- 1Competencies for Digitally-Enhanced Individualized Practice (CODIP), Faculty of Education, Leuphana University Lüneburg, Lüneburg, Germany
- 2Faculty of Education, Institute of Psychology in Education, Leuphana University Lüneburg, Lüneburg, Germany
- 3Faculty of Sustainability, Institute of Sustainability Psychology, Leuphana University Lüneburg, Lüneburg, Germany
Introduction: Though technologies for individualization appear to benefit primary school students’ learning, studies suggest that their integration remains sparse. Technology acceptance research has largely focused on exploring teachers’ general acceptance of educational technologies, although factors might predict usage intentions differently depending on the specific usage purpose of an educational technology. Digital learning platforms for individualized practice are comparably challenging and complex to use and so far, predictors of primary school teachers’ intention to integrate such technologies into lessons are largely unknown. Meanwhile, research on teachers’ technology acceptance generally lacks comparability due to the absence of a shared theoretical model and usage purpose specification.
Methods: In a sample of 272 German primary school teachers, this study aims to identify predictors of teachers’ acceptance of digital learning platforms for students’ individualized practice in consideration of the unified theory of acceptance and use of technology (UTAUT). To ensure a shared understanding, teachers were provided with a video which specified the addressed usage purpose. Regarding teachers’ usage intention, the explanatory power of the standard UTAUT predictors was investigated and compared with an extended UTAUT model accounting for seven additional context-specific predictors.
Results: The standard UTAUT significantly explained teachers’ usage intention, with performance expectancy, effort expectancy, and the availability of the necessary technical infrastructure showing significant associations with intention. However, neither a significant nor meaningful increase in explained variance was observed for the extended UTAUT model.
Discussion: Results suggest that the standard UTAUT model is sufficient in explaining teachers’ usage intention and that its extension by context-specific predictors provides no added value. Acceptance facilitating interventions should therefore target performance and effort expectancy as well as the availability of technical infrastructure. Thus, underlining that successful implementation of complex educational technologies should consider both, individual and structural factors.
1 Introduction
The potentials of digital technology to improve education are being increasingly recognized and initial meta-analytical findings demonstrate that educational technology can benefit student learning (Sung et al., 2016; Chauhan, 2017). Especially in primary education, teachers face increased heterogeneity among students (Kopp, 2009), which underlines their need to find ways to effectively address students’ individual learning needs. Digital technologies can help primary school teachers to individualize students’ learning and practice (Vanbecelaere et al., 2020) and findings from systematic reviews and meta-analyses encourage such technology’s potential to beneficially impact student learning outcomes (Major et al., 2021; van Schoors et al., 2021). Still, though results from the latest International Computer and Information Literacy Studies (ICILS) (Fraillon et al., 2014, 2020) indicate that the frequency of digital media usage is increasing, educational technology is still not used to its full potential. The international average of teachers using technology for individualized support (35.5%) reported in ICILS (Fraillon et al., 2020) remains low. In Germany, only 14.8% of teachers use technology for individualization (Eickelmann et al., 2019; Fraillon et al., 2020). During the COVID-19 pandemic, the lack of individualization was especially visible in German primary education, as digital technologies were hardly used for individual feedback or for learning and practice processes monitored by teachers (Forsa Politik- und Sozialforschung GmbH, 2020; Steinmayr et al., 2021). To identify ways to promote usage, this study investigates primary school teacher’s intention to use digital technologies for the specific purpose of enhancing students’ individualized practice, as intention to use is a major component of technology acceptance.
Teachers’ technology acceptance has been researched intensely in recent years (Scherer and Teo, 2019) and when the COVID-19 pandemic hit in 2020, the interest to investigate teachers intention and actual use of digital technology in education, for example, toward learning management systems (Dindar et al., 2021), e-learning (Fülöp et al., 2023), and cloud-based learning technology (Utami et al., 2022) further increased globally. As some studies reported an increased usage of educational technologies during the pandemic, teachers’ post-pandemic intention to maintain usage behavior was investigated as well (Chun and Yunus, 2023; Khong et al., 2023; Altan et al., 2024). Furthermore, more studies acknowledged that the purpose for using the focused technology should to be stated, e.g., Khlaisang et al. (2023) who investigated primary school teachers’ acceptance of mobile technologies for teaching thinking skills and Wohlfart et al. (2021) who interviewed teachers regarding their acceptance to use digital tools for distance teaching and learning. Since predictors of technology acceptance appear to differ depending on the reason behind (Mavroudi et al., 2021) and level of experience using it (De Smet et al., 2012; Dindar et al., 2021), the present study investigates intention to use technology for a specific purpose: students’ individualized practice. As the specification of usage purpose of a technology is often missing or vague in technology acceptance research in education (Kahnbach and Lehr, 2023) and technology acceptance research in education generally was found to be undertheorized (Hew et al., 2019), results regarding the relevant predictors of teachers’ acceptance of digital technology for this specific purpose are inconsistent. Therefore, we focused on explaining teachers’ intention to use such technologies as usage remains low (Eickelmann et al., 2019; Forsa Politik- und Sozialforschung GmbH, 2020; Fraillon et al., 2020). To do so, we chose the unified theory of acceptance and use of technology (UTAUT), a well-accepted model in technology acceptance research in education (Nistor et al., 2013; Granić, 2023), as the theoretical basis. As the UTAUT model has often been extended by context-specific predictors to increase the amount of explained variance in teachers’ technology acceptance (Pynoo et al., 2011; Gellerstedt et al., 2018; Balkaya and Akkucuk, 2021; Dindar et al., 2021; Mavroudi et al., 2021), we also evaluated whether context-specific predictors, for example, self-efficacy and attitude, meaningfully add to the explained variance in teachers’ intentions, also addressing the gap in theory-based technology acceptance research in education.
1.1 Digital learning platforms for individualized practice
Researchers have investigated potential advantages of using technology for individual support (Ghysels and Haelermans, 2018; Dietrich et al., 2021). One potential advantage is students receiving timely, detailed feedback on completed practice tasks via digital technology, which can benefit student learning performance (van der Kleij et al., 2015). Yet, there remains no uniform terminology for technology for individualization (van Schoors et al., 2021); and, therefore, no general term for technology for individualized practice. To start, van Schoors et al. (2021) emphasized distinguishing digital technologies that merely provide digitalized learning and practice materials from those that directly aim to support students’ individualized learning and practice. The latter is addressed by various educational technologies. For example, one functionality of digital learning platforms is employing student data to individualize student learning (Kerssens and van Dijck, 2021). Intelligent tutoring systems (ITS) can provide practice tasks and assistance completing them, based on individual students’ needs (Holmes et al., 2018). Smart learning management systems (smart LMS) are software-based platforms that aid education administration and the individualization of student learning, since student data can be analyzed and processed (Holmes et al., 2018). Hence, we chose to integrate each technology’s functionalities concerning individualized student practice within the term digital learning platforms for individualized practice (DLPiP) to specify the target technology. Consequently, DLPiP not only provide individualized support and tasks for students, selected by the teacher or system (van Schoors et al., 2021), they also can provide individualized feedback to the students and the teacher on students’ completion of tasks, as they are capable of generating and reporting data back. This enables teachers to engage in a more informed communication with students (Voss and Wittwer, 2020).
Furthermore, compared to the use of other educational technologies (e.g., tablets or smart boards), using DLPiP seems more complex. Since DLPiP not only replace analog educational material (reading from a tablet instead of a book) but analyze data, provide reports on students’ learning performance, give feedback, and personalize learning paths, they amplify and transform teaching and student learning (Hughes et al., 2006). Hence, it can be assumed that teachers need more in-depth competency and experience to use DLPiP to their full potential compared to, for instance, using a smart board. This assumption that DLPiP are particularly complex to use and require teachers to have a high level of technology competency is supported by the framework for exploring technology-enabled practice by Prestridge and Aldama (2016). Examples for DLPiP are “Anton” (Schaumburg, 2021) and “BetterMarks” (Holmes et al., 2018).
1.2 Technology acceptance of DLPiP
Research on teachers’ acceptance of educational technology for individualized practice is scarce. Most studies have focused on technology use in general, on computers, or on other digital hardware (Scherer and Teo, 2019; Buerger et al., 2021). Furthermore, technological factors like complexity or usage purpose have rarely been specified and considered in teacher technology acceptance research (Scherer et al., 2019) despite the relevance of predictors explaining technology acceptance varying, depending on the reason for use (De Smet et al., 2012; Mavroudi et al., 2021). Therefore, relevant predictors for the intention to use DLPiP may differ from those for teachers’ intention to use digital technologies that provide digitalized learning materials only (e.g., as investigated by Kreijns et al., 2013).
Several theoretical models have been applied to explain teachers’ acceptance of educational technologies. One leading model in technology acceptance research is the unified theory of acceptance and use of technology (UTAUT; Venkatesh et al., 2003) which is based on the technology acceptance model (TAM; Davis et al., 1989). Both TAM and UTAUT are well established to determine technology acceptance in education (Granić, 2023). However, since previous studies on teachers’ acceptance of technology which could be used for individualized practice often considered predictors from the UTAUT model, we chose it as our theoretical basis (Pynoo et al., 2011; De Smet et al., 2012; Abu-Shanab, 2014; Balkaya and Akkucuk, 2021; Dindar et al., 2021).
The UTAUT model (Figure 1) aims to explain both the intention to use a technology and actual use behavior. Intention refers to a person’s assumptions regarding their likelihood utilizing a technical system within a defined time frame and primarily predicts actual use of that technology (Venkatesh et al., 2003). Within the UTAUT are four predictors which explain intention to use a technology. Performance expectancy is the degree to which an individual believes that using a technology will benefit their job performance (Venkatesh et al., 2003); for teachers, this could relate to their expectation that the technology may enhance student performance or provide more efficient communication with students and parents. Effort expectancy is “the degree of ease associated with the use of [a technical] system” (Venkatesh et al., 2003, p. 550); in education, this could mean the set up and maintenance of the technology or potentially increased effort preparing materials online. Social influence is the degree to which a teacher perceives that important others (e.g., students, parents, and principal) believe the teacher should use educational technology (Venkatesh et al., 2003). Whether a teacher has all the resources necessary to use educational technologies, like the technical infrastructure (internet, software licenses, etc.), knowledge necessary to use them, and someone to support them is encapsulated within the facilitating conditions construct (Venkatesh et al., 2003). Since technology use by most teachers for educational purposes is voluntary in Germany, this study investigates technology acceptance in a voluntary use scenario. The UTAUT2 (Venkatesh et al., 2012) alters the UTAUT model to a voluntary use context. Consequently, facilitating conditions are also assumed to have direct influences on intention. During the COVID-19 pandemic, the technical infrastructure component of facilitating conditions was found to be an especially important prerequisite for teachers’ willingness to use educational technologies (Wohlfart et al., 2021).
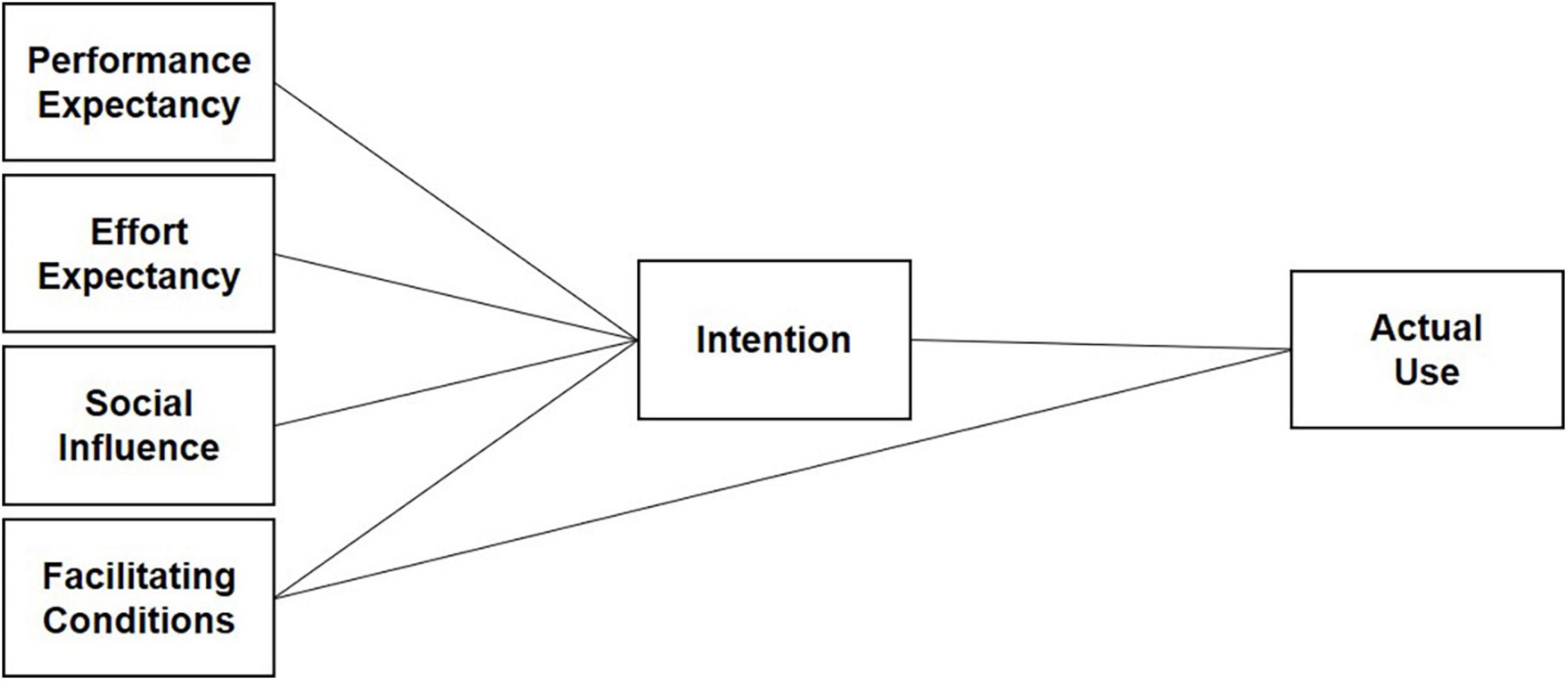
Figure 1. Unified theory of acceptance and use of technology in voluntary contexts (UTAUT, Venkatesh et al., 2003; UTAUT2, Venkatesh et al., 2012).
1.3 Context-specific predictors of intention
Technology acceptance models, like UTAUT, have often been altered and extended by adding further predictors to fit the research context (e.g., target group or technology) and enhance the models’ explanatory power (Granić, 2023). Earlier studies on teachers’ acceptance of technologies which could be used for individualized practice (Pynoo et al., 2011; De Smet et al., 2012; Abu-Shanab, 2014; Balkaya and Akkucuk, 2021; Dindar et al., 2021; Mavroudi et al., 2021) examined potentially relevant context-specific predictors for intention. However, when an established theory is extended, principles of theory construction methodology (Borsboom et al., 2021) must be considered to ensure robust theoretical advancement. An extended theoretical model should be substantively plausible, must meaningfully impact explanatory power, and should follow the principle of parsimony (Borsboom et al., 2021). As recommended by latest review-based and meta-analytical findings from technology acceptance research, we considered UTAUT as baseline model and reviewed additional context-specific predictors for potential extension, but also aimed to adhere to the principle of parsimony (Venkatesh et al., 2016; Blut et al., 2022).
Based on the literature, seven potential additional predictors—teaching style (individualization, practice, and feedback), previous experience in using technology, self-efficacy, attitude and work-related effort—of primary school teachers’ intention to use DLPiP were identified:
1.3.1 Teaching style
Bloemeke et al. (2006) described that teachers’ use of intelligent communication technology (ICT) relates to their teaching style (student- vs. teacher-centered). A student-centered teaching style is assumed to both efficiently integrate ICT and differentiate between students, in terms of task difficulty (Bloemeke et al., 2006). Eickelmann (2011, p. 78) concluded that […] “the more the computer-assisted teaching concepts resemble the subjective teaching style, the more likely teachers are to incorporate ICT into their teaching practice” […]. Gellerstedt et al. (2018) drew attention to the need to refine technology acceptance constructs and extend technology acceptance models in teaching; hence, teachers’ teaching style. As the use of digital technology for individualized practice refers to a student-centered teaching style which considers individualization, practice, and feedback, it is assumed that teachers who already integrate such aspects into their teaching are more likely to use technology for individualized practice. The characterization of different teaching styles was initially coined by Grasha and Yangarber-Hicks (2000), who differentiated teaching styles. Of these, the formal authority and facilitator teaching styles especially integrate individualization, practice, and feedback (Grasha and Yangarber-Hicks, 2000).
1.3.2 Experience with educational technology
The important role of precious mastery experiences on the initiation of new behavior was outlined as part of the social cognitive theory by Bandura (1986). Prior experiences with other educational technologies are therefore assumed to increase an optimistic self-belief in their own digital competencies to apply further educational technologies, such as DLPiP, successfully. The relevance of previous experience with educational technology to explain teachers’ intention to use DLPiP is underlined by De Smet et al. (2012), who categorized educational technology use into informational and communicational use, the latter described as more complex and favored by experience obtained through informational use. For example, communicational use of a learning-management system means utilizing assessment modules or learning paths (De Smet et al., 2012), which appears comparable to employing digital learning platforms for individualized practice. Therefore, experiences with learning platforms and other educational technology used for delivering information, sending announcements, or uploading exercises (informational usage, De Smet et al., 2012) could favor the intention of a communicational use of educational technology like DLPiP.
1.3.3 Self-efficacy
Closely related to the role of previous experiences is a person’s self-efficacy, the actual belief in their ability to achieve a goal successfully. According to Bandura’s (1986) social cognitive theory, it can be assumed that self-efficacy using DLPiP is a prerequisite for the intention to use DLPiP. Balkaya and Akkucuk (2021) and Dindar et al. (2021) found self-efficacy in using technology, appropriate instructional designs and in classroom management to be relevant to explaining teachers’ intention to adopt a learning management system. A systematic literature review regarding teachers’ acceptance of various educational technologies underlines the relevance of self-efficacy (Buerger et al., 2021). Therefore, we expected teachers’ self-efficacy employing DLPiP in class to contribute to explaining teachers’ intention to use DLPiP.
1.3.4 Attitude
According to the theory of reasoned action (Fishbein and Ajzen, 1975) and the theory of planned behavior (Ajzen, 1991), attitude toward a behavior is relevant to explain the intention to engage in the behavior. Findings from a systematic literature review and meta-analysis identified teachers’ attitude toward (educational) technology as significantly linked to intention (Scherer and Teo, 2019; Buerger et al., 2021). Therefore, we assumed that attitudes toward the use of digital technologies in education meaningfully contribute to explaining teachers’ intention to employ DLPiP.
1.3.5 Work-related effort
Approaches concerning resource-based theories of stress (e.g. conservation of resources theory; Hobfoll, 2001) imply the association of (work-related) stressors, a lack of resources and the ability to engage in a new activity which requires resources. Therefore, we expected teachers’ work-related efforts to be predictive of their intention to use DLPiP. There is evidence that teachers are experiencing increased occupational strain, generally and facing additional work-load due to the COVID-19 pandemic (Agyapong et al., 2022; Hansen et al., 2022), which may keep them from engaging in the digital transformation of education (Blume, 2020). In a study by Skaalvik and Skaalvik (2015), teachers primarily felt stressed due to workload and time pressures. This is followed by their need to adapt their teaching to the different needs of children. As insufficient time was found to be a barrier to integrate student-centered ICT use (Tondeur et al., 2017), high work-related effort could inhibit intentions to use DLPiP. Since changes, like using DLPiP, are accompanied by necessary resource requirements (e.g., time) (Hobfoll, 2001), we assumed that work-related effort (expressed in terms of time and resource scarcity) is negatively associated with teachers’ intention to use DLPiP.
To summarize, DLPiP utilization remains low among primary school teachers, despite its potential to enhance student learning, and research on teachers’ intention to use DLPiP is sparse. The UTAUT model comprises a well-established and parsimonious set of factors that explain the usage intentions and actual usage. However, it is not known whether the UTAUT is also useful in explaining intentions to use DLPiP. The importance of employing shared theoretical frameworks for advancing the understanding of the acceptance of educational technology was highlighted by Hew et al. (2019). Extensions to established models, e.g., by taking context-specific factors into account, can reveal shortcomings in the existing theory and help to identify necessary changes to the theory. However, potential extension of a theory should adhere to the principle of parsimony and be justified by predictive success (Borsboom et al., 2021).
1.4 Research questions and hypotheses
The two main research questions were: (1) Can a UTAUT model – encompassing performance expectancy, effort expectancy, social influence and facilitating conditions as predictors (c.f. Figure 1) – predict primary school teachers’ intention to use DLPiP to a meaningful extent? We expected the UTAUT model to explain at least 20% of the variance in teachers’ intention to use, based on meta-analysis findings (Scherer and Teo, 2019). (2) Can a UTAUT model – extended by context-specific predictors – meaningfully contribute to explaining primary school teachers’ intentions? We defined “meaningful contribution” as an increase in variance prediction of ≥5%, based on discussions with teachers and experts in this research field. This criterion was defined to avoid focusing on a merely statistically significant increase in explanatory power. Instead, to justify a more complex model through a meaningful increase in explanatory power, we considered the principles of theory evaluation, as described in theory construction methodology by Borsboom et al. (2021), especially minding the principle of parsimony.
Pertaining to the two main research questions, we postulated the following hypotheses:
H1: The predictors considered within the UTAUT model would explain a meaningful percentage (≥20%) of the variance in primary school teachers’ intention to use DLPiP.
H2: The UTAUT model – extended using additional context-specific predictors – would explain an additional meaningful percentage of variance (≥5%) in teachers’ intention.
2 Materials and methods
2.1 Study pre-registration
This study is part of shared research project, the present part considering technology acceptance and the other focusing on data acceptance among primary school teachers (Hase et al., 2022). This study was pre-registered prior to accessing the data via the open science framework (OSF). Furthermore, the study was approved by the Ethics Committee of the Leuphana University Lüneburg and by the respective education offices of each involved federal state.
An a priori sample size calculation revealed that n = 266 primary school teachers were needed to test the two hypotheses. This sample size calculation considered effect sizes reported in prior research performed to define realistic effects, expert consultations to specify meaningful effects, and the number of hypotheses to be tested to achieve a family-wise alpha error of 5% and 80% power to identify existing associations. More details are provided within the pre-registration.
2.2 Participants
After the ministries of education in each northern federal state (Bremen, Hamburg, Lower Saxony, Mecklenburg-Western Pomerania, and Schleswig Holstein) provided permission, we contacted all 2,684 public primary schools in northern Germany by e-mail between October and December 2021. The schools received precise information on the study and a flyer which summarized all necessary information and contained a QR-Code which led to the online survey. The flyer was designed to be printed out. We also recruited teachers via social media (Instagram) and commissioned a panel partner for teacher recruitment. In total, N = 439 teachers finished the questionnaire, with N = 167 excluded because they did not work in primary schools, resulting in a sample of N = 272 primary school teachers (86% female), exceeding the necessary sample size of n = 266. With 28% each, the age groups 30–39 and 40–49 years old were the largest age groups. Most participating teachers had ≥10 years teaching experience. These distributions are comparable to teacher demographics currently reported in Germany by the Federal Statistical Office of Germany (2022). Table 1 summarizes the teacher sample.
2.3 Survey instrument
An online survey was conducted via LimeSurvey. The survey consisted of an instructional and informative video and 55 items to assess teachers’ level of technology acceptance. Completing the survey took roughly 20 min.
2.3.1 Explanatory video
To start, participants watched a 3-min video produced by the authors which explained DLPiP (see Supplementary Figure A). The video was mandatory to watch to ensure that teachers related survey questions to the same type of technology and specific purpose of individualized practice. Afterward, teachers were surveyed on their acceptance of DLPiP.
2.3.2 Questionnaires
All constructs, including an example item, item scales, Cronbach’s alpha, and scale source are summarized in Table 2. Participants were asked about their intention to use DLPiP within the current school semester using three items extracted from Venkatesh et al. (2003). Performance expectancy, measured using six items extracted from Venkatesh et al. (2003), assessed teachers’ beliefs concerning the benefits of DLPiP for their students and lesson planning. Effort expectancy, measured via four items extracted from Venkatesh et al. (2003), assessed teachers’ perceived effort using DLPiP relative to not using it. Following Sugar et al. (2004), teachers experience social influence via principals, colleagues, parents, and students. Therefore, in four items teachers were asked to indicate which expectations each of these groups had of them to use DLPiP. Facilitating conditions, measured adopting three items from Venkatesh et al. (2003), evaluated the presence of all resources necessary for DLPiP use—technical infrastructure, knowledge, and support. Based on Schweizer and Horn (2014), we measured self-efficacy using DLPiP. Teachers’ attitude toward employing digital media for educational purposes was assessed with a scale of eight items based on Venkatesh et al. (2003), Petko et al. (2018), and Schaumburg and Prasse (2019). The differentiation of educational technologies introduced by Schmid et al. (2017) were used to assess teachers’ previous use of educational technology. Teachers indicated which types of technology they had already used for educational purposes. The total number of educational technology types used was then calculated.
To assess teaching styles related to individualized practice, we measured three central components: Practice was measured based on Jaeger and Helmke (2008) and Baumert et al. (2009). Individualization was assessed using a scale published by the Institute for Quality Development Hessen (2012), as its items were suitable for the German educational system. To assess feedback, we used a scale from the Program for International Student Assessment (PISA, 2017), while items from the effort-reward-imbalance scale (Rödel et al., 2004) measured teachers’ work-related effort. All scale characteristics, including examples, are summarized in Table 2.
2.4 Data analysis
2.4.1 Missing data
For data analysis, R-Studio was used. Data were first screened for missing data, which comprised 2.7% of the dataset. Non-response was defined as either participants skipping an answer or selecting the response option “I cannot tell.” Data were both missing completely at random (MCAR) and missing at random (MAR) as indicated by Little’s test for MCAR (Little, 1988). Missing data were replaced by multiple imputations in R-Studio, generating 10 datasets with imputed data using the “mice package” and “predictive mean matching” with non-normal data distribution (van Buuren, 2018). Non-normality of the data was detected using the Henze-Zirkler and Anderson-Darling test (“MVN package,” Korkmaz et al., 2021). For all statistical tests, we reported pooled results from the 10 imputed data sets.
2.4.2 Reliability analysis
Internal consistency of the 11 constructs was investigated by calculating Cronbach’s alpha. All but one scale exceeded α > 0.70, which indicates acceptable internal consistency (Hulin et al., 2001). The construct facilitating conditions had a Cronbach’s alpha <0.60, suggesting that its items should not be summed into one scale. Therefore, the three components of facilitating conditions – technical infrastructure, knowledge, and support – were considered separately. Furthermore, as reliability could be improved for certain scales, certain items from these scales were dropped. For example, item four for performance expectancy was the only item to address the perception of usefulness for a teacher to more efficiently plan out lessons. The other five items addressed the usefulness of technology for students. Reliability measures and final numbers of items per scale are listed in Table 2.
2.4.3 Multiple linear regression analysis
Following the prespecified study protocol, we tested the first hypothesis on the explanatory power of the standard UTAUT model on primary school teachers’ intention to use DLPiP by performing multiple linear regression analyses in R-Studio, using the “lm” function from the mice package (van Buuren, 2023). Normally distributed residuals were a prerequisite for multiple linear regression analyses, and inspecting histograms and pp-plots of all residuals for the UTAUT and context-specific predictors within complete datasets (N = 149) revealed normal distributions.
To test the second hypotheses on whether an UTAUT model extended by adding context-specific predictors would meaningfully improve its explanatory power, we performed further multiple linear regression analysis. To test whether additional context-specific predictors meaningfully increased the explained variance on intention (≥5%), analysis of variance (ANOVA) was used to determine whether the two models were significantly different.
Bivariate correlations of all UTAUT variables and additional context-specific factors were calculated using either Pearson or Spearman correlation coefficients, depending on whether variables were continuous or ordinal.
2.5 Deviations from pre-registration
Based on findings regarding the low rates of DLPiP use in Germany described in the introduction, a bottom effect was expected for actual use, suggesting that this was not an appropriate outcome. After data collection, an unexpectedly high rate of teachers actually using DLPiP became evident. Therefore, three additional analyses were conducted. First, we considered DLPiP use an additional outcome and conducted additional bivariate correlation analyses, particularly to identify the strength of the intention-behavior association. Second, to account for the associations of facilitating conditions, we performed binary logistic regression analysis. Third, since the additional outcome variable suggested that we consider a structural equation model (SEM) to account for both outcome variables, we chose to test a SEM in R-studio using the lavaan package for sensitivity analysis.
3 Results
3.1 Description of teachers’ intention and potential predictors
Most teachers reported having the intention to either use or continue to use DLPiP during the current school semester, indicated by a score of M = 3.31 (SD = 0.82) for intention. Teachers generally agreed on the predictors performance expectancy, social influence, and knowledge [M = 3.43 (SD = 0.46), M = 2.58 (SD = 0.61), M = 3.12 (SD = 0.72), respectively]. For effort expectancy, technical infrastructure, and support, the teachers’ average answers were below the scale midpoint of 2.50 [M = 2.39 (SD = 0.70), M = 2.43 (SD = 0.98), M = 2.42 (SD = 1.02)] indicating that teachers anticipated expending moderate effort for DLPiP use, but generally did not have access to the necessary technical infrastructure or technical support. Pertaining to context-specific predictors, teachers averaged strongly agreeing to each scale, indicated by mean measures above M = 3.00. Therefore, the teachers, on average, reported high self-efficacy [M = 3.28 (SD = 0.56)], a positive attitude toward digital media use in education [M = 3.05 (SD = 0.55)], regular use of practice [M = 3.21 (SD = 0.49)], individualization [M = 3.43 (SD = 0.56)] and feedback [M = 3.53 (SD = 0.49)] within their teaching, and expending high work-related effort [M = 3.20 (SD = 0.55)].
The top three digital technologies used by the teachers were applications/platforms for learning, learning videos, and Microsoft Office products. On average, the teachers used five different types of technology for educational purposes.
Additionally, 172 teachers (63%) indicated to use DLPiP by the time they took the survey. Furthermore, 36% of the teachers claimed to have used DLPiP before the pandemic, while 70% used DLPiP during the pandemic (home-schooling). The percentage of teachers who used DLPiP declined when face-to-face education was possible again (63%). Yet, there was a stable increase of 27% in DLPiP use after teaching face-to-face resumed.
Table 3 lists the mean and standard deviation for each construct and bivariate correlations between all constructs, while considering actual use.
3.2 Predicting teachers’ intention
To prepare for the main analysis, we investigated bivariate correlations of all constructs considered. They showed no signs of multicollinearity as no correlation coefficient was >0.80 (Field, 2018). Bivariate correlations are summarized in Table 3.
All UTAUT variables, except support, correlated significantly with low-to-moderate strength (Cohen, 1992) with the intention to use DLPiP. From the context-specific predictors, the number of previously used educational technologies correlated strongest with intention. This factor correlated to a lesser extent with other UTAUT and context-specific predictors, indicating little conceptual overlap and good discriminant validity. Attitude toward digital media use in education correlated moderately with intentions. However, from a discriminant validity perspective, attitude correlated even more strongly with two other UTAUT variables: effort expectancy and knowledge. A similar pattern was observed for self-efficacy, with moderate correlations with intentions and stronger correlations with the UTAUT variables effort expectancy and knowledge. Significant correlations also were observed between intention and both individualization and feedback. The use of practice in teaching and work-related effort did not correlate significantly with intention to use DLPiP.
3.2.1 Standard UTAUT model
Regarding the first hypothesis, the multiple linear regression model considering the standard UTAUT predictors explained R2 = 21% of the variance in intention to use DLPiP, with F(6, 2,050.83) = 12.568, p < 0.001. This met the expected amount of explained variance; consequently, hypothesis 1 is accepted. The results of multiple linear regression analysis for the standard UTAUT model are summarized in Table 4.
Standardized regression coefficients revealed performance expectancy having the strongest association with intention to use. Likewise, effort expectancy exhibited a significant association with intention to use, indicating that the more effort teachers perceive toward the usage of DLPiP, the more unlikely they have the intention to use them. Availability of the necessary technical infrastructure appeared especially relevant to explaining intention, indicating that the intention to use DLPiP is more likely to be formed if the technological infrastructure, internet, and/or software licenses are available. Though social influence and knowledge correlated significantly with intention during bivariate analysis, no significant regression coefficients for these factors were evident in the regression model. Support consistently exhibited no significant association with intention, either in the regression model or during bivariate analyses.
3.2.2 Extended UTAUT model
The extended UTAUT model explained 23% of the variance in the intention to use DLPiP, with F(13, 3,042.33) = 7.082, p < 0.001. Therefore, hypothesis 2 was rejected since the difference in R2 from the first model (ΔR2) was <5%. Using the Wald test, the extended UTAUT model did not significantly differ from the standard UTAUT model with F(7, 255.8318) = 1.999, p = 0.056. Results for the extended UTAUT model are summarized in Table 4. Results from the rejected second regression model were consistent with the important roles found for the two UTAUT variables performance expectancy and infrastructure, and partially consistent with the results for effort expectancy. Previous usage behavior was a significant predictor in the rejected model and, thereby, confirmed high associations found during bivariate correlation analysis. Attitude and self-efficacy exhibited no significant associations with intention.
3.2.3 Overall sensitivity analysis and exploratory prediction of actual use
Following modifications to the study protocol, we considered actual DLPiP use a second outcome variable (usage/non-usage). During bivariate correlation analysis, intention to use correlated strongly with actual use (r = 0.53***). All three aspects of facilitating conditions – infrastructure, knowledge, and support – correlated significantly with actual use when investigated separately.
From the results of binary logistic regression analysis (Supplementary Table A), intention, knowledge, and support appeared especially relevant to explaining actual use, as all were significantly associated with it. Within the context of all variables, technical infrastructure failed to exhibit any significant associations with actual use.
Finally, we were able to replicate the relative importance of each predictor explaining intention to use and actual use, with significant associations identified during multiple regression and binary logistic regression analysis performed utilizing a SEM (Supplementary Figure B and Supplementary Table B).
4 Discussion
The present study considered three novel aspects in teacher technology acceptance research: First, the investigation of primary school teachers’ intention to use digital learning platforms for individualized practice (DLPiP). Second, the utilization of a video as a method to ensure a shared understanding of the target technology and its usage purpose. And third, the comparison of an established theoretical model to a model extended by context-specific predictors. Results showed that a small set of predictors specified using a UTAUT model (Venkatesh et al., 2003, 2012) predicted teachers’ intention to use DLPiP. Noteworthy is that the UTAUT model, extended to more than twice as many predictors, failed to meaningfully add to the explained variance in intention. This suggests that understanding teachers’ intentions to use digital learning platforms can focus on only six UTAUT derived predictors without losing accuracy.
The UTAUT thereby explains teachers’ intention to use digital learning platforms significantly and meaningfully. This was true regardless of the analytic approach employed. However, the variance explained during regression analyses using the six UTAUT predictors was less than in previous studies with a comparable research focus (Pynoo et al., 2011; Abu-Shanab, 2014; Balkaya and Akkucuk, 2021; Dindar et al., 2021). Differences might be explained by methodological and conceptual factors. Since the present study was the first to focus on the functionality of digital learning platforms for individualized student practice, differences might be explained by different usage purposes (De Smet et al., 2012). In one systematic review, previous studies on the acceptance of technology which could be used for individualized practice mostly failed to clarify the actual usage purpose of the focused technology (Kahnbach and Lehr, 2023). As the importance of predictors to explain technology acceptance may depend on usage purpose (De Smet et al., 2012; Mavroudi et al., 2021), its clarification may contribute to the comparability of results between studies. To ensure our teachers had the same understanding of the technology and underline the reasons for using DLPiP, we provided an informational video for them to watch before starting the online survey.
Moreover, comparing the amount of variance explained might be misleading as other studies either employed a different theoretical framework (e.g., TAM) (Abu-Shanab, 2014) or did not strictly adhere to the predictors specified by the UTAUT (Dindar et al., 2021). Finally, it must be considered that analysis employing latent variables generally leads to greater explained variance than using manifest variables (Black et al., 2013).
Beyond testing the overall model, our results suggest different levels of importance of the different UTAUT predictors. Although some predictors in the multivariate model have more explanatory power than others, it would be premature to conclude that the other factors are irrelevant. Nonetheless, all predictors of the UTAUT model showed a significant bivariate correlation with the usage intention.
Consistent with earlier research (Pynoo et al., 2011; Abu-Shanab, 2014; Dindar et al., 2021), the most relevant predictor we found for intention was performance expectancy. Hence, teachers are more likely to intend to use DLPiP if they perceive that using it during lessons may be beneficial for their students. It seems that high performance expectancy is generally of importance in increasing intentions to use digital tools in education (Scherer and Teo, 2019). Still, as significant associations in regression models just point out the “loudest in the orchestra,” it is important to underline that the other predictors are as well relevant to explain intention.
Furthermore, following UTAUT assumptions, effort expectancy was significantly linked to intention. This is interesting, since previous studies did not show significant associations between effort expectancy and the intention to use digital technology (Pynoo et al., 2011; Balkaya and Akkucuk, 2021; Dindar et al., 2021). One potential explanation might be found within research on goal setting, highlighting the difference between generally- and specifically defined goals (Schunk, 1990). A globally defined and inherently positive goal like using digital technologies in education might lead to general approach tendencies without reflection on concrete obstacles (perceived effort). Conversely, specifying a concrete purpose (individualized practice) might stimulate thinking about the effort needed. Likewise, since the present study used a salient method, a video clip, to specify the purpose of DLPiP, the association between effort expectancy and intention might have become stronger.
Although the UTAUT assumes that facilitating conditions are a single characteristic and other studies measured facilitating conditions with a single scale (Pynoo et al., 2011), our results suggest that facilitating conditions have three independent components: technical infrastructure availability; perceived knowledge on how to use DLPiP; and the availability of technical assistance support. However, the lack of homogeneity might be an artificial effect of item selection in this study and replication studies on convergent and discriminant validity are needed to confirm our results. Nevertheless, from a practical perspective, such differentiation seems reasonable, as different interventions targeting either equipment, human resources, or occupational education are needed to improve each aspect of facilitating conditions.
Interestingly, we found that the availability of necessary technical infrastructure was particularly important explaining intentions, while other aspects were not. The relevance of a sufficient technical infrastructure for teachers’ willingness and ability to use digital tools in education is also supported by findings from a qualitative study by Wohlfart et al. (2021). Furthermore, only half of the participating teachers claimed they had the necessary infrastructure to utilize DLPiP. Technical infrastructure could thus be considered a “basic need” for teachers to develop an intention to use digital technology for individualized practice, rather than a “facilitating” condition for intention building, like the availability of support. This might explain our results revealing a stronger impact of technical infrastructure than support and knowledge on intention. However, as schools’ equipment is likely to progress in upcoming years, the availability of required technical infrastructure might not play as crucial a role in the future. Still, at this point in time, it goes in line with what has been brought to light when the COVID-19 pandemic hit that infrastructure and skill development have been neglected (Blume, 2020). As the availability of the necessary technical infrastructure partially explains (continuous) usage intention it may reflect one of the reasons for the lack of educational technology use during the pandemic.
One alternative explanation why support availability did not significantly correlate with intention could be our sample’s comparably high rates of prior experience with DLPiP. Significant associations between support and intention have already been reported for less-experienced samples (Dindar et al., 2021) or have become relevant when inexperienced samples start to actually use a new system (Pynoo et al., 2011). Therefore, support availability might be especially important in the early phases of intention building. Nevertheless, the small but significant association we observed with actual use might indicate a positive role maintaining such behavior.
Similarly, knowledge seems more important for maintaining DLPiP use than for developing an intention. This might be explained by the significant overlap with effort expectancy, a pattern also identified by Venkatesh et al. (2003).
4.1 Context-specific predictors
As prior literature highlights the influence of context-specific predictors, like self-efficacy (Balkaya and Akkucuk, 2021; Dindar et al., 2021), attitude (Scherer and Teo, 2019; Buerger et al., 2021), prior experience with educational technologies (De Smet et al., 2012), teaching style in terms practice, feedback, and individualization (Bloemeke et al., 2006; Gellerstedt et al., 2018) and work-related effort (Tondeur et al., 2017) on teachers’ technology acceptance, we considered seven additional predictors. Surprisingly, however, this comprehensive set of additional predictors failed to improve the predictive power of the standard UTAUT model. To the best of our knowledge, this was the first time the standard UTAUT model was tested in a preregistered and adequately powered study against an extended model predicting teachers’ intentions to use digital applications. Taking the principle of parsimony seriously (Borsboom et al., 2021), the standard UTAUT model should now be preferred, challenging current trends to take more and more factors into account.
Nevertheless, it seems worthwhile to explore in more detail the impact of previous usage behavior with other educational technology on future intentions for DLPiP. On average, our teachers reported prior experience with five other digital applications, supporting the dictum that “past behavior is the best predictor of future behavior.” Perhaps past behavior does not substantially overlap with all other predictors and might contribute something unique. Overall, our findings underline the UTAUT model’s robustness and challenge the practice of extending measurement models with context-specific factors.
Within our sample, the average intention to use DLPiP was high, with most teachers already using it regularly. This was unexpected, given the low usage reported for several prior studies (Eickelmann et al., 2019; Forsa Politik- und Sozialforschung GmbH, 2020; Fraillon et al., 2020). This implies that our teachers were more technology-savvy than average. Also, that most of our teachers reported their intention to either start or continue to use DLPiP indicates motivation and openness for technology usage in education. Furthermore, since increased experience using educational technology could result in different predictors becoming important for continuous intention (De Smet et al., 2012; Dindar et al., 2021), the knowledge gained from this study regarding teachers’ intention to use DLPiP may especially contribute to maintaining intention.
Also noteworthy is that we identified no association between work-related effort (e.g., constant time pressure) and the intention to use DLPiP with any of our analysis approaches. Considering that learning to apply DLPiP requires an investment of resources (Hobfoll, 2001), especially time, and that teaching is often considered a high-stress occupation (Agyapong et al., 2022), this finding was surprising. However, work-related stressors may be more important in the initial phase of intention building, and most of the teachers in the present sample had already started using DLPiP.
4.2 Strengths and limitations
Among the present study’s many strengths is that its design, hypotheses, and planned analyses were pre-registered to avoid HARKing “Hypothesizing After the Results are Known” (Kerr, 1998). Specific efforts also were made to ensure participants had a concrete understanding of the purpose regarding the use of the technology under investigation (the respective video’s script is available within the Supplementary Figure A). For the first time in teachers’ technology acceptance research, we tested if an extended version of the UTAUT is justified by a meaningful increase in explained variance or if parsimony is preferable. Ultimately, the results narrowed 13 potential predictors down to six, thereby confirming the UTAUT’s validity. Finally, different analytical approaches were employed, particularly regression analysis and structural equation modeling, to ensure our results are independent of a specific analytic approach.
Despite these strengths, several limitations must be acknowledged. First, our sample was considerably more technology-savvy than average primary school teachers in Germany (Eickelmann et al., 2019; Forsa Politik- und Sozialforschung GmbH, 2020), possibly because some participants were recruited through social media and the survey was online. The survey being conducted in an online format and the digital research topic itself may have caused a selection effect leading to an over-representation of DLPiP users in the present study as compared to low rates in the general teacher population. This casts doubt upon the generalizability of our findings. That said, our sample’s demographics (age, gender, and job experience) were similar to the German primary teacher population. Hence, our findings might better describe factors of relevance to teachers maintaining, rather than initiating, intentions to use DLPiP.
Second, we were unable to report a response rate for study participation. Although all primary schools in northern Germany were contacted, we have no information if and how the invitations for study participation were distributed within the schools. Contacting teachers personally was infeasible due to the schools’ data safety regulations, especially as recruitment took place from October to December 2021, amidst the second wave of the COVID-19 pandemic. Third, although an extended UTAUT model did not contribute to better understanding of teachers’ intentions to use DLPiP, other results might be observed when different digital applications or purposes are investigated. Fourth, our findings regarding the predictors of intentions and use are a snapshot in time and the data observational in nature. Prospective trials are needed to draw causal conclusions. Nevertheless, our study did identify potential focal points for future research. The present findings apply to situations where teachers have the option to use or not use educational technology voluntarily, but in situations where teachers are obliged to use technology, other factors may be more important for technology acceptance.
4.3 Implications
Several implications for future research and policy-making arise from this study. To make systematic progress understanding teachers’ technology acceptance, it seems essential to adhere to theoretical frameworks since, otherwise, results from multivariate analyses may be incomparable across studies. Changes or extensions to theoretical frameworks like the UTAUT should be justified empirically. If changes are made, reporting results for the original model also will ensure cross-study comparability.
A clearer description of the usage purpose would further contribute to cross-study comparability. This is especially important as the many different functionalities of educational technology could address several usage purposes. Adequately powered structural equation modeling is needed to investigate all associations within complex technology acceptance models in one overall analysis.
More in-depth investigations into structural and temporal issues of facilitating conditions are worthwhile. Separating structural and individual characteristics might be advantageous, especially for deriving and justifying subsequent measures (e.g., investments at a system level or addressing individual teacher’s knowledge). Moreover, whether providing infrastructure functions as a sine-qua-non condition for intention development or if the different aspects of facilitating conditions enfold their impact differently over time (intention building, starting use, and maintaining use) requires further investigation. Establishing temporal sequences between infrastructure provision and intention building is especially important for policy-makers. Equipping only those with digital devices and existing intentions is a diametrically different strategy than ensuring that the “basic need” of an available device is met and observing whether intentions develop.
The strong association between intention and actual use strengthens the notion that intentions are an appropriate proxy for usage. Nevertheless, that this association is far from complete indicates that intentions are not always translated into behavior, a phenomenon called the “intention-behavior-gap” (Sheeran and Webb, 2016). A promising approach for future research is to investigate the impact of interventions like implementation intentions (Gollwitzer, 1999) and coping plans (Schwarzer, 2016) to reduce this gap.
It also seems worthwhile to consider the stage of technology use (intention) to a greater extent as predictors might impact intention and actual use differently depending on teachers’ level of intention building or usage stage. Here, stage models like the Transtheoretical Approach (TTA, Prochaska and DiClemente, 2005) and Stages of Concern (SoC, Hall and Hord, 2011) might be considered. The SoC represents, in detail, the process of forming an intention, while the TTA highlights the processes necessary to undergo change at different levels from intention forming to maintaining behavior.
Our findings may also be considered for updated regulations and reforms concerning the digitalization strategy in German (primary) education. First, implementation strategies of DLPiP should not rely on equipping schools with DLPiP only, but find ways to address performance and effort expectancy as well. Secondly, primary school teachers who regularly use DLPiP should be considered as a resource for large-scale implementation of DLPiP and be part of the digitalization strategy in primary schools, e.g., by establishing regulations that enable teachers using DLPiP to serve as role models and multipliers.
Still, beyond observations research, experimental and interventional studies are needed to establish causality regarding the impacts of, for example, performance and effort expectancy. Targeting identified predictors employing a variety of acceptance-facilitating interventions, like testimonials from other teachers or experts (Apolinário-Hagen et al., 2021) should be evaluated to develop evidence-based strategies for implementing educational technology in schools. Teachers’ pre-employment and on-the-job education provide opportunities to evaluate and apply acceptance-facilitating interventions. Considering the important role of prior experience with technology, teachers should receive training on the appropriate and effective integration of DLPiP to enhance their acceptance and persistent use (Walsh and Farren, 2018; Moreno, 2022). Providing information on the benefits of technology use and permitting teachers-in-training to observe classes already using such technology might increase performance expectancies and should be evaluated as acceptance-facilitating interventions.
Lastly, it appears worthwhile to investigate if the regression model is also valid for explaining the intention to use DLPiP in other contexts, e.g., secondary school, including the investigation of potential differences regarding the impact of the various predictors, e.g., effort expectancy.
5 Conclusion
Adopting the UTAUT model to explain German primary school teachers’ intention to use digital learning platforms for individualized practice, we identified evidence supporting the importance of performance expectancy, effort expectancy, and technical infrastructure. Additional predictors considered important to explaining primary school teachers’ technology acceptance – especially teaching-style, attitude, and self-efficacy – failed to additionally explain variance in DLPiP-use intentions. Our study underlines the robustness of the UTAUT model explaining primary school teachers’ intention to use technology and argues for adhering to the principle of parsimony before extending research models.
Overall, it seems beneficial to communicate the added value of DLPiP for teaching and student learning during teacher training to enhance teachers’ performance expectancy, as well as to provide opportunities for them to try DLPiP to reduce effort expectancy. Additionally, it is important that teachers are sufficiently equipped with the necessary technical infrastructure and to consider teacher’s different stages of intention – from initially forming intentions through maintaining usage, as this may result in different importance levels for different predictors of technology acceptance.
Data availability statement
The raw data supporting the conclusions of this article will be made available by the authors, without undue reservation.
Ethics statement
The studies involving humans were approved by the Ethics Committee of the Leuphana University Lüneburg. The studies were conducted in accordance with the local legislation and institutional requirements. The participants provided their written informed consent to participate in this study.
Author contributions
LK: Conceptualization, Data curation, Formal analysis, Investigation, Methodology, Writing – original draft, Writing – review & editing. AH: Conceptualization, Data curation, Investigation, Methodology, Writing – review & editing. PK: Conceptualization, Methodology, Supervision, Writing – review & editing. DL: Conceptualization, Methodology, Supervision, Writing – review & editing.
Funding
The author(s) declare financial support was received for the research, authorship, and/or publication of this article. This study developed within the CODIP project. CODIP was funded by the Quality Initiative Teacher Training (Qualitätsoffensive Lehrerbildung), a joint initiative of Federal Government and the German states. The financial means were provided by the Federal Ministry of Education and Research (BMBF) (Support code: 01JA2002). This publication was funded by the German Research Foundation (DFG).
Acknowledgments
We thank our development team teachers for their help in revising the questionnaire and our student assistants for supporting the data evaluation.
Conflict of interest
The authors declare that the research was conducted in the absence of any commercial or financial relationships that could be construed as a potential conflict of interest.
Publisher’s note
All claims expressed in this article are solely those of the authors and do not necessarily represent those of their affiliated organizations, or those of the publisher, the editors and the reviewers. Any product that may be evaluated in this article, or claim that may be made by its manufacturer, is not guaranteed or endorsed by the publisher.
Supplementary material
The Supplementary Material for this article can be found online at: https://www.frontiersin.org/articles/10.3389/feduc.2024.1353020/full#supplementary-material
References
Abu-Shanab, E. (2014). E-learning system’s acceptance. Int. J. Web Based Learn. Teach. Technol. 9, 1–13. doi: 10.4018/ijwltt.2014100101
Agyapong, B., Obuobi-Donkor, G., Burback, L., and Wei, Y. (2022). Stress, burnout, anxiety and depression among teachers: A scoping review. Int. J. Environ. Res. Public Health 19:17. doi: 10.3390/ijerph191710706
Ajzen, I. (1991). The theory of planned behavior. Organ. Behav. Hum. Dec. Process. 50, 179–211. doi: 10.1016/0749-5978(91)90020-T
Altan, B. A., Yorulmaz, A., and Karalar, H. (2024). Modelling primary school teachers’ acceptance of distance-based educational technologies: A post-pandemic perspective. Educ. Inf. Technol. doi: 10.1007/s10639-024-12509-1
Apolinário-Hagen, J., Fritsche, L., Wopperer, J., Wals, F., Harrer, M., Lehr, D., et al. (2021). Investigating the persuasive effects of testimonials on the acceptance of digital stress management trainings among university students and underlying mechanisms: A randomized controlled trial. Front. Psychol. 12:738950. doi: 10.3389/fpsyg.2021.738950
Balkaya, S., and Akkucuk, U. (2021). Adoption and use of learning management systems in education: The role of playfulness and self-management. Sustainability 13:1127. doi: 10.3390/su13031127
Bandura, A. (1986). Social foundations of thought and action: A social cognitive theory. Englewood Cliffs, NJ: Prentice Hall.
Baumert, J., Blum, W., Brunner, M., Dubberke, T., Jordan, A., Klusmann, U., et al. (2009). Professionswissen von Lehrkräften, kognitiv aktivierender Mathematikunterricht und die Entwicklung von mathematischer kompetenz (COACTIV): Dokumentation der Erhebungsinstrumente [Teachers’ professional knowledge, cognitively activating mathematics instruction, and the development of mathematical competence (COACTIV): Documentation of survey instruments]. Berlin: Max-Planck-Institut für Bildungsforschung.
Black, W. C., Babin, B. J., and Anderson, R. E. (2013). Multivariate data analysis. London: Pearson Education.
Bloemeke, S., Mueller, C., and Eichler, D. (2006). Unterricht mit digitalen medien – zwischen innovation und tradition? [Teaching with digital media – between innovation and tradition?]. Z. Erziehungswiss. 9, 632–650. doi: 10.1007/s11618-006-0172-3
Blume, C. (2020). German teachers’ digital habitus and their pandemic pedagogy. Post. Sci. Educ. 2, 879–905. doi: 10.1007/s42438-020-00174-9
Blut, M., Chong, A. Y. L., Tsigna, Z., and Venkatesh, V. (2022). Meta-analysis of the unified theory of acceptance and use of technology (UTAUT): Challenging its validity and charting a research agenda in the red ocean. J. Associ. Inf. Syst. 23, 13–95. doi: 10.17705/1jais.00719
Borsboom, D., van der Maas, H. L. J., Dalege, J., Kievit, R. A., and Haig, B. D. (2021). Theory construction methodology: A practical framework for building theories in psychology. Perspect. Psychol. Sci. 16, 756–766. doi: 10.1177/1745691620969647
Buerger, N., Haselmann, S., Baumgart, J., Prinz, G., Girnat, B., Meisert, A., et al. (2021). Jenseits von professionswissen. Eine systematische überblicksarbeit zu einstellungs– und motivationsbezogenen einflussfaktoren auf die nutzung digitaler technologien im unterricht [beyond professional knowledge: A systematic literature review on attitude- and motivation-related factors influencing the use of digital technology in teaching]. Z. Erziehungswiss. 24, 1087–1112. doi: 10.1007/s11618-021-01050-3
Chauhan, S. (2017). A meta-analysis of the impact of technology on learning effectiveness of elementary students. Comput. Educ. 105, 14–30. doi: 10.1016/j.compedu.2016.11.005
Chun, T. W., and Yunus, M. M. (2023). Factors affecting Malaysian ESL teachers’ behavioral intentions for technology use in the post-COVID-19 era. Front. Psychol. 14:1127272. doi: 10.3389/fpsyg.2023.1127272
Davis, F. D., Bagozzi, R. P., and Warshaw, P. R. (1989). User acceptance of computer technology. A comparison of two theoretical models. Manag. Sci. 35, 982–1003.
De Smet, C., Bourgonjon, J., Wever, B., de, Schellens, T., and Valcke, M. (2012). Researching instructional use and the technology acceptation of learning management systems by secondary school teachers. Comput. Educ. 58, 688–696. doi: 10.1016/j.compedu.2011.09.013
Dietrich, J., Greiner, F., Weber-Liel, D., Berweger, B., Kämpfe, N., and Kracke, B. (2021). Does an individualized learning design improve university student online learning? A randomized field experiment. Comput. Hum. Behav. 122:106819. doi: 10.1016/j.chb.2021.106819
Dindar, M., Suorsa, A., Hermes, J., Karppinen, P., and Näykki, P. (2021). Comparing technology acceptance of K-12 teachers with and without prior experience of learning management systems: A Covid-19 pandemic study. J. Comput. Assist. Learn. 37, 1553–1565. doi: 10.1111/jcal.12552
Eickelmann, B. (2011). Supportive and hindering factors to a sustainable implementation of ICT in schools. J. Educ. Res. 1, 75–103.
Eickelmann, B., Bos, W., Gerick, J., Goldhammer, F., Schaumburg, H., Schwippert, K., et al. (2019). ICILS 2018 #Deutschland: Computer– und informationsbezogene kompetenzen von schülerinnen und schülern im zweiten internationalen vergleich und kompetenzen im bereich computational thinking [ICILS 2018 # Germany: Computer and information-related competencies of students in the second international comparison and competencies in the area of computational thinking]. Münster: Waxmann.
Federal Statistical Office of Germany (2022). Allgemeinbildende schulen: Fachserie 11 Reihe 1 – Schuljahr 2020/2021 [General education schools: Subject matter series 11 series 1 – school year 2020/2021]. Wiesbaden: Federal Statistical Office of Germany.
Field, A. (2018). Discovering statistics using IBM SPSS statistics, 5th Edn. New York, NY: SAGE Publications.
Fishbein, M., and Ajzen, I. (1975). Belief, attitude, intention and behaviour: An introduction to theory and research. Reading, MA: Addison-Wesley.
Forsa Politik- und Sozialforschung GmbH (2020). Das Deutsche schulbarometer spezial corona-Krise: Ergebnisse einer befragung von lehrerinnen und lehrern an allgemeinbildenden schulen [the german school barometer special corona crisis: Results of a survey of teachers at general education schools]. On behalf of robert bosch foundation in cooperation with ZEIT. Available online at: https://deutsches-schulportal.de/deutsches1-schulbarometer/downloads/Deutsches_Schulbarometer_Lehrkraeftebefragung_Dezember_2020_Final-1.pdf (accessed September 23, 2023).
Fraillon, J., Ainley, J., Schulz, W., Friedman, T., and Duckworth, D. (2020). Preparing for life in a digital world. Cham: Springer International Publishing.
Fraillon, J., Ainley, J., Schulz, W., Friedman, T., and Gebhardt, E. (2014). Preparing for life in a digital age. Cham: Springer International Publishing.
Fülöp, M. T., Breaz, T. O., Topor, I. D., Ionescu, C. A., and Dragolea, L.-L. (2023). Challenges and perceptions of e-learning for educational sustainability in the “new normality era”. Front. Psychol. 14:1104633. doi: 10.3389/fpsyg.2023.1104633
Gellerstedt, M., Babaheidari, S. M., and Svensson, L. (2018). A first step towards a model for teachers’ adoption of ICT pedagogy in schools. Heliyon 4:e00786. doi: 10.1016/j.heliyon.2018.e00786
Ghysels, J., and Haelermans, C. (2018). New evidence on the effect of computerized individualized practice and instruction on language skills. J. Comput. Assist. Learn. 34, 440–449. doi: 10.1111/jcal.12248
Gollwitzer, P. M. (1999). Implementation intentions: Strong effects of simple plans. Am. Psychol. 54, 493–503. doi: 10.1037/0003-066X.54.7.493
Granić, A. (2023). “Technology acceptance and adoption in education,” in Handbook of open, distance and digital education, eds O. Zawacki-Richter and I. Jung (Singapore: Springer Nature Singapore), 183–197. doi: 10.1007/978-981-19-2080-6_11
Grasha, A. F., and Yangarber-Hicks, N. (2000). Integrating teaching styles and learning styles with instructional technology. College Teaching 48:2–10. doi: 10.1080/87567550009596080
Hall, G. E., and Hord, S. M. (2011). Implementing change: Patterns, principles, and potholes, 3rd Edn. London: Pearson.
Hansen, J., Klusmann, U., and Hanewinkel, R. (2022). Emotionale erschöpfung und berufszufriedenheit von lehrpersonal während der COVID-19-pandemie [Emotional exhaustion and job satisfaction among teaching staff during the COVID-19 pandemic]. Bundesgesundheitsblatt Gesundheitsforschung Gesundheitsschutz 65, 776–783. doi: 10.1007/s00103-022-03554-7
Hase, A., Kahnbach, L., Kuhl, P., and Lehr, D. (2022). To use or not to use learning data: A survey study to explain German primary school teachers’ usage of data from digital learning platforms for purposes of individualization. Front. Educ. 7:920498. doi: 10.3389/feduc.2022.920498
Hew, K. F., Lan, M., Tang, Y., Jia, C., and Lo, C. K. (2019). Where is the “theory” within the field of educational technology research? Br. J. Educ. Technol. 50, 956–971. doi: 10.1111/bjet.12770
Hobfoll, S. E. (2001). The influence of culture, community, and the nested-self in the stress process: Advancing conservation of resources theory. Appl. Psychol. 50, 337–421. doi: 10.1111/1464-0597.00062
Holmes, W., Anastopoulou, S., Schaumburg, H., and Mavrikis, M. (2018). Technology-enhanced personalised learning. Untangling the evidence. Stuttgart: Robert Bosch Stiftung GmbH.
Hughes, J., Thomas, R., and Scharber, C. (2006). “Assessing technology integration: The RAT–replacement, amplification, and transformation-framework,” in Proceedings of the society for information technology & teacher education international conference, eds C. Crawford, R. Carlsen, K. McFerrin, J. Price, R. Weber, and D. Willis (Orlando, FL: Association for the Advancement of Computing in Education (AACE)), 1616–1620.
Hulin, C., Netemeyer, R., and Cudeck, R. (2001). Can a reliability coefficient be too high? J. Consumer Psychol. 10, 55–58.
Institute for Quality Development Hessen (2012). Hessischer referenzrahmen schulqualitaet: Dokumentation der fragebogen [Hessian reference framework for school quality: Documentation of the questionnaires]. Hessen: Institute for Quality Development (IQ) Hessen.
Jaeger, R. S., and Helmke, A. (2008). Mathematik-gesamterhebung rheinland-pfalz: Kompetenzen, unterrichtsmerkmale, schulkontext (MARKUS) [Mathematics survey rhineland-palatinate: Competencies, instructional characteristics, school context (MARKUS)], 1st Edn. Berlin: IQB – Institute for Educational Quality Improvement, doi: 10.5159/IQB_MARKUS_v1
Kahnbach, L., and Lehr, D. (2023). “Welche faktoren bedingen die akzeptanz von technologien zum digital-gestützten üben durch lehrkräfte? Eine systematische literaturübersicht [What factors determine the acceptance of technologies for digitally-enhanced practice by teachers? A systematic literature review],” in Digitales lehren und lernen im fachunterricht: Aktuelle entwicklungen, gegenstände und prozesse [Digital teaching and learning in subject teaching: Current developments, objects and processes], eds M. Ahlers, M. Besser, C. Herzog, and P. Kuhl (Weinheim: Beltz Juventa), 133–158.
Kerr, N. L. (1998). HARKing: Hypothesizing after the results are known. Pers. Soc. Psychol. Rev. 2, 196–217. doi: 10.1207/s15327957pspr0203_4
Kerssens, N., and van Dijck, J. (2021). The platformization of primary education in The Netherlands. Learn Media Technol. 46, 250–263. doi: 10.1080/17439884.2021.1876725
Khlaisang, J., Songkram, N., Huang, F., and Teo, T. (2023). Teachers’ perception of the use of mobile technologies with smart applications to enhance students’ thinking skills: A study among primary school teachers in Thailand. Interact. Learn. Environ. 31, 5037–5058. doi: 10.1080/10494820.2021.1993933
Khong, H., Celik, I., Le, T. T. T., van Lai, T. T., Nguyen, A., and Bui, H. (2023). Examining teachers’ behavioural intention for online teaching after COVID-19 pandemic: A large-scale survey. Educ. Inf. Technol. 28, 5999–6026. doi: 10.1007/s10639-022-11417-6
Kopp, B. (2009). Inklusive überzeugung und selbstwirksamkeit im umgang mit heterogenität. Wie denken studierende des lehramts für grundschulen? Empirische Sonderpädagogik. 1:9461.
Korkmaz, S., Göksülük, D., and Zararsız, G. (2021). MVN: An R package for assessing multivariate normality. Available online at: https://cran.r-project.org/web/packages/MVN/vignettes/MVN.html (accessed September 23, 2023).
Kreijns, K., van Acker, F., Vermeulen, M., and van Buuren, H. (2013). What stimulates teachers to integrate ICT in their pedagogical practices? The use of digital learning materials in education. Comput. Hum. Behav. 29, 217–225. doi: 10.1016/j.chb.2012.08.008
Little, R. J. A. (1988). A test of missing completely at random for multivariate data with missing values. J. Am. Stat. Assoc. 83, 1198–1202. doi: 10.1080/01621459.1988.10478722
Major, L., Francis, G. A., and Tsapali, M. (2021). The effectiveness of technology-supported personalised learning in low- and middle-income countries: A meta-analysis. Br. J. Educ. Technol. 52, 1935–1964. doi: 10.1111/bjet.13116
Mavroudi, A., Papadakis, S., and Ioannou, I. (2021). Teachers’ views regarding learning analytics usage based on the technology acceptance model. TechTrends 65, 278–287. doi: 10.1007/s11528-020-00580-7
Moreno, G. (2022). Expanding definition of technology in special education: Impact of training on the adoption of iPad tablets by special educators. Int. J. Disabil. Dev. Educ. 69, 722–738. doi: 10.1080/1034912X.2020.1731432
Nistor, N., Göğüş, A., and Lerche, T. (2013). Educational technology acceptance across national and professional cultures: A European study. Educ. Tech Res. Dev. 61, 733–749. doi: 10.1007/s11423-013-9292-7
Petko, D., Prasse, D., and Cantieni, A. (2018). The interplay of school readiness and teacher readiness for educational technology integration: A structural equation model. Comput. Sch. 35, 1–18. doi: 10.1080/07380569.2018.1428007
PISA (2017). Teacher questionnaire for Pisa 2018: General teacher. Main Survey Version. Available online at: https://www.oecd.org/pisa/data/2018database (accessed July 29, 2021).
Prestridge, S., and Aldama, C. (2016). A classification framework for exploring technology-enabled practice-frameTEP. J. Educ. Comput. Res. 54, 901–921. doi: 10.1177/0735633116636767
Prochaska, J. O., and DiClemente, C. C. (2005). The transtheoretical approach, Handbook of psychotherapy integration, 2nd Edn. New York, NY: Oxford University Press, 147–171.
Pynoo, B., Devolder, P., Tondeur, J., van Braak, J., Duyck, W., and Duyck, P. (2011). Predicting secondary school teachers’ acceptance and use of a digital learning environment: A cross-sectional study. Comput. Hum. Behav. 27, 568–575. doi: 10.1016/j.chb.2010.10.005
Rödel, A., Siegrist, J., Hessel, A., and Brähler, E. (2004). Fragebogen zur messung beruflicher gratifikationskrisen [Questionnaire to measure occupational gratification crises]. Z. Differentielle und Diagn. Psychol. 25, 227–238. doi: 10.1024/0170-1789.25.4.227
Schaumburg, H. (2021). Personalisiertes lernen mit digitalen medien als herausforderung für die schulentwicklung: Ein systematischer forschungsüberblick [Personalized learning with digital media as a challenge for school development: A systematic research review]. MedienPädagogik 41, 134–166. doi: 10.21240/mpaed/41/2021.02.24.X
Schaumburg, H., and Prasse, D. (2019). Medien und schule: Theorie – forschung – praxis [Media and school: Theory – research – practice]. Bad Heilbrunn: Julius Klinkhardt.
Scherer, R., and Teo, T. (2019). Unpacking teachers’ intentions to integrate technology: A meta-analysis. Educ. Res. Rev. 27, 90–109. doi: 10.1016/j.edurev.2019.03.001
Scherer, R., Siddiq, F., and Tondeur, J. (2019). The technology acceptance model (TAM): A meta-analytic structural equation modeling approach to explaining teachers’ adoption of digital technology in education. Comput. Educ. 128, 13–35. doi: 10.1016/j.compedu.2018.09.009
Schmid, U., Goertz, L., Radomski, S., Thom, S., and Behrens, J. (2017). Monitor digitale bildung: Die hochschulen im digitalen zeitalter. Guetersloh: Bertelsmann Stiftung.
Schunk, D. H. (1990). Goal setting and self-efficacy during self-regulated learning. Educ. Psychol. 25, 71–86. doi: 10.1207/s15326985ep2501_6
Schwarzer, R. (2016). Coping planning as an intervention component: A commentary. Psychol. Health 31, 903–906. doi: 10.1080/08870446.2016.1158260
Schweizer, K., and Horn, M. (2014). “Kommt es auf die einstellung zu digitalen Medien an? Normative überzeugungen, personale faktoren und digitale medien im unterricht: Eine untersuchung mit lehrpersonen und lehramtsstudierenden [Does the attitude towards digital media matter? Normative beliefs, personal factors and digital media in the classroom: A study with teachers and student teachers],” in Bildung mit und über Medien: Perspektiven von Bildungsforschung und Medienpädagogik [Education with and about media: Perspectives from educational research and media education], 6th Edn, eds K. Demmler and B. Schorb (München: Kopaed), 50–62.
Sheeran, P., and Webb, T. L. (2016). The intention-behavior gap. Soc. Pers. Psychol. Compass 10, 503–518. doi: 10.1111/spc3.12265
Skaalvik, E. M., and Skaalvik, S. (2015). Job satisfaction, stress and coping strategies in the teaching profession-what do teachers say? Int. Educ. Stud. 8:3. doi: 10.5539/ies.v8n3p181
Steinmayr, R., Lazarides, R., Weidinger, A. F., and Christiansen, H. (2021). Teaching and learning during the first COVID-19 school lockdown: Realization and associations with parent-perceived students’ academic outcomes. Z. Pädagog. Psychol. 35, 85–106. doi: 10.1024/1010-0652/a000306
Sugar, W., Crawley, F., and Fine, B. (2004). Examining teachers’ decisions to adopt new technology. Educ. Technol. Soc. 7, 201–213.
Sung, Y.-T., Chang, K.-E., and Liu, T.-C. (2016). The effects of integrating mobile devices with teaching and learning on students’ learning performance: A meta-analysis and research synthesis. Comput. Educ. 94, 252–275. doi: 10.1016/j.compedu.2015.11.008
Tondeur, J., van Braak, J., Ertmer, P. A., and Ottenbreit-Leftwich, A. (2017). Understanding the relationship between teachers’ pedagogical beliefs and technology use in education: A systematic review of qualitative evidence. Educ. Technol. Res. Dev. 65, 555–575. doi: 10.1007/s11423-016-9481-2
Utami, I. Q., Fahmiyah, I., Ningrum, R. A., Fakhruzzaman, M. N., Pratama, A. I., and Triangga, Y. M. (2022). Teacher’s acceptance toward cloud-based learning technology in Covid-19 pandemic era. J. Comput. Educ. 9, 571–586. doi: 10.1007/s40692-021-00214-8
van Buuren, S. (2018). Flexible imputation of missing data, 2nd Edn. New York, NY: Chapman & Hall Book.
van Buuren, S. (2023). Multivariate imputation by chained equations. Package ‘mice‘. Available online at: https://cran.r-project.org/web/packages/mice/mice.pdf (accessed September 23, 2023).
van der Kleij, F. M., Feskens, R. C. W., and Eggen, T. J. H. M. (2015). Effects of feedback in a computer-based learning environment on students’ learning outcomes. Rev. Educ. Res. 85, 475–511. doi: 10.3102/0034654314564881
van Schoors, R., Elen, J., Raes, A., and Depaepe, F. (2021). An overview of 25 years of research on digital personalised learning in primary and secondary education: A systematic review of conceptual and methodological trends. Br. J. Educ. Technol. 52, 1798–1822. doi: 10.1111/bjet.13148
Vanbecelaere, S., Cornillie, F., Depaepe, F., Guerrero, R. G., Mavrikis, M., Vasalou, M., et al. (2020). “Technology-mediated personalised learning for younger learners,” in Proceedings of the 2020 ACM interaction design and children conference: Extended abstracts, eds S. Price, E. Rubegni, and A. Vasalou (New York, NY: ACM), 126–134.
Venkatesh, V., Morris, M. G., Davis, G. B., and Davis, F. D. (2003). User acceptance of information technology: Toward a unified view. MIS Q. 27, 425–478.
Venkatesh, V., Thong, J. Y. L., and Xu, X. (2012). Consumer acceptance and use of information technology: Extending the unified theory of acceptance and use of technology. MIS Q. 36:157. doi: 10.2307/41410412
Venkatesh, V., Thong, J., and Xu, X. (2016). Unified theory of acceptance and use of technology: A synthesis and the road ahead. J. Assoc. Inf. Syst. 17, 328–376. doi: 10.17705/1jais.00428
Voss, T., and Wittwer, J. (2020). Unterricht in zeiten von corona: Ein blick auf die herausforderungen aus der sicht von unterrichts- und instruktionsforschung [Teaching in times of corona: A look at the challenges from the perspective of research on learning and instruction]. Unterrichtswissenschaft 48, 601–627. doi: 10.1007/s42010-020-00088-2
Walsh, V., and Farren, M. (2018). Teacher attitudes regarding barriers to meaningfully implementing iPads in a primary school setting. Comput. Sch. 35, 152–170. doi: 10.1080/07380569.2018.1462674
Keywords: technology acceptance, individualization, digital learning platforms, primary school teacher, UTAUT
Citation: Kahnbach L, Hase A, Kuhl P and Lehr D (2024) Explaining primary school teachers’ intention to use digital learning platforms for students’ individualized practice: comparison of the standard UTAUT and an extended model. Front. Educ. 9:1353020. doi: 10.3389/feduc.2024.1353020
Received: 13 December 2023; Accepted: 19 April 2024;
Published: 15 May 2024.
Edited by:
Julie A. Delello, The University of Texas at Tyler, United StatesReviewed by:
Konstantinos Michos, University of Zurich, SwitzerlandShubham Pathak, Walailak University, Thailand
Copyright © 2024 Kahnbach, Hase, Kuhl and Lehr. This is an open-access article distributed under the terms of the Creative Commons Attribution License (CC BY). The use, distribution or reproduction in other forums is permitted, provided the original author(s) and the copyright owner(s) are credited and that the original publication in this journal is cited, in accordance with accepted academic practice. No use, distribution or reproduction is permitted which does not comply with these terms.
*Correspondence: Dirk Lehr, ZGlyay5sZWhyQGxldXBoYW5hLmRl