- 1Department of Life Sciences, Liaoning University, Shenyang, Liaoning, China
- 2Liaoning Hongshan Culture Heritage Key Laboratory, Archaeology and Museology School, Liaoning University, Shenyang, China
- 3Department of Bioengineering, University of California, Los Angeles, Los Angeles, CA, United States
- 4Institute of Data Science, Department of Engineering, Columbia University, New York, NY, United States
- 5Department of Statistical Science, University College London, London, United Kingdom
In the aftermath of the COVID pandemic, we have been promoting online learning as a new learning tool. This study investigated the design of the optimal structure of online self-assisting coursework for laboratory courses that can assist students to better prepare for hands-on experiments. Undergraduate students from Liaoning University, who took the “Biochemistry Experiments” laboratory class, were asked to evaluate a self-learning segment offered on the Rain Classroom, an online platform that was provided to students before each in-person lab session as a preparation. Survey questions consisting of both multiple-choice and free-response questions were carefully designed to help evaluate students' sentiment toward the online previewing platform. Cramér's V correlation was used to determine the correlation between multiple choice answers, WordCloud and topic modeling analysis were conducted after textmining to analyze the emotions students express through the free response questions, and an analysis of variance (ANOVA) was conducted to evaluate the relationship between students' usage of the online interface and overall performance in class. The majority of students believe the previewing of the content on Rain Classroom to be helpful for their upcoming hands-on practice in the in-person laboratory course. At the same time, helpful insights were drawn from students' feedback through sentiment analysis from their entries in free-response questions in the survey. We showed that having online tools to pre-expose students to the laboratory-related material is helpful in preparing the students for hands-on laboratory courses. At the same time, we also offer a few suggestions that may guide the design of future online resources for laboratory classes such as involving multi-modality media to improve engagement and perfecting the interactive feature to increase its usage by students.
1 Introduction
The coronavirus-19 (COVID-19) pandemic, which first broke out in the winter of 2019 and then rapidly spread across the world, has greatly impacted every aspect of people's lives. The economy went stagnant, traveling was abandoned, and, more importantly, the traditional education system underwent a dramatic change (Mishra et al., 2020). Due to the limitation imposed on travel, to avoid further spreading of the novel coronavirus virus that causes the COVID-19 disease, schools at all education levels were forced to transition to the online mode of teaching. Online learning, though not easy to adapt to initially, proved to be effective during the hardest times (Rahayu and Wirza, 2020; Mushtaha et al., 2022). Numerous studies have proven that online tools can effectively simulate in-person instructions (Yasir et al., 2022). Roque-Hernández et al. (2023) reported that, owing to the interactive features offered by online learning environments, students have more positive experiences and engagement during classes, even though the classes were held online. Kim and Kim (2022) investigated teachers' perception of integrating artificial intelligence in scientific writing instructions and set up a foundation for future integration of AI techniques in education systems.
In fact, online tools that are designed for education are not new. The idea of online learning has been around for over a decade, with Coursera, which was founded in 2012, dominating the massive open online course (MOOC) industry (Nguyen, 2022). During the pandemic, however, multiple variations and types of online learning platforms were gradually developed that was adopted by users. Some, like Tencent Meeting and Zoom are designed to offer a virtual instruction platform that gives the impression of actually presenting in a virtual classroom and ensures student–instructor interaction and real-time feedback (Jiang and Ning, 2020; Adeyeye et al., 2022); others, such as ClassPoint and Classin, allow embedding interactive features in slides and course modules, which allows students to learn by themselves through pre-recorded lectures and pop quizzes embedded within the course recording or slides (Abdelrady and Akram, 2022).
In the aftermath of the COVID-19 pandemic, we have gradually adopted online learning platforms as a substitute for traditional ways of education as communication technology eases the learning process. Among the online platforms and technologies that were used during the pandemic, Rain Classroom was one of the most prominent student–teacher interaction platforms. It is a new type of smart toolbox for teaching, jointly developed by Tsinghua University and XuetangX. It provides real-time and personalized analysis for students on their class performance (Li et al., 2020). Many universities and colleges in China adopted Rain Classroom as their main platform of instruction during the pandemic and some are still using it to supplement in-person instruction these days. Such widespread adoption can be attributed to the substantial influence of Rain Classroom on effective learning across various disciplines. Li et al. (2020) revealed that the application of Rain Classroom in computer-aided design courses for landscape architecture significantly enhanced students' engagement and academic performance compared to traditional lecture-based methods. Similarly, Li and Song (2018) reported higher levels of involvement and willingness among engineering students using Rain Classroom, complemented by positive evaluations of this online platform from both educators and academic institutions. The facilitation of learner–learner, learner–teacher, and learner–content interactions via Rain Classroom was also highlighted by Yu and Yu (2022). A further study by Yu and Yu (2019) explored the role of peer and superior influences within the Rain Classroom-assisted learning environment, showcasing the importance of these factors in students' acceptance and adaptation of this innovative online tool. While theoretical courses that instruct students about concepts and practices can be smoothly integrated into the online learning environment, courses that require extensive hands-on practice, such as various laboratory courses, cannot be easily taught online. There have been attempts to construct virtual laboratories that allow students to “drag-and-drop” components to simulate the process of experimenting. For example, some online tools that simulate electronic circuitry allow students to drag different electronic components to complete different circuitry. However, courses that involve more abstract concepts and finer control during operation, such as a biochemistry laboratory course that instructs the synthesis of aspirin, faced challenges in finding appropriate simulations that allowed students to understand the experimental process.
A considerable number of studies have demonstrated the successful adaptation of theoretical courses to online platforms and have also noted some progress in simulating practical components online. However, hands-on laboratory practical courses remain less explored in the existing research. To bridge this gap, our study seeks to specifically evaluate how Rain Classroom can support and potentially improve the laboratory learning experience, thereby providing a new perspective on the application of online educational tools in laboratory class settings. To achieve this objective, in addition to the statistical analysis of the multiple choice responses using Cramér's V correlation, we introduced an innovative component by integrating natural language processing (NLP) techniques with intuitive visual representation through WordCloud. These novel approaches allow for a deeper understanding of students' perceptions regarding the use of online platforms for laboratory courses based on their verbal feedback through open-ended responses.
Therefore, to investigate whether online tools can aid student learning in laboratory courses, a preliminary architecture was designed and experimented on a group of university students. We asked the following research questions:
1. Was the previewing components offered through online instruction platforms helpful for preparing students for actual hands-on experiments?
2. How do students feel about the online tool in their overall studying experience of using the online learning tool, and how are they taking advantage of the features?
3. How to maximize students' learning satisfaction through the online learning platform?
4. How can we design novel components and further improve the online tool to better prepare students for practice-intensive courses?
2 Materials and methods
2.1 Context of study
“Biochemistry Experiments” is a specialized practical course in the field of biotechnology. It is a highly practical and comprehensive applied discipline in which students learn various aspects of biotechnology. This course equips students with essential practical skills required for careers in areas such as bio-medicine, biotechnology, bio-manufacturing, bio-protection, and biotechnology services. It serves as a prerequisite and foundation for research and industrial development in various biological disciplines. The course is designed for students majoring in biotechnology in the seventh semester (senior year, first semester).
To encourage active learning, the teaching model for this course has been redesigned to cultivate students' practical application and professional skills. The focus of the redesigned course lies in teaching methods and instructional approaches. In the design of the teaching model, several key aspects have been incorporated:
1. Teaching methods and tools are aimed at stimulating and enhancing students' interest in the course.
2. The teaching model emphasizes the development of students' comprehensive abilities, including hands-on skills, such as the ability to combine and apply various purification techniques, as well as time and efficiency management.
3. Real-world production and research scenarios are incorporated into the classroom to broaden students' perspectives.
4. Multiple assessment methods are combined to promote the improvement of students' abilities, ultimately forming a course teaching model consistent with the requirements for training applied technology professionals.
To achieve the goals of the redesigned course, pre-lab contents were designed and distributed using Rain Classroom to students to be previewed before attending in-person lab sessions. The online user interface (UI) contains essential components that aim to prepare students conceptually, as depicted in Figure 1. The main modality of pre-lab material is a series of videos that are logically designed to build the theoretical framework starting from the introduction of tools being used and the biochemistry concepts involved in the experiment. The pre-lab content was further supported by textual information and section quizzes to better engage students and deliver the essential content.
2.2 Data collection
A survey consisting of eight questions, six multiple choice and two free response questions was designed to collect insights that may answer the research questions, as shown in Figure 2. Inspired by the questionnaire in the studies of Abuhassna et al. (2020) and Sari and Oktaviani (2021) that also evaluates online learning platforms, the survey questions were designed to evaluate students' subjective feeling toward the online preview material via metrics, including material accessibility, usefulness, and value in reviewing. Multiple choice questions feature three levels of options that denote positive, neutral, or negative feelings about the online learning scheme. Each of the options is supplemented with a description so that students have a better idea about the extent to which the option is described. The surveys were distributed to the target cohort through Rain Classroom as a quiz. The questions were delivered in Chinese language because the cohort being studied involved all Chinese students. Students were encouraged to participate in the survey and a disclaimer was issued that the quiz was not associated with their grade in any way, that the data collected will only be used for future course development, and that the survey result will be anonymized to protect personal privacy. The detail that each question asks is broken down as follows:
• Question 1 asks about how satisfied the students are with the online pre-lab content for the Biochemistry laboratory course. The three choices are “Very satisfied” if the student feels the pre-lab content completely covers the lab content and meets pre-lab requirements, “Somewhat satisfied” if the student feels the knowledge framework is generally established after going through the pre-lab material but still have some knowledge gaps, or “Dissatisfied” if the students think that the pre-lab content is entirely not helpful and does not provide a reasonable understanding of the lab content.
• Question 2 asks about how the student rates the user-friendliness of the online pre-lab content interface on Rain Classroom. The three options are “Very user-friendly” if the student thinks that the interface is designed to find relevant information easily, “Moderately user-friendly” if the students think that they sometimes face difficulties when trying to find some information and it takes time to become familiar with the operation of the interface, or “Not user-friendly” if the student think the interface is cluttered and they frequently cannot find the desired information.
• Question 3 asks about how students feel about their ability to perform hands-on experiments after the pre-lab training through Rain Classroom. The three options are “Completely confident” if the students feel they can fluently transfer the knowledge from pre-lab to actual practices, or “Need further study and practice to feel confident” if the student feels somewhat confident about the practice but may require some instructions during in-person meetings from the instructor or teaching assistants (TAs), or “No confidence at all”.
• Question 4 asks about the usage of the pre-lab material through Rain Classroom during the in-person lab sessions. The three options are “Refer to the platform's content for every step” if the students are checking the pre-lab material on Rain Classroom while conducting hands-on experiments simultaneously at each step, “Occasionally check the platform for specific concepts” if students are referring to the pre-lab material occasionally only when they want to check certain specific points rather than following the pre-lab step by step, or “Do not check the platform's content at all” when students only focus on the in-lab material.
• Question 5 asks how students use the pre-lab material after in-person lab sessions. The three options are “Review pre-lab platform content to reinforce knowledge” if the student often refers back to the pre-lab content for review purposes after in-person lab meetings, “Refer to the platform or unclear knowledge gaps” if the student only revisits the pre-lab content when they find some individual concepts they failed to absorb, or “Do not use the platform's content anymore” if the student never revisits the pre-lab content after in-person lab sessions.
• Question 6 asks about students' opinions on the comparison between traditional pre-lab content (such as reading textbooks and lab procedures) and the online content delivered via Rain Classroom. The three options provided are “Online platform is more systematic and can provide a clearer introduction to lab content in a logical way,” “No significant difference, both methods have equal effects,” or “Traditional methods are better.”
• Question 7 is a free-response question to allow students to brainstorm any additional components to add to the current online pre-lab via Rain Classroom.
• Question 8 is a free-response question that asks students to summarize their overall feelings toward the online pre-lab material via Rain Classroom.
Having distributed the surveys via Rain Classroom, the platform automatically exports all the responses in the form of spreadsheet files. Eighty-six responses were collected in total, with one submitting a blank response for all the questions and one completing all the multiple choice questions but leaving the free response questions blank. The entry from the complete blank respondent was removed and the multiple choice response from the one submitted without free response was kept, resulting in 85 records of multiple choice survey responses and 84 free response survey responses. Rain Classroom also keeps track of each student's usage of the online material, such as the percentage of slides viewed and the percentage of videos watched, as well as the final grade in the class in a spreadsheet form. The two spreadsheet files were merged by the student ID and saved for further analysis.
2.3 Study participants
Students from the biotechnology and bio-informatics program at Liaoning University who took the “Biochemistry Experiments” laboratory course using the course structure with pre-lab content were surveyed. Among the 102 students who originally took the class, 85 responses were collected. Among the 85 respondents, there were 47 female respondents, 40 from the biotechnology program and seven from the bioinformatics program, and 38 male respondents, 33 from the biotechnology department and 5 from the bioinformatics program. The demographic information is given in Figure 3.
2.4 Analysis techniques
2.4.1 Statistical analysis
When determining the correlation between the multiple choice questions, Cramér's V correlation (ϕc) was used. Different from Pearson correlation (ϕp), which is used for measuring the strength and direction of a linear relationship between two numerical variables, ϕc is used for measuring the association between two or more categorical variables. It provides a value between 0 and 1, where 0 indicates no association, and 1 indicates a perfect association. Values that are over 0.5 indicate a strong association between the variables, while a correlation between 0.3 and 0.5 indicates a moderate association.
When analyzing the relationship between students' usage of the online platform and overall performance in the class, a linear model was first constructed between the two variables, with platform usage being the independent variable and performance being the dependent variable. Then, the analysis of variance (ANOVA) was conducted for the linear model to determine the significance of the effect of the dependent variable on the independent variable. The significance value (α) is 0.05 unless otherwise specified.
2.4.2 Text-mining analysis
To process the free response data from the students, several text-mining and NLP techniques were implemented. Text-mining refers to the process of analyzing large bodies of texts to find hidden underlying patterns, as shown in Figure 4.
Text-mining starts by collecting data or scrapping data from external sources. The texts are then tokenized, or broken down into individual words, before removing any stopping words. Stopping words refers to the words that are conventions of the language and fulfill grammatical purposes but do not contribute to the concept of information being conveyed in the text. In other words, stopping words are redundant information included in the text to allow fluent expression but should be removed for the sake of understanding the content of the body of texts. At the same time, the punctuation marks and numbers in the original text are also removed because they do not contribute to contextual information. Furthermore, the texts that have stopping words removed will be lemmatized. Lemmatization refers to the process of aggregating words that are of the same origin. For example, the words “start” and “started” represented the same meaning, but different tenses. Thus, to avoid interpreting the two words, which are actually of the same origin but different in meaning, lemmatization will be conducted to “simplify” all words down to their root word (i.e., “started” to “start”). Then, two mining techniques, namely word cloud analysis and topic modeling, are used to further analyze the extracted, cleaned content to better understand the textual information.
2.4.2.1 WordCloud
A word cloud is a visual representation of text data, where the size of each word in the visualization is proportional to its frequency or importance in the given text. It is a popular way to depict the most frequently occurring words in a body of text, providing a quick and intuitive way to identify key terms. First, all the responses were aggregated and the words were all tokenized (i.e., separating each word from a single string or sentence into individual strings/tokens). Then, the frequency of each word was accounted for and the word cloud constructed using the WordCloud function in Python. In the word cloud, the larger the text, the more frequently the word appears in the responses, indicating higher importance.
2.4.2.2 Topic modeling
Topic modeling refers to the process of clustering the collected texts into different groups and determining the entities (represented as texts) that give rise to the determined clusters. It is a way of automatically discovering hidden thematic structures in a collection of documents. The goal is to uncover the main themes or topics within the text data and understand how different documents are related to these topics. After the data acquisition and preprocessing that produce the tokenized words are lemmetized and having the stopping words removed, a term-document matrix (TDM) was constructed to create an association between all the words, as a corpus, and each response. Then, the TDM was further transformed into the term frequency inverse document frequency (TF-IDF) format, which is a standard practice in information retrieval and text mining. The TF-IDF is a numerical statistic that reflects the importance of a word in a document relative to a collection of documents, often a larger corpus, which can avoid problems with commonly occurring words across all the responses or articles that were not removed as stopping words but still do not contribute useful information. Furthermore, the CV coherence score, proposed by Röder et al. (2015), was used to determine the optimal number of clusters to divide the free responses into during the topic modeling process. Coherence score is a measurement of how well the cluster of data can convey the maximum amount of valuable information; it is usually used to determine the optimal number of clusters to separate the data into.
2.4.3 Analysis tools
The data preprocessing and text-mining were conducted in a Google Colab environment, which allows anyone to write and execute Python code through the browser. Specifically, text-mining was achieved using the NLTK module, which is a suite of libraries and programs for symbolic and statistical natural language processing for English written in the Python programming language. Statistical analysis and data visualization were performed using R. More specifically, the dplyr and ggplot2 packages were used for creating visualizations.
3 Results
Figure 5 summarizes the proportions of each of the choices from question 1 to question 6 in the survey. First, from the results of question 1, we learned that the 85 students who responded to the survey all displayed certain degrees of satisfaction with the pre-lab content. The majority of the students, which accounts for 83.5% of the responses, are very satisfied with the preparation that the pre-lab content offers and feel that the content covers the actual in-person lab content. According to the responses collected for question 2, we learned that the Rain Classroom interface still requires further improvements in terms of operation clarity and easiness, since a non-trivial 28.2% of the responses showed that sometimes students have trouble finding the desired information and need to go through a learning curve before getting used to the user interface (UI). Moreover, there is one response saying that it is really hard to navigate around the current user interface because the information given cannot guide the students to the components they want. Furthermore, from the response distribution of question 3, we can see that over 5-fold of the students indicated in their responses that they would need further instructions from either the instructor or TA during in-person meetings to become fully confident about transitioning to hands-on lab practices after reviewing the pre-lab contents. Roughly the same distribution of choices was displayed for questions 4 and 5, with the majority of students reporting they are using the pre-lab content to resolve some lingering knowledge gap during or after the in-person lab section, indicating that the content design can serve both preview and review purposes and can be an exemplary framework for future lab course design. Finally, according to the distribution of responses for question 6, the majority of students feel the online preparatory content is superior to traditional preview content, suggesting the plausibility of widely adopting online platforms for practical courses.
Figure 6 represents the Cramér's V value between the five multiple-choice questions. According to the figure, the correlation of Q1–Q2, Q2–Q4, and Q3–Q5 are all over 0.5, which is considered strongly correlated. We can conclude that students who share the same opinion on the knowledge structure of the pre-lab material might also have the same opinion on the design of the UI. Furthermore, there appears to be a high correlation between the frequency with which the student uses the online material for in-lab assistance or post-lab review and the interactiveness of the UI. Thus, we suggest developers work closely with education specialists and course content creators to create a student-user-friendly interface that would guarantee higher content use for both preview and review purposes. The correlation of Q1–Q3, Q2–Q3, Q3–Q4, Q1–Q5, Q4–Q5, Q3–Q6, and Q5–Q6 are in the range of 0.3–0.5, suggesting a moderate correlation between the choices for the questions. The correlation of Q1–Q4, Q2–Q5, Q1–Q6, Q2–Q6, and Q4–Q6 are < 0.3, which has a weaker correlation than the others. Overall, we can see that students are affected by the physical design of the website. If the interface is clearly structured, the likelihood of frequently referring to the pre-lab content for in-lab assistance and post-lab knowledge reinforcement is also higher.
The free response questions Q7 and Q8 give students a space to freely express themselves and assist with brainstorming more helpful components that can be added to the online platform. Word cloud diagrams were constructed for both free response questions and are shown as Figure 7. According to Figure 7A which shows the word cloud for students' brainstorming ideas about additional components to add to the platform, we can see that the keywords “follow” and “exercise” have equally high occurrences and stand out from the other words, indicating that many students feel that having exercises embedded in the tutorial videos might be more engaging and deepen their understanding of the content. This type of instruction component is already practiced in some online learning platforms. For example, some courses on Coursera embed quiz questions within the tutorial videos that are in form of a test on the concepts just mentioned in the video. Thus, questions can be embedded in the videos that closely adhere to the pre-lab content and are engaging enough for students to further investigate through the videos. In addition, another word that also requires attention is “operation”. Even though the word does not stand out from the rest of the keywords like “follow” and “exercise”, we can still conclude that students want to have a content that focuses more on the operation procedures, which may also include actual experiment procedures conducted by students from previous quarters. We can also integrate the operating component in the embedded questions such as a drag-and-drop interactive quiz to test if the students know how the apparatuses combine with one another. Moreover, according to Figure 7B, which shows students' overall sentiment regarding the online platform, we can see that the majority of the feedback is positive, and students are reporting that they feel the pre-lab content is convenient and good for the experimental practices, suggesting a huge potential to integrate online components for future laboratory course instructions.
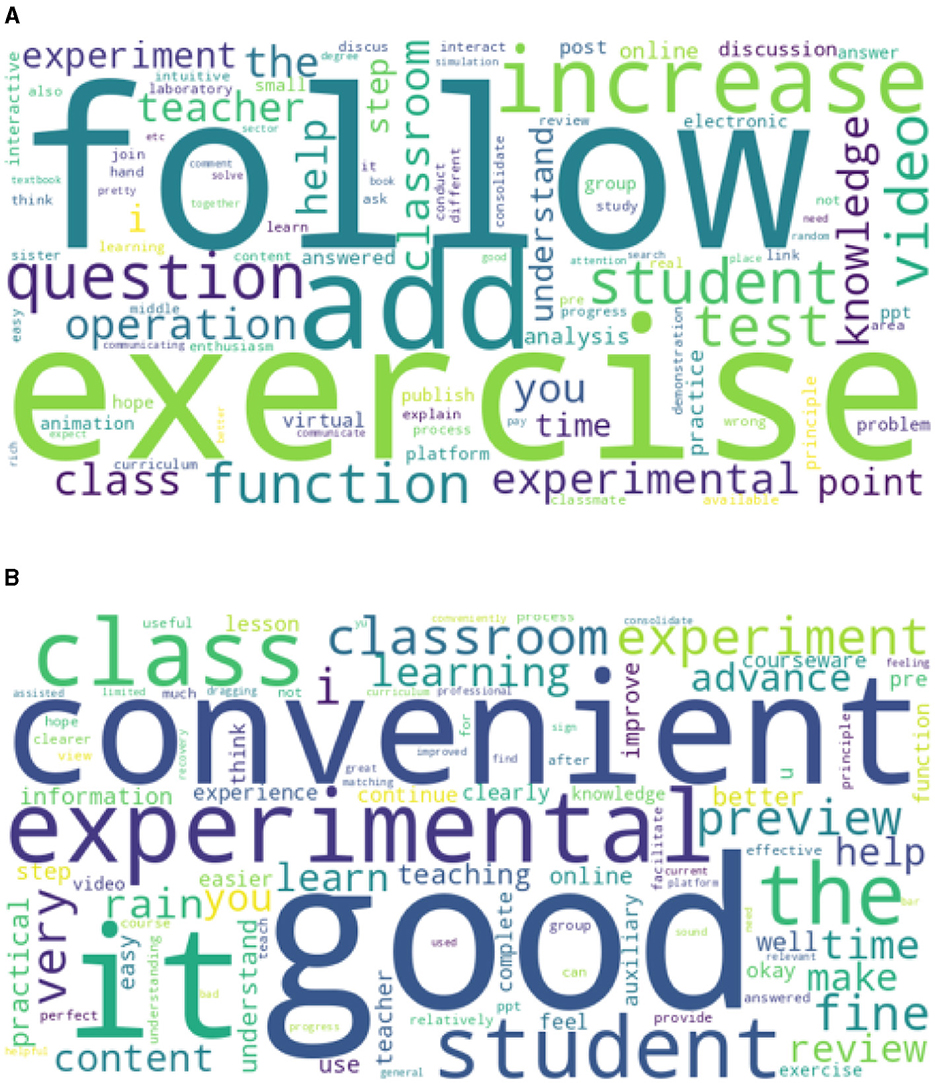
Figure 7. Free response WordCloud result: (A) What element to add to the platform?; (B) Overall feeling about the current platform.
Topic modeling revealed some further insights into the sentiment and suggestions that students have according to the responses from the last two free response questions. During the topic modeling process, the number of clusters was set to three according to the CV coherence score, which achieved the highest at three clusters, as shown in Figure 8. Using three clusters, topic modeling resulted in the text patterns shown in Table 1. The three topics were identified as three main directions of discussions, namely, “question-related,” “forum-related,” and “content-related.” In the first cluster, the keywords such as test, practice, review, and content all indicate that students want to have questions that are associated with the lab content that can help them reinforce their knowledge and understanding. The second cluster contains keywords such as help, group, teacher, student, online, and post, which centers around the idea that students want to have more interactions with their classmates and the instructors in addition to the in-person lab meetings, and they also want to have the option to ask their questions through the online platform whenever they have doubts about some concepts. The third cluster is mainly suggestions about what the pre-lab content should focus on. According to the identified keywords, such as operation, enthusiasm, and analysis, students want to have a pre-lab content that can deliver operation-related topics (e.g., how to perform a certain procedure) in an analytical way that can inspire enthusiasm among students.
Finally, the results of the ANOVA for the relationship between usage of the online platform and students' overall performance in class is summarized in Table 2. The coefficient for the online-grade variable (0.4443) suggests a positive relationship between the usage of online resources and overall performance, with each additional unit of usage associated with an increase in performance by ~0.4443 units. The very small p-value indicates the statistical significance of the effect. Thus, the results suggest a significant positive association between students' utilization of online resources on Rain Classroom and their overall performance in the laboratory course, highlighting the potential benefits of utilizing online resources for enhancing academic outcomes.
4 Discussion
The results of the survey provide valuable insights into students' perceptions and experiences with the online pre-lab content in laboratory courses. The sentiment analysis indicates an overall positive feedback from the students, emphasizing the potential for the successful integration of online components in future laboratory courses. However, we also noted that the online platforms need to be constructed by prioritizing student satisfaction since the correlation analysis of the multiple-choice questions reveals strong associations between students' opinions on pre-lab content and the UI design. As Gopal et al. (2021) suggest, student satisfaction is often a key determinant of the effectiveness of educational tools. The online platform to assist laboratory classes thus becomes a customer-sensitive system where the customer is the student and the product needs to be designed to provide maximum student satisfaction (Korableva et al., 2019). To maximize the success of online platforms in laboratory courses, it is essential to anticipate factors that would contribute to students' satisfaction. Adhering to the findings proposed by Abuhassna et al. (2020), our study has concluded that students' satisfaction with the online platform is highly correlated with the interactiveness that the platform offers, user-friendliness of the UI when navigating the platform, and efficient coursework assistance.
First, the layout of the interface needs to be further improved to become more intuitive to navigate, as our survey suggests that students sometimes find the interface challenging to navigate, emphasizing the need for collaboration between developers of the interface front-end, education professionals or education psychology specialists, and the instructors of the course to design the interface that is the most intuitive for students and allows more efficient and satisfactory interaction. This aligns with the broader trend in educational technology, where intuitive design becomes paramount for effective learning experiences (Tao et al., 2022). At the same time, we need to realize the obstacle that education faces with integrating the online component in pedagogy and the potential learning curve of using the interfaces. Thus, future course designs could include specific training to familiarize students with the interaction with digital tools (Langegård et al., 2021).
Second, the word cloud analysis indicates that students desire more interactive components, such as embedded exercises in tutorial videos. This aligns with modern trends in online education, where interactive elements enhance engagement and deepen understanding (Ha and Im, 2020; Muzammil et al., 2020). Thus, online platforms, as a supplement for in-person hands-on experiments, can include more interactive features to familiarize students with the concepts. There have been several attempts to integrate interactive features for practical courses. For example, Schlupeck et al. (2021) evaluated an interactive, video, and case-based online instruction scheme for medical students regarding wound care, suggesting the plausibility of online instruction components for courses that focus extensively on hands-on abilities. Patete and Marquez (2022) proposed an interactive tool for control systems engineering instruction during the pandemic, which allows students to play around with computer animations by changing mathematical models and design parameters of control laws. Their study proved the effectiveness of interactive platforms that make the instruction of abstract ideas playful and memorable. Sahin and Kara (2022) investigated the educational effect of embedding comic components in history courses and proved that comic content can positively affect students' attitudes toward lessons. Their result showed that computer-based material enables students to have more control of the content they study, making the education experience student-centered. Additionally, forums where students can post questions and chat with others to resolve course-related issues can also be integrated into the platform according to students' feedback in the survey. Having online forums that allow students to freely interact with others and the instructors not only allows the problem-solving process to be more efficient but also can be used to determine user's emotions, as proposed by Yee et al. (2023).
Moreover, our study suggests a correlation between students' confidence in transitioning to hands-on lab practices and their engagement with the pre-lab content. This underscores the potential of online materials to bridge the gap between theoretical understanding and practical application. Admittedly, the pre-lab content cannot offer the amount of technical experience that can be offered by hands-on operations. As communication tools are advancing over time, more human senses can be incorporated during the instruction. For example, Meta launched the Horizon Workrooms to engage more online collaborations for coworkers from all across the world, providing a real-life feeling as people are sitting around the same table through virtual reality (VR) technologies. In this case, reality-enhancement techniques can more efficiently represent knowledge, as Saidani Neffati et al. (2021) suggested integrating augmented reality, an extension of virtual reality with e-learning using mobile devices. An experimental VR-pedagogy scheme has already been designed in the field of medicine, where instructors proposed to involve VR in Doctor of Medicine (MD) surgical training to bring as much exposure as they would have similar to in-person training when learning remotely (Zhao et al., 2021). Thus, future online learning platforms for practical courses may involve VR and augmented reality (AR) technologies to create more immersive features for students to experience.
It is also essential to take into account individual learning styles (El-Sabagh, 2021) during the platform design process. Depending on the type of learner a student is, whether a visual learner or a hands-on learner, different modalities of learning resources can be helpful (Pashler et al., 2008). GPT, which empowers individuals to express themselves authentically, can be utilized to fit the different learning styles of students. For example, Javaid et al. (2023), in response to the emerging trend of ChatGPT, investigated the possibility of integrating ChatGPT in student education design. The authors showed that ChatGPT has the potential to create a personalized education experience because the algorithm can create educational resources and content that are tailored to student's unique interests, skills, and learning goals. Students, when using ChatGPT, can learn at their their own pace, paving the way for self-directed research and practices.
5 Conclusion
The survey results shed light on the current state of online pre-lab content integration in laboratory courses. While students express an overall satisfaction with the pre-lab online learning, there are clear opportunities for improvement, particularly in the usability of the online platform. The findings emphasize the importance of a collaborative approach between different stakeholders to design interfaces that enhance the learning experience. Moreover, the survey highlights the potential of online materials to complement hands-on experiences, offering a valuable resource for both preview and review purposes. The correlation analysis highlights the interconnectedness of students' opinions on pre-lab content and the user interface, emphasizing the need for a holistic approach to platform development. The free-response questions provide specific suggestions for future improvements, including the incorporation of interactive elements and a focus on operation-related content. These insights can be used to guide the development of future laboratory course designs, ensuring that online components align with student preferences and enhance the overall educational experience. In conclusion, the study provides valuable insights for educators, developers, and education specialists aiming to integrate online pre-lab content into laboratory courses. By addressing the identified areas for improvement and capitalizing on the positive feedback, educators can create a more effective and satisfying learning environment for students in laboratory settings.
Data availability statement
The datasets presented in this study can be found in online repositories. The names of the repository/repositories and accession number(s) can be found below: https://github.com/ChenshuLiu/RainClassroom-Biochemistry-Pre-lab-Evaluation.git.
Ethics statement
Written informed consent was obtained from the individual(s) for the publication of any potentially identifiable images or data included in this article.
Author contributions
SB: Conceptualization, Data curation, Funding acquisition, Project administration, Resources, Supervision, Writing – review & editing, Investigation. CL: Conceptualization, Data curation, Formal analysis, Investigation, Methodology, Project administration, Software, Supervision, Visualization, Writing – original draft, Writing – review & editing. PY: Data curation, Formal analysis, Investigation, Methodology, Software, Visualization, Writing – original draft, Writing – review & editing. JG: Data curation, Formal analysis, Investigation, Methodology, Software, Visualization, Writing – original draft, Writing – review & editing. YH: Data curation, Writing – review & editing, Resources.
Funding
The author(s) declare financial support was received for the research, authorship, and/or publication of this article. This study was sponsored by 2022 Liaoning Province First-Class Undergraduate Course Construction Project, 2022 Undergraduate Teaching Reform Project at Liaoning University, and Financial Support for Outstanding Lecturers in Undergraduate Programs at Liaoning University for the Academic Years 2020–2022.
Acknowledgments
The authors gratefully acknowledge the contributions of specific colleagues, institutions, or agencies that aided their efforts.
Conflict of interest
The authors declare that the research was conducted in the absence of any commercial or financial relationships that could be construed as a potential conflict of interest.
Publisher's note
All claims expressed in this article are solely those of the authors and do not necessarily represent those of their affiliated organizations, or those of the publisher, the editors and the reviewers. Any product that may be evaluated in this article, or claim that may be made by its manufacturer, is not guaranteed or endorsed by the publisher.
References
Abdelrady, A. H., and Akram, H. (2022). An empirical study of ClassPoint tool application in enhancing EFL students' online learning satisfaction. Systems 10:154. doi: 10.3390/systems10050154
Abuhassna, H., Al-Rahmi, W. M., Yahya, N., Zakaria, M. A. Z. M., Kosnin, A. B. M., and Darwish, M. (2020). Development of a new model on utilizing online learning platforms to improve students' academic achievements and satisfaction. Int. J. Educ. Technol. High. Educ. 17:38. doi: 10.1186/s41239-020-00216-z
Adeyeye, B., Ojih, S. E., Bello, D., Adesina, E., Yartey, D., Ben-Enukora, C., et al. (2022). Online learning platforms and covenant university students' academic performance in practical related courses during COVID-19 pandemic. Sustainability 14:878. doi: 10.3390/su14020878
El-Sabagh, H. A. (2021). Adaptive e-learning environment based on learning styles and its impact on development students' engagement. Int. J. Educ. Technol. High. Educ. 18, 1–24. doi: 10.1186/s41239-021-00289-4
Gopal, R., Singh, V., and Aggarwal, A. (2021). Impact of online classes on the satisfaction and performance of students during the pandemic period of COVID 19. Educ. Inf. Technol. 26, 6923–6947. doi: 10.1007/s10639-021-10523-1
Ha, Y., and Im, H. (2020). The role of an interactive visual learning tool and its personalizability in online learning: flow experience. Online Learn. 24:1620. doi: 10.24059/olj.v24i1.1620
Javaid, M., Haleem, A., Singh, R. P., Khan, S., and Khan, I. H. (2023). Unlocking the opportunities through ChatGPT Tool towards ameliorating the education system. BenchCouncil Transact. Benchmarks Stand. Eval. 3:100115. doi: 10.1016/j.tbench.2023.100115
Jiang, X., and Ning, Q. (2020). The impact and evaluation of COVID-19 pandemic on the teaching model of medical molecular biology course for undergraduates major in pharmacy. Biochem. Mol. Biol. Educ. 49, 346–352. doi: 10.1002/bmb.21471
Kim, N. J., and Kim, M. K. (2022). Teacher's perceptions of using an artificial intelligence-based educational tool for scientific writing. Front. Educ. 7:755914. doi: 10.3389/feduc.2022.755914
Korableva, O., Durand, T., Kalimullina, O., and Stepanova, I. (2019). Studying User Satisfaction with the MOOC Platform Interfaces Using the Example of Coursera and Open Education Platforms. New York, NY: Association for Computing Machinery.
Langegård, U., Kiani, K., Nielsen, S. J., and Svensson, P.-A. (2021). Nursing students' experiences of a pedagogical transition from campus learning to distance learning using digital tools. BMC Nurs. 20, 1–10. doi: 10.1186/s12912-021-00542-1
Li, D., Li, H., Li, W., Guo, J., and Li, E. (2020). Application of flipped classroom based on the Rain Classroom in the teaching of computer-aided landscape design. Comp. Appl. Eng. Educ. 28, 357–366. doi: 10.1002/cae.22198
Li, X., and Song, S. (2018). Mobile technology affordance and its social implications: a case of “Rain Classroom”. Br. J. Educ. Technol. 49, 276–291. doi: 10.1111/bjet.12586
Mishra, L., Gupta, T., and Shree, A. (2020). Online teaching-learning in higher education during lockdown period of COVID-19 pandemic. Int. J. Educ. Res. Open 1:100012. doi: 10.1016/j.ijedro.2020.100012
Mushtaha, E., Abu Dabous, S., Alsyouf, I., Ahmed, A., and Raafat Abdraboh, N. (2022). The challenges and opportunities of online learning and teaching at engineering and theoretical colleges during the pandemic. Ain Shams Eng. J. 13:101770. doi: 10.1016/j.asej.2022.101770
Muzammil, M., Sutawijaya, A., and Harsasi, M. (2020). Investigating student satisfaction in online learning: the role of student interaction and engagement in distance learning university. Turk. Online J. Dist. Educ. 21(Special Issue-IODL), 88–96. doi: 10.17718/tojde.770928
Nguyen, L. Q. (2022). Learners' satisfaction of courses on Coursera as a massive open online course platform: a case study. Front. Educ. 7:1086170. doi: 10.3389/feduc.2022.1086170
Pashler, H., McDaniel, M., Rohrer, D., and Bjork, R. (2008). Learning styles: concepts and evidence. Psychol. Sci. Public Int. 9, 105–119. doi: 10.1111/j.1539-6053.2009.01038.x
Patete, A., and Marquez, R. (2022). Computer animation education online: a tool to teach control systems engineering throughout the COVID-19 pandemic. Educ. Sci. 12:253. doi: 10.3390/educsci12040253
Rahayu, R. P., and Wirza, Y. (2020). Teachers' perception of online learning during pandemic Covid-19. J. Penelitian Pendidikan 20, 392–406. doi: 10.17509/jpp.v20i3.29226
Röder, M., Both, A., and Hinneburg, A. (2015). “Exploring the space of topic coherence measures,” in Proceedings of the Eighth ACM International Conference on Web Search and Data Mining, WSDM '15 (New York, NY: Association for Computing Machinery), 399–408.
Roque-Hernández, R. V., Díaz-Roldán, J. L., López-Mendoza, A., and Salazar-Hernández, R. (2023). Instructor presence, interactive tools, student engagement, and satisfaction in online education during the COVID-19 Mexican lockdown. Interact. Learn. Environ. 31, 2841–2854. doi: 10.1080/10494820.2021.1912112
Sahin, A. N. E., and Kara, H. (2022). A digital educational tool experience in history course: creating digital comics via Pixton Edu. J. Educ. Technol. Online Learn. 5:983861. doi: 10.31681/jetol.983861
Saidani Neffati, O., Setiawan, R., Jayanthi, P., Vanithamani, S., Sharma, D. K., Regin, R., et al. (2021). An educational tool for enhanced mobile e-learning for technical higher education using mobile devices for augmented reality. Microprocess. Microsyst. 83:104030. doi: 10.1016/j.micpro.2021.104030
Sari, F. M., and Oktaviani, L. (2021). Undergraduate students' views on the use of online learning platform during COVID-19 pandemic. Teknosastik 19, 41–47. doi: 10.33365/ts.v19i1.896
Schlupeck, M., Stubner, B., and Erfurt-Berge, C. (2021). Development and evaluation of a digital education tool for medical students in wound care. Int. Wound J. 18, 8–16. doi: 10.1111/iwj.13498
Tao, D., Fu, P., Wang, Y., Zhang, T., and Qu, X. (2022). Key characteristics in designing massive open online courses (MOOCs) for user acceptance: an application of the extended technology acceptance model. Interact. Learn. Environ. 30, 882–895. doi: 10.1080/10494820.2019.1695214
Yasir, M., Ullah, A., Siddique, M., Hamid, Z., and Khan, N. (2022). The capabilities, challenges, and resilience of digital learning as a tool for education during the COVID-19. Int. J. Interact. Mobile Technol. 16, 160–174. doi: 10.3991/ijim.v16i13.30909
Yee, M., Roy, A., Perdue, M., Cuevas, C., Quigley, K., Bell, A., et al. (2023). AI-assisted analysis of content, structure, and sentiment in MOOC discussion forums. Front. Educ. 8:1250846. doi: 10.3389/feduc.2023.1250846
Yu, Z., and Yu, L. (2022). Identifying tertiary students' perception of usabilities of rain classroom. Technol. Knowl. Learn. 27, 1215–1235. doi: 10.1007/s10758-021-09524-3
Yu, Z., and Yu, X. (2019). An extended technology acceptance model of a mobile learning technology. Comp. Appl. Eng. Educ. 27, 721–732. doi: 10.1002/cae.22111
Keywords: online learning tool, interactive platform design, self-learning scheme, sentiment analysis, natural language processing, laboratory course
Citation: Ben S, Liu C, Yang P, Gong J and He Y (2024) A practical evaluation of online self-assisted previewing architecture on rain classroom for biochemistry lab courses. Front. Educ. 9:1326284. doi: 10.3389/feduc.2024.1326284
Received: 23 October 2023; Accepted: 07 March 2024;
Published: 26 March 2024.
Edited by:
Subramaniam Ramanathan, Nanyang Technological University, SingaporeReviewed by:
Agus Ramdani, University of Mataram, IndonesiaMaria Victoria Velarde Aliaga, Universidad de Valparaiso, Chile
Copyright © 2024 Ben, Liu, Yang, Gong and He. This is an open-access article distributed under the terms of the Creative Commons Attribution License (CC BY). The use, distribution or reproduction in other forums is permitted, provided the original author(s) and the copyright owner(s) are credited and that the original publication in this journal is cited, in accordance with accepted academic practice. No use, distribution or reproduction is permitted which does not comply with these terms.
*Correspondence: Chenshu Liu, chenshu0615@g.ucla.edu; Songbin Ben, bensongbin@lnu.edu.cn
†These authors have contributed equally to this work