- 1Department of Science and Mathematics Education, Umeå University, Umeå, Sweden
- 2Umeå Mathematics Education Research Centre (UMERC), Umeå University, Umeå, Sweden
- 3Department of Applied Educational Science, Umeå University, Umeå, Sweden
Research has shown that students’ learning gains in mathematics are greater when they work with problems rather than routine tasks. These learning gains from problem-solving activities may be enhanced by providing feedback that does not give away the solutions to the problems, but helps students construct their solution methods themselves and anchor their reasoning in intrinsic properties of the mathematical components involved in the reasoning. However, in order to use feedback, students would need to perceive it as useful, and not all students may find such feedback useful. In this study, we investigate how students’ ability and motivational beliefs affect how useful they perceive feedback aimed at supporting mathematical reasoning to be. In the study, students worked with mathematical problems and received metacognitive and heuristic feedback when they needed help. We used structural equation modeling (SEM) to analyze the effects. The results show that students’ mastery goals had a direct effect on the perceived usefulness of the feedback, but no such effects were found for students’ national test grades, self-efficacy beliefs, performance goals, or intrinsic or extrinsic forms of motivation. The proportion of successful use of feedback did not mediate the effects.
1 Introduction
Teaching that supports students’ learning may be accomplished in several ways. One suggested teaching design is underpinned by research claiming that to develop mathematical knowledge students need to struggle (in a positive sense), for example by engaging in problem-solving. Problems are then understood as tasks for which students do not have a known solution method available to them in advance. Instead, they need to construct (parts of) the solution methods through their own reasoning (Brousseau, 1997; Lithner, 2008, 2017).
Research has shown that students’ learning outcomes are greater when they work with problems compared to when working with tasks that can be solved by applying given algorithms (routine tasks) (e.g., Terwel et al., 2009; Kapur, 2014; Olsson and Granberg, 2019; Jonsson et al., 2020). Different explanations for this have been suggested. Kapur (2014) theorizes that problem-solving, compared to solving routine tasks, will lead students to recall and differentiate useful prior knowledge needed to explore the problem’s mathematical relationships to a greater extent. Furthermore, constructing methods is more likely to encourage exploration of the mathematics inherent in the task. For example, Norqvist et al. (2019) used eye-tracking to show that students who solved tasks by constructing the solution methods through their own reasoning focused on the task information (figures, etc.) needed to investigate the mathematics inherent in the task to a greater extent than students who solved tasks using a provided method (e.g., a formula) who merely looked at the method. Problem-solving is also more likely to result in efficient encoding and memory consolidation. Constructing new knowledge and creating new memories involves relating new information to prior knowledge (memories) that is retrieved during, for example, a learning activity. Studies using functional magnetic resonance imaging (fMRI) have shown that active learning, such as problem-solving, leads to higher levels of activity in brain network areas important for memory formation and retrieval of strong memories (Stillesjö et al., 2021).
However, solving problems is difficult for students (Lorenzo, 2005; Lester and Cai, 2016; Verschaffel et al., 2020), and when they work with mathematical problems, they encounter difficulties they cannot overcome on their own more often than when working with routine tasks (e.g., Olsson and Granberg, 2019; Jonsson et al., 2020). Feedback may provide support for students to overcome these difficulties, which may both enhance learning, and hinder some students from losing their motivation due to repeated failure to solve tasks. However, there is a risk that feedback may either reveal a substantial part of the solution or deprive students of their responsibility to solve the problems and the opportunity to learn from them (Brousseau, 1997), or be too vague and not help students to solve the problems. Feedback needs to help students to construct solution methods and anchor their reasoning in intrinsic properties of the mathematical components involved in the problems, rather than providing guidance that they can use to solve the problems without having to use reasoning based on conceptual understanding. However, research has shown that not all students want feedback that does not specify exactly what to do (Winstone et al., 2016; Sidenvall et al., 2022), so teachers needs to support students in becoming active rather than passive receivers of feedback (Webb and Jones, 2009; Winstone et al., 2017; Jonsson et al., 2020), which is an important part of being a self-regulated learner (Nicol and Macfarlane-Dick, 2006).
Feedback may be conceptualized as “information provided by an agent (e.g., teacher, peer, book, parent, self, experience) regarding aspects of one’s performance or understanding” (Hattie and Timperley, 2007, p. 81). It may target intended learning goals, students’ current progress toward these goals, and how to proceed to attain the goals (Hattie and Timperley, 2007). Metacognitive feedback may be particularly suitable for supporting students to become active receivers of feedback using it to construct (parts of) the solution methods through their own reasoning. Such feedback addresses “the way students monitor, direct, and regulate actions toward the learning goal” (Hattie and Timperley, 2007, 93). Another type of feedback that may be suitable is heuristic feedback, comprising general suggested strategies such as making a drawing. Indeed, research shows that metacognitive feedback is often most effective for enhancing student learning (Hattie and Timperley, 2007; Shute, 2008; Van der Kleij et al., 2015; Wisniewski et al., 2020). However, the number of studies on the effects on student achievement in the specific subject of mathematics is moderate, and the sizes of these effects vary substantially (Van der Kleij et al., 2015; Lee et al., 2020; Koenka et al., 2021). Van der Kleij et al. (2015) concluded that “it must be mentioned that the literature does not report any consistent positive effects of feedback in mathematics” and that “the positive results from studies conducted in the field of mathematics must be interpreted with caution” (p. 502). Thus, there is a need for further studies on when, how, and why feedback in mathematics is successful.
In general, several research reviews have concluded that the mechanisms by which feedback affects educational outcomes such as learning are poorly understood (e.g., Shute, 2008; Van der Kleij and Lipnevich, 2021). One reason for this may be that differences in the effects of feedback on student achievement may be due to a number of variables, such as the students themselves, the tasks, the teachers, the characteristics of the feedback, the context in which the feedback is given, and the interaction between these variables (Shute, 2008; Thurlings et al., 2013; Brooks et al., 2021). It has also been argued that one potential explanation for the lack of understanding about these mechanisms is a lack of systematic research and understanding in the research community about how students interpret and respond to feedback (e.g., Leighton, 2019). In particular, there is a lack of research on how students interpret and respond to feedback aimed at supporting their own mathematical reasoning, and the role of student characteristics (e.g., ability and motivational beliefs) for these responses. Students with certain characteristics may not be able to use this kind of feedback to solve their learning tasks, or may not be motivated to use the feedback for productive efforts. If so, the type of feedback that presumably would act as advantageous learning support for students with some characteristics may be detrimental for students with other characteristics.
Models of student responses to feedback commonly include initial states, which are factors and processes internal to the learner, such as students’ motivational beliefs and mathematical ability; internal responses to feedback that include interpretations and perceptions of the feedback, and decisions that are made about next steps; and observable external responses to feedback, which include students’ behavioral responses and performance (Lui and Andrade, 2022). Several models posit that students’ initial states have an impact on their internal responses to feedback. For example, students with low mathematical ability or low self-efficacy may not find feedback that primarily aims to support their mathematical reasoning, and does not give them a more direct solution method, very useful. The perception of feedback as useful is one of the fundamental aspects of feedback perception (e.g., Brett and Atwater, 2001), and is an internal student response to feedback that has been argued to influence external responses to feedback, such as students’ decisions to ask for and use feedback and their success in using it to solve tasks (Lui and Andrade, 2022). Hence, in order to take advantage of the significant learning opportunities inherent in problem-solving activities, it is important to investigate how students’ ability and motivational beliefs (e.g., self-efficacy beliefs, achievement goals, extrinsic and intrinsic motivation) affect how useful they perceive feedback aimed at supporting mathematical reasoning to be.
There is a range of research investigating influences of feedback on students’ motivational beliefs such as self-efficacy (e.g., Wang and Wu, 2008) and intrinsic motivation (e.g., Jurik et al., 2014). However, studies looking into the opposite relationship, that is how students’ perceptions of feedback depend on their individual characteristics, are more scarce. Van der Kleij and Lipnevich (2021) identified 27 studies on how perceived feedback might depend on students’ characteristics, but these studies concerned a variety of school subjects rather than mathematics specifically.
However, some student characteristics pertaining to the initial states may be hypothesized as affecting how useful students perceive feedback in mathematics to be, and there exists a few studies investigating such hypotheses. Students’ mathematical ability is one characteristic that might affect the perceived usefulness of feedback. Students with low ability in relation to the tasks they are set to solve may be less able than students with a higher mathematical ability to use feedback that requires them to think deeply about how to use it to solve the task. This, in turn, may be due to both a lower ability to tackle difficult tasks and greater difficulties in processing feedback information. Consequently, these students may find this feedback less useful. Another student characteristic pertaining to their initial state is their self-efficacy beliefs. Self-efficacy expectation is “the conviction that one can successfully execute the behavior required to produce the outcomes” (Bandura, 1977, p. 141). Most commonly, students with higher self-efficacy expectations will expend more effort on an activity, will persevere when confronting obstacles, and will be resilient in the face of adverse situations (Schunk and Pajares, 2009). Thus, students with low self-efficacy expectations may not believe they will be able to use feedback successfully if it requires them to engage in a productive struggle, and will therefore not find it useful. However, in the study by Rakoczy et al. (2019), students’ perception of the usefulness of written process-oriented feedback in mathematics—which included what they had done well, the areas in which they could improve, and content-specific strategies on how they could improve—was not related to their self-efficacy.
Students’ achievement goals may also moderate how they respond to feedback. Students who have performance goals are driven by a desire to demonstrate competence and have a normative standard for evaluating competence, while students with mastery goals are driven by the goal of developing competence evaluated against either task-based or intra-personal standards. Although both performance goals and mastery goals are considered to have an approach aspect and an avoidance aspect, a trichotomous model subdividing only performance goals in relation to this aspect is frequently used in educational research (Murayama and Elliot, 2009). Since performance goals have been shown to be less adaptive than mastery goals when solving challenging tasks (Linnenbrink-Garcia et al., 2008), students with performance goals may perceive feedback that requires a productive struggle and new thinking to be less useful. Studies investigating this hypothesis are hard to find, but the students in a study by Rakoczy et al. (2013) perceived process-oriented feedback to be more useful than social-comparative feedback, and mastery goal orientation moderated the effect of feedback on perceived usefulness.
Finally, other types of motivation may affect students’ perceptions of the usefulness of feedback. While achievement goal theory distinguishes between goals of learning and goals that focus on being better than peers, self-determination theory (SDT) (Ryan and Deci, 2020) distinguishes between the extent to which students have internalized goals and pursue them of their own volition. SDT distinguishes between two major categories of motivation: intrinsic and extrinsic motivation. Intrinsically motivated students engage in an activity because they find the activity in itself inherently interesting or enjoyable, while extrinsically motivated students engage in an activity because it may lead to a separable outcome. Extrinsic motivation differs in terms of the extent to which the reasons for students’ actions are self-determined or autonomous. Externally motivated students engage in a task because of external rewards or to avoid discomfort or punishment, and this is the least autonomous form of extrinsic motivation. Students may also engage in an activity to avoid feeling guilt, or to attain ego enhancement or pride. In such introjected regulation, the students’ reasons for engaging in an activity are a little more autonomous, but they still experience these reasons as being imposed on them. Students may also have more autonomous forms of extrinsic motivation, engaging in an activity because they personally find it valuable and have identified its regulation as their own (identified and integrated extrinsic motivation). Students with less autonomous forms of extrinsic motivation (external and introjected motivation) may find feedback requiring a productive struggle to be less useful than students with intrinsic motivation or identified or integrated extrinsic motivation, because this requires substantial effort and they have not identified engagement in task-solving as being personally valuable or enjoyable. However, we have not found any studies investigating this hypothesis. Hence, in summary, there is a need for further research examining how students’ characteristics affect the perceived usefulness of feedback in mathematics.
2 Research questions
In the present study, upper-secondary school students were administered mathematical problems, presented to them on their laptops. If they needed help, they could click and receive metacognitive and heuristic feedback. We asked the following research questions:
1. To what extent do the students perceive the feedback as useful?
2. To what extent are the students successful in solving the tasks for which they receive feedback?
3. Do students’ mathematical ability, self-efficacy, achievement goals, and type of motivation have a direct effect on their perceived usefulness of the feedback?
4. Do the students’ mathematical ability, self-efficacy, achievement goals, and type of motivation have an indirect effect on their perceived usefulness of the feedback via their success in solving the tasks for which they receive feedback?
3 Methods
Students were invited to solve mathematical problems, supported by metacognitive and heuristic feedback when they needed it. Data consisted of students’ answers to the problems, and their responses to questionnaire items about their national test grades, self-efficacy beliefs, achievements goals, intrinsic and extrinsic forms of motivation, and their perceptions of the usefulness of the feedback they received. To answer Research questions 1 and 2, mean values were calculated, and to answer Research question 3 structural equation modeling (SEM) was conducted to assess the relationships between variables.
3.1 Participants
The participants (N = 134, 82 females, 52 males), were upper-secondary school students enrolled in the business program at a public high school in a mid-sized city in Sweden. The average age of the participants was 17.3 (SD = 0.76). Of the participants, 128 were of Swedish origin and six were of foreign origin. At the time of the study, students enrolled in the business program at this upper-secondary school had a higher proportion of university educated parents compared to the national average. Two data sets were excluded due to missing data from the task-solving session. As a result, 132 data sets have been used in this study. Participation was voluntary and the students had given their informed consent to participate. All participants received a gift card (40 euros) as an incentive to participate.
3.2 Study procedure
Data were collected during May and June 2022. Before that, a pilot study was conducted with 23 participants in February 2022. The results from the pilot study were used to ensure an appropriate level of difficulty for the tasks, that feedback and questionnaires were formulated in an understandable way, and that the web application had good functionality. The study was conducted outside ordinary school hours, and the participants used their personal laptops to answer the questionnaires and solve the tasks. Calculators, pens, and paper were allowed when solving the tasks. After an introduction, the students logged in to a web survey to answer Questionnaire 1. After completing the questionnaire, the students were automatically transferred to the web application. The instructions were shown on the screen, and the students could choose when to start the task-solving session (details are provided in Section 3.3.2). The students had a maximum of 10 min to solve each of the six tasks. After completing the six tasks (see Appendix A), the students were automatically transferred to the web survey to answer Questionnaire 2 (see Table 1 for an overview and Section 3.3 for more details). After completing Questionnaire 2, they received compensation for their participation.
3.3 Materials
Problems, feedback, and all questionnaires were administrated to the students digitally.
3.3.1 Questionnaire 1
Questionnaire 1 included items about students’ national test grades in mathematics, self-efficacy, achievement goals, and intrinsic and extrinsic forms of motivation. With regard to self-efficacy, goals and type of motivation, all items were statements that the students were asked to rate the extent of their agreement with, on a five-point scale ranging from 1 (completely disagree) to 5 (completely agree). The items used in the questionnaire (see Appendix B) are adapted from a study by Hofverberg et al. (2022).
3.3.1.1 National test grade
The students were asked to provide their national test grade in mathematics from school year 9 (their most recent national test performance, 2 years before the study). The national test grades range from A to F, with A being the highest grade and F being a failing grade.
3.3.1.2 Self-efficacy
Four items were used to measure the students’ self-efficacy. The key item was “I feel that I can do well in mathematics.” Internal consistency reliability of the scale was measured using Cronbach’s alpha coefficient. Alpha was calculated at 0.86, which suggests good internal consistency.
3.3.1.3 Achievement goals
Twelve items were used to measure the students’ achievement goals. Four items concerned performance approach goals (Cronbach’s alpha was 0.85) with the key item “In math, it is important for me to perform better on tests than the other students,” four items concerned performance avoidance goals (alpha was 0.88) with the key item “In math, it is important for me to not perform worse than other students on tests,” and four items concerned mastery goals (alpha was 0.79) with the key item “In math, I want to learn things, even if they are not assessed on tests or affect my grades.” All alpha values suggest good internal consistency of the scales.
3.3.1.4 Intrinsic and extrinsic forms of motivation
Eight items were used to measure the students’ type of motivation. Each of the variables external, introjected, identified, and intrinsic motivation was measured by two items. Cronbach’s alpha was 0.77, 0.72, 0.67, and 0.93, respectively. The alpha values suggest sufficient to good consistency of the scales.
3.3.2 Web application including tasks, diagnosis, and feedback
3.3.2.1 Web application
A website was constructed for the study. After logging in and reading the instructions—which described how to choose and receive feedback, along with encouragement to use the feedback—the students clicked “Start” to initiate the task-solving session. The web application presented the first task together with a box to submit the answer, a timer counting down from 10 min, and four diagnosis statements (i.e., descriptions of difficulties a student might have) (Figure 1). If students encountered a difficulty, they could click on the description that best corresponded to their difficulty and metacognitive feedback was shown (Figure 2). If the metacognitive feedback did not help, students could choose “More help” and heuristic feedback was shown. The students had 10 min per task. If the answer was correct, a new task was presented. If the answer was incorrect, they could try again as many times as they needed, within a timeframe of 10 min. The diagnoses and feedback were the same for all tasks (Appendix C).
3.3.2.2 Tasks
Ten tasks (see Appendix A) were selected from a set of 24 tasks used in an earlier study (Jonsson et al., 2020). The tasks were designed as problems, i.e., as tasks for which the students are unlikely to have a known solution method available and therefore must create a method by themselves. The tasks were selected in such a way that no mathematical content knowledge beyond the four basic arithmetic operational calculation methods would be required. Since the study aimed to investigate the effects of student characteristics on their perceptions of feedback usefulness, tasks were furthermore chosen to range from easy to difficult, making it likely that students to at least some of the problems would need feedback to be able to solve them. Two pilot studies were conducted to ensure that the difficulty level of the selected tasks was suitable for the purpose of the study, and thereafter six of the 10 tasks were selected to be included in the study.
3.3.2.3 Diagnosis and feedback
To support students when they got stuck solving any of the six tasks, each task was accompanied by four diagnoses statements, i.e., descriptions of different kinds of difficulties the students might have. These diagnosis statements were developed and formulated to correspond to the four activities that students are expected to engage in during a problem-solving process (see Figure 1 and Appendix C). Depending on which diagnosis statement the students chose, they were provided with suitable feedback. Based on the chosen diagnosis, students first received metacognitive feedback formulated as general (not task-specific) questions or suggestions that aimed to encourage them to check for mistakes, or to explain what the task was asking for (see Figure 2). In other words, the aim was to initiate monitoring and control of the task-solving process. If metacognitive feedback was not sufficient, the student could choose “I need more help” to receive heuristic feedback, formulated in terms of general suggestions for strategies, such as making a drawing, solve a simpler example, etc. (see feedback details in Appendix C).
3.3.3 Questionnaire 2
To measure students’ overall perceived usefulness of feedback, the students were asked after the task-solving session to rate three items on a five-point scale ranging from 1 (completely disagree) to 5 (completely agree). The key item was “I would consider this feedback useful.” Cronbach’s alpha was calculated at 0.94, which suggests good internal consistency of the scale. These items (see Appendix B) are adapted from the study by Strijbos et al. (2021).
3.4 Analysis method
To answer the research questions, the analysis proceeded in three stages. Firstly, computer-logged data from each student’s task-solving activities were analyzed to determine (a) whether students received feedback, and (b) whether students managed to solve the tasks for which they received feedback. After that, the proportion of successfully solved tasks for which feedback was received (PSTF) was computed for each student. Students receiving feedback is in this study conceptualized as it is reasonable to assume that they have actually read the feedback and possibly used it in their task-solving endeavors. To determine whether students had received feedback in this sense, two conditions needed to be fulfilled: (i) At least 60 s had to have passed between the time of clicking on the request for feedback and the time of submitting an answer. We noticed from the pilot study that students needed at least 1 min to read and try to utilize the feedback, (ii) After clicking on the request for feedback, the students did not submit unrealistic answers or a sequence of random numbers. The log file shows the answers that were entered. If a student tried to submit a random number as the answer more than five times, then this was counted as not receiving feedback. It was noted that those students who gave a random number as an answer usually did so within 30 s of clicking on the request for feedback.
Secondly, the descriptive statistics (e.g., mean, standard deviation, and Pearson correlation) were computed for all variables (Table 2). Before computing the descriptive analysis, 12 students’ data sets were removed from the data, since these 12 students did not use any feedback.
Thirdly, we used structural equation modeling (SEM) to assess the relationships between independent variables (i.e., grade, self-efficacy beliefs, extrinsic and intrinsic forms of motivation, and achievement goals) and dependent variables (i.e., perception of usefulness of feedback and proportion of successfully solved tasks when receiving feedback). Three different models were specified (see Figures 3 –5). The dependent variables were the same in all models, but the independent variables varied. For all models, the independent variables were correlated and specified as having direct effects on both dependent variables, and the proportion of successfully solved tasks when receiving feedback was specified as having a direct effect on the perceived usefulness of feedback.
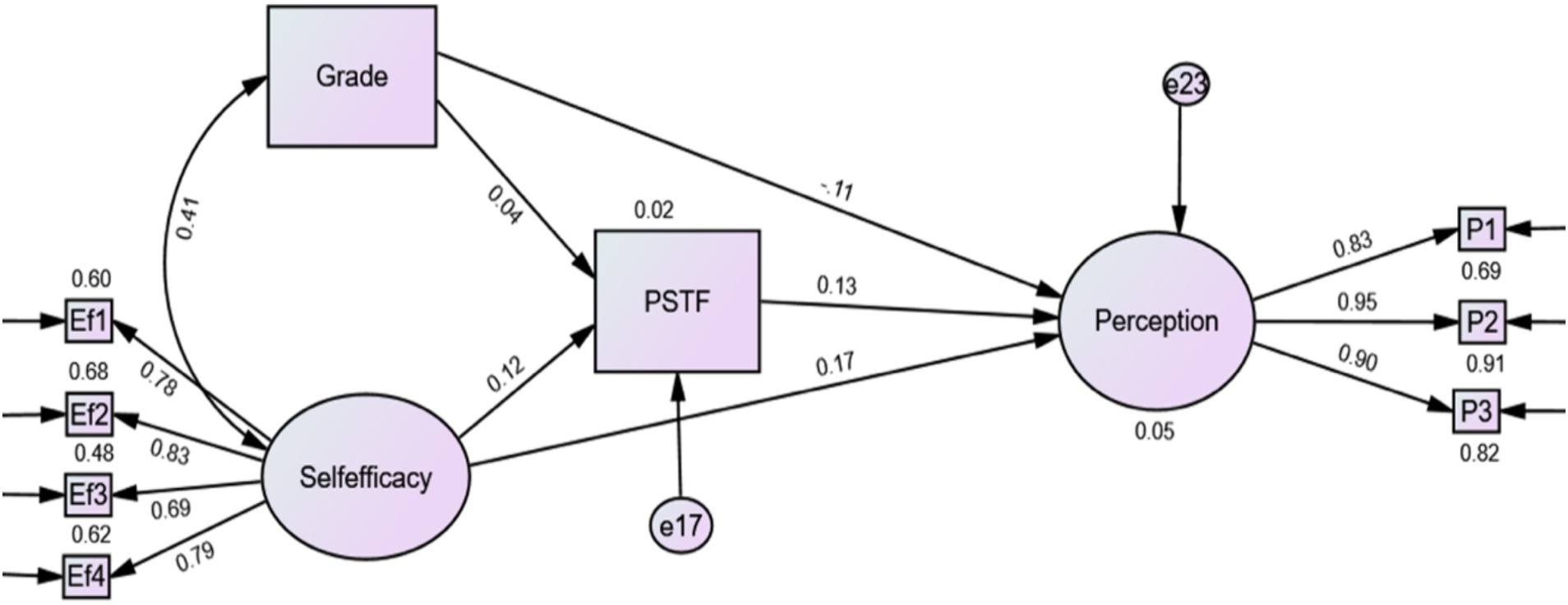
Figure 3. Path diagram describing structural relationships between the variables of Model 1. Standardized coefficients. PSTF, Proportion of solved tasks when receiving feedback.
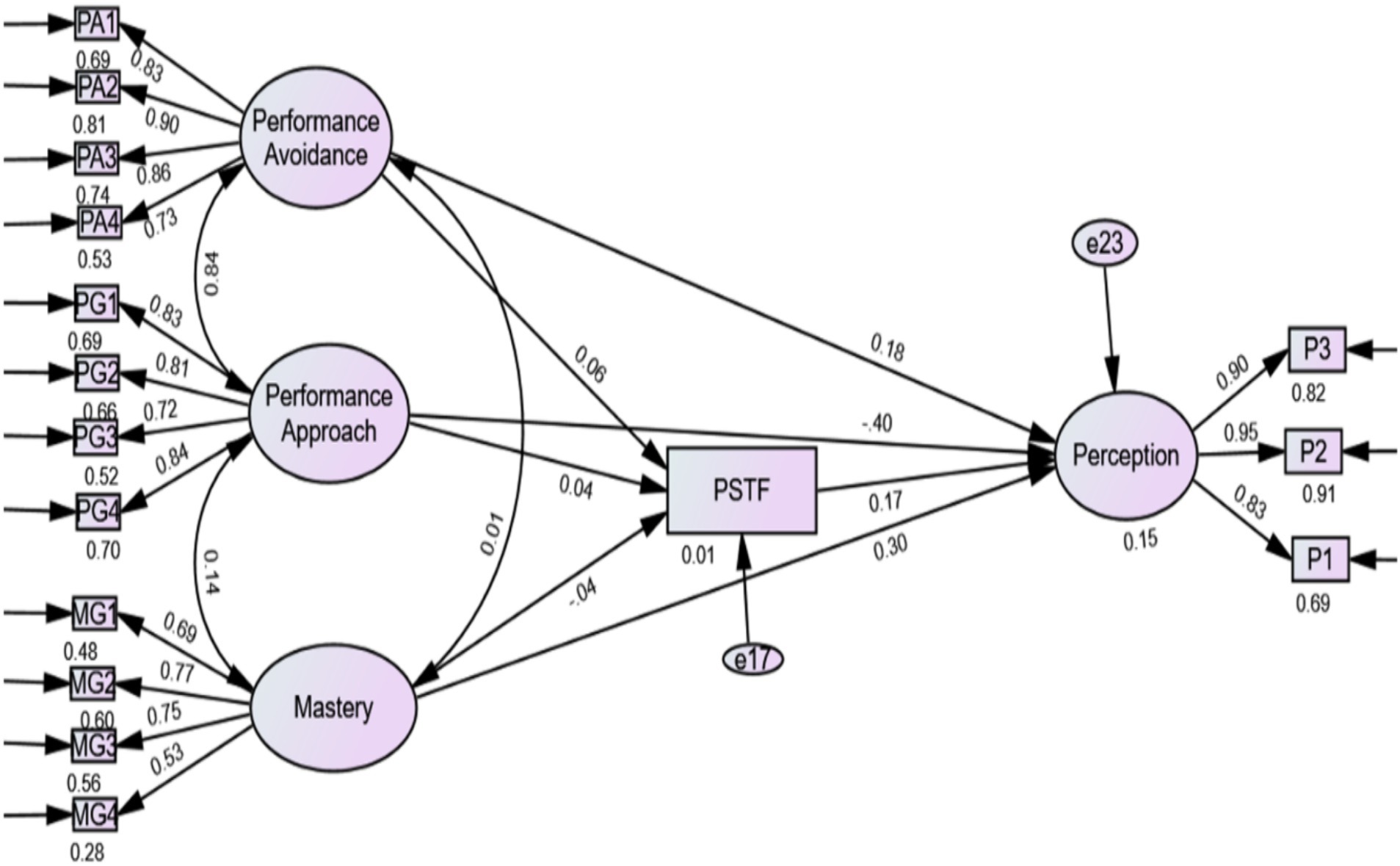
Figure 4. Path diagram describing structural relationships between the variables of Model 2. Standardized coefficients. PSTF, Proportion of solved tasks when receiving feedback.
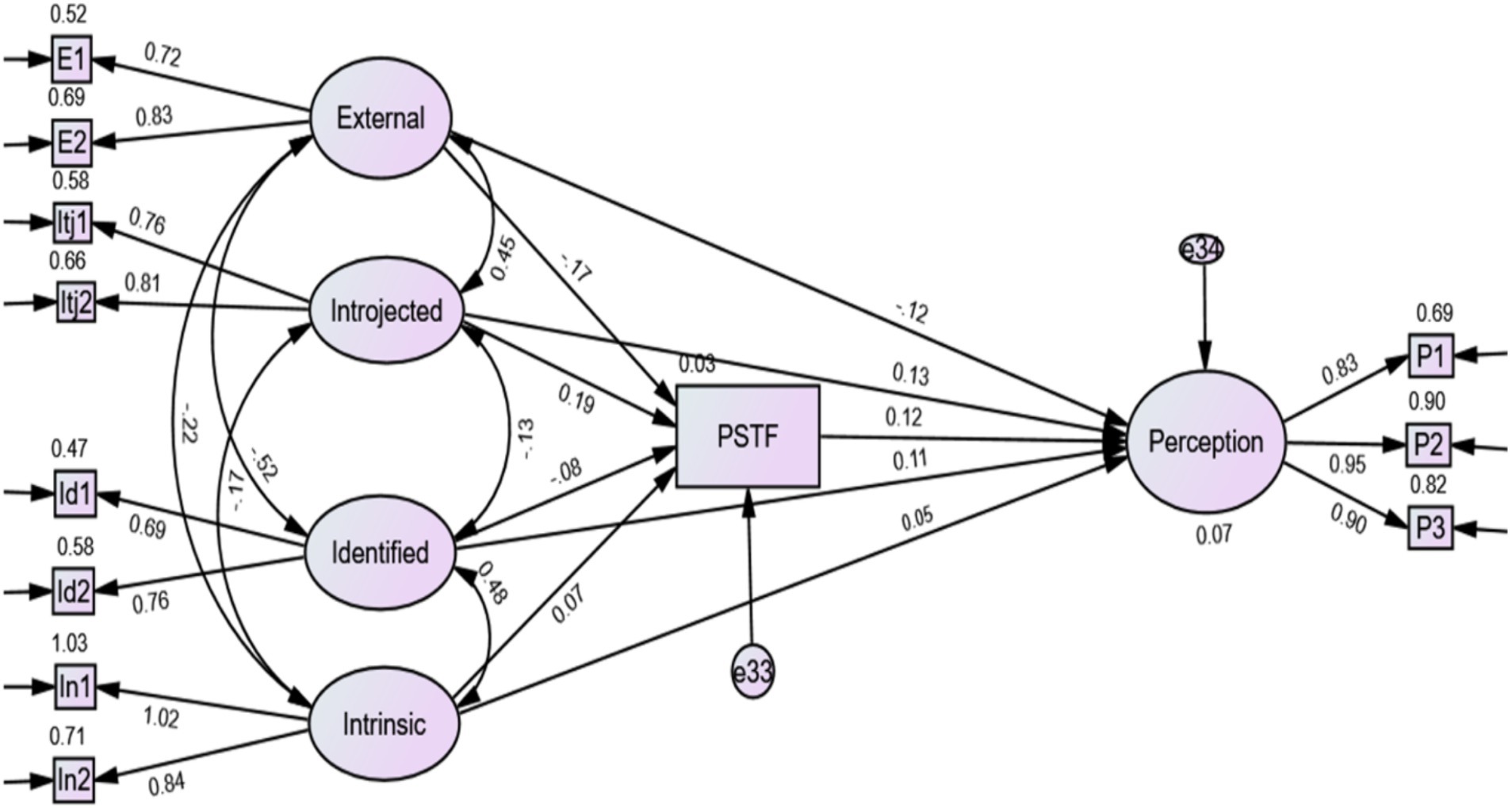
Figure 5. Path diagram describing structural relationships between the variables of Model 3. Standardized coefficients. PSTF, Proportion of solved tasks when receiving feedback.
Based on the specification of the models described above, the SEM analysis was carried out using Amos 28.0 software. The SEM analysis proceeded using a two-step model (e.g., Anderson and Gerbing, 1988). To do so, we first specified a full measurement model for all the latent variables in each of the three models (i.e., how the observed variables relate to the latent variables). Confirmatory factor analysis (CFA) was conducted to test the suitability of the model. Secondly, the structural model was specified (i.e., how the constructs are related to one another) to test the hypothesized model. The CFA measurement and structural models are provided in Table 3. We used the maximum likelihood estimator because of its unbiased, consistent, and efficient nature (e.g., Bollen, 1989). The chi-square (χ2) test, the comparative fit index (CFI), the Tucker–Lewis’s index (TLI), the incremental fit index (IFI), the normed fit index (NFI), and the root mean square error of approximation (RMSEA) were used to evaluate the level of fit for structural models. The CFI, IFI, NFI, and TLI values greater than 0.90 and 0.95 and the RMSEA value lower than 0.08 and 0.06 indicate an adequate or good model fit, respectively (Hu and Bentler, 1999). To test the significance of indirect effects, we used 1,000 bootstrap samples with Monte Carlo simulation and estimated 95% confidence intervals (CIs) for the effects. Additionally, to determine the achieved power of each model we used the R-package semPower (Moshagen and Erdfelder, 2016).
4 Results
No data were missing from the analysis. Three different models were computed where Model 1 comprises the effects of national test grade and self-efficacy on perceived usefulness, Model 2 comprises the effects of achievement goals on perceived usefulness, and Model 3 comprises the effects of intrinsic and extrinsic forms of motivation on perceived usefulness. Table 3 shows fit indices for the measurement models of the CFA, and structural models. The standardized factor loadings of the items ranged from 0.6 to 0.9 for each model.
4.1 Task solution success when receiving feedback and perception of feedback usefulness
Table 2 comprises descriptive statistics for the variables used in the analysis. The mean and the standard deviation of the students’ responses to individual items for each latent variable is used in the correlation analysis. The table shows that the mean value of the students’ perceptions of the usefulness of the feedback was 1.9, and the students succeeded in solving approximately half of the tasks for which they received feedback. Table 2 also displays the Pearson product–moment correlation coefficient for the relationship between variables. From the table, it can be seen that there are several strong positive relationships between the variables in the study. For example, there is a very strong correlation between performance avoidance goals and performance approach goals (r = 0.74), and self-efficacy are significantly and positively correlated with mastery goals, identified and intrinsic motivation, grade, and score. However, the students’ perception of the usefulness of feedback is only significantly correlated with having mastery goals.
4.2 Effects of national test grade and self-efficacy on perceived usefulness
Model 1 (Figure 3) showed a good fit to the data (Chi-square/df = 1.30, p = 0.15, CFI = 0.99, TLI = 0.98, NFI = 0.95, IFI = 0.99, RMSEA = 0.05). National test grade and self-efficacy show no statistically significant direct effects on proportion of solved tasks when receiving feedback (PSTF) and the standardized coefficients are small (see Figure 3). PSTF has no significant effect on student perceived usefulness of the feedback (b = 0.13, p = 0.16). Looking at indirect and direct effects on perceived usefulness, we find no statistically significant effects from national test grade and self-efficacy. National test grade has a combined standardized path coefficient of −0.11 (direct effect: b = −0.11, p = 0.29; indirect effect: b = 0.005, p = 0.53) and self-efficacy has a combined standardized path coefficient of 0.19 (direct effect: b = 0.17, p = 0.13; indirect effect: b = 0.02 p = 0.24). We found a positive correlation (b = 0.41) between self-efficacy and grade. A post-hoc power analysis, conducted with semPower (Moshagen and Erdfelder, 2016), indicated that Model 1, with an alpha of 0.05, an RMSEA of 0.08, and the current sample size, achieved a power of 0.75.
4.3 Effects of achievement goals on perceived usefulness
Model 2 (Figure 4) showed an adequate fit to the data (Chi-square/df = 1.14, p = 0.17, CFI = 0.99, TLI = 0.99, NFI = 0.91, IFI = 0.99, RMSEA = 0.03). Mastery goals, performance approach goals, and performance avoidance goals show no statistically significant effects on PSTF and the standardized coefficients are small (see Figure 4). PSTF does not have a statistically significant direct positive effect on students’ perceived usefulness of the feedback (b = 0.17, p = 0.06). Looking at indirect and direct effects on perceived usefulness, there is a statistically significant positive direct effect from mastery goals, and a nearly significant negative direct effect from performance approach goals, on perceived usefulness of the feedback. Mastery goals has a combined standardized path coefficient of 0.29 (direct effect: b = 0.30, p = 0.01; indirect effect: b = −0.008, p = 0.54), performance approach goals has a combined standardized path coefficient of −0.39 (direct effect: b = −0.40, p = 0.08; indirect effect: b = 0.007, p = 0.73), and performance avoidance goals has a combined standardized path coefficient of 0.19 (direct effect: b = 0.18, p = 0.42; indirect effect: b = 0.01, p = 0.61). We found a strong correlation (b = 0.84) between performance approach goals and performance avoidance goals. The post-hoc power analysis, conducted with semPower, indicated that Model 2, with an alpha of 0.05, an RMSEA of 0.08, and the current sample size, achieved a power of 0.99.
4.4 Effects of intrinsic and extrinsic forms of motivation on perceived usefulness
Model 3 (Figure 5) showed a good fit to the data (Chi-square/df = 0.83, p = 0.77, CFI = 1.00, TLI = 1.00, NFI = 0.95, IFI = 1.0, RMSEA = 0.00). Intrinsic, identified, introjected, and external motivation show no statistically significant effects on PSTF and the standardized coefficients are small (see Figure 5). PSTF has no significant effect on students’ perceived usefulness of the feedback (b = 0.12, p = 0.2). Looking at indirect and direct effects on perceived usefulness, we find no statistically significant effects from any type of motivation. We see that intrinsic motivation has a combined standardized path coefficient of 0.06 (direct effect: b = 0.05, p = 0.68; indirect effect: b = 0.008, p = 0.47), identified motivation has a combined standardized path coefficient of 0.096 (direct effect: b = 0.11, p = 0.54; indirect effect: b = −0.01, p = 0.39), introjected motivation has a combined standardized path coefficient of 0.16 (direct effect: b = 0.13, p = 0.32; indirect effect: b = 0.02, p = 0.16), and external motivation has a combined standardized path coefficient of −0.14 (direct effect: b = −0.12, p = 0.48; indirect effect: b = −0.02, p = 0.22). We found a positive correlation (b = 0.48) between identified and intrinsic motivation, a negative correlation (b = −0.52) between external and identified motivation, and a positive correlation (b = 0.45) between external and introjected motivation. The post-hoc power analysis, conducted with semPower (Moshagen and Erdfelder, 2016), indicated that Model 3, with an alpha of 0.05, an RMSEA of 0.08, and the current sample size, achieved a power of 0.85.
5 Discussion
To use feedback, students need to perceive it as useful (Lui and Andrade, 2022), and not all students will find feedback that requires them to think deeply and engage in a sustained productive struggle to be useful. The present study is, to our knowledge, the first to investigate how the ability and motivational beliefs of students affect their perceived usefulness of feedback that aims to support them in constructing their solution methods themselves and help them anchor their reasoning in intrinsic properties of the mathematical components involved in the reasoning. The results of the study extend the current research base by providing evidence that students may not perceive this type of feedback to be useful, and are not very successful at using it. Foremost, however, the study contributes by providing evidence on the role of students’ mathematical ability and motivational beliefs for their perception of the usefulness of this kind of feedback. The results showed that students having mastery goals was the only variable that affected their perceived usefulness of the feedback.
5.1 Perception of feedback usefulness
The results of the study show that the students did not perceive the provided feedback to be very useful. The mean of the students’ answers was only 1.9. One reason for this may be that the students are used to receiving feedback that tells them what to do to solve the tasks they are working with, and they have therefore formed the belief that the primary purpose of working with tasks is to solve them, not to learn from working with them. From this belief, they may have formed the perception that useful feedback is feedback that helps them solve tasks without much thinking or struggling. Indeed, students have been shown to typically want feedback that specifies exactly what they should do (Winstone et al., 2016) rather than asking them to narrate their own thoughts. The latter might even bring students to demand the teacher to provide the method (Sidenvall et al., 2022). If this is so, teachers need to challenge their students’ beliefs about the purpose of task-solving, and their beliefs about what constitutes useful feedback. Teachers need to support students’ in taking the role of an active, rather than a passive, receiver of feedback who is consciously in the pursuit of learning (Winstone et al., 2017), which is an important part of being a self-regulated learner (Nicol and Macfarlane-Dick, 2006). This would require teachers themselves to act in accordance with the belief that the main purpose of working with tasks is learning, and that the purpose of feedback is to support students’ thinking because this will enhance learning the most. This would mean consistently providing feedback that both requires and supports students to engage in reasoning based on conceptual mathematical understanding.
5.2 Task solution success when receiving feedback
The students only succeeded in solving half of the tasks for which they received feedback. These results are in line with conclusions that solving problem is challenging for students (Verschaffel et al., 2020) and more difficult than solving routine tasks (e.g., Olsson and Granberg, 2019; Jonsson et al., 2020). and that when students ask for help, they expect the teacher to tell them how to do (Winstone et al., 2016) This might have been another reason for students not perceiving the provided feedback as useful. However, this reason is not supported by data. The correlation between the proportion of tasks they solved when needing feedback and the extent to which they found the feedback useful did not reach statistical significance. But, on average, the students only clicked for feedback on two tasks, which brings significant uncertainty to the measure of this correlation.
Nevertheless, if students are to use feedback, they would both need to want, and be able, to use the feedback successfully (Jonsson, 2013; Winstone et al., 2017). Thus, the study’s finding that the students neither found the feedback useful nor were able to use it successfully to a large extent is important. The former was discussed above, and the latter means that the students were often not able to use metacognitive and heuristic hints to construct mathematical reasoning based on conceptual understanding to solve the tasks, although they should possess the mathematical content knowledge required for this reasoning. These findings have some important implications for teaching and providing feedback. Many teachers are aware that feedback that supports students’ thinking rather than describing how to solve the tasks would provide better learning opportunities. However, the results of this study imply that teachers cannot assume that students are able to use this type of feedback without practice, and many students may not have very much experience of receiving and processing this kind of feedback. These results are in line with a study by Winstone et al. (2016) who argue that students are more used to feedback telling them how to do, and the study by Webb and Jones (2009) who points out the challenge students experience when teachers, instead of giving feedback that tells the students how to do, require the students to explain their ideas and taking an active part in a dialogue about how to proceed in their task solving.
In order to take advantage of the significant learning opportunities that are presumably inherent in teacher feedback during problem-solving activities, it would be important for teachers to teach, and let students practice, how to use metacognitive and heuristic feedback to construct mathematical reasoning based on conceptual understanding. Constructing such reasoning is not trivial; it is a rather advanced skill that needs to be practiced in order to be mastered. Although intervention programs focusing on training students to use metacognitive self-directed questions and heuristic problem-solving strategies have shown to be able to accomplish positive effects on students’ mathematical problem-solving performance, these effects have typically been moderate (Verschaffel et al., 2020). In accordance with such results, Lester and Cai (2016) conclude that, as valuable as problem solving is for learning, it takes a long time to master.
5.3 Effects of student characteristics on the perception of the feedback’s usefulness
The only student characteristic that had a statistically significant effect on perceived usefulness of the feedback was students’ mastery goals. However, while mastery goals had a positive direct effect on perceived usefulness, performance approach goals had a nearly significant but negative effect. These results may be understood through the properties of these goals. Students with mastery goals are driven by the goal of learning, and evaluate goal attainment in relation to task-based or intra-personal standards. Thus, feedback that focuses on conceptual issues and requires students to think instead of providing solution methods may be consistent with mastery goal students’ ideas of feedback usefulness. By contrast, students with performance goals who are driven by demonstrating competence in relation to others may not perceive feedback that makes it difficult for them to continuously demonstrate competence as useful. The results are also consistent with studies that have found performance goals to be less adaptive than mastery goals when solving challenging tasks (Linnenbrink-Garcia et al., 2008). This means that to support students’ perception that the metacognitive and heuristic feedback is useful, it may be productive for teachers to support the students’ mastery goals and not their performance approach goals. This would include a teaching behavior focusing on each student’s learning in relation to themselves and specific standards, and not focusing on competition and students’ learning in relation to each other. Such a focus may also be particularly useful for students who use passive rote learning approaches, which is an approach that have been argued to be encouraged by examination-based assessment systems and to be negatively associated with student achievement (Saha et al., 2024). Such a passive learning approach is not likely to be consistent with students perceiving feedback that requires active thinking and constructing solution methods themselves as useful.
Studies on the effects of students’ characteristics in mathematics on their perceptions of the usefulness of different kinds of feedback are scarce, so there are few studies with which to compare our results. Thus, there is a significant need for more studies investigating this topic. We have not found any studies focusing on the effects of intrinsic or extrinsic forms of motivation on students’ perceptions of the usefulness of feedback. However, students in the study by Rakoczy et al. (2013) perceived process-oriented feedback to be more useful than social-comparative feedback, and mastery goal orientation moderated the effect of feedback on perceived usefulness (Rakoczy et al., 2013). As in the present study, students’ self-efficacy did not affect their perception of the usefulness of the process-oriented feedback investigated in the study of Rakoczy et al., 2019. Since students with higher self-efficacy beliefs commonly expend more effort on an activity and persevere when they confront obstacles to a higher degree (Schunk and Pajares, 2009), we had expected self-efficacy to be positively associated with both the students’ success in using the feedback and the feedback’s perceived usefulness. However, self-efficacy is domain-specific so a possible reason for this lack of effects may be that their self-efficacy expectations of doing well in mathematics did not extend to this new situation, which included types of tasks and feedback they were not used to.
5.4 Limitations of the study
The metacognitive and heuristic feedback used in the present study aimed to provide sufficient support for students to construct their own solutions based on reasoning anchored in the intrinsic properties of the mathematical components involved in the reasoning, and not to give away the solution so the students could solve the tasks without mathematical reasoning based on conceptual understanding. However, there is a fine line between not revealing a substantial part of the solution and providing feedback that is too vague and does not help the students to come closer to solving the tasks. In this study, the average number of tasks for which the students used the feedback was only two, and the students only succeeded in solving half of the tasks for which they received feedback. The use of tasks rendering such small numbers of successful feedback use will restrict the possible variation in this variable. This is a limitation of the study because it increases the risk of not detecting a potential mediating role of successful feedback use in effects of students’ characteristics on their perception of the feedback’s usefulness. It may also be worth noting that the students in this study participated in their spare time and received financial compensation. These circumstances might have had both a positive and a negative influence on their motivation to engage in the problem-solving activity, and hence on the results of the study. The students might have acted differently in an ordinary classroom situation. The financial compensation may, for example, have increased their effort when using the feedback, while solving the tasks in their spare time without having their teacher present may have decreased their motivation to struggle and persist with using the feedback. Effort and persistence may influence their success in using the feedback and their perception of its usefulness.
5.5 Suggestions for future research
Future studies may try to develop tasks and feedback with the same aim as in the present study, but with properties that encourage students to use the feedback in a larger number of tasks and succeed in using it more often even though they have to construct the solutions using their own reasoning. Such studies would provide a wider variation in the proportion of tasks the students manage to solve with the help of feedback. Such a wider variation may diminish the risk present in this study that a low variation in the proportion of successfully solved tasks for which feedback was received is a reason why this proportion did not significantly mediate the effects of the students’ characteristics on their perception of the feedback’s usefulness. Future studies could also benefit from being conducted during ordinary teaching of mathematics to better resemble the students’ normal learning situation. Furthermore, studies may also be conducted over a longer time period (weeks or months instead of an hour), since more encounters with this kind of feedback may influence both students’ success in using the feedback and their perception of its usefulness.
Data availability statement
The raw data supporting the conclusions of this article will be made available by the authors, without undue reservation.
Ethics statement
The study was conducted in accordance with local legislation and institutional requirements. All participants in the study were older than 15 years. In Sweden, for students of that age legal guardians informed consent is not necessary, it suffices with the written consent of the students. Such consent was obtained. This kind of project with this kind of Swedish data does not require approval from the Swedish Ethics review board.
Author contributions
SS: Conceptualization, Data curation, Formal analysis, Investigation, Methodology, Resources, Software, Supervision, Validation, Visualization, Writing – original draft, Writing – review & editing. TP: Conceptualization, Data curation, Supervision, Writing – original draft, Writing – review & editing. CG: Conceptualization, Data curation, Supervision, Writing – original draft, Writing – review & editing.
Funding
The author(s) declare that no financial support was received for the research, authorship, and/or publication of this article.
Conflict of interest
The authors declare that the research was conducted in the absence of any commercial or financial relationships that could be construed as a potential conflict of interest.
Publisher’s note
All claims expressed in this article are solely those of the authors and do not necessarily represent those of their affiliated organizations, or those of the publisher, the editors and the reviewers. Any product that may be evaluated in this article, or claim that may be made by its manufacturer, is not guaranteed or endorsed by the publisher.
Supplementary material
The Supplementary material for this article can be found online at: https://www.frontiersin.org/articles/10.3389/feduc.2024.1374664/full#supplementary-material
References
Anderson, J. C., and Gerbing, D. W. (1988). Structural equation modeling in practice: a review and recommended two-step approach. Psychol. Bull. 103, 411–423. doi: 10.1037/0033-2909.103.3.411
Bandura, A. (1977). Self-efficacy: toward a unifying theory of behavioral change. Psychol. Rev. 84, 191–215. doi: 10.1037/0033-295X.84.2.191
Brett, J. F., and Atwater, L. E. (2001). 360° feedback: accuracy, reactions, and perceptions of usefulness. J. Appl. Psychol. 86, 930–942. doi: 10.1037//0021-9010.86.5.930
Brooks, C., Burton, R., van der Kleij, F., Carroll, A., Olave, K., and Hattie, J. (2021). From fixing the work to improving the learner: an initial evaluation of a professional learning intervention using a new student-centred feedback model. Stud. Educ. Eval. 68:100943. doi: 10.1016/j.stueduc.2020.100943
Brousseau, G. (1997). Theory of Didactical Situations in Mathematics: Didactique des Mathématiques, 1970–1990. Netherlands: Springer.
Hattie, J., and Timperley, H. (2007). The power of feedback. Rev. Educ. Res. 77, 81–112. doi: 10.3102/003465430298487
Hofverberg, A., Winberg, M., Palmberg, B., Andersson, C., and Palm, T. (2022). Relationships between basic psychological need satisfaction, regulations, and behavioral engagement in mathematics. Front. Psychol. 13:829958. doi: 10.3389/fpsyg.2022.829958
Hu, L., and Bentler, P. M. (1999). Cutoff criteria for fit indexes in covariance structure analysis: conventional criteria versus new alternatives. Struct. Equ. Model. Multidiscip. J. 6, 1–55. doi: 10.1080/10705519909540118
Jonsson, A. (2013). Facilitating productive use of feedback in higher education. Act. Learn. High. Educ. 14, 63–76. doi: 10.1177/1469787412467125
Jonsson, B., Granberg, C., and Lithner, J. (2020). Gaining mathematical understanding: the effects of creative mathematical reasoning and cognitive proficiency. Front. Psychol. 11:574366. doi: 10.3389/fpsyg.2020.574366
Jurik, V., Gröschner, A., and Seidel, T. (2014). Predicting students' cognitive learning activity and intrinsic learning motivation: how powerful are teacher statements, student profiles, and gender? Learn. Individ. Differ. 32, 132–139. doi: 10.1016/j.lindif.2014.01.005
Kapur, M. (2014). Productive failure in learning math. Cogn. Sci. 38, 1008–1022. doi: 10.1111/cogs.12107
Koenka, A. C., Linnenbrink-Garcia, L., Moshontz, H., Atkinson, K. M., Sanchez, C. E., and Cooper, H. (2021). A meta-analysis on the impact of grades and comments on academic motivation and achievement: a case for written feedback. Educ. Psychol. 41, 922–947. doi: 10.1080/01443410.2019.1659939
Lee, H., Chung, H. Q., Zhang, Y., Abedi, J., and Warschauer, M. (2020). The effectiveness and features of formative assessment in US K-12 education: a systematic review. Appl. Meas. Educ. 33, 124–140. doi: 10.1080/08957347.2020.1732383
Leighton, J. P. (2019). Students’ interpretation of formative assessment feedback: three claims for why we know so little about something so important. J. Educ. Meas. 56, 793–814. doi: 10.1111/jedm.12237
Lester, F. K. Jr., and Cai, J. (2016). “Can mathematical problem solving be taught?” in Preliminary Answers From 30 Years of Research. Posing and Solving Mathematical Problems: Advances and New Perspectives, eds. P. Felmer, E. Pehkonen, and J. Kilpatrick Springer. 117–135.
Linnenbrink-Garcia, L., Tyson, D. F., and Patall, E. A. (2008). When are achievement goal orientations beneficial for academic achievement? A closer look at main effects and moderating factors. Rev.Int. Psychol. Soc. 21, 19–70.
Lithner, J. (2008). A research framework for creative and imitative reasoning. Educ. Stud. Math. 67, 255–276. doi: 10.1007/s10649-007-9104-2
Lithner, J. (2017). Principles for designing mathematical tasks that enhance imitative and creative reasoning. ZDM 49, 937–949. doi: 10.1007/s11858-017-0867-3
Lorenzo, M. (2005). The development, implementation, and evaluation of a problem solving heuristic. Int. J. Sci. Math. Educ. 3, 33–58. doi: 10.1007/s10763-004-8359-7
Lui, A., and Andrade, H. (2022). Inside the next black box: examining students’ responses to teacher feedback in a formative assessment context. Front. Educ. 7:751548. doi: 10.3389/feduc.2022.751549
Moshagen, M., and Erdfelder, E. (2016). A new strategy for testing structural equation models. Struct. Equ. Model. Multidiscip. J. 23, 54–60. doi: 10.1080/10705511.2014.950896
Murayama, K., and Elliot, A. J. (2009). The joint influence of personal achievement goals and classroom goal structures on achievement-relevant outcomes. J. Educ. Psychol. 101, 432–447. doi: 10.1037/a0014221
Nicol, D. J., and Macfarlane-Dick, D. (2006). Formative assessment and self-regulated learning: a model and seven principles of good feedback practice. Stud. High. Educ. 31, 199–218. doi: 10.1080/03075070600572090
Norqvist, M., Jonsson, B., Lithner, J., Qwillbard, T., and Holm, L. (2019). Investigating algorithmic and creative reasoning strategies by eye tracking. J. Math. Behav. 55:100701. doi: 10.1016/j.jmathb.2019.03.008
Olsson, J., and Granberg, C. (2019). Dynamic software, task solving with or without guidelines, and learning outcomes. Technol. Knowl. Learn. (2019) 24, 419–436. doi: 10.1007/s10758-018-9352-5
Rakoczy, K., Harks, B., Klieme, E., Blum, W., and Hochweber, J. (2013). Written feedback in mathematics: mediated by students' perception, moderated by goal orientation. Learn. Instr. 27, 63–73. doi: 10.1016/j.learninstruc.2013.03.002
Rakoczy, K., Pinger, P., Hochweber, J., Klieme, E., Schütze, B., and Besser, M. (2019). Formative assessment in mathematics: mediated by feedback's perceived usefulness and students’ self-efficacy. Learn. Instr. 60, 154–165. doi: 10.1016/j.learninstruc.2018.01.004
Ryan, R. M., and Deci, E. L. (2020). Intrinsic and extrinsic motivation from a self-determination theory perspective: definitions, theory, practices, and future directions. Contemp. Educ. Psychol. 61:101860. doi: 10.1016/j.cedpsych.2020.101860
Saha, M., Islam, S., Akhi, A. A., and Saha, G. (2024). Factors affecting success and failure in higher education mathematics: Students' and teachers' perspectives. Heliyon 10:e29173. doi: 10.1016/j.heliyon.2024.e29173
Schunk, D. H., and Pajares, F. (2009). “Self-efficacy theory” in Handbook of Motivation at School. eds. K. Wentzel and A. Wigfield (New York: Routledge/Taylor & Francis Group), 35–53.
Shute, V. J. (2008). Focus on formative feedback. Rev. Educ. Res. 78, 153–189. doi: 10.3102/0034654307313795
Sidenvall, J., Granberg, C., Lithner, J., and Palmberg, B. (2022). Supporting teachers in supporting students’ mathematical problem solving. Int. J. Math. Educ. Sci. Technol., 1–21. doi: 10.1080/0020739X.2022.2151067
Stillesjö, S., Wirebring, L. K., Andersson, M., Granberg, C., Lithner, J., Jonsson, B., et al. (2021). Active math and grammar learning engages overlapping brain networks. Proc. Natl. Acad. Sci. USA 118:e2106520118. doi: 10.1073/pnas.2106520118
Strijbos, J.-W., Pat-El, R., and Narciss, S. (2021). Structural validity and invariance of the feedback perceptions questionnaire. Stud. Educ. Eval. 68:100980. doi: 10.1016/j.stueduc.2021.100980
Terwel, J., van Oers, B., van Dijk, I., and van den Eeden, P. (2009). Are representations to be provided or generated in primary mathematics education? Effects on transfer. Educ. Res. Eval. 15, 25–44. doi: 10.1080/13803610802481265
Thurlings, M., Vermeulen, M., Bastiaens, T., and Stijnen, S. (2013). Understanding feedback: a learning theory perspective. Educ. Res. Rev. 9, 1–15. doi: 10.1016/j.edurev.2012.11.004
Van der Kleij, F. M., Feskens, R. C. W., and Eggen, T. J. H. M. (2015). Effects of feedback in a computer-based learning environment on students’ learning outcomes: a Meta-analysis. Rev. Educ. Res. 85, 475–511. doi: 10.3102/0034654314564881
Van der Kleij, F. M., and Lipnevich, A. A. (2021). Student perceptions of assessment feedback: a critical scoping review and call for research. Educ. Assess. Eval. Account. 33, 345–373. doi: 10.1007/s11092-020-09331-x
Verschaffel, L., Schukajlow, S., Star, J., and Van Dooren, W. (2020). Word problems in mathematics education: a survey. ZDM 52, 1–16. doi: 10.1007/s11858-020-01130-4
Wang, S.-L., and Wu, P.-Y. (2008). The role of feedback and self-efficacy on web-based learning: the social cognitive perspective. Comput. Educ. 51, 1589–1598. doi: 10.1016/j.compedu.2008.03.004
Webb, M., and Jones, J. (2009). Exploring tensions in developing assessment for learning. Assess. Educ. Princip. Policy Pract. 16, 165–184. doi: 10.1080/09695940903075925
Winstone, N. E., Nash, R. A., Rowntree, J., and Menezes, R. (2016). What do students want most from written feedback information? Distinguishing necessities from luxuries using a budgeting methodology. Assess. Eval. High. Educ. 41, 1237–1253. doi: 10.1080/02602938.2015.1075956
Winstone, N. E., Nash, R. A., Rowntree, J., and Parker, M. (2017). ‘It'd be useful, but I wouldn't use it’: barriers to university students’ feedback seeking and recipience. Stud. High. Educ. 42, 2026–2041. doi: 10.1080/03075079.2015.1130032
Keywords: motivation, feedback, perceived usefulness, problem-solving, mathematics, mathematical ability, motivational beliefs
Citation: Söderström S, Palm T and Granberg C (2024) The effects of mathematical ability and motivational beliefs on students’ perceptions of feedback usefulness. Front. Educ. 9:1374664. doi: 10.3389/feduc.2024.1374664
Edited by:
Wei Wei, Macao Polytechnic University, ChinaReviewed by:
Heni Pujiastuti, Sultan Ageng Tirtayasa University, IndonesiaGoutam Saha, University of Dhaka, Bangladesh
Copyright © 2024 Söderström, Palm and Granberg. This is an open-access article distributed under the terms of the Creative Commons Attribution License (CC BY). The use, distribution or reproduction in other forums is permitted, provided the original author(s) and the copyright owner(s) are credited and that the original publication in this journal is cited, in accordance with accepted academic practice. No use, distribution or reproduction is permitted which does not comply with these terms.
*Correspondence: Sharmin Söderström, sharmin.soderstrom@umu.se