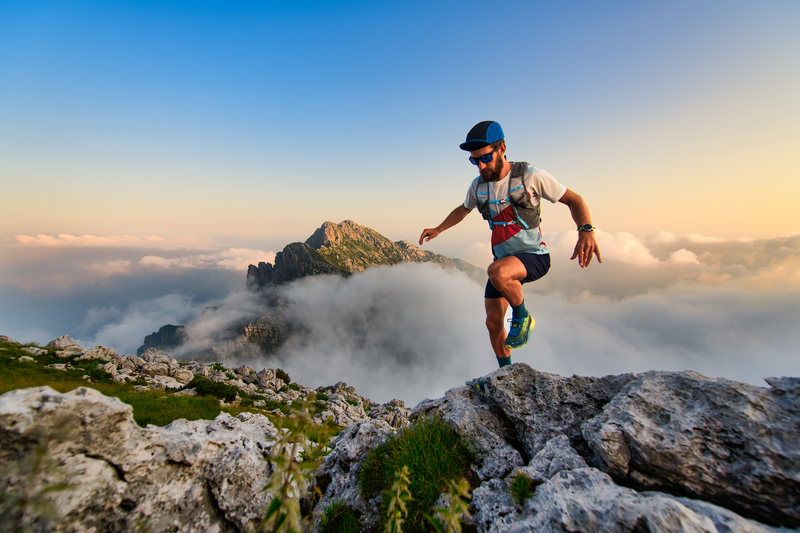
94% of researchers rate our articles as excellent or good
Learn more about the work of our research integrity team to safeguard the quality of each article we publish.
Find out more
ORIGINAL RESEARCH article
Front. Educ. , 06 February 2024
Sec. Teacher Education
Volume 9 - 2024 | https://doi.org/10.3389/feduc.2024.1328864
In this paper, we examined whether it is possible to predict German teacher students’ study specialization (i.e., type of school) from data on their personality (in terms of the Big Five) and vocational interests (in terms of the RIASEC model) using multinomial logistic regression. Gender and intelligence were included as control variables. Two studies are reported. The first study (N = 1,145 teacher students) took place at a German university, while Study 2 used data from the German National Education Panel Study (NEPS; data from N = 944 teacher students). In both studies, it was found that the model fit increased significantly after adding personality and vocational interests as predictors (compared with a baseline model containing only gender and intelligence as predictors). Findings show that the model of vocational interests and the Big Five personality model can be used to differentiate between teacher students with different specializations. In the long run, results like these could be used in the field of counseling to help clients who are determined to become a teacher but unsure about which specialization might be most appropriate for them.
Choosing a career when graduating from high school is one of the most important decisions a person will ever have to make (Porter and Umbach, 2006). For many high school graduates, their career choice will require them to choose a program of study at a university or college. This decision has strong implications for career paths; dissatisfaction and detrimental effects on well-being (even burnout) might result from choosing a college (Gilbreath et al., 2011) or a career path (Tong et al., 2015) that does not fit one’s individual needs. Finding the right study program for their clients is an important task for high school counselors (Cimsir, 2019; University of Massachusetts Global, 2021). However, in order to give sound advice, counselors need reliable information on how to use individual interests and other individual characteristics when giving advice. For example, Moakler and Kim (2014) investigated individual characteristics that made choosing a study program from the fields of science, technology, engineering, or mathematics (STEM) more likely. The authors argued that their findings could help counselors find the right study program for their clients and bring more talented people into STEM fields.
Reasons to pursue a career in teaching have been examined thoroughly. While earlier research (e.g., Schutz et al., 2001) relied on qualitative data, later studies often used standardized questionnaires, especially the FIT-Choice instrument (Watt and Richardson, 2007) which is based on the expectancy-value approach (cf. Wigfield and Eccles, 2000). Using that instrument in an international comparison study, Watt et al. (2012) examined the reasons to become a teacher in a sample of teacher students from the US, Australia, Germany, and Norway. For example, in all countries, perceived teaching abilities and the intrinsic value of that career were among the most highly rated motivations. Personal utilitarian motivations (e.g., job security or time for family) were rated as less important. Results from these and related studies can be used by counselors to determine whether a career in teaching is a good choice for their clients. However, after choosing a career in teaching in general, individuals typically have to decide which type of school they want to teach in. Results on differences among teachers or teacher students are quite rare. Retelsdorf and Möller (2012) examined differences among teacher students intending to teach at different types of school in Germany using a questionnaire similar to the FIT-Choice. For example, they found that students intending to teach at primary schools indicated more pedagogical motives (e.g., working with children) than those intending to teach at secondary schools. Regarding subject-related interests as a motive (interest in the chosen school subjects), the result pattern pointed in the opposite direction with students intending to teach at secondary schools scoring higher. Weiß et al. (2009) also compared students intending to teach at different types of (German) schools using a self-developed questionnaire.
A different line of research deals with individual characteristics (in particular personality and vocational interests). Several studies investigated individual characteristics of teacher students and students not intending to become a teacher (e.g., Neugebauer, 2013; Savage et al., 2021). Findings on differences in individual characteristics among teacher students by the type of school are rare. In one of the few studies on this issue, Klusmann et al. (2009) examined differences in personality and vocational interests between German teacher students intending to teach at different types of schools. Substantial differences were found on the Openness and Agreeableness dimensions of personality as well as the Social and Investigative dimensions of vocational interests; however, several types of schools were grouped together in the comparisons. More research was done on differences in personality (Hartmann and Ertl, 2021) and vocational interests (Kaub et al., 2014; Hartmann et al., 2022) between teacher students intending to teach different subjects. Nonetheless, the question whether a career decision (i.e., decision for a specific type of school or school subject) could be predicted (i.e., explained) by differences in individual characteristics has only been examined by Klusmann et al. (2009).
The aim of the present studies was to examine individual differences in vocational interests and personality between German teacher students intending to teach at different types of school. We hope that this reduces the scarcity of research on this issue and provides better insights into the decision on the type of school made by teacher students. We also examined whether individual differences in vocational interests and personality could be used to predict the type of school that teacher students aim to teach in. These results might later be used to help counselors to find the right type of school for their clients.
Two studies are reported. In Study 1, data from our institution is used; Study 2 draws on data from the German National Educational Panel Study (NEPS; FDZ-LIfBi, 2023). Other studies have already demonstrated that students’ study program can be predicted by vocational interests and personality (e.g., Larson et al., 2010; Pinxten et al., 2015), but these studies did not include teacher students with the exception of the study by Klusmann et al. (2009). So, our aim was to examine whether this approach of predicting can be applied to teacher students using a wide range of types of schools as dependent variable.
According to John Holland’s model of the structure of interests, individuals’ vocational interests can be described with six distinct dimensions (Holland, 1966; Darcy and Tracey, 2007). Each dimension entails preferences for specific work environments and tasks and can be characterized and operationalized by a set of activities. Despite the availability of competing models (see, e.g., Nagy et al., 2010), Holland’s model is the most widely used of the models representing the structure of vocational interests (Ertl et al., 2022). One reason for this popularity is the assumption that environments can also be described using these six dimensions (Darcy and Tracey, 2007). Holland further proposed that interests guide individuals’ acquisition of skills in such a way that they learn the abilities that make it easier for them to execute activities that are in line with their interests (Darcy and Tracey, 2007). Holland (1966) described the six interest dimensions as follows (see Kaub et al., 2016 for a more detailed description): Realistic interests typically involve activities that require the ordered or systematic manipulation of objects or tools. Individuals with high Realistic interests typically possess manual or technical competencies and might work as craftspeople or engineers. Individuals with high Investigative interests prefer activities that require the observation and investigation of things. They often possess mathematical or scientific competencies and might work as scientists. Individuals with strong Artistic interests, by contrast, are attracted by activities that can be executed in an ambiguous or unsystematic way and might possess competencies in the fields of language or writing. Social interests lead individuals to work in an environment that requires interactions with other people. These individuals often possess competencies that support human relationships (e.g., in the field of education) and might work as a teacher. Individuals with strong Enterprising interests have a preference for activities that entail leadership or persuasion. They typically possess interpersonal and persuasive competencies and might work as a manager or run their own business. Individuals with pronounced Conventional interests are mainly interested in activities that require the ordered and systematic processing of data (e.g., documentation). These individuals typically have high administrative competencies and might choose clerical or administrative work.
Based on the first letter of each dimension (Realistic, Investigative, Artistic, Social, Enterprising, and Conventional), this model is often called the RIASEC model. Due to the fact that environments can be described using the same six dimensions (Darcy and Tracey, 2007), this model can be applied in counseling contexts. In that case, individual and occupational interest profiles have to be compared to determine fit [for an overview of older comparison indices, see Brown and Gore (1994)]. Kroustalis et al., 2010 describe a later developed index. Nagy et al. (2010) could confirm the factorial validity of the RIASEC model using confirmatory factor analysis methodology.
In the following, we discuss research on teacher students’ vocational interests. Vocational interests of teacher students have mainly been investigated in the German context so far. Therefore, we briefly explain the German system of teacher education. For the academic part of teacher education in Germany, teacher students enroll in a teacher training program at university and select their specialization in terms of the type of school they want to teach in as well as (typically) two school subjects they intend to teach later. Typically, the candidates can choose one of five types of schools: primary schools (Grades 1 to 4 in Germany; Grade 1 is the first year of mandatory schooling), special schools (schools for students who have special educational needs, e.g., due to learning difficulties), “Hauptschule”/“Realschule” (Grades 5 to 10; in the following, the term K-10 is used as an abbreviation), “Gymnasium” (Grades 5 to 13; in the following: K-13), or vocational schools (students who choose vocational training have to attend this school in addition to getting trained on the job). The teacher education program entails courses that prepare teacher students to teach the two selected school subjects as well as general educational content courses that prepare them to work as a teacher. Teacher students in Germany study for around 5 years before starting a paid traineeship (“Referendariat”) of typically 18 months (duration differs by federal state; range: 12 to 24 months) to obtain the required certification. A state certification is awarded after successful completion of the study program (in some federal states, students also earn a Bachelor’s and a Master’s degree during their studies).
The model of vocational interests proposed by Holland (1966) can be understood in terms of the person-environment fit approach which has already been applied to student populations (e.g., Etzel and Nagy, 2016). The premise is that performance and well-being depend on the fit (congruence) between individual interests and the environment (e.g., Brown and Gore, 1994). Therefore, it is assumed that individuals choose study programs and career paths that fit their individual characteristics (i.e., vocational interests) or withdraw (i.e., change their study program) if they do not see fit. The occupation as a teacher differs by the type of school; for example, primary school teachers are expected to teach a multitude of subjects in one or two classes while teachers in secondary schools (K-10 and K-13 school) are specialized in specific subjects (Blömeke, 2006). These differing requirements are mirrored in the teacher training programs at university. For example, study programs for future secondary school teachers typically focus on two subjects in Germany while study programs for primary school teachers offer broader content. These differences in the characteristics of study programs (and later work environments) should attract individuals with different characteristics. Klusmann et al. (2009) reported differences in vocational interests between German teacher students striving to teach at different types of schools; however, students intending to teach at primary schools, special schools, and K-10 schools were treated as one group and compared with those intending to teach at K-13 schools. The authors found that future K-13 teachers showed higher Investigative interests, but lower Social interests than the other group. Roloff Henoch et al. (2015) and Hartmann et al. (2022) also examined vocational interests among German teacher students, but focused on differences between teacher students who would later teach a STEM subject and students enrolled in study programs preparing for other school subjects. In the latter study, it was found that future STEM teachers had higher Realistic and Investigative and lower Artistic interests than teacher students intending to teach non-STEM subjects at school. Relationships between the combination of school subjects chosen and the vocational interest dimensions among German teacher students were examined by Kaub et al. (2012). They found significant main effects on four of the six vocational interest dimensions. For example, students intending to teach two STEM subjects scored significantly higher on the Investigative dimension than the other groups of teacher students. However, the authors did not examine whether vocational interests differed as a function of the type of school the students intended to teach at later, and their sample did not include future primary or special school teachers. Similar results were reported in later publications by the same research group (Kaub et al., 2014, 2016). Regarding research on teacher students’ vocational interests, it can be summarized that differences in vocational interests between teacher students intending to teach at different types of schools or striving to teach different (combinations of) school subjects have been found. However, research examining differences in vocational interests across teacher students intending to teach at different types of schools is quite rare.
Another important way to capture individual differences is through personality. Regarding the description of individual differences in personality, consensus has grown in recent decades that most important individual differences can be described in terms of the Big Five: Extraversion, Agreeableness, Conscientiousness, Neuroticism, and Openness (Soto and John, 2017).
Personality has often been understood as predictor of performance in educational settings; especially the Conscientiousness dimension has been found to be a predictor of performance (O’Connor and Paunonen, 2007). However, personality has not that often been examined in terms of person-environment fit (e.g., Etzel and Nagy, 2016). Applying that approach to career choice, it is assumed that individuals choose study programs which are in line with their personality or withdraw from study programs as soon as they realize that it does not fit their personality (cf. Vedel, 2016). Therefore, it can be assumed that differences in personality can be found between individuals enrolled in different study programs. In a systematic review, Vedel (2016) summarized 12 studies that examined differences on the Big Five dimensions between students enrolled in different study programs. Most studies included reported such differences. Vedel (2016) argues that these findings could be used in guiding students to find the study program that fits their personality best. As described above, German teacher training programs differ by the type of school to mirror differences in the later work environments. So, individuals with different personality characteristics should be attracted by the different teacher training programs. Differences in personality among German teacher students can therefore be expected and were examined by Klusmann et al. (2009); the authors reported that students intending to teach at primary schools, special schools, or K-10 schools scored lower on Openness and higher on Agreeableness than students intending to teach at K-13 schools. Hartmann and Ertl (2021) examined differences on the Big Five dimensions among German teacher students striving to teach different subjects. The most notable differences were reported for the Openness dimension where one finding was that teacher students intending to teach German had higher scores than those intending to teach mathematics.
Research has shown that the Big Five and the RIASEC dimensions share a substantial amount of variance. However, the amount of unshared variance is large enough to warrant the simultaneous use of both constructs (de Fruyt and Mervielde, 1996). Individual differences in characteristics between tertiary education students from different study programs can be used to predict an individual’s study program. The study program then becomes the dependent variable. To our knowledge, there are only a few studies that have chosen that approach. The first study to systematically examine this issue was conducted by de Fruyt and Mervielde (1996) using a sample of Belgian university graduates. Students were grouped by their study program, resulting in a total of 21 groups. Predictor variables were personality in terms of the Big Five and vocational interest scores. Discriminant function analysis was used to determine whether participants’ study program could be predicted from their vocational interests and personality. The instruments used allowed for the calculation of scores for broad domains as well as smaller facets of personality and vocational interests. A combination of the 30 personality facets and the 18 vocational interest scales was most effective (nearly 51% of participants were classified correctly). When the broader domains for personality and vocational interests were used (these predictors are equivalent to the predictor variables used in the present research), nearly 29% of the participants could be classified correctly. Given that the likelihood of correct classification by chance was around 5%, this means that the combination of predictors proved useful.
In a similar study, Larson et al. (2010) examined whether U.S. undergraduate students’ family of majors could be predicted from personality, vocational interests, and self-efficacy regarding occupational activities that could be mapped onto the six dimensions of Holland’s model. The Multidimensional Personality Questionnaire (for a description, see, e.g., Patrick and Kramer, 2017) was used to assess personality. The affiliation with one of nine families of majors was the dependent variable in a set of discriminant function analyses; gender was entered as a control (predictor) variable. A combination of gender, personality, vocational interests, and self-efficacy led to the correct classification of 53% of the participants (exceeding the likelihood of correct classification by chance, which was 11%). By successively adding predictor variables, the authors showed that including vocational interests and self-efficacy significantly improved the prediction over a model containing gender and personality as the only predictors.
In a study with students from German institutions, Päßler and Hell (2012) examined whether the field of study (study programs were assigned to one of four clusters by the authors) could be predicted from vocational interests and ability scores (scores on verbal, numerical, and spatial ability were available). Using vocational interests alone, the prediction of the field of study was correct in 61% of the cases while the likelihood of correct classification by chance was only 25%. When vocational interests and ability measures were used together, nearly 64% of the cases could be classified correctly.
Using multinomial logistic regression, a recent study of Belgian university students (Pinxten et al., 2015) examined whether students’ field of study (study programs were grouped into eight clusters by the authors) could be predicted from a range of variables including not only vocational interests, but also school subject uptake in high school as well as knowledge of mathematics and Dutch. The authors found that the prediction of field of study could be improved by adding vocational interests as predictor variables. However, the instrument used to assess vocational interests did not rely on the RIASEC model, so the results can be compared to the results of other studies only to a limited extent. Nonetheless, they confirm the important role of vocational interests in the selection of a study program.
One of the analyses conducted by Klusmann et al. (2009) entailed the prediction of study program among German students using multinomial logistic regression. Students were assigned to one of four groups: (1) teacher students intending to teach at primary schools, K-10 schools or special schools, (2) teacher students intending to teach at K-13 schools, (3) non-teaching students at universities and (4) non-teaching students at universities of applied sciences (German “Fachhochschulen”) or vocational colleges (German “Berufsakademie”). Aside from vocational interests and personality, the final grade from K-13 school, intelligence, performance test scores in mathematics and English, the socio-economic status and gender were included as predictors. The coefficients of five vocational interest dimensions reached significance for at least two comparisons.
The aim of the present research was to examine whether vocational interests and Big Five personality dimensions predict teacher students’ specialization in terms of the type of school.1 Furthermore, we included gender and intelligence as control variables. There is research evidence suggesting that the intelligence scores of teacher students differ by the type of school they intend to teach in Klusmann et al. (2009) and by the combination of school subjects (Kaub et al., 2012). Furthermore, in a sample of students (including teacher students), Roloff Henoch et al. (2015) found that students studying at least one STEM subject had significantly higher cognitive abilities than students enrolled in other study programs. Additionally, the gender ratio among German teacher students differs by the type of school (Statistisches Bundesamt, 2021) and by the school subjects (Roloff Henoch et al., 2015).
Two studies are reported. In study 1, data from our institution is used; Study 2 draws on data from the German National Educational Panel Study (NEPS; Blossfeld and Roßbach, 2019). To make sure that our findings from Study 1 are relevant in the context of counseling, we included only data from students with scores that were above the midpoint on the satisfaction with course contents scale. A similar case selection criterion was applied by Päßler and Hell (2012).2 Nonetheless, satisfaction with course content does not necessarily lead to successful completion of the study program. Furthermore, data was collected at a single institution. Study 2 can tackle these limitations as data used for Study 2 was collected at several institutions and data is longitudinal. So, it was possible to include only data from those students who completed their studies successfully without changing the intended type of school. The analytical approach will be the same in both studies. Using multinomial logistic regression, we computed three models to investigate unique effects (incremental contributions of specific predictors beyond the effects of the other predictors): The first model contained only gender and intelligence as predictors. The second model additionally contained personality variables, and the third model additionally contained vocational interest variables.
The hypotheses apply to both studies. Hypothesis 1 stated that the rate of correct classification would increase with each group of predictors (i.e., personality and vocational interest variables) additionally included. As vocational interests were the focus of this research, these variables were added last to examine their incremental effects. As in previous studies (e.g., Pinxten et al., 2015), interactions among the predictor variables were not included as there was no theoretical foundation for including them. Hypotheses 2a, 2b, 3a, and 3b referred to the personality variables (i.e., the dimensions of the personality model). Based on the findings by Klusmann et al. (2009), we expected the personality dimensions Openness (Hypothesis 2a) and Agreeableness (Hypothesis 2b) to be important predictors of the type of school in the multinomial logistic regression analysis (a variable is considered to be an important predictor in this context if it’s coefficients reach significance for two or more comparisons). Additionally, we expected future K-13 school teachers to score higher on Openness (Hypothesis 3a) and lower on Agreeableness (Hypothesis 3b) than students intending to teach at other types of schools. Hypotheses 4a, 4b, 5a and 5b referred to the vocational interest variables. Also based on Klusmann et al. (2009), we expected the Investigative (Hypothesis 4a) and Social (Hypothesis 4b) dimensions to be important predictors of the type of school. Additionally, we expected future K-13 school teachers to score higher on the Investigative (Hypothesis 5a) and lower on the Social dimension (Hypothesis 5b) than students intending to teach at other types of schools. The study by Klusmann et al. (2009) did not include students intending to teach at vocational schools, so our hypotheses do not apply to this group.
Study 1 took place at a German university. The aim was to examine whether the hypotheses could be confirmed before they would be applied to a sample from multiple institutions.
Data were collected using paper-and-pencil questionnaires during the 2018–2019 winter term at a German university. Participants of courses for teacher students were asked to complete the questionnaire voluntarily during their regular course time; no compensation was offered. Among other measures, we collected data on personality, vocational interests, intelligence, satisfaction with course contents, and some demographic information. Overall, N = 1,789 students completed questionnaires. However, n = 228 participants indicated that they did not intend to become a teacher (these courses are also open to students from a wide range of study programs), so data from these cases were excluded from further analyses. Further data from n = 16 cases had to be discarded as they were not decided yet regarding their future type of school or the information given regarding the school subjects selected was implausible (e.g., the two subjects were identical). Data from n = 5 participants who had indicated their gender to be diverse were also excluded because this group size was too small for analysis. Further data from n = 12 participants were excluded because these participants had given wrong answers on more than 15 (out of 64) items on an intelligence screening instrument (which can be regarded as careless responding). Next, data from n = 202 participants with missing data regarding their satisfaction with course content, gender or the type of school they intend to teach in were removed. In the last step of data screening, data from participants whose satisfaction with course contents score was below the midpoint of the scale (5.5 as the scale ranged from 1 to 10) were excluded from the analysis, reducing the sample size by a further n = 160 participants. Missing data on personality, vocational interest and intelligence variables3 was handled using multiple imputation (see the data analysis section for details). As the multiple imputation procedure chosen required that at least one item of each scale relevant in the analyses was not missing, data from n = 16 participants had to be discarded in this step. After multiple imputation, n = 5 cases were identified as multivariate outliers and hence removed from all further analyses (see the data analysis section for details). Hence, the available sample consisted of N = 1,145 participants.4 The majority (74%) of the final sample was female; the average age was M = 21.47 years (SD = 2.87; Range: 16–38, information was missing from 8 participants). Out of these, 83% were enrolled in a Bachelor’s program, and the remaining 17% were enrolled in a Master’s program. Most students intended to teach at a primary school (44%)5 or a K-13 school (German Gymnasium; 27%). Around 18% intended to teach at a special school (German Förderschule), approximately 9% at a K-10 school (German Hauptschule/Realschule), and around 2% at a vocational school (German Berufsbildende Schule). The vast majority of students were in their first year (around 59%), around 15% were in their second year, and around 7% were in their third year. Around 10% were in their fourth year (Master level students were asked to include the number of years they had studied to obtain their Bachelor’s degree), and around 5% were in their fifth year. The remaining students were in their sixth year or had already studied for more than 6 years (the standard period of study to obtain a Bachelor’s and a Master’s degree is 5 years at this university).
To assess vocational interests, we used a 48-item instrument that was developed for research purposes (Astrid Schütz, personal communication, October 02, 2018). Its advantages are that it can be used without license fees, and it is somewhat shorter than established German instruments, such as the 60-item AIST-3 (“Allgemeiner Interessen-Struktur-Test” [General Interest-Structure-Test]; Bergmann and Eder, 2019). Each item on the instrument described a work activity6 (sample item for the Social dimension: “to manage other people’s affairs,” translation by the authors), and participants were asked to indicate how much they would be interested in that activity on a 5-point Likert scale (1 = not at all to 5 = very interested). The instrument included eight items for each of the six RIASEC scales; for each scale, the mean of the corresponding items was computed and used as an individual’s interest score. An unpublished previous study (N = 149) showed that the internal consistency (Cronbach’s α) of the six RIASEC scales ranged from α = 0.81 to α = 0.95. Correlations between the scales from this instrument and the AIST-3 scales (Bergmann and Eder, 2019) were computed as part of this previous study; the correlations between the corresponding scales (convergent correlations) ranged from 0.77 to 0.92 (all correlations were significant after the alpha-level adjustment). Internal consistencies were acceptable in the present Study 1 as well: McDonald’s Omega ranged from ω = 0.79 to ω = 0.88.
The German short version of the Big Five Inventory (Rammstedt and John, 2005) was used to assess personality. This instrument measures five broad domains of personality with 21 items (k = 4 items for each domain except for Openness with k = 5). Each item consisted of a statement (e.g., “I get nervous easily” for the Neuroticism dimension, translation by the authors) and had to be answered on a 5-point rating scale. Results regarding internal consistency (Cronbach’s alpha) reported by the authors (i.e., α between 0.59 and 0.86) can be considered acceptable as this is a short version, and the reliability is usually negatively affected by the length of the scale (Niemi et al., 1986). They also reported part-whole correlations between the scales from the short version and the corresponding scales from the long version of the instrument (k = 45) that ranged from r = 0.85 to r = 0.95, supporting the validity of the instrument. In the present Study 1, McDonald’s Omega ranged from ω = 0.66 to ω = 0.84. As in the publication by Rammstedt and John (2005), the Agreeableness scale showed the lowest internal consistency.
To assess intelligence efficiently, the mini-q instrument (Baudson and Preckel, 2016) was used. The instrument consists of 64 items. Each item consists of a figure and an accompanying statement. Each figure shows three geometric forms (a circle, a triangle and a square) with the triangle being in between the two other objects. In each figure, the triangle is closer to one of the two other objects and the participants are told that two objects being closer to each other is an indication of mutual liking or preference. The accompanying statement (e.g., “the triangle prefers the circle,” translation by the authors) describes a possible relationship between the objects and participants are asked to indicate whether the statement matches the figure (i.e., whether it is true). Following the instructions by Baudson and Preckel (2016), participants were given 3 min to complete as many items as possible; the number of correctly answered items was used as the intelligence score in further analyses (see Baudson and Preckel, 2016 for sample items). Regarding convergent validity, the authors reported correlations with the CFT 20-R (Weiß, 2008) of 0.51 (0.60 when corrected for attenuation) and with a newly developed short instrument based on the established German language instrument I-S-T 2000 R (Amthauer et al., 2001) of 0.67 (0.74 when corrected for attenuation).
To examine satisfaction with course content, the respective subscale (k = 3) of the instrument developed by Spies et al. (1996) was used. Participants were asked to indicate how well each item (sample item: “Overall, I am satisfied with my current course of study,” translation by the authors) applied to them on a 10-point rating scale. In the sample of the present Study 1, McDonald’s ω was 0.79. For participants who had omitted any of the three items, no mean score was computed.
Participants were also asked to provide some demographic information. Data on gender and selected type of school (primary school, special school, K-13 school, K-10 school, and vocational school) were used in the analyses.
All computations were conducted using the R statistical environment (R Core Team, 2023). Missing data were handled by multiple imputation (see, e.g., Enders, 2010) using the R-package mice (van Buuren and Groothuis-Oudshoorn, 2011). As only a small percentage of the data was missing (less than 2%), we decided to use five imputations (i.e., five datasets were created). So, we did not follow the recommendation by van Buuren (2018) to set the number of imputations to the percentage of missing data; however, we did not want to use only two imputations. To preserve the relationships between item variables and scale variables (scale variables typically represent the mean of the corresponding item variables), we followed the three-step-imputation approach described by Enders (2010, pp. 271–272). First, before the imputation phase, temporary scale (mean) scores were computed by averaging the relevant item scores available. As the mice package only accepts complete predictor variables in the imputation process, data from participants who did not answer any item of a certain scale had to be discarded. Second, during the imputation phase, these temporary scale scores were used to impute missing data on item variables and the intelligence test score, creating multiple datasets. In the third step, those temporary scale scores were deleted from each imputed dataset and the final scale scores were computed based on the corresponding item variables in each imputed dataset. All imputed variables (the item variables measuring vocational interests and personality as well as the intelligence test score variable) were metric; predictive mean matching was used as imputation method (see van Buuren, 2018). Only variables without any missing values were used as predictors for the imputation phase (these were type of school, gender, the three satisfaction with course content item variables and the temporary scale scores of the vocational interests and personality instruments).
Next, data were screened for multivariate outliers for each dataset separately. As described by Tabachnick and Fidell (2014, p. 108), the Mahalanobis distance was computed for the set of variables including all predictor variables (interval scale variables were z-standardized for data screening). Mahalanobis distances follow a chi-square distribution with degrees of freedom equal to the number of variables (13 in our case); cases significant at the p < 0.001 level can be considered outliers (Tabachnick and Fidell, 2014, p. 133). The same five cases met this criterion in each dataset; data from these cases were excluded from each dataset.
For the multinomial logistic regression analyses, the categorical predictor variable gender was coded 0/female and 1/male (i.e., female was the reference category). The nnet package [version 7.3–19, see Venables and Ripley (2002)] was used to compute the multinomial logistic regression analyses. The effect of a predictor variable in (multinomial) logistic regression is typically interpreted in terms of the odds ratio (OR), which indicates the change in the odds of the target category of the outcome variable for a 1-unit increase in the predictor variable (controlling for all other predictor variables in the model). If the OR is above (below) 1, the odds of the outcome category in question increase (decrease) as the predictor variable increases. For example, an OR of 2.75 indicates that the odds of the target category (i.e., the ratio of the probability of the target category over the probability of the reference category) increase by a factor of 2.75 for a 1-unit increase in the predictor variable (Tabachnick and Fidell, 2014, p. 507). Primary school was selected as the reference category because it was the largest group of students. Pooling of the results from the five imputed datasets depended on the statistic in question.7
Table 1 presents mean scores and standard deviations for the relevant variables for each type of school and for the whole sample. As can be seen in Table 1, significant group differences were found for three dimensions of personality as well as for all dimensions of the model of vocational interests, except for the Realistic dimension. Intelligence did not differ between school types, but gender did. A table of the intercorrelations of the relevant metric variables can be found in Section A of the supplementary online materials.
Table 1. Mean scores and standard deviations for relevant variables and percentage of female students by type of school (Study 1).
Hypothesis 1 stated that the type of school could be predicted from vocational interests and personality while controlling for the effects of gender and intelligence. Specifically, we expected that the prediction would become better with each group of predictors added. Multinomial logistic regression was used for the analysis. The type of school was the dependent variable with five categories: primary school (reference category), special school, K-10 school, K-13 school, and vocational school.
The first model contained only gender and intelligence as predictor variables. This model classified 49% of the cases correctly (exceeding a rate of 20%, which would be expected by chance); the LRT (likelihood-ratio test; comparison of the model with a baseline model that contains only the intercept) resulted in F(8, 3866783.79) = 14.26 (p < 0.001) with an average χ2 (df = 8) of 114.17, and the Nagelkerke R2 was 0.10.
The second model additionally contained the personality variables. This model classified 51% of the cases correctly; the LRT resulted in F(28, 424915.67) = 6.90 (p < 0.001) with an average χ2 (df = 28) of 193.40, and the Nagelkerke R2 was 0.17. A comparison of Model 1 and Model 2 revealed that Model 2 fit the data significantly better: F(20, 448131.35) = 3.96, p < 0.001 (average χ2 (df = 20) = 79.23).
The third model additionally contained the vocational interest variables. The percentage of correctly classified participants increased to 55%; the LRT resulted in F(52, 8298.67) = 9.28 (p < 0.001) with an average χ2 (df = 52) of 486.32, and the Nagelkerke R2 was 0.37. A comparison of Model 2 and Model 3 revealed that Model 3 fit the data significantly better, F(24, 7973.93) = 12.09, p < 0.001 (average χ2 (df = 24) = 292.93). Coefficients for the predictors along with the OR from Model 3 can be obtained from Table 2.
Table 2. Regression coefficients for the prediction of type of school using all predictor variables (Study 1 Model 3).
Hypotheses 2a, 2b, 4a and 4b predicted that two dimensions from the personality and vocational interest models each would be important predictors of the type of school. Agreeableness as well as Investigative and Social interests were important predictors of the type of school (two significant coefficients in the multinomial logistic regression analysis) while Openness was not (see Table 2). Regarding Hypotheses 3a, 3b, 5a and 5b, significant differences (overall Anova) by type of school occurred for Agreeableness as well as Investigative and Social interests, but not for Openness (see Table 1).
Hypothesis 1 stated that the prediction of type of school would improve by adding personality traits and vocational interests as predictors. Even though the rates of correctly classified participants improved only marginally by adding predictors, model comparison tests and the increasing Nagelkerke R2 indicated that adding personality and vocational interest variables both increased model fit. Therefore, Hypothesis 1 could be considered confirmed.
Regarding the dimensions of the Big Five model, it was specifically assumed that Openness (Hypothesis 2a) and Agreeableness (Hypothesis 2b) would be important predictors of the type of school. While Agreeableness met our criterion (two or more significant coefficients), this cannot be said about Openness; therefore, only Hypothesis 2b could be confirmed. This is mirrored by the findings regarding Hypothesis 3a (assuming that future K-13 school teachers would score higher on Openness) which could not be confirmed. Hypothesis 3b could be confirmed, though, as K-13 school teacher students showed lower values on Agreeableness than teacher students intending to teach at primary or special schools.
Regarding the role of vocational interests as predictors, it was expected that Investigative (Hypothesis 4a) and Social (Hypothesis 4b) interests would be important predictors of the type of school. Both hypotheses could be confirmed. Hypothesis 5a stated that future K-13 school teachers would score higher on Investigative interests than students intending to teach at other types of schools which was found. Hypothesis 5b stated that future K-13 school teachers would score lower regarding Social interests than students intending to teach at other types of schools which could also be found. So, Hypotheses 5a and 5b could be confirmed.
Some of our findings were not part of our hypotheses (especially, significant group differences regarding Neuroticism and Enterprising interests). Regarding neuroticism, it was found that future primary school students showed higher values. A different study reported that students who chose teaching as a career because they perceived the study program as less demanding were more likely to choose to primary school than other types of school (Retelsdorf and Möller, 2012). Maybe, this can be interpreted in the sense that students aiming to teach at primary schools are more skeptical and apprehensive regarding their own capabilities. Future research might investigate this further.
Nonetheless, findings reported above stem from a population recruited at a single university. Thus, the generalizability of our findings remains an open question. Further, it remains unclear whether participants actually finished their studies successfully. The decision to only include participants who indicated that they were at least somewhat satisfied with their studies was made to mitigate this problem. However, satisfaction with studies does not guarantee successful completion. Additionally, the sample was heterogeneous regarding their study progress. As data was cross-sectional, this implied that individual characteristics were assessed among students with differing study progress. The latter can be considered problematic as there is evidence that vocational interests change during the course of studies (Abel, 2011; Ertl et al., 2023). Study 2 was conducted to overcome these limitations.
Study 2 draws on data collected in the course of the German National Education Panel Study (NEPS, see FDZ-LIfBi, 2023). This allows us to overcome the limitations of Study 1 mentioned above. First, data was collected at multiple institutions. Second, data is of longitudinal nature, so it is possible to analyze only data from students who completed their studies successfully without changing the intended type of school. Third, vocational interests were assessed during the first year of studies for all participants. A small difference between the studies is that a measure of reasoning skills (in the following cognitive abilities) was used in Study 2 instead of the measure of intelligence used in Study 1.
For our analyses, we used publicly available data from the NEPS starting cohort 5 which focused on students who started their tertiary education in the winter term 2010/11 (NEPS-Netzwerk, 2023). If a higher education institution declared cooperativeness, possible participants were recruited via conventional mail or via personal information in lectures for first year students (FDZ-LIfBi, 2023). Data on vocational interests was obtained during wave 1 (2010/2011); data on personality during wave 3 (2012) and data on cognitive abilities during wave 5 (2013).
In total, starting cohort 5 consists of data from N = 17,909 students, out of which n = 5,824 indicated that they studied to become a teacher. We further excluded data from students with inconsistent information regarding their year of birth or gender and data from students born before 1980 or after 1992, leading to n = 5,701. A similar criterion regarding the year of birth was used by Ertl et al. (2022) to make sure that the population examined was homogenous regarding age. Additionally, only data from those students who indicated at later data collection waves that they had finished their studies successfully without changing the type of school were retained, what reduced the sample available for analysis further to n = 1,379. Next, only data from participants with complete information regarding all relevant variables was retained what led to n = 947. Finally, screening for multivariate outliers on basis of the Mahalanobis distance as described by Tabachnick and Fidell (2014, p. 133) identified three cases as multivariate outliers. So, the sample used for analysis was n = 944.
The average age of our sample was M = 21.24 (SD = 1.54), 76% were female. The sample included participants from 57 institutions across all 16 federal states of Germany. The majority of the sample intended to teach at a K-13 school (66%), followed by K-10, special and primary schools (10% each). Around 4% of the participants studied to teach at a vocational school.
For the assessment of vocational interests following the RIASEC model, the IILS (Interest Inventory Life Span; see Wohlkinger et al., 2011) was used. Each dimension was measured using k = 3 items, which results in a total of k = 18 items. Participants responded to items using a Likert scale ranging from 1 to 5 (1 = little interested to 5 = very interested). Internal consistency in terms of Cronbach’s α ranged from 0.46 to 0.61 in our sample which can be considered acceptable given the small number of items per scale (see, Niemi et al., 1986).
For the assessment of personality in terms of the Big Five, a short version of the Big Five Inventory was used which contains k = 11 items (Rammstedt and John, 2007). There are two items for each dimension, except for the Agreeableness dimension which is measured using three items. Participants responded to items using a Likert scale ranging from 1 to 5 (1 = does not apply to me to 5 = fully applies to me). Internal consistency in terms of Cronbach’s α ranged from 0.33 to 0.77 in our sample which can also be considered acceptable given that items were selected to capture core aspects of each dimension without being highly redundant (Rammstedt and John, 2007). As further indication of reliability the authors reported the stability of the scale scores across a six weeks interval for a German sample; correlations ranged from 0.66 to 0.87.
The NEPS-MAT (Haberkorn and Pohl, 2013) is a matrix test which assesses reasoning skills. Each item shows several fields in which different geometrical elements are depicted. One field is empty; participants are shown multiple possible solutions and have to identify the right one. In total, there are 12 items. However, as the test was administered using three modalities (paper and pencil, computer based [both proctored] and web based [unproctored]) and mean test scores differed substantially by modality. To make the scores comparable, scores were z-standardized separately for each modality and the result of this transformation was later used for analysis.
Participants were asked to indicate whether they had completed their studies successfully at wave 11 (2016/2017) and at later waves. For the type of school selected at the time of enrollment, information provided during waves 1 or 2 (2010/2011) was used. This was compared to the type of school indicated at wave 8 (2014) or at later waves to identify participants who did not change the type of school they wanted to teach in.
All analyses were computed using the R statistical software (R Core Team, 2023). For the multinomial logistic regression analyses, the categorical predictor variable gender was coded 0/female and 1/male (i.e., female was the reference category). The type of school was the dependent variable with five categories: primary school (reference category), special school, K-10 school, K-13 school, and vocational school. Primary school was chosen as reference category to make findings comparable to those from Study 1. The nnet package (version 7.3–19, see Venables and Ripley, 2002) was used for the multinomial logistic regression analyses.
Descriptive statistics for the relevant variables grouped by type of school and for the whole sample are displayed in Table 3. As can be seen in Table 3, significant group differences were found for the Openness and Agreeableness dimensions of personality as well as for all dimensions of the model of vocational interests, except for Realistic interests. Additionally, the gender ratio differed substantially by type of school. Cognitive abilities, though, did not differ significantly by type of school.
Table 3. Mean scores and standard deviations for relevant variables and percentage of female students by type of School (Study 2).
To examine Hypothesis 1, three models with an increasing number of predictor variables were computed. The first model contained only gender and cognitive abilities as predictor variables. This model classified 66% of the cases correctly (exceeding a rate of 20%, which would be expected by chance); the LRT resulted in χ2 (df = 8) = 44.46 (p < 0.001), and the Nagelkerke R2 was 0.05.
The second model additionally contained the personality variables. This model classified 66% of the cases correctly; the LRT resulted in χ2 (df = 28) = 106.69 (p < 0.001), and the Nagelkerke R2 was 0.12. A comparison of Model 1 and Model 2 revealed that Model 2 fit the data significantly better, χ2 (df = 20) = 62.23 (p < 0.001).
The third model additionally contained the vocational interest variables. The percentage of correctly classified participants was 66%; the LRT resulted in χ2 (df = 52) = 272.69 (p < 0.001), and the Nagelkerke R2 was 0.28. A comparison of Model 2 and Model 3 revealed that Model 3 fit the data significantly better, the LRT resulted in χ2 (df = 24) = 166.00 (p < 0.001). Coefficients for the predictors along with the OR from Model 3 can be obtained from Table 4.
Table 4. Regression coefficients for the prediction of type of school using all predictor variables (Study 2 Model 3).
The remaining hypotheses referred to dimensions of personality and vocational interests. Out of the dimensions of the Big Five model, only Agreeableness proved to be an important predictor of the type of school (see Table 4). Nonetheless, significant group differences could be found for Agreeableness and Openness (see Table 3). Almost all of the vocational interest dimensions were important predictors of the type of school (see Table 4). The only dimension for which no significant group differences could be found was Realistic (see Table 3).
Hypothesis 1 stated that the prediction of type of school would improve by adding personality traits and vocational interests as predictors. Even though the rates of correctly classified participants did not improve by adding predictors, model comparison tests and the increasing Nagelkerke R2 indicate that adding personality and vocational interest variables both increased model fit. Therefore, Hypothesis 1 could be considered confirmed based on results from Study 2. It should be noted that the types of schools were distributed quite unevenly in our sample with more than half of the participants intending to teach at a K-13 school. This can explain why gender and cognitive abilities alone could reach a correct classification rate of more than 50%.
Out of the personality dimensions, only Agreeableness was an important predictor of the type of school. So, Hypothesis 2a could not be confirmed, while Hypothesis 2b could. In contrast to our expectations, K-13 school teacher students did not score significantly higher on Openness than most other groups, Hypothesis 3a could also not be confirmed. However, K-13 school teacher students scored significantly lower on Agreeableness than most other groups which confirmed Hypothesis 3b.
Regarding vocational interests, Investigative and Social interests were important predictors of the type of school. Hence, Hypotheses 4a and 4b were confirmed. As expected, K-13 school teacher students showed significantly higher Investigative interests and significantly lower Social interests when compared to most other groups. Hence, Hypotheses 5a and 5b were also confirmed.
The aim of this research was to examine whether German teacher students’ specialization could be predicted from their vocational interests and personality, after controlling for the effects of gender and intelligence. To our knowledge, this is the first research to examine whether this prediction is feasible among teacher students when differentiating between five school types. It is also one of few studies to examine the same set of hypotheses using two separate larger datasets.
In both studies (i.e., both datasets), the same result pattern could be found.8 Hypothesis 1 could be confirmed in both studies. Adding personality and vocational interest variables improved model fit (and Nagelkerke R2). Applying the guidelines for the interpretation of R2 by Cohen (1988, p. 412) showed that a small effect could be observed when only gender and intelligence were used as predictors. In both studies, this increased to a large effect when all predictor variables were included. Nonetheless, the actual rate of correctly classified participants only increased marginally in Study 1 and did not change in Study 2. This can be explained by the unequal distribution of types of schools in both studies which was even more pronounced in the dataset used for Study 2. While students intending to become primary school teachers were the largest group in Study 1 (around 44%), students intending to become K-13 school teachers were the largest group in Study 2 (around 66%).
The further hypotheses specifically addressed one of the personality and vocational interest dimensions. Hypotheses 2a and 3a could not be confirmed in both studies while the remaining hypotheses could be confirmed. It should be noted that those hypotheses were derived from the results by Klusmann et al. (2009) in whose study students intending to teach at primary schools, special schools, and K-10 schools were treated as one group and compared to those intending to teach at K-13 schools. Future vocational school teachers were not part of the sample examined by Klusmann et al. (2009). This different grouping of participants might explain why these two hypotheses could not be confirmed in our studies.
Regarding Agreeableness, it was found that future K-13 school teacher scored significantly lower than future primary or special school teachers. The same pattern could be observed regarding Social interests. Investigative interests, though, were more pronounced among the K-13 school group. The same pattern was found by Klusmann et al. (2009). This is further in line with the requirements of the occupations the students will later work in: It can be assumed that Agreeableness and Social interests are more important when working with younger children (primary school) and when dealing with individuals with special educational needs (special school). Contrastingly, the occupation as K-13 school teacher requires more scientific work as this type of school leads to the German university entrance qualification, hence higher Investigative interests among future teachers. As mentioned in the introduction, a different line of research investigates motives to become a teacher and differences regarding these motives by the type of school. Retelsdorf and Möller (2012) found that future primary school teachers showed higher pedagogical motives, while future K-13 school teachers showed higher subject-related interest as a motive. It seems plausible to assume that pedagogical motives and Social interests are highly related. The same applies to subject-related interest as a motive and Investigative interests.
To sum up, our findings show that vocational interests and personality can be used to predict teacher students’ specialization, extending the findings of Larson et al. (2010) and others to teacher students. The rates of correct classification (between 55 and 66%) were better than chance and similar to or higher than the correct classification rates reported by similar studies: Using comparable predictors, de Fruyt and Mervielde (1996) reported the correct classification of around 30% of participants without using gender as a predictor. Larson et al. (2010) reported a correct classification rate of around 54%, and Päßler and Hell (2012) reported the correct classification of 61% of the cases. It can be assumed that choosing a study program is influenced by a range of other factors that were not part of the present studies (e.g., peers’ choices, parents’ occupations, study programs offered by institutions in the vicinity). Nonetheless, our findings show that personality and Holland’s (1966) model of vocational interests can be applied to differentiate between teacher students with different specializations. A possible application of these findings could be found in the field of career counseling. It could be used to give advice to clients who are determined to become teachers but unsure which type of school might be most appropriate for them.
Finally, several limitations of this research should be mentioned. First, the selection of predictors (gender, intelligence, personality, and vocational interests) might seem somewhat arbitrary. However, previous research has shown that especially vocational interests were helpful in distinguishing between study programs (see Hartmann et al., 2022). Second, our research is based on differences in personality and vocational interests. We did not take motives to become a teacher (cf. Watt and Richardson, 2007; Watt et al., 2012) into account. However, Retelsdorf and Möller (2012) reported differences regarding these motives between teacher students intending to teach at different types of schools. Future research might therefore investigate the combined effect as well as incremental effects of those predictors (i.e., personality, vocational interests and motives to become a teacher). Third, participants were not equally distributed across the levels of the criterion variable used in the analyses. This was even more marked in Study 2 where more than 60% of the sample intended to teach at a K-13 school. So even without any predictor variables, a substantial correct classification rate could be achieved. Future research on this issue might therefore use a quota sampling strategy.
In conclusion, the aim of this research was to address the question of whether teacher students’ study program could be predicted by personality and vocational interests. Two studies drawing on different datasets were conducted which both showed this is possible. As data for Study 1 was collected at a single university while data used in Study 2 was collected at several German institutions, our research provides evidence that the results are generalizable. Furthermore, study 2 only included data from participants who completed their studies successfully without changing the type of school. So findings are more relevant for counseling. It should be noted that result patterns were nearly identical in both studies.
The datasets presented in this study can be found in online repositories. The names of the repository/repositories and accession number(s) can be found at: http://doi.org/10.17605/OSF.IO/VB3JD.
The studies involving humans were approved by RPTU Kaiserslautern-Landau, Campus Landau – Psychology Institutional Review Board. The studies were conducted in accordance with the local legislation and institutional requirements. Written informed consent for participation was not required from the participants or the participants’ legal guardians/next of kin because participation was completely voluntarily and it was assumed that participation would not lead to harm or distress to the participants (at least not stronger distress than everyday activities). Furthermore, data was collected anonymously. Under these circumstances, informed consent is not required by the current ethical guidelines of the German Psychological Society (DGPs).
NL: Conceptualization, Data curation, Formal analysis, Investigation, Writing – original draft, Writing – review & editing. CO: Formal analysis, Writing – original draft. SW: Supervision, Writing – review & editing. MS: Writing – review & editing. TL: Supervision, Writing – review & editing.
The author(s) declare financial support was received for the research, authorship, and/or publication of this article. This project is part of the “Qualitätsoffensive Lehrerbildung,” a joint initiative of the Federal Government and the Länder which aims to improve the quality of teacher training. The program is funded by the Federal Ministry of Education and Research. The authors are responsible for the content of this publication.
The authors declare that the research was conducted in the absence of any commercial or financial relationships that could be construed as a potential conflict of interest.
All claims expressed in this article are solely those of the authors and do not necessarily represent those of their affiliated organizations, or those of the publisher, the editors and the reviewers. Any product that may be evaluated in this article, or claim that may be made by its manufacturer, is not guaranteed or endorsed by the publisher.
The Supplementary material for this article can be found online at: https://www.frontiersin.org/articles/10.3389/feduc.2024.1328864/full#supplementary-material
1. ^In this research, we examined whether teacher student’s type of school selected can be predicted. We conducted a second analysis on a subset of the data used in Study 1 and examined whether the combination of school subjects could also be predicted. Results can be found in section B of the Supplementary materials.
2. ^It should be noted that Päßler and Hell (2012) used satisfaction with the chosen major as variable for case selection. However, as German teacher students choose two school subjects when enrolling in teacher training, assessing satisfaction with the chosen major seemed inappropriate.
3. ^We decided not to impute data on the satisfaction with course content variables (as this score was used as case selection criterion) as well as on all relevant categorical variables. Instead, cases with missing data on these variables were excluded.
4. ^The data set as well as the program code for conducting the analyses can be obtained from http://doi.org/10.17605/OSF.IO/VB3JD
5. ^The high percentage of future primary and special school teachers can easily be explained as this is the only institution in the federal state offering the corresponding study programs.
6. ^All items as well a translation into English can be found in the codebook which can be downloaded together with data and code.
7. ^For the descriptive statistics, mean scores from the five datasets were averaged. Standard deviations were pooled by calculating the square root of the mean of the five squared standard deviations see Cohen (1988). Parameters from analyses of variance (Anova) were pooled using the corresponding function from the miceadds package Robitzsch and Grund (2022) while parameters from independent samples t-tests were pooled using the corresponding function of the MKmisc package Kohl (2022). McDonald’s ω scores were averaged. Pearson correlations were pooled using the corresponding function from the miceadds package Robitzsch and Grund (2022) while the coefficients of the multinomial logistic regression analysis were pooled using the pooling function from the mice package van Buuren and Groothuis-Oudshoorn (2011). OR and the corresponding confidence intervals were computed from the pooled coefficients of the multinomial logistic regression analysis. Hit ratios were averaged while model comparisons and model tests (comparison to a baseline model) were pooled using the micombine.chisquare function from the miceadds package Robitzsch and Grund (2022). Nagelkerke R2 was pooled as described by van Buuren (2018).
8. ^As expected, findings from both studies were not completely identical. A table in section C of the supplementary online materials compares odds ratios for all predictors (model 3) from the two studies.
Abel, J. (2011). “‘Differentielle Entwicklung von Interessen bei Lehramtsstudierenden für das Grundschullehramt’ [development of interests among teacher candidates for primary school]” in Sozialwissenschaftliche Forschung in Diskurs und Empirie [social science research in discourse and empiricism]. ed. C. Tarnai (Waxmann: Münster), 103–117.
Amthauer, R., Brocke, B., Liepmann, D., and Beauducel, A. (2001) I-S-T 2000 R - Intelligenz-Struktur-Test 2000 R [intelligence structure test 2000 R], Göttingen: Hogrefe.
Baudson, T. G., and Preckel, F. (2016). ‘mini-q: Intelligenzscreening in drei Minuten’ [intelligence screening in three minutes]. Diagnostica 62, 182–197. doi: 10.1026/0012-1924/a000150
Bergmann, C., and Eder, F. (2019) AIST-3. Allgemeiner Interessen-Struktur-Test mit Umwelt-Struktur-Test (UST-3) - Version 3 [Interest-Structure-Test (AIST-3) and Environmental-Structure-Test (UST-3) - Version 3] Göttingen: Hogrefe.
Blömeke, S. (2006). ‘Struktur der Lehrerausbildung im internationalen Vergleich: Ergebnisse einer Untersuchung zu acht Ländern’ [structure of teacher education in an international comparison among eight countries]. Zeitschrift für Pädagogik 52, 393–416.
Blossfeld, H.-P., and Roßbach, H.-G. (2019) Education as a lifelong process: The German National Educational Panel Study (NEPS), 2nd, Wiesbaden, Springer VS.
Brown, S. D., and Gore, P. A. Jr. (1994). An evaluation of interest congruence indices: distribution characteristics and measurement properties. J. Vocat. Behav. 45, 310–327. doi: 10.1006/jvbe.1994.1038
Cimsir, E. (2019). Insight, academic major satisfaction and life satisfaction among college students majoring in education: implications for career counselling. J. Psychol. Couns. Sch. 29, 206–218. doi: 10.1017/jgc.2019.15
Cohen, J. (1988). Statistical power analysis for the behavioral sciences. 2nd Edn. Hillsdale, NJ: Lawrence Erlbaum.
Darcy, M. U. A., and Tracey, T. J. G. (2007). Circumplex structure of Holland's RIASEC interests across gender and time. J. Couns. Psychol. 54, 17–31. doi: 10.1037/0022-0167.54.1.17
de Fruyt, F., and Mervielde, I. (1996). Personality and interests as predictors of educational streaming and achievement. Eur. J. Personal. 10, 405–425. doi: 10.1002/(SICI)1099-0984(199612)10:5<405::AID-PER255>3.0.CO;2-M
Ertl, B., Hartmann, F. G., and Wunderlich, A. (2022). Impact of interest congruence on study outcomes. Front. Psychol. 13:620. doi: 10.3389/fpsyg.2022.816620
Ertl, B., Hartmann, F. G., and Wunderlich, A. (2023). Stability of vocational interests during university studies. J. Individ. Differ. 44, 143–162. doi: 10.1027/1614-0001/a000392
Etzel, J. M., and Nagy, G. (2016). Students’ perceptions of person–environment fit. J. Career Assess. 24, 270–288. doi: 10.1177/1069072715580325
FDZ-LIfBi (2023). Data manual: NEPS starting cohort 5 - first-year students: Scientific use file version 18.0.0 [online]. Bamberg, Germany.
Gilbreath, B., Kim, T.-Y., and Nichols, B. (2011). Person-environment fit and its effects on university students: a response surface methodology study. Res. High. Educ. 52, 47–62. doi: 10.1007/s11162-010-9182-3
Haberkorn, K., and Pohl, S. (2013) Cognitive basic skills (non-verbal): data in the scientific use file [online]. Available at: https://www.neps-data.de/Portals/0/NEPS/Datenzentrum/Forschungsdaten/SC3/com_cogbasic2013_en.pdf (Accessed 2 August 2023).
Hartmann, F. G., and Ertl, B. (2021). Big five personality trait differences between students from different majors aspiring to the teaching profession. Curr. Psychol. 42, 12070–12086. doi: 10.1007/s12144-021-02528-3
Hartmann, F. G., Mouton, D., and Ertl, B. (2022). The big six interests of STEM and non-STEM students inside and outside of teacher education. Teach. Teach. Educ. 112:103622. doi: 10.1016/j.tate.2021.103622
Holland, J. L. (1966). A psychological classification scheme for vocations and major fields. J. Couns. Psychol. 13, 278–288. doi: 10.1037/h0023725
Kaub, K., Karbach, J., Biermann, A., Friedrich, A., Bedersdorfer, H.-W., Spinath, F. M., et al. (2012). ‘Berufliche Interessensorientierungen und kognitive Leistungsprofile von Lehramtsstudierenden mit unterschiedlichen Fachkombinationen’ [vocational interests and cognitive ability of first-year teacher candidates as a function of selected study major]. Zeitschrift für Pädagogische Psychologie 26, 233–249. doi: 10.1024/1010-0652/a000074
Kaub, K., Karbach, J., Spinath, F. M., and Brünken, R. (2016). Person-job fit in the field of teacher education – an analysis of vocational interests and requirements among novice and professional science and language teachers. Teach. Teach. Educ. 55, 217–227. doi: 10.1016/j.tate.2016.01.010
Kaub, K., Stoll, G., Biermann, A., Spinath, F. M., and Brünken, R. (2014). ‘Interessenkongruenz, Belastungserleben und motivationale Orientierung bei Einsteigern im Lehramtsstudium’ [interest congruence, stress experience and motivational orientations of teacher student freshmen]. Zeitschrift für Arbeits- und Organisationspsychologie A&O 58, 125–139. doi: 10.1026/0932-4089/a000149
Klusmann, U., Trautwein, U., Lüdtke, O., Kunter, M., and Baumert, J. (2009). ‘Eingangsvoraussetzungen beim Studienbeginn: Werden die Lehramtskandidaten unterschätzt?’ [cognitive and psychosocial characteristics upon entry into teacher training: are teacher candidates underestimated?]. Zeitschrift für Pädagogische Psychologie 23, 265–278. doi: 10.1024/1010-0652.23.34.265
Kohl, M. (2022) MKmisc: Miscellaneous functions from M. Kohl: R package version 1.9 [online]. Available at: https://cran.r-project.org/web/packages/MKmisc/index.html (Accessed 9 September 2023).
Kroustalis, C., Lewis, P., and Rivkin, D. (2010) Linking client assessment profiles to O*NET® occupational profiles within the O*NET interest profiler short form [online]. Available at https://www.onetcenter.org/dl_files/IPSF_Linking.pdf (Accessed 9 September 2023).
Larson, L. M., Wu, T. F., Bailey, D. C., Gasser, C. E., Bonitz, V. S., and Borgen, F. H. (2010). The role of personality in the selection of a major: with and without vocational self-efficacy and interests. J. Vocat. Behav. 76, 211–222. doi: 10.1016/j.jvb.2009.10.007
Moakler, M. W., and Kim, M. M. (2014). College major choice in STEM: revisiting confidence and demographic factors. Career Dev. Q. 62, 128–142. doi: 10.1002/j.2161-0045.2014.00075.x
Nagy, G., Trautwein, U., and Lüdtke, O. (2010). The structure of vocational interests in Germany: different methodologies, different conclusions. J. Vocat. Behav. 76, 153–169. doi: 10.1016/j.jvb.2007.07.002
NEPS-Netzwerk (2023) Nationales Bildungspanel, scientific use file der Startkohorte Studierende (SC5 18.0.0) [National Education Panel Study, scientific use file of starting cohort 5, version 18.0.0] [online], Bamberg, Leibniz-Institut für Bildungsverläufe.
Neugebauer, M. (2013). ‘Wer entscheidet sich für ein Lehramtsstudium – und warum? Eine empirische Überprüfung der these von der Negativselektion in den Lehrerberuf’ [who decides to become a teacher - and why? An empirical investigation of the negative selection hypothesis regarding teacher candidates]. Z. Erzieh. 16, 157–184. doi: 10.1007/s11618-013-0343-y
Niemi, R. G., Carmines, E. G., and McIver, J. P. (1986). The impact of scale length on reliability and validity. Qual. Quant. 20, 371–376. doi: 10.1007/BF00123086
O’Connor, M. C., and Paunonen, S. V. (2007). Big five personality predictors of post-secondary academic performance. Personal. Individ. Differ. 43, 971–990. doi: 10.1016/j.paid.2007.03.017
Päßler, K., and Hell, B. (2012). Do interests and cognitive abilities help explain college major choice equally well for women and men? J. Career Assess. 20, 479–496. doi: 10.1177/1069072712450009
Patrick, C. J., and Kramer, M. D. (2017). “Multidimensional personality questionnaire (MPQ)” in Encyclopedia of personality and individual differences. eds. V. Zeigler-Hill and T. K. Shackelford (Cham: Springer International Publishing)
Pinxten, M., de Fraine, B., van den Noortgate, W., van Damme, J., Boonen, T., and Vanlaar, G. (2015). I choose so I am’: a logistic analysis of major selection in university and successful completion of the first year. Stud. High. Educ. 40, 1919–1946. doi: 10.1080/03075079.2014.914904
Porter, S. R., and Umbach, P. D. (2006). College major choice: an analysis of person-environment fit. Res. High. Educ. 47, 429–449. doi: 10.1007/s11162-005-9002-3
R Core Team (2023) R: A language and environment for statistical computing [online], Vienna, Austria, R Foundation for Statistical Computing.
Rammstedt, B., and John, O. P. (2005). ‘Kurzversion des big five inventory (BFI-K)’ [short version of the big five inventory]. Diagnostica 51, 195–206. doi: 10.1026/0012-1924.51.4.195
Rammstedt, B., and John, O. P. (2007). Measuring personality in one minute or less: a 10-item short version of the big five inventory in English and German. J. Res. Pers. 41, 203–212. doi: 10.1016/j.jrp.2006.02.001
Retelsdorf, J., and Möller, J. (2012). ‘Grundschule oder gymnasium? Zur motivation ein Lehramt zu studieren’ [primary school or K-13 school? The motivation to become a teacher]. Zeitschrift für Pädagogische Psychologie 26, 5–17. doi: 10.1024/1010-0652/a000056
Robitzsch, A., and Grund, S. (2022) Miceadds: Some additional multiple imputation functions, especially for 'mice'. [online]. Available at https://cran.r-project.org/package=miceadds (Accessed 9 September 2023).
Roloff Henoch, J., Klusmann, U., Lüdtke, O., and Trautwein, U. (2015). Who becomes a teacher? Challenging the “negative selection” hypothesis. Learn. Instr. 36, 46–56. doi: 10.1016/j.learninstruc.2014.11.005
Savage, C., Ayaita, A., Hübner, N., and Biewen, M. (2021). Who chooses teacher education and why? Evidence from Germany. Educ. Res. 50, 483–487. doi: 10.3102/0013189X211000758
Schutz, P. A., Crowder, K. C., and White, V. E. (2001). The development of a goal to become a teacher. J. Educ. Psychol. 93, 299–308. doi: 10.1037/0022-0663.93.2.299
Soto, C. J., and John, O. P. (2017). The next big five inventory (BFI-2): developing and assessing a hierarchical model with 15 facets to enhance bandwidth, fidelity, and predictive power. J. Pers. Soc. Psychol. 113, 117–143 [Online]. doi: 10.1037/pspp0000096
Spies, K., Westermann, R., Heise, E., and Schiffler, A. (1996). ‘Diskrepanzen zwischen Bedürfnissen und Angeboten im Studium und ihre Beziehungen zur Studienzufriedenheit’ [discrepancies between student needs and course offerings and their relationship with study satisfaction]. Empirische Pädagogik 10, 377–409.
Statistisches Bundesamt (2021) 21311–0004: Studierende: Deutschland, Semester, Nationalität, Geschlecht, Angestrebte Abschlussprüfung [Students in Germany: Semester, nationality, gender and degree seeked] [Online]. Available at: https://www-genesis.destatis.de/genesis//online?operation=table&code=21311-0004&bypass=true&levelindex=0&levelid=1632998920777#abreadcrumb (Accessed 9 September 2023).
Tong, J., Wang, L., and Peng, K. (2015). From person-environment misfit to job burnout: theoretical extensions. J. Manag. Psychol. 30, 169–182. doi: 10.1108/JMP-12-2012-0404
University of Massachusetts Global (2021) What does a school counselor do? A closer look at this student-centric role [online]. Available at: https://www.umassglobal.edu/news-and-events/blog/what-does-a-school-counselor-do (Accessed 9 September 2023).
van Buuren, S. (2018) Flexible imputation of missing data [online version] [online], 2nd, Milton, CRC Press LLC.
van Buuren, S., and Groothuis-Oudshoorn, K. (2011). Mice: multivariate imputation by chained equations in R. J. Stat. Softw. 45, 1–67. doi: 10.18637/jss.v045.i03
Vedel, A. (2016). Big five personality group differences across academic majors: a systematic review. Personal. Individ. Differ. 92, 1–10. doi: 10.1016/j.paid.2015.12.011
Venables, W. N., and Ripley, B. D. (2002) Modern applied statistics with S, 4th, New York: NY, Springer.
Watt, H. M. G., and Richardson, P. W. (2007). Motivational factors influencing teaching as a career choice: development and validation of the FIT-choice scale. J. Exp. Educ. 75, 167–202. doi: 10.3200/JEXE.75.3.167-202
Watt, H. M. G., Richardson, P. W., Klusmann, U., Kunter, M., Beyer, B., Trautwein, U., et al. (2012). Motivations for choosing teaching as a career: an international comparison using the FIT-choice scale. Teach. Teach. Educ. 28, 791–805. doi: 10.1016/j.tate.2012.03.003
Weiß, R. H. (2008) Grundintelligenztest Skala 2 – Revision (CFT 20-R) mit Wortschatztest (WS) und Zahlenfolgentest (ZF) – Revision (WS/ZF-R) [basic intelligence test scale 2 with vocabulary and number sequences test - revised], Göttingen: Hogrefe.
Weiß, S., Braune, A., Steinherr, E., and Kiel, E. (2009). ‘Studium Grundschullehramt: Zur problematischen Kompatibilität von Studien-/Berufswahlmotiven und Berufsvorstellungen’ [studying to become a primary school teacher: on the problematic match between motivs for that career choice and career expectations]. Zeitschrift für Grundschulforschung 2, 126–138.
Wigfield, A., and Eccles, J. S. (2000). Expectancy-value theory of achievement motivation. Contemp. Educ. Psychol. 25, 68–81. doi: 10.1006/ceps.1999.1015
Keywords: teacher education, college counseling, vocational interests, personality, multinomial logistic regression
Citation: Leichner N, Ottenstein C, Weis S, Schmitt M and Lischetzke T (2024) Interests and personality matter in the choice of teacher education program. Front. Educ. 9:1328864. doi: 10.3389/feduc.2024.1328864
Received: 27 October 2023; Accepted: 22 January 2024;
Published: 06 February 2024.
Edited by:
Elsie L. Olan, University of Central Florida, United StatesReviewed by:
Michael Schurig, Technical University Dortmund, GermanyCopyright © 2024 Leichner, Ottenstein, Weis, Schmitt and Lischetzke. This is an open-access article distributed under the terms of the Creative Commons Attribution License (CC BY). The use, distribution or reproduction in other forums is permitted, provided the original author(s) and the copyright owner(s) are credited and that the original publication in this journal is cited, in accordance with accepted academic practice. No use, distribution or reproduction is permitted which does not comply with these terms.
*Correspondence: Nikolas Leichner, bi5sZWljaG5lckBycHR1LmRl
†ORCID: Nikolas Leichner, https://orcid.org/0000-0003-0804-4027
Charlotte Ottenstein, https://orcid.org/0000-0003-0592-765X
Susanne Weis, https://orcid.org/0000-0001-7327-3366
Manfred Schmitt, https://orcid.org/0000-0002-9978-8357
Tanja Lischetzke, https://orcid.org/0000-0002-4326-5127
Disclaimer: All claims expressed in this article are solely those of the authors and do not necessarily represent those of their affiliated organizations, or those of the publisher, the editors and the reviewers. Any product that may be evaluated in this article or claim that may be made by its manufacturer is not guaranteed or endorsed by the publisher.
Research integrity at Frontiers
Learn more about the work of our research integrity team to safeguard the quality of each article we publish.