- Free University of Berlin, Berlin, Germany
Collaborative online learning became a necessity for universities during the COVID-19 pandemic. Even though it is known from research that online collaboration is an effective way of learning, digital interaction can be challenging for learners. Group members have to create a high-quality interaction to ensure the success of the collaborative learning process. Based on a theoretical model of collaborative learning, high-quality interaction can be determined with regard to cognitive group activities (prior knowledge activation, transactivity), meta-cognitive group activities (organization of the work process), and relational group activities (group climate, participation and task-related communication). Our study aims to examine how students manage a self-directed collaborative learning setting, how they perceive the process quality of digital interaction and how the interaction quality is related to self-reported outcomes (learning gain and satisfaction). We use a newly developed questionnaire to assess the quality of digital interaction in terms of the aforementioned dimensions. Furthermore, we focus on associations with the beliefs about web-based learning and the ability of perspective-taking at the individual level as well as the sense of community at the group level. We conducted a quantitative study within online university courses that were implemented asynchronously due to the COVID-19 pandemic. N = 298 undergraduate students in teacher education rated the quality of a digital collaborative learning settings (response rate of 72%). The students worked on collaborative tasks autonomously without any guidance from the teacher. We find differences between (meta-)cognitive and relational factors of interaction quality, and differences in the strength of the associations with outcomes and individual and group-related factors. Our study provides insights into students´ collaborative online learning and examines the relationships between different dimensions of group interaction quality and the input and outcome variables. Limitations and areas for further research are discussed.
1 Introduction
Collaborative learning settings have been implemented worldwide for decades in educational contexts (Hmelo-Silver et al., 2013). Collaboration is anticipated to foster learners social competencies and motivation, as well as to promote deeper learning and understanding (Gillies, 2016; Ginsburg-Block et al., 2006; Hanson et al., 2016; Kyndt et al., 2013). Through knowledge co-construction, learners are expected to achieve more than they would individually achieve in a teacher-centered environment (Johnson and Johnson, 2009).
Along with innovations in educational technology over the last few years (Huang et al., 2019), collaborative online learning has gained importance since the early 2000s (Zawacki-Richter and Latchem, 2018) and has become particularly relevant during the COVID-19 pandemic. Due to the lockdown, universities and schools were forced to move into the digital space (García-Morales et al., 2021; Vahle et al., 2023) amid temporary restrictions on face-to-face teaching. Consequently, collaborative learning formats must be implemented online. Collaborative online learning is connected to specific affordances and challenges (Jeong and Hmelo-Silver, 2016) and can be realized in various forms (Jeong et al., 2019). High-quality interaction is one of the most important factors in determining the effectiveness of online learning (van Dorresteijn et al., 2024). There is a lack of empirical studies investigating the process characteristics of digital interactions in authentic learning situations (Vuopala et al., 2016).
In this study, we used a newly developed questionnaire to assess the quality of online collaborative learning among higher education students. The purpose of this study was to analyze the process quality of digital student interactions in a university setting. Students worked in groups during a university course that was implemented asynchronously due to the pandemic. Furthermore, the associations between interaction quality, individual and group-related factors, and outcomes were analyzed. This is essential for gaining a better understanding of how digital collaborative learning can be effectively implemented in higher education. The aim was to determine the factors that impact the success of collaborative learning to gain a better comprehension of the learning process.
This paper begins by presenting the theoretical framework, followed by a description of the research design and methods.
2 Theoretical background
2.1 Collaborative online learning
Collaborative learning is a widely used teaching format in higher education that allows students to share knowledge in self-directed learning environments. While some authors distinguish between collaborative and cooperative learning (Dillenbourg, 1999), others highlight their similarities (Kreijns et al., 2003). We follow the conclusions of Kirschner (2001), who emphasizes commonalities, and use the term collaborative learning as “an umbrella term for various instructional approaches to small group learning” (Yang, 2023, p. 718) throughout this study. In a collaborative learning setting, learners work in small groups to solve problems by exchanging and discussing ideas. They are stimulated to deal with others´ opinions, reflect on their own considerations, and take responsibility for their own and the group’s learning (e.g., Johnson and Johnson, 2009).
Based on research conducted over the last few decades, there is sufficient empirical evidence that collaborative learning is effective in enabling the co-construction of knowledge and deep learning (Johnson et al., 2007). It offers socially shared learning experiences and promotes motivation, social competencies, and learning achievements (e.g., Kyndt et al., 2013). At its best, collaborative learning can foster skills that are essential for 21st-century learning needs and success in future employment (OECD, 2019; Robbins and Hoggan, 2019). Beyond randomized controlled trial evidence for its effectiveness, collaborative learning “is underused in practice” (Scager et al., 2016, p. 1). True collaborative learning is challenging, and high-quality productive group interactions rarely exist in practice. The cognitive level of questions and explanations is often low; learners try to minimize their amount of work and interact at a low cognitive level (e.g., Antil et al., 1998; Kreijns et al., 2003; Ross, 2008).
Shifting from collaborative learning formats to digital environments presents additional challenges to group interaction and communication. Before the pandemic, Computer-Supported Collaborative Learning (CSCL) research focused on the use of technology for collaborative learning in higher education and its effectiveness (Cress et al., 2021; Dillenbourg et al., 2009). During the pandemic, a shift to online collaborative learning became imperative for universities. Digital collaboration allows students to continue to interact remotely with their peers. Since the pandemic began, researchers have examined how universities have managed to transition to online teaching (e.g., Crawford et al., 2020). However, the implementation of collaborative learning formats resulting from this transition has been investigated less frequently to date (Kalmar et al., 2022).
Online learning environments offer a range of possibilities for interaction, allowing groups of learners to engage in collaborative learning (Ku et al., 2013). Various digital tools can be used to support digital communication. Synchronous real-time video conferencing, chats and emails, discussion forums, document collaboration, and visual representation tools are the most common (Jeong and Hmelo-Silver, 2016). Regarding framework conditions, one essential requirement for the successful implementation of online learning is the absence of technical issues. Technical difficulties in online learning have been discussed as important factors impeding the effectiveness of collaborative learning (Sitzmann et al., 2010).
In principle, digital collaborative learning can be expected to be as effective as face-to-face collaboration, as shown by previous studies in higher education contexts (e.g., Chen et al., 2018; Graham and Misanchuk, 2004; Jonassen and Kwon, 2001). Findings from meta-analyses indicate differences in group performance and the quality of group interaction depending on the digital tools and learning environments used by learners (Chen et al., 2018; Jeong et al., 2019). Research also reveals that for online forms, the quality of interaction is often inadequate in educational practice, especially in the absence of tutor-led guidance and the structuring of collaborative activities (Kreijns et al., 2003; van Leeuwen and Janssen, 2019).
High-level group interaction can be considered the core process in collaborative (online) learning (Janssen and Kirschner, 2020). This determines the presumed outcomes demonstrated in several meta-analyses (e.g., Pai et al., 2015). Whether a group succeeds in establishing a high-quality interaction depends on the different factors of individuals, groups, and contexts (Gillies, 2016; Scager et al., 2016). To illustrate the relationships between the dependent factors, processes, and outcomes of collaborative learning, a theoretical model of collaborative learning is presented in the following chapter.
2.2 Theoretical model of collaborative learning: an input-process-outcome-model
A theoretical model of collaborative learning processes is shown in Figure 1. The model refers to the Implementing Collaborative Learning in the Classroom Framework (ICLC; Kaendler et al., 2015) and Input-Process-Outcome-Frameworks, which are established and empirically validated in team effectiveness research in work and organizational psychology (e.g., Dulebohn and Hoch, 2017). While Kaendler et al. (2015) focused on the teacher level, our study addressed the student level. The ICLC framework includes both teacher and student levels but primarily focuses on and specifies the teacher level. The student level is described in the interactive phase (with reference to Molenaar et al., 2011) by interaction quality, and more precisely, by collaborative, cognitive, and meta-cognitive group activities. We integrate these three factors into our model at the process stage. The quality of group interaction is conceptualized in our model as the key process variable at the student level, predicting the cognitive, motivational and social-communicative outcomes of individuals and groups.
The quality of the group process is impacted by the framework at the input stage. Early research on collaborative learning focused on contextual conditions such as collaborative tasks, resources, and communication media (Dillenbourg et al., 1996). Individual preconditions and group-level variables also influence whether a group succeeds in creating high-quality interactions. The following section focuses on the variables relevant to this study. In collaborative learning, social skills are essential for maintaining effective communication and interpersonal interactions (Johnson and Johnson, 2009; Prichard et al., 2006). Research also stresses the importance of individual motivational factors that are considered to be associated with students´ participation in collaborative interaction (Meyer and Turner, 2006). Individual motivational beliefs seem to affect group behavior in collaborative learning settings (Ahola et al., 2023). Furthermore, shared beliefs of the group as a social system predict the interaction behaviors of group members (van den Bossche et al., 2006).
Following our model, these different input variables directly affect the quality of the process stage, or more precisely, the group interaction quality. What factors constitute high-quality, productive group interactions? How can we describe high-quality group interactions? Social interaction is the key to collaborative learning. Grounded in social constructivist learning theory, studies have addressed how interactions in collaborative learning should be designed to ensure meaningful learning (Roschelle and Teasley, 1995; Vuopala et al., 2016). High-quality interaction between groups, representing the core condition for learning success, can be defined through cognitive, metacognitive and relational group activities (Kaendler et al., 2015; Molenaar et al., 2011).
Cognitive group activities refer to interactive learning processes and knowledge co-construction. The design of a co-constructive learning process (Webb, 2010) is integral to the success of group learning. Group interactions can only be productive if cognitive processes are stimulated in the learning process through exchange and collaborative reasoning in the sense of a learning talk (Alexander, 2017). This form of interaction challenges the activation of prior knowledge and stimulates mutual questioning, explanations, and the formulation and justification of hypotheses and opinions (Weinberger and Fischer, 2006). Confronting differing arguments challenges learners to compare them and justify and elaborate on their own (Chi and Wylie, 2014). Through interrelated and coherent interactions between group members, a transactive learning process that is superior to individual learning can be established (e.g., King, 1998; Fischer et al., 2013). The transactivity of group member interactions is the centerpiece of co-constructive collaboration (Janssen and Kirschner, 2020).
Metacognitive group activities focus on the joint organization of the group work process. Effective work organization is highly important for the success of collaboration (Kwon et al., 2014). The extent to which a group succeeds in structuring the work process through planning, goal-setting, and appropriate time allocation for collaborative tasks guides collaborative discussions. These elements are in line with strategies that function as group-based metacognition (Hadwin and Oshige, 2011; Järvelä and Hadwin, 2013; Järvelä et al., 2015). The organization of work processes indicates whether socially shared regulated learning (De Backer et al., 2022a) occurs in the group.
Relational group activities involve interpersonal components of the interaction. The participation of all group members is an important prerequisite for productive learning processes of the whole group (Isohätälä et al., 2017). Beebe and Masterson (2003) described domination or lack of participation by individual group members (e.g., due to uncertainty or poor commitment) as central factors that can impede the collaborative learning process (Kirschner et al., 2015). Whether communication in the group is task-related (i.e., focused on the learning goal and content) is also relevant (Chinn et al., 2000). The more effectively a group succeeds in reducing dysfunctional and task-irrelevant topics, the greater the likelihood of fostering productive interaction (Kauffeld and Lehmann-Willenbrock, 2012). A respectful group climate is a key factor in effective collaboration (e.g., Johnson and Johnson, 1999). Mutual appreciation and support are indispensable (Huang and Lajoie, 2023). West (1990) introduced the term “participative safety” for a trusting climate and defined it as a prerequisite for group members to contribute their ideas and develop solutions to problems (Edmondson, 1999).
Collaborative learning processes involve cognitive, metacognitive, and relational group activities that promote learning. Although several studies, particularly in the field of CSCL, address specific aspects (e.g., socially shared regulation, Järvelä et al., 2015), empirical knowledge regarding these factors remains limited. Few studies have simultaneously analyzed group interactions with regard to different process characteristics (Vuopala et al., 2019). There is also still a lack of research investigating the process quality of digital interactions in authentic online collaborative learning settings (Vuopala et al., 2016).
To gain a more comprehensive insight into digital interaction in a higher education context, we conducted a quantitative study using a new questionnaire developed by our research group. The questionnaire assesses the quality of interaction from students’ perspectives along the aforementioned dimensions and has been validated in a study investigating face-to-face collaborative learning (Bach and Thiel, 2024). To our knowledge, there is no instrument available that simultaneously consider the different factors of group interaction from a learner’s perspective. There is a need for instruments that make group interaction quality visible in a differentiated way and with which collaborative learning can be evaluated in higher education practice. The results of the validation study show that it is possible to differentiate empirically between various factors and that there are differential correlations with input factors or outcomes. The questionnaire was used to investigate students´ digital collaborative learning to answer the following research questions.
2.3 Research questions
In this study, we focus on three research questions that empirically investigate the theoretically assumed relationships depicted in the theoretical model of collaborative learning, as shown in Figure 1.
The first research question (RQ-1) focuses on framework conditions at the input stage. As the framework conditions in our study are not regulated by teachers, the students are completely autonomous in organizing the collaborative learning phases. Against this background, it is necessary to investigate how students manage the collaborative learning setting. These questions aimed to gain insight into the context of the collaborative learning process. As the collaborative learning phases were black boxes due to the design, it was first necessary to clarify whether the students were collaborating appropriately.
(RQ-1) How do students organize collaborative learning processes? Specifically, the following subquestions were addressed: How much time do groups invest in collaborative learning tasks? Are technical difficulties impeding digital collaboration? How often do students use different online tools for collaborative tasks, and are there associations between the different types of online tools and group interaction quality? Research indicates that group processing depends on the digital tools chosen for collaboration (Jeong et al., 2019).
The second research question (RQ-2) examines the quality of digital group interaction in association with outcomes (i.e., self-reported learning gain and satisfaction with the group process). Collaborative learning can enhance the competence gain and motivation of learners (Johnson and Johnson, 2009; Kyndt et al., 2013). The impact of different group activities on outcomes has not yet been examined simultaneously.
(RQ-2) Is the quality of digital interaction in groups related to outcomes (i.e., self-reported learning gain and satisfaction with the group process)? From a theoretical perspective, cognitive and metacognitive group activities are presumably more strongly related to cognitive learning gains than to satisfaction, whereas relational group activities are more strongly related to satisfaction (Chi and Wylie, 2014; Johnson and Johnson, 1999).
The third research question (RQ-3) examines the associations of group interaction quality and the outcomes with individual and group-level characteristics of the input stage. We focused on three potential determinants of the quality and effectiveness of collaborative learning.
First, the associations with cognitive perspective-taking ability of group members were investigated. As outlined above, social competencies are essential for appropriate behaviors in collaborative settings. They enable individuals to achieve their own goals while considering the interests of their group members. Cognitive perspective-taking ability, defined as the ability to understand and empathize with another person’s perspective, is crucial for effective group communication (Mouw et al., 2020). A high level of perspective-taking increases the group’s problem-solving competence and leads to more productive group interactions (Webb and Mastergeorge, 2003). However, empirical evidence supporting the significance of perspective-taking ability is lacking. This may be due to the fact that previous studies often linked it to achievement-related factors and did not investigate the association in relation to process characteristics of group interaction.
Second, associations within the context of digital learning relevant individual motivational variables were examined. Collaborative work is generally considered a highly motivating instructional approach. By integrating technology into collaborative learning, personal beliefs about online learning become increasingly relevant. Computer-related motivational attitudes have been a research topic since the 1980s (Coffin and MacIntyre, 1999). There is evidence that beliefs about online learning influence individual behavior and engagement in digital learning environments (Yang and Tsai, 2008).
Third, we focus on the groups´ shared beliefs about the group as a functioning social system (van den Bossche et al., 2006). With the sense of community, we consider an important motivational factor at group level that can predict how learners engage in collaborative learning (Delahunty et al., 2014). McMillan and Chavis (1986, p. 9) established a conceptual definition of the sense of community as “a feeling that members have of belonging, a feeling that members matter to one another and to the group, and a shared faith that members’ needs will be met through their commitment to be together.” Particularly in digital collaborative settings, a strong sense of community helps mitigate the isolation and absence of face-to-face interactions (Lowenthal et al., 2023). A strong sense of community increases the probability of engaging in collaborative learning (Reeves and Gomm, 2015), leads to a more positive perception of online courses (Baker and Moyer, 2019), and contributes to student learning outcomes (Battistich et al., 1995). Empirical studies are lacking to examine the relationships between a group’s sense of community, group interaction quality, and outcomes (Han et al., 2022).
(RQ-3) Are individual and group-related factors associated with the quality of digital interactions? It is assumed that high level of the ability of perspective-taking, beliefs about online learning, and a sense of community within the group are positively related to the quality of digital interaction in the groups and outcomes.
3 Methods
3.1 Research design
In the summer term of 2020, a quantitative standardized survey was conducted to examine the online collaborative learning of teacher education students in 13 undergraduate courses. In their second semester, the students attended an asynchronous course that was delivered online in the Blackboard learning management system due to the university’s closure during the COVID-19 pandemic. Every week, the students had access to recorded PowerPoint slides with inputs about the principles of teaching and learning and individual tasks that had to be completed autonomously. Furthermore, students were randomly assigned to fixed learning groups consisting of three or four persons. Eight collaborative learning tasks were conducted over a 12-week semester. The students decided which collaborative online tools they had used to complete the tasks. The students worked on collaborative tasks autonomously without any guidance from the teacher. An example of a collaborative learning task is shown in Figure 2. The collaborative task was embedded in a session on teaching quality and support for student knowledge acquisition in the classroom. After theoretical and audio-recorded input on the theories of memory, information processing, and text comprehensibility, a text vignette was provided to the students. A classroom scenario was described in the vignette. In preparation for the collaborative tasks, the students read the vignette independently. They were then instructed to make individual notes on how the teacher in the vignette could have better supported the students in their knowledge acquisition. They should refer to the previously presented theoretical content. After completing the individual pre-work, the students were instructed to organize group meetings online. They discussed their suggestions in their group and agreed on five measures. The measures were written in a template, justified in detail, and with reference to theoretically gained knowledge. After completing the collaborative task, the jointly created documents are uploaded to the learning management platform.
The other seven collaborative tasks were similarly structured; after the theoretical input, the students had to apply the newly acquired knowledge to a collaborative task. The tasks were explained using audio-recorded slides by a lecturer. Additional materials, such as templates or written work instructions, were provided. Other tasks included motivating students in the classroom, developing ethical standards for teaching, discussing classroom videos, and providing collaborative ratings of teaching quality. Approximately 60–90 min were scheduled for each collaborative task.
At the end of the semester, the students were asked to complete a standardized questionnaire on collaborative learning activities. The survey was conducted online using the Unizensus software supplied by Blubbsoft GmbH. The students were given 3 weeks to complete the online questionnaire and were reminded twice via email to participate. Participation was voluntary and anonymous.
3.2 Sample
The sample consists of N = 413 students nested in 129 groups. The mean group size was 3.3 persons: 60% of the groups consisted of three members, 34% of four members, and 6% of only two persons. We achieved a response rate of 72% at the individual level and included N = 298 students nested in 119 groups in our study (average cluster size was 2.3 group members). The mean age of the participants was 22.47 years (SD = 5.59, Mdn = 21 years), and 78% were female. Participants in the sample represented a broad range of academic subjects (e.g., mathematics/science, German/foreign languages). Forty-six percent were primary school students, and 54 % were secondary school students. Most students had worked together in groups for the first time; only 11% of respondents self-reported that they had “previously worked with one or more persons in a group.”
3.3 Measures
To address RQ-1 (framework conditions of collaborative learning settings), one question about the online tools that have been used for digital collaboration and one question regarding the extent of technical problems that have occurred during the collaborative activities (4-point Likert scale, 1: “Never,” 2: “Rarely, “3: “Often,” 4: “Always”) were included in our questionnaire. We also added one question to assess the time invested in the collaborative learning tasks (“How much time did you invest on average per week in the collaborative learning tasks?,” 8-point Likert scale, 1: “30 min” to 8: “240 min”).
To answer RQ-2 and RQ-3, group interaction quality and outcomes were assessed using a newly developed questionnaire for collaborative learning settings (Bach and Thiel, 2024). The first version of the questionnaire, designed to assess the quality of interaction, originally consisted of 48 items. It was pretested and validated on several samples of students in secondary schools (N = 932 students) and higher education (N = 333) to investigate its psychometric properties. The final questionnaire used in the current study contains a total of 31 items in German language. For this article, the authors translated all items into English. The questionnaire measured the collaborative learning processes along the dimensions of the groups´ cognitive, metacognitive, and relational activities. It includes six dimensions that are relevant for collaborative learning processes: Joint activation of prior knowledge (three items) and transactivity (six items) as cognitive group activities; organization (four items) as metacognitive; group climate (six items), participation (three items), and task-related communication (three items) as relational group activities. Regarding the outcomes, we examined the learning gain (three items) and overall satisfaction of learners with digital collaboration (three items).
To address RQ-3, the following additional scales were included in the survey. The ability of perspective-taking was assessed using the German subscale of the Interpersonal Reactivity Index (Davis, 1996; Paulus, 2009). Beliefs about online learning were captured using six items (with reference to Yang and Tsai, 2008). To capture the groups´ sense of community, we used three items to measure social entities (with reference to Jason et al., 2015). This subscale captures the experience of having performed in a well-functioning and effective group while having developed a strong sense of belonging within the group. All items were measured on a 6-point Likert scale (1: “Does not apply at all” to 6: “Fully applies”).
Finally, 5-digit group codes that were given to the groups a priori were asked in an open question to allow the assignment of individual ratings to the group level.
Example items are reported in Table 1.
3.4 Data analysis
The data were analyzed using quantitative methods in SPSS Statistics 28 (IBM Corp, 2021) and Mplus (Muthén and Muthén 1998–2017). Because of the nested data structure (individuals at Level 1 clustered in groups at Level 2), intraclass correlation coefficients, ICC(1), for the dimensions were calculated based on the null model within multilevel analyses. These values indicate the proportion of the total variance accounted for by the clustering of individuals into groups.
For RQ-1, descriptive results are presented. To answer RQ-2, we report the results of a multivariate path analysis with two dependent variables. Although the number of clusters is relatively high at Level 2, the average cluster size is very small, resulting in overestimated standard errors; thus, using multilevel analysis would lead to problems by underestimating the p-values. Applying the Mplus command “type is complex” ensures that the estimations are corrected for the standard errors considering the multilevel data structure and between-class variance. To address RQ-3, the results of the correlation analysis (Pearson’s coefficients) at Levels 1 and 2 are presented.
4 Results
4.1 RQ-1: framework conditions
The first research question aimed to determine the framework conditions for digital collaboration. On average, the groups invested about 1.5 to 2 h per week in digital collaborative tasks (M = 98.14 min, SD = 34.20, Mdn = 90 min). Regarding the digital tools the groups used to complete the collaborative tasks, the results showed that the groups in our sample mainly used video conferences (M = 2.72, SD = 1.21, N = 119) and messenger services (M = 3.66, SD = 0.51, N = 119). Emails (M = 2.09, SD = 0.69, N = 119), shared online documents (M = 1.59, SD = 0.81, N = 119), and online tools integrated into the learning management platform (M = 1.27, SD = 0.53, N = 119) were rarely applied to collaborative tasks. Technical difficulties did not occur often in the groups; 95% of the students reported that group communication was never or seldom impeded by technical issues (M = 1.67, SD = 0.48, N = 119).
At the group level, significant correlations with self-reported group interaction quality were found in relation to the online tools used by groups. The more frequently video conferencing was used for working on group tasks, the more likely the groups were able to establish elaborate and in-depth discussions. The results showed positive, small-to-medium (Cohen, 1988) correlations for transactivity (r = 0.38, p < 0.001), prior knowledge activation (r = 0.27, p = 0.003), and organization (r = 0.24, p = 0.009). No significant associations were found with group climate (r = 0.15, p = 0.106), participation (r = −0.14, p = 0.126), or task-related communication (r = 0.13, p = 0.152).
4.2 RQ-2: associations of interaction quality with learning gain and satisfaction
To determine whether the digital collaborative learning sessions were conducted effectively, the second research question focused on the quality of interaction and its associations with outcomes.
Descriptive statistics for all dimensions assessing the quality of digital interactions are shown in Table 2. While most groups succeeded in establishing a positive group climate, working focused on the tasks, and integrating all group members in the discussion, we found lower mean values for the cognitive and metacognitive group activities, that is, the joint activation of prior knowledge, transactivity, and organization of collaborative work. The ICC(1) values indicate that a substantial part of the variance (10–29%) was explained by group membership. We found higher values for the group climate, participation, and task-related communication than for (meta-) cognitive group activities.
We investigated whether the quality of group interactions led to better outcomes. Self-reported learning gain (ML1 = 4.23, SDL1 = 1.24, α = 0.89, ML2 = 4.22, SDL2 = 0.93, ICC(1) = 0.12) and overall satisfaction with the group process (ML1 = 4.65, SDL1 = 1.35, α = 0.93, ML2 = 4.58, SDL2 = 1.16, ICC(1) = 0.36) are considered cognitive and motivational outcomes.
Results of multivariate path analyses (see Figure 3) reveal that learning gain is greater for groups who systematically activate their prior knowledge (b = 0.16, SE = 0.06, β = 0.19, p = 0.005), and structure their learning process through meta-cognitive strategies (b = 0.29, SE = 0.07, β = 0.28, p < 0.001). If the group succeeds in establishing transactive group discussions, we find significant, positive associations with the satisfaction (b = 0.22, SE = 0.18, β = 0.20, p = 0.006), but not with the learning gain.
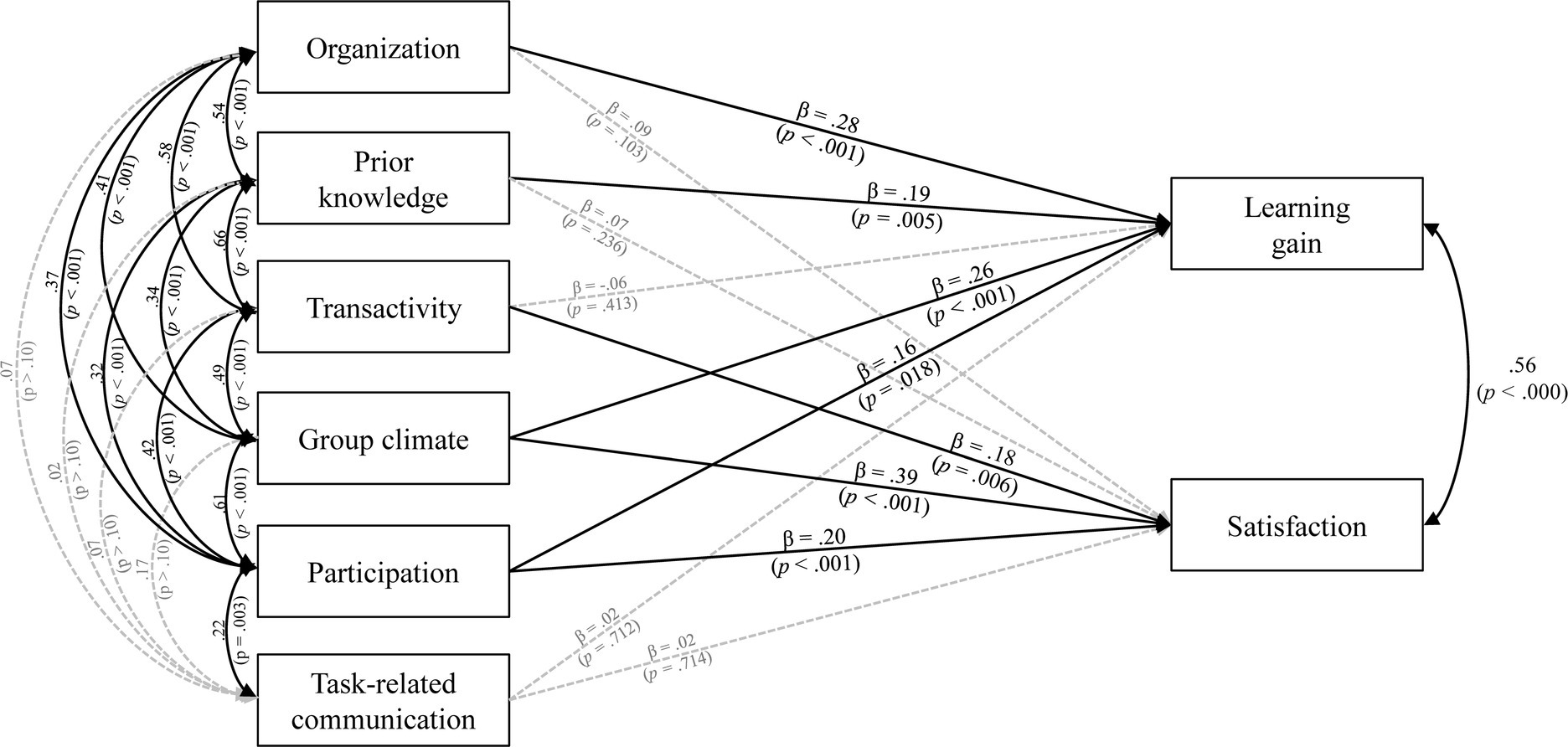
Figure 3. Results of path analysis with two dependent variables, learning gain and satisfaction, predicted by the six dimensions of interaction quality. Reported values are standarized coefficients with p-values in parantheses. Significant paths are depicted by solid lines, while non-significant lines are depicted by dotted lines (in grey). R2 (learning gain)=.40 (p < 0.001); R2 (satisfaction) =.55 (p < 0.001).
A positive group climate (b = 0.47, SE = 0.11, β = 0.26, p < 0.001) and the involvement of all group members (b = 0.13, SE = 0.06, β = 0.16, p = 0.018) also significantly predict the extent of the learning gain. Both variables have, in line with the expectations, a greater effect on the satisfaction with the group process (group climate: b = 0.79, SE = 0.13, β = 0.39, p < 0.001; participation: b = 0.19, SE = 0.05, β = 0.20, p < 0.001). No significant paths were found for the sub-dimensions of task-related communication. The independent variables accounted for 40% of the variance in predicting learning gains and 55% of the variance in predicting satisfaction. Model fit indices are not reported because the estimated path model was saturated (i.e., df = 0, perfect fit to data), as we expected associations between all variables.
4.3 RQ-3: associations between interaction quality and the ability of perspective-taking, beliefs about online learning, and the group’s sense of community
The third research question concerns how individual and group-related factors were associated with the quality of interaction in the groups. Table 3 presents the correlation coefficients at individual and aggregate group levels.
Concerning the ability of perspective-taking (ML1 = 5.00, SDL1 = 0.76, α = 0.70, ICC(1) = 0.06), we find moderate positive and significant correlations with the (meta-)cognitive subdimensions and small positive values for group climate participation and task-related communication. Except for the small positive correlations with the outcomes, mostly no significant correlations were observed between beliefs about online learning (ML1 = 3.55, SDL1 = 1.53, α = 0.93, ICC(1) = 0.11) and dimensions of interaction quality, which is contrary to expectations. At the group level, the sense of community (ML1 = 5.16, SDL1 = 1.21, α = 0.92, ML2 = 5.10, SDL2 = 1.01, ICC(1) = 0.30) is highly positively correlated, especially with group climate, participation, and overall satisfaction with the digital collaborative learning process.
5 Discussion
This study investigated online collaborative learning based on the perceptions of students attending an asynchronous university course. Groups of three or four students worked collaboratively throughout one semester. Lecturers introduced group tasks on recorded slides, and collaborative learning phases were conducted without teacher guidance. The groups decided autonomously how to complete the collaborative tasks. At the end of the semester, students rated the collaborative learning process. A new student questionnaire was used to assess the quality of collaborative learning (Bach and Thiel, 2024). Group interaction was operationalized via cognitive, metacognitive, and relational group activities relevant to the productivity of collaborative learning settings. Furthermore, questions about framework conditions, as well as individual and group characteristics, were included in the survey. Our study aimed to gain insight into self-directed collaborative online learning and empirically investigate the different pathways that contribute to the success of collaborative learning.
The results revealed that the groups, according to their self-reports, spent the expected amount of time on the collaborative learning tasks; on average, the groups invested slightly more than 1.5 h per week over the 12-week semester on the collaborative tasks. Video conferencing and messenger services were primarily used. Considering that videoconferencing is associated with more in-depth group interactions, it may be beneficial to specify in advance which tools should be used for collaborative learning. Technical difficulties identified in previous studies as the main barriers to digital collaboration (Sitzmann et al., 2010) were rare in our study. Consequently, the interaction quality ratings are consequently not affected by technical limitations.
Regarding the digital interaction quality, we found higher mean values for relational group activities than for cognitive or metacognitive activities. Establishing a respectful group climate, the participation of all group members, and task-related communication showed comparatively high averages, which is in line with findings from empirical instructional research (Fauth et al., 2014). According to our findings, relational group activities were also unaffected by the digital tools that the groups used to work on collaborative tasks. However, the use of video conferencing can stimulate the (meta-)cognitive learning process in a group. The quality of interaction with regard to the joint activation of prior knowledge, organization, and transactivity is associated with the increasing use of video conferencing. In line with the findings of Jeong et al. (2019), we found that fully asynchronous formats may not achieve the intended level of in-depth exchange and should be supplemented by synchronous formats (Joksimović et al., 2015). Furthermore, ICC-1 also showed higher values for relational activities than for (meta-)cognitive activities. This implies that group members tend to be more consistent in their ratings and that the instrument differentiates better between groups.
According to our results, the group climate and participation of all group members positively impacted learning gains and satisfaction with the collaborative learning process. This emphasizes the importance of these characteristics in digital collaboration group processes. However, we found no significant impact of task-related communication on the outcomes, perhaps because a certain level of task-irrelevant conversation in groups could foster group cohesion and even effectiveness, as suggested in the literature (O’Keefe, 1995). In this respect, a non-linear relationship may exist, which should be examined in follow-up studies. Furthermore, the study was conducted during the COVID-related lockdown, strongly impacted social contacts (Long et al., 2022). Working in online groups may have had a buffering effect; therefore, conversations about topics unrelated to the tasks compensated for social isolation.
Our results show stronger associations between the organization and prior knowledge dimensions with learning gain than with satisfaction with the collaborative learning process, which is in line with expectations. Contrary to expectations, the impact of transactivity on satisfaction was greater than that on cognitive learning gain. While the intercorrelation between transactivity and learning gain was significant (r = 0.45, p < 0.001), it disappeared when all factors were included in the model. This could indicate mediation effects, which need to be further investigated using with larger sample size.
Furthermore, only a limited number of factors associated with group interaction quality and outcomes could be empirically included. According to previous findings, an important factor could be the individual’s prior knowledge, which impacts the success of the learning process (Slof et al., 2021). Differences in group composition were also not considered (e.g., collective efficacy that could have a positive impact on group discussion and performance, see Wang and Lin, 2007).
According to our results, the ability of perspective-taking is also a precondition for the success of collaborative learning processes in digital interactions. At the individual level, there were consistently positive correlations of medium size between the ability of perspective-taking and the (meta-)cognitive group activities. This finding is also evident at the group level: groups with a higher average level of perspective-taking ability are also more effective in transactive communication and organization of group processes with regard to metacognitive group activities. This is a reasonable finding because the ability to empathize with others’ thoughts is a genuine aspect of transactive communication. With the ability of perspective-taking, we captured the cognitive part of the multidimensional construct of empathy rather than the emotional aspect (Davis, 1996), which may correlate more strongly with the motivation factors of the group process.
Our findings confirmed that creating a sense of community in digital interactions significantly affects the quality of digital interactions and outcomes. In line with expectations, there were strong associations with relational group activities, such as group climate, participation, and satisfaction. We found lower values for task-related communication only. This result confirms the aforementioned conclusion and is consistent with the assumption that a certain amount of private communication can positively affect group cohesion, particularly in digital collaborative learning processes.
According to our findings, beliefs about web-based learning do not seem meaningfully related to the quality of group interactions. The strongest association was observed with learning gains. However, a reciprocal relationship is also possible here; groups that have worked virtually successfully may also have improved their attitudes toward online learning as an outcome. Causal direction can only be ascertained using experimental and longitudinal studies. In addition, item formulations may lack specificity because they generally refer to learning through digital courses. In this respect, it is a distal factor in the collaborative learning process, which could explain the weaker relationship.
Overall, our study provides insights into digital collaborative learning in higher education during the COVID-19 pandemic. Using the new student questionnaire, group interaction quality can be assessed in a differentiated manner. The consideration of different group activities allows for the examination of specific, theoretically assumed relationships. However, this brings up an important limitation: the questionnaire is being used for the first time for the assessment of digital collaborative learning; thus far, it has only been validated in face-to-face settings (Bach and Thiel, 2024). The empirical findings can be considered as evidence of the construct validity of the questionnaire for digital collaborative learning. Nevertheless, follow-up studies should include further validation of this questionnaire. Objective data (e.g., student or group achievement) could be used for validation as well as observational studies to assess the quality of group interaction for comparison with self-ratings. Also, in terms of construct operationalization, it should be reflected that the questionnaire could be improved regarding the metacognitive group activities. The organization sub-dimension in our questionnaire could be expanded to include additional factors, such as collaborative reflection on the group process (De Backer et al., 2022b).
Further limitations include this study’s cross-sectional design with only a single measurement, which does not allow for the derivation of causal relationships. High-quality group interaction can also enhance the social competencies and the ability of perspective-taking of group members. Longitudinal studies are required to investigate the direction of these associations. Future studies could address this problem by adopting a longitudinal approach to enhance our understanding of the underlying mechanisms. Additionally, the study was conducted with only one cohort of students at a single university and, therefore, has restrictions in terms of the generalizability of the findings.
Furthermore, the limited sample size (particularly the average cluster size) poses an additional methodological challenge by restricting the use of latent multilevel analyses. This problem already occurred in the population because the group size was small in our collaborative learning setting. A low average cluster size in the sample was available for data analysis, resulting in statistical underpowering, which compromised the reliability of the group level. Increasing the sample size would also allow for multilevel confirmatory factor analyses and analyses of mediation effects.
In addition, a larger sample could also be used to analyze the gender differences identified in collaborative learning research (see Cai et al., 2017, for technology use; Costa et al., 2001, for social competencies). Our findings align with this perspective, showing different means in variance analyses (higher values for females in perspective-taking ability and for males regarding beliefs about web-based learning). However, due to the different group sizes in our sample (232 females and 66 males), the results should be validated in subsequent studies.
Finally, all analyses relied on self-reported data, which could have included response bias. Despite these limitations, our study provides insights into students´ collaborative online learning and examines the relationships between different dimensions of group interaction quality and the input and outcome variables.
Data availability statement
The data are available upon request to the authors. Requests to access the datasets should be directed to YW5hYmVsLmJhY2hAZnUtYmVybGluLmRl.
Ethics statement
Ethical approval was not required for the studies involving humans because criteria requiring an ethical approval were not given in accordance with the institutional requirements (no examination of patients, no risks, harm or discomfort to the participants, no vulnerable groups, use only non-identifiable data etc.). The studies were conducted in accordance with the local legislation and institutional requirements. The participants provided their written informed consent to participate in this study.
Author contributions
AB: Conceptualization, Software, Writing – original draft, Writing – review & editing, Data curation, Formal analysis, Investigation, Methodology, Project administration. FT: Conceptualization, Writing – review & editing, Project administration, Resources, Supervision.
Funding
The author(s) declare that financial support was received for the research, authorship, and/or publication of this article. We acknowledge support by the Open Access Publication Fund of Freie Universität Berlin.
Conflict of interest
The authors declare that the research was conducted in the absence of any commercial or financial relationships that could be construed as a potential conflict of interest.
Publisher’s note
All claims expressed in this article are solely those of the authors and do not necessarily represent those of their affiliated organizations, or those of the publisher, the editors and the reviewers. Any product that may be evaluated in this article, or claim that may be made by its manufacturer, is not guaranteed or endorsed by the publisher.
References
Ahola, S., Malmberg, J., and Järvenoja, H. (2023). Investigating the relation of higher education students’ situational self-efficacy beliefs to participation in group level regulation of learning during a collaborative task. Cogent Educ. 10:2164241. doi: 10.1080/2331186X.2022.2164241
Alexander, R. J. (2017). Towards dialogic teaching: Rethinking classroom talk. 5th Edn. York: Dialogos.
Antil, L. R., Jenkins, J. R., Wayne, S. K., and Vadasy, P. F. (1998). Cooperative learning. Prevalence, conceptualizations, and the relation between research and practice. Am. Educ. Res. J. 35, 419–454. doi: 10.3102/00028312035003419
Bach, A., and Thiel, F. (2024). Erfassung gruppenbezogener Lernprozesse–Validierung eines standardisierten Fragebogens für kooperative Lernsettings. [collaborative learning processes – validation of a standardized questionnaire for collaborative learning settings]. Z pädagog Psychol., 1–12. doi: 10.1024/1010-0652/a000388
Baker, K. Q., and Moyer, D. M. (2019). The relationship between students’ characteristics and their impressions of online courses. Am. J. Dist. Educ. 33, 16–28. doi: 10.1080/08923647.2019.1555301
Battistich, V., Solomon, D., Kim, D., Watson, M., and Schaps, E. (1995). Schools as communities, poverty levels of student populations, and students’ attitudes, motives, and performance: A multilevel analysis. Am. Educ. Res. J. 32, 627–658. doi: 10.3102/00028312032003627
Beebe, S. A., and Masterson, J. T. (2003). Communicating in small groups. Principles and practices. Boston: Pearson Education, Inc.
Cai, Z., Fan, X., and Du, J. (2017). Gender and attitudes toward technology use: A meta-analysis. Comput. Educ. 105, 1–13. doi: 10.1016/j.compedu.2016.11.003
Chen, J., Wang, M., Kirschner, P. A., and Tsai, C.-C. (2018). The role of collaboration, computer use, learning environments, and supporting strategies in CSCL: A meta-analysis. Rev. Educ. Res. 88, 799–843. doi: 10.3102/0034654318791584
Chi, M. T., and Wylie, R. (2014). The ICAP framework: linking cognitive engagement to active learning outcomes. Educ. Psychol. 49, 219–243. doi: 10.1080/00461520.2014.965823
Chinn, C. A., O'Donnell, A. M., and Jinks, T. S. (2000). The structure of discourse in collaborative learning. J. Exp. Educ. 69, 77–97. doi: 10.1080/00220970009600650
Coffin, R. J., and MacIntyre, P. D. (1999). Motivational influences on computer-related affective states. Comput. Hum. Behav. 15, 549–569. doi: 10.1016/S0747-5632(99)00036-9
Cohen, J. (1988). Statistical power analysis for the behavioral sciences. 2nd Edn. Hillsdale, NJ: Erlbaum.
Costa, P. T., Terracciano, A., and McCrae, R. R. (2001). Gender differences in personality traits across cultures: robust and surprising findings. J. Pers. Soc. Psychol. 81, 322–331. doi: 10.1037/0022-3514.81.2.322
Crawford, J., Butler-Henderson, K., Rudolph, J., Malkawi, B., Glowatz, M., Burton, R., et al. (2020). COVID-19: 20 countries' higher education intra-period digital pedagogy responses. J. Appl. Learn. Teach. 3, 1–20.
Cress, U., Rosé, C., Wise, A. F., and Oshima, J. (2021). International handbook of computer-supported collaborative learning. Cham, Switzerland: Springer Nature.
De Backer, L., Van Keer, H., De Smedt, F., Merchie, E., and Valcke, M. (2022a). Identifying regulation profiles during computer-supported collaborative learning and examining their relation with students’ performance, motivation, and self-efficacy for learning. Comput. Educ. 179:104421. doi: 10.1016/j.compedu.2021.104421
De Backer, L., Van Keer, H., and Valcke, M. (2022b). The functions of shared metacognitive regulation and their differential relation with collaborative learners’ understanding of the learning content. Learn. Instr. 77:101527. doi: 10.1016/j.learninstruc.2021.101527
Delahunty, J., Verenikina, I., and Jones, P. (2014). Socio-emotional connections: identity, belonging and learning in online interactions. A literature review. Technol. Pedagog. Educ. 23, 243–265. doi: 10.1080/1475939X.2013.813405
Dillenbourg, P. (1999). “What do you mean by 'collaborative learning'?” in Collaborative Learning. ed. P. Dillenbourg (Oxford: Elsevier), 1–19.
Dillenbourg, P., Baker, M., Blaye, A., and O’Malley, C. E. (1996). “The evolution of research on collaborative learning” in Learning in humans and machine: Towards an interdisciplinary learning science. eds. E. Spada and P. Reiman (Oxford: Elsevier), 189–211.
Dillenbourg, P., Järvelä, S., and Fischer, F. (2009). “The evolution of research on computer supported collaborative learning: from design to orchestration” in Technology-enhanced Learning: Principles and products. eds. N. Balacheff, S. Ludvigsen, and T. Jong (New York: Springer), 3–19.
Dulebohn, J. H., and Hoch, J. E. (2017). Virtual teams in organizations. Hum. Resour. Manag. Rev. 27, 569–574. doi: 10.1016/j.hrmr.2016.12.004
Edmondson, A. C. (1999). Psychological safety and learning behavior in work teams. Adm. Sci. Q. 44, 350–383. doi: 10.2307/2666999
Fauth, B., Decristan, J., Rieser, S., Klieme, E., and Büttner, G. (2014). Student ratings of teaching quality in primary school: dimensions and prediction of student outcomes. Learn. Instr. 29, 1–9. doi: 10.1016/j.learninstruc.2013.07.001
Fischer, F., Kollar, I., Stegmann, K., and Wecker, C. (2013). Toward a script theory of guidance in computer-supported collaborative learning. Educ. Psychol. 48, 56–66. doi: 10.1080/00461520.2012.748005
García-Morales, V. J., Garrido-Moreno, A., and Martín-Rojas, R. (2021). The transformation of higher education after the COVID disruption: emerging challenges in an online learning scenario. Front. Psychol. 12:616059. doi: 10.3389/fpsyg.2021.616059
Gillies, R. M. (2016). Cooperative learning, review of research and practice. Aust. J. Teach. Educ. 41, 39–54. doi: 10.14221/ajte.2016v41n3.3
Ginsburg-Block, M. D., Rohrbeck, C. A., and Fantuzzo, J. W. (2006). A meta-analytic review of social, self-concept, and behavioral outcomes of peer-assisted learning. J. Educ. Psychol. 98, 732–749. doi: 10.1037/0022-0663.98.4.732
Graham, C. R., and Misanchuk, M. (2004). “Computer-mediated learning groups: benefits and challenges to using groupwork in online learning environments” in Online collaborative Learning: Theory and practice. ed. T. S. Roberts (US: IGI global), 181–202.
Hadwin, A., and Oshige, M. (2011). Self-regulation, coregulation, and socially shared regulation. Exploring perspectives of social in self-regulated learning theory. Teach. Coll. Rec. 113:240264.
Han, J., Jiang, Y., Mentzer, N., and Kelley, T. (2022). The role of sense of community and motivation in the collaborative learning: an examination of the first-year design course. Int. J. Technol. Des. Educ. 32, 1837–1852. doi: 10.1007/s10798-021-09658-6
Hanson, J. M., Trolian, T. L., Paulsen, M. B., and Pascarella, E. T. (2016). Evaluating the influence of peer learning on psychological well-being. Teach. High. Educ. 21, 191–206. doi: 10.1080/13562517.2015.1136274
Hmelo-Silver, C. E., Chinn, C. A., Chan, C. K. K., and O’Donnell, A. (2013). The international handbook of collaborative learning. New York, NY: Routledge.
Huang, X., and Lajoie, S. P. (2023). Social emotional interaction in collaborative learning: why it matters and how can we measure it? Soc Sci Humanit. 7:100447.
Huang, R., Spector, J. M., and Yang, J. (2019). Educational technology a primer for the 21st century. Singapore: Springer.
Isohätälä, J., Järvenoja, H., and Järvelä, S. (2017). Socially shared regulation of learning and participation in social interaction in collaborative learning. Int. J. Educ. Res. 81, 11–24. doi: 10.1016/j.ijer.2016.10.006
Janssen, J., and Kirschner, P. A. (2020). Applying collaborative cognitive load theory to computer-supported collaborative learning: towards a research agenda. Educ. Technol. Res. Dev. 68, 783–805. doi: 10.1007/s11423-019-09729-5
Järvelä, S., and Hadwin, A. F. (2013). New frontiers: regulating learning in CSCL. Educ. Psychol. 48, 25–39. doi: 10.1080/00461520.2012.748006
Järvelä, S., Kirschner, P. A., Panadero, E., Malmberg, J., Phielix, C., Jaspers, J., et al. (2015). Enhancing socially shared regulation in collaborative learning groups: designing for CSCL regulation tools. Educ. Technol. Res. Dev. 63, 125–142. doi: 10.1007/s11423-014-9358-1
Jason, L. A., Stevens, E., and Ram, D. (2015). Development of a three-factor psychological sense of community scale: sense community scale. J. Community Psychol. 43, 973–985. doi: 10.1002/jcop.21726
Jeong, H., and Hmelo-Silver, C. E. (2016). Seven affordances of computer-supported collaborative learning: how to support collaborative learning? How can technologies help? Educ. Psychol. 51, 247–265. doi: 10.1080/00461520.2016.1158654
Jeong, H., Hmelo-Silver, C. E., and Jo, K. (2019). Ten years of computer-supported collaborative Learning: A meta-analysis of CSCL in STEM education during 2005-2014. Educ. Res. Rev. 28:100284. doi: 10.1016/j.edurev.2019.100284
Johnson, D. W., and Johnson, R. T. (1999). Learning together and alone. Cooperative, competitive and individualistic learning. Boston, MA: Allyn and Bacon.
Johnson, D. W., and Johnson, R. T. (2009). An educational psychology success story: social interdependence theory and cooperative learning. Educ. Res. 38, 365–379. doi: 10.3102/0013189X09339057
Johnson, D. W., Johnson, R. T., and Smith, K. A. (2007). The state of cooperative learning in postsecondary and professional settings. Educ. Psychol. Rev. 19, 15–29. doi: 10.1007/s10648-006-9038-8
Joksimović, S., Gašević, D., Kovanović, V., Riecke, B. E., and Hatala, M. (2015). Social presence in online discussions as a process predictor of academic performance. J. Comput. Assist. Learn. 31, 638–654. doi: 10.1111/jcal.12107
Jonassen, D. H., and Kwon, H. (2001). Communication patterns in computer mediated versus face-to-face group problem solving. Educ. Technol. Res. Dev. 49, 35–51. doi: 10.1007/BF02504505
Kaendler, C., Wiedmann, M., Rummel, N., and Spada, H. (2015). Teacher competencies for the implementation of collaborative learning in the classroom: A framework and research review. Educ. Psychol. Rev. 27, 505–536. doi: 10.1007/s10648-014-9288-9
Kalmar, E., Aarts, T., Bosman, E., Ford, C., de Kluijver, L., Beets, J., et al. (2022). The COVID-19 paradox of online collaborative education: when you cannot physically meet, you need more social interactions. Heliyon. 8:e08823. doi: 10.1016/j.heliyon.2022.e08823
Kauffeld, S., and Lehmann-Willenbrock, N. (2012). Meetings matter. Effects of team meetings on team and organizational success. Small Group Res. 43, 130–158. doi: 10.1177/1046496411429599
King, A. (1998). Transactive peer tutoring. Distributing cognition and metacognition. Educ. Psychol. Rev. 10, 57–74. doi: 10.1023/A:1022858115001
Kirschner, P. A. (2001). Using integrated electronic environments for collaborative teaching/learning. Learn. Instr. 10, 1–9. doi: 10.1016/S0959-4752(00)00021-9
Kirschner, P. A., Kreijns, K., Phielix, C., and Fransen, J. (2015). Awareness of cognitive and social behaviour in a CSCL environment. J. Comput. Assist. Learn. 31, 59–77. doi: 10.1111/jcal.12084
Kreijns, K., Kirschner, P. A., and Jochems, W. (2003). Identifying the pitfalls for social interaction in computer-supported collaborative learning environments: A review of the research. Comput. Hum. Behav. 19, 335–353. doi: 10.1016/S0747-5632(02)00057-2
Ku, H. Y., Tseng, H. W., and Akarasriworn, C. (2013). Collaboration factors, teamwork satisfaction, and student attitudes toward online collaborative learning. Comput. Hum. Behav. 29, 922–929. doi: 10.1016/j.chb.2012.12.019
Kwon, K., Liu, Y.-H., and Johnson, L. P. (2014). Group regulation and social-emotional interactions observed in computer supported collaborative learning: comparison between good vs. poor collaborators. Comput. Educ. 78, 185–200. doi: 10.1016/j.compedu.2014.06.004
Kyndt, E., Raes, E., Lismont, B., Timmers, F., Cascallar, E., and Dochy, F. (2013). A meta-analysis of the effects of face-to-face cooperative learning. Do recent studies falsify or verify earlier findings? Educ. Res. Rev. 10, 133–149. doi: 10.1016/j.edurev.2013.02.002
Long, E., Patterson, S., Maxwell, K., Blake, C., Bosó Pérez, R., Lewis, R., et al. (2022). COVID-19 pandemic and its impact on social relationships and health. J. Epidemiol. Community Health 76, 128–132. doi: 10.1136/jech-2021-216690
Lowenthal, P. R., Horan, A., DeArmond, M. C., Lomellini, A., Egan, D., Johnson, M., et al. (2023). Classroom community and online learning: A synthesis of Alfred Rovai’s research. TechTrends 67, 931–944. doi: 10.1007/s11528-023-00904-3
McMillan, D., and Chavis, D. (1986). Sense of community: A definition and theory. J. Community Psychol. 14, 6–23. doi: 10.1002/1520-6629(198601)14:1<6::AID-JCOP2290140103>3.0.CO;2-I
Meyer, D. K., and Turner, J. C. (2006). Re-conceptualizing emotion and motivation to learn in classroom contexts. Educ. Psychol. Rev. 18, 377–390. doi: 10.1007/s10648-006-9032-1
Molenaar, I., Chiu, M. M., Sleegers, P., and van Boxtel, C. (2011). Scaffolding of small groups’ metacognitive activities with an avatar. Int. J. Comput.-Support. Collab. Learn. 6, 601–624. doi: 10.1007/s11412-011-9130-z
Mouw, J., Saab, N., Gijlers, H., Hickendorff, M., Paridon, Y. V., and Broek, P. (2020). The differential effect of perspective-taking ability on profiles of cooperative behaviours and learning outcomes. Frontline Learn. Res. 8, 88–113. doi: 10.14786/flr.v8i6.633
Muthén, L. K., and Muthén, B. O. (1998–2017). Mplus statistical analysis with latent variables: User’s guide. Los Angeles (CA): Muthén and Muthén.
O’Keefe, V. P. (1995). Speaking to think, thinking to speak: The importance of talk in the learning process. Portsmouth, NH: Boynton/Cook Publishers.
Pai, H.-H., Sears, D. A., and Maeda, Y. (2015). Effects of small-group learning on transfer: A meta-analysis. Educ. Psychol. Rev. 27, 79–102. doi: 10.1007/s10648-014-9260-8
Paulus, C. (2009). Der Saarbrücker Persönlichkeitsfragebogen SPF (IRI) zur Messung von Empathie Psychometrische Evaluation der deutschen Version des Interpersonal Reactivity Index. Uni Saarland. :9249. doi: 10.23668/psycharchives.9249
Prichard, J. S., Stratford, R. J., and Bizo, L. A. (2006). Team-skills training enhances collaborative learning. Learn. Instr. 16, 256–265. doi: 10.1016/j.learninstruc.2006.03.005
Reeves, T., and Gomm, P. (2015). Community and contribution: factors motivating students to participate in an extra-curricular online activity and implications for learning. E-Learn Digit Media 12, 391–409. doi: 10.1177/2042753015571828
Robbins, S., and Hoggan, C. (2019). Collaborative learning in higher education to improve employability: opportunities and challenges. New Dir Adult Contin Educ. 2019, 95–108. doi: 10.1002/ace.20344
Roschelle, J., and Teasley, S. D. (1995). “The construction of shared knowledge in collaborative problem solving” in Computer Supported Collaborative Learning. ed. C. O’Malley (Berlin: Springer), 69–97.
Ross, J. A. (2008). “Explanation giving and receiving in cooperative learning groups” in The Teacher’s role in implementing cooperative Learning in the classroom. Computer-supported collaborative Learning. eds. R. M. Gillies, A. F. Ashman, and J. Terwel, vol. 8 (Boston, MA: Springer), 222–237.
Scager, K., Boonstra, J., Peeters, T., Vulperhorst, J., and Wiegant, F. (2016). Collaborative learning in higher education: evoking positive interdependence. CBE Life Sci. Educ. 15, 1–9. doi: 10.1187/cbe.16-07-0219
Sitzmann, T., Ely, K., Bell, B. S., and Bauer, K. (2010). The effects of technical difficulties on learning and attrition during online training. J. Exp. Psychol. Appl. 16, 281–292. doi: 10.1037/a0019968
Slof, B., van Leeuwen, A., Janssen, J., and Kirschner, P. A. (2021). Mine, ours, and yours: whose engagement and prior knowledge affects individual achievement from online collaborative learning? J. Comput. Assist. Learn. 37, 39–50. doi: 10.1111/jcal.12466
Vahle, C., de Araujo, Z., Han, J., and Otten, S. (2023). Teachers’ instructional responses to the COVID-19 pandemic. Teach. Teach. Educ. 124:104040. doi: 10.1016/j.tate.2023.104040
van den Bossche, P., Gijselaers, W. H., Segers, M., and Kirschner, P. A. (2006). Social and cognitive factors driving teamwork in collaborative learning environments: team learning beliefs and behaviors. Small Group Res. 37, 490–521. doi: 10.1177/1046496406292938
van Dorresteijn, C., Fajardo-Tovar, D., Pareja Roblin, N., Cornelissen, F., Meij, M., Voogt, J., et al. (2024). What factors contribute to effective online higher education? A Meta-Review. Technol. Knowl. Learn., 1–32. doi: 10.1007/s10758-024-09750-5
van Leeuwen, A., and Janssen, J. (2019). A systematic review of teacher guidance during collaborative learning in primary and secondary education. Educ. Res. Rev. 27, 71–89. doi: 10.1016/j.edurev.2019.02.001
Vuopala, E., Hyvönen, P., and Järvelä, S. (2016). Interaction forms in successful collaborative learning in virtual learning environments. Act. Learn. High. Educ. 17, 25–38. doi: 10.1177/1469787415616730
Vuopala, E., Näykki, P., Isohätälä, J., and Järvelä, S. (2019). Knowledge co-construction activities and task-related monitoring in scripted collaborative learning. Learn. Cult. Soc. Interact. 21, 234–249. doi: 10.1016/j.lcsi.2019.03.011
Wang, S. L., and Lin, S. S. (2007). The effects of group composition of self-efficacy and collective efficacy on computer-supported collaborative learning. Comput. Hum. Behav. 23, 2256–2268. doi: 10.1016/j.chb.2006.03.005
Webb, N. M. (2010). “Peer learning in the classroom” in International encyclopedia of education. eds. P. Peterson, E. Baker, and B. McGaw (Oxford: Elsevier), 636–642.
Webb, N. M., and Mastergeorge, A. (2003). Promoting effective helping behavior in peer-directed groups. Int. J. Educ. Res. 39, 73–97. doi: 10.1016/S0883-0355(03)00074-0
Weinberger, A., and Fischer, F. (2006). A framework to analyze argumentative knowledge construction in computer-supported collaborative learning. Comput. Educ. 46, 71–95. doi: 10.1016/j.compedu.2005.04.003
West, M. A. (1990). “The social psychology of innovation in groups” in Innovation and creativity at work: Psychological and organizational strategies. eds. M. A. West and J. L. Farr (Chichester: Wiley), 4–36.
Yang, X. (2023). A historical review of collaborative learning and cooperative learning. TechTrends 67, 718–728. doi: 10.1007/s11528-022-00823-9
Yang, F.-Y., and Tsai, C.-C. (2008). Investigating university student preferences and beliefs about learning in the web-based context. Comput. Educ. 50, 1284–1303. doi: 10.1016/j.compedu.2006.12.009
Keywords: collaborative learning, digital interaction, group interaction, collaborative online learning, group activities, cooperative learning
Citation: Bach A and Thiel F (2024) Collaborative online learning in higher education—quality of digital interaction and associations with individual and group-related factors. Front. Educ. 9:1356271. doi: 10.3389/feduc.2024.1356271
Edited by:
Henrik Bellhäuser, Johannes Gutenberg University Mainz, GermanyReviewed by:
Victor Marrahi-Gomez, Universidad Francisco de Vitoria, SpainSiem Buseyne, Faculty of Psychology and Educational Sciences, Belgium
Copyright © 2024 Bach and Thiel. This is an open-access article distributed under the terms of the Creative Commons Attribution License (CC BY). The use, distribution or reproduction in other forums is permitted, provided the original author(s) and the copyright owner(s) are credited and that the original publication in this journal is cited, in accordance with accepted academic practice. No use, distribution or reproduction is permitted which does not comply with these terms.
*Correspondence: Anabel Bach, YW5hYmVsLmJhY2hAZnUtYmVybGluLmRl