- Department of Educational Psychology, Leadership, and Higher Education, University of Nevada, Las Vegas, Las Vegas, NV, United States
Introduction: There is a growing concern about the threat of distractions in online learning environments. It has been suggested that mindfulness may attenuate the effects of distraction. The extent to which this translates to academic performance is under investigation. We aimed to investigate the relationship between task-irrelevant visual distraction, time pressure, and mindful self-regulated learning in the context of a low-stake computer-based assessment.
Methods: The study sampled 712 registered users of Prolific.co who were prescreened, current undergraduate university students. After data quality screening, 609 were retained for analyses. A 2 × 2 between-subjects design was used. Participants were randomly assigned to the following groups: (1) a control condition, (2) a distract condition, (3) a time pressure condition, or (4) a distract and time pressure condition. All participants completed reading comprehension questions, demographic questions, and the Mindful Self-Regulated Learning Scale.
Results: Presenting a visual distraction increased self-reported distraction and having a clock present increased self-reported time pressure. The distraction did not have a statistically significant effect on test performance. Mindfulness was negatively correlated with test performance, self-reported distraction, and self-reported time pressure.
Discussion: Continuous task-irrelevant visual distractions may not be distracting enough to influence low-stakes testing performance, but they do influence self-perceptions.
Introduction
Educational technologies have and continue to transform education. In 2022, 53.3% of college students enrolled in at least one online course, up from 34.7% in 2018 (National Center for Education Statistics, 2024). Online learning necessitates online assessment. Online learning as “a form of distance education where technology mediates the learning process” provides time-independent and place-independent accessibility and convenience for students of diverse backgrounds (Siemens et al., 2015, p. 100). However, what students enjoy about learning online—time flexibility and home comforts—may also increase the threat of distractions (Kostaki and Karayianni, 2022). The COVID-19 pandemic accelerated an already rising shift from face-to-face educational practices to online and hybrid modes of learning, but the rising demand for remote teaching and learning practices remains steady (Clary et al., 2022). With the widespread acceptance that online education is here to stay, research interests have shifted to the design of online assessment methods (Park and Shea, 2020). Here, we examine a commonplace assessment method in online learning environments, the computer-based assessment (CBA).
Computer-based assessment and distractions
Online assessments provide appealing benefits to educators such as automated scoring, reduced costs, immediate feedback opportunities, and adaptive capabilities (Farrell and Rushby, 2016). Early studies indicated performance differences between paper-based and computer-based testing (“test mode-effects”), which were attributed to individual learner differences such as computer familiarity and individual attitudes (Clariana and Wallace, 2002; Goldberg and Pedulla, 2002; Ricketts and Wilks, 2002). Follow up research suggests that this is because CBAs may require more cognitive effort than their paper-based counterparts (Noyes et al., 2004). Many recent investigations suggest little to no evidence of these test mode effects in both proctored and unproctored settings (Hewson et al., 2007; Escudier et al., 2011; Hewson and Charlton, 2019). However, clinical research shows that during remote online cognitive assessments, environmental distractions, such as looking away from the webcam, are associated with poorer performance (Madero et al., 2021). In addition, on timed reading comprehension tasks, people reading on screens show increased mind-wandering compared to those reading on the article (Delgado and Salmerón, 2021). It is suggested that this is because people associate screens with entertainment behaviors (i.e., reading as social media scrolling vs. reading for comprehension).
These findings intersect with cognitive load theory, which has had a profound influence on instructional practice within education (Kalyuga et al., 1999; Sweller, 1999). A key aspect of complex learning is that students need to manage materials that include a vast number of interacting elements (van Merriënboer and Sweller, 2005). The human mind is particularly sensitive to overload from irrelevant distractions (Baddeley, 1986; Paas et al., 2003). For example, media multitasking often results in poorer task performance due to unrelated distractions (Uncapher et al., 2016). In the context of test-taking, visual, auditory, and perceptual distractions have been linked to the depletion of cognitive resources and reduced test performance (Corneil and Munoz, 1996; Elliott, 2002; Trimmel and Poelzl, 2006). CBAs conducted in non-classroom environments present additional challenges related to these kinds of distractions.
Increased laptop, tablet, and mobile device use allows students to take tests virtually anywhere. This portability produces greater opportunities for task-irrelevant distractors to consume mental resources above and beyond simple home distractions such as the dog barking or the cat jumping onto your lap. Students can take a test in the parking lot outside of their workplace or in the backseat of a friend’s car on their way to go camping. Studies show that auditory distractions (e.g., background noise) impair reading comprehension (Sörqvist et al., 2010). Visual distractions occur when unrelated visuals appear in the same view as the main task, causing the eyes to move away from the primary display area (Corbett et al., 2016). This movement leads to multitasking, which increases the working memory load (SanMiguel et al., 2008). Rodrigues and Pandeirada (2019) compared cognitive performance among adolescents in high- and low-visual load environments, while Traylor et al. (2021) compared tests taken in indoor and outdoor environments across both desktop and mobile devices. In all cases, the more visually distracting environments were associated with lower test performance.
Visual distractions may be further amplified in digital environments. For example, Chen et al. (2022) compared distraction-caused eye-wandering in virtual classrooms with high- and low-visual saliency. Analyzing eye movements showed that higher distractibility in online learning, due to multitasking and visually busy layouts, leads to worse task performance, while simpler interfaces with fewer distractions help improve academic performance. Copeland and Gedeon (2015) presented English as a first language readers and English as a second language readers with a computer-based reading comprehension test alongside a fixed rapidly changing image sidebar to mimic the kind of advertisements that appear frequently on social media. They found that English as first language students had higher fixations on digital visual distraction and longer fixation times compared to English as second language students. This contributed to a compensation effect for the distraction as measured with eye tracking. In addition, devices used to take these assessments may also provide attention-attractive push notifications (e.g., Sarah Jane Smith liked your recent post) and the result is a tax on student awareness (Duke and Montag, 2017; Wilmer et al., 2017). The online environment and our effortless access to it have propelled us forward from the Age of Information to the Age of Interruption (Friedman, 2006; Jarrahi et al., 2023). Calls to examine ways to mitigate the threat of continuous partial attention in the context of online education and testing continue (Rose, 2010; Elliott et al., 2022).
Mindfulness and distractions
Mindfulness has been described as a particular enhanced form of self-regulation (Shapiro et al., 2006; Masicampo and Baumeister, 2007; Tang et al., 2015; Zhou et al., 2023). It is conscious attention that amplifies feedback through a quality of intention that is impartial. The difference between self-regulation or self-control training and mindfulness training lies in the quality of reperceiving and intention (Shapiro et al., 2006). This implies an ability to experience present-moment occurrences in the mind and environment without clinging to them. Researchers suggest that this particular kind of metacognition may allow for more sustained self-regulation and improved ability to allocate and shift attention with greater speed and cognitive efficiency, especially in the presence of negative affect (Lutz et al., 2008; Jankowski and Holas, 2014; Elkins-Brown et al., 2017). This quality of mindfulness may allow for more adaptive affective, behavioral, and sustained attentional self-control and energize these processes in the service of goals over and above self-control training alone (Elkins-Brown et al., 2017). Mindfulness researchers in education suggest that the utility of mindfulness in education lies within its ability to support enhanced self-regulation (Roeser et al., 2022). Mindful self-regulated learning has since been defined as “the adaptive and active self-monitoring of one’s thoughts, feelings, and behaviors, characterized by a quality of re-perception and acceptance, in the conscious service of the learning process” (Wolff, 2023).
Mindfulness practice is associated with decreased tactile distraction (Wang et al., 2020) and auditory and visual interference (van den Hurk et al., 2010). Other studies point to more mixed evidence. For example, in one study, self-reported trait mindfulness is negatively associated with self-reported cognitive failures, however not significantly related to a reading with distraction or operation span task (Rosenberg et al., 2013). In another study, brief mindfulness meditation did not significantly improve attention switch cost under a stressful condition (negative mood induction), yet did improve reaction times (Jankowski and Holas, 2020). This was both contrary and confirmatory to researcher hypotheses made based on attentional control theory (Eysenck et al., 2007).
In the context of education, mindfulness has been associated with higher scores on both simulated and non-simulated high-stakes testing in the college classroom and improvements on the Graduate Record Examination (GRE), required for admission to many graduate programs globally (Mrazek et al., 2013; Bellinger et al., 2015). There is widespread enthusiasm for mindfulness, as a specific enhanced attentional, emotional, and behavioral skill to protect us against the rising tax of distraction in the digital age (Berthon and Pitt, 2019; Jarrahi et al., 2023). This enthusiasm extends to online education, where supporters suggest mindfulness may reduce the attentional costs of doing schoolwork in distracting environments and promote improved performance and engagement (Palalas, 2018). Investigations are still needed to determine whether or not the intended cognitive benefits of mindfulness (see Chiesa et al., 2011 for review) translate into improved performance and reduced distraction. There is some recent evidence to suggest that mindfulness may attenuate digital distraction. Khan (2024) found that mindfulness indirectly affected exhaustion and, subsequently, academic performance during online instruction amid the COVID-19 pandemic. Another study has shown that a mindful digital attention training program enhanced student focus and reduced mind-wandering (Mrazek et al., 2022). This is particularly relevant to the current investigation if we consider Delgado and Salmerón's (2021) findings that screen reading is more susceptible to mind-wandering than paper reading.
The present study
The current study investigated whether self-reported mindful self-regulated learning moderated the effect of a task-irrelevant distraction in a computer-based assessment. The primary interest is to understand the influence of a digital distraction, our proxy being an animated.gif, and time pressure, on different groups of students’ test performance. This holds the potential to inform online test practices and future education practices surrounding digital distraction and its relationship to mindfulness. The relationship between time and accuracy is complex (e.g., Ratcliff, 1978; Wright, 2019). We hypothesize both of these may be affected by digital distractions and mindfulness, measured with the Mindful Self-regulated Learning Scale (m-SRLS), may moderate (i.e., if the interaction is substantial) the effects of distraction and time pressure. Answering these questions will allow us to better understand the relationships between distraction and performance.
Materials and methods
Participants and design
Prolific.co, an online behavioral research platform, was used to recruit study participants (N = 712). Due to the online study context relevance, the unique subject management features, and the robust pre-screening criteria of Prolific.co (see Palan and Schitter, 2018), it was considered an appropriate source of participants for this study. The quality of participant responses on Prolific.co has been studied in relation to other online sampling platforms and has shown to be both superior and satisfactory in quality (Litman et al., 2021; Peer et al., 2022; Douglas et al., 2023).
Participants electronically consented and were prescreened for the following criteria: (1) above 18 years of age, (2) US residents, (3) fluent in English, (4) currently students, (5) currently in their first 4 years of undergraduate study, and (5) had a study approval rating of 95%. A user’s study approval rating reflects the rate of satisfactory completion of other studies and is used as a data quality filter. One participant was removed for non-consent, 47 were removed for not finishing the survey, and the other 55 were removed for rapid responses, discussed further in the results, resulting in 609 participants who were retained for further analyses. Participants age ranged from 18 to 57 years, with a median age of 21 years and 71.1% aged 24 years and below. Detailed demographics are provided in Table 1.
The study used a 2×2 between-subjects design. The factors are whether there is an irrelevant distraction and whether there is a time clock counting down on the screen. The irrelevant distraction is a.gif of a door opening and closing that rotates between having a psychedelic color motion, a statue, and a hotdog. It was chosen for its oddity and irrelevance to the assessment. When presented, it is on the right side of the screen next to every question. The clock appears on the left side of the screen and is a clock that flips down each second. A screenshot of each element is provided in Figure 1. Participants were randomly assigned to one of four conditions: (1) a control condition, (2) a distract condition in which a.gif of an opening and closing door appears next to all questions, (3) a time pressure condition in which a clock counting down the remaining time they have to complete the questions is fixed to the screen, and (4) a distract/time pressure condition in which the same.gif appears in conjunction with the countdown clock.
All groups completed eight multiple-choice reading comprehension questions taken from the previous versions of the SAT and were given 12 min to complete this task. Afterward, they were asked to rate how distracted they felt they were and how much time pressure they felt on a scale from 0 to 10. In addition, participants were asked to complete the m-SRLS and some demographic questions. Within 24 h of study completion, participants were compensated US $4 to approximate a $12/h wage (approximately £10/h).
Measures
Demographic questionnaire
Participants were asked to select their age, choose their undergraduate year of study, select their biological sex at birth, gender identification, and the race/ethnicity they identified with most. In addition, participants were asked to rate how distracted they felt they were and how much time pressure they felt during the study on a scale of 0–10.
Reading comprehension questions
Reading comprehension questions were five alternative multiple-choice items taken from tests 5 and 9 in The Official SAT Study Guide (The College Board, 2009). There are two sets of four items. Each set presents the participant with two short passages that are about a common topic. Participants then answer four multiple-choice questions that require them to synthesize information from both passages. A high load on cognitive control processes degrades the ability to focus attention (Lavie, 2010). Because the items involve reading from multiple sources, readers deal with the cognitive demands of processing the texts both individually and together (Cerdan et al., 2018). Thus, the multiple-source question type seemed appropriate for eliciting a distraction effect. A full list of questions used is provided in Appendix A.
Mindful self-regulated learning scale (m-SRLS)
The m-SRLS is a newly developed scale that consists of 20 items measured on a 7-point Likert scale that ranges from 1—very untrue of me to 7—very true of me. Participants are prompted: Below are a set of statements about some general learning experiences in school. Using the 1–7 scale provided, please rate each of the following statements with the number that best describes your own opinion of what has been most true of your recent experiences in school. It is designed to measure mindfulness as an enhanced form of self-regulated learning and is conceptualized as a multidimensional construct that consists of three interrelated components: intention, attention, and attitude. The m-SRLS was chosen as a measure of mindfulness due to item context-specificity to education populations over and above other existing self-report mindfulness scales. A total score is produced by taking the average item response. The scale has demonstrated strong internal consistency and adequate validity in undergraduate student populations, including those sampled on Prolific.co (Wolff, 2023).
Data analyses
This study was pre-registered with the Open Science Foundation at: https://osf.io/k8hvf/. Deviations from the pre-registration are noted in the results. Here are the three main sets of analyses:
1. The preliminary analysis involves removing data according to the exclusion criteria (e.g., duplicate IP address) and descriptive statistics for the individual items including the self-reported measures (see Wright, 2003, for a description of good practices). This includes creating aggregate measures of accuracy from IRT.
2. ANOVAs are the main inferential procedure used for analyzing differences in accuracy, time spent, self-reported distraction, and time pressure for the 2×2 between-subjects design. We report effect sizes (ηg2) and produce 95% error bar plots for these.
3. Mindfulness was measured using the m-SRLS. We examine the correlations between these and the accuracy, time spent, self-reported distraction, and time pressure used. In addition, we explore these relationships using different experimental conditions, where analyses are exploratory, they are denoted as pexp (Spiegelhalter, 2017).
Results
Preliminary analyses
In total, 712 participants started the survey, but 49 did not complete the task, leaving 663 participants. No responses were excluded for coming from duplicate IP addresses. There were rapid response times. The pre-registration stated that we would exclude participants who responded to the test questions, on average, faster than 10 s. As there were eight questions, we excluded the 18 who finished these questions in less than 80 s. There was also evidence of rapid response to the mindfulness scale. There are 17 questions on this version of the mindfulness scale. The pre-registration did not set a threshold for treating these as being completed too rapidly, but answering on average faster than 3 s per question is unlikely to be given sufficient attention. As such, all mindfulness responses from the 26 participants who completed this part of the study more quickly than 17 × 3 s = 51 s are treated as missing.
The mindfulness scale can be divided into three components: intention, attention, and attitude. In the form used here, these are composed of seven items, four items, and six items. Standardized Cronbach’s α (Cronbach, 1951, see also Guttman, 1945) and their 95% confidence intervals using the Feldt method, found using the psych (Revelle, 2018) package, were as follows: intention (α = 0.789, 95% CI = [0.763, 0.813]), attention (α = 0.852, 95% CI = [0.832, 0.870]), and attitude (α = 0.806, 95% CI = [0.781, 0.828]). The values for the whole scale are as follows: α = 0.884, 95% CI = [0.871, 0.897]. The mean response for each of the components and the scale values were found. Therefore, each had a mean of 0 and a standard deviation of 1 so that they were weighted equally. Then, the mean of these three scaled scores was used for an overall mindfulness score. More details on this measure, its development, and alternative scoring methods, are available in Wolff (2023).
Test performance can be estimated in a number of different ways. The proportion that each group answered correctly for each item, discriminability, and item difficulty are recorded in Table 2. The correct values and difficulty correlations were r > 0.994. These are also included in Supplementary material. Total score means and standard deviations are also reported in Table 2. Questions 4 and 7 stand out as low proportions correct. Item response theory (IRT), as implemented in the package mirt (Chalmers, 2012), was used to examine response accuracy. One- and two-trait IRT models that include one-item parameter (difficulty), two-item parameters (difficulty and discriminability), and three-item parameters (difficulty, discriminability, and a guessing parameter) were estimated. The best fitting of these models in terms of the lowest AIC was the single-trait model with two parameters. Examining this model, all items had increasing probabilities of accurate responding as the person’s ability estimate increased. The latent trait from the model was calculated. Its correlation with the number correct was as follows: r = 0.985, 95% CI = (0.983, 0.987). Cronbach’s α value was 0.57. All pairwise odds ratios were above 1 with a median of 2.03. Here, the latent variable from the IRT model will be used to estimate test performance.
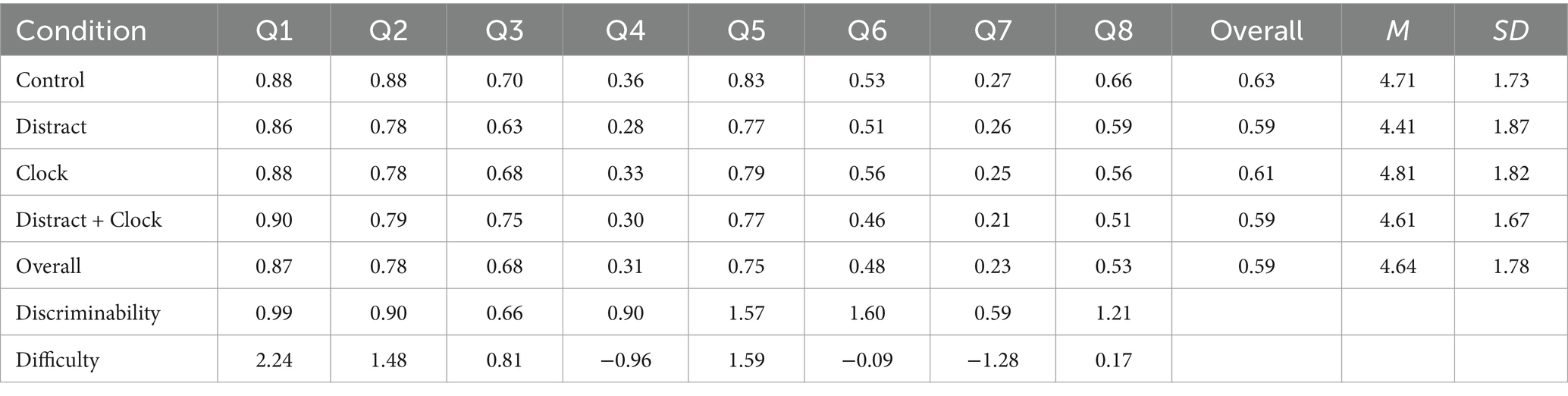
Table 2. Proportion correct for each item and total score means and standard deviations, split by experimental group. IRT parameter estimates for difficulty and discriminability are printed in the final two rows.
ANOVAs and experimental manipulations
Figure 2A shows the means for the latent variable for test performance, and their 95% confidence intervals, for the four conditions. An ANOVA was conducted using the package afex (Singmann et al., 2020), and the results are shown in the top part of Table 3. Neither the main effect nor the interaction were statistically significant at the traditional level, α = 5%, as specified in the pre-registration. The Supplementary material includes these analyses with and without transforming the response variable with the norm rank transformation (Wright, 2024). The results lead to the same conclusions, thus the analyses using the untransformed data are reported here as this is the more common approach.
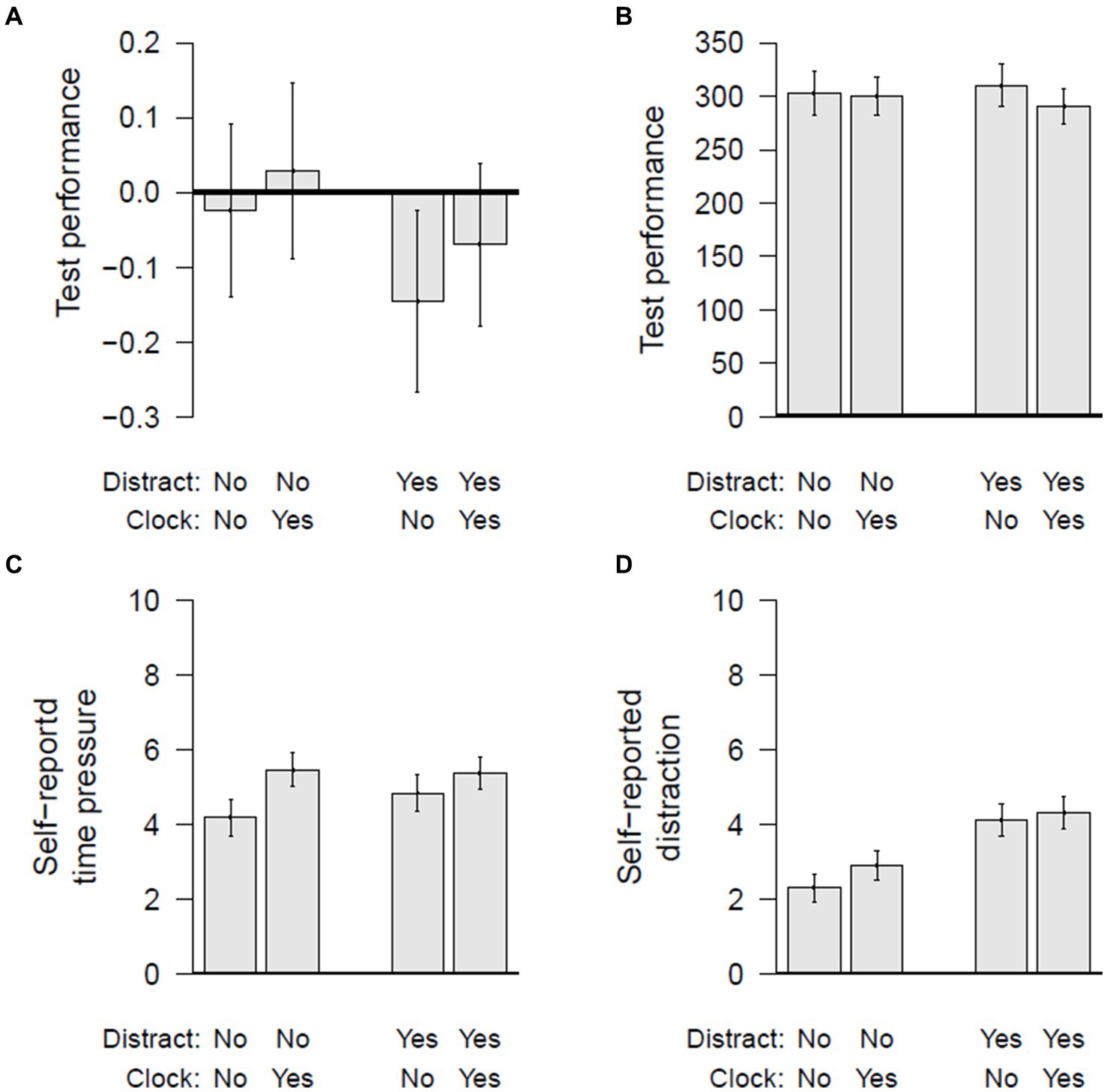
Figure 2. Means and 95% confidence intervals for the different conditions for (A) test performance (from the 2PL IRT model), (B) time taken, (C) self-reported time pressure, and (D) self-reported distraction.
Then, we examined whether the conditions influenced the self-reported time pressure and amount of distractions. These are shown in Figures 2B,C, and the statistics are reported in the second two sections of Table 3. These measures are influenced in predictable ways by the experimental manipulation. The distraction increased self-reported distraction and the clock increased self-reported time pressure. It is worth noting that the experimental manipulations have neither significant main effects nor interactions on the overall mindfulness value or any of the three components: pmin = 0.381, unadjusted for the 12 comparisons.
Relationships between self-reported mindfulness and outcome variables
Figure 2D shows the means for self-reported distraction by condition. The overall mindfulness score was negatively correlated with test performance: r = −0.114, 95% CI = (−0.191, −0.036), p = 0.004. Mindfulness was also negatively associated with self-reported distraction: r = −0.212, 95% CI = (−0.286, −0.135), p < 0.001, and time pressure: r = −0.090, 95% CI = (−0.168, −0.011), p = 0.025. The self-reported distraction and time pressure were negatively correlated with test performance, r = −0.207, 95% CI = (−0.279, −0.131) and r = −0.150, 95% CI = (−0.224, −0.073), and positively correlated to each other, r = 0.310, 95% CI = (0.239, 0.378).
The negative relationship between mindfulness and test performance is worth noting. We wanted to explore this further and any moderating relationship between mindfulness and distraction manipulation and the three outcome variables (test performance, self-reported time pressure, and self-reported distraction). To denote these as exploratory analyses and not in the pre-registration, we follow Spiegelhalter’s (2017, p. 952) advice in his presidential address to the Royal Statistical Society to denote these with pexp. For each of these, we predicted the outcome variables from mindfulness (accuracy/test performance, self-reported time pressure, and self-reported distraction), which we know from the correlations is significant. We then added the distraction manipulation, which was non-significant for test performance (pexp = 0.072) and self-reported time pressure (pexp = 0.473), and significant for self-reported distraction (pexp < 0.001). These are all as expected based on the bivariate relationships between the manipulation and each outcome variable. The interactions were then included. These were non-significant for test performance (pexp = 0.428) and distraction (pexp = 0.378). The interaction was significant for self-reported time pressure (pexp = 0.002). This relationship is shown in Figure 3. The difference is notable. The correlation when there is no distraction is as follows: rexp = −0.212, 95% CI = (−0.317, −0.103), pexp < 0.001, but when there is a distraction is as follows: rexp = 0.041, 95% CI = (−0.070, 0.151), pexp = 0.468. We remind readers this is exploratory and not part of the pre-registration.
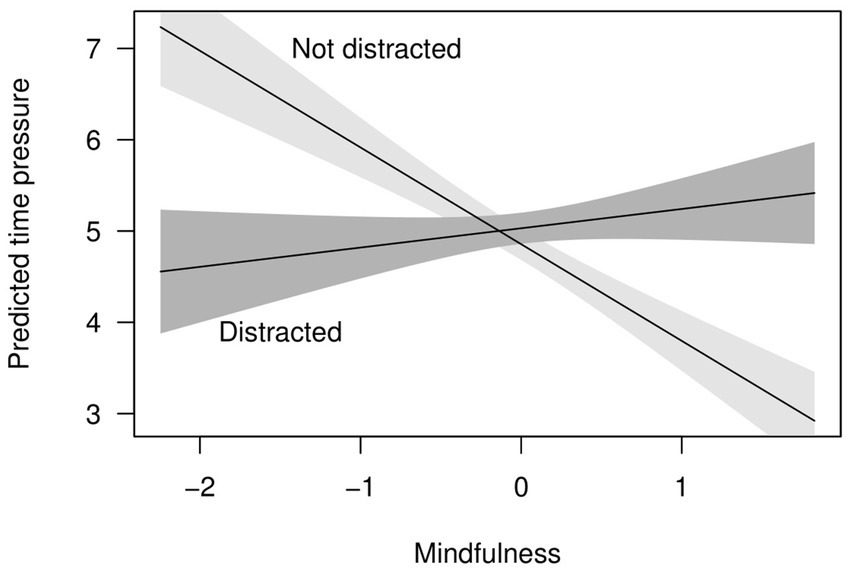
Figure 3. The predicted values of self-reported time pressure and the ± one standard error bands by whether people were distracted or not.
Discussion
This study explored the test performance of four groups: a control group, a group provided a distraction, a group provided a countdown clock, and a group provided both a distraction and a countdown clock. This experimental manipulation did not affect accuracy as much as was expected. This could be explained in a number of ways. First, this may be a reflection of the low stakes. It is known that test performance and motivation decrease in the absence of personal consequences (Wise and DeMars, 2005). In addition, students who worry more are more susceptible to examination-relevant threatening distractions, but less susceptible to examination-irrelevant threatening distractions (Keogh et al., 2004). It is also possible that the task-irrelevant distractor implemented was not distracting enough to burden cognitive resources or that online participants were already engaged in other distracting behaviors (i.e., multitasking) while taking place in this study. The more freedom of choice in test environments, the greater the potential for baseline distraction, and this may have influenced the results here (Lawrence et al., 2017). Important to the context of online learning, however, is exactly this variability in how/when/where students will engage with their online test-taking, and investigating this in a less controlled manner was intentional.
Group membership did affect self-reported distraction and time pressure in expected ways. Those who were provided the distraction felt more distracted and those who were provided the clock felt more time pressure. This suggests that even in an uncontrolled low-stakes testing environment, a continuous irrelevant visual distractor is intrusive enough to cause notable distraction and the same is true with the presence of the clock and time pressure. While this study was restricted to examining the effects of one continuous visual distraction, in real-world testing situations, there are far more complex distractions such as notifications from texts, social media, and email or the presence of others in the room, like someone’s children or roommates. These distractions may be emotionally charged, and this may intensify their effects.
We anticipated that mindfulness, measured as mindful self-regulated learning, would moderate the effects of distraction and clock presence on performance if there was a substantial interaction. Here, we found no moderating effects on test performance. This was not surprising provided that the overall experimental manipulation did not produce significant differences in accuracy to begin with.
It was surprising to note that the overall relationship between mindfulness and accuracy was negative. Hartley et al. (2022) found that regulation of cell phone use (i.e., turning off notifications while studying) was strongly correlated with self-regulated learning and distraction reduction while studying. By forcing the distraction, it is possible we removed the self-regulatory strategy of distraction avoidance from mindful people. In theory, more mindful individuals are greater tuned to the present moment and able to continually re-perceive their immediate reactions and intentions with non-judgment. It is possible that this heightened state of consciousness, while it could attenuate distraction, could also make people notice distractions more or notice intrusive thoughts more, a function of increased present-moment awareness. Here, however, mindfulness did not moderate the effects of distraction and clock presence on self-reported distraction. This suggests that more mindful participants were not more or less distracted than others despite that mindfulness was overall negatively associated with self-reported distraction. How mindful someone was did, however, alleviate time pressure in the not distracted conditions.
Time pressure compounds text anxiety for already anxious students (Raugh and Strauss, 2008). Hillgaar (2011) showed that students who scored high on self-focused attention alone vs. self-focused attention paired with accepting attitudes (mindfulness) had more test anxiety. The implication is that mindfulness may reduce test anxiety over attentional control due to the affective qualities. It is possible that the relevance of the distractor to negative affect is important in inducing or “turning on” someone’s use of mindful strategies. This has been echoed in recent investigations of the primary mechanisms by which mindfulness may act on self-regulated learning components, where affective variables emerged most relevant (Opelt and Schwinger, 2020). We suggest that mindfulness may need to be activated, just as other metacognitive prompting is used in encouraging students to monitor their off-task technology use or self-regulated learning strategies (Cheever et al., 2014).
Limitations
Our interest in computer-based online assessment and the variability of those environments led to a study design that limited the amount of control we imposed on subjects. There are inherent environmental limitations that arise because of this. Moreover, the test questions used in this study were taken from a high-stakes test. They were, therefore, difficult questions rather than a broader array of question difficulties.
The present research investigated one kind of distraction that was chosen for its irrelevance and neutrality. The idea was to understand whether a rather benign interference could still influence online test-takers. It did not influence their performance but did appear to influence their self-perceptions. Much research suggests that mindfulness enhances affective, behavioral, and attentional self-regulation (Masicampo and Baumeister, 2007; Friese et al., 2012; Zhou et al., 2023). The neutral valence of the visual distractor implemented here, paired with the low-stakes environment, may just not have been enough of a burden to induce a mindfulness effect; however, we note that the experimental manipulations also failed to influence accuracy in the expected ways. Exploring emotionally charged visual distractors in continued work is one avenue for future research.
Conclusion
The experimental conditions did not affect test performance but did affect relationships with self-report measures. The results from this study suggest that continuous irrelevant visual distractions may not be distracting enough to influence performance in low-stakes testing. While previous study suggests that irrelevant images and animations negatively impact online learning, decorative images do not (Sung and Mayer, 2012). In another study, images related covertly to the reading topic altered reading behavior enough to make easy-to-read texts as challenging as hard-to-read ones, suggesting the degree of distraction was sufficient despite participants rarely fixating on the distracting images and no significant effect on comprehension performance (Copeland and Gedeon, 2015). Despite the irrelevance and neutral valence of the distractor here, however, we also find that participants report increases in self-reported distraction and time pressure in predictable ways across conditions. In the presence of the distractor, participants were more distracted, and in the presence of the clock, they experienced more time pressure. Self-reported mindfulness did not moderate these effects but was associated with decreased self-reported distraction and decreased self-reported time pressure.
Recent research suggests mindful self-regulation of technology is beneficial in educational settings. Internet addiction is associated with higher test anxiety (Naeim et al., 2020). Students who use fewer applications during lectures are less distracted and achieve higher academic success (Limniou, 2021), while regulation of smartphone use is strongly correlated with self-regulated learning and distraction reduction while studying (Hartley et al., 2022). Those who regulate their technology usage preemptively eliminate distractions and other contributors to test anxiety. By removing the ability to “turn off” the distractor, we removed one of the mechanisms that more mindful people might use to avoid distraction.
Our findings that even an irrelevant and emotionally neutral visual distractor affected self-perceptions of distraction and time pressure in low-stakes testing lead us to endorse that efforts to limit the amount of distraction in online testing environments should be taken. This may take a number of forms. Barring the use of resource-intensive proctoring, which may not be appropriate in many circumstances, test-takers may be prompted to remove distractors from their environment prior to testing. Further research should investigate whether different kinds of distractions (e.g., relevant vs. irrelevant, to both examination and person) heighten the effect or non-effect of mindfulness in these conditions. Our results suggest that mindfulness was negatively associated with performance despite its association with distraction and time pressure in predicted ways. This is contrary to the limited literature surrounding the mindfulness–achievement link and suggests that this relationship is more nuanced than is purported (Howell and Buro, 2011; Chiang and Sumell, 2019).
Future research may first look to explore whether the associations observed here exist in other test settings. Determining whether different kinds of distractions (e.g., relevant versus irrelevant, emotionally charged versus not emotionally charged, both to exam and to person) is important for assessing the true role of mindfulness in moderating these threats. Investigations may also include inducing mindful states preceding distracting test environments. An important implication of the current research is that mindfulness may not be a blanket solution to all kinds of awareness interference. We note that more mindful individuals, while potentially better at attenuating distraction, might also notice distractions or intrusive thoughts more due to their heightened state of present-moment awareness. Exploring whether this heightened awareness is an intrinsic characteristic could provide further insights.
Data availability statement
The datasets presented in this study can be found in online repositories. The names of the repository/repositories and accession number(s) can be found at: Open Science Foundation, doi: 10.17605/osf.io/k8hvf, available at: https://osf.io/k8hvf.
Ethics statement
The studies involving humans were approved by University of Nevada Las Vegas Social/Behavioral IRB. The studies were conducted in accordance with the local legislation and institutional requirements. The participants provided their written informed consent to participate in this study.
Author contributions
SW: Conceptualization, Data curation, Formal analysis, Investigation, Methodology, Project administration, Resources, Software, Supervision, Validation, Visualization, Writing – original draft, Writing – review & editing. DW: Formal analysis, Funding acquisition, Methodology, Supervision, Writing – original draft, Writing – review & editing. WH: Writing – original draft, Writing – review & editing.
Funding
The author(s) declare that no financial support was received for the research, authorship, and/or publication of this article.
Conflict of interest
The authors declare that the research was conducted in the absence of any commercial or financial relationships that could be construed as a potential conflict of interest.
Publisher’s note
All claims expressed in this article are solely those of the authors and do not necessarily represent those of their affiliated organizations, or those of the publisher, the editors and the reviewers. Any product that may be evaluated in this article, or claim that may be made by its manufacturer, is not guaranteed or endorsed by the publisher.
Supplementary material
The Supplementary material for this article can be found online at: https://www.frontiersin.org/articles/10.3389/feduc.2024.1360848/full#supplementary-material
References
Bellinger, D. B., DeCaro, M. S., and Ralston, P. A. (2015). Mindfulness, anxiety, and high-stakes mathematics performance in the laboratory and classroom. Conscious. Cogn. 37, 123–132. doi: 10.1016/j.concog.2015.09.001
Berthon, P. R., and Pitt, L. F. (2019). Types of mindfulness in an age of digital distraction. Bus. Horiz. 62, 131–137. doi: 10.1016/j.bushor.2018.10.003
Cerdan, R., Candel, C., and Leppink, J. (2018). Cognitive load and learning in the study of multiple documents. Front Educ 3:59. doi: 10.3389/feduc.2018.00059
Chalmers, R. P. (2012). Mirt: A multidimensional item response theory package for the R environment. J. Stat. Softw. 48, 1–29. doi: 10.18637/jss.v048.i06
Cheever, N. A., Rosen, L. D., Carrier, L. M., and Chavez, A. (2014). Out of sight is not out of mind: the impact of restricting wireless mobile device use on anxiety levels among low, moderate and high users. Comput. Hum. Behav. 37, 290–297. doi: 10.1016/j.chb.2014.05.002
Chen, S., Wu, X., and Li, Y. (2022). Exploring the Relationships between Distractibility and Website Layout of Virtual Classroom Design with Visual Saliency. International Journal of Human-Computer Interaction, 38, 1291–1306. doi: 10.1080/10447318.2021.1994212
Chiang, E. P., and Sumell, A. J. (2019). Are your students absent, not absent, or present? Mindfulness and student performance. J. Econ. Educ. 50, 1–16. doi: 10.1080/00220485.2018.1551096
Chiesa, A., Calati, R., and Serretti, A. (2011). Does mindfulness training improve cognitive abilities? A systematic review of neuropsychological findings. Clin. Psychol. Rev. 31, 449–464. doi: 10.1016/j.cpr.2010.11.003
Clariana, R., and Wallace, P. (2002). Paper-based versus computer-based assessment: key factors associated with the test mode effect. Br. J. Educ. Technol. 33, 593–602. doi: 10.1111/1467-8535.00294
Clary, G., Dick, G., Akbulut, A. Y., and Van Slyke, C. (2022). The after times: college students’ desire to continue with distance learning post pandemic. Commun. Assoc. Inf. Syst. 50, 122–142. doi: 10.17705/1CAIS.05003
Copeland, L., and Gedeon, T. (2015). Visual distractions effects on reading in digital environments: A comparison of first and second English language readers. In: Proceedings of the Annual Meeting of the Australian Special Interest Group for Computer Human Interaction, 506–516.
Corbett, B., Nam, C. S., and Yamaguchi, T. (2016). The effects of haptic feedback and visual distraction on pointing task performance. Int J Hum Comput Interact 32, 89–102. doi: 10.1080/10447318.2015.1094914
Corneil, B. D., and Munoz, D. P. (1996). The influence of auditory and visual distractors on human orienting gaze shifts. J. Neurosci. 16, 8193–8207. doi: 10.1523/JNEUROSCI.16-24-08193.1996
Cronbach, L. J. (1951). Coefficient alpha and the internal structure of tests. Psychometrika 16, 297–334. doi: 10.1007/BF02310555
Delgado, P., and Salmerón, L. (2021). The inattentive on-screen Reading: Reading medium affects attention and reading comprehension under time pressure. Learn. Instr. 71:101396. doi: 10.1016/j.learninstruc.2020.101396
Douglas, B. D., Ewell, P. J., and Brauer, M. (2023). Data quality in online human-subjects research: comparisons between MTurk, prolific, CloudResearch, Qualtrics, and SONA. PLoS One 18:e0279720. doi: 10.1371/journal.pone.0279720
Duke, É., and Montag, C. (2017). Smartphone addiction, daily interruptions and self-reported productivity. Addict. Behav. Rep. 6, 90–95. doi: 10.1016/j.abrep.2017.07.002
Elkins-Brown, N., Teper, R., and Inzlicht, M. (2017). “How mindfulness enhances self-control” in Mindfulness in social psychology. eds. J. C. Karremans and E. K. Papies (London, UK: Routledge/Taylor & Francis Group), 65–78.
Elliott, E. M. (2002). The irrelevant-speech effect and children: theoretical implications of developmental change. Mem. Cognit. 30, 478–487. doi: 10.3758/bf03194948
Elliott, E. M., Bell, R., Gorin, S., Robinson, N., and Marsh, J. E. (2022). Auditory distraction can be studied online! A direct comparison between in-person and online experimentation. J. Cogn. Psychol. 34, 307–324. doi: 10.1080/20445911.2021.2021924
Escudier, M. P., Newton, T. J., Cox, M. J., Reynolds, P. A., and Odell, E. W. (2011). University students' attainment and perceptions of computer delivered assessment: A comparison between computer-based and traditional tests in a “high-stakes” examination. J. Comput. Assist. Learn. 27, 440–447. doi: 10.1111/j.1365-2729.2011.00409.x
Eysenck, M. W., Derakshan, N., Santos, R., and Calvo, M. G. (2007). Anxiety and cognitive performance: attentional control theory. Emotion 7, 336–353. doi: 10.1037/1528-3542.7.2.336
Farrell, T., and Rushby, N. (2016). Assessment and learning technologies: an overview. Br. J. Educ. Technol. 47, 106–120. doi: 10.1111/bjet.12348
Friedman, L. (2006). The age of interruption. The New York Times. Available online at: https://www.nytimes.com/2006/07/05/opinion/05friedman.html(Accessed July, 2024).
Friese, M., Messner, C., and Schaffner, Y. (2012). Mindfulness meditation counteracts self-control depletion. Conscious. Cogn. 21, 1016–1022. doi: 10.1016/j.concog.2012.01.008
Goldberg, A. L., and Pedulla, J. J. (2002). Performance differences according to test mode and computer familiarity on a practice graduate record exam. Educ. Psychol. Meas. 62, 1053–1067. doi: 10.1177/0013164402238092
Guttman, L. (1945). A basis for analyzing test-retest reliability. Psychometrika 10, 255–282. doi: 10.1007/BF02288892
Hartley, K., Bendixen, L. D., Shreve, E., and Gianoutsos, D. (2022). Smartphone usage and studying: investigating relationships between type of use and self-regulatory skills. Multimodal Technol Interact 6:44. doi: 10.3390/mti6060044
Hewson, C., and Charlton, J. P. (2019). An investigation of the validity of course-based online assessment methods: the role of computer-related attitudes and assessment mode preferences. J. Comput. Assist. Learn. 35, 51–60. doi: 10.1111/jcal.12310
Hewson, C., Charlton, J., and Brosnan, M. (2007). Comparing online and offline administration of multiple choice question assessments to psychology undergraduates: do assessment modality or computer attitudes influence performance? Psychol. Learn. Teach. 6, 37–46. doi: 10.2304/plat.2007.6.1.37
Hillgaar, S. D. (2011). Mindfulness and self-regulated learning. [Master’s thesis: the University of Science and Technology]. Norges teknisk-naturvitenskapelige universitet, Fakultet for samfunnsvitenskap og teknologiledelse, Psykologisk institutt.
Howell, A. J., and Buro, K. (2011). Relations among mindfulness, achievement-related self-regulation, and achievement emotions. J Happiness Stud 12, 1007–1022. doi: 10.1007/s10902-010-9241-7
Jankowski, T., and Holas, P. (2014). Metacognitive model of mindfulness. Conscious. Cogn. 28, 64–80. doi: 10.1016/j.concog.2014.06.005
Jankowski, T., and Holas, P. (2020). Effects of brief mindfulness meditation on attention switching. Mindfulness 11, 1150–1158. doi: 10.1007/s12671-020-01314-9
Jarrahi, M. H., Blyth, D. L., and Goray, C. (2023). Mindful work and mindful technology: redressing digital distraction in knowledge work. Digit Bus 3:100051. doi: 10.1016/j.digbus.2022.100051
Kalyuga, S., Chandler, P., and Sweller, J. (1999). Managing split-attention and redundancy in multimedia instruction. Appl. Cogn. Psychol. 13, 351–371. doi: 10.1002/(SICI)1099-0720(199908)13:4<351::AID-ACP589>3.0.CO;2-6
Keogh, E., Bond, F. W., French, C. C., Richards, A., and Davis, R. E. (2004). Test anxiety, susceptibility to distraction and examination performance. Anxiety Stress Coping 17, 241–252. doi: 10.1080/10615300410001703472
Khan, A. N. (2024). Students are at risk? Elucidating the impact of health risks of COVID-19 on emotional exhaustion and academic performance: role of mindfulness and online interaction quality. Curr. Psychol. 43, 12285–12298. doi: 10.1007/s12144-023-04355-0
Kostaki, D., and Karayianni, I. (2022). Houston, we have a pandemic: technical difficulties, distractions and online student engagement. Student Engage Higher Educ J 4, 105–127. doi: 10.31219/osf.io/6mrhc
Lavie, N. (2010). Attention, distraction, and cognitive control under load. Curr. Dir. Psychol. Sci. 19, 143–148. doi: 10.1177/0963721410370295
Lawrence, A., Kinney, T., O'Connell, M., and Delgado, K. (2017). Stop interrupting me! Examining the relationship between interruptions, test performance and reactions. Personnel Assess Decis 3:2. doi: 10.25035/pad.2017.002
Limniou, M. (2021). The effect of digital device usage on student academic performance: A case study. Educ. Sci. 11:121. doi: 10.3390/educsci11030121
Litman, L., Moss, A., Rosenzweig, C., and Robinson, J. (2021). Reply to MTurk, prolific or panels? Choosing Right Audience Online Res. doi: 10.2139/ssrn.3775075
Lutz, A., Slagter, H. A., Dunne, J. D., and Davidson, R. J. (2008). Attention regulation and monitoring in meditation. Trends Cogn. Sci. 12, 163–169. doi: 10.1016/j.tics.2008.01.005
Madero, E. N., Anderson, J., Bott, N. T., Hall, A., Newton, D., Fuseya, N., et al. (2021). Environmental distractions during unsupervised remote digital cognitive assessment. J. Prev Alzheimers Dis. 8, 263–266. doi: 10.14283/jpad.2021.9
Masicampo, E. J., and Baumeister, R. F. (2007). Relating mindfulness and self-regulatory processes. Psychol. Inq. 18, 255–258. doi: 10.1080/10478400701598363
Mrazek, M. D., Franklin, M. S., Phillips, D. T., Baird, B., and Schooler, J. W. (2013). Mindfulness training improves working memory capacity and GRE performance while reducing mind wandering. Psychol. Sci. 24, 776–781. doi: 10.1177/0956797612459659
Mrazek, A. J., Mrazek, M. D., Brown, C. S., Karimi, S. S., Ji, R. R., Ortega, J. R., et al. (2022). Attention training improves the self-reported focus and emotional regulation of high school students. Technol Mind Behav 3:92. doi: 10.1037/tmb0000092
Naeim, M., Rezaeisharif, A., and Zandian, H. (2020). The relationship between internet addiction and social adjustment, and test anxiety of the students of Ardabil University of Medical Sciences. Shiraz E-Med J 21:209. doi: 10.5812/semj.99209
National Center for Education Statistics. (2024). Enrollment and degrees conferred trends. IPEDS trend generator. U.S. Department of Education, Institute of Education Sciences. Available online at: https://nces.ed.gov/ipeds/TrendGenerator/app/answer/2/42 (Accessed July, 2024).
Noyes, J. M., Garland, K. J., and Robbins, E. L. (2004). Paper-based versus computer-based assessment: is workload another test mode effect? Br. J. Educ. Technol. 35, 111–113. doi: 10.1111/j.1467-8535.2004.00373.x
Opelt, F., and Schwinger, M. (2020). Relationships between narrow personality traits and self- regulated learning strategies: Exploring the role of mindfulness, contingent self-esteem, and self-control. AERA Open, 6, 11–5. doi: 10.1177/2332858420949499
Paas, F., Renkl, A., and Sweller, J. (2003). Cognitive load theory and instructional design: recent developments. Educ. Psychol. 38, 1–4. doi: 10.1207/S15326985EP3801_1
Palalas, A. (2018). “Mindfulness in mobile and ubiquitous learning: harnessing the power of attention” in Mobile and ubiquitous learning. Perspectives on rethinking and reforming education. eds. S. Yu, M. Ally, and A. Tsinakos (Singapore: Springer).
Palan, S., and Schitter, C. (2018). Prolific.Ac—A subject pool for online experiments. J. Behav. Exp. Financ. 17, 22–27. doi: 10.1016/j.jbef.2017.12.004
Park, H., and Shea, P. (2020). A ten-year review of online learning research through co-citation analysis. Online Learn 24, 225–244. doi: 10.24059/olj.v24i2.2001
Peer, E., Rothschild, D., Gordon, A., Evernden, Z., and Damer, E. (2022). Data quality of platforms and panels for online behavioral research. Behav. Res. Methods 54, 1643–1662. doi: 10.3758/s13428-021-01694-3
Ratcliff, R. (1978). Theory of memory retrieval. Psychol. Rev. 85, 59–108. doi: 10.1037/0033-295X.85.2.59
Raugh, I. M., and Strauss, G. P. (2024). Integrating mindfulness into the extended process model of emotion regulation: The dual-mode model of mindful emotion regulation. Emotion, 24, 847–866. doi: 10.1037/emo0001308
Revelle, W. (2018). Psych: Procedures for psychological, psychometric, and personality research [computer software manual]. Evanston, Illinois. Available online at: https://CRAN.R-project.org/package=psych (R package version 1.8.10) (Accessed July, 2024).
Ricketts, C., and Wilks, S. J. (2002). Improving student performance through computer-based assessment: insights from recent research. Assess. Eval. High. Educ. 27, 475–479. doi: 10.1080/0260293022000009348
Rodrigues, P. F. S., and Pandeirada, J. N. S. (2019). The influence of a visually-rich surrounding environment in visuospatial cognitive performance: A study with adolescents. J. Cogn. Dev. 20, 399–410. doi: 10.1080/15248372.2019.1605996
Roeser, R. W., Greenberg, M. T., Frazier, T., Galla, B. M., Semenov, A. D., and Warren, M. T. (2022). Beyond all splits: envisioning the next generation of science on mindfulness and compassion in schools for students. Mindfulness 14, 239–254. doi: 10.1007/s12671-022-02017-z
Rose, E. (2010). Continuous partial attention: reconsidering the role of online learning in the age of interruption. Educ. Technol. 50, 41–46.
Rosenberg, M., Noonan, S., DeGutis, J., and Esterman, M. (2013). Sustaining visual attention in the face of distraction: a novel gradual-onset continuous performance task. Atten. Percept. Psychophys. 75, 426–439. doi: 10.3758/s13414-012-0413-x
SanMiguel, I., Corral, M. J., and Escera, C. (2008). When loading working memory reduces distraction: behavioral and electrophysiological evidence from an auditory-visual distraction paradigm. J. Cogn. Neurosci. 20, 1131–1145. doi: 10.1162/jocn.2008.20078
Shapiro, S. L., Carlson, L. E., Astin, J. A., and Freedman, B. (2006). Mechanisms of mindfulness. J. Clin. Psychol. 62, 373–386. doi: 10.1002/jclp.20237
Siemens, G., Gašević, D., and Dawson, S. (2015). Preparing for the digital university: A review of the history and current state of distance, blended, and online learning. Alberta, Canada: Athbasca University.
Singmann, H., Bolker, B., Westfall, J., and Aust, F. (2020). afex: Analysis of factorial experiments [Computer software manual]. Available online at: https://CRAN.R-project.org/package=afex (R package version 0.27–2) (Accessed July, 2024).
Sörqvist, P., Halin, N., and Hygge, S. (2010). Individual differences in susceptibility to the effects of speech on reading comprehension. Appl. Cogn. Psychol. 24, 67–76. doi: 10.1002/acp.1543
Spiegelhalter, D. J. (2017). Trust in numbers. J. R. Stat. Soc. Ser. A 180, 948–965. doi: 10.1111/rssa.12302
Sung, E., and Mayer, R. E. (2012). When graphics improve liking but not learning from online lessons. Comput. Hum. Behav. 28, 1618–1625. doi: 10.1016/j.chb.2012.03.026
Tang, Y. Y., Hölzel, B. K., and Posner, M. I. (2015). The neuroscience of mindfulness meditation. Nat. Rev. Neurosci. 16, 213–225. doi: 10.1038/nrn3916
Traylor, Z., Hagen, E., Williams, A., and Arthur, W. (2021). The testing environment as an explanation for unproctored internet-based testing device-type effects. Int. J. Sel. Assess. 29, 65–80. doi: 10.1111/ijsa.12315
Trimmel, M., and Poelzl, G. (2006). Impact of background noise on reaction time and brain DC potential changes of VDT-based spatial attention. Ergonomics 49, 202–208. doi: 10.1080/00140130500434986
Uncapher, M. R., Thieu, K., and Wagner, A. D. (2016). Media multitasking and memory: differences in working memory and long-term memory. Psychon. Bull. Rev. 23, 483–490. doi: 10.3758/s13423-015-0907-3
van den Hurk, P. A. M., Giommi, F., Gielen, S. C., Speckens, A. E. M., and Barendregt, H. P. (2010). Greater efficiency in attentional processing related to mindfulness meditation. Q. J. Exp. Psychol. 63, 1168–1180. doi: 10.1080/17470210903249365
van Merriënboer, J. J. G., and Sweller, J. (2005). Cognitive load theory and complex learning: recent developments and future directions. Educ. Psychol. Rev. 17, 147–177. doi: 10.1007/s10648-005-3951-0
Wang, M. Y., Freedman, G., Raj, K., Fitzgibbon, B. M., Sullivan, C., Tan, W. L., et al. (2020). Mindfulness meditation alters neural activity underpinning working memory during tactile distraction. Cogn. Affect. Behav. Neurosci. 20, 1216–1233. doi: 10.3758/s13415-020-00828-y
Wilmer, H. H., Sherman, L. E., and Chein, J. M. (2017). Smartphones and cognition: A review of research exploring the links between mobile technology habits and cognitive functioning. Front. Psychol. 8:605. doi: 10.3389/fpsyg.2017.00605
Wise, S. L., and DeMars, C. E. (2005). Low examinee effort in low-stakes assessment: problems and potential solutions. Educ. Assess. 10, 1–17. doi: 10.1207/s15326977ea1001_1
Wolff, S. M. (2023). Development and initial validation of the mindful self-regulated learning scale (m-SRLS) (publication no. 30422987) [doctoral dissertation, University of Nevada las Vegas]. Las Vegas, NV: ProQuest Dissertations Publishing.
Wright, D. B. (2003). Making friends with your data: improving how statistics are conducted and reported. Br. J. Educ. Psychol. 73, 123–136. doi: 10.1348/000709903762869950
Wright, D. B. (2019). Speed gaps: exploring differences in response latencies among groups. Educ. Meas. Issues Pract. 38, 87–98. doi: 10.1111/emip.12286
Wright, D. B. (2024). Normrank correlations for testing associations and for use in latent variable models. Open Educ Stud 6, 1–18. doi: 10.1515/edu-2024-0003
Keywords: computer-based assessment, mindful self-regulated learning, task-irrelevant distraction, online learning, digital distraction
Citation: Wolff SM, Wright DB and Hatcher WJ (2024) Task-irrelevant visual distractions and mindful self-regulated learning in a low-stakes computer-based assessment. Front. Educ. 9:1360848. doi: 10.3389/feduc.2024.1360848
Edited by:
Shirley Miedijensky, Oranim Academic College, IsraelReviewed by:
Pengcheng Wang, Singapore Institute of Technology, SingaporeYun Tang, Central China Normal University, China
Copyright © 2024 Wolff, Wright and Hatcher. This is an open-access article distributed under the terms of the Creative Commons Attribution License (CC BY). The use, distribution or reproduction in other forums is permitted, provided the original author(s) and the copyright owner(s) are credited and that the original publication in this journal is cited, in accordance with accepted academic practice. No use, distribution or reproduction is permitted which does not comply with these terms.
*Correspondence: Sarah M. Wolff, c2FyYWgud29sZmZAdW5sdi5lZHU=