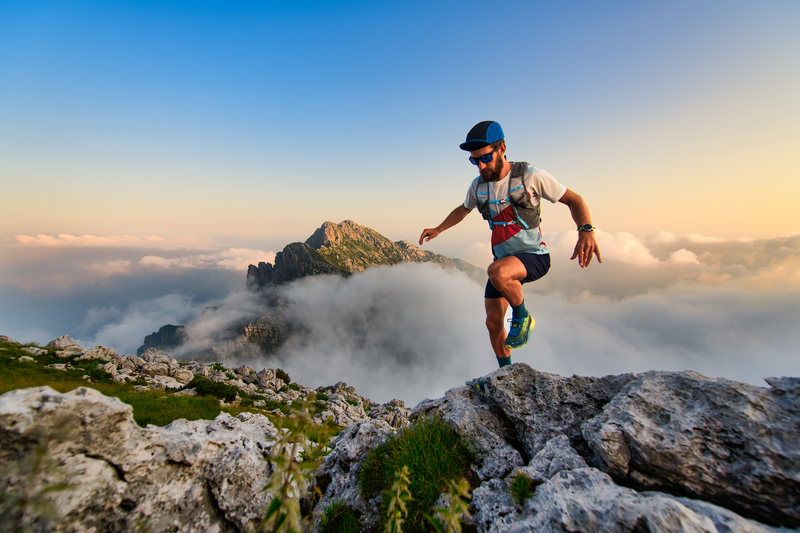
95% of researchers rate our articles as excellent or good
Learn more about the work of our research integrity team to safeguard the quality of each article we publish.
Find out more
HYPOTHESIS AND THEORY article
Front. Educ. , 10 July 2024
Sec. STEM Education
Volume 9 - 2024 | https://doi.org/10.3389/feduc.2024.1372832
This article is part of the Research Topic Mathematical Thinking, Practices, and Processes in Non-formal Learning Environments View all 8 articles
We offer this synthesized framework as a tool to reveal mathematical activity in a non-formal making-space. In particular, we connect research at different grain sizes to illustrate and explain how mathematics plays a crucial, if often implicit, role in making activities. We begin by describing the Approximate Number System and the Ratio-Processing System, and explaining how those systems connect to both embodied cognition and Thompson’s (1994) conceptualization of quantities. Then, we examine how prediction and anticipation relate, with a particular emphasis on how social feedback guided the emergent mathematical activity. We offer this framework as a way to perceive, account for, and understand the multi-layered nature of experiencing mathematical activities.
Four years ago, the first and third author were crowded around a laptop, watching the video of a preschool-aged boy trying to put together pieces of plastic and metal to build a battery-powered car in a non-formal making-space. We watched him twist and turn and snap pieces together, trying different shapes and angles. He failed to fit two pieces together, put one of the pieces down, picked up another piece, failed again, and then rotated the new piece until it finally fit. He paused, looked at the shape of the car with the new piece attached, then cast around for another piece to add. We thought that perhaps mathematical play scholars would identify him as engaging in mathematical activities (e.g., Wager and Parks, 2014).
But then we watched a video of 4th grade students placing pieces of tape on the floor, and a video following two high school students creating a robotics car as part of a Science Olympiad1 competition. In both videos, the students fit (and failed to fit) pieces together, rotated and twisted pieces and their bodies, thought and talked about their robots moving in a variety of ways, compared what they saw with what they wanted to happen, and revised their activities accordingly. We started to wonder how much mathematical activity the field of mathematics education would see, even as they were engaging in similar actions to the little boy.
“There’s definitely mathematical play happening,” Caro said. “But I’m not sure how we can convince other mathematics educators - their mathematical activity does not look or sound enough like math.”
“Even though it’s just as much math as the young boy. So how do we describe the mathematics they are experiencing and experimenting with?” asked Amber.
This manuscript is our answer to that question - a theoretical synthesis across the scales of mathematical activity, in order to provide layers of different types and grain sizes of evidence. We know that identifying mathematical cognition in non-formal contexts where formal math representations play little to no role can be a difficult proposition. Often, spoken, written, and notation-based performances are taken as evidence of mathematical activity, and a tempting corollary is that mathematical activity is thus evidenced by and through the same representations. Furthermore, formal educational contexts focus primarily on explicit learning - that is, learning that falls within the spectrum of consciousness (Nathan, 2021), where learners.
are given the goals explicitly, directed to learn a specific skill or pattern, shown its structure, and then engage in the learning activity. … Conscious learning processes are generally well remembered, subject to explicit monitoring and control, and can often be accurately described with words (p. 75).
However, the mathematics in activities that have low-to-no formal representations can be easily missed, even when the learners are experiencing a mathematical activity and experimenting with mathematics. This is because a hallmark of the low-to-no notation environments we examine (such as non-formal making-spaces2) is centering the participants’ interests and goals throughout the activity - in fact, we could say that our learners ‘are not given the goals explicitly, not directed to learn a specific skill or pattern, not shown its structure, and then engage in the learning activity.’ As a result, our participants often engage in implicit learning, outside of the spectrum of consciousness, which is characterized as generally opposite to explicit learning. The most important feature of such implicit learning is that describing such learning is difficult - to remember and/or to verbalize (Nathan, 2021). Consequently, in our work (Katirci et al., 2021; Shokeen et al., 2021), we link between different scales of learning in order to reveal and understand mathematical experiences that may not be otherwise visible to the learners themselves, much less observers who rely upon the externalization of learning as evidence of learning. During this previous work, we developed and subsequently used a new framework for identifying mathematical activity in such low-to-no notation environments, and we share, illustrate, and argue for the importance of such a framework here.
Our theoretical development of this framework will begin here, by focusing on the participant within a mathematical activity. We do so by describing two primitive structures present in the typical human brain (the Approximate Number System and the Ratio-Processing System; e.g. Matthews et al., 2015), tying those neural structures to Alibali and Nathan’s (2012) embodied cognition view of perception and action, and interpreting both of those frameworks through Thompson’s (1994) conceptualization of quantities. Then, we expand from a participant-centered perspective to the social and material context of that participant. We introduce prediction (Bieda and Nathan, 2009), and participation and anticipation (Tzur, 2007) as components of mathematical activity, and describe how our adaptation of failure paired with feedback (e.g., Williams-Pierce et al., 2021) manifest within the collaborative youth making activity under examination. In short, we begin ‘zoomed in’ at the neuron level, then increasingly ‘zoom out’ layer by layer in increasing grain size until we reach interpersonal interactions and their roles in the mathematical activity (see Figure 1). We conclude this article by exhorting researchers to use such a layered lens in low-to-no notation mathematical activity environments, and emphasizing the need to connect this framework to mathematical learning in future theoretical work.
We took this approach of ‘zooming out’ as a way to understand the layered experience of mathematical activities at each increase in grain size, even though this layering is not linear. For example, the first layer may contribute to the second layer, which then contributes to both the first and the third layer, and so on. We have left the circles in Figure 1 open instead of closed to so indicate, and move fluidly between the layers in our analyses later in the paper, to better emphasize this nonlinearity of mathematical activity.
We conceptualize each increase in grain size as an increase in timescale, as “every process, action, social practice, or activity occurs on some timescale” (Lemke, 2000, p. 275). While most research theorizes at a particular layer of process timescale, our framework threads from the fast (e.g., neurons) outside the conscious spectrum, to the slower (e.g., tools in use) that occurs within the conscious spectrum. We consider this threading to be crucial to centering the human experience in our work - after all, being human at a moment in time begins with our perceptions, our embodiedness, and then spans out to include the material and social context of our body.3 At each layer of human experience, ranging from milliseconds to years, different theories of mathematical learning and being in the world have been proposed. In order to better account for a singular human moment and mathematical experience, we leveraged appropriate theories at each timescale. While developing stronger connections in the liminal space between each timescale is a future goal, we offer this paper as a way to share the theoretical and methodological work we have already done and applied.
Our primary approach for this paper is theoretical, but built directly through observing and analyzing video data with the aim of examining it for evidence of mathematical play (e.g., Katirci et al., 2021; Williams-Pierce et al., 2021). The research team who analyzed the data is composed of members with varying areas of expertise. Three are experts in embodied cognition, in both physical and digital learning contexts; one specializes in mathematics learning in maker- and making-spaces (and originally collected the video data); one specializes in mathematical play. All five have considerable expertise with mathematics learning in both formal and non-formal contexts. The multidisciplinary nature of the team is how we developed our comprehensive framework over time, as our collaboration during analysis revealed both the need and the expertise for developing this framework. In the remainder of this section, we describe the source of the video data, in order to contextualize the illustrative example we use.
Our video data is composed of three video records of the same 20 min of a collaborative robotics activity with a group of five 4th grade students (3 boys and 2 girls, pseudonyms Ryan, Peter, Aaron and Fawn, Almond, respectively). Two of the video records were from the perspective of two students wearing GoPro cameras on their chest, while the third was a standing camera that captured the entire group’s activity from a slight distance. The activity took place within the context of a physical classroom located in a public school as part of a broader TinkerLab activity series. Consequently, the classroom was treated as a non-formal making-space (Simpson et al., 2023) by the facilitating teachers and students alike, and the students had all volunteered to participate in the TinkerLab. The robotics activity had two phases: Phase 1, the groups put masking tape on the floor to establish a path; and Phase 2, the groups switched places, seeking to measure the path built by the other group, and to program a robot, Dash, to successfully travel it. Crucially, while the students were given the goal of and tools for each Phase, they were not instructed specifically on how to achieve the goals with the given tools – rather, each goal required the students, as a group, to interpret the goals in order to collaboratively complete each Phase. We describe each phase in more detail later in the paper, with Phase 1 used to illustrate the first three layers of our framework, and Phase 2 used to illustrate the next two layers. The final layer is illustrated using data from both phases. We have split the phases and layers up in this fashion to try to simplify what is quite a complex data set.
We will now introduce the first three layers of our framework, then describe two specific data excerpts while sharing what each layer reveals about the excerpt. Our review of these first three layers will focus on each individually, but the following vignettes will describe in more detail how the layers interact and intertwine during mathematical activity. These data excerpts were selected specifically because of their clarity - that is, Phase 1 is composed of multiple moments that are as richly layered as these examples, but not all are as simple to reveal and describe to a newcomer.
We begin at the smallest grain size: the neurons that compose the learner’s brain (Figure 2). In particular, the Approximate Number System (ANS) ties estimation of a number of objects directly to certain animal neuron activation patterns, including humans (e.g., Dehaene, 1997; Matthews et al., 2015). A human adult, glancing at a set of three objects on a table, immediately subitizes: they know automatically and without conscious thought that there are three objects present (e.g., Miller, 1956). If that human adult is shown three objects repeatedly, the part of their brain responding to those three objects begins firing less actively as the perceiver becomes habituated to the number of objects being subitized. In such a situation, if a fourth object is added, there is a small increase in relevant brain activity; whereas if three more objects are added (making six in total), a larger increase in relevant activity occurs. In other words, when the number of objects being perceived increases slightly, there is little increase in brain activity; but if the number increases considerably, so does the brain activity (e.g., Dehaene, 1997; Piazza et al., 2004).
Building upon the ANS, Matthews et al. (2015) describe the Ratio-Processing System (RPS) as a neural system in which we intuitively and immediately perceive and compare magnitudes4 of objects. With the ANS and the RPS as primitive structures that perceive and compare magnitudes, certain components of perception are built directly into our brains, while our bodies have additional perceptual systems that build upon those neuronal perceptions of magnitude or otherwise influence our cognition. Alibali and Nathan (2012) describe perception and simulations of perception:
When humans perceive objects, they automatically activate actions appropriate for manipulating or interacting with those objects (Ellis & Tucker, 2000; Tucker & Ellis, 1998). Thus, imagining an object can evoke simulations of perception (i.e., of the actions associated with perceiving the object) or of potential actions involved in interacting with the object. (p. 254).
Perception, whether based upon primitive numerical structures or otherwise, leads to action (such as gesture, physical movement upon the environment, or spoken language), and that action leads back into our perception. This feedback loop of perception, action, and imagining is described as mental simulation (Alibali and Nathan, 2012), and together compose the embodied nature of our cognition (Figure 3). This feedback loop can be evidenced through spoken or written language, physical movements that impact the physical world, or - often - can only be inferred by an outside observer through expression of gestures. These gestures are communicative acts that reveal perception and action in a variety of ways, such as through pointing (deictic) gestures that connect spoken language with objects or people in the physical environment or representational (such as iconic or metaphoric) gestures that directly reflect the state of perceptions and planned actions of the gesture. Consequently, we rely upon action and gesture as both composing and revealing perception, action, and their composite into cognition.
Lastly, our theoretical bridge between perception and action and the mathematical characteristics of the external world, Thompson (1994) specifically defines quantity as a conceptual entity - that is, quantity does not reside in the object, but rather in the perceiver (Figure 4). As noted above, our references to magnitude should be taken to refer to both Matthews et al.’s (2015) use of the term quantity, and to the perceived quality of an object or representation of taking up space [re: Thompson (1994) definition]. Thompson (1994) goes on to define quantity as a schematic that involves “an object, a quality of the object, an appropriate unit or dimension, and a process by which to assign a numerical value to the quality” (p. 184). We further describe these three layers using two vignettes in the next section.
We use examples solely from Phase 1 in this section, and consequently describe the Phase 1 activity here in more detail (Phase 2 is described when we analyze the remaining layers). In this phase of activity, our five students are given a roll of masking tape and told to create a path from one end of the classroom to the other. The students, knowing that the path they made would be used by the other group in Phase 2, decided to create a challenging path. As a result, throughout the entire phase, the students negotiated what ‘challenging’ meant, in terms of the lengths of the path and the angles between two neighboring lengths (e.g., Shokeen et al., 2021). Our illustrative examples for this manuscript come from this phase.
Our first data excerpt begins with Aaron (crouching on the floor in red shorts), who placed the sticky edge of the tape on the end of the already placed path, and unrolling the still-attached roll of tape, smoothing it down as he goes, to create the next length of path (Figure 5A). Peter then says, “Too big!” about the piece of tape Aaron is placing, as longer pieces of path are not as ‘challenging’ as short pieces connected by angles. Then (Figure 5B) Aaron starts rolling the tape back up to shorten the path, and (Figure 5C) Peter helps him rip and place the final piece.
Figure 5. (A) Aaron unrolls the tape; Peter says, “Too big!”; (B) Aaron starts rolling the tape back up to shorten the piece; (C) Aaron and Peter rip the tape (length represented by red line).
First, Peter’s judgment of “too big” indicates that the speaker is perceiving the length of the masking tape as a quantity by Thompson’s (1994) definition: the masking tape is the object; the length of the piece of masking tape is the quality they are considering; and the internal standard for magnitude is an appropriate unit or dimension. Although our participants did not have access to a measuring tape in order to assign a numerical value to the quality of length, they may have done such measuring if the tool had been present (as we have evidence they appropriately used such a tool in Phase 2). In other words, Peter is using quantity as conceptualized by Thompson (1994), and that quantity is perceived and then compared with a simulated perception (Alibali and Nathan, 2012) of appropriate unit or dimension. This perception and comparison of length is rooted in the speaker’s ANS and RPS: although a lack of discrete or explicit measurement makes it difficult to determine how their ANS is contributing, the comparison of the physical length’s magnitude with their imagined unit’s magnitude can be directly attributed to their RPS.
The speaker’s comparison of the magnitude of the tape with their internal standard presents a communication problem, as they must externalize their internal standard in some fashion for their group mates. One potential method of externalizing might be gesturing what “too long” is - while this does not externalize the internal standard, it indicates what magnitude the speaker is considering to be too much, which implies that the desired length of tape should be shorter. Another potential method was to shorten the piece by ripping the tape: this would serve to indicate what an appropriate length of tape would be, while requiring fellow perceivers to examine the magnitude of a resulting piece of tape in order to evaluate whether the new pieces are perhaps “too short.” When a piece was too short, the choice of actions was different: they were crumpled up and thrown away, or used to extend a pre-existing length of tape already on the floor. While these actions and gestures may differ from each other and from the action of rolling the tape back up as shown in the vignette, they each indicate the same perception of magnitude, the quantification of that magnitude, and a comparison to an internal standard. Our next illustrative excerpt comes from the same group of participants and the same phase of activity, but focuses on perception of magnitude of angle, instead of length.
In this vignette, two pieces of tape have already been placed, and Ryan tentatively places a third strip of tape down at what he mentally simulates to be a “10-degree” angle (Figure 6, left). As the group does not have access to tools for measuring angles (e.g., a protractor), Ryan uses two modalities to communicate the magnitude of the angle which he is simulating: verbally, through mathematical language, and concretely, by lightly placing the tape down. Pairing these two modalities means that other participants who may have different mental simulations of a “10-degree” angle from Ryan’s, can use their own perception of the magnitude of angle that he has created as an external representation of his simulation and perception.
Figure 6. Ryan begins to lay the next piece of tape down at a tight angle to the previous length (left); Fawn moves the tape over to increase the angle (right).
Following the tightness of Ryan’s proposed angle, the group discussed whether such an angle could actually be navigated by Dash. As Dash brings its own constraints - through mobility and the design of the controlling software - the participants compared their mental simulation of Dash’s ability to take angles with their perception of the magnitude of angle as manifested by Ryan’s tape. Fawn said, “Can we do a ten degree angle? I do not think Dash can do ten degree angles,” and was confirmed by the teacher, who had overheard her: “Dash cannot do like really tight angles.” Almond suggested making the angle “a little wider,” pairing her language with a gesture that moved her hands apart to make the magnitude of her ‘a little wider’ visually available to everyone else. Fawn followed her suggestion up by moving the end of the piece of tape further to the right and expanding the angle (Figure 6, right) to what became the established location of the third piece of tape.
Similarly to Vignette #1, the speaker’s comparison of the magnitude of the angle with their internal standard presents a communication problem, as they must externalize their internal standard in some fashion for their group mates. Ryan externalized his preferred magnitude in language and in concrete action by placing the tape, perhaps because the magnitude represented by “10-degrees” may not share consensus (that is, Ryan’s mental simulation of 10 degrees may be different from the mental simulations of others in the group). Another potential method of externalizing could be gesturing what “10-degrees” is - much as Almond externalized the magnitude of “a little wider” through gesturing. Then, participants introduced a new constraint based on their past experience with Dash. They mentally simulated Dash’s ability to turn, and compared that simulation to their simulation of the turn that would be required with Ryan’s placement of the tape. As the comparison of those two magnitudes found Dash’s maneuverability wanting, they then communicated Dash’s angle movement constraints through language and gesture, and proposed a different magnitude of angle that Dash could navigate.
This vignette highlights how each participant perceived and quantified the magnitude of angles, compared with their own internal standard of the desired angle. While the language, actions, and gestures may differ from each other and from those shown in Vignette #1, they each indicate the same perceptual bases for magnitude, quantification of that magnitude, a comparison to an internal standard, and resulting communication about preferred magnitudes, whether of length or angle.
We use Tzur’s (2007) description of two stages in mathematical activity (participation and anticipation) operationalized through Bieda and Nathan’s (2009) description of prediction to specifically identify moments of failure and feedback (e.g., Williams-Pierce, 2019). In this section, we focus on the next two layers of mathematical activity. See Figure 7 for the added layer.
Tzur’s (2007) two stages in mathematical activity are defined as participation and anticipation5. During the participatory first stage, the learner has a mathematical understanding that emerges only when prompted by the activity at hand, and cannot be independently demonstrated without the contextual cues or tools. During the anticipatory second stage, however, “the learner can independently call up and utilize an anticipated activity-effect relationship proper for solving a given problem situation” (p. 278) - in other words, they are able to use their mathematical understanding without engaging in the activity first. Since we are examining a single group activity rather than a single student engaging in multiple mathematical learning contexts, we operationalize Tzur’s definition using Bieda and Nathan’s (2009) conceptualization of prediction.
Bieda and Nathan (2009) describe prediction as looking at a pattern, and predicting a later instance of that pattern, whether near or far. While Bieda and Nathan focused on a function represented on a Cartesian plane, and having students predict a point on the function that appears beyond the represented plane, we adapted their work to our context and content. In particular, we consider every piece of programming to be an indication of prediction - the students are predicting that the code they input will result in Dash traversing the tape path. Although this may seem quite different at first blush, Bieda and Nathan’s focus on mathematics, overt predictive work by students prior to execution, and representational fluency - that is, engaging in mathematical activities that involve moving between multiple forms of the same phenomena – aligns powerfully with our own work (e.g., Simpson et al., 2021; Katirci et al., 2022).
When students engage in prediction, we consider them to be engaging in the anticipation stage; when their predictions fail to manifest as expected, we examine how they adjust in the moment. In those moments of failure, we use an adapted version of Williams-Pierce’s (2019) failure and feedback framework, originally developed for mathematical play within videogames. In this case, a moment of failure is indicated by what Tzur (2007) describes the “the well-known ‘oops’ experience” (p. 277) in the participatory first stage, where a student does something, notices a mistake as it manifests in their activity, and goes on to adjust it in the moment. We consider this ‘oops experience’ to be a moment of recognizing the failure of their prediction, alongside the attendant feedback of what actually happened - that is, an in-activity adjustment due to the failure and feedback pairing. Such failure and feedback can take multiple forms - here, we focus on a single form: the physical manifestation of failure and feedback through Dash enacting code. See Figure 8 for the added layer.
In this phase of the activity, the goal for the group was to make a single code-based program for Dash, so that the robot would travel accurately on a new path (designed by the other group). The tools available were a roll of measuring tape, a pencil, an iPad loaded with Blockly (the software for programming Dash; see Figure 9, left), and Dash itself. Figure 9, right shows the path and Dash, as well as Ryan, the student in the red shirt, actively measuring a segment of the path. To achieve this goal, students needed to measure the path, then program those measurements into Blockly, as well as the direction and angle between segments.
Figure 9. (Left) The app Blockly on an iPad; (right) has multiple important components – the path in yellow tape; Dash, the blue robot on the yellow tape; Ryan, in the red shirt with the measuring tape laid over a segment of the path.
In this excerpt, the students were engaged in the activity of measuring, then programming Dash to travel over the first length of tape, turn 90 degrees to the right, and then travel over the second piece of tape. We now analyze this excerpt with our additional theoretical framings to help reveal what happened as a result of that activity.
This vignette involves students in the anticipatory phase using the measuring tape and pencil to measure segments of the path. We consider them to be in the anticipatory phase because they are efficiently measuring and annotating their measurements on the path, anticipating (predicting) that those measurements will be accurate and appropriate for programming Dash. Other indicators of the anticipatory phase are evidenced by a lack of indicators of the participatory phase: for example, the students do not discuss in detail how to use the measuring tape, explicate any moments of failure during their measuring, or make adjustments to their measuring in-activity in order to improve their measuring practices. The activity of measuring simply occurs, smoothly and without remark, as they engage in the Thompson’s quantification process: the object is the tape path; the quality of the object is the length of a segment of the tape path; the appropriate unit is centimeters; and the process by which to assign a numerical value to the quality is to place the measuring tape over the segment and annotate the result on the tape path itself.
However, a complication with this quantification emerges during the programming of Dash. Although the measuring process is occurring within the anticipatory phase, the use of those measurements - and the programming and enacting of Dash’s code - is occurring within the participatory phase, as students recognize various aspects of the activity they did not expect. In particular, as the students program Dash to travel over the first part of the path, which has been measured to be 90 centimeters, they do not account for the fact that Dash is a broad object. In other words, how Dash is placed on the starting point - perhaps it is just at the very tip of the tape, or perhaps Dash’s center is located on the tip of the tape - greatly influences its final location. Dash will go 90 centimeters regardless, but placing Dash at the very tip of the tape will result in a closer location to the start than if Dash is centered on the beginning of the tape.
In this part of this vignette, students programmed Dash to travel over the first length of tape, turn 90 degrees to the right, and then travel over the second piece of tape. However, when they placed Dash on the starting point of the line, they placed Dash’s ‘front’ at a slight angle to the tape, resulting in Dash getting further to the left of the tape path with every movement forward (see Figure 10A). After Dash turned 90 degrees to the right (Figure 10B), its forward movement just brought it to the end of the first part of the tape path - that is, essentially correcting for the angled start (see Figure 10C), instead of traversing the second length of tape.
Figure 10. (A) Dash is slightly off the path after traveling the first length; (B) Dash turns 90 degrees to the right; (C) Dash travels forward and stops right at the end of the first length of the path.
The students inputting Dash’s code indicates their prediction that Dash will - in enacting their code - result in Dash correctly traversing the first part of the path, turning, and finishing at the end of the second part of the path. However, as their prediction failed to materialize and they experienced an ‘oops’ moment (a moment of failure), they realized that they failed to arrange Dash’s ‘front’ correctly so that it was aligned with the tape path. Dash ending up off the path is their visual feedback (paired with that moment of failure), which supported them in adjusting their activity and prediction successfully. As additional evidence that their input code acts as a prediction, when they put Dash back at the beginning of the path, carefully make sure the front is aligned with the path, and then re-run the same program, they chant “perfect, perfect, perfect” with each move, confirming that Dash is traveling exactly how they wanted and predicted.
This ‘oops’ moment indicates that they are in the participatory phase, recognizing in the moment the visual feedback that indicates their failure. In particular, the students are perceiving the path Dash is traveling, and comparing that path with the path they predicted – this comparison leads to the students experiencing the failure of their prediction and correctly interpreting that attendant in-activity visual feedback. This recognition and interpretation supports the students in being able to shift into the anticipatory phase, as Dash’s directionality requires that they attend to exactly how Dash is placed before executing the code. In other words, if they did not perfectly line up Dash in all future attempts, Dash would end up in a variety of different places each time, which would prevent the students from seeing whether or not their code was actually correct. This one instance of this error was sufficient, however, and the students never again placed Dash without correctly positioning the front. In summary, the result of failure and feedback in the participatory phase helped the students recognize a requirement of the activity that made it actually possible for them to achieve the anticipatory phase.
Our final layer extends failure and feedback to the social environment, resulting in a description of how social feedback influenced the collaborative mathematical activity (Figure 11). In other words, we shift to describing how failure and feedback also manifest socially, as a key component of collaboration and interaction within this low-to-no notation environment. Social feedback is our operationalization of how these participants engaged in collaboration throughout the activity, with each participant bringing their own perception of quantity, anticipation, and so on from the previous layers. As a result, each layer interleaves complexly with the other layers from the other participants, and consequently, we have given this final layer its own section, and we present examples from both phases here.
The role of social feedback was particularly crucial in Phase 1, as there was a lack of mathematizing tools: each student had to use their own perception and mental simulation of quantities, as no more precise method was at hand. For example, at one point the students decided that they wanted to lay the path underneath two chairs that are tucked under a table. As one student began laying the tape underneath the chairs, another student, Hannah, said something in a doubtful tone (not captured on audio), while tracing the floor under the chairs to indicate that there might not be enough room for Dash (Figure 12, left). Aaron says, “No no, that would work” and Ryan agrees, also tracing the floor under the chairs. As Hannah spoke, she was mentally simulating her perception of the size (quantities) of Dash, comparing that mental simulation with her perception of the space available underneath the chair, and visualizing a conflict between those two perception-based simulations such that Dash would run into the chair, rather than go smoothly underneath it. Aaron and Ryan, though, are either engaging in different mental simulations – one in which Dash fits under the chairs – or are merely thinking of Dash following the path (a participatory view), while Hannah was anticipating, and using that anticipation to predict that some issues would arise. Aaron and Ryan keep placing the tape, and then Peter joins to place the last piece of tape that brings the path out from under the chairs (Figure 12, middle). As Peter finishes, he says, “We should move the chairs out, too, if it does not fit,” and Ryan says, “Yeah.” Then, when Phase 1 is ending, and the group is leaving their tape path for the other group to use, this group runs back to remove the chair from the path, indicating that the mental simulations of Peter and Hannah have convinced the others that Dash probably will not fit (Figure 12, right). In other words, this is a moment of social feedback, in which members of the group convinced other members that a moment of failure (Dash getting stuck under a chair) was imminent unless adjustments were made.
Figure 12. Hannah tracing the tape under the chairs (left); Peter placing the last piece of tape (middle); and moving the chairs at the end of Phase 1 (right).
As another example from Phase 2, students are using a measuring tape and a pencil to measure a part of the path, and then write their measurement of that strip of tape on the path. After measuring and writing down the measurement, they move directly on to measuring the next part of the path without commenting, because they have successfully completed a step of the measurement. Measuring by itself is an activity that can be successful or unsuccessful in itself, even before Dash enacts the measurement – but the data showed no example of the students accidentally flipping the measuring tape to the inches side, or noticing any other potential measuring issues that could happen. This illustrates the other side of the ‘oops moment,’ because it is a ‘we measured the path appropriately and are not surprised by it’ moment. Sometimes, the students are successful but remark on their success, such as when Aaron coded Dash to traverse the first three lines and the angles within them, and after Dash ended up in the correct spot, Aaron said, “That’s perfect!” We consider this to be an example of social feedback paired with success, rather than failure, and an illustration of the participatory stage rather than anticipatory, because they were at least mildly surprised that it worked (e.g., they lacked confidence in their prediction), unlike when using the measuring tape.
We have described all six of our layers for theorizing about mathematical activity (Figure 11), each building upon the previous layers, using one of the many rich moments of mathematical activity to describe what each layer looked like in situ. These first three layers describe how quantities in the world are perceived by our neuronal structures, and we mentally simulate and act upon those perceived quantities. In other words, this part of the framework describes a perceiver-centered view of how different layers of experience are involved in implicitly mathematizing our world, in order to better reveal the mathematical activity within a low-to-no notation environment. Then, we moved up to the next two layers, which expanded further around the participants into their shared spaces and community, and provided insight into how the implicit mathematizing from the first three layers builds the foundation for the next layers of mathematical activity. In particular, we focused on how mathematical predictions and anticipation of certain mathematical results contribute to mathematical activity, and how feedback contributed to the shifting shape of mathematical activity.
We then separated out the final layer, social feedback, because the relationship of social feedback to other aspects of our theoretical layers can be quite complex. For example, prediction, anticipation, and social feedback combined in an example from Phase 2 where Dash went too far and moved off the tape path. The students had input a centimeter measurement that they anticipated and predicted would lead Dash to the correct location on the tape. Consequently, when Dash stopped at the wrong place, the students received that paired feedback and failure, and amplified that feedback and failure through talking about it (e.g., social feedback). However, often feedback and failure are not clearly evident, because if what the students predicted would happen did happen, they had no need to remark upon it. In situations like this, where feedback and failure are missing, and the students move on to the next step, we concluded that they were content with their previous work. We also suggest that this is an indicator that students have shifted from participatory to anticipatory, because they have learned/internalized what to do or not to do, which results in no failure and often no social feedback. However, how social feedback relates to the other layers deserves further attention in future research.
The primary goal of this framework is to support scholars in identifying rich mathematical activity that may not look very mathematical at first glance, such as occurs in non-formal making spaces. Threading through multiple scales of the human experience serves to build up layers of evidence of mathematical activity, revealing the complex interplay of perception and simulation of quantities underlying choices about simple pieces of tape. These layers offer a multi-layered view of activity that can serve as a guide for understanding mathematical learning in similar contexts. In particular, we anticipate that the six layers can act as guideposts for researchers seeking evidence of learning in low-to-no notation environments. Examining the role of perception, simulation of magnitude, and quantification can both reveal, establish, and value the implicit mathematization of activities that otherwise lack explicit characteristics of mathematical activity and learning. Then, the additional three layers incorporate the embodied and social nature of mathematical learning - how tools and community can influence our learning as we move through mathematical activities with other learners. We hope that future research investigates the relationship between often-implicit mathematical activity, as we describe here, and often-explicit mathematical learning, as is particularly valued in formal learning contexts.
With this work, we hope to contribute to the “domestication of the eye” described by Radford (2010, p. 4) in his work on understanding the embodied nature of mathematical seeing and objectifying. However, the eye we wish to domesticate is our own - that of mathematics educators and researchers who likewise seek to connect up and through the layers of human mathematical experience. In particular, we hope the field will use these layers when examining low-to-no notation environments for mathematical activity and value, regardless of the age group, context, or mathematical content. Future work will focus specifically on connecting the layers of mathematical activity directly to how mathematical learning occurs within and between each layer – but for now, the goal is to merely see and respect the mathematical activity.
The data analyzed in this study is subject to the following licenses/restrictions: Video data of children - not to be distributed. Requests to access these datasets should be directed to Dr. Simpson, YXNpbXBzb25AYmluZ2hhbXRvbi5lZHU=.
CW-P: Conceptualization, Formal analysis, Investigation, Methodology, Writing – original draft, Writing – review & editing. NK: Conceptualization, Formal analysis, Investigation, Methodology, Writing – original draft, Writing – review & editing. AS: Investigation, Methodology, Resources, Writing – original draft, Writing – review & editing. ES: Conceptualization, Formal analysis, Investigation, Visualization, Writing – original draft, Writing – review & editing. JB: Conceptualization, Investigation, Writing – original draft, Writing – review & editing.
The studies involving humans were approved by University of Binghamton SUNY IRB. The studies were conducted in accordance with the local legislation and institutional requirements. Written informed consent for participation in this study was provided by the participants’ legal guardians/next of kin. Written informed consent was obtained from the minor(s)’ legal guardian/next of kin for the publication of any potentially identifiable images or data included in this article.
The author(s) declare that financial support was received for the research, authorship, and/or publication of this article. Partial funding for open access provided by the UMD Libraries’ Open Access Publishing Fund.
Previous portions of this paper were presented at the 2021 Psychology of Mathematics Education - North American Chapter conference (Simpson et al., 2021; Williams-Pierce et al., 2021).
The authors declare that the research was conducted in the absence of any commercial or financial relationships that could be construed as a potential conflict of interest.
All claims expressed in this article are solely those of the authors and do not necessarily represent those of their affiliated organizations, or those of the publisher, the editors and the reviewers. Any product that may be evaluated in this article, or claim that may be made by its manufacturer, is not guaranteed or endorsed by the publisher.
1. ^More information about Science Olympiad available here: https://www.soinc.org/about/mission.
2. ^Our use of making-space (instead of makerspace) is to emphasize the active process of making while also engaging in the active process of becoming a maker. That is, our participants do not necessarily identify as makers, but are still engaging in the process of making. This distinction is further outlined by Simpson et al. (2020, accepted).
3. ^And can be spanned out again to include the larger timescales, such as the socio-historical context of our body, and so on. However, these further layers are beyond the immediate scope of this paper.
4. ^Although Matthews et al. (2015) describes ‘quantities’ as an inherent quality of magnitude of an object or representation, we instead refer to that as magnitude, and reserve the term quantity for Thompson’s (1994) definition.
5. ^Tzur (2007) actually titled these stages participatory and anticipatory. He uses ‘anticipation’ as a form of ‘anticipatory,’ but avoids using ‘participation’ as a form of ‘participatory’ (we hypothesize because of the very common usage of the word ‘participation’ with quite a different definition). We switch regularly between both forms of both terms throughout this manuscript.
Alibali, M. W., and Nathan, M. J. (2012). Embodiment in mathematics teaching and learning: evidence from learners' and teachers' gestures. J. Learn. Sci. 21, 247–286. doi: 10.1080/10508406.2011.611446
Bieda, K. N., and Nathan, M. J. (2009). Representational disfluency in algebra: evidence from student gestures and speech. ZDM 41, 637–650. doi: 10.1007/s11858-009-0198-0
Dehaene, S. (1997). The number sense: How the mind creates mathematics. NY, New York: Penguin Press.
Katirci, N., Shokeen, E., Simpson, A., and Williams-Pierce, C. (2022). Attending to the missing role of gestures in representational fluency. Roundtable presented at the 2022 American Educational Research Association Annual Meeting and Exhibition. San Diego, CA.
Katirci, N., Shokeen, E., Simpson, A., and Williams-Pierce, C. (2021). Making with math: Extending a mathematical play framework to informal makerspaces. Paper presented at the American Educational Research Association Annual Meeting and Exhibition
Lemke, J. L. (2000). Across the scales of time: artifacts, activities, and meanings in ecosocial systems. Mind Cult. Act. 7, 273–290. doi: 10.1207/S15327884MCA0704_03
Matthews, P. G., Lewis, M. R., and Hubbard, E. M. (2015). Individual differences in nonsymbolic ratio processing predict symbolic math performance. Psychol. Sci. 27, 191–202. doi: 10.1177/0956797615617799
Miller, G.A. (1956). The magical number seven, plus or minus two: Some limits on our capacity for processing information. Psychological Review, 63, 81–97. doi: 10.1037/h0043158
Nathan, M. J. (2021). Foundations of embodied learning: A paradigm for education. 1st Edn. NY, New York: Routledge.
Piazza, M., Izard, V., Pinel, P., Le Bihan, D., and Dehaene, S. (2004). Tuning curves for approximate numerosity in the human intraparietal sulcus. Neuron 44, 547–555. doi: 10.1016/j.neuron.2004.10.014
Radford, L. (2010). The eye as a theoretician: seeing structures in generalizing activities. Learn. Math. 30, 2–7.
Shokeen, E., Simpson, A., Katirci, N., and Williams-Pierce, C. (2021). “Use of zig-Zag to represent mathematical thinking about angle” in Proceedings of the forty-third annual meeting of the north American chapter of the International Group for the Psychology of mathematics education. eds. D. Olanoff, K. Johnson, and S. Spitzer (PME-NA: Philadelphia, PA), 323–324.
Simpson, A., Kastberg, S., and Williams-Pierce, C. (2023). Norms and collaboration in hybrid making spaces. J. Educ. Res. 116, 134–146. doi: 10.1080/00220671.2023.2207191
Simpson, A., Katirci, N., Shokeen, E., Bih, J., and Williams-Pierce, C. (2021). Representational fluency of angle during an educational robotics task. in Proceedings of the 15th International Conference of the Learning Sciences. Eds. E. de Vries, E., Y. Hod, and J. Ahn. Bochum, Germany: International Society of the Learning Sciences. 529–532.
Simpson, A., Williams-Pierce, C., and Kastberg, S. (2020). “When figured worlds fracture: a collaborative environment splintered by a non-collaborative tool” in Proceedings of the 14th international conference of the learning sciences. eds. M. Gresalfi and I. S. Horn, vol. 3 (Nashville, TN: International Society of the Learning Sciences), 1673–1676.
Thompson, P. W. (1994). Images of rate and operational understanding of the fundamental theorem of calculus. Educ. Stud. Math. 26, 229–274. doi: 10.1007/BF01273664
Tzur, R. (2007). Fine grain assessment of students’ mathematical understanding: participatory and anticipatory stages in learning a new mathematical conception. Educ. Stud. Math. 66, 273–291. doi: 10.1007/s10649-007-9082-4
Wager, A. A., and Parks, A. N. (2014). “Learning mathematics through play” in The SAGE handbook of play and learning in early childhood. eds. L. Brooker, M. Blaise, and S. Edwards (London, UK: SAGE Publications), 216–227.
Williams-Pierce, C. (2019). Designing for mathematical play: Failure and feedback. Information and Learning Sciences 120, 589–610. doi: 10.1108/ILS-03-2019-0027
Williams-Pierce, C., Katirci, N., Simpson, A., Shokeen, E., and Bih, J. (2021). Revealing mathematical activity in non-formal learning spaces. In D. Olanoff, K. Johnson, and S. Spitzer (Eds.), Proceedings of the forty-third annual meeting of the north American chapter of the International Group for the Psychology of mathematics education (pp. 1830–1838). Philadelphia, PA
Keywords: informal education, learning theory, technology, mathematical activities, feedback
Citation: Williams-Pierce C, Katırcı N, Simpson A, Shokeen E and Bih J (2024) It’s mathematics all the way down: revealing mathematical activity in non-formal learning spaces. Front. Educ. 9:1372832. doi: 10.3389/feduc.2024.1372832
Received: 18 January 2024; Accepted: 20 June 2024;
Published: 10 July 2024.
Edited by:
André Bresges, University of Cologne, GermanyReviewed by:
Sandra Nite, Texas A&M University, United StatesCopyright © 2024 Williams-Pierce, Katırcı, Simpson, Shokeen and Bih. This is an open-access article distributed under the terms of the Creative Commons Attribution License (CC BY). The use, distribution or reproduction in other forums is permitted, provided the original author(s) and the copyright owner(s) are credited and that the original publication in this journal is cited, in accordance with accepted academic practice. No use, distribution or reproduction is permitted which does not comply with these terms.
*Correspondence: Caro Williams-Pierce, Y2Fyb3dwQHVtZC5lZHU=
Disclaimer: All claims expressed in this article are solely those of the authors and do not necessarily represent those of their affiliated organizations, or those of the publisher, the editors and the reviewers. Any product that may be evaluated in this article or claim that may be made by its manufacturer is not guaranteed or endorsed by the publisher.
Research integrity at Frontiers
Learn more about the work of our research integrity team to safeguard the quality of each article we publish.