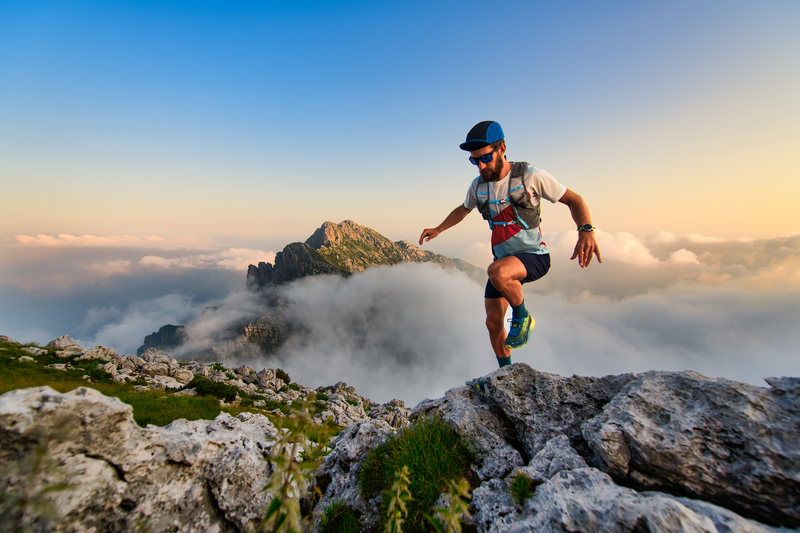
95% of researchers rate our articles as excellent or good
Learn more about the work of our research integrity team to safeguard the quality of each article we publish.
Find out more
ORIGINAL RESEARCH article
Front. Educ. , 02 May 2024
Sec. Educational Psychology
Volume 9 - 2024 | https://doi.org/10.3389/feduc.2024.1307485
This study aimed to investigate students’ metacognitive knowledge and reported use of surface and deep learning strategies. It also explored the extent to which students recall their teachers’ recommendations for learning strategies and the relationship between these recollections and students’ knowledge and reported use of strategies. A scenario-based questionnaire was used to set a learning goal in the area of biology. Students’ metacognitive knowledge was assessed through perceived effectiveness and reported use of learning strategies. Additionally, open-ended questions allowed students to recall and report recommendations given by their teachers. We used person-centered methods to explore whether different types of recollections were related to reported strategy use. Among students who recollected that their teachers have recommended deep learning strategies, it was typical to value deep strategies higher than surface strategies and report using deep strategies. Also, it was atypical among those students to value surface level strategies and not use deep strategies.
Fostering the growth of students into self-regulated learners (SRL) is considered an important goal in contemporary education. Students do not spontaneously become self-regulated learners; instead, they require explicit instruction to develop these skills (Kirschner et al., 2006). The concept of SRL refers to students who have knowledge and skills to manage effectively their own learning activities (Bjork et al., 2012). Managing one’s own learning encompasses many aspects, but an important component is the metacognitive knowledge of the effectiveness of different learning strategies in relation to specific learning tasks (Veenman, 2017). Several empirical studies have shown that students’ metacognitive knowledge of learning strategies is weak and that students frequently tend to use surface rather than deep strategies (Karpicke et al., 2009; McCabe, 2011; Hennok et al., 2022). So far, some of the possible reasons of students’ low understanding of the effectiveness of learning strategies have rarely examined. Also, the majority of studies have used variable-oriented methods. However, it is possible that general trends and average effects identified by variable-oriented methods may not capture all relevant aspects of this phenomenon. Hence, we used a person-oriented approach (Stemmler, 2020).
An important source of students’ metacognitive knowledge of learning and learning strategies is teacher instruction. Explicit teaching of learning strategies has indicated positive changes in strategy use and metacognition (Zepeda et al., 2019; Rogiers et al., 2020), but several observational studies have shown that teachers rarely explicitly teach about memory, learning, and learning strategies (Granström et al., 2023). However, it is not enough to examine how and what teachers teach about learning strategies; it is more important to study what students learn, i.e., how they perceive instruction, comprehend information, and memorize details (e.g., Liu and Uesaka, 2022). Although the role of explicit teaching in students’ metacognitive knowledge has been shown in some studies (Mizumoto and Takeuchi, 2009), much less is known about students’ recollections of teacher instruction regarding learning strategies in regular classrooms (but see Kikas and Jõgi, 2016).
Our study investigates students’ metacognitive knowledge by examining their perceptions of the effectiveness of various learning strategies and their reported use. We conceptualized all strategies as either promoting deep or surface information processing. The influence of teacher guidance as a significant source of this knowledge led us to explore students’ recollections of instructional advice on learning strategies. Despite the recognized importance of teacher guidance on student learning, there remains a notable research gap in specifically investigating students’ recollections of teachers’ strategic instructions in regular classrooms. This research is important for understanding how students perceive instructional guidance toward better learning practices, which, in turn, can guide the development of more effective educational approaches. We selected upper middle school students for our study because they are required to take exams. We reasoned that, in preparing students for these exams, teachers are likely to recommend learning strategies. We aim to determine if there are relations between students’ recollections of teachers’ advice and their metacognitive knowledge. Utilizing person-oriented methods, our study aims to identify sub-groups of students who, based on their recollections of teachers’ advice, exhibit distinct response patterns to learning strategies.
Learning strategies have been defined as goal-directed activities that students use to enhance their learning, acquire new skills, and memorize new information (Hattie and Donoghue, 2016; Van Meter and Campbell, 2020). Learning strategies are based on procedural knowledge about what one must do to learn something, and the activities and processes themselves are captured by the concept of strategic information processing (Van Meter and Campbell, 2020). There are multiple models and theoretical frameworks examining learning strategies. Most of them distinguish different levels of information processing — i.e., surface and deep strategies (Dinsmore, 2017), which tend to support either surface- or deep-level information processing (Craik and Lockhart, 1972; Marton and Säljö, 1976). Deep strategies enable deep information processing to understand content (i.e., what is signified) and surface strategies enable surface information processing that is directed toward memorization of surface characteristics (the signifiers; Marton and Säljö, 1976). For example, when students employ elaboration, a deep learning strategy, they create new and more intricate connections with previously acquired knowledge, thereby enhancing retention and supporting formation of long-term memories. In contrast, when students rely only on rehearsal, no novel associations are formed, hindering the future retention process and formation of long-term memories. This distinction is useful as variations in the level of information processing lead to variations in outcomes (Marton and Säljö, 1976). Even further, Dumas (2020) has pointed out that the use of different learning strategies is the most proximal factors that contributes to learning outcomes and academic development.
Deep learning strategies tend to result better long-term learning outcomes across different learning situations, and the use of these strategies promotes longer-term recall (Dinsmore and Alexander, 2012; Dunlosky et al., 2013; Barzagar Nazari and Ebersbach, 2019; Badali et al., 2022). Deep learning involves active engagement with learning material, whereby students create connections between concepts and develop the ability to apply knowledge in real-life situations (Fiorella and Mayer, 2015; Agarwal and Bain, 2019). Additionally, deep learning promotes metacognitive awareness, which is essential for effective learning (Rivers et al., 2020; Stanton et al., 2021). Metacognitive awareness or metacognitive knowledge involves understanding one’s own learning processes and strategies, and making adjustments where necessary (Stanton et al., 2021; Gurung et al., 2022). Through the use of deep learning strategies, students can develop metacognitive awareness, which helps in identifying knowledge gaps (Rivers, 2021). Examples of learning strategies that support deep learning include creating connections, self-testing, structuring learning material, making concept maps or schemas, using visuals and examples, elaborating on learning material, and distributing (spacing) learning over time (Dunlosky et al., 2013). However, there is no infinite list of strategies that support deep information processing.
Learning strategies that support surface learning are comparatively simple and tend to support mainly short-term memorization of learning material (Frey et al., 2017). In the case of surface learning, new knowledge is rarely integrated with prior knowledge, which inhibits future recall and the use of new knowledge in novel situations (Weinstein et al., 2011; Roediger and Pyc, 2012). At the same time, it is important to note that using surface learning strategies is necessary, especially among younger learners, to later progress toward using more complex learning strategies (Brod, 2020). Surface learning strategies include massing, rereading, underlining, and linear learning (Dunlosky et al., 2013). Studies have shown that using a combination of surface-learning and deep-learning strategies can support effective learning (Frey et al., 2017; Weinstein et al., 2019). Therefore, it is important that students know about and are able to flexibly use both types of learning strategies depending on the learning goals.
Deep learning strategies support understanding of learning material and the formation of long-term memories (Marton and Säljö, 1976). Various studies have shown that students prefer using learning strategies that support surface rather than deep learning (Karpicke et al., 2009; McCabe, 2011; Dirkx et al., 2019). Dirkx et al. (2019) conducted a survey among Dutch primary school students (N = 316). When provided with a list of learning strategies and asked to mark which strategies they used, most students marked rereading (87.7%), summarizing (76.9%), testing (60.1%), and underlining (26.6%). Answers to open-ended questions indicated that students additionally used practice problems (47.8%), copying (12.3%), and thinking of real-life examples (6%).
Een (2021) asked American high school students (N = 2,082) to rate their use of and the effectiveness of various strategies. For almost all strategies (i.e., retrieval practice, spacing, elaboration, self-explanation, rereading, highlighting, note taking, and outlining), mean ratings were in the middle range. One exception was note-taking, where usage rates were very high. Note-taking is a popular learning strategy among students because it feels easy and familiar (Dunlosky et al., 2013). Note-taking can support surface learning or deep learning, depending on whether the notes cover the important aspects of a given topic or text (Liu and Uesaka, 2022).
In Estonia, several studies have used a specific learning task (i.e., asking students to memorize a list of words during a short period of time) to examine which learning strategies students use as well as students’ metacognitive awareness of the effectiveness of different strategies (Kikas and Jõgi, 2016; Hennok et al., 2022; Kikas et al., 2022). This learning task can be carried out using simple reading and rehearsal (i.e., surface learning strategies) or by grouping words into sentences, stories, or abstract categories (i.e., deep learning strategies). Findings indicate that students report using surface strategies even at the end of upper middle school, and that they value the effectiveness of surface strategies more than deep strategies. Still, Hennok et al. (2022) have shown that upper middle school students tend to associate information more — and value rehearsal less — than younger students.
In the current study students’ metacognitive knowledge of learning strategy effectiveness is evaluated implicitly by reported use of deep learning strategy and evaluations of deep level strategies over surface level strategies.
Effective and meaningful learning in educational contexts does not happen without intention. Students need a well-organized environment and explicit instructions to form healthy study habits and to promote genuine learning (Kirschner et al., 2006). However, research indicates that between teachers’ and students’ knowledge of learning strategies may be disconnect. Several studies have shown that teachers’ knowledge of learning strategies tends to be good (Granström et al., 2022; Surma et al., 2022), but nonetheless, teachers typically cover learning strategies only indirectly and rarely discuss the process of learning (Kistner et al., 2010; Granström et al., 2023). This means that teachers use different learning strategies in teaching (e.g., models, examples from daily life) but do not practice the use of these strategies with students, nor do they discuss the benefits of various learning strategies with students (Granström et al., 2023). This, however, suggests that teachers lack the skills to teach learning strategies and to link them to learning, which also affects students’ knowledge of learning strategies.
As a result of their lower-level cognitive skills (e.g., limited working memory resources and reasoning skills) and poorer subject-related knowledge (Schwenck et al., 2009; Schleepen and Jonkman, 2012), younger students often rely on easily applicable surface strategies like re-reading and rehearsal, and have probably experienced their effectiveness. Gaining knowledge about complex, or deep learning strategies, and understanding when to employ them, necessitates a shift in existing implicit knowledge and beliefs, often referred to as conceptual change. Empirical studies have emphasized the key role of explicit teaching about learning strategies, discussing learning processes, and practicing deep strategies (Dignath et al., 2008; Hattie and Donoghue, 2016). Without explicit instruction, many students may not understand how to effectively learn, or how and when to use deep learning strategies. Moreover, even if students are explicitly taught about strategies, they may perceive this information differently depending on their earlier knowledge and reasoning skills (Brod, 2020).
Thus, in addition to examining teacher knowledge and practices, it is important to study students’ recollections of what they have been taught. Starting from 2016, Estonian ninth grade students could fill out a survey before mandatory basic school math and Estonian language exams that taps their learning skills and motivation related to these academic areas. Every year, an overview of findings is provided on The Education and Youth Board website (HARNO, 2021). Results cover metacognitive knowledge and reported application of learning strategies as well as information about which strategies teachers have taught. The findings have been consistent since the beginning of this project in 2016. To illustrate, 4,642 students participated in 2016 (Kikas and Jõgi, 2016), and 94% reported that teachers had taught rehearsal, while just 22% reported that teachers had taught abstract grouping. In contrast, 77% of teachers reported teaching rehearsal and 71% abstract grouping. In general, these background questionnaires suggest that, for students at the end of upper middle school, learning strategies that support surface learning are the most memorable and often used strategies.
The disconnect between teachers’ and students’ knowledge of learning strategies is worth further study, particularly if students who report having been taught about certain strategies have better knowledge of those strategies. To the best of our knowledge, no prior study has explicitly asked students to recall teacher recommendations about how to prepare for a comprehensive exam and compared those recollections with students’ knowledge and use of learning strategies. In addition, variable-centered approaches tend to rely on linear relations and general trends. As it is known that there is variation in students’ knowledge and outcomes, we used person-centered techniques to identify relevant subgroups.
This study aimed to examine students’ metacognitive knowledge and reported use of learning strategies as well as their recollections of what strategies teachers recommend when preparing for a comprehensive test. The study was carried out among Estonian students in Grades 8 and 9. We selected these grades because, in Estonia, students have to take tests at the end of upper middle school (i.e., at the end of Grade 9), and we reasoned that, when preparing students for these tests, teachers are likely to recommend strategies for learning. We used a Configural Frequency Analysis to tap specific subgroups of response patterns in an effort to uncover if recollections of teachers’ recommendations are related to metacognitive knowledge and reported use of learning strategies.
Students’ metacognitive knowledge and reported use of learning strategies was assessed with a scenario-based questionnaire (McCabe, 2011; Granström et al., 2022; Surma et al., 2022; Granström and Kikas, 2023). Specifically, we asked students about their preparation for a comprehensive biology exam which was scheduled to take place 2 months thereafter. The questionnaire focused on biology because it is known for its unique subject-specific vocabulary and multidisciplinary approach, where models and schemas complement theoretical descriptions. When learners do not fully comprehend new vocabulary, or they lack the ability to use visual aids effectively, their ability to follow and understand the subject is compromised. Thus, effective learning of biology requires the employment of diverse learning strategies (Nordell, 2009). Metacognitive knowledge of learning strategies was further examined via students’ ability to differentiate between strategies that promote deep learning versus strategies that promote surface learning. Reported use of effective learning strategies was examined via the number of deep learning strategies students had selected. Students’ recollections of strategy instruction (i.e., perceived instruction) were categorized into three types – deep strategies, surface strategies and general advice on learning (see Appendix A).
Our research was guided by the following questions:
1. How do students evaluate and differentiate deep and surface learning strategies? Which strategies do students prefer to use (surface vs. deep strategies)? Given prior research indicating poor metacognitive knowledge of strategy effectiveness (McCabe, 2011; Kikas and Jõgi, 2016), we expected students to favor surface strategies in both evaluations and reported use.
2. What learning strategies do students recall having been taught? Do students recall a greater number of deep or surface strategies? On the one hand, teachers have good knowledge of effective learning strategies (Granström et al., 2022), but prior research suggests students typically recall surface strategies (e.g., rereading text and rehearsal of tasks; Karpicke et al., 2009).
3. How do recollections of teacher recommendations correspond to students’ ability to differentiate between deep and surface learning strategies and their reported use of deep learning strategies? We expected that students who report using deep learning strategies more frequently would evaluate deep strategies higher than surface and would prefer using deep strategies.
Participants included 1,504 students (890 from Grade 8 and 614 from Grade 9; 786 boys and 718 girls) from 34 schools across Estonia. Of these, 871 students studied in Estonian-speaking schools and 633 in Russian-speaking schools. The mean age of participants was 14.94 years (min = 13 years; max = 17 years; SD = 0.73).
Students were assessed with a web-based assessment tool as part of the project “Developing tools for assessing science-related motivation.” In March 2022, the Education and Youth Board (HARNO) invited schools to participate in the study, after which specific instructions were given to teachers regarding the questionnaire. Students were assessed in April–May 2022. Teachers were able to choose the most suitable testing day during a predetermined period of time. Students took the tests in computer labs during regular school days and were supervised by their teachers. Completing the entire test took approximately 45 min. Before the assessment began, students and their parents were informed about the content of the questionnaire, and students were permitted to discontinue the test at any time. Only questions used in this study are described below.
All tasks and questions were developed specifically for this questionnaire based on prior scenario-based studies (McCabe, 2011; Surma et al., 2022; Granström and Kikas, 2023). The questionnaire consisted of three parts: recollections of teachers’ recommendations, knowledge of strategy effectiveness and reported use of strategies, which were all related to the same learning scenario. Students were given a description of the learning task, i.e., learning scenario: “In two months, you will have to take a complex biology exam. There will be questions and problems covering all chapters in your textbook. Your aim is to learn about these topics so that you can get a good grade on the exam.”
Students were asked if their teachers had given recommendations about how to prepare for this kind of exam. If they answered yes, students were asked to write down a maximum of three teacher recommendations they recalled.
Responses were categorized by the three authors of the paper. A total of 17 distinct categories of descriptions were identified (see Appendix A). The interrater reliability statistic Kappa was 0.89. Descriptions included 14 learning strategies that were further categorized as either supporting deep or surface learning (six supported surface learning and eight supported deep learning). Other answers included general advice on learning (e.g., “pay attention”), classroom activities (e.g., “teacher used visual aids”), and descriptions of motivational and emotional support from teachers (e.g., “be calm,” “take it easy”).
For Configural Frequency Analysis, students were classified into three groups: (1) No recollection (i.e., students who did not report any learning strategy, or reported general advice that could not be accounted as a specific strategy); (2) Surface (i.e., students who reported only surface learning strategies); and (3) Deep (i.e., students who reported at least one deep learning strategy).
Next, students evaluated the effectiveness of eight descriptions of learning strategies that were related to deep learning and surface learning for achieving the aim (i.e., to acquire good knowledge and get a good grade on the exam). Students answered on a 5-point scale (1 — not at all effective […] 5 — very effective). Four of these strategies supported deep learning and four supported surface learning (see Appendix B). Since the eight descriptions were related and comprised of four pairs, we created a composite variable (differential variable) to capture this relationship. We conceptualized metacognitive knowledge of learning strategies by how well students distinguished the effectiveness of deep and surface strategies. The composite variable was derived by subtracting the evaluation of deep learning strategy score from the evaluation of surface learning strategy score and summing up the different scores. The theoretical range of the score was −16 to +16, and the actual range was −12 to +16. Low scores indicated students who considered surface strategies more effective than deep strategies; middle scores indicated students who slightly preferred one over other or did not distinguish between learning strategies; and high scores indicated students who considered deep strategies more effective than surface strategies. Internal reliability of the composite score was acceptable (Cronbach’s alpha = 0.65).
The spread of the data indicated that there were deviations in responses. Three groups of students were differentiated after further analyses. A low group included 27.3% of students who scored −12 to 0 (i.e., they valued surface strategies over deep strategies or did not distinguish between the two types of strategies; low knowledge group). A moderate group included 50.7% of students who scored 1 to 7 (i.e., they slightly valued deep strategies over surface strategies; moderate knowledge group). A high group included 22% of students who scored 8 to 16 (i.e., they valued deep strategies over surface strategies; high knowledge group).
Last, students were presented with the same eight strategies in four pairs, with one deep and one surface strategy in each pair. Then, students were asked to mark which of the two strategies they would use. Reported use was the sum of marked deep learning strategies. For subsequent analyses, we grouped students into two groups. The low reported application group included 54.4% of students who scored 0–2 (low use), and the high reported application group included 45.6% of students who scored 3–4 (high use).
To answer the first two research questions (i.e., metacognitive knowledge and students’ perceived instruction), we looked at descriptive statistics, distributions, and percentages of student responses. The difference between deep and surface strategy evaluations were tested with Paired Samples t-Test.
To answer the third research question (i.e., the relationship between response patterns and perceived instruction), a configural frequency analysis (CFA) was conducted. CFA is a person-oriented method to analyze contingency tables. The goal is to identify significant types and antitypes (Stemmler, 2020; von Eye and Wiederman, 2021). A pattern in a contingency table is considered a type if it occurs more often than expected. A pattern is considered an antitype if it occurs less often than expected. For testing the difference between observed patterns and expected patterns, the Chi-square approximation to the z-test (Z.Chi) was used (Stemmler, 2020). To control alpha-level inflation, we used Bonferroni alpha adjustment. Locally significant types and antitypes were also discussed.
Descriptive statistics for students’ raw evaluations of the effectiveness of learning strategies are provided in Appendix B. Central tendencies indicate a trend among students to evaluate strategies that support deep learning higher (M = 14.4; SD = 3.22) than strategies that support surface learning (M = 10.7; SD = 2.92). A paired samples t-test was conducted, revealing a statistically significant difference, t (1,502) = 30.6, p < 0.001, between the two measures (average score of deep strategies and average score of surface strategies; see Appendix B). Overall, averages were centered toward the middle of the scale, meaning students tended to evaluate all strategies as moderately effective. The same is reflected in Table 1 which presents descriptive statistics for score differences (pairs are described in Appendix B). The central tendencies are close to zero, indicating overall low differentiation between the effectiveness of deep and surface strategies.
Table 1. Descriptive statistics for distinguishing the effectiveness of surface and deep strategies.
For students’ reported use of deep learning strategies, the mean score was 2.80 (SD = 1.15). The distribution of scores were as follows: 0 = 4.3%; 1 = 10.2%; 2 = 20.9%; 3 = 30.2%; 4 = 34.3%.
In total, 755 (50.2%) of students reported that teachers had provided recommendations how to learn. Students who reported that their teachers had given them recommendations were further asked to list up to three strategies that their teacher had recommended. Among these students 62.5% students provided three recommendations, 23.8% two recommendations, and 13.6% one recommendation. Overall, we gathered 1,879 students’ recollections of teachers’ recommendations. From these recollections 676 (35.9%) referred to deep strategies, 603 (32%) surface strategies and 600 (31.9%) to more general advise. Among students who recalled receiving recommendations from teachers, the most commonly mentioned strategies were surface-level, including methods like rereading (reported by 218 students, 28.9%) and rehearsal (reported by 217 students, 28.7%). However, it is noteworthy that deep learning strategies held prominence, with systematization or summarization of material being recollected by 188 students (24.9%), distribution of learning (reported by 127 students, 16.8) and self-testing (reported by 119 students, 15.8%). See the full list of frequencies of students reported strategies in Appendix A.
Students’ metacognitive knowledge of learning strategy effectiveness and reported use were assessed as a joint response pattern, distinguishing six patterns of responses. In other words, students were classified into groups based on their evaluations of strategies and reported use of deep strategies (see the Measures section). The distribution of response patterns is as follows: low knowledge-low use (19.23%); low knowledge-high use (7.98%); moderate knowledge-low use (14.3%); moderate knowledge-high use (36.26%); high knowledge-low use (1.93%); and high knowledge-high use (20.29%).
The original contingency table that the CFA is based on can be found in Appendix D. The CFA, employing a Bonferroni-adjusted alpha, revealed a type (response pattern and recollection occurring more frequently than by chance) and an antitype (response pattern and recollection occurring less commonly than by chance) (see Table 2).
Table 2. Configural frequency analysis of student knowledge, reported use, and classification by type of recollection.
Among students who could recall at least one deep strategy, they tended to belong to the high knowledge and high usage response pattern group. Conversely, it was rare for individuals in this group to belong to the low knowledge and low usage group. Without accounting for Bonferroni-adjustment of alpha, it was typical for students who did not have any strategy recollection to belong to the low knowledge and low use group, and it was atypical for these students to belong to the high knowledge and high use group.
Our study explored students’ metacognitive knowledge, their reported use of learning strategies and the extent to which they recall their teachers’ recommendations for learning strategies. Furthermore, we examined the relationship between these recollections and the students’ knowledge and use of learning strategies. The findings refer to differences in knowledge and use of learning strategies among students who remember that their teachers have recommended deep learning strategies. Namely, in this group, there are more students who value and report using deep strategies and do not value and use surface strategies. This indicates a possible impact of teachers’ instructional guidance of learning strategies (Dignath et al., 2008). However, it is worth noting that among all respondents, this applies to only a small minority of students. Additionally, our results indicate a general lack of instructional guidance regarding learning strategies and its possible impact on students’ knowledge and preferences.
The study identified a modest trend in which students slightly preferred deep learning strategies over surface strategies. However, the distinction in effectiveness between deep and surface strategies was minimal. This observation is consistent with prior research, which suggests that students’ preference for surface strategies may stem from an insufficient understanding of the effectiveness of various learning strategies (Karpicke et al., 2009; McCabe, 2011). By enhancing students’ metacognitive knowledge, we can empower them to make more informed choices regarding the most appropriate strategies to apply in different learning contexts. When students were given the choice between employing deep or surface learning strategies, a substantial proportion reported predominantly or nearly exclusively opting for deep strategies. However, the presence of students scoring at the low end of the scale points to a critical subset that may utilize only surface level strategies (see also the Limitations and Conclusions sections for further discussion).
Regarding student recollections, we sought to investigate how students perceive the instruction of learning strategies. After categorizing students’ descriptions of perceived instruction, results were similar to prior studies (Karpicke et al., 2009; Kikas and Jõgi, 2016; Kikas et al., 2022). Namely, students report most frequently surface strategy instruction, such as rereading and rehearsal. One explanation for this phenomenon is that these strategies are rather domain general; that is, they are applicable in a wide variety of situations (Dumas, 2020) and they are easy to use (Dunlosky et al., 2013).
Our approach differs from the more common inquiry into what strategies students report using (e.g., Karpicke et al., 2009); instead, we asked about the strategies teachers recommended to them. The results of our study raise some concerns. A significant majority of students reported that their teachers had not provided any specific recommendations on how to study for the test described in the scenario. Even among students who recalled receiving recommendations, their descriptions often included generic advice like “pay attention,” “learn,” or “be smart,” none of which provide explicit instruction about learning strategies. These findings may indicate that students may not have fully understood the question we posed to them, possibly because they have not conceptualized learning as a series of procedures aimed at enhancing their understanding in specific contexts. This could be due to the absence of explicit strategy instruction.
In addition, the results on students’ recollections and metacognitive knowledge refer to the role of teacher recommendations in shaping students’ learning strategy preferences. Students who could recall recommendations for deep learning strategies were more likely to report high metacognitive knowledge and usage of these strategies. This aligns with previous research highlighting the influence of explicit instruction on students’ strategy use and metacognitive awareness (Rogiers et al., 2020). It also addresses the research gap in studying students’ recollections of teachers’ strategic instructions in regular classrooms and demonstrates the importance of explicit teaching in guiding students toward more effective learning practices (Granström et al., 2023). Furthermore, among students who did not recall receiving any strategy instruction, the patterns were not as pronounced as those observed in students who reported receiving instruction on deep learning strategies. Our analysis revealed that the preferences of these students were more randomly distributed. Regardless, there was some evidence indicating that students who reported not receiving any strategy instruction tended to have less knowledge of effective strategies and showed a lower preference for using them. This finding is consistent with the notion that without explicit instruction, students are unlikely to spontaneously become self-regulated learners or develop an understanding of cognitive processes involved in the learning process, as suggested by Bjork et al. (2012).
Our study showed that about half of 8th–9th grade students could not name any learning strategy that teachers had recommended for preparing for complex exam. Moreover, more than third of students gave general recommendations (learn, pay attention), not specific learning strategies. Also, the most frequently mentioned learning strategies were those supporting surface learning – rereading, rehearsal. These are easy-to-use strategies that students have possibly learnt already in primary school and used for long time (Schwenck et al., 2009; Schleepen and Jonkman, 2012). Although earlier studies have indicated that teachers tend to have good knowledge of deep learning strategies (Granström et al., 2022; Surma et al., 2022), this knowledge is not visible in students’ recollections. Differently from surface strategies, learning about deep learning strategies presumes explicit teaching, time, and practicing (Dignath et al., 2008; Hattie and Donoghue, 2016). An earlier observational study in Estonia showed that teachers rarely discuss about learning and learning strategies explicitly (Granström et al., 2023). Our findings indicate that without explicit discussions, many students cannot even name learning strategies. Furthermore, when students are able to recall effective strategies, they tend to these higher and report preferring them to use. This highlights the necessity to encourage teachers to explicitly discuss and give instructions on effective learning strategies.
The study has several limitations. First, although we asked about teacher recommendations, students’ answers might include not only recommendations but what they thought is appropriate for learning. Thus, even fewer students might really recall what teachers had taught. Second, students had to evaluate and choose between pairs of learning strategies, one of which supports deep and another surface learning. The wording of the strategies might have introduce some imbalance. Still, the strategies were formulated in the way that students could recognize the more adaptive deep learning strategy. Earlier studies in secondary school have used different methodology (McCabe, 2011; Dirkx et al., 2019). While the latter studies showed students’ preferences toward strategies supporting surface learning, our results indicate a more balanced evaluation, with students rating the effectiveness of both strategy types as moderately effective. This is evidenced in student responses clustering around the middle of the rating scale (Een, 2021), and students’ ratings of deep strategies are slightly higher than surface strategies. As we recognize the possible limitations, we maintain that these aspects do not detract from the core conclusions of our research. Third, we studied students in the end of middle school and further studies are needed with younger and older students.
In conclusion, our study showed that about half of students could name learning strategies that teachers had recommended. Moreover, only a minority of students recalled deep learning strategies. However, students who recalled deep strategies rated typically these strategies higher and reported preferring using them over surface strategies. In line with an earlier observational study (Granström et al., 2023), the findings refer to the shortages in teaching about learning and learning strategies. Teachers need practical courses how to integrate teaching of learning strategies in their subject lessons. Furthermore, we maintain that the explicit instruction of deep learning strategies not only enhances effective learning but also encourages students to become self-regulated learners.
The raw data supporting the conclusions of this article will be made available by the authors, without undue reservation.
Ethical approval was not required for the study involving human samples in accordance with the local legislation and institutional requirements. Written informed consent for participation in this study was provided by the participants’ legal guardians/next of kin.
JO: Conceptualization, Methodology, Writing – original draft, Writing – review & editing. MG: Conceptualization, Writing – original draft, Writing – review & editing. EK: Conceptualization, Data curation, Methodology, Supervision, Writing – original draft, Writing – review & editing.
The author(s) declare financial support was received for the research, authorship, and/or publication of this article. This study was supported by the grant from Tallinn University, Estonia (TF3818) to JO and EK and by School of Natural Sciences and Health, Tallinn University.
The authors declare that the research was conducted in the absence of any commercial or financial relationships that could be construed as a potential conflict of interest.
All claims expressed in this article are solely those of the authors and do not necessarily represent those of their affiliated organizations, or those of the publisher, the editors and the reviewers. Any product that may be evaluated in this article, or claim that may be made by its manufacturer, is not guaranteed or endorsed by the publisher.
The Supplementary material for this article can be found online at: https://www.frontiersin.org/articles/10.3389/feduc.2024.1307485/full#supplementary-material
Agarwal, P. K., and Bain, P. M. (2019). Powerful teaching: Unleash the science of learning : John Wiley & Sons.
Badali, S., Rawson, K. A., and Dunlosky, J. (2022). Do students effectively regulate their use of self-testing as a function of item difficulty? Educ. Psychol. Rev. 34, 1651–1677. doi: 10.1007/s10648-022-09665-6
Barzagar Nazari, K., and Ebersbach, M. (2019). Distributing mathematical practice of third and seventh graders: applicability of the spacing effect in the classroom. Appl. Cogn. Psychol. 33, 288–298. doi: 10.1002/acp.3485
Bjork, R. A., Dunlovsky, J., and Kornell, N. (2012). Self-regulated learning: beliefs, techniques, and illusions. Annu. Rev. Psychol. 64, 417–444. doi: 10.1146/annurev-psych-113011-143823
Brod, G. (2020). Generative learning: which strategies for what age? Educ. Psychol. Rev. 33, 1295–1318. doi: 10.1007/s10648-020-09571-9
Craik, F. I. M., and Lockhart, R. S. (1972). Levels of processing: a framework for memory research. J. Verbal Learn. Verbal Behav. 11, 671–684. doi: 10.1016/S0022-5371(72)80001-X
Dignath, C., Buettner, G., and Langfeldt, H.-P. (2008). How can primary school students learn self-regulated learning strategies most effectively?: a meta-analysis on self-regulation training programmes. Educ. Res. Rev. 3, 101–129. doi: 10.1016/j.edurev.2008.02.003
Dinsmore, D. L. (2017). Toward a dynamic, multidimensional research framework for strategic processing. Educ. Psychol. Rev. 29, 235–268. doi: 10.1007/s10648-017-9407-5
Dinsmore, D. L., and Alexander, P. A. (2012). A critical discussion of deep and surface processing: what it means, how it is measured, the role of context, and model specification. Educ. Psychol. Rev. 24, 499–567,
Dirkx, K. J. H., Camp, G., Kester, L., and Kirschner, P. A. (2019). Do secondary school students make use of effective study strategies when they study on their own? Appl. Cogn. Psychol. 33, 952–957. doi: 10.1002/acp.3584
Dumas, D. (2020). Strategic processing within and across domains of learning in handbook of strategies and strategic processing. London: Routledge.
Dunlosky, J., Rawson, K. A., Marsh, E. J., Nathan, M. J., and Willingham, D. T. (2013). Improving students’ learning with effective learning techniques: promising directions from cognitive and Educational Psychology. Psychol. Sci. Public Interest 14, 4–58. doi: 10.1177/1529100612453266
Een, E. (2021). Changes in usage and perceptions of effectiveness of learning strategies of high school students during a rigorous academic experience.
Fiorella, L., and Mayer, R. E. (2015). Eight ways to promote generative learning. Educ. Psychol. Rev. 28, 717–741. doi: 10.1007/s10648-015-9348-9
Frey, N., Fisher, D., and Hattie, J. (2017). Surface, deep, and transfer? Considering the role of content literacy instructional strategies. J. Adolesc. Adult. Lit. 60, 567–575. doi: 10.1002/jaal.576
Granström, M., Härma, E., and Kikas, E. (2022). Teachers’ knowledge of learning strategies. Scand. J. Educ. Res. 290–308. doi: 10.1080/00313831.2022.2074536
Granström, M., and Kikas, E. (2023). Õpetajate ja õpilaste hinnangud õpistrateegiate tõhususele: ülevaade Eesti koolide tulemustest. Eesti Haridusteaduste Ajakiri 11, 98–128. doi: 10.12697/eha.2023.11.2.05
Granström, M., Kikas, E., and Eisenschmidt, E. (2023). Classroom observations: how do teachers teach learning strategies? Front. Educ. 8:9519. doi: 10.3389/feduc.2023.1119519
Gurung, R. A. R., Mai, T., Nelson, M., and Pruitt, S. (2022). Predicting learning: comparing study techniques, perseverance, and metacognitive skill. Teach. Psychol. 49, 71–77. doi: 10.1177/0098628320972332
HARNO, T.E. (2021). Lõpueksamite taustaküsitluse tulemused 2021. Available at: https://harno.ee/eksamite-taustakusitlused
Hattie, J. A. C., and Donoghue, G. M. (2016). Learning strategies: a synthesis and conceptual model. NPJ Sci. Learn. 1, 1–13. doi: 10.1038/npjscilearn.2016.13
Hennok, L., Mädamürk, K., and Kikas, E. (2022). Memorization strategies in basic school: grade-related differences in reported use and effectiveness. Eur. J. Psychol. Educ. doi: 10.1007/s10212-022-00630-z
Karpicke, J. D., Butler, A. C., and Roediger, H. L. (2009). Metacognitive strategies in student learning: do students practise retrieval when they study on their own? Memory 17, 471–479. doi: 10.1080/09658210802647009
Kikas, E., and Jõgi, A.-L. (2016). Assessment of learning strategies: self-report questionnaire or learning task. Eur. J. Psychol. Educ. 31, 579–593. doi: 10.1007/s10212-015-0276-3
Kikas, E., Mädamürk, K., Hennok, L., Sigus, H., Talpsep, T., Luptova, O., et al. (2022). Evaluating the efficacy of a teacher-guided comprehension-oriented learning strategy intervention among students in grade 4. Eur. J. Psychol. Educ. 37, 509–530. doi: 10.1007/s10212-021-00538-0
Kirschner, P. A., Sweller, J., and Clark, R. E. (2006). Why minimal guidance during instruction does not work: an analysis of the failure of constructivist, discovery, problem-based, experiential, and inquiry-based teaching. Educ. Psychol. 41, 75–86. doi: 10.1207/s15326985ep4102_1
Kistner, S., Rakoczy, K., Otto, B., Dignath, C., Büttner, G., and Klieme, E. (2010). Promotion of self-regulated learning in classrooms: investigating frequency, quality, and consequences for student performance. Metacogn. Learn. 5, 157–171. doi: 10.1007/s11409-010-9055-3
Liu, M., and Uesaka, Y. (2022). Factors that influence deep/shallow lecture notetaking: Japanese and Chinese students’ strategies in math class. J. Appl. Res. Mem. Cogn. doi: 10.1037/mac0000075
Marton, F., and Säljö, R. (1976). On qualitative differences in learning: I—outcome and process. Br. J. Educ. Psychol. 46, 4–11. doi: 10.1111/j.2044-8279.1976.tb02980.x
McCabe, J. (2011). Metacognitive awareness of learning strategies in undergraduates. Mem. Cogn. 39, 462–476. doi: 10.3758/s13421-010-0035-2
Mizumoto, A., and Takeuchi, O. (2009). Examining the effectiveness of explicit instruction of vocabulary learning strategies with Japanese EFL university students. Lang. Teach. Res. 13, 425–449. doi: 10.1177/1362168809341511
Nordell, S. E. (2009). Learning how to learn: a model for teaching students learning strategies. Biosci. J. Coll. Biol. Teach. 35, 35–42,
Rivers, M. L. (2021). Metacognition about practice testing: a review of learners’ beliefs, monitoring, and control of test-enhanced learning. Educ. Psychol. Rev. 33, 823–862. doi: 10.1007/s10648-020-09578-2
Rivers, M. L., Dunlosky, J., and Persky, A. M. (2020). Measuring metacognitive knowledge, monitoring, and control in the pharmacy classroom and experiential settings. Am. J. Pharm. Educ. 84:7730. doi: 10.5688/ajpe7730
Roediger, H. L., and Pyc, M. A. (2012). Inexpensive techniques to improve education: applying cognitive psychology to enhance educational practice. J. Appl. Res. Mem. Cogn. 1, 242–248. doi: 10.1016/j.jarmac.2012.09.002
Rogiers, A., Merchie, E., and Van Keer, H. (2020). Learner profile stability and change over time: the impact of the explicit strategy instruction program “learning light”. J. Educ. Res. 113, 26–45. doi: 10.1080/00220671.2019.1711005
Schleepen, T. M. J., and Jonkman, L. M. (2012). Children's use of semantic organizational strategies is mediated by working memory capacity. Cogn. Dev. 27, 255–269. doi: 10.1016/j.cogdev.2012.03.003
Schwenck, C., Bjorklund, D., and Schneider, W. (2009). Developmental and individual differences in young Children's use and maintenance of a selective memory strategy. Dev. Psychol. 45, 1034–1050. doi: 10.1037/a0015597
Stanton, J. D., Sebesta, A. J., and Dunlosky, J. (2021). Fostering metacognition to support student learning and performance. CBE—life sciences. Education 20:fe3,
Stemmler, M. (2020). Person-centered methods: Configural frequency analysis (CFA) and other methods for the analysis of contingency tables. Cham, Switzerland: Springer Nature.
Surma, T., Camp, G., de Groot, R., and Kirschner, P. A. (2022). Novice teachers’ knowledge of effective study strategies. Front. Educ. 7:39. doi: 10.3389/feduc.2022.996039
Van Meter, P., and Campbell, J. M. (2020). “Commentary: a conceptual framework for defining strategies and strategic processing” in Handbook of strategies and strategic processing. eds. D. L. Dinsmore, L. K. Fryer, and M. M. Parkinson (New York, USA: Routledge).
Veenman, M. V. J. (2017). “Learning to self-monitor and self-regulate” in Handbook of research on learning and instruction. eds. R. Mayer and P. Alexander. 2nd ed (New York, NY: Routledge).
von Eye, A., and Wiederman, W. (2021). Configural frequency analysis. Foundations, models, and applications. Berlin, Heidelberg: Springer.
Weinstein, Y., McDermott, K. B., and Szpunar, K. K. (2011). Testing protects against proactive interference in face–name learning. Psychon. Bull. Rev. 18:518. doi: 10.3758/s13423-011-0085-x
Weinstein, Y., Sumeracki, M., and Caviglioli, O. (2019). Understanding how we learn: a visual guide. Florence, KY, USA: Routledge.
Keywords: metacognitive knowledge, learning strategies, teacher instruction, configural frequency analysis, strategy effectiveness
Citation: Olop J, Granström M and Kikas E (2024) Students’ metacognitive knowledge of learning-strategy effectiveness and their recall of teachers’ strategy instructions. Front. Educ. 9:1307485. doi: 10.3389/feduc.2024.1307485
Received: 04 October 2023; Accepted: 22 April 2024;
Published: 02 May 2024.
Edited by:
Paul Steendijk, Leiden University Medical Center (LUMC), NetherlandsReviewed by:
Florian Krieger, Technical University Dortmund, GermanyCopyright © 2024 Olop, Granström and Kikas. This is an open-access article distributed under the terms of the Creative Commons Attribution License (CC BY). The use, distribution or reproduction in other forums is permitted, provided the original author(s) and the copyright owner(s) are credited and that the original publication in this journal is cited, in accordance with accepted academic practice. No use, distribution or reproduction is permitted which does not comply with these terms.
*Correspondence: Joosep Olop, am9vc2Vwb2xAdGx1LmVl
Disclaimer: All claims expressed in this article are solely those of the authors and do not necessarily represent those of their affiliated organizations, or those of the publisher, the editors and the reviewers. Any product that may be evaluated in this article or claim that may be made by its manufacturer is not guaranteed or endorsed by the publisher.
Research integrity at Frontiers
Learn more about the work of our research integrity team to safeguard the quality of each article we publish.