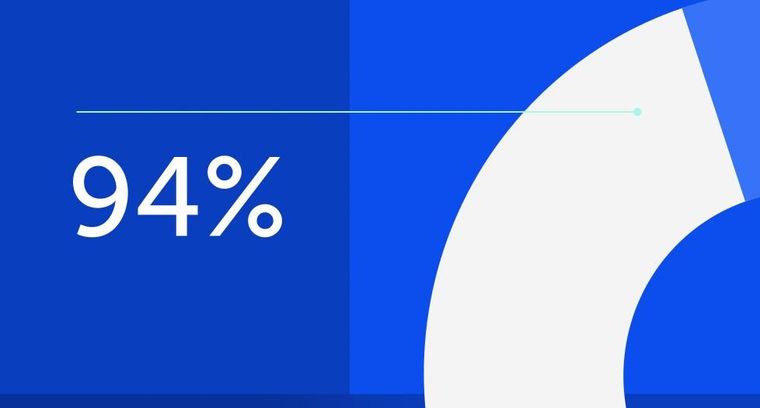
94% of researchers rate our articles as excellent or good
Learn more about the work of our research integrity team to safeguard the quality of each article we publish.
Find out more
ORIGINAL RESEARCH article
Front. Educ., 15 March 2024
Sec. STEM Education
Volume 9 - 2024 | https://doi.org/10.3389/feduc.2024.1356626
Introduction: Models are a primary mode of science communication and preparing university students to evaluate models will allow students to better construct models and predict phenomena. Model evaluation relies on students’ subject-specific knowledge, perception of model characteristics, and confidence in their knowledge structures.
Methods: Fifty first-year college biology students evaluated models of concepts from varying biology subject areas with and without intentionally introduced errors. Students responded with ‘error’ or ‘no error’ and ‘confident’ or ‘not confident’ in their response.
Results: Overall, students accurately evaluated 65% of models and were confident in 67% of their responses. Students were more likely to respond accurately when models were drawn or schematic (as opposed to a box-and-arrow format), when models had no intentional errors, and when they expressed confidence. Subject area did not affect the accuracy of responses.
Discussion: Variation in response patterns to specific models reflects variation in model evaluation abilities and suggests ways that pedagogy can support student metacognitive monitoring during model-based reasoning. Error detection is a necessary step towards modeling competence that will facilitate student evaluation of scientific models and support their transition from novice to expert scientists.
Models are fundamental to all forms of science (Lehrer and Schauble, 2000; Gilbert, 2004; Papaevripidou and Zacharia, 2015) and allow scientists to describe, understand, and ultimately predict phenomena (Odenbaugh, 2005; Gouvea and Passmore, 2017; Seel, 2017). Experts in science learn their discipline through these models – from testing and revising, determining missing components and relationships, and by generalizing across models (Windschitl et al., 2008; Magnani et al., 2012). We separate the process of modeling from model objects by referring to the process of building, evaluating, using, and revising models as modeling, and the object being constructed, evaluated, or revised as the model (Krell et al., 2013). As scientists often communicate with models, being able to identify incongruencies between one’s knowledge and observed models is a critical component of one’s progression as an expert in the discipline. Understanding the factors that facilitate students’ efficient error detection may offer valuable insights into how to guide students in this progression.
Modeling ability is predicated on prior knowledge because modeling is always done in a context and for a purpose (Nielsen and Nielsen, 2021). Prior knowledge, when organized in an explanatory model of the phenomena in working memory (Oh, 2019), is the comparator to observed phenomena. During model sense-making, students evaluate the strengths and weaknesses of their explanatory model (aka, mental model) and whether to revise their explanatory model (Schwarz et al., 2009). While most, if not all, modeling frameworks include the element of model evaluation and revision (Löhner et al., 2005; Upmeier zu Belzen et al., 2019), there are sparse details about the cognitive processes inherent to model evaluation.
Biology knowledge provides the foundation for class performance and plays an important role in how students do model-based reasoning and modeling. According to the passive activation principle, knowledge is activated regardless of its importance to comprehension (Myers and O’Brien, 1998), and the overabundance of knowledge must be evaluated. While retrieving this prior knowledge increases the opportunity to develop an explanatory model of phenomena, it simultaneously requires greater effort to discern the most relevant and scientifically sound knowledge.
Students’ knowledge contains incorrect or incomplete understanding, i.e., misconceptions. When one stores scientifically incorrect knowledge (e.g., the inaccuracy that CO2 is absorbed by plants but not respired), these misconceptions are indefinitely encoded in memory (Kendeou et al., 2019). Experts, by definition, possess exceptional knowledge and the skills to evaluate it (Allaire-Duquette et al., 2021). The ability to evaluate one’s knowledge for scientific inaccuracies is one of the ways expert scientists and novices diverge and is the object of this study. Model evaluation is a critical element of modeling, but little is known about how students use their conceptual knowledge to judge and evaluate models.
Studies have shown repeatedly that the ability to detect conflict and inhibit intuitive scientific misconceptions correlates both with more effective reasoning and with scientific expertise (Pennycook et al., 2012; Brookman-Byrne et al., 2018). Relative to student novices, experts are drawing on well-established, scientifically-sound knowledge that supports rapid error identification. Students have had fewer opportunities to evaluate scientifically accurate, robust, and inter-connected knowledge and therefore have less-developed error detection abilities compared to experts.
In science classrooms, students frequently must compare their conceptual knowledge to canonical knowledge presented in the form of a model. The presented knowledge is most often shown as a scientifically-sound explanatory model for a phenomenon. Zhang and Fiorella (2023) propose a theoretical model for how students learn as they generate errors during retrieval of prior knowledge then detect errors when comparing their mental model with the reference information. Specifically, these authors propose that students learn from errors when those errors are semantically related to the target content and prompt self-feedback and evaluation of current knowledge. Conversely, students do not learn from errors when the errors are semantically unrelated to the target content or when students are unmotivated to reflect on their mental models. Our work focuses on the specific process of error detection that occurs during the comparison of one’s mental model to the reference information. In terms of model evaluation, we consider the reference information to be the presented, scientifically sound model of phenomena and the mental model to be the product of concepts elicited by the task, and which resides in long-term or working memory. Consistent with Zhang and Fiorella, model attributes such as the format of the model (e.g., pictures or schematic models) may act as cues for prior knowledge and alter the likelihood of students’ error detection, as well as inspiring different levels of self-monitoring and reflection. Therefore, we focus on model attributes as a potentially potent factor that may affect students’ error detection. Moreover, we expand upon the Zhang and Fiorella model by examining students’ confidence in their responses as a function of accuracy and model attributes.
We predict that students’ abilities to detect errors will be mediated by their confidence in their knowledge of the concept, and that the alignment between student confidence and accuracy in error detection approximates their level of self-monitoring. A learner adept at self-monitoring is more likely to systematically determine when their knowledge is scientifically sound and when there are errors, and therefore may be “primed” for disequilibrium and associated conceptual change (D’Mello et al., 2014). Conversely, students who do not perform self-monitoring, or have low knowledge or motivation, only do surface level reasoning (Zhang and Fiorella, 2023) and therefore miss the first step in generating the productive confusion that serves as an entry point for conceptual change (VanLehn et al., 2003; D’Mello et al., 2014). Students may also display low self-monitoring that results in over- or under-confidence in their own knowledge of the topic. For example, the “Dunning-Kruger effect” describes the phenomenon where people are overconfident in their lower quality performance (Kruger and Dunning, 1999). Therefore, confidence acts as a critical mediator in the process of error checking and model evaluation.
Different forms of alignment between model accuracy and students’ evaluation of models offer clues as to the nature of students’ knowledge (Table 1). When knowledge aligns with the presented model, students will likely respond accurately. Misalignment can occur when students are presented with an explanatory model and perceive an error where none existed, or when the student fails to notice an intentionally-introduced error. In both misalignments, it is possible the students’ conceptual understanding is incomplete or incorrect. Again, students’ confidence responses can indicate the level of self-monitoring as students assess their knowledge of the concept.
Considering student confidence leads to a far more complicated picture of students’ self-monitoring abilities (Table 1). Confidence and error detection interact in ways that suggest significant variation in how students perceive the observed models (Dinsmore and Parkinson, 2013). A student who is an “ideal metacognitive observer” of their performance (has high self-monitoring; Table 1) will show high correspondence between their performance and their confidence. That is, they will know what they know and know what they do not know (Fleming and Lau, 2014). Conversely, a mismatch between student confidence and accuracy reflects low self-monitoring either in the form of over- or under- confidence. Whether students display variation in self-monitoring as a function of model attributes, including the conceptual content or the format of the model, may yield insights into which types of models elicit self-monitoring and offer guideposts for instructors on teaching metacognitive skills. For instance, if students routinely are over-confident when evaluating models presented as pictures, this may provide an entry point for prompting further reflection or presenting content in an alternative format.
In our study, students evaluated explanatory models of biology phenomena with different model attributes like whether they had intentionally-introduced errors, the subject area, or the format. Some of the presented models were scientifically sound and some contained scientifically incorrect information that rendered the model empirically inaccurate. We acknowledge all models are incomplete and there is more than one explanatory model to represent phenomena while also noting that phenomena have core conceptual ideas that must be shared by these “correct” models. For example, to represent relatedness in a phylogenetic tree, nodes and branch tips have scientifically accepted interpretations even though an individual could conceive of a novel format and create an alternative “correct” model depicting the same information. In this study, we aimed to capture the core conceptual components and relationships inherent to phenomena rather than to discern or compare alternative representations.
Representation format may impact how a student perceives a model, especially if presented in a modality that contrasts with their prior knowledge format. In past research, we have adapted the Goel and Stroulia (1996) Structure-Behavior-Function (S-B-F) framework when constructing biology models, where structures of a system are in boxes (nodes) and the behaviors/relationships among them are described on connecting arrows (links, edges), to illustrate how the system produces a function (Dauer et al., 2013; Long et al., 2014; Clark et al., 2020). The symbolic nature of SBF models places attention on the text within the boxes and on the labeled arrows (Figure 1).
Figure 1. Examples of biology conceptual models used in the error detection task in SBF (boxes and arrows) format and schematic (drawn) format. Shown models are one of three versions for each concept. Model 405 contains an intentionally-introduced error. Silhouette images from https://www.phylopic.org/.
Some concepts were difficult to represent in this SBF format and biology norms often represent some concepts in drawn format, what we term schematic (Figure 1; Table 2). Schematic model objects often contained variation in components (e.g., bacterial cells shown as circles with and without fill patterns to illustrate phenotypic variation in traits, such as antibiotic resistance) that change over time. All the model objects in this study were in formats that undergraduate students would regularly have encountered during the course.
Detecting and correcting errors in one’s own mental models requires comparing and evaluating one’s own mental models with diverse scientific explanatory models (Zhang and Fiorella, 2023) coupled with one’s self-awareness, through confidence, of their knowledge of the concept presented in the model. This study sought to describe the variation in students’ abilities to detect errors in presented models, describe the variation in self-monitoring during model evaluation, and identify the model attributes that contribute to the variation. We ask two research questions: (a) how do model attributes (intentionally-introduced errors, subject area, format) impact students’ accuracy and confidence when detecting errors in explanatory models; (b) how do individuals vary in their abilities to detect intended errors in explanatory models?
Students were recruited for this neuroimaging study from the second in a two-part introductory biology course at a large, doctoral-granting institution in higher education in the United States. The course content included, in order of instruction, evolution, phylogeny/diversity, physiology, and ecology. Students were recruited from two sections of the course led by two instructors: the lead author (Instructor 1) and another instructor not involved in the study (Instructor 2). Both instructors have taught the course for more than 9 years and use models regularly during instruction and assessment. Students in the class of Instructor 1 also constructed and evaluated their own and each other’s models. The specific models used in this study were never seen or used in the course, although the concepts were a focus of instruction.
Students were recruited from four sections of the course in Spring 2021 and Spring 2022 terms. Instructor 1 taught two sections in Spring 2021 and one section in Spring 2022; Instructor 2 taught one section in Spring 2022. Spring 2021 students (only Instructor 1) were, as per university policy at the time, taught in an online format similar to the approach in Spring 2022. Participating students from different instructors did not differ in their grade point average (GPA) entering the course (4-point scale, p < 0.91) or their final course grades (p < 0.58). Similarly, for Instructor 1, students did not differ between years for GPA (p < 0.31) or final course grade (p < 0.44). Student privacy was maintained and the identity of students participating in the study was never known to either instructor. A total of 51 students consented to participate.
Students were screened for learning disabilities, Attention-Deficit/Hyperactivity Disorder, experience of concussion, and other neurological diagnoses that might impact neural response patterns. One participant was excluded from analyses because they consistently gave the same response to every trial. Of the final analytic sample (N = 50, Mage = 19.62, SDage = 0.90), 35 (70%) were first-year freshmen, 12 (24%) were sophomores, and three (6%) were juniors. Seven (14%) were first-generation college students. Forty-three were European American/White, three were Hispanic, three were Asian, and one identified as both European American/White and Hispanic. Thirty-eight were female, 10 were male, and two identified as non-binary.
The model database started with a large set of models from textbooks and student-constructed models from past courses related to the concepts presented in the course. Twelve concepts (each one designated as a series, e.g., 1XX and 10XX, Table 2) were selected from this database: four each in evolution and ecology, two each in physiology and genetics. While genetics was not a specific course content area, central dogma and origins of alleles are concepts fundamental to the evolutionary mechanisms that are present in the course. Undergraduate teaching assistants from the course were recruited to pilot the evaluation task which led to revisions that simplified chosen models. Each concept modeled was represented in correct and incorrect versions, totaling 3 models per concept (Table 2; Supplementary material). Scientifically-sound explanatory models were numbered XX01 and XX02, e.g., 101 or 502, while explanatory models with intentionally-introduced errors were numbered XX05 or XX06, e.g., 105 or 1,106.
This work is complemented by a study identifying neural networks associated with error detection in models (Behrendt et al., 2024). In that study, students who displayed greater metacognitive calibration activated lateral prefrontal brain regions that have been associated with expert STEM reasoning. The experimental design accounts for the challenges and recruiting constraints inherent to neuroimaging studies. Recruited students completed the task inside an MRI scanner at the Center for Brain, Biology, and Behavior located at the University of Nebraska-Lincoln, during the last third of the course. Scheduling the MRI meant students were at different places in the course content although we did not observe differences in date of task on accuracy [Z(49) = 0.115, p = 0.909].
The task consisted of three runs of 12 models. For each model, students were first shown the binary prompt (“error” or “no error”), followed by the prompt + model, and then allowed up to 30 s to select a response. After each response, students were prompted to reflect on their level of confidence in their response by selecting “confident” or “not confident.” At the conclusion, students were debriefed by providing them with paper copies of each of the models in the order they had seen them. If the student had indicated an error for a model, the student was now instructed to circle the error they had observed. In some cases, students added additional details like what word they expected to see or a brief explanation.
Behavioral accuracy in this study was defined as selecting “no error” when presented with a model that had no intended error (i.e., a correct model), or selecting “error” on models that had an intended error (i.e., an incorrect model, Table 3). We recognized that students may be misidentifying errors, i.e., responding with an error when, in fact, it is correct, but the limitations of the MRI machine forced us to determine these cases using the debrief. Therefore, the behavioral accuracy does not capture whether students identified the intentionally introduced error or misidentified an error. A generalized linear model with binomial error distribution was fit to the accuracy data, assuming repeated measures, to analyze the effects of model types (SBF vs. schematic), subject area, confidence response, and course grade on behavioral accuracy. Students’ modeling abilities can parallel course performance in introductory biology and we expected high performing students (based on final course grade) to perform well on the model evaluation task because they likely had greater biology knowledge (Couch et al., 2019). To determine the likelihood (odds) of correctly responding to a particular model, a generalized linear regression model with logit link function and binomial error distribution was fit to the combined correct/incorrect responses.
Debrief responses were characterized in terms of what students noticed. Occasionally, a student would change their mind during the debrief and this was always in the direction of no longer feeling the model had an error and therefore there was no error to identify. The researchers discussed what to do for these cases and decided to not alter the within-MRI response. During the debrief, students either noticed the intended error or misidentified errors. Misidentified errors occurred both when identifying an error where none existed (i.e., in models with no intended error) or identifying an error that was different than the intended error (i.e., in models with an intended error). For all ambiguous cases (fewer than 20 out of 1,800 responses), the lead author decided whether students indicated the intended error or misidentified an error, conservatively characterizing these as noticing the intended error.
Two metrics were calculated to further clarify the relation of student confidence, accuracy, and noticing during the debrief: A modified knowledge corruption index (KCI) value and the noticing gap. KCI reveals whether students are misaligned and therefore overly confident in their responses rather than calibrated to their knowledge of the concept (Moritz et al., 2005). KCI is calculated as the proportion of all “confident” trials that were inaccurate. Greater KCI values suggest greater frequency of incorrect interpretation held with high confidence. The KCI is calculated from behavioral data (selecting “error”/“no error” and “confident”/“not confident”) during the MRI portion and the data were not connected to neuroimaging for the purpose of this study. During the debrief after the MRI scan, a noticing gap was calculated as the proportion of trials where students noticed intended errors (i.e., errors intentionally introduced into models) minus trials where they misidentified errors (i.e., identified errors in models with no intended error). The noticing gap during the debrief reveals students who notice intended errors more readily than misidentify errors. Trials where students responded “error” and “confident” would have overlapped between the KCI and the noticing gap and therefore we calculate the modified KCI as the proportion of inaccurate to accurate confident responses for models with no intended error. The relationship provides insight into which students are more calibrated because they are confident they know the errors and which students are overconfident and misidentifying errors in the models, i.e., students who confidently hold corrupted knowledge.
Overall, students were most accurate when presented with schematic and scientifically sound models, and in responses where they expressed confidence. Subject area did not affect the accuracy of responses. Students were accurate on 65% of models (M = 23.4 models, Mdn = 23) with a range of 16–33 accurate responses out of 36 models (Figure 2). Students with a higher final course grade were more accurate in their responses [Z(29) = 2.79, p < 0.01]. For each increment in course grade (i.e., course grade of 2.0 vs. 3.0), the proportion of correct responses increased by 0.34, or 34%. Students were confident in their responses on 67% of the models (Mdn = 25 responses) with a range of 17–36 out of 36 responses (Figure 2). Students with higher course grade did not have greater confidence in their responses [Z(49) = −0.83, p > 0.05]. Student accuracy was significantly greater on responses where they expressed confidence in their response [χ2(1) = 19.55, p < 0.001].
Figure 2. Effect of course grade on accuracy and confidence. Students with higher performance in the course had responses that were significantly more accurate (y = 0.34x-0.5, p < 0.01), yet these students were no more confident in their responses than lower performing students (p < 0.3).
Model format affected accuracy [χ2(1) = 6.20, p < 0.02] with schematic models resulting in accurate responses in 10.4 of 15 models and SBF models resulting in accurate responses to 13 of 21 models (Figure 3A). Students were 1.24 times more likely to respond accurately to schematic models than SBF models. The model format affected confidence [χ2(1) = 5.80, p < 0.02] and students reported confidence in their response to 11.8 of 15 schematic models and in 15.5 of 21 SBF models (Figure 3B).
Figure 3. Proportion of accurate responses by (A) model type and (C) subject area and proportion of confident responses for (B) model type and (D) subject area. Students were more likely to respond accurately and confidently to schematic models than box and arrow (SBF) models. Students were less likely to respond accurately and had lower confidence on physiology models. Bars represent standard error of means.
Students were significantly more accurate on models with no intended error [Z(1) = 8.40, p < 0.01] where they accurately responded to 10.3 out of 14 models and responded accurately to 13.1 of 22 models with an intended error. Students were 1.85 times more likely to respond accurately to a model with no intended error. Confidence in their responses was no different for models having no intended error and models having an intended error [Z(1) = −0.43, p > 0.05].
There was no significant effect of subject area on proportion of correct responses [χ2(3) = 5.27, p < 0.15], recognizing that within a subject area, concepts are not necessarily independent (Figure 3C). Ecology models (63% accurate, 7.6 accurate, SD = 2.02) and evolution models (64% accurate, 7.8 accurate, SD = 2.25) were intermediate with genetics models having the greatest accuracy (68% accurate, 4.1 accurate, SD = 1.4) and physiology models the lowest accuracy (61% accurate, 3.6 accurate, SD = 1.1). The subject area significantly affected confidence in their responses [χ2(3) = 20.45, p < 0.001]; physiology prompts decreased confidence by about 0.54 (SE = 0.26) compared to confidence in ecology models (Figure 3D). Students were more confident in responses to genetics responses (4.2 confident out of 6, 69%) and less confident in physiology responses (3.7 confident out of 6, 61%) with ecology (7.7 confident out of 12, 64%) and evolution (7.9 confident out of 12, 66%) intermediate.
Student accuracy varied with the model they were evaluating (Figure 4), with a median accuracy of 65%. Twelve models frequently elicited accurate responses for more than 75% of the students, half of these models having no intended errors (101, 401, 801, 901, 1,001, 1,101) and half having an intended error (606, 806, 906, 1,005, 1,106, 1,205). Two models frequently elicited inaccurate responses, 205 at 25%, and 405 at 27% accurate. Accuracy varied even within a series. For example, in the series related to the pathway of carbon (1XX series), 92% of students responded accurately when evaluating 101, while only 38% responded accurately on model 102, and 33% responded accurately on model 105. Series related to carbon cycle (2XX), evolution of antibiotic resistance (4XX), pathway of fat atoms (7XX), human ancestry (8XX), central dogma (11XX) also show sizable variation in accurate responses (Figure 3).
Figure 4. Confidence and accuracy per model. Values are proportion of students to accurately respond and percent of students to select “confident” for each model (gray lines represent median). Models right of the median line suggest student prior knowledge is strongly connected into an explanatory model (above median confidence) or weakly connected (below median confidence). Models left of median accuracy suggest gaps in prior knowledge or misconceptions leading to overconfidence (above median confidence) and uncertainty (below median confidence).
Students’ confidence in their responses was higher than accuracy with a median of 69%. One model series (601, 605, 606), tracing the pathway of glucose from absorption to muscle, elicited “not confident” responses from 47, 45, and 42% of students. Conversely, models about the central dogma (1,101, 1,105, 1,106), and models of the energy pyramid in communities (901, 905, 906), elicited high confidence in responses (Figure 4).
During the debrief, students were asked to circle the error in the models where they had responded “error.” Two students were not debriefed because of time constraints and results represent responses from 48 students. Students were asked to identify errors on a range of 8–31 models (Mdn = 22 models) depending on how many models they had determined to have errors. Students noticed the intended errors 60% of the times they were asked, although there was considerable variation in this with one student only identifying the intended error on 1 of 8 models they were asked, and another identifying the intended error on 15 of 16 models they were asked.
Students regularly failed to notice the intended errors. For model 206, students often misidentified “plants respire CO2” and models 102, 201, and 601 often elicited students misidentifying errors in models where none existed. For 102, students often misidentified “plants absorb O2,” for 201 students misidentified “plants respire CO2,” and for 601 students circled parts of the model related to the placement of the liver in the sequence and the relationship “carried to.”
The noticing gap reveals students who noticed intended errors more readily and the median gap was 0.21, or 21%, with a range of −0.75 (mostly noticed intended errors) to 0.88 (mostly misidentified errors, Figure 5). The noticing gap was unaffected by final course grade [t(49) = 1.83, p > 0.05].
Figure 5. Noticing gap and modified knowledge corruption index. Students who tended to be overconfident during the task in the MRI scanner also tended to notice unintended errors when debriefed on the errors they had seen during the MRI scan. Noticing gap is proportion of models where students had responded “error,” where they noticed the intended versus unintended errors. The modified knowledge corruption index was proportion of inaccurate responses when student responded confident to models with no intended error.
We carefully interpret the modified knowledge corruption index because this metric has been used diagnostically in medical studies where the number of trials is large and participants may have cognitive challenges. Our interest is in how the modified KCI varies by student relative to the noticing gap that was calculated from data collected during the debrief (Figure 5). The modified KCI does capture a range of students from calibrated to overconfident in their knowledge. The median modified KCI value was 0.44 with a range of 0.14 (mostly accurate when confident, i.e., highly calibrated) to 0.69 (mostly inaccurate when confident, i.e., overconfident) was unaffected by final course grade [t(49) = −1.88, p > 0.05]. The correlation coefficient for noticing gap and the modified KCI was r = −0.64.
Student evaluation of models is a complicated interplay of subject-specific knowledge, perception of model characteristics that match their knowledge of the phenomena, and confidence in their own knowledge structures. We start by exploring how prior knowledge and model-based reasoning affect performance on the task before examining how these results explain variation in student error detection, and the implications for learning research and teaching practices.
We hypothesized high performing students would perform well on the model evaluation task because they likely had greater biology knowledge. Final course grade did positively relate to student accuracy on the model evaluation task although the student sample overrepresents high performing students. The 65% accuracy rate, lower if accounting for misidentifying errors, was lower than might be expected given students were concurrently enrolled in the course and the model contexts would be recent.
Students encountered different model formats, subject areas, and models with and without intended errors and our a priori hypothesis was that variation in the model attributes would correspond with variation in students’ biology knowledge. The study occurred during weeks 10–16 of the term, depending on student and MRI availability, following class content on the genetic basis of evolution and biodiversity (includes human evolution and phylogeny), and during the physiology and ecology units. Given the recency of genetics and evolution topics, we expected higher performance on models focused on these subject areas. Although no significant subject area differences were found in accuracy, physiology models had a lower accuracy (Figure 3). Physiology models were a particular challenge for students and confidence in physiology responses was significantly lower than other subject areas. Students may have recognized their limited knowledge of the represented physiology processes (Scott et al., 2023) affecting both accuracy (slightly) and confidence in their responses. While we can find nuance in the results, students’ overall accuracy on ecology and physiology was similar to evolution and genetics, perhaps suggesting students were using domain-general error detection strategies to compensate for variance in knowledge.
When looking at model formats, schematic models resulted in higher accuracy and more confident responses, acknowledging the limits of our experimental design to cross format and subject area (Figure 3). SBF formatted models may emerge from different pedagogical goals or to show different structures or processes (Quillin and Thomas, 2015). In our study, when presented with SBF models students had fewer accurate responses and fewer confident responses. This effect may be the byproduct of the experimental design where we were unable to represent all models in a single format. For example, we could not create an SBF model of a phylogenetic tree. This effect needs to be more systematically tested to determine the interaction of model format on student error detection abilities. It may also have more general implications for thinking about how to present scientific information and how to time and scaffold curricular content to leverage students’ abilities to achieve across model formats.
Two models (102 and 205) provoked frequent discussion among the researchers (Figure 1). Both models related to challenges associated with research on model-based reasoning and students’ limited knowledge about gas exchange between plants and atmosphere. In model 102, the arrow from Atmosphere to Plants is labeled “O2 absorbed by.” This is a scientifically sound proposition as plants facilitate gas exchange with the atmosphere and they both absorb and release O2 (and CO2) in respiration and photosynthesis. Twenty-nine students noticed this as an error and five students wrote or verbally responded with a variation of “plants release O2 rather than absorb it.” Model 205 raised a different question, also related to gas exchange in plants. In model 205, a critical arrow, from plants to atmosphere labeled “respire CO2,” is missing from the presented model (Figure 1). The case could also be made for model 206, where the arrow from atmosphere to plants labeled “photosynthesis,” is missing. Are missing arrows, relationships, or components, errors when they are central to the purpose of the model as described in the prompt? A few students clearly noticed the missing arrows. During the debrief, students 14, 36, and 52 circled the area without an error and wrote in “respire” and “photosynthesis,” and student 32 noticed the omission of “photosynthesis.” In model 201, 20 students circled, incorrectly, that plants “respire CO2” to the atmosphere. All students were focusing on this portion of the model as none of the other portions of the model were mentioned. Misconceptions around plants and their interactions with the atmosphere remain prevalent in university biology students (Parker et al., 2012). It is likely that students concurrently hold scientifically sound knowledge of these interactions and misconceptions about the matter flows and accumulations (Scott et al., 2023). Pedagogically, this plays an important role as we look to support students to critically evaluate their knowledge about these processes. While cognitively it is critical that students hold misconceptions and scientific knowledge (Kendeou et al., 2019) the nuance in this phenomena may be at too fine a scale to facilitate conceptual change.
Despite its brevity, the debrief provided more clarity about when students notice intended errors or misidentified errors. In the antibiotic resistance models (401, 405, 406) and the energy pyramid models (901, 905, 906) rarely resulted in misidentifying errors. The ecosystem carbon cycle models (201, 205, 206) and the pathway of glucose models (601, 605, 606) elicited students misidentifying errors, but for different reasons. The ecosystem carbon cycle elicited almost universal circling of the “primary producers respiring CO2” while the pathway of glucose elicited circling a variety of combinations of structures and relationships that appeared to be haphazard. The impact of model format or subject area will require a more systematic investigation across many more concepts to clarify student perceptional differences.
Our findings suggest that attributes of models may enhance or detract from students’ ability to detect errors. If broadly generalizable, these attributes have real consequences for learners, particularly in model-based instructional contexts. An inability to detect errors means students will be unable to perform sense-making during model construction or evaluation and be unable to make accurate predictions when applying models. Most consequentially, students will continue to rely on scientific inaccuracies that remain unchallenged.
The combination of accuracy, confidence, and noticing results provide clues about student variation in self-monitoring during model evaluation and gaps in knowledge or misconceptions. For instance, it is clear that student confidence in and of itself is not related to course performance (Figure 2), suggesting that students with the lowest model evaluation performance are not necessarily aware of their knowledge limitations. Relationships between accuracy and confidence for particular models reflect the variation in students’ metacognitive monitoring of their prior knowledge (Figure 3). Models represented in the Confident in Knowledge quadrant generated both accurate and confident responses. These included models about human ancestry, the energy pyramid, selection, and central dogma, although specific models in these series are represented elsewhere with lower confidence and/or accuracy. Models in the Underconfident in Knowledge quadrant (Figure 3), generated student responses suggestive that students had knowledge of these concepts because they accurately evaluated the models, however, they lacked confidence in their responses. Most models in this quadrant were scientifically sound versions with no intended errors and students may have been hedging their bets, tentatively registering a “no error” response because the model appeared “close enough” to their knowledge. These students were underconfident in their own knowledge (Table 1) and may be in the process of encoding new neural pathways that reflect more scientifically sound knowledge (Kendeou and O’Brien, 2014). The lower confidence of these students suggests that many students have prior knowledge that is weakly connected and may benefit from pedagogies that improve confidence in their foundational knowledge.
Models in the Overconfident in Misconceptions quadrant (Figure 4) align with persistent misconceptions, including those related to ecosystem carbon cycling (gas exchange in primary producers), antibiotic resistance (antibiotics cause mutations), genetic variation (meaning of transcription and translation), and animal physiology (matter converted into energy). Models in the Uncertain of Knowledge quadrant (Figure 4) elicited low confidence and low accuracy responses reflective of incomplete knowledge of the concepts for students to confidently determine the accuracy of the presented model. The level of self-monitoring is difficult to discern based on responses to these models with students likely showing low self-monitoring.
Students have many foundational knowledge structures that allow them to perform in class despite misconceptions related to the same topics. Physics experts exhibit tendencies of retaining misconceptions, and inhibiting them to more accurately perform on physics, but not biology tasks (Allaire-Duquette et al., 2021). Students in our study exist on a novice to expert spectrum for subject areas with generally higher performing students still holding, but likely inhibiting critical misconceptions. Course performance and final course grades, surrogates for general biology knowledge, did impact performance, with model attributes and self-monitoring acting as moderating factors in the performance differences.
Students with high KCI values often misidentified errors (Figure 5). Students who noticed errors where none existed exhibited a high incidence of misconceptions. Student 33 is a good example, writing during the debrief: only producers respire (model 201), respiration decreases greenhouse gases, not increases (301), mammals more closely related to amphibians [than reptiles] (501), and fat only turns into CO2 (701). Once a student performed a partial mapping of new information to their biology knowledge, and decided there was a misalignment with their prior knowledge, they stopped evaluating the model and were confident in their response (Cook et al., 2018). On the other side are students who were very good at noticing intended errors (upper left, Figure 5). Across the suite of concepts, at least seven students were excellent at both noticing intended errors and not misidentifying errors. These students likely held strong, well-connected explanatory models for the concepts and high self-monitoring, often matching their correct responses with confidence in their responses. These students exemplify an upper bound for expectations on this task—not perfect accuracy, confident in their knowledge, and able to identify the intended errors across subject areas. While these students likely encountered similar misconceptions in their schooling or lived experiences, they have also been able to create neural traces with scientifically sound knowledge that allows them to inhibit the misconceptions.
Recent work on evaluative mindset has provided key insights into how students may operate when encountering scientifically incorrect, or even purposefully false information. When people have sufficient background knowledge of the topic, they are fast and efficient at rejecting false information and routinely do this when reading text (Richter et al., 2009). Further clarifying this work, Wiswede et al. (2013) showed that evaluation of the validity of a text is dependent on the evaluative mindset of the participant, and can be thought of as a deliberate evaluation. The evaluative mindset reinforces the claim that “shallow processing is simply the result of an incomplete validation process” (Cook et al., 2018, p. 119). Presumably students whose responses placed them toward the top left (Figure 5) are doing evaluation differently than students who misidentify errors and are prone to overconfidence. Better error detection ability can support students being more calibrated as they become more aware of their prior knowledge.
The study design was a balance between behavioral cognitive and cognitive neuroscience designs in an educational context. To this end, the models we used were more simplistic than an interview study but lays the foundation for using a broader suite of formats and subject areas. Similarly, the binary response for accuracy and confidence was necessitated by the neuroscience constraints, yet still provides patterns of error detection ability and levels of self-monitoring that are infrequently found in authentic cognitive educational neuroscience studies (Fleur et al., 2021). As is typical in neuroimaging research (e.g., Masson et al., 2014; Declercq et al., 2022) students with neurological conditions likely to alter their neural response patterns, including ADHD, concussion, and learning disabilities, were excluded from this sample. This means that the sample is relatively homogeneous and does not reflect the full variation in cognitive responses that would occur in a typical undergraduate life sciences classroom. Studies that reflect the diversity of students within the typical classroom will be necessary before drawing conclusions for educational practice. Lastly, we acknowledge that error detection does not equal modeling but retain that error detection is a critical step in model-based reasoning and the transition from model construction to model application (Upmeier zu Belzen et al., 2021). Despite the limitations from combining study designs and theoretical constraints, the results provide incremental advancement of how teaching can be advanced through a biological understanding of how students learn.
Detecting the errors in one’s prior knowledge is a difficult but necessary step before students can create a new neural trace for the scientifically sound knowledge (Kendeou et al., 2019). This is especially true for the Uncertain quadrant (Figure 4) where students also had low confidence in their responses. People exhibit a bias toward accepting new information as true (Brashier and Marsh, 2020), encoding this information as true, creating a neural pathway that will need to be re-evaluated to change. When students in our study encountered these basic biology concepts, multiple times over many years, they may have encoded the misconceptions, setting a path that will require significant effort to change.
Ultimately, scientists use models and engage in modeling as a part of their work and students enrolled in science courses are developing productive ways to do the same. University instructors can be instrumental in providing opportunities for students to critically examine their knowledge and the reasons they know it. Importantly, instructors must normalize having, identifying, and learning from errors since we all possess and frequently inhibit many of the same misconceptions our students hold (Masson et al., 2014; Allaire-Duquette et al., 2021; Wan et al., 2023). Identifying errors in models, and the inferences about our own internal models, requires comparison that is time-intensive and effortful and must be made explicit for students. By framing the error detection process as common, expected, and beneficial, students receive many benefits including increased motivation (Steele-Johnson and Kalinoski, 2014) and improved connections between instructors and students (Cooper et al., 2018). Students, as with all people, remain curious and clearly do not want to hold scientifically incorrect knowledge. Learning effective self-monitoring skills to allow evaluation of their own knowledge structures is a necessary step that will allow students to transition from novice toward expert scientists and become better purveyors of scientific models.
The datasets presented in this study can be found in online repositories. The names of the repository/repositories and accession number(s) can be found at: https://doi.org/10.32873/unl.dr.20230419.1.
The studies involving humans were approved by the University of Nebraska Institutional Review Board (UNL IRB 20401). The studies were conducted in accordance with the local legislation and institutional requirements. The participants provided their written informed consent to participate in this study.
JD: Conceptualization, Data curation, Formal analysis, Funding acquisition, Investigation, Methodology, Project administration, Validation, Visualization, Writing – original draft, Writing – review & editing. MB: Conceptualization, Data curation, Investigation, Methodology, Software, Writing – original draft, Writing – review & editing. ME: Data curation, Investigation, Writing – original draft, Writing – review & editing. BG: Conceptualization, Data curation, Validation, Writing – original draft, Writing – review & editing. TL: Conceptualization, Data curation, Funding acquisition, Investigation, Validation, Visualization, Writing – original draft, Writing – review & editing. CC: Conceptualization, Data curation, Formal analysis, Funding acquisition, Investigation, Methodology, Project administration, Resources, Software, Supervision, Validation, Visualization, Writing – original draft, Writing – review & editing.
The author(s) declare financial support was received for the research, authorship, and/or publication of this article. This work was supported by the National Science Foundation (Grant DUE 2000549).
We would like to recognize the cooperation and support of Instructor 2. We had meaningful discussions about the methodology with E. Brewe, C. Hmelo-Silver, and J. Fugelsang. We appreciate the challenging conversations about the theoretical framing, results, and implications of this work from N. Faivre, A. Upmeier zu Belzen, D. Krüger, S. Bennett, A. Mashmoushi. And we gratefully acknowledge the students who volunteered for this study.
The authors declare that the research was conducted in the absence of any commercial or financial relationships that could be construed as a potential conflict of interest.
All claims expressed in this article are solely those of the authors and do not necessarily represent those of their affiliated organizations, or those of the publisher, the editors and the reviewers. Any product that may be evaluated in this article, or claim that may be made by its manufacturer, is not guaranteed or endorsed by the publisher.
The Supplementary material for this article can be found online at: https://www.frontiersin.org/articles/10.3389/feduc.2024.1356626/full#supplementary-material
Allaire-Duquette, G., Foisy, L.-M. B., Potvin, P., Riopel, M., Larose, M., and Masson, S. (2021). An fMRI study of scientists with a Ph.D. in physics confronted with naive ideas in science. Npj Sci. Learn. 6, 11–12. doi: 10.1038/s41539-021-00091-x
Behrendt, M. G., Dauer, J., and Clark, C. A. C. (2024). Relation of biology students’ metacognitive monitoring to neural activity during model-based scientific reasoning. NPJ Sci. Learn. doi: 10.21203/rs.3.rs-2874829/v1
Brashier, N. M., and Marsh, E. J. (2020). Judging truth. Annu. Rev. Psychol. 71, 499–515. doi: 10.1146/annurev-psych-010419-050807
Brookman-Byrne, A., Mareschal, D., Tolmie, A. K., and Dumontheil, I. (2018). Inhibitory control and counterintuitive science and Maths reasoning in adolescence. PLoS One 13:e0198973. doi: 10.1371/journal.pone.0198973
Clark, C. A. C., Helikar, T., and Dauer, J. (2020). Simulating a computational biological model, rather than Reading, elicits changes in brain activity during biological reasoning. CBE Life Sci. Educ. 19:ar45. doi: 10.1187/cbe.19-11-0237
Cook, A. E., Walsh, E. K., Bills, M. A. A., Kircher, J. C., and O’Brien, E. J. (2018). Validation of semantic illusions independent of anomaly detection: evidence from eye movements. Q. J. Exp. Psychol. 71, 113–121. doi: 10.1080/17470218.2016.1264432
Cooper, K. M., Downing, V. R., and Brownell, S. E. (2018). The influence of active learning practices on student anxiety in large-enrollment college science classrooms. Int. J. STEM Educ. 5:23. doi: 10.1186/s40594-018-0123-6
Couch, B. A., Wright, C. D., Freeman, S., Knight, J. K., Semsar, K., Smith, M. K., et al. (2019). GenBio-MAPS: a programmatic assessment to measure student understanding of vision and change Core concepts across general biology programs. CBE Life Sci. Educ. 18:ar1. doi: 10.1187/cbe.18-07-0117
D’Mello, S., Lehman, B., Pekrun, R., and Graesser, A. (2014). Confusion can be beneficial for learning. Learn. Instr. 29, 153–170. doi: 10.1016/j.learninstruc.2012.05.003
Dauer, J., Momsen, J. L., Speth, E. B., Makohon-Moore, S. C., and Long, T. M. (2013). Analyzing change in students’ gene-to-evolution models in college-level introductory biology. J. Res. Sci. Teach. 50, 639–659. doi: 10.1002/tea.21094
Declercq, M., Bellon, E., Sahan, M. I., Fias, W., and De Smedt, B. (2022). Arithmetic learning in children: An fMRI training study. Neuropsychologia 169:108183. doi: 10.1016/j.neuropsychologia.2022.108183
Dinsmore, D. L., and Parkinson, M. M. (2013). What are confidence judgments made of? Students’ explanations for their confidence ratings and what that means for calibration. Learn. Instr. Calibr. Calibr. 24, 4–14. doi: 10.1016/j.learninstruc.2012.06.001
Fleming, S. M., and Lau, H. C. (2014). How to measure metacognition. Front. Hum. Neurosci. 8:443. doi: 10.3389/fnhum.2014.00443
Fleur, D. S., Bredeweg, B., and van den Bos, W. (2021). Metacognition: ideas and insights from neuro- and educational sciences. NPJ Sci. Learn. 6, 13–11. doi: 10.1038/s41539-021-00089-5
Gilbert, J. K. (2004). Models and modelling: routes to more authentic science education. Int. J. Sci. Math. Educ. 2, 115–130. doi: 10.1007/s10763-004-3186-4
Goel, A., and Stroulia, E. (1996). Functional device models and model-based diagnosis in adaptive design. Artif. Intell. Eng. Design Anal. Manuf. 10, 355–370. doi: 10.1017/S0890060400001670
Gouvea, J., and Passmore, C. (2017). ‘Models of’ versus ‘models for’. Sci. & Educ. 26, 49–63. doi: 10.1007/s11191-017-9884-4
Kendeou, P., Butterfuss, R., Kim, J., and Van Boekel, M. (2019). Knowledge revision through the lenses of the three-pronged approach. Mem. Cogn. 47, 33–46. doi: 10.3758/s13421-018-0848-y
Kendeou, P., and O’Brien, E. (2014). “The knowledge revision components framework: processes and mechanisms” in Processing inaccurate information: theoretical and applied perspectives from cognitive science and the educational sciences. eds. D. N. Rapp and J. L. G. Braasch (Cambridge, MA: MIT Press), 353–377.
Krell, M., Upmeier zu Belzen, A., and Krüger, D. (2013). Students’ understanding of the purpose of models in different biological contexts. Int. J. Biolo. Educ. 2, 1–34.
Kruger, J., and Dunning, D. (1999). Unskilled and unaware of it: how difficulties in recognizing one’s own incompetence lead to inflated self-assessments. J. Pers. Soc. Psychol. 77, 1121–1134. doi: 10.1037/0022-3514.77.6.1121
Lehrer, R., and Schauble, L. (2000). Developing model-based reasoning in mathematics and science. J. Appl. Dev. Psychol. 21, 39–48. doi: 10.1016/S0193-3973(99)00049-0
Löhner, S., van Joolingen, W. R., Savelsbergh, E. R., and Hout-Wolters, B. (2005). Students’ reasoning during modeling in an inquiry learning environment. Comput. Hum. Behav. 21, 441–461. doi: 10.1016/j.chb.2004.10.037
Long, T. M., Dauer, J., Kostelnik, K. M., Momsen, J. L., Wyse, S. A., Speth, E. B., et al. (2014). Fostering Ecoliteracy through model-based instruction. Front. Ecol. Environ. 12, 138–139. doi: 10.1890/1540-9295-12.2.138
Magnani, L., Nersessian, N., and Thagard, P. (Eds.) (2012). Model-based reasoning in scientific discovery. Springer New York, NY: Kluwer Academic/Plenum Publishers.
Masson, S., Potvin, P., Riopel, M., and Foisy, L.-M. B. (2014). Differences in brain activation between novices and experts in science during a task involving a common misconception in electricity. Mind Brain Educ. 8, 44–55. doi: 10.1111/mbe.12043
Moritz, S., Woodward, T. S., Whitman, J. C., and Cuttler, C. (2005). Confidence in errors as a possible basis for delusions in schizophrenia. J. Nerv. Ment. Dis. 193, 9–16. doi: 10.1097/01.nmd.0000149213.10692.00
Myers, J. L., and O’Brien, E. J. (1998). Accessing the discourse representation during reading. Discourse Process. 26, 131–157. doi: 10.1080/01638539809545042
Nielsen, S. S., and Nielsen, J. A. (2021). A competence-oriented approach to models and modelling in lower secondary science education: practices and rationales among Danish teachers. Res. Sci. Educ. 51, 565–593. doi: 10.1007/s11165-019-09900-1
Odenbaugh, J. (2005). Idealized, inaccurate but successful: a pragmatic approach to evaluating models in theoretical ecology. Biol. Philos. 20, 231–255. doi: 10.1007/s10539-004-0478-6
Oh, P. S. (2019). Features of modeling-based abductive reasoning as a disciplinary practice of inquiry in earth science. Sci. & Educ. 28, 731–757. doi: 10.1007/s11191-019-00058-w
Papaevripidou, M., and Zacharia, Z. C. (2015). Examining how students’ knowledge of the subject domain affects their process of modeling in a computer programming environment. J. Comput. Educ. 2, 251–282. doi: 10.1007/s40692-015-0034-1
Parker, J. M., Anderson, C. W., Heidemann, M., Merrill, J., Merritt, B., Richmond, G., et al. (2012). Exploring undergraduates' understanding of photosynthesis using diagnostic question clusters. CBE Life Sci. Educ. 11, 47–57. doi: 10.1187/cbe.11-07-0054
Pennycook, G., Fugelsang, J. A., and Koehler, D. J. (2012). Are we good at detecting conflict during reasoning? Cognition 124, 101–106. doi: 10.1016/j.cognition.2012.04.004
Quillin, K., and Thomas, S. (2015). Drawing-to-learn: a framework for using drawings to promote model-based reasoning in biology. CBE Life Sci. Educ. 14:es2. doi: 10.1187/cbe.14-08-0128
Richter, T., Schroeder, S., and Wöhrmann, B. (2009). You don’t have to believe everything you read: background knowledge permits fast and efficient validation of information. J. Pers. Soc. Psychol. 96, 538–558. doi: 10.1037/a0014038
Schwarz, C., Reiser, B. J., Davis, E. A., Kenyon, L., Achér, A., Fortus, D., et al. (2009). Developing a learning progression for scientific modeling: making scientific modeling accessible and meaningful for learners. J. Res. Sci. Teach. 46, 632–654. doi: 10.1002/tea.20311
Scott, E. E., Cerchiara, J., McFarland, J. L., Wenderoth, M. P., and Doherty, J. H. (2023). How students reason about matter flows and accumulations in complex biological phenomena: an emerging learning progression for mass balance. J. Res. Sci. Teach. 60, 63–99. doi: 10.1002/tea.21791
Seel, N. M. (2017). Model-based learning: a synthesis of theory and research. Educ. Technol. Res. Dev. 65, 931–966. doi: 10.1007/s11423-016-9507-9
Steele-Johnson, D., and Kalinoski, Z. T. (2014). Error framing effects on performance: cognitive, motivational, and affective pathways. J. Psychol. 148, 93–111. doi: 10.1080/00223980.2012.748581
Upmeier zu Belzen, A., Engelschalt, P., and Krüger, D. (2021). Modeling as scientific reasoning—the role of abductive reasoning for modeling competence. Educ. Sci. 11:495. doi: 10.3390/educsci11090495
Upmeier zu Belzen, A., van Driel, J., and Krüger, D. (2019). “Introducing a framework for modeling competence” in Towards a competence-based view on models and modeling in science education, Models and modeling in science education (Cham: Springer International Publishing), 3–19.
VanLehn, K., Siler, S., Murray, C., Yamauchi, T., and Baggett, W. B. (2003). Why do only some events cause learning during human tutoring? Cogn. Instr. 21, 209–249. doi: 10.1207/S1532690XCI2103_01
Wan, T., Doty, C. M., Geraets, A. A., Saitta, E. K. H., and Chini, J. J. (2023). Responding to incorrect ideas: science graduate teaching assistants’ operationalization of error framing and undergraduate students’ perception. Int. J. STEM Educ. 10:5. doi: 10.1186/s40594-023-00398-8
Windschitl, M., Thompson, J., and Braaten, M. (2008). Beyond the scientific method: model-based inquiry as a new paradigm of preference for school science investigations. Sci. Educ. 92, 941–967. doi: 10.1002/sce.20259
Wiswede, D., Koranyi, N., Müller, F., Langner, O., and Rothermund, K. (2013). Validating the truth of propositions: behavioral and ERP indicators of truth evaluation processes. Soc. Cogn. Affect. Neurosci. 8, 647–653. doi: 10.1093/scan/nss042
Keywords: model-based learning, modeling, conceptual models, metacognition, confidence
Citation: Dauer J, Behrendt MG, Elliott M, Gettings B, Long T and Clark C (2024) Individual variation in undergraduate student metacognitive monitoring and error detection during biology model evaluation. Front. Educ. 9:1356626. doi: 10.3389/feduc.2024.1356626
Received: 15 December 2023; Accepted: 07 March 2024;
Published: 15 March 2024.
Edited by:
Miriam Segura, University of North Georgia, United StatesReviewed by:
Lisa Limeri, Texas Tech University, United StatesCopyright © 2024 Dauer, Behrendt, Elliott, Gettings, Long and Clark. This is an open-access article distributed under the terms of the Creative Commons Attribution License (CC BY). The use, distribution or reproduction in other forums is permitted, provided the original author(s) and the copyright owner(s) are credited and that the original publication in this journal is cited, in accordance with accepted academic practice. No use, distribution or reproduction is permitted which does not comply with these terms.
*Correspondence: Joseph Dauer, am9zZXBoLmRhdWVyQHVubC5lZHU=
Disclaimer: All claims expressed in this article are solely those of the authors and do not necessarily represent those of their affiliated organizations, or those of the publisher, the editors and the reviewers. Any product that may be evaluated in this article or claim that may be made by its manufacturer is not guaranteed or endorsed by the publisher.
Research integrity at Frontiers
Learn more about the work of our research integrity team to safeguard the quality of each article we publish.