- 1Institute for the Future of Education, Tecnologico de Monterrey, Guadalajara, Mexico
- 2Fundación Universitaria Konrad Lorenz, Bogotá, Colombia
- 3School of Humanities and Education, Tecnologico de Monterrey, Monterrey, Mexico
- 4Institute for the Future of Education, Tecnologico de Monterrey, Mexico City, Mexico
The eComplexity instrument aims to measure the perception of achievement in the complex thinking competency and its sub-competencies. To ensure the reliability of this instrument, validation processes like the one presented in this article are necessary. Methodologically, this study evaluates data from 1,037 university students in Mexico, confirming the statistical validity and reliability of the instrument. As a result, the demonstrated reliability of the eComplexity instrument as a tool for measuring perceived achievements in complex thinking provides a valuable resource for assessing the effectiveness of educational interventions. Consequently, this research contributes to a more informed approach to fostering critical thinking skills, benefiting both theoretical exploration and practical application in educational settings. The study employs the Partial Least Squares Structural Equation Modeling (PLS-SEM) to evaluate students’ self-perceptions of their performance in complex thinking and its sub-competencies, thus advancing the field of educational measurement. Academically, it enriches the discourse on the design and validation of instruments, offering a rigorous model for future efforts in measuring cognitive competencies. Practically, the study’s results inform educational practice by identifying systemic and scientific thinking as key to developing complex thinking skills. This knowledge enables educators to more effectively adapt teaching strategies and curricular designs, aiming to enhance students’ ability to navigate the complexities of the modern world.
Introduction
In recent decades, the educational paradigm supported by the priority acquisition of information has given way to a vision that, without neglecting knowledge, pays more attention to developing necessary skills (Bancin and Ambarita, 2019). Parallel to this new formative approach is the need for new measuring criteria for achievement since exams or instruments only verifying knowledge may be insufficient, given that the development of competencies implies necessary attitudes and skills, which need to be assessed (Alt et al., 2023).
Therefore, competency-based education must consider the design and validation of instruments that identify students’ development of professional and transversally relevant skills beyond acquiring and retaining information (AlMunifi and Aleyani, 2019). Measuring competencies is essential to ensure the quality of contemporary education, adapting the academic offerings to students’ particular needs to prepare them best to face the challenges of today’s world (Riahi, 2022). In addition, these instruments also impact the continuous improvement of the pedagogical models themselves, making it possible to identify advantages, attributes, and areas of opportunity for better decision-making at the educational level (Castillo-Martínez et al., 2024a). However, designing a competency measurement instrument is not easy since constant revision is necessary in addition to theoretical, academic, and expert validation, especially during implementation (Wild and Schulze, 2021).
So, this article presents the analysis of the validity of the eComplexity instrument, which was designed to measure students’ perceived achievement of complex thinking competency and its sub-competencies. Although there already was an initial conceptual and expert validation, this article proposes a structural equation model using the PLS-SEM multivariate analysis methodology. The intention was to ratify the validity and reliability of the instrument at the statistical level and to understand profoundly and precisely the interactions and dependencies between the variables of the instrument.
Literature review
The complex thinking competency and its sub-competencies
In general terms, complex thinking is a cognitive approach proposed by the philosopher Edgar Morin to understand and address problems, situations, or systems that are intricate, interconnected, and irreducible to simplistic or linear solutions (Tobón and Luna, 2021). This type of cognitive ability rests on a holistic approach that reflects on problems in their totality, incorporating the natural uncertainty of the environment and the various perspectives and actors involved (Baena et al., 2022). Thus, a complex thinker develops an integrated vision of the world around him/her and is open to contradiction, dialog, multidisciplinarity, and continuous learning (Horn et al., 2022).
Considering the above, complex thinking is a precious skill in the contemporary world, a cognitive competency that allows individuals to make decisions in flexible, uncertain, and constantly changing environments (Rios and Suarez, 2017). In this sense, it is increasingly common to find complex thinking in the training programs of educational institutions, proposed as a cognitive competency associated with their curricula (Silva and Iturra, 2021).
However, to speak of complex thinking as a formative competency implies the need to deconstruct this skill into the different cognitive elements that an individual needs to develop, theoretically proposed as the sub-competencies of critical thinking, systemic thinking, scientific thinking, and innovative thinking (Vázquez-Parra et al., 2023).
Critical thinking is a cognitive process that involves actively and systematically analyzing, evaluating, and reflecting on information, ideas, situations, or problems (Cruz-Sandoval et al., 2023). It aims to develop a deeper understanding, informed decision-making, and practical problem-solving, employing a critical and questioning attitude toward previous assumptions and established criteria (Cui et al., 2021). This skill facilitates constructing clear, objective, and persuasive arguments that respond to challenges and decisions faced by the individual (Hapsari, 2016). For its part, systems thinking is the ability to focus on the understanding and analysis of complex systems rather than their isolated parts, based on the principle that many contemporary situations, problems, and challenges result from interconnected networks of interacting elements (Carlos-Arroyo et al., 2023). In this sense, systems thinking allows understanding how these systems work as a whole, the parts that comprise them, and how all the elements interact, providing an integrated vision of reality (Abuabara et al., 2023).
Scientific thinking refers to the systematic and rational ability to understand the world through methodologies based on observation, experimentation, logic, and the formulation of hypotheses and assumptions (Vázquez-Parra et al., 2022a). Cognitively, it is a fundamental ability to analyze information objectively and reliably in the knowledge-acquisition process (Koerber et al., 2015). Finally, innovative thinking, also known as creative thinking, refers to the ability to generate new ideas, approaches, and original solutions to existing problems or challenges, proposing unconventional solutions from the perspective of existing paradigms (Ramírez-Montoya et al., 2022). This sub-competency goes beyond creativity, including developing flexibility, curiosity, openness to risks, and collaboration as relevant elements for creating new visions of the environment (Saienko et al., 2021).
Thus, complex thinking, as an educational competency, comprises multiple elements that make it a valuable cognitive skill, both professionally and individually, regardless of the discipline of study (Luna et al., 2020). However, due to the breadth of its components, its assessment is a challenge because its measurement must consider different but complementary aspects (Sherblom, 2017). Thus, although there are multiple instruments focused on this competency, most of them specialize in one or more of its sub-competencies, remaining as limited efforts that do not consider the integrated vision of the overall competency (Vázquez-Parra et al., 2022b).
eComplexity and the importance of self-perceived competence
The study and measurement of complex thinking is not something new or isolated because, academically, various texts refer to instruments with this same approach (Castillo-Martínez and Ramírez-Montoya, 2022). The design of the eComplexity instrument refers to other instruments that precede it, such as the one by Ossa-Cornejo et al. (2018) that measured critical and scientific thinking in Chilean students, the one by Cui et al. (2021), who developed a test for disposition to critical thinking among a sample of Chinese medical student, the one by Diana et al. (2021), who developed an e-learning-based assessment of critical thinking, and that of Nagahi et al. (2020), who analyzed various skills associated with systems thinking and proactive personality in a sample of engineering students. However, as mentioned above, these studies focus primarily on some or some of the subcompetencies of complex thinking, which provides an important area of opportunity for eComplexity.
As for the instrument itself, eComplexity is a Likert-scale questionnaire designed to measure students’ perceived mastery of complex thinking and its sub-competencies (Castillo-Martínez and Ramírez-Montoya, 2022). Initially, the instrument only considered systemic, scientific, and critical thinking; innovative or creative thinking came later in revising the questionnaire. The choice of the subcompetencies proposed for this first instrument had as a conceptual basis the definition used by the Tecnologico de Monterrey (2019) in the document “Transversal Competencies. A vision from the TEC21 educational model. Guiding Document for Higher Education Teachers,” which addresses the transversal competencies that must be developed in university students who are part of the TEC21 Model, which in turn was based on various authors such as those mentioned previously in this section in the definition of the subcompetencies, but above all, it is based on the conception of Morin (1990), which states that society is complex and characterized by dialectics, and addresses the importance of complex reasoning and how it is constituted. This definition establishes that the student is capable of applying integrative thinking that enables analysis, synthesis and problem solving and continuous learning through the mastery of the cognitive skills necessary to use scientific, critical and systemic thinking according to the challenges that their current and future context will demand in the exercise of their profession and in the commitment as a citizen with the transformation of the environment. Innovative or creative thinking came later in revising the questionnaire, since at one stage of the research evaluation there was a recommendation from a synod member to include innovative thinking as part of the complex reasoning competency. The eComplexity instrument in its second version, which is presented in this article, comprises 25 Likert-scale statements with five degrees of agreement or disagreement. Table 1 below shows the theoretical basis for the construction of the eComplexity tool.
Although there are other instruments that address complex reasoning or thinking, some limitations were identified. In the case of the Complex21 instrument (Tobón and Luna, 2021), the same authors mentioned that it is an exploratory study and that there was a recommendation from experts who validated their instrument that, in addition to the five skills they handle, two more skills should be included: conceptual analysis and knowledge management, skills that are addressed in the eComplexity instrument presented in this article, specifically in the subcompetence of Scientific Thinking. The Silva and Iturra (2023) instrument was also analyzed and it was found that this instrument did not integrate the subcompetences of scientific thinking and systemic thinking. Thus, it was possible to establish that there are differentiating elements in the eComplexity instrument.
This instrument underwent an initial two-part validation process: a theoretical validation and a content validation with experts. The theoretical validation derived from the analysis of instruments measuring complex reasoning and its sub-competencies. This analysis revealed the absence of an integrative instrument, which revealed an opening to design an instrument that integrates reasoning for complexity with its sub-competencies. Thus, the instrument’s design conceived this competency and its sub-competencies (Castillo-Martínez et al., 2024a).
Regarding content validation by experts, they determined the degree to which the instrument items represented the entire content domain (Rutherford-Hemming, 2018). The experts considered three criteria for the evaluation: clarity, coherence, and relevance (Escobar-Pérez and Cuervo-Martínez, 2008). They answered an online questionnaire and rated the items according to the three criteria. For the clarity criterion, the mean of the experts’ rating was 3.31, representing 82.7% of the scale from 1 to 4. For coherence, the mean was 3.38 (84.5%), and for relevance, the mean was 3.54 (88.5%). Thus, all three criteria scored above 60%, which placed them at a high level (3–4). The correlations of the experts’ means for clarity, coherence, and relevance were low, indicating considerable independence.
This validation process led to modifying some items to improve their clarity, considering the comments made by the evaluators. The qualitative analysis of the experts’ judgment consisted of a detailed analysis of the information and suggestions provided by the experts. The analysis was delimited by the inclusion or exclusion of items, the structure of the indicators, or the creation of new items or dimensions (Juárez and Tobón, 2018). Statistical analysis and the qualitative descriptions in the experts’ comments were vital to improving the instrument’s design. With this 2-part validation process and the support of the R4C Complex Thinking Research Group, eComplexity was ready to apply to student samples to have data that would allow a statistical validation like the one proposed in this article.
In the pilot study conducted with 999 participants, the instrument demonstrated both validity and internal consistency. Exploratory factor analysis revealed a Kaiser-Meyer-Olkin (KMO) index greater than 0.80, p-value less than 0.05, and a Cronbach’s Alpha of 0.93. Additionally, confirmatory factor analysis confirmed the validity of the instrument’s internal structure. Reliability was further assessed using McDonald’s Omega and Guttman’s Lambda coefficients, alongside Cronbach’s Alpha. The findings indicated overall high reliability and internal consistency, with scores for each subcompetency surpassing 0.8. Based on this statistical analysis, the instrument is considered to have sufficient reliability and internal consistency across all subcompetencies.
Following the initial analysis, a correlational study was carried out to determine the relationships among the items of the instrument. The results showed no high correlations (0.8 to 1.0), five moderate correlations (0.6 to 0.8), and 121 low correlations (0.4 to 0.6), suggesting a general independence between the items.
Subsequently, a cluster analysis was conducted to verify the proper placement of elements within their designated clusters. The analysis found that items 1, 3, 7, 10, and items 4, 14, 16, 19 were outliers, positioned far from clusters 4 and 1, respectively. Based on these findings from the pilot study, it is recommended to reassess these outliers to determine whether they should remain in their current clusters or be relocated to other clusters.
In summary, the pilot study established that the instrument possesses a high degree of validity, reliability, and internal consistency, with minimal collinearity across both the overall instrument and its individual subcompetencies. This is evidenced by a Cronbach’s Alpha of 0.93 and a Spearman-Brown coefficient of 0.95. The instrument’s four subcompetencies—systemic thinking, scientific thinking, critical thinking, and inventive thinking—were found to be independent. Each of these subcompetencies, as well as the instrument as a whole, exhibited high internal consistency. Additionally, the average score for student perception on complexity reasoning competence was 3.94, utilizing 78.8% of the scale intended for measuring the instrument. This highlights a potential area for educational enhancement and intervention. It is relevant to note that this pilot, theoretical and expert validation is currently in the process of publication by Castillo-Martínez et al. (2024b), and therefore, only its main results are presented in this article.1
Methods
Data
To fulfill the objective of this study, the authors gathered information from applying the eComplexity to 1,037 students from a university in western Mexico to measure their perceived achievement of the complex thinking competency and its sub-competencies. The sample size is deemed appropriate, in accordance with the recommendations of Westland (2010) and Cohen (1988), as the minimum recommended size should be 355.
It was calculated taking into consideration the following parameters:
i. Minimum size of the absolute effect predicted for the structural equation model: 0.3 (medium level).
ii. Number of latent variables: 5.
iii. Number of observed variables: 26.
iv. p-value, which refers to the level of probability for the study: 0.01.
v. Statistical power level: 0.99.
The calculation was performed using the “Structural Equation Model Sample Size Calculator” by Soper (2024) and follows:
i = number of observed variables
n = number of latent variables
= estimated Gini correlation for a bivariate normal random vector
= anticipated effect size
= Sidak-corrected Type I error rate
= Type II error rate
z = a standard normal score
This student population had candidates who would graduate from all the university’s disciplines: Engineering and Sciences, Humanities and Education, Social Sciences, Health Sciences, Architecture and Arts, and Business. The implementation occurred during the academic semester February–July 2023, using a digitalized Google Forms application.
The R4C research group regulated the study, which had the technical support of the Writing Lab of the Institute for the Future of Education at Tecnologico de Monterrey. This study adhered to institutional parameters regarding ethical research principles, so all participants consented to be included as part of the sample and for their responses to contribute to academic and research purposes. Before answering the instruments, each participant agreed to the use of their information for research and academic dissemination purposes. The consent and information collected in this study is protected by the terms and conditions of the SEL4C privacy notice, which was communicated to the participants (Tecnologico de Monterrey, 2023).
Procedure
The statistical management of the data was carried out with Stata program version 18 (StataCorp, 2023), focusing on six stages of analysis (Figure 1).
Initially, the Shapiro–Wilk test determined whether the data followed a normal distribution. Since it did not (Appendix 1), the authors decided to use the partial least squares SEM (PLS-SEM) estimation method (Mohd Rahim et al., 2022; Widyastuti et al., 2023) due to its robustness in handling non-normal data and its suitability to the study data.
PLS-SEM was chosen for this case for several reasons:
1. PLS-SEM is a non-parametric method that does not assume a specific distribution (Hair et al., 2017), which is crucial as the data in this case did not follow a normal distribution.
2. PLS-SEM is an explanatory approach that facilitates the understanding of relationships between variables (Rigdon, 2012).
3. Henseler et al. (2014) demonstrated that PLS-SEM is suitable for estimating models of composite factors, as it provides unbiased implicit covariances. Additionally, construction scores generated by PLS-SEM are more reliable than summation scores when indicators vary in quality, and it can help identify various model misspecifications.
4. Wijaya et al. (2023) emphasized the robustness of PLS-SEM, especially in limited and non-normally distributed datasets. Although the sample size was not small in this case, the data did not follow a normal distribution.
5. PLS-SEM shows good accuracy in the analysis of psychometric models. Furthermore, it allows hypothesis testing in non-normal distributions, can be applied when an item has fewer than 3 factors, and is used without restrictions regarding sample size (Wijaya et al., 2022).
Imjai et al. (2024) utilized PLS-SEM to examine the interrelationships among three independent variables: (i) Logical thinking skills; (ii) Digital literacy; and (iii) Self-learning ability, along with one dependent variable—The Effectiveness of Internships in Thailand. In this instance, PLS-SEM was employed to explore intricate models involving multiple variables and to navigate scenarios with limited or non-normal data, as might be the case in this study.
Also, In the study of Hsu et al. (2023), the utilization of Partial Least Squares Structural Equation Modeling (PLS-SEM) was pivotal in developing and validating an assessment tool for STEAM (Science, Technology, Engineering, Arts, and Mathematics) creations in K-12 education. PLS-SEM facilitated the analysis of intricate relationships among Computational Thinking (CT) levels, Design Thinking (DT) levels, STEAM interdisciplinary levels, and Literacy-Oriented (LO) levels. Moreover, it was discovered that STEAM served as a mediator between CT and LO, indicating a partial mediation effect. The research underscored the significance of PLS-SEM in ensuring the validity, reliability, and adequacy of the structural model, thereby providing a robust and effective means of evaluating and improving STEAM creations within K-12 educational environments.
Due to the instrument’s characteristics, the authors developed a specific structural model for this analysis (Figure 2). Table 2 shows the items comprising the instrument.
The McDonald’s Omega reliability coefficient was approximately 0.9155, indicating excellent reliability of the scale. Consequently, it can be asserted that the scale is highly consistent in measuring the construct. Ninety-one point 55 % (91.55%) of the variability in the total scale scores is accounted for by the factorial loadings of the items, while the remaining 8.45% is attributed to error variance.
From this, it was necessary to transform the endogenous variable (Complex Thinking) into a categorical variable according to the ranges in Table 3.
This model assesses the relationships between latent and observed variables taken as indicators. In other words, the latent variables represent the underlying theoretical constructs measured through multiple observed variables (Figure 2). The latent variables considered were systemic thinking (SyT), scientific thinking (ScT), critical thinking (CrT), and innovative thinking (IT), proposed to elucidate the perception of achievement in the overall complex thinking (CoT) competency.
When estimating the model and performing a discriminant validity assessment, we observed that the variance explained by the indicator variables of a factor (AVE) was lower than the variance shared with other factors (squared correlations). To address this issue, we analyzed the standardized loadings of each observed variable on their respective latent variables. These loadings indicate the contribution of each observed variable to the measurement of the corresponding latent variable. Those with lower contributions were eliminated, which allowed us to propose a new structural model. The Results section explains this process in depth in the results section.
See also the article “Psychometric properties of eComplexity scale” (Castillo-Martínez et al., 2024b) for other calculations that support the validity and reliability of the eComplexity instrument.
Results
The model results indicate that the observed variables explain 76.28% of the variability in the latent variables, which is corroborated by the average redundancy. In addition, the Relative Global Degree of Fit (Relative GoF) measures how well the model fits compared to a null model (i.e., a model with no relationships), and this value was 0.99768, suggesting an adequate fit relative to the null model (Table 4). Additionally, the tolerance was small (1.00e − 07), indicating no significant convergence problems during model estimation. This positive result suggests that the model was estimated accurately (Table 4).
The standardized loadings provided a measure of the strength of the relationship between the latent variables and their (observed) indicators, where higher values indicate a more robust definition and measurement of these specific constructs. In other words, this analysis highlights the most crucial variables in measuring each type of thinking, demonstrating their importance in defining the respective latent constructs.
In the context of Systems Thinking (SyT), the variable that exhibits the most influence is the ability to “identify associations between variables, conditions, and constraints in a project, challenge, or problem faced” (variable b). In Scientific Thinking (ScT), the most influential variable is the “identification of elements to formulate research questions or hypotheses” (variable j). Within Critical Thinking (CrT), the highest load was in the “ability to identify the basis of one’s own and others’ judgments in order to recognize false arguments” (variable o). Finally, in Innovative Thinking (IT), the most influential variables in the measurement were “Apply innovative solutions to various problems” (variable v) and “Evaluate with a critical and innovative sense the solutions derived from a problem” (variable y; Table 5).
Regarding the internal reliability of the variables, Cronbach’s Alpha coefficient produced values indicating greater consistency in the responses to the questions comprising each latent variable. In addition, the DG index (Dillon-Goldstein rho) supported this internal reliability of the latent variables. When examining rho_A, it was evident that the model presented a composite reliability for each latent variable, thus underscoring more consistency in the responses to the questions that comprise the latent variable (Table 5). In summary, the results indicate that the PLS-SEM model fits the data well, with the latent variables exhibiting robust standardized loadings on their indicators and the reliability measures pointing to the reliability of these latent variables.
To evaluate the discriminant validity in a Partial Simultaneous Equations model, the authors compared the squared inter-factor correlation (squared inter-factor correlation) and the average variance extracted (AVE). Discriminant validity is the ability to distinguish different latent constructs in a model clearly. The values on the main diagonal represent the squared correlation between the latent factors. This squared correlation is a measure of the shared variance between two factors. In general, this shared variance is desired to be low so that the factors are clearly distinct from each other. If the values on the main diagonal are substantially less than 1 (as in this case), it indicates that the latent factors have a low correlation with each other, which is a positive result for discriminant validity.
Table 6 shows that:
• SCT: The squared correlation is 0.47, while the AVE of SyT is 0.5668 and the AVE of SCT is 0.5643. The squared correlation is lower than the corresponding AVE values, suggesting adequate discriminant validity between these two factors.
• SyT vs. CrT: The squared correlation is 0.3783, while the AVE of SyT is 0.5668, and the AVE of CrT is 0.6382. The squared correlation is lower than the corresponding AVE values, indicating adequate discriminant validity.
• SyT vs. CoT: The squared correlation is 0.5383, while the AVE of SyT is 0.5668, and the AVE of CoT is 1. The squared correlation is lower than the AVE of CoT, suggesting discriminant validity.
• SyT vs. IT: The squared correlation is 0.469, while the AVE of SyT is 0.5668, and the AVE of IT is 0.5874. The squared correlation is lower than the corresponding AVE values, indicating adequate discriminant validity.
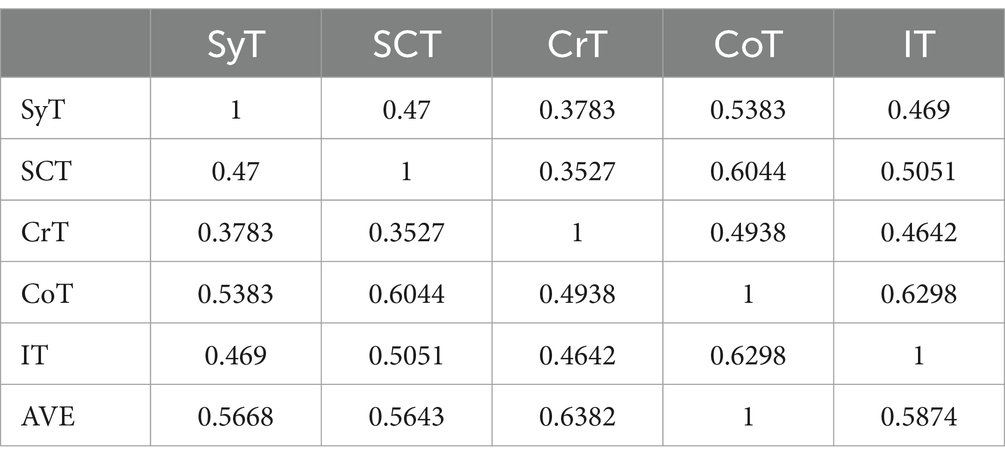
Table 6. Discriminant validity—squared interfactor correlation vs. average variance extracted (AVE).
In summary, the squared correlations are lower than the corresponding AVE values for all pairs of factors, suggesting that this model has good discriminant validity. The factors have relatively low shared variance compared to the total variance of their indicators, which supports the construct validity of this model.
The Standardized Path Coefficients indicate the strength and direction of the relationships between the latent variables [Systems Thinking (SyT), Scientific Thinking (ScT), Critical Thinking (CrT), and Innovative Thinking (IT)] and the dependent variable [Complex Thinking (CoT)]. The coefficients are on a scale from −1 to 1 and show how much a latent variable changes in standard deviations when another latent variable increases by one standard deviation. A positive coefficient indicates a positive relationship, meaning that an increase in the first variable correlates with an increase in the second. On the other hand, a negative coefficient indicates an inverse relationship, meaning that an increase in the first variable correlates with a decrease in the second (Table 7).
Therefore, the results in Table 7 are interpreted as follows, considering that all p-values are less than 1%:
• The standardized path coefficient for Systemic Thinking (SyT) is 0.1885, suggesting a positive relationship between this variable and Complex Thinking (CoT), but it is not a very strong relationship.
• The standardized path coefficient for Scientific Thinking (ScT) is 0.3143, indicating a positive relationship with Complex Thinking (CoT), which is a little stronger than the relationship with Systemic Thinking (SyT).
• The standardized path coefficient for Critical Thinking (CrT) is 0.1858, suggesting a positive relationship with Complex Thinking (CoT), but again, it is not a very strong relationship.
• The standardized path coefficient for Innovative Thinking is 0.3146, indicating a positive relationship with Complex Thinking (CoT), which is similar in strength to the relationship with Scientific Thinking (ScT).
• The adjusted R-squared value (r2_a) for Complex Thinking (CoT) is 0.7619. This means that these latent variables can explain a substantial part (76.19%) of the variations in this type of thinking.
In the correlation matrix of the latent variables (Table 8), the results show moderate positive correlations between the different latent variables in the model, suggesting correlations between these constructs (Table 8).
The cross-loadings in a Partial Simultaneous Equations model show the strength of the relationship between the indicator variables (rows in Table 9: a, b, d, f, g, h, i, j, k, l, n, o, p, t, u, v, w, x, y) and the latent constructs (columns in Table 9). A high cross-loading (greater than 0.7) indicates that the indicator strongly correlates with a latent construct. Table 9 shows that the indicator variables have the highest loadings on their corresponding latent construct (type of thinking to which it is related).
Finally, to interpret the VIF (Variance Inflation Factor) table in a PLS-SEM model, one must remember that VIF measures multicollinearity between constructs. A high VIF indicates that the constructs are highly correlated with each other, which can lead to estimation and model interpretation problems. Table 10 shows that the VIF of latent constructs was less than 5.0, which indicates no significant multicollinearity.
These results determined that the eComplexity instrument proved valid and reliable in the knowledge-based educational framework and for measuring the perceived achievement of the Complex Thinking competency and its sub-competencies. The Partial Least Squares (PLS-SEM) method was employed due to the non-normal nature of the data, showing that (i) the observed variables explained 76.28% of the variability in the latent variables; (ii) the discriminant validity evidenced low correlations between squared factors compared to the Average Variance Extracted (AVE), which indicated good discriminant validity.
Although the normalized path coefficients revealed positive relationships between the predictor variables (systemic thinking, scientific thinking, critical thinking, and innovative thinking) and the dependent variable (complex thinking), systemic and scientific thinking had the most impact on complex thinking.
Conclusion
This study on the validation of the eComplexity instrument marks a significant milestone in the field of education, particularly concerning the measurement and understanding of complex thinking and its sub-competencies. Through a meticulous validation process that included constructing a structural equation model, the study not only confirms the validity and reliability of the instrument for measuring perceived achievement in complex thinking but also methodologically contributes to the design and validation of educational measurement tools. The ability of this study to offer a complementary methodology aimed at achieving greater objectivity in results is particularly valuable, setting a precedent in educational assessment. It paves the way for enhancing the quality and precision in the measurement of complex educational competencies.
Beyond its methodological contributions, the significance of the study extends to its practical implications. By identifying scientific and systemic thinking as key influences on complex thinking, the study provides educators and curriculum designers with concrete data on which areas require more emphasis within educational program design. This information is crucial for developing pedagogical strategies that effectively promote complex thinking among students, better preparing them for the challenges of an interconnected and constantly changing world. Additionally, by highlighting the need for future research to validate the instrument across different contexts and teaching methodologies, the study not only acknowledges its limitations but also outlines a roadmap for the expansion of educational research. This ensures that the tools and strategies developed are truly effective and applicable on a global scale, emphasizing the importance of adapting measurement tools and educational interventions to the diversity of contexts and needs—an essential step toward advancing toward an education that is inclusive, equitable, and capable of fostering complex thinking skills in all students.
The study’s acknowledgment of the subjective nature of self-assessment in measuring complex thinking competencies raises critical considerations for the field of educational psychology and assessment. This aspect introduces an avenue for integrating innovative approaches, such as incorporating artificial intelligence and machine learning algorithms, to analyze and predict educational outcomes with greater accuracy. By addressing this limitation, future research could revolutionize how educational achievements are measured and understood, moving beyond self-reported measures to more objective and nuanced assessments. Such advancements would not only enhance the reliability of educational assessments but also provide deeper insights into the cognitive processes underpinning complex thinking, thereby informing more targeted and effective educational practices. This evolution in educational measurement and evaluation underscores the dynamic interplay between technological innovation and educational research, heralding a new era of precision in understanding and nurturing complex thinking in learners across diverse educational landscapes.
The potential for generalizability of this study is anchored in its comprehensive methodology and the universal relevance of complex thinking competencies in education. The use of Partial Least Squares Structural Equation Modeling (PLS-SEM) for validation not only demonstrates a rigorous approach to instrument assessment but also suggests that such a methodology can be adapted and applied across various educational contexts and cultures. Given the global challenge of equipping students with the skills necessary to navigate an increasingly complex world, the focus on systemic and scientific thinking as crucial components of complex thinking is of universal importance. Furthermore, the study’s emphasis on validating the eComplexity instrument in diverse educational and geographical contexts underlines its potential applicability and effectiveness worldwide. By proposing a methodological framework that can accommodate different teaching methodologies and learning environments, the study paves the way for its findings to be tested and implemented globally. This approach, combined with the call for integrating advanced technologies for more objective assessments, positions the study at the forefront of educational innovation, offering a blueprint for future research aimed at universal educational improvement. Hence, the study’s insights and methodologies hold the promise of generalizability, contributing to the global endeavor to enhance educational practices and outcomes.
Data availability statement
The raw data supporting the conclusions of this article will be made available by the authors, without undue reservation.
Ethics statement
The studies involving humans were approved by Writing Lab, Institute for the Future of Education, Tecnológico de Monterrey. The studies were conducted in accordance with the local legislation and institutional requirements. Written informed consent for participation was not required from the participants or the participants’ legal guardians/next of kin because no personal data, such as names, addresses, or any other identifying information, was collected from the participants. As a result, written informed consent was not deemed necessary for this research, as there was no risk of compromising the confidentiality or privacy of the individuals involved.
Author contributions
JV-P: Conceptualization, Methodology, Project administration, Writing – original draft, Writing – review & editing. LH: Formal analysis, Visualization, Writing – review & editing. JL-G: Formal analysis, Visualization, Writing – review & editing. IC-M: Methodology, Writing – review & editing. PS-B: Methodology, Supervision, Writing – review & editing.
Funding
The author(s) declare financial support was received for the research, authorship, and/or publication of this article. The authors would like to acknowledge the financial support of the “Challenge-Based Research Funding Program 2022” (project ID # I004-IFE001-C2-T3-T) in the production of this work.
Acknowledgments
The authors acknowledge the technical and financial support of Writing Lab, Institute for the Future of Education, Tecnologico de Monterrey, Mexico, in the production of this work.
Conflict of interest
The authors declare that the research was conducted in the absence of any commercial or financial relationships that could be construed as a potential conflict of interest.
Publisher’s note
All claims expressed in this article are solely those of the authors and do not necessarily represent those of their affiliated organizations, or those of the publisher, the editors and the reviewers. Any product that may be evaluated in this article, or claim that may be made by its manufacturer, is not guaranteed or endorsed by the publisher.
Footnotes
1. ^In contrast to the approach taken by Castillo-Martínez et al. (2024b), our study boasts a larger sample size, exceeding theirs by 38 individuals, and delves deeper into the non-normal distribution of the data. Consequently, we opted for a non-parametric technique, namely PLS-SEM. Moreover, we transformed the endogenous variable into quartiles, enabling interpretation across five distinct levels: Very Low, Low, Medium, High, and Very High.While Castillo-Martínez et al. (2024b) predominantly rely on descriptive statistical analyses, such as exploratory factor analysis (EFA), confirmatory factor analysis (CFA), correlation, and cluster analysis, our study takes a different trajectory. We not only focus on validating measurement instruments but also delve into assessing the internal consistency of subcompetencies. Additionally, we extend our approach by utilizing PLS-SEM, which combines principal component analysis and regression analysis to model relationships between latent and observed variables. This methodological choice affords us the opportunity to elucidate causal relationships between latent variables, thereby facilitating a deeper understanding of the theoretical model’s underlying structure and enabling the measurement and validation of latent constructs through observed indicators.
References
Abuabara, L., Paucar, A., Werne, K., and Villas, D. (2023). Enhancing systemic thinking by sharing experiences of reading literary fiction using causal mapping. J. Oper. Res. Soc. 75, 158–172. doi: 10.1080/01605682.2023.2180448
AlMunifi, A., and Aleyani, A. (2019). Knowledge and skills level of graduate civil engineers employers and Graduates' perceptions. Int. J. Eng. Pedagog. 9, 84–101. doi: 10.3991/ijep.v9i1.9744
Alt, D., Naamati, L., and Weishut, D. (2023). Competency-based learning and formative assessment feedback as precursors of college students' soft skills acquisition. Stud. High. Educ. 48, 1901–1917. doi: 10.1080/03075079.2023.2217203
Baena, J., Ramírez, M., Mazo, D., and López, E. (2022). Traits of complex thinking: a bibliometric review of a disruptive construct in education. J. Intelligence 10:3. doi: 10.3390/jintelligence10030037
Bancin, A., and Ambarita, B. (2019). Education model based on life skill (a Meta-synthesis). In: 4th Annual International Seminar on Transformative Education and Educational Leadership.
Borroto-Lopez, L. T. (2019). Universidad, comunidad y desarrollo sostenible. Una aproximación. Estudios del Desarrollo Social 7, 291–294. https://revistas.uh.cu/revflacso/article/view/6323
Carlos-Arroyo, M., Vázquez-Parra, J., Cruz-Sandoval, M., and Echaniz-Barrondo, A. (2023). Male chauvinism and complex thinking: a study of Mexican university students. Societies. 13:5. doi: 10.3390/soc13050104
Castillo-Martínez, I. M., and Ramírez-Montoya, M. S. (2022). eComplexity: Medición de la percepción de estudiantes de educación superior acerca de su competencia de razonamiento para la complejidad. Monterrey Available at: https://hdl.handle.net/11285/643622
Castillo-Martínez, I., Ramírez-Montoya, M., and Torres-Delgado, G. (2024a). Reasoning for complexity competency instrument (e-complexity): Content validation and expert judgment : Cogent Education. [In press].
Castillo-Martínez, I. M., Velarde-Camaqui, D., Ramírez-Montoya, M. S., and Sanabria-Z, J. (2024b). Psychometric properties of eComplexity scale. J Soc Stud Educ Res. [In press].
Cohen, J. (1988). Statistical power analysis for the behavioral sciences. 2nd Edn: New York: L. Erlbaum Associates.
Cruz-Sandoval, M., Vázquez-Parra, J. C., Carlos-Arroyo, M., and Del Angel-González, M. (2023). Complex thinking and its relevance in professional training: an approach to engineering students in a Mexican university. Int. J. Eng. Pedagog. 13, 100–119. doi: 10.3991/ijep.v13i3.36885
Cui, L., Zhu, Y., Qu, J., Tie, L., Wang, Z., and Qu, B. (2021). Psychometric properties of the critical thinking disposition assessment test amongst medical students in China: a cross-sectional study. BMC Med. Educ. 21:10. doi: 10.1186/s12909-020-02437-2
Diana, N., Latifah, S., Yuberti Komikesari, H., Rohman, M. H., and Tiyan, L. (2021). Developing an e-learning-based critical-thinking assessment as a physics learning evaluation media with Kahoot! Interactive quiz. J. Phys. Conf. Ser. 1796:012055. doi: 10.1088/1742-6596/1796/1/012055
Escobar-Pérez, J., and Cuervo-Martínez, A. (2008). Content validity and expert judgments. An approach to their use. Avances en Medición. 6, 27–36. https://www.humanas.unal.edu.co/lab_psicometria/application/files/9416/0463/3548/Vol_6._Articulo3_Juicio_de_expertos_27-36.pdf
Gallón, L. (2019). “Systemic thinking” in Quality education. Encyclopedia of the UN sustainable development goals. eds. F. W. Leal, A. Azul, L. Brandli, P. Özuyar, and T. Wall (Cham: Springer).
García-González, A., and Ramírez-Montoya, M. (2019). Higher education for social entrepreneurship in the quadruple helix framework: co-construction in open innovation. In: TEEM'19: Proceedings of the Seventh International Conference on Technological Ecosystems for Enhancing Multiculturality. (925–929).
Hair, J., Hult, G., Ringle, C., and Sarstedt, M. (2017). A primer on partial least squares structural equation modeling PLS-SEM. Los Angeles: SAGE.
Hapsari, S. (2016). A descriptive study of the critical thinking skills of social science at junior high school. J Educ Learn 10, 228–234. doi: 10.11591/edulearn.v10i3.3791
Henseler, J., Dijkstra, T., Sarstedt, M., Ringle, C., Diamantopoulos, A., and Straub, D. (2014). Common beliefs and reality about partial least squares: comments on Rönkkö & Evermann (2013). Organ. Res. Methods 17, 182–209. doi: 10.1177/1094428114526928
Horn, A., Scheffelaar, A., Urias, E., and Zweekhorst, M. (2022). Training students for complex sustainability issues: a literature review on the design of inter- and transdisciplinary higher education. Int. J. Sustain. High. Educ. 24, 1–27. doi: 10.1108/IJSHE-03-2021-0111
Hsu, T.-C., Chang, Y.-S., Chen, M.-S., Tsai, I.-F., and Yu, C.-Y. (2023). A validity and reliability study of the formative model for the indicators of STEAM education creations. Educ. Inf. Technol. 28, 8855–8878. doi: 10.1007/s10639-022-11412-x
Imjai, N., Aujirapongpan, S., and Yaacob, Z. (2024). Impact of logical thinking skills and digital literacy on Thailand’s generation Z accounting students’ internship effectiveness: role of self-learning capability. Int J Educ Res Open 6:100329. doi: 10.1016/j.ijedro.2024.100329
Izvorska, D. (2016). Educational researcher a model for development of students’ professional competence in technical universities. Educ. Res. 45, 961–974. https://www.researchgate.net/publication/314063005_Educational_Researcher_A_Model_for_Formation_and_Development_of_Students’_Technology_Competence_in_Technical_Universities
Jaaron, A., and Backhouse, C. (2018). Operationalisation of service innovation: a systems thinking approach. Serv Industr 38, 561–583. doi: 10.1080/02642069.2017.1411480
Juárez, L., and Tobón, S. (2018). Analysis of the elements implicit in the validation of content of a research instrument. Revista Espacios. 39:53. https://www.revistaespacios.com/cited2017/cited2017-23.pdf
Koerber, S., Mayer, D., Osterhaus, C., Schwippert, K., and Beate, S. (2015). The development of scientific thinking in elementary school: a comprehensive inventory. Child Dev. 86, 327–336. doi: 10.1111/cdev.12298
Koerber, S., and Osterhaus, C. (2019). Individual differences in early scientific thinking: assessment, cognitive influences, and their relevance for science learning. J. Cogn. Dev. 20, 510–533. doi: 10.1080/15248372.2019.1620232
Luna, J., Tobón, S., and Juárez, L. (2020). Sustainability-based on socioformation and complex thought or sustainable social development. Resourc Environ Sustain. 2:100007. doi: 10.1016/j.resenv.2020.100007
Mohd Rahim, N. I., Iahad, N. A., Yusof, A. F., and Al-Sharafi, M. A. (2022). AI-based chatbots adoption model for higher-education institutions: a hybrid PLS-SEM-neural network modeling approach. Sustain. For. 14, –726. doi: 10.3390/su141912726
Nagahi, M., Jaradat, R., Davarzani, S., Nagahisarchoghaei, M., and Goerger, S. (2020). The impact of systems thinking skills and proactive personality on academic performance of engineering students abstract. In: ASEE'S Virtual Conference.
Ossa-Cornejo, C., Palma-Luengo, M., Lagos-San Martín, N., and Díaz-Larenas, C. (2018). Critical and scientific thinking assessment in preservice teachers at a Chilean university. Rev. Electron. Educ. 22, 1–18. doi: 10.15359/ree.22-2.12
Ramírez-Montoya, M., Castillo-Martínez, I., Sanabria, J., and Miranda, J. (2022). Complex thinking in the framework of education 4.0 and open innovation—a systematic literature review. J. Open Innov.: Technol. Mark. Complex. 8:4. doi: 10.3390/joitmc8010004
Rebs, T., Brandenburg, M., and Seuring, S. (2019). System dynamics modeling for sustainable supply chain management: a literature review and systems thinking approach. J. Clean. Prod. 208, 1265–1280. doi: 10.1016/j.jclepro.2018.10.100
Reynders, G., Lantz, J., Ruder, S. M., Stanford, C. L., and Cole, R. S. (2020). Rubrics to assess critical thinking and information processing in undergraduate STEM courses. IJ STEM Ed 7:9. doi: 10.1186/s40594-020-00208-5
Riahi, S. (2022). Strengthening the teaching of soft skills in the pedagogical architecture of Moroccan universities. Int. J. Eng. Pedagog. 12, 47–62. doi: 10.3991/ijep.v12i4.22329
Rigdon, E. (2012). Rethinking partial least squares path modelling: in praise of simple methods. Long Range Plann. 45, 341–358. doi: 10.1016/j.lrp.2012.09.010
Rios, F., and Suarez, C. (2017). Intercultural contact, complex thinking, and intergroup attitudes. Group Process. Intergroup Relat. 20, 238–251.
Rutherford-Hemming, T. (2018). “Content validity ratio” in The SAGE encyclopedia of educational research, measurement, and evaluation. ed. B. Frey New York: (SAGE Publications), 397–398.
Saienko, N., Olizko, Y., and Cunha, A. (2021). Perceptions of fostering creative thinking skills in ESP classrooms in Ukraine and Portugal. Int. J. Eng. Pedagog. 11, 23–41. doi: 10.3991/ijep.v11i4.20129
Sellars, M., Fakirmohammad, R., Bui, L., Fishetti, J., Niyozov, S., Reynolds, R., et al. (2018). Conversations on critical thinking: can critical thinking find its way forward as the skill set and mindset of the century? Educ. Sci. 8:205. doi: 10.3390/educsci8040205
Sherblom, S. (2017). Complexity-thinking and social science: self-organization involving human consciousness. New Ideas Psychol. 47, 10–15. doi: 10.1016/j.newideapsych.2017.03.003
Silva, C., and Iturra, C. (2021). A conceptual proposal and operational definitions of the cognitive processes of complex thinking. Think. Skills Creat. 39:100794. doi: 10.1016/j.tsc.2021.100794
Silva, C., and Iturra, C. (2023). Development and validation of the complex thinking assessment. Think. Skills Creat. 48:101305. doi: 10.1016/j.tsc.2023.101305
Soper, D. S. . (2024) Structural equation model sample size calculator. Available at: https://www.analyticscalculators.com.
Straková, Z., and Cimermanová, I. (2018). Critical thinking development-a necessary step in higher education transformation towards sustainability. Sustainability 10, 1–18. doi: 10.3390/su10103366
Suryansyah, A., Kastolani, W., and Somantri, L. (2021). Scientific thinking skills in solving global warming problems. IOP Conf Series Earth Environ Sci 683:012025. doi: 10.1088/1755-1315/683/1/012025
Tecnologico de Monterrey. (2023). SEL4C. Aviso de Privacidad. Available at: https://tec.mx/es/aviso-de-privacidad-sel4c
Tobón, S., and Luna, J. (2021). Complex thinking and sustainable social development: validity and reliability of the COMPLEX-21 scale. Sustain. For. 13:12. doi: 10.3390/su13126591
Vázquez-Parra, J. C., Alfaro-Ponce, B., Guerrero-Escamilla, J., and Morales-Maure, L. (2023). Cultural imaginaries and complex thinking: impact of cultural education on the development of perceived achievement of complex thinking in undergraduates. Soc. Sci. 12:272. doi: 10.3390/socsci12050272
Vázquez-Parra, J., Castillo-Martínez, I., Ramírez-Montoya, M., and Millán, A. (2022a). Development of the perception of achievement of complex thinking: a disciplinary approach in a Latin American student population. Educ. Sci. 12:5. doi: 10.3390/educsci12050289
Vázquez-Parra, J., Cruz-Sandoval, M., and Carlos-Arroyo, M. (2022b). Social entrepreneurship and complex thinking: a bibliometric study. Sustain. For. 14:20. doi: 10.3390/su142013187
Wale, B. D., and Bishaw, K. S. (2020). Effects of using inquiry-based learning on EFL students’ critical thinking skills. Asian. J. Second. Foreign. Lang. Educ. 5:9. doi: 10.1186/s40862-020-00090-2
Westland, J. C. (2010). Lower bounds on sample size in structural equation modeling. Electron. Commer. Res. Appl. 9, 476–487. doi: 10.1016/j.elerap.2010.07.003
Widyastuti, P., Hadi, S., Daryono, R. W., and Abd Samad, N. B. (2023). The mediation role of university environment in the relationship between self-efficacy and family environment on entrepreneurial education interest: a PLS-SEM approach. Indones J Learn Adv Educ 5, 295–310. doi: 10.23917/ijolae.v5i3.22015
Wijaya, T. T., Cao, Y., Weinhandl, R., Yusron, E., and Lavicza, Z. (2022). Applying the utaut model to understand factors affecting micro-lecture usage by mathematics teachers in China. Mathematics 10:1008. doi: 10.3390/math10071008
Wijaya, T. T., Yu, B., Xu, F., Yuan, Z., and Mailizar, M. (2023). Analysis of factors affecting academic performance of mathematics education doctoral students: a structural equation modeling approach. Int. J. Environ. Res. Public Health 20:4518. doi: 10.3390/ijerph20054518
Wild, S., and Schulze, L. (2021). Re-evaluation of the D21-digital-index assessment instrument for measuring higher-level digital competencies. Stud. Educ. Eval. 68:100981. doi: 10.1016/j.stueduc.2021.100981
Zimmerman, C., and Croker, S. (2014). A prospective cognition analysis of scientific thinking and the implications for teaching and learning science. J. Cogn. Educ. Psychol. 13, 245–257. doi: 10.1891/1945-8959.13.2.245
Appendix 1: Shapiro–Wilk test
Keywords: professional education, educational innovation, future of education, complex thinking, structural equations, PLS-SEM, higher education
Citation: Vázquez-Parra JC, Henao-Rodriguez LC, Lis-Gutiérrez JP, Castillo-Martínez IM and Suarez-Brito P (2024) eComplexity: validation of a complex thinking instrument from a structural equation model. Front. Educ. 9:1334834. doi: 10.3389/feduc.2024.1334834
Edited by:
Diana Hernández Montoya, Universidad Estatal a Distancia, Costa RicaReviewed by:
Tommy Tanu Wijaya, Beijing Normal University, ChinaAli Murad Syed, University of Bahrain, Bahrain
Copyright © 2024 Vázquez-Parra, Henao-Rodriguez, Lis-Gutiérrez, Castillo-Martínez and Suarez-Brito. This is an open-access article distributed under the terms of the Creative Commons Attribution License (CC BY). The use, distribution or reproduction in other forums is permitted, provided the original author(s) and the copyright owner(s) are credited and that the original publication in this journal is cited, in accordance with accepted academic practice. No use, distribution or reproduction is permitted which does not comply with these terms.
*Correspondence: Paloma Suarez-Brito, cGFsb21hLnN1YXJlekB0ZWMubXg=
†ORCID: José Carlos Vázquez-Parra, orcid.org/0000-0001-9197-7826
Linda Carolina Henao Rodriguez, orcid.org/0000-0001-9414-001X
Jenny Paola Lis-Gutiérrez, orcid.org/0000-0002-1438-7619
Isolda Margarita Castillo-Martínez, orcid.org/0000-0003-3968-5775
Paloma Suarez-Brito, orcid.org/0000-0002-7169-6215