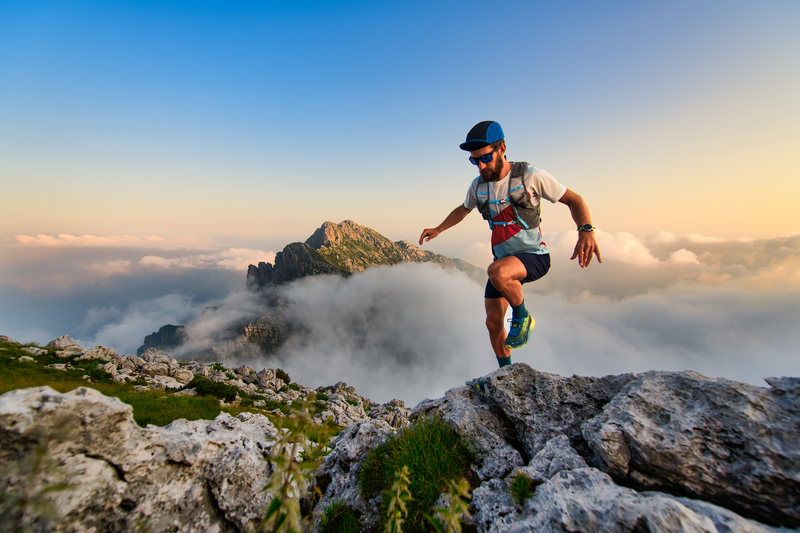
95% of researchers rate our articles as excellent or good
Learn more about the work of our research integrity team to safeguard the quality of each article we publish.
Find out more
ORIGINAL RESEARCH article
Front. Educ. , 19 November 2024
Sec. Digital Learning Innovations
Volume 9 - 2024 | https://doi.org/10.3389/feduc.2024.1288723
The widespread use of generative AI tools like ChatGPT has seen significant growth. This rise prompted discussions on integrating these technologies into school education. However, the practical implementation, testing, and assessment of generative AI in primary and secondary education remained largely unexplored. This article examines the application of ChatGPT-3.5 and 4 in primary school education. A study involving 110 students aged 8–14 across grades 4–6 in two Uruguayan schools was conducted. The focus was on using generative AI for dynamic personalization of educational content during classroom lessons. In these sessions, instructional content followed the curriculum goals, and text, illustrations, and exercises were generated and dynamically adjusted based on generative AI. The findings indicate that generative AI effectively tailors school materials to match varying pupil knowledge levels. Real-time adjustments during lessons cater to individual learning needs, enhancing cognitive ergonomics. This approach not only boosts pupil motivation but also improves their performance, facilitating more effective achievement of the curriculum’s learning objectives. These results suggest a promising avenue for leveraging generative AI to personalize and optimize primary school education.
Artificial intelligence (AI) and specifically generative pre-trained transformer (GPT) applications have garnered significant attention since 2023. GPT models, a subset of generative AI, excel in generating human-like text, spanning content creation, language translation, and conversational interactions. These models exhibit remarkable efficacy across various natural language processing tasks. Their strength lies in processing sequential data and accurately predicting the subsequent word in a sentence based on the input they receive (Kasneci et al., 2023).
The launch of ChatGPT-3.5, a specialized GPT developed by OpenAI in November 2022, marked a pivotal moment in the utilization of open-access AI technology. Subsequently, the media and the public showed a growing interest in generative AI and its associated tools. This surge was evident in the rapid increase of Google search queries related to terms such as “artificial intelligence” and “AI,” especially notable in regions across Europe, North America, and, notably, China. However, the conversation around AI and ChatGPT was conspicuously limited in developing nations, particularly in Africa, until it started to expand rapidly, but not in all African countries, in the autumn of 2023, according to Google Trends (2023). There was substantial media coverage on generative AI in many countries worldwide, but the widespread recognition and adoption of generative AI technologies happened in different places globally in 2023.
Several chat-based generative AI applications, such as Bard, ChatGPT, Claude, Copilot, Gemini, and Llama, and several image generation tools, such as DALL-E and Midjourney, have emerged. This fast technological development witnessed a significant rise in the adoption of these tools across all possible settings. In education, this spans from primary to secondary schools and further to high schools and universities. Education stands as a pivotal arena poised for transformation through the implementation of generative AI, as indicated by multiple sources (Abdelghani et al., 2023; Adiguzel et al., 2023; Baidoo-Anu and Owusu Ansah, 2023; Crawford et al., 2023; Dwivedi et al., 2023; Grassini, 2023; Jeon and Lee, 2023; Kasneci et al., 2023; Lambert and Stevens, 2023; Lozano and Blanco Fontao, 2023; Michel-Villarreal et al., 2023; Su and Yang, 2023). The evolving capabilities of GPT tools have sparked significant interest and intrigue among school administrators, educators, and pupils. In a concise case study, Jeon and Lee (2023) highlighted the multifaceted role of teachers concerning ChatGPT. Teachers are depicted as orchestrators of resources, making informed pedagogical decisions, supporters of pupils’ research endeavors, and awareness raisers of AI-related ethics (see Crawford et al., 2023). Teachers perceive ChatGPT as an interlocutor, content provider, teaching assistant, and evaluater.
Moreover, challenges and risks associated with generative AI in education have emerged. These encompass potential overreliance on the model leading to the propagation of unverified or inaccurate information; concerns regarding data privacy, security, and copyright; expenses linked with training and maintaining generative AI systems; digital divides; and the prevailing limited accessibility (Kasneci et al., 2023). Moreover, as of 2023, a noticeable dearth of comprehensive testing involving generative AI within school settings persists, with minimal published outcomes from primary or secondary school evaluations available for reference (Jauhiainen and Garagorry Guerra, 2023).
The article focuses on leveraging the potential of generative AI to aid and assess pupils’ learning processes during classroom lessons within the structured school environment. It explores how generative AI can be used to personalize learning materials, such as text, images, and exercises, to match the diverse knowledge levels among pupils. This customization seeks to align with each pupil’s abilities, enhancing motivation and learning efficacy to better achieve curriculum goals. Additionally, the study examines the feasibility of dynamically adapting these learning materials based on individual pupils’ real-time performance in class. Ultimately, the article illustrates how generative AI can effectively enhance pupils’ learning outcomes.
Following this introductory section, the article proceeds to its theoretical framework, which serves as the foundation of the study. This framework seamlessly integrates two distinct yet interlinked strands of motivational theories within the concept of cognitive ergonomics (Gaines and Monk, 2015). It adapts the flow theory (Csikszentmihalyi, 1990, 2014) and self-determination theory (Deci and Ryan, 1985; Pintrich, 1995; Ryan and Deci, 2000; Ryan and Vansteenkiste, 2023) to the educational context, specifically focusing on learning dynamics within school environments in the age of generative AI.
The empirical content presented comprises the outcomes derived from test lessons regarding the generative AI-processed learning material and learning processes in a school classroom context with 110 pupils spanning the 4th, 5th, and 6th grades. The lesson these pupils attended was curated using generative AI technology, specifically employing ChatGPT-3.5 and 4 and Midjourney to design the learning material, with subsequent evaluation capturing the pupils’ learning performance and perspectives. For this assessment, the Digileac framework was employed as the digital cloud-based designated tool (Digileac, 2023). The research was conducted quantitatively, employing descriptive statistical methods to illuminate key insights.
In the section regarding the results, we unveil the findings that provide comprehensive responses to our research questions. We elucidate the effective utilization of generative AI in segmenting pupils into distinct knowledge groups that align with their aptitudes and knowledge regarding the school classroom learning topics that were based on the curriculum. Furthermore, we comprehensively explore the challenges and potential pitfalls arising from the implementation of generative AI in school pupils’ learning. Finally, in conclusion, we emphasize the pivotal role generative AI has in the dynamic personalization of pupils’ learning material and tailoring it to the pupils’ existing and developing knowledge. This fosters heightened motivation among them, culminating in more effective learning outcomes.
The article builds upon and expands Csikszentmihalyi (1990, 2014) flow theory, adapting it to the context of education and learning within school environments amidst the emergence of generative AI. Concurrently, it aligns this renowned theory with the well-established self-determination theory in education. This approach prioritizes fostering students’ intrinsic motivation, in line with the insights of Pintrich (1995) as well as Deci and Ryan (1985), Ryan and Deci (2000), and Ryan and Vansteenkiste (2023). Within this article, these theories are contextualized through the prism of cognitive ergonomics (Gaines and Monk, 2015), particularly in the realm of learning amid the generative AI era. This framework interconnects pupils, the learning environment, and generative AI. Here, personalized learning materials serve as catalysts for enhancing pupils’ motivation, driving them toward heightened levels of learning achievement.
Pupils’ self-regulated learning has three main dimensions (Pintrich, 1995). Firstly, there is self-regulation of behavior, involving active oversight of pupils’ resources such as time and study environment and seeking help from peers and institution members. The second dimension is self-regulation of motivation and emotions, which includes adjusting pupils’ motivational beliefs and managing negative emotions along the curriculum learning topics. Positive motivation facilitates learning and achievement (Gottfried and Gottfried, 2004). The third dimension is self-regulation of cognition, utilizing cognitive strategies to enhance pupils’ performance (Pintrich, 1995). Self-regulation is influenced by the surrounding environment and can be developed over time (Pintrich, 1995). Teachers creating suitable classroom environments foster pupils’ autonomy and motivation for deep learning (León et al., 2015). Effective learning requires catering to pupils’ interests and abilities and building on existing knowledge (Hoekman et al., 1999).
Self-regulation is closely linked to intrinsic motivation, which is when pupils autonomously engage in activities for their interest and enjoyment (Ryan and Deci, 2000; Ryan and Vansteenkiste, 2023). It is self-determined and enhances engagement and effort (León et al., 2015). Intrinsic motivation involves tasks that are inherently captivating and aligned with pupils’ competence and self-direction (Deci and Ryan, 1985). Activities driven by intrinsic motivation frequently exhibit novelty, involve challenges that are appropriately balanced in terms of demand (Csikszentmihalyi, 2014), and stem from the pupil’s inherent desire for both competence and self-direction. Intrinsic motivation can be influenced by external factors such as assessment. Pupils anticipating assessments focus on memorization, while those learning without assessment expectations gain a deeper understanding (Grolnick and Ryan, 1987; Deci and Flaste, 1995).
In the 21st century, digitalization intensifies competition for children’s attention. Antonis Kocheilas, CEO of one of the world’s largest advertising agencies, Ogilvy Advertising, remarked, “In today’s world, we face media abundance because everything and everyone communicates. Simultaneously, there is attention scarcity because everything and everyone competes for a piece of people’s limited attention span” (Paulino, 2021).
Numerous studies explore how this competition affects children’s attention, influencing their food preferences, diet, and health (World Health Organization, 2022). Reward systems, such as the use of loot box systems offering randomized rewards in video games, are used to seize children’s attention and can contribute to the development of disordered gambling (Xiao, 2021). Brands often exploit pupils’ vulnerabilities, with over 70% of advertisements targeting school-aged children, particularly promoting unhealthy foods (Elliot et al., 2023). External rewards negatively impact pupils’ intrinsic motivation, diverging their focus toward video games, social media, etc., consequently limiting their dedicated learning time both at school and home. In addition, according to Joussemet et al. (2008), external incentives such as rewards and imposed control mechanisms such as school exams contribute to a negatively controlled educational environment. This environment impedes pupils’ wellbeing and fails to support their persistence in learning or enhance their school performance.
This divided attention significantly hampers pupils’ learning capabilities. To address this, the educational system must actively engage and sustain pupils’ attention to augment their cognitive skills. Csikszentmihalyi (1990, p. 33) asserts that attention is crucial “because attention determines what will or will not appear in consciousness, and because it is also required to make any other mental events – such as remembering, thinking, feeling, and making decisions – happen” … it is “like energy in that without it no work can be done, and in doing work, it is dissipated.” This attention allows for memory retrieval, situational assessment, and appropriate responses.
In addition, contemporary education faces a challenge as learning materials are designed for an average pupil, lacking adaptability. A Uruguayan study shed light on teachers’ struggles creating materials suitable for diverse student needs (Cura et al., 2022). Teachers often find themselves overwhelmed, juggling multiple roles, leading to excessive work and preparation at home. However, substantial and meaningful learning content within schools can elevate pupils’ intrinsic motivation (León et al., 2015). Generative AI uses can potentially assist pupils’ intrinsic motivation by aligning their skills with learning materials, empowering teachers to deliver targeted instruction to achieve curriculum objectives.
Misdirection of attention among pupils can lead to disengagement with the educational system. However, positive attention and engagement can be fostered through the flow theory. Flow is a state of energized focus where individuals intensely enjoy an activity that is neither easy nor challenging (Csikszentmihalyi, 1990). It nurtures intrinsic motivation, encouraging continued involvement.
Flow theory suggests that individual learners engaging in tasks matching their skills are more likely to experience flow states (Hoffman and Novak, 2009). Personalized learning activities, such as sports or hobbies, enhance learning performance and skill development (Csikszentmihalyi, 1990). The more one experiences flow, the more they actively seek it out (Nakamura and Cziksentmihalyi, 2009). Flow’s intrinsic rewards significantly bolster motivation across various domains, particularly education (Heutte et al., 2021). Therefore, education researchers strive to cultivate classroom environments rich in flow experiences (Gottfried and Gottfried, 2004).
Flow has nine key components: challenge-skill balance, action-awareness merging, clear goals, unambiguous feedback, current task concentration, sense of control, self-consciousness loss, time transformation, and autotelic experience (Csikszentmihalyi, 1990). The latter closely corresponds with the previously mentioned concepts of intrinsic motivation and self-regulation theory. Autotelic individuals engage in tasks for their intrinsic value, not solely driven by external goals. Steering pupils toward self-regulated experiences rooted in intrinsic motivation can effectively combat classroom disengagement.
Engaging in a flow experience during learning significantly enhances the learning process (Heutte et al., 2021). Shernoff et al. (2003) found that pupils exhibit higher engagement when they perceive a balance between their skills and task challenges, control over the learning environment, and relevance in instruction. Insufficient challenge leads to indifference, while excessive challenge leads to anxiety.
Generative AI in education introduces a novel form of cognitive ergonomics, enhancing the synergy between pupils, AI, and the education setting in schools (Figure 1). Cognitive ergonomics aims to improve human wellbeing and system performance by considering the interplay of human cognition, physical elements, and social factors (Zachary et al., 2001; Rodrigues et al., 2012). It centers on how perception, memory, reasoning, and motor response interact with diverse system components (Karwowski, 2012), analyzing their impact on interactions between humans and system elements.
Cognitive ergonomics is a cornerstone in designing human-system interactions, encompassing aspects such as mental workload, decision-making, human-computer interaction, human reliability, stress, and effective training (Zachary et al., 2001). It unravels the intricate interplay between task commitment and cognitive processes, showing how performance-centric tasks influence the mind and vice versa (Hollnagel, 2001).
A pupil’s performance relies on their comprehension of task elements such as objectives, methodologies, and system design, shaped by the designer’s perspective. Both performance reliability and cognitive progress are important (Hollnagel, 2001). Effective management of limited cognitive resources becomes pivotal, particularly in fostering the flow experience where pupils prefer challenging and engaging work. Within cognitive ergonomics, pupils express a personal interest in a specific topic or activity to trigger the flow experience. High-achieving pupils often encounter heightened flow states when engaging with their preferred subjects and determining the content (Borovay et al., 2019).
The introduction of technology in education often fails due to a lack of learning principles rooted in cognition and insufficient analysis of educational contexts (Zucchermaglio, 1993). In our evolving societal landscape, the significance of cognitive ergonomics grows, especially in the intersection between the educational environment, pupils, and generative AI technology (Kazemi and Smith, 2023).
Cognitive ergonomics extends to managing pupils’ limited cognitive resources for learning. Tailoring materials to their capabilities heightens their interest in the subject matter. Highly motivated students provided with clear learning materials are less likely to lose interest (Lazarides et al., 2018). Designing learning processes through cognitive ergonomics acknowledges pupils’ emotions and preferences, fostering optimal motivation by combining the derived from the enjoyment of learning material (intrinsic motivation) and its relevance (identified regulation) (Howard et al., 2021).
This article presents the findings stemming from a classroom lesson that scrutinized the integration of generative AI into primary school education. The lesson test was conducted as part of the Social Science curriculum for 4th, 5th, and 6th grade pupils, focusing on history. The tests were carried out in July of 2023 in Uruguay and involved four classes spanning two schools located in Montevideo, the capital city. These schools were chosen to represent diverse educational approaches: one was a public school predominantly catering to pupils from lower social strata, while the other was a private school mainly serving pupils from medium and higher social classes. As Spanish served as the language of tuition in these schools, it was also utilized during the test.
The primary dataset encompasses input gathered from a cohort of 110 pupils aged 8–14. Of them, 57.3% identified themselves as boys, 40.9% identified as girls, and 1.8% chose not to answer this question. These pupils actively engaged in the test lessons, which were part of their regular yearly curriculum in a classroom setting (Table 1).
The research questions investigated in this article, analyzing 110 pupils, were: How can ChatGPT be utilized to provide personalized learning materials for school pupils prior to and during lessons? How do pupils engage with the learning materials tailored by ChatGPT? How did the studied pupils articulate their learning experiences after using the materials customized by ChatGPT?
National and university guidelines on research integrity and ethics were strictly adhered to throughout the implementation of the test lesson, subsequent analysis, and reporting. Before commencing the test, permission was obtained from school directors, and the parents or guardians of the pupils had the option to prevent their child’s participation in the research. Additionally, pupils participated voluntarily and had the right to withdraw from the test at any moment or leave questions unanswered. Only results from pupils who completed the entire test lesson and responded to all questions are included in the analysis. Safety, data practices, and management were in accordance with the research code of conduct and discipline-specific principles.
Both teachers and pupils were thoroughly informed about the testing process, including research integrity and ethical guidelines. To ensure meticulous implementation of these procedures and prevent any potential harm or damage to research participants, one of the authors of this article personally supervised and guided the test procedures on-site. All test materials were automatically collected and recorded using the designated test platform and stored securely within a digital platform with a cloud-based digital folder. To maintain participant anonymity, respondents answered the test without providing their names, and their identities could not be determined from the results.
The integration of generative AI adaptation into the learning materials followed a structured process. Each pupil participated in a single test lesson focusing on social sciences, specifically history (see Appendix for detailed material formation). Each lesson was divided into two parts. Both consisted of personalized learning material (text and related questions) across three knowledge-level groups.
Within each test lesson and the knowledge level groups within these, there were two customized texts (adapted with ChatGPT-4), two images (previously designed using Midjourney), and a set of four open-ended questions and four multiple-choice questions related to the lesson’s text and illustrations, also adjusted with ChatGPT-4. On average, a single test lesson lasted around 15–20 min. It involved pupils familiarizing themselves with the initial test lesson text and illustrations, answering open-ended and multiple-choice questions, and then engaging with newly customized learning materials tailored to their proficiency levels along basic, medium, and advanced knowledge groups, and subsequently providing information about demography and subjective experiences related to the lesson.
To conduct the test lessons and gather empirical data, the Digileac platform was accessed via laptops within school premises (Digileac, 2023). Of the participants, 60 pupils utilized the national Ceibal program’s operating system, while an additional 50 pupils opted for the Chromebook platform. Both laptops and their respective operating systems were routinely integrated into the pupils’ learning experiences within their classrooms.
Collected data included various metrics. They included the time spent by each pupil (measured in seconds) reading the test lesson materials (text and figures) and answering multiple-choice and open-ended questions. The accuracy of their responses (whether correct or incorrect) to questions was individually measured for both the initial and modified test lesson materials. Additionally, pupils provided pre-test information about their subjective interest in the lesson topic and self-assessed knowledge. Post-test, they offered feedback on platform usability and self-perception of the acquired knowledge. Other gathered details encompassed demographic information, preferred subjects, and recent performance in the main study topic at school.
The analysis of the results followed a robust analytical approach. Descriptive statistics offered a comprehensive exploration of key data attributes, presenting a clear and concise summary of central tendencies and variations within the dataset. Concurrently, cross-tabulation techniques were employed to examine interdependencies, juxtaposing various demographic and contextual factors against pupils’ test performance. This method facilitated a nuanced understanding of how diverse aspects of pupils’ backgrounds potentially impacted their achievements, thereby enhancing the interpretation of findings regarding the utilization of generative AI in school education. However, the limited number of respondents prevented the application of advanced statistical testing.
The subsequent section details the empirical findings that respond to the research questions. Firstly, it illustrates the utilization of ChatGPT in providing customized and personalized learning materials tailored for pupils with varying levels of prior knowledge on the subject, accommodating differences in their learning pace during the lesson. Secondly, it indicates how pupils engaged with the learning materials customized by ChatGPT. Thirdly, it outlines pupils’ experiences regarding learning with materials adapted using ChatGPT.
The customization of learning materials occurred in two stages. Firstly, pupils were categorized into advanced, medium, and basic knowledge groups based on their understanding of the taught topic prior to and during the test lesson. Secondly, leveraging these three knowledge tiers, the learning materials were adapted using ChatGPT at the beginning of the test lesson and further refined during the session based on pupils’ performance. The objective was to ensure that pupils across different knowledge levels would attain the curriculum objectives by the lesson’s conclusion. This required consistency in the learning materials across all proficiency levels.
Three main methods were employed to categorize pupils according to their knowledge base. Initially, before the lesson commenced, the first method involved assessing each pupil’s prior school performance, specifically their achievement in social sciences on their school report certificates. Consequently, 18 pupils (18.2%) were categorized as having an excellent academic record, 79 pupils (71.8%) with a good or medium record, and 13 pupils (11.8%) with a satisfactory or poor record. While offering an “objective” measurement, this method pertained to broader subjects than the specific lesson at hand and depicted the situation before the lesson commenced.
The second method before the lesson relied on pupils’ self-perceived familiarity with the topic. Pupils categorized themselves as having advanced, intermediate, or basic knowledge. This subjective assessment identified 42 pupils (38.8%) as perceiving themselves to have advanced knowledge, 35 pupils (31.2%) with intermediate perceived knowledge, and 33 pupils (30.0%) possessing basic perceived knowledge. This subjective measurement was based on individual self-perception, which might have been influenced by limited prior knowledge, potentially leading to overestimation (Haselton et al., 2005). Pupils often lacked detailed knowledge about the lesson topic beforehand, making it challenging to accurately assess their knowledge base.
The analysis showed a linkage between pupils’ objective and subjective knowledge assessments. Pupils with excellent Social Sciences rates tended to perceive themselves as having greater knowledge about the lesson topic. For instance, among pupils with excellent social science rates, 38.9% believed they had extensive knowledge of the lesson topic, while only 5.6% felt they had no knowledge. Conversely, among pupils with poor or satisfactory social science rates, only 7.7% perceived to have extensive knowledge about the lesson topic, while 23.1% claimed to have no knowledge. This correlation was statistically significant, indicating a relationship between academic performance and perceived knowledge [t(DF = 16) = 00.00, p < 0.001].
The third categorization method was implemented during the test lesson. After reading the test lesson material, pupils responded to multiple-choice and open-ended questions. Based on the accuracy of their responses, pupils were categorized into advanced, medium, or basic knowledge groups regarding the lesson’s topic and to start with the second learning material. However, the first categorization had such an impact that pupils could remain in the same knowledge group or move up or down one knowledge group. After completing the multiple-choice and open-ended questions regarding the second part of the lesson, pupils were reassigned to knowledge groups based on their performance, enabling tailored adjustments in the learning material to match individual student needs.
Regarding personalizing the learning materials, ChatGPT played a pivotal role in tailoring the learning material content for each knowledge group. This included text content related to the lesson’s topic and crafting open-ended and multiple-choice questions aligned with the study material. In addition, Midjourney was utilized to create illustrations complementing the learning materials.
ChatGPT adjusted the learning materials using systematic and careful prompting across advanced, intermediate, and basic knowledge levels. Despite these adaptations, the length of learning materials remained consistent across these knowledge groups. However, notable differences emerged in the depth and complexity of the content. In the advanced knowledge group, the study materials displayed nuanced terminology, intricate sentence structures, and a more comprehensive exploration of the lesson’s topic. The intermediate knowledge group featured simplified terminology, fewer detailed examples, and a more straightforward expression of ideas. The material was presented in its simplest form for the basic knowledge group, focusing on fundamental concepts. These adjustments were implemented both before pupils began their learning and during the lesson after they responded to related questions.
As previously mentioned, pupils were initially divided into three knowledge categories—advanced, intermediate, and basic—based on their Social Sciences school grades. They began the lesson using accordingly tailored learning materials and completed open-ended and multiple-choice exercises, which were promptly assessed. Subsequently, each pupil was reassigned to a knowledge group aligned with their performance level, allowing them to continue with materials suited to their understanding. After the second segment of the lesson, they underwent another set of exercises and were immediately assessed as before, leading to a reevaluation of their knowledge groups based on their performance.
Our analysis found that using the official Social Science school grade as the initial criterion did not consistently match all pupils’ actual knowledge about the lesson topic. After completing the first part of the lesson, 9.1% of pupils were moved to a higher knowledge group, 73.4% remained in the same group, and 15.5% were moved to a lower group. After completing the second part of the lesson, 14.5% were moved up, 44.5% stayed in their group, and 41.0% were moved down. These shifts highlighted the necessity of offering more tailored learning materials—either simpler or more challenging. ChatGPT successfully customized materials to accommodate these varying learning needs.
Pupils exhibited diverse learning patterns during the class; some maintained their initial knowledge levels while progressing or found the topic too challenging.
At the end of the test lesson, after responding to the second learning materials, of pupils initially placed in the basic knowledge group, 68.8% remained in that group after the second test. In comparison, 31.2% were downgraded there from the intermediate group. Within the intermediate knowledge group, 76.5% stayed in that category across both lesson parts. Additionally, 11.7% were downgraded for not completing advanced-level exercises accurately, while 11.7% were upgraded for mastering tasks from the basic knowledge group. In the advanced knowledge group, 78.6% maintained their position due to consistent performance, and 21.4% were promoted from the intermediate level for superior performance. Overall, only slightly more than one out of four (28.1%) students remained in the same knowledge group from the beginning to the end of the test lesson.
The three most common learning trajectories of pupils observed during the test lesson were as follows: First, pupils initially placed in the intermediate knowledge group were downgraded to the basic knowledge group and remained there consistently (constituting 28.2% of pupils). Second, pupils who initially belonged to the intermediate knowledge group remained in that category throughout the tests (representing 19.1% of pupils). Finally, pupils initially categorized in the intermediate group were subsequently upgraded to the advanced knowledge group and maintained that level consistently (comprising 12.7% of pupils). These trajectories highlight the potential of generative AI tools such as ChatGPT in supporting a flow experience and intrinsic motivation in learning (Csikszentmihalyi, 1990).
Maintaining the same knowledge group indicated that the learning materials suited pupils’ learning, which is evident from the accuracy of their answers to the lesson material questions. These results suggest that after ChatGPT adjusted the learning materials, pupils’ performance aligned with their understanding of the topic and learning progression during the lesson. This adjustment rectified initial misplacements into either too high or low groups. Consequently, pupils were provided ChatGPT-tailored material matching their learning capacities while aligning with the curriculum goals. This suggests that it is feasible to utilize generative AI such as ChatGPT in a school educational context so that multiple adjustments in learning materials can be made within each lesson to attain optimally challenging material (Hoffman and Novak, 2009).
Before the test lesson began, each pupil assessed their knowledge regarding the lesson topic. Among those who claimed no prior knowledge, only a small fraction (4.5%) were placed in a higher knowledge group after both tests, while a majority (54.5 and 45.5%) were downgraded due to poor performance. A significant proportion of pupils who perceived fair knowledge showed alignment between their perceived and actual knowledge: 54.3% remained consistent in the first stage and 74.3% in the second stage of the lesson. Interestingly, many who were initially confident in their knowledge were later placed in lower knowledge groups (Table 2). This contrast highlights the diversity of knowledge within a classroom. It underscores the need to tailor learning material to each pupil’s cognitive abilities, irrespective of their self-perceived familiarity with the subject.
Table 2. Pupils’ (N = 110) perceived knowledge of test lesson topic and adjusting of pupils into knowledge groups (%).
The test lessons revealed varying levels of pupil engagement with the ChatGPT-modified learning materials, highlighting distinct patterns in their interaction. Self-regulated learning encompasses managing time and resources and sustaining motivation and engagement (Pintrich, 1995). Differences among pupils were observable in their levels of attention toward the learning materials, the duration taken to comprehend the text and illustrations, and the time allocated to answering both open-ended and multiple-choice questions.
Paying attention to learning materials is crucial for the learning process and improving the quality of the experience (Csikszentmihalyi, 1990, p. 33). Pupils’ attention to learning was measured by tracking the time spent studying the lesson material and addressing related questions throughout the lesson. Performances were categorized as fast, medium, or slow based on their durations. On average, for each task, roughly 15–20% of pupils displayed fast performance, while 60–70% exhibited medium performance, and the remaining 15–20% fell within the slow performance range. Certain attention patterns were observed among pupils. For instance, a proportionally larger share of fast readers tended to answer questions quickly compared to slow readers, and vice versa (see Table 3).
Pupils who are very interested in the lesson topic usually read the learning material faster than those who are less interested. This perhaps suggests the presence of autotelic pupils who engage with personalized study materials out of intrinsic interest rather than a mere obligation (see Csikszentmihalyi, 2014). The proportion of fast readers was substantially higher among those interested in the topic than those with fair, little, or no interest. Conversely, the share of slow readers was considerably smaller among pupils highly interested in the topic (Table 3).
A pupil’s self-perception of having good knowledge about the study topic significantly increased the likelihood of these pupils quickly acquainting themselves with the study materials. A statistically significant correlation was observed between reading the first learning material quickly, answering quickly, and having much or much knowledge about the topic [t(DF = 8) = 17.162, p = 0.028]. Conversely, a larger share of slow readers was observed among those who perceived having little knowledge. Nonetheless, almost two-thirds of pupils remained in the intermediate group (see Table 3).
Measuring the duration of learning does not straightforwardly indicate a pupil’s focused attention. A rapid pace might suggest disinterest, simply skimming through the material and quickly answering questions without much reflection. On the other hand, it could also indicate adeptness, with the pupil swiftly grasping the material and generating accurate responses, thus requiring less contemplation time.
The results reveal that ChatGPT facilitated the provision of dynamically tailored learning materials, consistently aligning with each pupil’s knowledge and learning progression regarding the lesson topic. Past research indicates that personalized learning materials amplify pupils’ motivation to engage with the content. This heightened interest and enjoyment foster intrinsic motivation, leading to higher-quality engagement with the learning materials (León et al., 2015; Ryan and Vansteenkiste, 2023). The support offered for pupils’ self-regulation, including clearer goals and dedicated learning time, coupled with heightened motivation and emotional resonance due to personalized materials, potentially bolstered each pupil’s self-efficacy. This enhancement in confidence regarding their learning capabilities and the deployment of diverse cognitive strategies can significantly impact overall performance (Pintrich, 1995). Such personalized learning experiences may contribute to pupils’ focused and energized engagement with the adapted material (Csikszentmihalyi, 1990; Hoffman and Novak, 2009).
Previous research among primary and secondary school pupils suggested optimistic perspectives on integrating ChatGPT into learning (Bitzenbauer, 2023; Lozano and Blanco Fontao, 2023), albeit without empirical evidence. This study, outlined in this article, assessed pupils’ subjective learning experiences while utilizing the provided learning materials and devices for the subject. Pupils’ inclination toward a specific learning method significantly impacts their motivation to engage with the topic.
Approximately two-thirds (66.4%) expressed substantial or high levels of enjoyment when using the provided learning materials and laptops. This suggests an enthusiastic and actively engaged learning process among the pupils during the test lesson.
Pupils who held a strong liking for the test lesson consistently exhibited particular patterns: they generally demonstrated high interest in the lesson topic [t(DF = 16) = 34.198, p = 0.005], found the test lesson learning material highly readable [t(DF = 16) = 39.127, p = 0.001], and recognized the test lesson’s illustrations as significantly beneficial for their learning [t(DF = 16) = 81.844, p < 0.001]. Conversely, a smaller fraction, one in 10 (10.0%), conveyed limited or negligible liking. These pupils, who expressed a lack of liking for the test, typically showcased contrasting tendencies: little to no interest in the covered test lesson topic [t(DF = 16) = 34.198, p = 0.005], negligible perceived learning during the lesson [t(DF = 16) = 61.426, p < 0.001], or placement in the intermediate knowledge group [t(DF = 8) = 16.727, p = 0.033].
Another key aspect to consider is the extent to which pupils learned the topic of the test lesson. A comprehensive, objective assessment was not feasible after this test lesson. However, as discussed earlier, pupils seemed to align more with their understanding of the topic and their learning progress throughout the lesson. This indicates an optimal challenge level in the learning materials tailored for each pupil, a crucial factor for enabling optimal learning experiences.
However, pupils provided subjective viewpoints on their learning about the topic. It is important to note that subjective perception might not precisely measure the actual amount learned, as it would be indicated in a standardized exam. Perceptions of having learned not at all or very much are also influenced by pupils’ prior knowledge about the topic.
A notable proportion of all pupils indicated having learned very much (23.6%) or much (33.6%) during the test lesson. This perception of having learned much or very much grew along the knowledge level groups: 42.9% in the basic, 54.2% in the intermediate, and 78.8% in the advanced knowledge group (Table 4). A small percentage reported learning not at all (4.5%) or only a little (9.1%) during the test lesson. Among pupils in the basic knowledge group, 19.0% indicated they had learned little or nothing, with this proportion decreasing in the intermediate knowledge group (14.3%) and further in the advanced knowledge group (6.0%) (Table 4).
Table 4. Pupils’ (N = 110) perceived learning during the test lesson and their knowledge level groups (%).
Overall, pupils’ perceptions of their learning during the test lesson varied. Differences were also noted between 4th, 5th and 6th grades. Among 4th and 5th graders, the average score leaned toward perceiving having learned much, while that score was lower among 6th graders (Table 5). The lower general score among 6th graders was impacted by a higher share of pupils who expressed that they had learned little or nothing during the test lesson, while that share was lower among 4th and 5th graders.
The impact of generative AI is anticipated to be substantial within school education, serving as a valuable tool for school administrators, teachers, and pupils alike. Several chat-based applications have emerged, such as Bard, ChatGPT, Claude, Copilot, Gemini, and Llama, along with image generation tools such as DALL-E and Midjourney. This surge in technology is projected to witness a significant rise in adoption across educational settings, from primary and secondary schools to higher education institutions such as universities.
This article examined the utilization of generative AI, specifically ChatGPT-3.5 and 4, for crafting customized learning materials catering to a wide spectrum of school pupils. The study centered on primary school pupils from grades 4 to 6, investigating test lessons focused on history topics conducted in July 2023. The subsequent sections address the three research questions, highlight the study’s limitations, and propose potential avenues for future research.
Firstly, in the conducted test lessons, the adaptability of generative AI, specifically ChatGPT-3.5 and 4, was effectively demonstrated. These AI models adeptly personalized learning materials both prior to and during the lessons, tailoring them to match individual pupils’ knowledge levels and align with the curriculum demands. Leveraging ChatGPT, pupils were categorized into distinct knowledge groups, enabling tailored adjustments in the learning materials to suit each pupil’s knowledge level.
Furthermore, ChatGPT demonstrated its capacity to provide personalized learning content to each pupil at the lesson’s outset and dynamically adapt this material throughout the session. This adaptive process accommodated both the curriculum requisites and the unique knowledge levels and performance of individual pupils. The dynamic adaptability is pivotal given the difficulty in accurately predicting pupils’ specific and general knowledge levels before the lesson and gauging their learning progress during the session.
This personalized adaptation maintained the lesson text length, requiring no adjustments in the quantity of illustrations or questions. This outcome suggests a pathway where learning material design can align with pupils’ learning performance rather than relying solely on exam results or self-perceived knowledge levels. Crafting engaging material with an optimal challenge level is crucial for motivating learning, and leveraging generative AI can enhance pupils’ autonomy in this process. This interconnectedness between pupils, generative AI, and educational goals is visualized in Figure 1, reflecting insights from various studies (Pintrich, 1995; Shernoff et al., 2003; Hoffman and Novak, 2009; León et al., 2015; Ryan and Vansteenkiste, 2023).
Secondly, using generative AI, particularly ChatGPT, to personalize learning materials effectively engaged pupils and captured their attention. It facilitated an environment with appropriately challenging learning tasks, promoting a focused approach toward curriculum goals while optimizing cognitive ergonomics. This personalized approach fostered a more engaging and motivating learning experience, aligning with the principles outlined in flow theory (Csikszentmihalyi, 1990). Nonetheless, there were variations in pupils’ attention to the tailored learning material by ChatGPT, as evidenced by the time spent studying across different knowledge level groups.
Thirdly, pupils shared their learning experiences with the customized materials generated by ChatGPT. A notable 66.4% expressed significant or high levels of enjoyment while using the provided learning materials and laptops. Within the studied group, a majority (57.2%) believed they had learned very much or much during the test lesson. Interestingly, this figure surged to 78.8% in the advanced knowledge group, which exhibited the highest performance and knowledge among the studied pupils. However, gauging objective learning measurements during this test lesson was unfeasible. The outcomes also highlight a discrepancy: pupils’ previous evaluation marks, self-perception of knowledge, or interest in the topic do not always align with their understanding of the school lesson topic. Dynamic adaptation of learning materials by ChatGPT could address this challenge, potentially enhancing the learning and accuracy of pupils’ learning and responses related to the school lesson topic.
Certainly, there are limitations to consider. The study involved a limited number (110) of pupils, each attending only one lesson modified with ChatGPT. Additionally, measuring pupils’ focused attention to learning materials might require more nuanced methods beyond simply tracking time usage. Given that generative AI is still in its early developmental stage, broader testing involving more pupils and diverse topics across various countries would be beneficial. Nonetheless, the case study conducted in 2023 illustrates that there already exists a major potential to utilize ChatGPT to adapt learning materials according to pupils’ knowledge levels. It suggests that when used thoughtfully, generative AI has the capacity to customize school learning materials, enhancing pupils’ learning performance and promoting educational efficiency, as supported by prior studies (Adiguzel et al., 2023; Kasneci et al., 2023; Lozano and Blanco Fontao, 2023).
Amid the global digitization of school settings, criticism has emerged regarding the expansion of digital tools, including mobile phones (Beland et al., 2023), and attempts have been launched to ban or restrict the use of ChatGPT in educational settings (Roose, 2023). However, these criticisms often stem from instances where these devices and technologies have been inadequately implemented. Generative AI, such as ChatGPT, is already part of our reality, making its complete ban in educational contexts unfeasible. Instead, the focus should be leveraging these tools to unlock their immense potential, fostering more engaging, inclusive, and effective learning experiences worldwide.
Taking a proactive and forward-looking approach is crucial in unlocking the potential of generative AI in education. Generative AI serves as a tool to tailor learning content and levels based on individual interactions within the school environment. Aligning curriculum content with each pupil’s distinct abilities and interests unlocks the potential for heightened motivation. This deeper engagement facilitates a more comprehensive exploration of school subjects, empowering pupils to better understand the educational materials provided.
As the use of generative AI in education is in its early stages, systematic studies are crucial to understanding its impact on designing school learning materials and, more broadly, its potential and pitfalls in education. Longitudinal tests and comparative analyses between pupils using generative AI-tailored learning materials and those who are not will shed light on the most effective ways to leverage this technology for optimal learning outcomes.
The datasets presented in this article are not readily available because anonymization and research integrity reasons. Requests to access the datasets should be directed to JJ, anVzYWphQHV0dS5maQ==.
The requirement of ethical approval was waived by the Ethics Committee for the Human Sciences at the University of Turku. The studies were conducted in accordance with the local legislation and institutional requirements. The Ethics Committee/Institutional Review Board also waived the requirement of written informed consent for participation from the participants or the participants’ legal guardians/next of kin.
JJ: Conceptualization, Data curation, Formal analysis, Funding acquisition, Investigation, Methodology, Project administration, Resources, Software, Supervision, Validation, Visualization, Writing – original draft, Writing – review & editing. AG: Conceptualization, Data curation, Formal analysis, Investigation, Methodology, Software, Visualization, Writing – original draft, Writing – review & editing.
The author(s) declare financial support was received for the research, authorship, and/or publication of this article. The research was partially funded by the University of Turku and by the Business Finland co-creation project Digileac.
The authors declare that the research was conducted in the absence of any commercial or financial relationships that could be construed as a potential conflict of interest.
All claims expressed in this article are solely those of the authors and do not necessarily represent those of their affiliated organizations, or those of the publisher, the editors and the reviewers. Any product that may be evaluated in this article, or claim that may be made by its manufacturer, is not guaranteed or endorsed by the publisher.
Abdelghani, R., Wang, Y., Yuan, X., Wang, T., Luca, P., Sauzéon, H., et al. (2023). GPT-3-driven pedagogical agents to train children’s curious question-asking skills. Int. J. Artific. Intell. Educ. 34, 483–518. doi: 10.1007/s40593-023-00340-7
Adiguzel, T., Kaya, M., and Cansu, F. (2023). Revolutionizing education with AI: exploring the transformative potential of ChatGPT. Contemp. Educ. Technol. 15:429. doi: 10.30935/cedtech/13152
Baidoo-Anu, D., and Owusu Ansah, L. (2023). Education in the era of generative artificial intelligence (AI): understanding the potential benefits of ChatGPT in promoting teaching and learning. J. AI 7, 52–62. doi: 10.61969/jai.1337500
Beland, L., Glass, A., Krutka, D., and Rose, S. (2023). Do smartphones belong in classrooms? Four scholars weigh in. The Conversation. Available at: https://theconversation.com/do-smartphones-belong-in-classrooms-four-scholars-weigh-in-210099 (Accessed July 26, 2023).
Bitzenbauer, P. (2023). ChatGPT in physics education: a pilot study on easy-to-implement activities. Contemp. Educ. Technol. 15:430. doi: 10.30935/cedtech/13176
Borovay, L., Shore, B., Caccese, C., Yang, E., and Hua, O. (2019). Flow, achievement level, and inquiry-based learning. J. Adv. Acad. 30, 74–106. doi: 10.1177/1932202X18809659
Crawford, J., Cowling, M., and Allen, K. (2023). Leadership is needed for ethical ChatGPT: character, assessment, and learning using artificial intelligence (AI). J. Univ. Teach. Learn. Prac. 20:2. doi: 10.53761/1.20.3.02
Csikszentmihalyi, M. (1990). Flow: The psychology of optimal experience. New York, Ny: Harper & Row.
Csikszentmihalyi, M. (2014). Flow and the foundations of positive psychology: The collected works of Mihaly Csikszentmihalyi. Dordrecht: Springer.
Cura, D., Scasso, M., Ribeiro Jorge, N., and Capano, G. (2022). Challenges and opportunities for equality in education. Main barriers to accessing and using Ceibal tools for children and adolescents in Uruguay. Ceibal & UNICEF Uruguay: Montevideo. Available at: https://www.unicef.org/uruguay/media/6976/file/Challenges%20and%20opportunities%20for%20equity%20in%20education.pdf (Accessed September 7, 2023).
Deci, E., and Flaste, R. (1995). Why we do what we do: The dynamics of personal autonomy. New York, NY: G.P. Putnam’s Sons.
Deci, E., and Ryan, R. (1985). Intrinsic motivation and self-determination in human behaviour. New York: Plenum.
Digileac (2023). Digital learning environments for all contexts. Available at: https://sites.utu.fi/digileac (Accessed December 15, 2023).
Dwivedi, Y., Kshetri, N., Hughes, L., Slade, E. L., Jeyaraj, A., and Kar, A. K. (2023). Opinion paper: “so what if ChatGPT wrote it?” multidisciplinary perspectives on opportunities, challenges and implications of generative conversational AI for research, practice and policy. Int. J. Inf. Manag. 71:102642. doi: 10.1016/j.ijinfomgt.2023.102642
Elliot, C., Truman, E., and Black, J. (2023). Tracking teen food marketing: participatory research to examine persuasive power and platforms of exposure. Appetite 186:106550. doi: 10.1016/j.appet.2023.106550
Gaines, B., and Monk, A. (2015). Cognitive ergonomics: Understanding, learning, and designing human-computer interaction. London: Academic Press.
Google Trends (2023). Search engine results for trending with “AI”, “artificial intelligence”, ChatGPT, bard, Google AI. Available at: https://trends.google.com/trends/explore?q=Artificial%20intelligence,chatgpt,AI,Bard,Google%20AI&hl=en-US (Accessed December 15, 2023).
Gottfried, A. E., and Gottfried, A. W. (2004). Toward the development of a conceptualization of gifted motivation. Gift. Child Q. 48, 121–132. doi: 10.1177/001698620404800205
Grassini, S. (2023). Shaping the future of education: exploring the potential and consequences of AI and ChatGPT in educational settings. Educ. Sci. 13:692. doi: 10.3390/educsci13070692
Grolnick, W., and Ryan, R. (1987). Autonomy in children's learning: an experimental and individual difference investigation. J. Pers. Soc. Psychol. 52, 890–898. doi: 10.1037/0022-3514.52.5.890
Haselton, M., Nettle, D., and Andrews, P. (2005). “The evolution of cognitive bias” in The handbook of evolutionary psychology. ed. D. Buss (Hoboken, NJ: John Wiley & Sons), 724–746.
Heutte, J., Fenouillet, F., Martin-Krumm, C., Gute, G., Raes, A., Gute, R., et al. (2021). Optimal experience in adult learning: conception and validation of the flow in education scale (EduFlow-2). Front. Psychol. 12:828027. doi: 10.3389/fpsyg.2021.828027
Hoekman, K., McCormick, J., and Gross, M. (1999). The optimal context for gifted students: a preliminary exploration of motivational and affective considerations. Gift. Child Q. 43, 170–193. doi: 10.1177/001698629904300304
Hoffman, D., and Novak, T. (2009). Flow online: lessons learned and future prospects. J. Interact. Mark. 23, 23–34. doi: 10.1016/j.intmar.2008.10.003
Hollnagel, E. (2001). Extended cognition and the future of ergonomics. Theor. Issues Ergon. Sci. 2, 309–315. doi: 10.1080/14639220110104934
Howard, J., Bureau, J., and Guay, F. (2021). Student motivation and associated outcomes: a meta-analysis from self-determination theory. Perspect. Psychol. Sci. 16, 1300–1323. doi: 10.1177/1745691620966789
Jauhiainen, J., and Garagorry Guerra, A. (2023). Generative AI and ChatGPT in school children’s education. Evidence from a school lesson. Sustainability 15:14025. doi: 10.3390/su151814025
Jeon, J., and Lee, S. (2023). Large language models in education: a focus on the complementary relationship between human teachers and ChatGPT. Educ. Inf. Technol. 28, 15873–15892. doi: 10.1007/s10639-023-11834-1
Joussemet, M., Landry, R., and Koestner, R. (2008). A self-determination theory perspective on parenting. Can. Psychol. 49, 194–200. doi: 10.1037/a0012754
Karwowski, W. (2012). A review of human factors challenges of complex adaptive systems: discovering and understanding chaos in human performance. Hum. Factors 54, 983–995. doi: 10.1177/0018720812467459
Kasneci, E., Sessler, K., Küchemann, S., Bannert, M., Dementieva, D., Fischer, F., et al. (2023). ChatGPT for good? On opportunities and challenges of large language models for education. Learn. Indiv. Differ. 103:102274. doi: 10.1016/j.lindif.2023.102274
Kazemi, R., and Smith, A. (2023). Overcoming COVID-19 pandemic: emerging challenges of human factors and the role of cognitive ergonomics. Theor. Issues Ergon. Sci. 24, 401–412. doi: 10.1080/1463922X.2022.2090027
Lambert, J., and Stevens, M. (2023). ChatGPT and generative AI technology: a mixed bag of concerns and new opportunities. Comput. Sch. 6, 1–25. doi: 10.1080/07380569.2023.2256710
Lazarides, R., Dietrich, J., and Taskinen, P. (2018). Stability and change in students’ motivational profiles in mathematics classrooms: the role of perceived teaching. Teach. Teach. Educ. 79, 164–175. doi: 10.1016/j.tate.2018.12.016
León, J., Nunez, J., and Liew, J. (2015). Self-determination and STEM education: effects of autonomy, motivation, and self-regulated learning on high school math achievement. Learn. Indiv. Differ. 43, 156–163. doi: 10.1016/j.lindif.2015.08.017
Lozano, A., and Blanco Fontao, C. (2023). Is the education system prepared for the irruption of artificial intelligence? A study on the perceptions of students of primary education degree from a dual perspective: current pupils and future teachers. Educ. Sci. 13:733. doi: 10.3390/educsci13070733
Michel-Villarreal, R., Vilalta-Perdomo, E., Salinas-Navarro, D., Thierry-Aguilera, R., and Gerardou, F. (2023). ChatGPT explains the challenges and opportunities of generative AI for higher education. Educ. Sci. 13:856. doi: 10.3390/educsci13090856
Nakamura, J., and Cziksentmihalyi, M. (2009). “Flow theory and research” in The Oxford handbook of positive psychology. eds. S. Lopez and C. Snyder. 2nd ed (Oxford: Oxford University Press), 195–206.
Paulino, B. (2021) Winning attention: a Q & a with Antonis Kocheilas, CEO of Ogilvy Advertising. Ogilvy. Available at: https://www.ogilvy.com/ideas/winning-attention-qa-antonis-kocheilas-ceo-ogilvy-advertising (Accessed September 7, 2023).
Pintrich, P. (1995). Understanding self-regulated learning. New Dir. Teach. Learn. 63, 3–12. doi: 10.1002/tl.37219956304
Rodrigues, M., Castello Branco, I., Shimioshi, J., Rodrigues, E., Monteiro, S., and Quirino, M. (2012). Cognitive-ergonomics and instructional aspects of e-learning courses. Work 41, 5684–5685. doi: 10.3233/WOR-2012-0919-5684
Roose, K. (2023). Don’t ban ChatGPT in schools. Teach with it. New York Times. Available at: https://www.nytimes.com/2023/01/12/technology/chatgpt-schools-teachers.html (accessed January 12, 2023).
Ryan, R., and Deci, E. (2000). Self-determination theory and the facilitation of intrinsic motivation, social development, and well-being. Am. Psychol. 55, 68–78. doi: 10.1037/0003-066X.55.1.68
Ryan, R., and Vansteenkiste, M. (2023). “Self-determination theory: metatheory, methods, and meaning” in The Oxford handbook of self-determination theory. ed. R. Ryan (Oxford: Oxford University Press), 3–32.
Shernoff, D., Csikszentmihalyi, M., Shneider, B., and Shernoff, E. (2003). Student engagement in high school classrooms from the perspective of flow theory. Sch. Psychol. Q. 18, 158–176. doi: 10.1521/scpq.18.2.158.21860
Su, J., and Yang, W. (2023). Unlocking the power of ChatGPT: a framework for applying generative AI in education. ECNU Rev. Educ. 6, 355–366. doi: 10.1177/20965311231168423
World Health Organization (2022). Protecting children from the harmful impact of food marketing: policy brief. Geneva: World Health Organization.
Xiao, L. (2021). Regulating loot boxes as gambling? Towards a combined legal and self-regulatory consumer protection approach. Int. Entertain. Law. Rev. 2, 27–47. doi: 10.4337/ielr.2021.01.02
Zachary, W., Campbell, G., Laughery, K., Glenn, F., and Cannon-Bowers, J. (2001). The application of human modeling technology to the design, evaluation, and operation of complex systems. Adv. Hum. Perform. Cogn. Eng. Res. 1, 201–250. doi: 10.1016/S1479-3601(01)01007-4
Zendle, D., Meyer, R., Cairns, P., Waters, S., and Ballou, N. (2020). The prevalence of loot boxes in mobile and desktop games. Addiction 115, 1768–1772. doi: 10.1111/add.14973
Zucchermaglio, C. (1993). “Toward cognitive ergonomics of educational theory” in Designing environments for constructive learning. eds. T. Duffy, J. Lowyck, and D. Jonassen (Heidelberg: Springer), 249–260.
The authors of this article initially selected reading materials for the test lessons: one for the 4th grade, another for the 5th grade, and a third for the 6th grade. The 5th-grade text was sourced from an article penned by Emma Groeneveld found in the World History Encyclopedia, later translated into Spanish by Waldo Reboredo Arroyo. Meanwhile, the texts for the 4th and 6th grades were drawn from an article authored by Teressa Kiss, covering the topics of “Los Indígenas” and “Imperio Romano.” To ensure content accuracy, the authors validated the correctness of these test lesson texts.
After the test lesson material was chosen, the authors engaged ChatGPT-3.5 and ChatGPT-4 to adapt the text, ensuring its suitability for pupils with varying levels of knowledge about the lesson topic—ranging from advanced to intermediate or basic. Additionally, two images pertinent to the test lesson were created using Midjourney, a tool generating images from natural language descriptions. Subsequently, a set of four open-ended questions and four multiple-choice questions related to the test lesson text and images were tailored by ChatGPT-4 for three distinct knowledge level groups, aiming to assess each pupil’s learning of the material. To verify accuracy, the authors rigorously validated the correctness of these test lesson texts, questions, and illustrations.
In the process of developing the learning material, we adjusted a particular parameter, which governs the randomness of predictions in the text generation. This adjustment was critical in curbing unforeseen or implausible text content—referred to as “hallucinations” in the generative AI vocabulary.
In the context of AI text generation and using AI models, we utilized zero-shot and few-shot learning scenarios to allow ChatGPT to perform tasks or generate text, as it had not been extensively trained on specific topics or tasks. In zero-shot learning, the AI model was trained to perform tasks without any specific examples or data related to that particular task. In the few-shot learning, the AI model was trained with a few examples from which it generated the content.
In this natural language processing, AI models were trained to identify or prioritize particular elements (called antibodies in the AI vocabulary) within a dataset or during a task. These included text, sentiment, names, and topics related to the prepared lesson. As a result, the AI model understood and emphasized the required aspects and features when generating or processing data.
Keywords: generative AI, ChatGPT, Midjourney, education, school, pupil, learning, flow
Citation: Jauhiainen JS and Garagorry Guerra A (2024) Generative AI and education: dynamic personalization of pupils’ school learning material with ChatGPT. Front. Educ. 9:1288723. doi: 10.3389/feduc.2024.1288723
Received: 04 September 2023; Accepted: 30 October 2024;
Published: 19 November 2024.
Edited by:
Charoula Angeli, University of Cyprus, CyprusReviewed by:
Elaine Khoo, Massey University, New ZealandCopyright © 2024 Jauhiainen and Garagorry Guerra. This is an open-access article distributed under the terms of the Creative Commons Attribution License (CC BY). The use, distribution or reproduction in other forums is permitted, provided the original author(s) and the copyright owner(s) are credited and that the original publication in this journal is cited, in accordance with accepted academic practice. No use, distribution or reproduction is permitted which does not comply with these terms.
*Correspondence: Jussi S. Jauhiainen, anVzYWphQHV0dS5maQ==
Disclaimer: All claims expressed in this article are solely those of the authors and do not necessarily represent those of their affiliated organizations, or those of the publisher, the editors and the reviewers. Any product that may be evaluated in this article or claim that may be made by its manufacturer is not guaranteed or endorsed by the publisher.
Research integrity at Frontiers
Learn more about the work of our research integrity team to safeguard the quality of each article we publish.