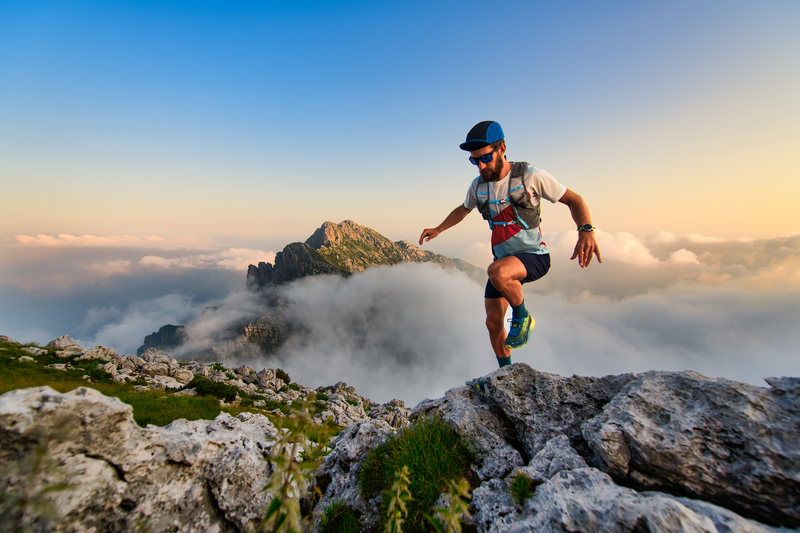
94% of researchers rate our articles as excellent or good
Learn more about the work of our research integrity team to safeguard the quality of each article we publish.
Find out more
ORIGINAL RESEARCH article
Front. Educ. , 08 March 2024
Sec. Educational Psychology
Volume 9 - 2024 | https://doi.org/10.3389/feduc.2024.1293437
This article is part of the Research Topic Chatgpt and Other Generative AI Tools View all 15 articles
Background: Individual beliefs about one’s ability to carry out tasks and face challenges play a pivotal role in academic and professional formation. In the contemporary technological landscape, Artificial Intelligence (AI) is effecting profound changes across multiple sectors. Adaptation to this technology varies greatly among individuals. The integration of AI in the educational setting has necessitated a tool that measures self-efficacy concerning the adoption and use of this technology.
Objective: To adapt and validate a short version of the General Self-Efficacy Scale (GSE-6) for self-efficacy in the use of Artificial Intelligence (GSE-6AI) in a university student population.
Methods: An instrumental study was conducted with the participation of 469 medical students aged between 18 and 29 (M = 19.71; SD = 2.47). The GSE-6 was adapted to the AI context, following strict translation and cultural adaptation procedures. Its factorial structure was evaluated through confirmatory factorial analysis (CFA). Additionally, the factorial invariance of the scale based on gender was studied.
Results: The GSE-6AI exhibited a unidimensional structure with excellent fit indices. All item factorial loads surpassed the recommended threshold, and both Cronbach’s Alpha (α) and McDonald’s Omega (ω) achieved a value of 0.91. Regarding factorial invariance by gender, the scale proved to maintain its structure and meaning in both men and women.
Conclusion: The adapted GSE-6AI version is a valid and reliable tool for measuring self-efficacy in the use of Artificial Intelligence among university students. Its unidimensional structure and gender-related factorial invariance make it a robust and versatile tool for future research and practical applications in educational and technological contexts.
Artificial Intelligence (AI) has emerged as a fundamental pillar in the current technological evolution, significantly impacting various sectors, including education, where its implementation promises to transform teaching and learning methodologies. As we enter this new era of technological changes, adapting and adopting AI becomes crucial. The acceptance of these technologies varies significantly, largely influenced by individual perceptions of technological competence, which becomes a determining factor in this process (Agarwal and Karahanna, 2000; Hsu and Chiu, 2004; Pütten and Von Der Bock, 2018). In the educational sector, tools like ChatGPT have stood out for their ability to optimize efficiency and personalize learning. Incorporating AI in education not only allows for learning that is more tailored to the needs of each student but also fosters self-efficacy and motivation, especially in complex areas such as programming (Zhai et al., 2021; McDiarmid and Zhao, 2023; Yilmaz and Karaoglan Yilmaz, 2023b).
Developing digital competencies and becoming familiar with AI among teachers are key steps to maximize its benefits, such as personalized learning and improved teaching efficiency. However, the integration of AI in education also poses ethical and practical challenges that must be addressed responsibly (Oran, 2023). Generative AI, especially in writing assistance, opens up possibilities for stimulating creativity and overcoming writer’s block, though it raises concerns about dependency and ethics (Washington, 2023). In the healthcare field, AI promises to improve diagnosis and decision-making, necessitating proper AI training and ethical awareness for its effective application (Kwak et al., 2022).
Technological self-efficacy, shaped by previous experiences and the perception of its usefulness, is crucial for the acceptance of AI. Demographic factors, such as income and education level, highlight the importance of overcoming gaps in access and use of AI, proposing a more inclusive approach (Hong, 2022). Self-efficacy has been established as a crucial concept in behavioral and educational psychology in the last decades of the 20th century. This term refers to confidence in one’s abilities to organize and execute actions necessary to manage future situations. Moreover, self-efficacy significantly impacts how individuals set goals, face challenges, and overcome obstacles, being a key element for motivation and human behavior (Bandura, 1977). This concept is relevant not only in educational contexts, where its direct relationship with performance and student motivation has been demonstrated (Pajares, 1996; Zimmerman, 2000), but also in the adoption and adaptation to new technologies, introducing the term “computer self-efficacy” (Compeau and Higgins, 1995).
Advanced technologies, such as Generative Artificial Intelligence (GAI), can enhance students’ self-efficacy, encouraging a deeper cognitive engagement and, consequently, improving their academic outcomes (Liang et al., 2023). This phenomenon highlights the need to carefully integrate AI into educational systems, balancing the benefits of these technologies with critical reflection on their potential drawbacks. Thus, self-efficacy with the use of AI refers to the confidence and individual perception of the ability to employ AI effectively to achieve personal and professional goals. This includes not only the technical handling of AI-based tools but also the ability to integrate these tools into solving specific challenges, adapting to changes, and overcoming difficulties through innovative use of AI. Essentially, this self-efficacy reflects an individual’s ability to apply AI effectively in varied contexts, both in managing daily tasks and in addressing unexpected situations, leveraging AI to enhance their performance.
This multidisciplinary approach to AI self-efficacy demonstrates its potential to mitigate the negative effects of overwork and stress, particularly in workplace and educational settings (Kim et al., 2024). By fostering a healthier and safer environment, AI self-efficacy not only benefits individual wellbeing but also contributes to organizational effectiveness and academic success (Yilmaz and Karaoglan Yilmaz, 2023a). The variability in adopting and adapting to AI among individuals suggests that, beyond technical skills, students’ perceptions of their ability to use AI are crucial in their willingness to adopt these technologies. With the increasing integration of AI across various areas of life and work, several scales have been developed, such as the General Attitudes Towards Artificial Intelligence Scale (GAAIS), which assesses general perceptions of AI. This scale, including subscales to reflect both positive and negative attitudes, was validated in the UK and reveals a division in public perception of AI applications, underscoring the need for future studies to validate the scale in broader and more varied contexts (Schepman and Rodway, 2020). On the other hand, the Medical AI Readiness Scale for Medical Students (MAIRS-MS), developed in Persian and consisting of 22 items across four dimensions, has been validated among medical students in Iran, highlighting the relevance of integrating AI into medical curricula (Moodi et al., 2023). The Artificial Intelligence Anxiety Scale (AIAS), with 21 items spread across four dimensions, was validated in Taiwan (Wang and Wang, 2022). Similarly, the Generative Artificial Intelligence Acceptance Scale, based on the UTAUT model and created with the participation of university students, provides a solid tool for measuring student acceptance of generative AI applications, whose four-factor structure was confirmed through factor analysis (Yilmaz et al., 2023). The Artificial Intelligence Literacy Scales (AILS), adapted to Turkish, assess AI understanding among non-expert adults and youth (Çelebi et al., 2023; Karaoğlan and Yilmaz, 2023).
In the context of self-efficacy, the Artificial Intelligence Self-Efficacy Scale (AISES) was developed, specifically designed to measure the perception of self-efficacy in handling AI technologies, consisting of 22 items covering four fundamental dimensions: assistance, anthropomorphic interaction, comfort with AI, and technological skills. Validated in Taiwan, this scale evaluates self-efficacy in the context of AI, highlighting the complexity of this technology and its impact on individuals in various contexts, both educational and professional. Conversely, the General Self-Efficacy Scale (GSE) is a widely used instrument measuring an individual’s belief in their ability to manage a variety of difficult or challenging situations (Schwarzer and Born, 1997). An abbreviated version of this scale is the GSE-6 (Romppel et al., 2013). Although not specifically focused on AI, its brevity and generalist approach make it useful for extensive studies and situations requiring a quick assessment of self-efficacy. Its use across multiple domains emphasizes the universality of the self-efficacy concept and its applicability in varied life situations.
The comparison between AISES and GSE-6 illustrates the dichotomy between the need for domain-specific measures and more general assessment tools. While AISES provides a detailed and contextual evaluation of self-efficacy in using AI, capturing the specific challenges and peculiarities of this technology, GSE-6 offers a general perspective that can be applied across a wide range of situations, including those related to AI. This distinction highlights the importance of developing and adapting scales that reflect the unique challenges and opportunities presented by AI, suggesting that adapting GSE-6 to the AI context could provide a concise and easily administered measure of AI-related self-efficacy. In this way, a more general tool that still reflects the specificity of the AI context could be offered, benefiting researchers, educators, and professionals interested in assessing and enhancing individuals’ readiness to interact with AI. Therefore, the aim of this study is to adapt and validate a scale of self-efficacy in using Artificial Intelligence among Peruvian students.
An instrumental and cross-sectional study was conducted with the purpose of examining the psychometric properties of a documentary measurement instrument (Ato et al., 2013). Furthermore, a non-random convenience sampling method was used to select medical students from three Peruvian universities who were between their first and tenth study cycles. Students already in the eleventh cycle or beyond, typically engaged in hospital practices, were excluded. An essential inclusion criterion was the use of Artificial Intelligence in their academic training, specifically those students who dedicate at least 8 h a week to activities involving AI use. The sample selection was based on a precise calculation using an electronic calculator (Soper, 2023), considering specific variables such as the number of observed and latent aspects in the proposed model, an expected effect size of λ = 0.10, a statistical significance of α = 0.05, and a statistical power level of 1–β = 0.80. Although the minimum sample size required for the model structure was 200 participants, a total of 469 students were recruited. These participants had ages ranging from 18 to 29 years (M = 19.71; SD = 2.47). It was observed that 53.3% were women, 26.2% were in their first cycle of studies, and 51% came from the coastal region of Peru (Table 1).
Self-Efficacy in Using Artificial Intelligence: The Self-Efficacy in Using Artificial Intelligence Scale was derived from the adaptation of the 6-item General Self-Efficacy Scale (GSE-6) (Romppel et al., 2013), representing a shortened version of the original 10-item GSE scale (Schwarzer and Born, 1997). The GSE-6 assesses an individual’s perceived level of self-efficacy with response options ranging from 1 = “not at all true” to 4 = “exactly true.” To obtain an overall score, responses to all items are summed. Initial values on the reliability of the GSE-6 were adequate, recording Cronbach’s alphas of 0.86, 0.88, and 0.88 in three consecutive evaluations. Moreover, the GSE-6 has demonstrated robust psychometric properties in various cultural contexts and in both clinical and non-clinical samples.
To adapt the GSE-6 to the specific context of Artificial Intelligence use and application, translation and cultural adaptation procedures were followed (Beaton et al., 2000). In the initial phase, three bilingual native Spanish speakers independently translated the GSE-6 into Spanish. This translated version was then back-translated into English by three native English speakers who were not familiar with the scale. Three psychologists and an educator thoroughly reviewed this Spanish translation and, after deliberations, decided to adjust the wording of the 6 items to align with the context of Artificial Intelligence use, resulting in the GSE-6AI version. Additionally, content validation was conducted through expert judgment. To test the readability and comprehensibility of this adaptation, it was administered to a pilot group of 13 medical students. The results indicated clear and readable comprehension (Table 2).
The study was conducted following stringent ethical standards, aligned with the Helsinki Declaration (Puri et al., 2009). It received approval from the Ethics Committee of a Peruvian university (2023-CEUPeU-044). Data collection was carried out in person at three Peruvian universities, ensuring participants that their participation was voluntary and all provided information would be treated anonymously to maintain their privacy and confidentiality. Before participating, informed consent was obtained from each individual, ensuring their rights were respected throughout the research process.
In the preliminary phase, the content validity of the items in the GSE-6AI was assessed by expert judges, who were selected and contacted through both electronic and face-to-face means. This review focused on evaluating three critical aspects of each item: its relevance, determining the importance and essentiality of the item for the construct under study; its coherence, assessing the consonance of the item with the construct it intends to measure; and its clarity, measuring the ease of understanding and the unambiguity of the item. The evaluation of these criteria was performed using a scale from 0 to 3, where 0 indicates the absence and 3 the total presence of the evaluated characteristic. Each item was assessed in an approximate period of 5 min. To quantify these aspects, the Aiken’s V coefficient, along with its 95% confidence intervals, was applied (Aiken, 1980). This procedure was carried out using software specifically designed in MS Excel©. The Aiken’s V coefficient ranges from 0 to 1, where values close to 1 indicate a high degree of clarity, coherence, and relevance. Items with an Aiken’s V coefficient ≥0.70 are considered positively rated at the sample level, and those whose lower limit of the confidence interval exceeds 0.59 are deemed appropriate at the population level (Penfield and Giacobbi, 2004).
Subsequently, a descriptive analysis of the items belonging to the General Self-Efficacy Scale with Artificial Intelligence (GSE-6AI) was conducted. This analysis followed the criteria of Pérez and Medrano (2010), where skewness (g1) and kurtosis (g2) were deemed adequate if their values were within ± 1.5. Items with a corrected item-test correlation [r(i-tc)] of < = 0.2 or that showed signs of multicollinearity (i-tc) < = 0.2 were excluded (Kline, 2016).
Following this descriptive analysis, a Confirmatory Factor Analysis (CFA) was implemented, focusing on the unidimensional aspect of the GSE-6AI scale, using the MLR estimator. This estimator is renowned for its robustness against potential deviations from normality (Muthen and Muthen, 2017). Fit criteria were based on metrics like the chi-square test (χ2). RMSEA and SRMR values below 0.08 and 0.05 indicate acceptable and optimal fit, respectively (Kline, 2011; Bandalos and Finney, 2019). For CFI and TLI, values above 0.90 are recommended, and those exceeding 0.95 denote an excellent model fit (Schumacker and Lomax, 2016).
To ensure the scale’s equivalence across different demographic groups, especially regarding gender, measurement invariance (MI) was evaluated using a multi-group confirmatory factor analysis. Four critical levels of invariance were considered: Configural, Metric, Scalar, and Strict. The criterion adopted for determining invariance between gender groups was based on ΔCFI differences less than 0.010 (Chen, 2007). In terms of internal consistency, both Cronbach’s alpha coefficient and McDonald’s omega coefficient (McDonald, 1999) were used, anticipating values above 0.70 as an indicator of reliability (Raykov and Hancock, 2005).
All statistical processing was performed using R, specifically version 4.1.1. For the CFA and structural equation modeling, the “lavaan” package was applied (Rosseel, 2012). Meanwhile, “semTools” facilitated the measurement invariance analysis, ensuring meticulous interpretation of the findings (Jorgensen et al., 2021).
The table displays the results of the evaluation for relevance, representativeness, and clarity of the items of the assessed instrument, quantified through the Aiken’s V coefficient and their respective 95% confidence intervals (CI 95%). At the sample level, all items showed Aiken’s V values indicating highly positive evaluations in terms of relevance, representativeness, and clarity, with values above 0.70, indicating a high valuation of these aspects. Specifically, items 2 and 6 stand out for achieving perfect scores in clarity (V = 1.00; CI 95%: 0.85–1.00) and representativeness (V = 1.00; CI 95%: 0.85–1.00) for item 3, highlighting their total comprehensibility and alignment with the measured construct. The consistency in high scores across different items reflects a uniformity in the experts’ perception of the content quality of the instrument. Furthermore, the lower limit of the CI 95% for all Aiken’s V values exceeds the established criterion for adequate valuation at the population level (Li > 0.59), underscoring the robustness of the items in terms of relevance, representativeness, and clarity from a broader perspective (Table 3).
In the GSE-6AI descriptive analysis (Table 4), item 4 “Thanks to my wit and AI, I know how to handle unforeseen situations.” reported the highest mean (M = 2.58, SD = 0.87). Meanwhile, items 1 “If someone opposes me, I can find ways to get what I want with AI’s help.” and 6 “No matter what comes up, I can usually handle it with Artificial Intelligence’s support.” shared the lowest mean (M = 2.42). Concerning data normality, all items showed skewness (g1) and kurtosis (g2) values within the acceptable range of ± 1.5, indicating a roughly normal distribution for each item’s responses. Specifically, skewness ranged from −0.21 to 0.08, and kurtosis from −0.55 to −0.70. Evaluating item-total correlations (r.cor), all items exceeded the 0.30 acceptability threshold, with values ranging from 0.67 to 0.77. This suggests each item’s significant contribution to the scale’s overall consistency, so there’s no need to exclude any item based on these correlations.
The GSE-6AI’s confirmatory factor analysis (Figure 1) displayed an adequate model fit to the data. Specifically, the obtained indices were as follows: χ2 = 17.480, df = 9, p < 0.01; CFI = 0.99; TLI = 0.98; RMSEA = 0.04 (90% CI: 0.02–0.07) and SRMR = 0.02. All indices indicate an excellent model fit, considering the generally accepted standards in the literature (Schumacker and Lomax, 2016). Furthermore, all item factor loadings (λ) exceeded the recommended threshold (> 0.50), suggesting each item’s significant contribution to the measured construct. In terms of reliability, the scale’s internal consistency was found to be high, with a Cronbach’s Alpha (α) and McDonald’s Omega (ω) of 0.91, exceeding the generally accepted 0.70 threshold (Raykov and Hancock, 2005).
The sequence of invariance models applied to the GSE-6AI scale among university students to assess its consistency across genders reveals significant findings that support its applicability and reliability in both groups. Starting with configural invariance (M1), which establishes a common baseline factorial structure between genders, the research progressed toward progressively more restrictive levels of invariance: metric (M2), scalar (M3), and strict (M4). The analysis of differences in the Comparative Fit Index (ΔCFI) shows minimal variations between models, with ΔCFI values of 0.003, −0.001, and 0 for the transitions from M1 to M2, M2 to M3, and M3 to M4, respectively. These results, consistently below the threshold of 0.010 proposed by Chen (2007), indicate a solid invariance of the scale across genders, confirming that the psychometric properties of the GSE-6AI are stable between men and women (Table 5).
AI has become a foundational pillar in technological evolution, significantly impacting the educational sector by promising a transformation of teaching and learning methodologies. The acceptance of AI varies according to individual perceptions of technological competence, and its implementation is optimizing both the efficiency and personalization of learning. It’s essential to develop digital skills and familiarize oneself with AI to maximize its benefits, though this entails facing ethical and practical challenges. Technological self-efficacy, determined by previous experiences and the perception of its utility, is crucial for adopting AI. Advances in Generative AI can increase students’ self-efficacy and improve their academic outcomes. The integration of AI in education demands a critical evaluation of its advantages and potential challenges. This underscores the importance of developing tools that address the specific challenges and opportunities presented by AI.
Our research aimed to adapt and validate the Artificial Intelligence Use Self-Efficacy Scale (GSE-6AI), derived from the 6-item General Self-Efficacy Scale (GSE-6). This study responds to the growing interest in understanding how self-efficacy perceptions affect the adoption and use of advanced technologies, such as artificial intelligence (AI). Previous research has examined self-efficacy in technological contexts, highlighting works such as those by Grassini (2023) and Wang and Chuang (2023), who developed scales for measuring self-efficacy and attitudes toward AI, respectively. These contributions are crucial for understanding individuals’ willingness to interact with emerging technologies, key to adopting AI. We adapted the GSE-6 to the realm of AI through a process of translation and content validation, assessing its clarity, coherence, and relevance with Aiken’s V coefficient. Unlike studies like that of Yilmaz et al. (2023), which focused on the acceptance of generative AI, our work concentrates on self-efficacy, emphasizing the role of individual beliefs in the ability to use AI efficiently. The content validity of the GSE-6AI was established through expert review, a crucial step also present in the creation of other instruments, such as the AI Anxiety Scale by Wang and Wang (2022). This process ensures that the items accurately reflect the concept of self-efficacy in using AI. The comparison with the study by Çelebi et al. (2023), on the adaptation of the AI Literacy Scale, highlights the need to address not only self-efficacy but also knowledge and understanding of AI. The results confirm the clarity and applicability of the adapted scale across different cultural contexts and populations, in line with research like that of Moodi et al. (2023), who analyzed the psychometric characteristics of a readiness scale for AI in medical students, demonstrating the usefulness of having specific assessment tools for different areas of AI application.
Additionally, a Confirmatory Factor Analysis (CFA) was conducted for the GSE-6AI, confirming its unidimensionality. When compared with similar studies, such as Wang and Chuang (2023), who developed and validated an AI self-efficacy scale, and Grassini (2023), who adapted a scale for attitudes toward AI, a common trend in the importance of validating the psychometric properties of these instruments across specific cultural contexts and various AI application domains was found. The consistency in the results of these studies highlights the importance of AI-specific scales in assessing psychological constructs within the technological realm, as well as their applicability in various contexts. In this regard, the GSE-6AI demonstrated superior fit indices compared to the previously established Artificial Intelligence Self-Efficacy Scale (AISES). While both instruments aim to measure aspects of self-efficacy, the GSE-6AI presents as a more concise and focused tool for the context of Artificial Intelligence. Moreover, the item factor loadings exceeded the recommended threshold (λ > 0.50), indicating that each item is relevant and reinforces the internal coherence of the scale.
Furthermore, the GSE-6AI has shown high internal consistency, with Cronbach’s Alpha (α) and McDonald’s Omega (ω) coefficients of 0.91, indicating adequate reliability for measuring self-efficacy in the context of artificial intelligence (Raykov and Hancock, 2005). This result is in line with findings from previous studies that evaluated the reliability of similar scales in various contexts, demonstrating the robustness of the GSE-6’s psychometric properties. Research such as that by Grassini (2023), Moodi et al. (2023), and Wang and Chuang (2023) generally report high reliability coefficients for scales related to self-efficacy and attitudes toward artificial intelligence. For instance, Wang and Chuang achieved a Cronbach’s Alpha of 0.852, while Grassini reported Cronbach’s Alpha and McDonald’s Omega values indicating good internal consistency for different factors. These findings underscore the need for reliable measurement tools in the field of artificial intelligence, facilitating accurate comparisons and generalizations across different studies. However, the importance of continuing research to address potential gaps, especially in adapting these scales to specific cultural and linguistic contexts, is recognized. Adaptation studies conducted by Çelebi et al. (2023) and Karaoğlan and Yilmaz (2023) demonstrated high levels of reliability in the adapted versions of the scale, proving the effectiveness of these efforts.
Moreover, the study presents factorial invariance by gender for the GSE-6AI, showcasing a thorough analysis of the scale’s factorial structure, focusing on group comparison by gender. Through a hierarchical methodology, different levels of invariance were tested: configural, metric, scalar, and strict, consistently showing good fits at all levels and suggesting that the scale maintains its structure and meaning across genders, indicating that the scale measures the general self-efficacy construct assisted by artificial intelligence equivalently in both men and women.
The validation of the GSE-6AI offers a significant contribution to the psychometric understanding of how individuals perceive their ability to interact with artificial intelligence technology. Adapting the GSE-6 scale to the AI context not only broadens its scope of applicability but also highlights the importance of domain specificity in evaluating self-efficacy. The rigor in the process of translation and cultural adaptation, followed by validation by experts, ensures that the GSE-6AI is a reliable and relevant tool for measuring self-efficacy in AI use, respecting sociolinguistic variations and adapting to the contextual reality where it is applied. The results of the psychometric validation of the GSE-6AI provide solid evidence of its utility in educational and professional environments, where AI is emerging as a critical tool. Since self-efficacy has been identified as a key predictor of technology adoption, self-directed learning, and the ability to face technological challenges, the GSE-6AI can be used in developing interventions aimed at improving AI-related self-efficacy among students and professionals, thereby facilitating a smoother transition toward integrating AI into various practices. The ability to accurately measure this self-efficacy may lead to a deeper understanding of how individual perceptions of the ability to use AI influence specific behaviors and, ultimately, success in AI adoption.
Identifying self-efficacy in AI use is essential for designing educational interventions aimed at enhancing the integration of these technologies into the classroom. Educators can use the GSE-6AI as a tool to assess and improve students’ confidence in using AI-based tools. Students with low levels of self-efficacy could benefit from specific training programs that provide them with the support and skills necessary to tackle current technological challenges. Educational institutions, in turn, might consider incorporating AI modules or workshops into their curricula, allowing students to become familiar with these technologies from early stages of their education.
Moreover, it is crucial for administrators to recognize the importance of self-efficacy in AI use. This implies promoting educational policies that prioritize training in emerging technologies and that ensure equitable access to these tools, thus preventing the widening of the technological gap. Additionally, considering gender equality is vital, as the scale has shown invariance between men and women, suggesting that both genders perceive their ability to use AI similarly.
We recommend that future research explore the relationship between AI self-efficacy and other relevant constructs, such as academic performance, satisfaction with the learning process, or student wellbeing. It would also be relevant to assess the GSE-6AI in other contexts, such as the workplace or recreational settings, to understand how these beliefs manifest in different areas of daily life.
However, it’s crucial to acknowledge the inherent limitations in developing pan-dialectal versions of psychometric instruments and the need for specific linguistic and cultural adaptations for particular contexts. The GSE-6AI, though validated in a specific context, requires ongoing validation across diverse cultural and educational settings to ensure its generalizability and accuracy in different populations. Additionally, the cross-sectional nature of the study prevents establishing causal relationships between the examined variables. Future research could benefit from longitudinal designs that provide a deeper understanding of the evolution and stability of self-efficacy beliefs in relation to AI over time. Also, the self-reported nature of the data. While self-reported scales are common and valuable tools, they are susceptible to biases such as social desirability. The inclusion of objective assessments, such as performance tests or interviews, could offer a more holistic view of AI-related self-efficacy. Lastly, although gender invariance was analyzed and confirmed, it would be fruitful to explore invariance across other demographic groups, such as different ages, educational levels, or cultural backgrounds. AI is a global tool, and understanding how different populations perceive their self-efficacy in this domain is essential for more inclusive implementation.
The adaptation and validation of the GSE-6AI in the Peruvian educational context represent a significant contribution to understanding individual perceptions of competence in using AI. This study, by confirming the psychometric validity and gender invariance of the GSE-6AI, underscores the importance of technological self-efficacy for successful integration of AI in education and demonstrates the scale’s universality and adaptability to different cultural and educational contexts. The findings support the idea that strengthening AI self-efficacy among students and professionals can facilitate greater acceptance and effective use of these technologies, enhancing associated educational and occupational benefits. However, exploring the implications of these perceptions on various academic and professional outcomes is essential. Longitudinal evaluation of AI self-efficacy can offer deeper insights into how specific interventions could improve technological readiness and overall performance in an increasingly digitalized world.
The raw data supporting the conclusions of this article will be made available by the authors, without undue reservation.
The research was evaluated by the Ethics Committee of the Universidad Peruana Unión (Code: 2023-CEUPeU-044). The studies were conducted in accordance with the local legislation and institutional requirements. The participants provided their written informed consent to participate in this study.
WM-G: Conceptualization, Data curation, Formal Analysis, Investigation, Methodology, Resources, Validation, Visualization, Writing – original draft, Writing – review and editing. LS-S: Conceptualization, Data curation, Formal Analysis, Methodology, Project administration, Resources, Visualization, Writing – original draft, Writing – review and editing. SM-G: Conceptualization, Data curation, Methodology, Resources, Software, Visualization, Writing – original draft, Writing – review and editing. MM-G: Conceptualization, Data curation, Methodology, Software, Validation, Visualization, Writing – original draft, Writing – review and editing.
The author(s) declare that no financial support was received for the research, authorship, and/or publication of this article.
The authors declare that the research was conducted in the absence of any commercial or financial relationships that could be construed as a potential conflict of interest.
All claims expressed in this article are solely those of the authors and do not necessarily represent those of their affiliated organizations, or those of the publisher, the editors and the reviewers. Any product that may be evaluated in this article, or claim that may be made by its manufacturer, is not guaranteed or endorsed by the publisher.
Agarwal, R., and Karahanna, E. (2000). Time flies when you’re having fun: Cognitive absorption and beliefs about information technology usage. MIS Quart. 24, 665–694. doi: 10.2307/3250951
Aiken, L. R. (1980). Content validity and reliability of single items or questionnaires. Educ. Psychol. Measure. 40, 955–959. doi: 10.1177/001316448004000419
Ato, M., López, J., and Benavente, A. (2013). Un sistema de clasificación de los diseños de investigación en psicología. Anal. Psicol. 29, 1038–1059. doi: 10.6018/analesps.29.3.178511
Bandalos, D. L., and Finney, S. J. (2019). “Factor analysis: Exploratory and confi rmatory,” in The reviewer’s guide to quantitative methods in the social sciences, eds G. R. Hancock, L. M. Stapleton, and R. O. Mueller (Berlin: Routledge).
Bandura, A. (1977). Self-efficacy: Toward a unifying theory of behavioral change. Psychol. Rev. 84, 191–215. doi: 10.1037/0033-295X.84.2.191
Beaton, D. E., Bombardier, C., Guillemin, F., and Ferraz, M. B. (2000). Guidelines for the process of cross-cultural adaptation of self-report measures. Spine 25, 3186–3191. doi: 10.1097/00007632-200012150-00014
Çelebi, C., Yilmaz, F., Demir, U., and Karakuş, F. (2023). Artificial intelligence literacy: An adaptation study. Instr. Technol. Lifelong Learn. [Epub ahead of prin]. doi: 10.52911/itall.1401740
Chen, F. F. (2007). Sensitivity of goodness of fit indexes to lack of measurement invariance. Struct. Equat. Model. 14, 464–504. doi: 10.1080/10705510701301834
Compeau, D. R., and Higgins, C. A. (1995). Computer self-efficacy: Development of a measure and initial test. MIS Quart. 19, 189–211. doi: 10.2307/249688
Grassini, S. (2023). Development and validation of the AI attitude scale (AIAS-4): A brief measure of general attitude toward artificial intelligence. Front. Psychol. 14:1191628. doi: 10.3389/fpsyg.2023.1191628
Hong, J. W. (2022). I was born to love AI: The influence of social status on AI self-efficacy and intentions to use AI. Int. J. Commun. 16:20.
Hsu, M. H., and Chiu, C. M. (2004). Internet self-efficacy and electronic service acceptance. Decis. Support Syst. 38, 369–381. doi: 10.1016/j.dss.2003.08.001
Jorgensen, T. D., Pornprasertmanit, S., Schoemann, A. M., and Rosseel, Y. (2021). semTools: Useful tools for structural equation modeling. In The Comprehensive R Archive Network. Available online at: https://CRAN.R-project.org/package=semTools
Karaoğlan, F. G., and Yilmaz, R. (2023). Yapay zekâ okuryazarliği ölçeğinin türkçeye uyarlanması. Bilg. Ýletişim Teknol. Derg. 5, 172–190. doi: 10.53694/bited.1376831
Kim, B.-J., Kim, M.-J., and Lee, J. (2024). Examining the impact of work overload on cybersecurity behavior: highlighting self-efficacy in the realm of artificial intelligence. Curr. Psycho. doi: 10.1007/s12144-024-05692-4
Kline, R. B. (2011). Principles and Practice of Structural Equation Modeling. London: Guilford Press.
Kline, R. B. (2016). Principles and practice of structural equation modeling. London: Guilford Press.
Kwak, Y., Ahn, J. W., and Seo, Y. H. (2022). Influence of AI ethics awareness, attitude, anxiety, and self-efficacy on nursing students’ behavioral intentions. BMC Nurs. 21:267. doi: 10.1186/s12912-022-01048-0
Liang, J., Wang, L., Luo, J., Yan, Y., and Fan, C. (2023). The relationship between student interaction with generative artificial intelligence and learning achievement: serial mediating roles of self-efficacy and cognitive engagement. Front. Psychol. 14:1285392. doi: 10.3389/fpsyg.2023.1285392
McDiarmid, G. W., and Zhao, Y. (2023). Time to rethink: Educating for a technology-transformed world. ECNU Rev. Educ. 6, 189–214. doi: 10.1177/20965311221076493
Moodi, A. A., Moghadasin, M., Emadzadeh, A., and Mastour, H. (2023). Psychometric properties of the persian version of the Medical Artificial Intelligence Readiness Scale for Medical Students (MAIRS-MS). BMC Med. Educ. 23:577. doi: 10.1186/s12909-023-04553-1
Muthen, L., and Muthen, B. (2017). Mplus Statistical Analysis with latent variables. User’s guide. Los Angeles: Muthen & Muthen.
Oran, B. B. (2023). Correlation between artificial intelligence in education and teacher self-efficacy beliefs: a review. RumeliDE Dil ve Edebiyat Araştırmaları Dergisi 34:1316378. doi: 10.29000/rumelide.1316378
Pajares, F. (1996). Self-efficacy beliefs in academic settings. Rev. Educ. Res. 66, 543–578. doi: 10.3102/00346543066004543
Penfield, R. D., and Giacobbi, P. R. (2004). Applying a score confidence interval to Aiken’s item content-relevance index. Measure. Phys. Educ. Exerc. Sci. 8, 213–225. doi: 10.1207/s15327841mpee0804_3
Pérez, E. R., and Medrano, L. (2010). Análisis factorial exploratorio: Bases conceptuales y metodológicas. Rev. Arg. Cienc. Comport. 2, 58–66.
Puri, K. S., Suresh, K. R., Gogtay, N. J., and Thatte, U. M. (2009). Declaration of Helsinki, 2008: Implications for stakeholders in research. J. Postgrad. Med. 55, 131–134. doi: 10.4103/0022-3859.52846
Pütten, A. R., and Von Der Bock, N. (2018). Development and validation of the self-efficacy in human-robot-interaction scale (SE-HRI). ACM Trans. Hum. Robot Interact. 7:3139352. doi: 10.1145/3139352
Raykov, T., and Hancock, G. R. (2005). Examining change in maximal reliability for multiple-component measuring instruments. Br. J. Math. Stat. Psychol. 58, 65–82. doi: 10.1348/000711005X38753
Romppel, M., Herrmann-Lingen, C., Wachter, R., Edelmann, F., Düngen, H.-D., Pieske, B., et al. (2013). A short form of the General Self-Efficacy Scale (GSE-6): Development, psychometric properties and validity in an intercultural non-clinical sample and a sample of patients at risk for heart failure. Psycho-Soc. Med. 10:Doc01. doi: 10.3205/psm000091
Rosseel, Y. (2012). lavaan: An R Package for Structural Equation Modeling. J. Stat. Softw. 48, 1–36. doi: 10.18637/JSS.V048.I02
Schepman, A., and Rodway, P. (2020). Initial validation of the general attitudes towards Artificial Intelligence Scale. Comput. Hum. Behav. Rep. 1:100014. doi: 10.1016/j.chbr.2020.100014
Schumacker, R. E., and Lomax, R. G. (2016). A Beginner’s Guide to Structural Equation Modeling, 4th Edn. Milton Park: Taylor & Francis.
Schwarzer, R., and Born, A. (1997). Optimistic self-beliefs: Assessment of general perceived self-efficacy in thirteen cultures. Berlin: Freie Universität Berlin.
Soper, D. (2023). A-priori Sample Size Calculator for structural equation models. Software. Available online at: http://wwwdanielsopercom/statcalc (accessed on 30 July 2023).
Wang, Y.-Y., and Chuang, Y.-W. (2023). Artificial intelligence self-efficacy: Scale development and validation. Educ. Inf. Technol. [Epub ahead of print]. doi: 10.1007/s10639-023-12015-w
Wang, Y. Y., and Wang, Y. S. (2022). Development and validation of an artificial intelligence anxiety scale: an initial application in predicting motivated learning behavior. Interact. Learn. Environ. 30:8812542. doi: 10.1080/10494820.2019.1674887
Washington, J. (2023). The impact of generative artificial intelligence on writer’s self-efficacy: A critical literature review. SSRN Electron. J. doi: 10.2139/ssrn.4538043
Yilmaz, F. G. K., Yilmaz, R., and Ceylan, M. (2023). Generative artificial intelligence acceptance scale: A Validity and Reliability Study. Int. J. Hum. Comput. Interact. [Epuba haed of print]. doi: 10.1080/10447318.2023.2288730
Yilmaz, R., and Karaoglan Yilmaz, F. G. (2023a). Augmented intelligence in programming learning: Examining student views on the use of ChatGPT for programming learning. Comput. Hum. Behav. 1:100005. doi: 10.1016/j.chbah.2023.100005
Yilmaz, R., and Karaoglan Yilmaz, F. G. (2023b). The effect of generative artificial intelligence (AI)-based tool use on students’ computational thinking skills, programming self-efficacy and motivation. Comput. Educ. 4:100147. doi: 10.1016/j.caeai.2023.100147
Zhai, X., Chu, X., Chai, C. S., Jong, M. S. Y., Istenic, A., Spector, M., et al. (2021). A review of artificial intelligence (AI) in education from 2010 to 2020. Complexity 2021:8812542. doi: 10.1155/2021/8812542
Keywords: self-efficacy, artificial intelligence (AI), invariance, technological, adaptation, GSE-6AI
Citation: Morales-García WC, Sairitupa-Sanchez LZ, Morales-García SB and Morales-García M (2024) Adaptation and psychometric properties of a brief version of the general self-efficacy scale for use with artificial intelligence (GSE-6AI) among university students. Front. Educ. 9:1293437. doi: 10.3389/feduc.2024.1293437
Received: 13 September 2023; Accepted: 19 February 2024;
Published: 08 March 2024.
Edited by:
Knut Neumann, IPN–Leibniz-Institute for Science and Mathematics Eduction, GermanyReviewed by:
Mariel Fernanda Musso, CONICET Centro Interdisciplinario de Investigaciones en Psicología Matemática y Experimental, ArgentinaCopyright © 2024 Morales-García, Sairitupa-Sanchez, Morales-García and Morales-García. This is an open-access article distributed under the terms of the Creative Commons Attribution License (CC BY). The use, distribution or reproduction in other forums is permitted, provided the original author(s) and the copyright owner(s) are credited and that the original publication in this journal is cited, in accordance with accepted academic practice. No use, distribution or reproduction is permitted which does not comply with these terms.
*Correspondence: Wilter C. Morales-García, d2lsdGVybW9yYWxlc0B1cGV1LmVkdS5wZQ==
Disclaimer: All claims expressed in this article are solely those of the authors and do not necessarily represent those of their affiliated organizations, or those of the publisher, the editors and the reviewers. Any product that may be evaluated in this article or claim that may be made by its manufacturer is not guaranteed or endorsed by the publisher.
Research integrity at Frontiers
Learn more about the work of our research integrity team to safeguard the quality of each article we publish.