- 1School of Business, Shenzhen City Polytechnic, Shenzhen, Guangdong, China
- 2Faculty of International Tourism and Management, City University of Macau, Taipa, Macao SAR, China
This study verifies the practical use of virtual reality (VR) technology in real-world educational settings. The evidence indicates that the unique encounter provided by technology can assist university scholars in mastering educational assignments with high motivation and satisfaction toward the innovative learning system. This study analyzes 82 college students who used VR technology in the classroom for 6 weeks. The collected data were further analyzed using SPSS24.0 and SMARTPLS 3 analysis software. The study found the use of VR technology in education led to high levels of satisfaction and motivation to learn. As a result, college students’ learning performance was improved. The research also found that learning motivation and satisfaction played a partial role in mediating the correlation between perceived coolness and blended learning performance. This finding highlights the importance of the blended learning task arrangement, which was further verified through actual learning tasks.
1 Introduction
Virtual reality (VR) technology presents a computer-generated simulation of a three-dimensional environment that offers users an immersive and realistic experience. VR technology has been widely applied in diverse fields, such as entertainment, education, healthcare, and tourism (Acosta Castellanos and Queiruga-Dios, 2022; Bazi et al., 2023; Chan, 2023; Matthes et al., 2023). VR technology can potentially significantly improve the quality and efficacy of tourism education. It allows students to experience realistic and authentic tourism scenarios that would otherwise be hard to access. One such example is the ability to virtually visit various destinations, cultures, and attractions, allowing for a deeper understanding of their history, geography, and ecology. Moreover, VR technology allows for practicing and applying vital skills and knowledge in simulated tourism. Numerous studies into use of VR in education have uncovered favorable predicts. These include heightened time-on-task (Johnson et al., 1998; Huang et al., 2010), increased enjoyment (Apostolellis and Bowman, 2014; Ferracani et al., 2014), boosted motivation (Jacobson, 2011; Cheung et al., 2013; Sharma et al., 2013), and deeper learning with improved long-term retention (Rizzo et al., 2009; Huang et al., 2010).
Virtual reality technology promises to transform education, offering students a more engaging, dynamic, and immersive experience. However, despite the rapid development of VR technology, there is a need for more empirical research on its application and predictions on tourism education. Virtual Reality is a digital experience that uses computer technology to create an artificially rendered digital experience in which the visual experience is entirely computer-generated. Although the technical feasibility of VR has been known for decades, it has been limited by mobile platforms (such as iOS, and VR has only recently become commercialized with the advent of Android) and the rapid development of consumer-grade hardware (Arici et al., 2019). While the potential benefits of VR are evident at all levels of education, as these technologies are increasingly integrated into learning environments, key aspects deserve attention. These include cognitive load, motion sickness, course challenges, and teaching strategies (Wu et al., 2018; Alalwan et al., 2020; Servotte et al., 2020; AlGerafi et al., 2023; Yu, 2023). Therefore, there is a requirement for more systematic and rigorous research to scrutinize the actual effects and predicts of VR technology on tourism students’ learning performance, motivation, and contentment. Additionally, there is a necessity for thorough and all-encompassing research to examine the elements and circumstances that predict the triumphant incorporation and execution of VR technology in tourism education.
Consequently, this investigation aims to address this research gap and contribute to the literature on VR technology and tourism education. The fundamental research inquiry of this study is: How does VR technology associate with the educational achievements and drive of tourism scholars in the classroom? This study employs quantitative data collection and analysis to address the research question.
Research question:
(1) Are college students using VR technology devices for learning because it is a novel and excellent technological experience?
(2) Does student interest in VR enhance learning performance?
(3) Does students’ interest in VR technology improve their learning motivation?
(4) Does students’ interest in VR technology associate with students’ love of learning?
This research holds both theoretical and practical value. The study will provide empirical evidence and insights into the predictions and outcomes of VR technology on the learning of tourism students. Additionally, it will enrich the existing literature on VR technology and tourism education. This study will prove advantageous for tourism educators, students, and practitioners by showcasing the potential and value of VR technology as a powerful and innovative tool in tourism education. Additionally, this research will provide recommendations for enhancing the quality and effectiveness of VR technology applications and their implementation in tourism education.
2 Literature review
2.1 Perceived coolness
Generally speaking, “cool” is a positive evaluation of a person, thing (such as a product or technology), or brand that deviates from everyday perceptions and provides a unique or trendy aspect desirable in society (Sundar et al., 2014). “Cool” is typically perceived as a favorable and attractive characteristic (Nan et al., 2022; Li et al., 2024). The term has been applied to describe numerous subjective entities. Whereas some researchers perceive it as a personal trait that people possess (Dar-Nimrod et al., 2012). Prior research indicates that consumers may exhibit favorable behavioral intentions if they perceive a product or technology as cool (Niu and Mvondo, 2024). Since VR technology is a relatively new IoT product at present, its “cool” features, such as compatibility with other devices (e.g., smartphones, computers), Bluetooth/WiFi connectivity, user-friendly interface, fashion, uniqueness, excellent quality, good performance (Park et al., 2018) may increase its functional value and overall perceived value. This study focuses on student’s “cool” factor, specifically virtual reality in learning, rather than on individuals such as teachers. This study examines the relationship between students’ use of communication technologies in the classroom and students’ perceptions of their coolness.
2.2 Learning motivation
According to Ford (1992), learning motivation is defined as a psychological state aimed at achieving goals, beliefs, and emotions. Motivation refers to a stimulus that encourages, instructs, and sustains behavior, endorsing students’ commitment to a particular subject. Given direction and persistence concerning learning (Fredricks et al., 2004; Reeves, 2006) is crucial for achieving successful academic performance. Learning motivation plays a significant role in this pursuit. Different predictions have been found regarding the predictions of VR on learning attitudes and motivation. The VR method based on spherical video did not significantly correlate with learners’ attitudes toward it (Chang et al., 2019). However, students in the VR-based group showed significantly more favorable attitudes than control groups (Van Ginkel et al., 2019). Students are very interested in VR-based learning methods (Lin et al., 2003; Ogbuanya and Onele, 2018; Winkelmann et al., 2020; Lee, 2023).
2.3 Learning satisfaction
Satisfaction, according to Bedggood and Donovan (2012), is commonly defined as the level to which students enjoy their studies and is influenced by factors such as engagement and skill enhancement. Assessing and evaluating learning predictions, including in blended learning environments (Arbaugh, 2014; Rahman et al., 2015), satisfaction with learning is crucial. Students who are content with their academic achievements tend to equate it with satisfaction in their academic experience. Key satisfiers for university students entail experiencing a sense of accomplishment with their learning (Douglass et al., 2012). Performance in subjects is assessed based on scores obtained on assessment items that measure achievement of learning outcomes, so student satisfaction depends on their perceptions of achievement of learning outcomes.
2.4 The perceived coolness of using VR technology is an antecedent of learning satisfaction and motivation
We propose “perceived coolness” as an antecedent factor in students’ learning motivation and satisfaction. Studies have demonstrated that consumers, when they feel they are at the forefront of a trend, tend to develop positive attitudes toward the related device (Kim, 2016). positive academic emotions part of the intermediary role between college students’ online learning motivation and online learning performance (Zhu et al., 2022). Past studies showed that it is also an essential perceptual factor technology can motivate students to engage in learning and stimulate intense learning motivation (Bracq et al., 2019; Chang et al., 2019, 2020a; Shi et al., 2019; Taranilla et al., 2019; Bourgeois-Bougrine et al., 2020; Singh et al., 2020). VR applications can improve students’ quality of learning (McGovern et al., 2020) and perceived satisfaction (Chang et al., 2020a) in presentation skills. VR technology can improve learning satisfaction (Jung et al., 2012; Chang et al., 2019; Makransky et al., 2019; Meyer et al., 2019; Winkelmann et al., 2020). VR technology did not significantly change learners’ self-efficacy (Chang et al., 2020a), but a positive prediction of self-efficacy was found in VR-based conditions (Meyer et al., 2019; Shu et al., 2019; Strzelecki, 2023).
We explored the concept of perceived cool technology using iPads in educational research. Teachers’ and children’s perceptions heavily predicted our project, particularly regarding the iPad as a cool technology (Culén and Gasparini, 2012). Our study considers the application of VR technology in the classroom as a scenario of perceived cool application. Whether it is an iPad, iPhone, or VR technology, they represent the emergence of new technologies and practical application scenarios. These technologies can be used by anyone as application scenarios, enabling individuals to experience the coolness factor and conduct follow-up behavioral research. Therefore, we formulated these hypotheses.
H1: The perceived coolness of using VR technology positively relates to learning motivation.
H2: The perceived coolness of using VR technology positively relates to learning satisfaction.
2.5 Blended learning performance
Blended learning utilizes the Internet’s convenience and valuable resources in conjunction with the benefits of traditional learning, enhancing teaching by incorporating online computer and network activities into conventional courses. This new educational mode has been proposed to serve as a supplement to traditional face-to-face learning (Benbunan-Fich, 2008). The implementation of blended learning represents a restructuring of curriculum design with the aim of encouraging student engagement in online learning. Research has demonstrated that the incorporation of information technology into the teaching and learning process fosters the development of enhanced educational outcomes. Acquisition of course resources and improving the learning experience have been identified as key factors in enhancing learning outcomes (Bai et al., 2016; Darling-Aduana and Heinrich, 2018; Turvey and Pachler, 2020; Deng et al., 2023).
Blended learning performance can be defined in various ways. For example, it may relate to students’ examination predicts (Law and Geng, 2019), or satisfaction with learning. Yang conducted research on online learning and measured students’ performance using an online learning system. The study relied on the definition of learning performance from the Association for Educational Communications and Technology. In 2004, the definition of learning performance was established, stating that it measures a learner’s ability to apply newly acquired knowledge or skills. This definition emphasizes not only the acquisition of basic knowledge and skills but also the capacity to use them effectively.
2.6 Blended learning performance as a consequence of perceptions
The VR virtual technology stimulates students’ interest in learning, increases their enthusiasm for learning, and improves their learning performance (Geng et al., 2018). Chang et al. (2020b) used VR technology for interactive learning in primary school classrooms, and compared with students using traditional learning, students who studied using VR achieved positive predicts in learning satisfaction and motivation. Research results show that this teaching method can improve students’ learning performance and motivation, enable them to solve problems, and actively improve their academic performance.
Blended learning methods have proven to significantly enhance learning. The learning method has a higher course satisfaction rate than traditional classroom teaching (Darling-Aduana and Heinrich, 2018). The learning method has a higher course satisfaction rate than traditional classroom teaching. As stated by Yildirim (2005), it enhances student devotion to the learning process. Greater flexibility and convenience are appreciated by students, making it their preferred option (Hogarth, 2010). So, we formulated these hypotheses.
H3: The perceived coolness of using VR technology positively relates to blended learning performance.
H4: Learning motivation positively relates to blended learning performance.
H5: Learning satisfaction positively relates to blended learning performance.
2.7 Mediation role of learning motivation and learning satisfaction
As shown in the literature review above, the application of VR technology in education has been proven to stimulate students’ learning motivation (Bracq et al., 2019; Chang et al., 2019, 2020a; Shi et al., 2019; Taranilla et al., 2019; Bourgeois-Bougrine et al., 2020; Singh et al., 2020) and improve students’ learning satisfaction (Jung et al., 2012; Chang et al., 2019; Makransky et al., 2019; Meyer et al., 2019; Winkelmann et al., 2020). At the same time, students’ strong motivation (Law et al., 2010; Law and Breznik, 2017; Law and Geng, 2019) and high learning satisfaction can improve students’ learning performance (Meyer et al., 2019; Shu et al., 2019). In this study, we investigated how VR technology associates with students’ blended learning performance in a class by mixing online and offline learning, so we formulated these hypotheses.
H6: Learning motivation mediates between the perceived coolness of using VR technology and blended learning performance.
H7: Learning satisfaction mediates between the perceived coolness of using VR technology and blended learning performance.
Based on the above literature review of the relationship between the perceived coolness of using VR technology, learning motivation, learning satisfaction and blended learning performance, (Figure 1) illustrates the hypothetical relationship between the theoretical model and various structures (perceived coolness of using VR technology, learning motivation, learning satisfaction, and blended learning performance) of all indicators tested in our research.
3 Research method
3.1 Data collection and sample characteristics
This research is grounded in a sample size of 82 second-year college students studying international tourism and management in 2021 at City Polytechnic of Shenzhen, encompassing varying genders, and ages. The participants were selected through a convenient sampling method and data was collected through a detailed survey, graded on a 5-point Likert-type scale ranging from “strongly disagree” to “strongly agree,” and fully implemented in the questionnaire. We conducted data analysis using SPSS 24.0 and PLS 3. The questionnaire survey is a form of offline data collection and analysis undertaken after the mid-term examination of students between the dates of November 10th, 2023 and November 15th, 2023.
3.2 Measurements
The measurement scale employed to gauge multiple aspects of the suggested model (i.e., Perceived coolness, learning satisfaction, learning motivation, and Blended learning performance) is derived from existing literature. Four items from (Yin and Yuan, 2021) were used to measure learning motivation with good reliability, its internal consistency coefficient Cronbach α = 0.87; while five items from (Sun et al., 2008) were adopted to evaluate learning satisfaction with good reliability, its internal consistency coefficient Cronbach α = 0.91. Blended learning performance was assessed via seven items taken from Yin and Yuan (2021) with good reliability, its internal consistency coefficient Cronbach α = 0.88. The paper utilized a four-item Perceived Coolness Scale adapted from Sundar et al. (2014) with good reliability, its internal consistency coefficient Cronbach α = 0.89. All items are measured using a 5-point Likert scale.
3.3 Data analysis
Partial Least Squares-Structural Equation Modeling (PLS-SEM) analysis was preferred over traditional covariance-based structural equation models (CB-SEM) due to the complexity of the model, which includes many constructs and indicators, set against the sample size (N = 200). Such complexity, when using CB-SEM, would cause our model to be empirically under-identified (Kline, 2004). Furthermore, using PLS-SEM is also useful in overcoming any deviations from normality in data sets (Chin, 1998). It is contended that PLS-SEM produces reliable and sturdy outcomes with limited sample sizes of roughly 100 observations and non-normal data, making it well-suited for the current investigation (Valle and Assaker, 2016). SEM-PLS processing using smart pls 3 is conducted in two stages: (1) The first step is to test the outside model. (2) The second stage is testing the inner model. At this stage the aim is to find out whether there is an influence between variables. Tests are carried out using the t-test.
Firstly, we distributed and recycled the scales, followed by the employment of SPSS23.0 to perform demographic analysis and measure reliability and validity on the collected data from the scales. The data was then imported into smartpls3 to execute a confirmatory factor analysis to establish the research hypothesis.
3.4 Pilot test
In September 2023, a questionnaire was prepared, and a pilot study was carried out to evaluate the validity and reliability of the scales employed. In the pilot study, 30 students were surveyed and requested to provide feedback on the comprehensibility of the scales. The findings indicated that all variables met the pre-determined criteria for validity and reliability, affirming the consistent reliability of the developed scale in measuring students’ levels of acceptance toward VR technology in higher education. This conclusion was supported by composite reliability, Cronbach’s alpha, reliability coefficients exceeding 0.7, and average variance extracted (AVE) surpassing 0.5. It was proved that the experimental scale has good reliability and validity.
3.5 Research result
The first part of the questionnaire represents the demographic characteristics of respondents. A total of 82 copies were disbursed, and all were recovered, yielding a 100% recovery rate. Female group with 67.1% are 55 students and males with 32.9% are 27 students. Of the total, 76.8% are 63 graduate students aged between 20 and 25 years old. The remaining 23.2% are 19 graduate students above 26 years old.
3.5.1 Convergent validity testing
A reliable model fit analysis considers the convergent validity of items, measuring how closely they align with each other. According to Table 1, composite reliability values range from 0.918 to 0.944, indicating that they all exceed the recommended threshold of 0.70. Furthermore, the Cronbach’s alpha values surpass the suggested value of 0.70, ranging from 0.873 to 0.921. An alpha value of 0.70–0.80 or higher indicates an exceptional level of reliability (Ursachi et al., 2015). The AVE values range from 0.664 to 0.828, all exceeding the recommended value of 0.50 as suggested by Fornell and Larcker (1981).
3.5.2 Discriminant validity testing
Discriminant validity is a test that assesses the extent to which the constructs in the model are close to each other or how they differ from one another (Bagozzi et al., 1991). As it can be seen in Table 2, the AVE values exceed the proposed 0.50 loading, indicating that discriminant validity is supported for the construct (Fornell and Larcker, 1981). Besides Hair Jr et al. (2021) suggested the use of the Heterotrait-Monotrait ratio of correlations (HTMT) to measure discriminant validity. The guideline is that a value above 0.90 indicates poor discriminant validity, while a value below 0.90 indicates good discriminant validity.
3.5.3 Testing hypotheses and the moderating effect
An SEM model was employed to perform a multiple regression analysis to investigate the correlation between students’ perception coolness of using technology, motivation to learn, satisfaction with VR technology, and blended learning performance. The outcome of the analysis is presented in Table 3, where a significant association was found between all factors. The findings indicate that students who are considered ‘cool’ and use VR technology have a direct impact on their overall blended Learning performance. Furthermore, there is a significant correlation between the use of VR technology, student motivation and satisfaction.
The study also revealed a positive correlation between students feeling ‘cool’ when using VR technology and their learning motivation (path coefficient = 0.768, t = 14.891, p < 0.000), thereby supporting the proposed hypothesis H1. The findings indicate that H2 demonstrates a favorable correlation between students’ sense of enjoyment when utilizing VR technology and satisfaction with their blended Learning performance, with a path coefficient = 0.767, t = 14.640, and p < 0.000, substantiating support for H2.
Additionally, the outcomes presented in Table 4 reveal significant progress in blended learning performance among students, with a path coefficient = 0.731, t = 10.446, and p < 0.000, directly linking to enhanced student enjoyment when using VR technology, endorsing H3. The results suggest a significant relationship between students’ learning motivation and satisfaction with blended learning performance (path coefficient = 0.570, t = 4.599, p < 0.000), supporting H4. Then, H5 was supported as there was a correlation found between students’ learning satisfaction and blended learning performance (path coefficient = 0.257, t = 2.174, p = 0.030<0.1).
Finally, the SEM model analyzed the mediating effects of learning motivation and satisfaction on the enhancement of students’ overall blended Learning performance. The results in Table 4 demonstrate that learning motivation had a significant impact on both students’ sense of enjoyment when using VR technology and their overall blended Learning performance improvement, path coefficient = 0.438, t = 4.777, p < 0.000, thereby confirming hypothesis H6.
The same as, the satisfaction of learning only has a significant impact on students’ perception of the appeal of utilizing VR technology and the variation in students’ performance improvement. The path coefficient = 0.197, t = 2.078 and p = 0.038, which is less than the prescribed level of 0.1. Therefore, based on these findings, it may be inferred that supported the hypothesis H7. Additionally, the t-values for H5 and H7 are 2.174 and 2.078 correspondingly. If the critical ratio of the difference falls outside of the range of −1.96 to +1.96, we may infer a significant difference between the hypothesis groups. However, if the ratio falls within that range, we cannot conclude that there is a significant difference.
3.5.4 Intermediary effect test
Based on Table 4, a student learning motivation and satisfaction act as mediating variables between students’ positive engagement with VR technology and their blended learning performance improvement. The next step will be to investigate whether these two variables only partially mediate or fully mediate the relationship. We calculate the value of variance accounted for (VAF) in PLS 3 to judge (Hayes and Preacher, 2014) (VAF = Indirect Effect/Total Effect; VAF > 80% indicates full mediation; 20% < VAF < 8% shows partial mediation; VAF < 20% indicates no mediation).
Table 4 presents data for two intermediary responses. Firstly, the p values of the intermediary responses as represented by H6 and H7 are all less than 0.1, indicating they hold statistical significance. Abbreviations are explained where used for clarity. Subsequently, we utilize a tool to calculate VAF values for analysis purposes.
The VAF is dividing import effect by total effect, with VAF (LM) = 0.438 ÷ 1.169 = 0.375 = 37.5% > 20%; VAF (LS) = 0.197 ÷ 0.928 = 0.212 = 21.2% > 20%. Therefore, the perceived coolness of employing VR technology partially mediates blended learning performance through the intermediate factors of motivation and satisfaction. Thus, H6 and H7 are supported.
3.5.5 Structural model result
After verifying the research hypothesis, we analyze and sort out the obtained data according to PLS 3 software and improve it to our structural model, as shown in Figure 2, which is the structural result of our model.
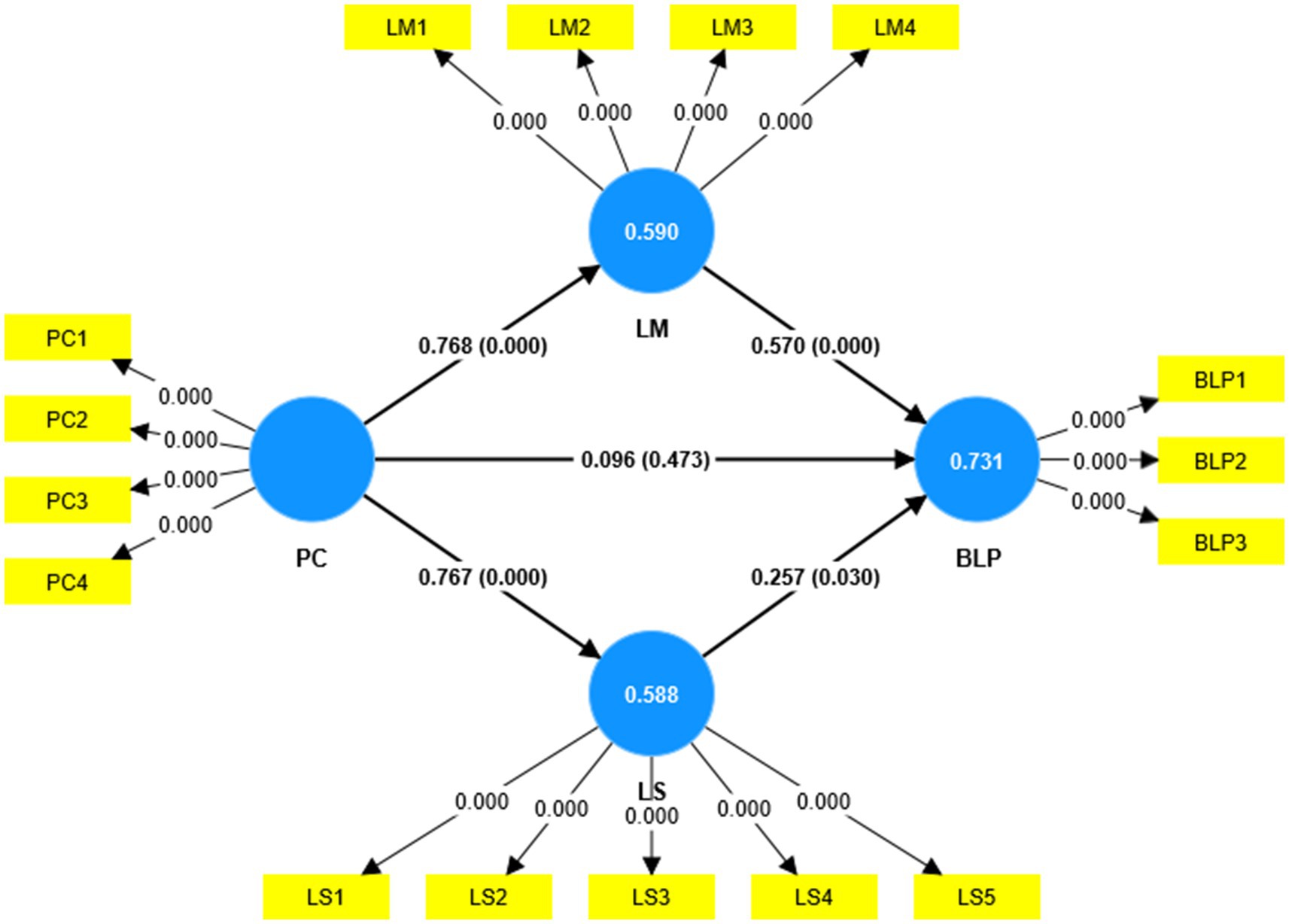
Figure 2. Structural model. PC, perceived coolness using VR Technology; BLP, blended learning performance; LM, learning motivation; LS, learning satisfaction.
4 Discussion and conclusion
The study’s key findings align with prior research, indicating that students’ keenness to explore new technologies and engage with them actively has a favorable impact on their learning motivation and satisfaction (Yin and Yuan, 2021). Much technological innovation has a primary purpose but is often applied in various other contexts and for other objectives. This is particularly evident in the case of mobile devices equipped with cloud computing and applications. The iPad serves as a prime illustration of a ubiquitous technological trend. Its widespread acceptance in education makes it an apt example of contextual usage. What students find appealing are innovative things that they can sufficiently master, perform impressive tasks with, and which provide them with added value. Moreover, customization or self-creation is often considered captivating (Culén and Gasparini, 2012). When comparing iPad and VR technology, it was discovered that referring to new technologies elicits a positive emotional response (Liu et al., 2023). To corroborate our findings, we will compare our research with existing literature. The results of this study are consistent with Dunford and Miller’s analysis of first-, fourth-year, and fifth-year college students. The experiment found that VR technology can significantly improve the interaction between teachers and students, enhance students’ learning satisfaction (Dumford and Miller, 2018), and thus improve learning performance because VR technology can simulate face-to-face interaction while retaining some of the convenience of online learning. A recent quasi-experimental study of virtual reality technology concluded that wearable VR was more effective in increasing situational awareness and interest, engagement, and overall learning experience in the context of physical laboratory courses (Sun et al., 2023). This is also consistent with our research findings that the experience provided by the new VR technology stimulates students’ motivation to learn and positively impacts improving students’ learning performance. Furthermore, demonstrating curiosity and experimenting with technology positively contribute to their learning performance. In our empirical research, we utilized VR technology as a blended teaching tool, and the experimental results achieved our expected results. The use of VR technology can help advanced students improve their learning performance. The findings indicate that college students exhibit curiosity toward the integration of new technologies in classroom teaching and strive to implement them. The novel experience promotes students’ motivation toward learning and enhances their interest therein. There is a higher degree of classroom teaching contentment resulting from this. Such findings have the potential to enhance students’ academic performance in college settings. This study holds significant value to schools as the results can foster students’ learning motivation and proficiency in learning within teaching contexts.
The empirical analysis has identified future research directions and areas of concern. One of the survey variables based on empirical results is that the use of innovative technologies by students appears to be the most significant factor in enhancing learning satisfaction. From the data analysis results, it can be concluded that learning satisfaction partially mediates between perceived coolness using VR Technology and hybrid learning performance, and the value approaches the limit, proving that learning satisfaction is not the best mediating variable, which raises the question of whether there are other variables that play a crucial role in the relationship between learning satisfaction and performance. Additionally, the application of new technologies generates great curiosity, prompting students to explore their use actively and igniting their enthusiasm and motivation for learning. This study found that learning motivation accounts for only 37.5% of the performance of new technology applications and blended learning. This indicates the presence of other critical variables that must be actively explored.
Thus, the outcomes indicate that blended learning enhances students’ learning and attitude toward education and increases their satisfaction. and at the same time motivates them for the blended learning procedure. VR technology synchronizes Internet search engines to students’ headsets. When teachers assign course tasks and students complete classwork and self-study, students can self-check the most accurate information and knowledge online when they need help, significantly promoting Accelerated understanding and application of knowledge. Secondly, teachers can also work with various fields to design interdisciplinary courses, add tasks and challenges with clear goals for students’ learning, and achieve better learning results by deepening knowledge learned across fields and applying what they have learned through collaboration and hands-on practice. Third, teachers can apply “teaching students by their aptitude” in students’ daily learning activities. In previous teaching activities, it took much work for teachers to designate corresponding learning plans for students with different learning progress and abilities. Now, through VR technology, online Assign learning tasks to improve student learning satisfaction and ultimately improve learning performance. In general, blended learning simplifies the process of publishing resources, assigning independent tasks, and managing courses, thereby increasing student satisfaction and ultimately improving academic performance.
5 Limitation
One limitation of this study is that it solely analyzes students’ perceptions of blended learning without incorporating the viewpoint of teachers. A more comprehensive and reciprocal approach in future research will produce more pertinent outcomes. Enlarging the sample would enhance the generalizability of the findings and allow for the inclusion of an evaluation of teachers and students perceptions of blended learning. Further studies are advised to examine and identify additional key factors involved in enhancing student satisfaction and academic achievement. Additionally, future research may concentrate on emerging information technology trends and how to evaluate the adoption processes of these trends by teachers and students. The integration of ChatGPT in HEIs holds the potential to deliver personalized and relevant learning experiences to students, streamline administrative procedures, and advance research (Strzelecki and ElArabawy, 2024). Future research can cooperate with VR and ChatGPT to contribute to developing higher education.
Data availability statement
The raw data supporting the conclusions of this article will be made available by the authors, without undue reservation.
Ethics statement
The studies involving humans were approved by Shenzhen City Polytechnic, Shenzhen, Guangdong Province, 518116, China. The studies were conducted in accordance with the local legislation and institutional requirements. The participants provided their written informed consent to participate in this study.
Author contributions
XL: Conceptualization, Funding acquisition, Resources, Writing – original draft. HZ: Conceptualization, Data curation, Visualization, Writing – original draft. LL: Methodology, Supervision, Writing – review & editing.
Funding
The author(s) declare that financial support was received for the research, authorship, and/or publication of this article. This study was supported by Achievements of Key Research Base of Humanities and Social Sciences in Shenzhen: Skilled Society Research Center of Shenzhen Institute of Technology.
Conflict of interest
The authors declare that the research was conducted in the absence of any commercial or financial relationships that could be construed as a potential conflict of interest.
Publisher’s note
All claims expressed in this article are solely those of the authors and do not necessarily represent those of their affiliated organizations, or those of the publisher, the editors and the reviewers. Any product that may be evaluated in this article, or claim that may be made by its manufacturer, is not guaranteed or endorsed by the publisher.
References
Acosta Castellanos, P. M., and Queiruga-Dios, A. (2022). From environmental education to education for sustainable development in higher education: a systematic review. Int. J. Sustain. High. Educ. 23, 622–644. doi: 10.1108/IJSHE-04-2021-0167
Alalwan, N., Cheng, L., Al-Samarraie, H., Yousef, R., Alzahrani, A. I., and Sarsam, S. M. (2020). Challenges and prospects of virtual reality and augmented reality utilization among primary school teachers: a developing country perspective. Stud. Educ. Eval. 66:100876. doi: 10.1016/j.stueduc.2020.100876
AlGerafi, M. A., Zhou, Y., Oubibi, M., and Wijaya, T. T. (2023). Unlocking the potential: a comprehensive evaluation of augmented reality and virtual reality in education. Electronics 12:3953. doi: 10.3390/electronics12183953
Apostolellis, P., and Bowman, D. A., (2014), November. Evaluating the effects of orchestrated, game-based learning in virtual environments for informal education. In Proceedings of the 11th Conference on Advances in Computer Entertainment Technology (1–10).
Arbaugh, J. B. (2014). System, scholar or students? Which most influences online MBA course effectiveness? J. Comput. Assist. Learn. 30, 349–362. doi: 10.1111/jcal.12048
Arici, F., Yildirim, P., Caliklar, Ş., and Yilmaz, R. M. (2019). Research trends in the use of augmented reality in science education: content and bibliometric mapping analysis. Comput. Educ. 142:103647. doi: 10.1016/j.compedu.2019.103647
Bagozzi, R. P., Yi, Y., and Phillips, L. W. (1991). Assessing construct validity in organizational research. Adm. Sci. Q. 36, 421–458. doi: 10.2307/2393203
Bai, Y., Mo, D., Zhang, L., Boswell, M., and Rozelle, S. (2016). The impact of integrating ICT with teaching: evidence from a randomized controlled trial in rural schools in China. Comput. Educ. 96, 1–14. doi: 10.1016/j.compedu.2016.02.005
Bazi, S., Filieri, R., and Gorton, M. (2023). Social media content aesthetic quality and customer engagement: the mediating role of entertainment and impacts on brand love and loyalty. J. Bus. Res. 160:113778. doi: 10.1016/j.jbusres.2023.113778
Bedggood, R. E., and Donovan, J. D. (2012). University performance evaluations: what are we really measuring? Stud. High. Educ. 37, 825–842. doi: 10.1080/03075079.2010.549221
Benbunan-Fich, R. (2008). Blended learning in higher education: framework, principles, and guidelines. Acad. Manag. Learn. Edu. 7, 135–137.
Bourgeois-Bougrine, S., Richard, P., Burkhardt, J., Frantz, B., and Lubart, T. (2020). The expression of users’ creative potential in virtual and real environments: an exploratory study. Creat. Res. J. 32, 55–65. doi: 10.1080/10400419.2020.1712162
Bracq, M. S., Michinov, E., Arnaldi, B., Caillaud, B., Gibaud, B., Gouranton, V., et al. (2019). Learning procedural skills with a virtual reality simulator: an acceptability study. Nurse Educ. Today 79, 153–160. doi: 10.1016/j.nedt.2019.05.026
Chan, C. K. Y. (2023). A comprehensive AI policy education framework for university teaching and learning. Int. J. Educ. Technol. High. Educ. 20:38. doi: 10.1186/s41239-023-00408-3
Chang, S. C., Hsu, T. C., Chen, Y. N., and Jong, M. S. (2020a). The effects of spherical video-based virtual reality implementation on students’ natural science learning effectiveness. Interact. Learn. Environ. 28, 915–929. doi: 10.1080/10494820.2018.1548490
Chang, S. C., Hsu, T. C., Kuo, W. C., and Jong, M. S. Y. (2020b). Effects of applying a VR-based two-tier test strategy to promote elementary students’ learning performance in a geology class. Br. J. Educ. Technol. 51, 148–165. doi: 10.1111/bjet.12790
Chang, C. Y., Sung, H. Y., Guo, J. L., Chang, B. Y., and Kuo, F. R. (2019). Effects of spherical video-based virtual reality on nursing students’ learning performance in childbirth education training. Interact. Learn. Environ. 30, 400–416. doi: 10.1080/10494820.2019.1661854
Cheung, S. K., Fong, J., Fong, W., and Wang, F. L. eds., (2013). Blended learning and continuing education: 6th International conference, ICHL 2013, Toronto, ON, Canada, August 12–14, 2013, Proceedings (8038). Springer.
Culén, A. L., and Gasparini, A. A. (2012). Situated techno-cools: factors that contribute to making technology cool in a given context of use. PsychNology J. 10, 117–139.
Darling-Aduana, J., and Heinrich, C. J. (2018). The role of teacher capacity and instructional practice in the integration of educational technology for emergent bilingual students. Comput. Educ. 126, 417–432. doi: 10.1016/j.compedu.2018.08.002
Dar-Nimrod, I., Hansen, I. G., Proulx, T., Lehman, D. R., Chapman, B. P., and Duberstein, P. R. (2012). Coolness: an empirical investigation. J. Individ. Differ. 33, 175–185. doi: 10.1027/1614-0001/a000088
Deng, X., Liu, L., and Liu, X. (2023). Research on service quality of professional education based on the PZB service quality model. Front. Educ. 8:1325256. doi: 10.3389/feduc.2023.1325256
Douglass, J. A., Thomson, G., and Zhao, C. M. (2012). The learning outcomes race: The value of self-reported gains in large research universities. Higher education. 64, 317–335. doi: 10.1007/s10734-011-9496-x
Dumford, A. D., and Miller, A. L. (2018). Online learning in higher education: exploring advantages and disadvantages for engagement. J. Comput. High. Educ. 30, 452–465. doi: 10.1007/s12528-018-9179-z
Ferracani, A., Pezzatini, D., and Del Bimbo, A., (2014), November. A natural and immersive virtual interface for the surgical safety checklist training. In Proceedings of the 2014 ACM International Workshop on Serious Games (27–32).
Ford, M. E. (1992). Human motivation: goals, emotions, and personal agency beliefs. Newbury Park, CA: Sage.
Fornell, C., and Larcker, D. F. (1981). Structural equation models with unobservable variables and measurement error: algebra and statistics. J. Mark. Res. 18, 382–388. doi: 10.1177/002224378101800313
Fredricks, J. A., Blumenfeld, P. C., and Paris, A. H. (2004). School engagement: potential of the concept, state of the evidence. Rev. Educ. Res. 74, 59–109. doi: 10.3102/00346543074001059
Geng, J., Jong, M. S. Y., Luk, E., and Jiang, Y. (2018). Comparative study on the pedagogical use of interactive spherical video-based virtual reality: the EduVenture-VR experience. In 2018 International Symposium on Educational Technology (ISET) (261–263). Osaka, Japan: IEEE.
Hair, J. Jr., Hair, J. F. Jr., Hult, G. T. M., Ringle, C. M., and Sarstedt, M. (2021). An Introduction to Structural Equation Modeling. In: Partial Least Squares Structural Equation Modeling (PLS-SEM) Using R. Classroom Companion: Business. Springer, Cham.
Hayes, A. F., and Preacher, K. J. (2014). Statistical mediation analysis with a multicategorical independent variable. Br. J. Math. Stat. Psychol. 67, 451–470. doi: 10.1111/bmsp.12028
Hogarth, A. (2010). Education in a competitive and globalising world: adopting blended learning for collaborative work in higher education, 1st. New York: Nova Science Publishers.
Huang, H. M., Rauch, U., and Liaw, S. S. (2010). Investigating learners’ attitudes toward virtual reality learning environments: based on a constructivist approach. Comput. Educ. 55, 1171–1182. doi: 10.1016/j.compedu.2010.05.014
Jacobson, S. (2011). Leadership effects on student achievement and sustained school success. International Journal of Educational Management. 25, 33–44. doi: 10.1108/09513541111100107
Johnson, A., Roussos, M., Leigh, J., Vasilakis, C., Barnes, C., and Moher, T., (1998). The NICE project: learning together in a virtual world. In Proceedings. IEEE 1998 Virtual Reality Annual International Symposium (Cat. No. 98CB36180) (176–183). Atlanta, GA, USA: IEEE.
Jung, E. Y., Park, D. K., Lee, Y. H., Jo, H. S., Lim, Y. S., and Park, R. W. (2012). Evaluation of practical exercises using an intravenous simulator incorporating virtual reality and haptics device technologies. Nurse Educ. Today 32, 458–463. doi: 10.1016/j.nedt.2011.05.012
Kim, K. J. (2016). Round or square? How screen shape affects utilitarian and hedonic motivations for smartwatch adoption. Cyberpsychol. Behav. Soc. Netw. 19, 733–739. doi: 10.1089/cyber.2016.0136
Kline, R. B. (2004). Principles and practice of structural equation modeling. Guilford Publications.
Law, K. M. Y., and Breznik, K. (2017). Impacts of innovativeness and attitude on entrepreneurial intention: among engineering and non-engineering students. Int. J. Technol. Des. Educ. 27, 683–700. doi: 10.1007/s10798-016-9373-0
Law, K. M. Y., and Geng, S. (2019). How innovativeness and handedness affect learning performance of engineering students? Int. J. Technol. Des. Educ. 29, 897–914. doi: 10.1007/s10798-018-9462-3
Law, K. M. Y., Lee, V. C. S., and Yu, Y. T. (2010). Learning motivationine-learning facilitated computer programming courses. Comput. Educ. 55, 218–228. doi: 10.1016/j.compedu.2010.01.007
Lee, I. J. (2023). Applying virtual reality for learning woodworking in the vocational training of batch wood furniture production. Interact. Learn. Environ. 31, 1448–1466. doi: 10.1080/10494820.2020.1841799
Li, Y., Bao, S., and Yang, Y. (2024). Research on college students’ behavior in calligraphy courses within a virtual learning environment based on SVVR: a convergent model approach of coolness theory, VAM and TAM. IEEE Access. 12, 28538–28553. doi: 10.1109/ACCESS.2024.3365556
Lin, Y. G., McKeachie, W. J., and Kim, Y. C. (2003). College student intrinsic and/or extrinsic motivation and learning. Learn. Individ. Differ. 13, 251–258. doi: 10.1016/s1041-6080(02)00092-4
Liu, L., Liu, Y., Lai, I. K. W., and Liu, X. (2023). “Research on the practice of VR entering the classroom–taking City University students of Macau as an example” in International Conference on Blended Learning (Cham: Springer Nature Switzerland), 191–201.
Makransky, G., Terkildsen, T. S., and Mayer, R. E. (2019). Adding immersive virtual reality to a science lab simulation causes more presence but less learning. Learn. Instr. 60, 225–236. doi: 10.1016/j.learninstruc.2017.12.007
Matthes, J., Heiss, R., and van Scharrel, H. (2023). The distraction effect. Political and entertainment-oriented content on social media, political participation, interest, and knowledge. Comput. Hum. Behav. 142:107644. doi: 10.1016/j.chb.2022.107644
McGovern, E., Moreira, G., and Luna-Nevarez, C. (2020). An application of virtual reality in education: can this technology enhance the quality of students’ learning experience? J. Educ. Bus. 95, 490–496. doi: 10.1080/08832323.2019.1703096
Meyer, O. A., Omdahl, M. K., and Makransky, G. (2019). Investigating the effect of pre-training when learning through immersive virtual reality and video: a media and methods experiment. Comput. Educ. 140:103603. doi: 10.1016/j.compedu.2019.103603
Nan, D., Lee, H., Kim, Y., and Kim, J. H. (2022). My video game console is so cool! A coolness theory-based model for intention to use video game consoles. Technol. Forecast. Soc. Chang. 176:121451. doi: 10.1016/j.techfore.2021.121451
Niu, B., and Mvondo, G. F. N. (2024). I am ChatGPT, the ultimate AI Chatbot! Investigating the determinants of users' loyalty and ethical usage concerns of ChatGPT. J. Retail. Consum. Serv. 76:103562. doi: 10.1016/j.jretconser.2023.103562
Ogbuanya, T. C., and Onele, N. O. (2018). Investigating the effectiveness of desktop virtual reality for teaching and learning of electrical/electronics technology in universities. Comput. Sch. 35, 226–248. doi: 10.1080/07380569.2018.1492283
Park, K., Kwak, C., Lee, J., and Ahn, J.-H. (2018). The effect of platform characteristics on the adoption of smart speakers: empirical evidence in South Korea. Telematics Inform. 35, 2118–2132. doi: 10.1016/j.tele.2018.07.013
Rahman, N. A. A., Hussein, N., and Aluwi, A. H. (2015). Satisfaction on blended learning in a public higher education institution: what factors matter? Procedia Soc. Behav. Sci. 211, 768–775. doi: 10.1016/j.sbspro.2015.11.107
Reeves, J. R. (2006). Secondary teacher attitudes toward including English-language learners in mainstream classrooms. J. Educ. Res. 99, 131–143. doi: 10.3200/joer.99.3.131-143
Rizzo, A. A., Bowerly, T., Buckwalter, J. G., Klimchuk, D., Mitura, R., and Parsons, T. D. (2009). A virtual reality scenario for all seasons: the virtual classroom. CNS Spectr. 11, 35–44. doi: 10.1017/S1092852900024196
Servotte, J. C., Goosse, M., Campbell, S. H., Dardenne, N., Pilote, B., Simoneau, I. L., et al. (2020). Virtual reality experience: immersion, sense of presence, and cybersickness. Clin. Simul. Nurs. 38, 35–43. doi: 10.1016/j.ecns.2019.09.006
Sharma, S., Agada, R., and Ruffin, J., (2013), April. Virtual reality classroom as an constructivist approach. In 2013 Proceedings of IEEE Southeastcon (1–5). Jacksonville, FL, USA: IEEE.
Shi, A., Wang, Y., and Ding, N. (2019). The effect of game-based immersive virtual reality learning environment on learning outcomes: designing an intrinsic integrated educational game for pre-class learning. Interact. Learn. Environ. 30, 721–734. doi: 10.1080/10494820.2019.1681467
Shu, Y., Huang, Y. Z., Chang, S. H., and Chen, M. Y. (2019). Do virtual reality head–mounted displays make a difference? A comparison of presence and self–efficacy between head–mounted displays and desktop computer–facilitated virtual environments. Virtual Reality 23, 437–446. doi: 10.1007/s10055-018-0376-x
Singh, G., Mantri, A., Sharma, O., and Kaur, R. (2020). Virtual reality learning environment for enhancing electronics engineering laboratory experience. Comput. Appl. Eng. Educ. 29, 229–243. doi: 10.1002/cae.22333
Strzelecki, A. (2023). Students’ acceptance of ChatGPT in higher education: an extended unified theory of acceptance and use of technology. Innov. High. Educ., 1–23. doi: 10.1007/s10755-023-09686-1
Strzelecki, A., and ElArabawy, S. (2024). Investigation of the moderation effect of gender and study level on the acceptance and use of generative AI by higher education students: comparative evidence from Poland and Egypt. Br. J. Educ. Technol., 1–22. doi: 10.1111/bjet.13425
Sun, P. C., Tsai, R. J., Finger, G., Chen, Y. Y., and Yeh, D. (2008). What drives a successful e-learning? An empirical investigation of the critical factors influencing learner satisfaction. Comput. Educ. 50, 1183–1202. doi: 10.1016/j.compedu.2006.11.007
Sun, J. C. Y., Ye, S. L., Yu, S. J., and Chiu, T. K. (2023). Effects of wearable hybrid AR/VR learning material on high school students’ situational interest, engagement, and learning performance: the case of a physics laboratory learning environment. J. Sci. Educ. Technol. 32, 1–12. doi: 10.1007/s10956-022-10001-4
Sundar, S. S., Tamul, D. J., and Wu, M. (2014). Capturing “cool”: measures for assessing coolness of technological products. Int. J. Hum. Comput. Stud. 72, 169–180. doi: 10.1016/j.ijhcs.2013.09.008
Taranilla, R. V., Cózar-Gutiérrez, R., González-Calero, J. A., and Cirugeda, I. L. (2019). Strolling through a city of the Roman empire: an analysis of the potential of virtual reality to teach history in primary education. Interact. Learn. Environ. 30, 608–618. doi: 10.1080/10494820.2019.1674886
Turvey, K., and Pachler, N. (2020). Design principles for fostering pedagogical provenance through research in technology supported learning. Comput. Educ. 146:103736. doi: 10.1016/j.compedu.2019.103736
Ursachi, G., Horodnic, I. A., and Zait, A. (2015). How reliable are measurement scales? External factors with indirect influence on reliability estimators. Procedia Econ. Financ. 20, 679–686. doi: 10.1016/S2212-5671(15)00123-9
Valle, P. O., and Assaker, G. (2016). Using partial least squares structural equation modeling in tourism research: a review of past research and recommendations for future applications. J. Travel Res. 55, 695–708. doi: 10.1177/0047287515569779
Van Ginkel, S., Gulikers, J., Biemans, H., Noroozi, O., Roozen, M., Bos, T., et al. (2019). Fostering oral presentation competence through a virtual reality-based task for delivering feedback. Comput. Educ. 134, 78–97. doi: 10.1016/j.compedu.2019.02.006
Winkelmann, K., Keeney-Kennicutt, W., Fowler, D., Lazo Macik, M., Perez Guarda, P., and Ahlborn, C. J. (2020). Learning gains and attitudes of students performing chemistry experiments in an immersive virtual world. Interact. Learn. Environ. 28, 620–634. doi: 10.1080/10494820.2019.1696844
Wu, P. H., Hwang, G. J., Yang, M. L., and Chen, C. H. (2018). Impacts of integrating the repertory grid into an augmented reality-based learning design on students’ learning achievements, cognitive load and degree of satisfaction. Interact. Learn. Environ. 26, 221–234. doi: 10.1080/10494820.2017.1294608
Yildirim, Z. (2005). Hypermedia as a cognitive tool: student teachers’ experiences in learning by doing. Educ. Technol. Soc. 8, 107–117.
Yin, B., and Yuan, C. H. (2021). Precision teaching and learning performance in a blended learning environment. Front. Psychol. 12:141. doi: 10.3389/fpsyg.2021.631125
Yu, Z. (2023). A meta-analysis of the effect of virtual reality technology use in education. Interact. Learn. Environ. 31, 4956–4976. doi: 10.1080/10494820.2021.1989466
Keywords: VR technology, perceived coolness, learning motivation, learning satisfaction, blended learning performance, tourism education
Citation: Liu X, Zhang H and Liu L (2024) The perceived coolness of using virtual reality technology in blended learning performance can improve learning motivation and learning satisfaction. Front. Educ. 9:1346467. doi: 10.3389/feduc.2024.1346467
Edited by:
Manyu Li, University of Louisiana at Lafayette, United StatesReviewed by:
Thomas Chan, Hong Kong Shue Yan University, Hong Kong SAR, ChinaManfred Man-Fat Wu, Hong Kong Metropolitan University, Hong Kong SAR, China
Copyright © 2024 Liu, Zhang and Liu. This is an open-access article distributed under the terms of the Creative Commons Attribution License (CC BY). The use, distribution or reproduction in other forums is permitted, provided the original author(s) and the copyright owner(s) are credited and that the original publication in this journal is cited, in accordance with accepted academic practice. No use, distribution or reproduction is permitted which does not comply with these terms.
*Correspondence: Liang Liu, VDIzMDkyMTAwMzI5QGNpdHl1LmVkdS5tbw==