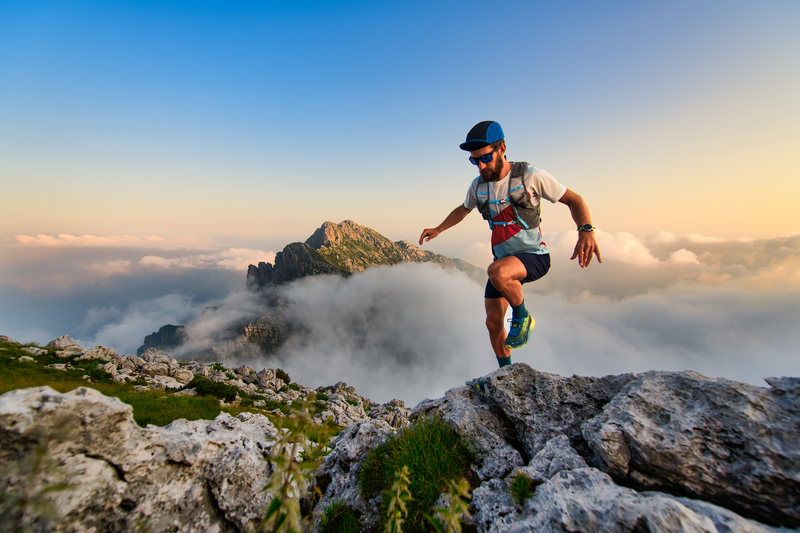
94% of researchers rate our articles as excellent or good
Learn more about the work of our research integrity team to safeguard the quality of each article we publish.
Find out more
ORIGINAL RESEARCH article
Front. Educ. , 31 January 2024
Sec. Educational Psychology
Volume 9 - 2024 | https://doi.org/10.3389/feduc.2024.1286132
Introduction: The present longitudinal study tested the hypotheses that (i) learners become faster readers after intensive English language instruction, and that (ii) learners who read more English texts tend to make larger gains in reading speed.
Methods: Study participants were 142 L1 Cantonese or Mandarin English learners enrolled in an eight-month university bridging program. Participants completed a reading habits log each week, reporting information about their reading activity, including the type of texts they read, the amount of time they spent reading each text, and the number of pages they read.
Results: It was found that English language learners spent less time reading per page of text by program end, as shown by a significant linear weekly increase in reading speed. Critically, there was also a significant effect of reading experience: learners who read more pages of text than their peers during the bridging program tended to make the largest net gains in reading speed.
Discussion: The results support the idea that reading experience is a factor that contributes to reading speed development in English language learners.
International university students who speak English as an additional language (EAL) account for a large proportion of students in English-speaking Western universities (Juffs, 2020). For instance, in Canada, international students made up 25% of all enrolments in higher education institutions in the 2021–2022 academic year, with students from China making up an 18% share of these enrolments (Project Atlas, 2023). Studies that compare the academic outcomes of EAL students with those of students who use English as a first language (L1) report that EAL students underperform academically compared to their L1 English peers in English-medium institutions (e.g., Thorpe et al., 2017). There are at least three sources of evidence that suggest reading skill is a critical factor in explaining this discrepancy. First, there are large group differences between international students’ reading and reading-related skills and those of domestic students (Trenkic and Warmington, 2019). Second, EALs with stronger English reading and reading-related abilities tend to achieve higher grades (Oliver et al., 2012; Daller and Phelan, 2013; Masrai and Milton, 2017). Third, reading (and language skills in general) are predictive only of international students’ academic outcomes but not those of L1 English (domestic) students (Trenkic and Warmington, 2019). It is therefore crucial to understand the factors that contribute to second language (L2) reading ability in EAL students to gain insight into how to support their reading development.
The present study addresses an under-researched aspect of EAL reading: namely, the impact of reading experience on the development of EAL reading speed. We collected week-by-week longitudinal data on the reading habits of Chinese-speaking EAL students enrolled in a university bridging program. The reading habits data were used to examine (i) the impact of 26 weeks of language instruction (13 weeks in the classroom per semester) on developmental change in reading speed, and (ii) whether individual differences in reading experience translate into individual variability in reading speed gains. We addressed the hypothesis that more exposure to written materials, i.e., more reading experience, is linked to a faster rate of improvement in reading speed over time.
Our focus on reading experience is motivated by research that overwhelmingly demonstrates that exposure to printed materials critically shapes the development of reading outcomes and various component skills of reading (Stanovich, 1986; Stanovich and West, 1989; Share, 1995; Stanovich et al., 1995; Acheson et al., 2008; Mol and Bus, 2011; Schiefele et al., 2012; Moore and Gordon, 2015; Locher and Pfost, 2020). For example, reading experience has been linked to vocabulary knowledge growth (Nagy et al., 1987; Beech, 2002; Sternberg, 2014; Duff et al., 2015), lexical processing speed gains (i.e., change in word reading speed; Martin-Chang and Gould, 2008; Moore and Gordon, 2015), and stronger reading comprehension in an L1 (e.g., Cain and Oakhill, 2014) and an L2 (e.g., Hu and Nation, 2000; Grabe, 2017; Dong et al., 2020). These patterns are consistent with Mol and Bus’ (2011) meta-analyses which concluded that developing readers who are exposed to greater quantities of printed materials also tend to be more skilled readers and are also likely to perform better academically.
An explanation for the relationship between print exposure and reading speed is that experience with texts supports the consolidation and automatization of word identification ability (Stanovich, 1986; Ehri, 1995; Share, 1995). It is argued that developing readers must first learn to map orthographic codes to phonological codes, i.e., decoding. Share (1995) argues that, once the basic principles of word decoding are in place, a “self-teaching” mechanism facilitates the acquisition of an autonomous orthographic lexicon. Thus, under this hypothesis, the successful learning of word-specific print-to-meaning connections is driven by repeated exposures to novel orthographic forms. As print-to-sound translation becomes more efficient via the application of grapheme-phoneme correspondences to novel words, this in turn leads to a broader and more autonomous orthographic lexicon, and greater word processing fluency (Jorm and Share, 1983; Wolf and Katzir-Cohen, 2001; Share, 2004). Of relevance to the L2 population in the current study, learning the grapheme-to-phoneme correspondence of English represents a unique challenge for Chinese learners of English whose L1 is Mandarin or Cantonese. Chinese is a morpho-syllabic writing system in which characters represent morphemes, whereas English is an alphabetic orthography which uses graphemes to represent phonemes. Thus, the reading strategies used by Chinese EALs when reading in their native language (Cantonese or Mandarin) may not be so easily applied when reading in English.
The important role of exposure to printed language is also emphasized in the Lexical Quality Hypothesis (LQH; Perfetti, 1985, 2007; Perfetti and Hart, 2002). According to Perfetti, high quality lexical representations are crucial for efficient reading comprehension. Under the LQH, high quality lexical representations consist of “highly specified” and “partly redundant” representations of form (orthography and phonology) and “flexible” representations of meaning (Perfetti, 2007, p. 357). The key prediction of the LQH is that developing high quality lexical representations is a gradual process that relies upon repeated exposures to the printed forms of words. With repeated exposure to words, the lexical representations associated with those words become more entrenched in the mental lexicon, requiring less cognitive effort to retrieve from memory during reading.
Evidence in support of the role of exposure in shaping L2 reading speed stems from (i) cross-sectional reading studies demonstrating that larger amounts of prior L2 reading experience predict faster word processing reading speed (e.g., Whitford and Titone, 2012, 2015; Kaushanskaya et al., 2020), and (ii) longitudinal studies in which word processing and reading rates (measured in words per minute) become faster after a period of language instruction (Schmidtke and Moro, 2021), or after an extensive reading intervention (e.g., Beglar and Hunt, 2014; Huffman, 2014; Sakurai, 2015; McLean and Rouault, 2017).
Further support for the importance of lexical quality in shaping L2 reading development in English comes from studies that assess the role of individual differences in cognitive linguistic skills, such as phonological awareness and orthographic skills, on reading comprehension (e.g., Raudszus et al., 2018; O’Connor et al., 2019; Schmidtke and Moro, 2021; Xue, 2021). For instance, phonological awareness and vocabulary knowledge were found to be critical determinants of reading speed development among Chinese EALs (Schmidtke and Moro, 2021). In sum, it is expected that greater experience with printed language leads to more efficient decoding (Share, 1995) and stronger lexical representations (Perfetti, 2007), which in turn leads to the faster processing of texts.
Prior research has examined the effect of L2 reading speed as a function of either (i) inter-individual differences in L2 language exposure (e.g., estimated via an L2 language experience survey: Whitford and Titone, 2012), or (ii) intra-individual change in reading experience via language exposure (e.g., in a pre- and post-test design: Sakurai, 2015; McLean and Rouault, 2017; Schmidtke et al., 2023). The novel aspect of the present study is that we quantify the effect of both sources of variability as predictors of change in reading speed. First, we examine intra-individual change in L2 reading speed during the 26 weeks of English language instruction. Based on prior research (e.g., Beglar and Hunt, 2014; Schmidtke and Moro, 2021; Schmidtke et al., 2023), we expect text processing speed to become faster within this duration. Second, we gauge the impact of inter-individual differences in exposure to printed materials on change in L2 text reading speed during the bridging program. Since the study period is tied to the fixed duration of the bridging program (8 months), it naturally affords the ability to study the impact of individual differences in print exposure within a controlled window of time. Addressing this research question also has practical importance: it is not known whether students who consume a greater volume of English reading material also tend to make additional boosts in reading speed during bridging programs. If additional print exposure does lead to a greater developmental advantage, the bridging program developers may wish to identify and provide support to those students who cover fewer reading materials than their peers.
We conducted a reading habits study in which EAL students logged their weekly reading habits over the course of the program (26 weeks in the classroom). Each week, students were asked to report the number of pages they read, the amount of time spent reading the text, and the type of text they read. We used this data to compute a measure of reading speed for each reading activity, defined as the number of minutes spent reading a text divided by the number of pages of the text they read (minutes per page). To control for general L2 ability, we assessed written receptive vocabulary size using the Vocabulary Size Test (VST; Coxhead et al., 2014, 2015) at the outset of the study as a proxy of prior experience with English printed materials (Stanovich and Cunningham, 1992).
Our first research question asked whether EAL reading speed is increases by the end of English language instruction. We tested this hypothesis in a growth model that assessed whether week of instruction affected reading speed. We expected students to become progressively faster at processing text over time, i.e., that we would see a gradual reduction in minutes per page as the instructional program progressed. Based on prior work (Stanovich, 1986; Stanovich and West, 1989; Acheson et al., 2008; Mol and Bus, 2011; Whitford and Titone, 2012; Moore and Gordon, 2015), greater coverage of written text, i.e., more reading experience, is expected to confer an additional advantage to individual gains in reading speed. Therefore, our second research question asked whether individual variability in total reading coverage translates into individual differences in reading speed gains. We tested this hypothesis by assessing the relationship between rate of change in reading speed and total amount of experience with written materials during the program. We hypothesized that additional reading experience is associated with reading speed change in such a way that students who accumulate more experience with written materials over time tend to make greater gains in reading speed.
Data was collected from a total of 142 (60 Female, 76 Male, 6 undisclosed) EAL students enrolled in an 8-month university bridging program at McMaster University. This English language bridging program accepts students that meet the academic requirement for undergraduate studies but do not meet the English language proficiency requirement. To study at the undergraduate level, students must have obtained an overall score of 6.5 on the International English Language Testing System (IELTS), with a minimum of 6.0 in each of the four language components (Reading, Writing, Speaking and Listening). To qualify for the bridging program, students must have obtained a minimum overall IELTS score of 5.0. The median overall incoming IELTS score was 5.75. Study participants were all native speakers of Mandarin or Cantonese. The average age at the end of testing was 19.64 years (SD = 0.92; min = 17.72; max = 24.55). None of the participants had a diagnosed reading or learning disability. Participation in the experiment was voluntary. The reading habits log was conducted as part of a reading assessment in the bridging program and participants received course credit for completion. This study was approved by the McMaster Research Ethics Board. All participants gave their informed consent prior to their inclusion in the study.
Participants completed a reading habits log twice a week for a total of 26 weeks. During the first academic term participants completed logs by pencil and paper in physical reading log booklets. Participants were instructed to complete the reading habits log upon completion of their English reading activities each week. Booklets were assessed each week for completion by course instructors. At the end of the first term, data contained within the booklets were then digitized. During the second academic term participants completed the reading habits log in an online platform. The total duration of each term was 14 weeks, but we did not collect data during the reading week of each term.1 Thus, we examined reading habits over 26 weeks (13 weeks each term) of classroom instruction.
Participants were asked to complete two log entries per week, where each entry was specific to a single reading activity, i.e., a passage of a book or a homework reading. The log included a series of questions that required free-text responses which required students to report aspects of their reading habits. The reading log questions that are pertinent to our research goals required students to indicate the brief title of the reading, the number of pages they read, and the length of time they read for (in minutes or hours). A page of text could be a single sheet of paper or a digital screen displaying written or printed content. There was also a multiple-choice question that required participants to indicate the type of reading. This question provided seven options following the phrase This week I read:. The options included the following text types: Reading for the Reading course, Reading for other courses, An academic article (non-course readings), A newspaper/magazine article, An article from a website, A short story or novel, or A chapter from a novel or non-fiction book.
Reading speed was defined as the number of pages per reading activity divided by number of minutes per reading activity (see above). Larger values indicate longer reading times. This measure provides an estimate of reading speed per each reading activity. We refer to this measure as minutes per page (mpp), rather than reading rate, to avoid confusion with other studies in the literature that use reading rate to refer to words per minute.
Total number of pages read was defined as the number of pages participants read during the bridging program. This measure was computed by summing the number of pages read across all reading log entries per participant.
Due to overlap between the text types that were originally collected, some text types were collapsed into broader categories. Reading for the Reading course and Reading for other courses were collapsed into the category of Course reading. A short story or novel and A chapter from a novel or non-fiction book were combined into the Fiction text category. Only 6% (76 titles) of the titles in the A chapter from a novel or non-fiction book category were non-fiction titles. We removed these non-fiction entries. After these changes in text type categories, there were five text types in total: Academic article, Newspaper/magazine article, Website article, Course reading, and Fiction text.
Incoming vocabulary size was assessed using the Vocabulary Size Test (VST; Coxhead et al., 2014, 2015). The VST measures an individual’s written receptive vocabulary size in English. The test is composed of 100 items that are presented in order of word frequency (most to least frequent). For each item, participants are provided a word in a sentence followed by four descriptions. Participants are required to choose the description that correctly describes or defines the word. Although the word is provided within a sentence, it is provided in a non-defining context. One point is received for each correctly answered item. Vocabulary size, measured as number of word families, is estimated by multiplying 200 by the number of correctly identified items (out of a maximum of 100 items). The greater the word family size, the greater the written receptive vocabulary size. Version A of the test was administered in a classroom setting to all students upon entry to the bridging program. As recommended in the testing guidelines, students were given 45 min to complete the test. Cronbach’s alpha was 0.75.
The initial data set contained 6,649 data points, where each data point represents the response to a single entry in the reading log. The dataset included responses from 142 consenting participants who completed at least 10 reading logs in total. The median total number of weeks a participant provided a response in the reading log was 21. We removed extreme outlying data points by excluding submissions within the top 1% of the distribution of number of pages read per log (i.e., any log entries for which a student read more than 58 pages in a single reading session) and the top 1% of the distribution of minutes spent reading per session (i.e., any entries for which a student read for longer than 180 min). We also removed data points within the top and bottom 1% of the distribution of minutes per page (i.e., any entries for which a student spent less than 40 s or more than 40 min reading per page). These clean-up steps led to a total loss of 232 data points (including all data collected from 1 participant). The final data set contained 6,417 valid data points from 142 participants.
We used a linear mixed-effects regression model approach since it accounts for group-level effects of multiple factors on the dependent variable (fixed effects), as well as individual variation between different participants (random effects) (Baayen et al., 2008; Linck, 2016). The random effects components of mixed-effects models offer a powerful and reliable analysis tool for our research hypotheses since they provide individual estimates of change for an individual (Long, 2012). The analyses were conducted in R, the open-source software for statistical computing (R version 4.0.5; R Core Team, 2021; RStudio Team, 2021), with the lme4 package (Version 1.1–31, Bates et al., 2015). We used restricted maximum likelihood (REML) estimations for all models and the BOBYQA algorithm for optimization. We obtained p-values for model fits with the lmerTest package, which uses Satterthwaite’s degrees of freedom method (Kuznetsova et al., 2017). Model comparisons were conducted by performing a Chi-square test on the deviance statistics of models. Additional parameters were included if the more complex model showed a statistically significant improvement in model fit. All continuous predictor variables were z-transformed prior to model inclusion. Text type was added as a dummy coded fixed effect, with academic article as the reference level. We refitted models after removing outlying residuals exceeding ±2.5 standard deviations from the mean (Baayen and Milin, 2010).
Descriptive statistics for all continuous reading habits measures and incoming vocabulary knowledge are provided in Table 1. Table 2 provides breakdowns of the number of readings by text type. We present the results of two analyses. In the first analysis, we assessed change in reading speed across the duration of the bridging program. In the second analysis, we examined whether individual differences in reading experience across the study period translate into individual variability in the rate of reading speed gains.
We used linear mixed-effects regression modelling to evaluate whether week of instruction contributed significantly to the prediction of reading speed, in addition to incoming vocabulary knowledge and other control measures. We fitted a model to minutes per page with week as a fixed effect; we also included incoming vocabulary size, text type, and number of pages per reading as control variables. By-participant random intercepts were included, as were by-participant random slopes for week of instruction (min = 1, max = 26). Based on Schramm and Rouder’s (2019) recommendation, we did not log-transform minutes per page.2 A Type III ANOVA was computed to estimate the omnibus effects of the model (using the Anova function in the car package in R; Fox and Weisberg, 2011) and is presented in Table 3. The full fixed and random effects of the final model are presented in the Appendix Table A1.
There was a significant main effect of Week on reading speed: reading speed increased throughout the duration of the program [ = −1.85; SE = 0.47; t = −3.95; p < 0.001]. The result indicates a predicted net increase in reading speed (i.e., shorter reading times) of 3 min, 4 s per page between week 1 (M = 11 min, 59 s) and week 26 (M = 8 min, 55 s). Thus, EAL students gradually become faster readers, shortening reading times each week by approximately 7 s per page between the beginning and the end of the study period (see Figure 1).
Figure 1. Observed mean reading speed per week of the study. Error bars represent the standard error. Slope represents the partial main effect of week in Analysis 1.
We did not find a significant effect of Vocabulary size assessed at the outset of the study [ = −0.524; SE = 0.39; t = −1.33; p = 0.18]. Although the effect was not significant, the numerical trend was as expected: faster readers tended to have larger vocabulary sizes. There was a significant main effect of Text type on reading speed. Contrasts revealed that, compared to academic articles (M = 8.96 mpp; SD = 6.81 mpp), bridging program students were significantly faster when reading fiction texts (M = 6.52, mpp; SD = 6.02 mpp) and newspaper/magazine articles (M = 9.71 mpp; SD = 7.12 mpp) [both ps < 0.001, see Appendix Table A1]. However, there was no significant difference in reading speed between academic articles and course readings (M = 11.87 mpp; SD = 7.91 mpp) or website articles (M = 10.1 mpp; SD = 7.1 mpp) [both ps > 0.05, see Appendix Table A1]. These results indicate that text processing speed is contingent on the kind of reading activity students engage with. More challenging texts (e.g., academic articles and course readings) are processed more slowly than texts such as fiction or newspaper/magazine articles. This finding accords with research showing that more complex texts tend to require more mental effort to process during reading (Crossley et al., 2014; Kim et al., 2018).
Furthermore, there was a significant interaction between Text type and Week. The interaction terms [see Table 1] revealed that, compared to gains in reading speed for academic articles, improvement in reading speed was significantly slower for all text types [all ps < 0.05] except for newspaper magazine articles. Importantly, post-hoc significance tests on the slopes for each text type indicated that the effect of Week was reliable for each text type [ps < 0.05], except for fiction texts [p > 0.05]. This indicates that even though change in reading speed depends on the types of text that were read, reading rate improved significantly for virtually all text types (see interaction plot in the Appendix Figure A1). Since the effect of text type is not central to our research questions, we do not address these findings further.
In the second analysis, we evaluated whether the amount of experience with print materials made a unique contribution to the prediction of longitudinal change in reading speed. We implemented a two-step approach based on information from students’ individual predicted growth curves (Welten et al., 2018). In step 1, we obtained the individual growth curves from the random effects of the linear mixed-effects model fitted to change in reading speed (see model in prior analysis). In step 2, the student-specific predicted random effects were then entered into a new difference score regression model (Rogosa, 1988; Fitzmaurice et al., 2011; Thomas and Zumbo, 2012; Castro-Schilo and Grimm, 2018). The difference score model included the predicted random effects for change in reading speed as an outcome measure and total reading experience as a critical predictor [see Gunn et al., 2002 for difference score models applied to reading data].
The first step involved extracting the predicted random effects from a mixed-effects model fitted to reading speed. The model included week as a fixed effect and random intercepts for participants with random slopes for week. The predicted random effects of the model provide the mean-corrected conditional modes or best linear unbiased predictors (BLUPs). In the context of longitudinal data analysis, the BLUPs provide a reliable participant-specific metric of change in the response variable (Long, 2012). The main advantage of using BLUPs for evaluating change in an individual is that extreme values (extreme change scores in the present study) are shrunk towards the population mean (Robinson, 1991). Another advantage of using BLUPs in the present study is that they provide the estimated growth of participants with incomplete observations, i.e., participants who may not have answered the reading habits log on a particular week. Numerically, BLUPs are mean-centered and index a participant’s change relative to the estimated change curve of the entire cohort (the fixed effect). More extreme negative values indicate that weekly reading speed increases at a faster rate than the group on average, while higher positive values mean that weekly reading rate increases at a slower rate than the group on average.
We fitted an ordinary least squares multiple regression model with predicted change in reading speed, estimated via BLUPs as an outcome measure. The total number of pages read during the study period was the measure of reading experience and served as the critical predictor variable. We logarithmically transformed total number of pages because this variable was positively skewed. Control covariates included the random intercepts extracted from the linear mixed-effects model for reading speed (a measure of baseline status) and incoming vocabulary size. Correlations between all measures are displayed in Table 4.
Table 4. Correlations of baseline reading speed, incoming vocabulary size and reading experience (log total number of pages read).
The multiple regression model (Table 5) contributed significantly to explaining variance in the data: adjusted R2 = 0.68, F(3,138) = 99.12, p < 0.001. As shown in Table 5, reading experience had a significant effect on change in reading speed [β = −0.108; SE = 0.028; t = −3.925; p < 0.001]. Baseline reading speed made a significant contribution as well, indicating that students who started out the slowest made the largest gains in reading speed [β = −0.041; SE = 0.002; t = −16.797; p < 0.001]. Change in reading speed was not explained by vocabulary size [β = 0.011; SE = 0.020; t = −0.531; p = 0.6]. In sum, this analysis supports the hypothesis that total reading experience, as measured by total number of pages read throughout the duration of the study period, is a predictor of change in reading speed. Students who read the most printed materials also experienced the greatest increase in reading speed.
Table 5. Multiple regression analysis predicting change in reading speed by print reading experience after controlling for initial reading speed and incoming vocabulary size.
The model-based predicted relationship between change in total reading speed over 26 weeks and reading experience (log scale), is visualized in Figure 2 (for reading experience plotted on the linear scale, refer to Appendix Figure A2). To aid interpretability, uncorrected BLUPs are shown in the plot, and were computed by summing the corrected BLUPs with the associated fixed effect of week from the model fitted in step 1 of this analysis. Figure 2 shows that a student who reads more than 128 pages in total (roughly more than 5 pages per week) is expected to surpass the average net reading speed increase (Δ = −3 min, 4 s per page, Analysis 1).
Figure 2. The partial effect of reading experience (log scale) on change in reading speed. Change measure based on individual predicted growth curves.
We conducted a longitudinal study on the reading habits of EAL students enrolled in a university bridging program. In Analysis 1, we found that average reading speed became progressively faster during the 26-week bridging program. In Analysis 2, we found that students who read more texts over the program also made larger net gains in reading speed. We also found that students with larger incoming vocabularies, as a proxy of prior reading experience, tended to be faster readers overall. Two key findings therefore emerge from the present study: (i) EAL students are faster readers after a period of 26 weeks of language instruction, and (ii) the amount of reading experience during a period of intense language instruction is linked to the rate of reading speed gains.
As predicted, we observed that reading speed became faster over time. The weekly rate of change in reading speed was characterized by a predicted linear change over time: the average time spent reading a page of text decreased steadily at a rate of approximately 7 s per week, amounting to a 33% decrease in minutes spent reading between the first and last week of the bridging program (Figure 1). We interpret this finding as support for the idea that practice with reading promotes greater reading fluency, either via the development of word decoding skills through self-teaching (Share, 1995), or through more efficient word identification resulting from stronger mappings between orthographic form and meaning (LQH; Perfetti, 2007), or via a combination of both. Though the measure of minutes per page may serve as a crude index of reading speed, the estimated proportional increase in reading speed agrees well with estimates from studies that have used words per minute to examine within-individual change in reading speed. For example, McLean and Rouault (2017) observed a proportional increase in words per minute of 31% among a cohort of L1 Japanese EALs after a period of 30 weeks of extensive reading. A similar percentage change of 34% was found by Beglar and Hunt (2014; group 1) in a study of reading rate gains in L1 Japanese EAL university students after 28 weeks of reading practice. Furthermore, our observed increases in reading speed are also consistent with longitudinal eye movement studies from a similar cohort of Mandarin and Cantonese speaking EALs (Schmidtke and Moro, 2021; Schmidtke et al., 2023). We add to these findings, demonstrating reading speed gains after a fixed period of 26 weeks of classroom instruction and within a relatively homogeneous cohort of EALs: all students were L1 Cantonese or Mandarin speakers, were aged 17–24 years, and all began the program with similar overall English competency (Overall IELTS score of between 5.0 and 6.5).
An original contribution of this research is that we found that EAL students who read a greater quantity of English texts also saw greater net increases in reading speed. Bridging program students who read more than 128 pages of text by the end of the bridging program exhibited greater boosts to reading speed than the average student. To give a sense of scale, if a student reads an average of eight pages of English per week during the bridging program, their net reading speed increase is predicted to be an average of 1 min per page greater than a student who read 5.5 pages per week (the sample median).
While there is a substantial positive impact of reading experience on change in reading speed, it is important to note that its effect diminishes with increasing reading coverage. As Appendix Figure A2 shows, the boosting effect of additional exposure to written materials gradually plateaus, pointing to ‘a window of opportunity’ of between 0 and 250 pages as an amount of reading practice that is associated with the greatest net gains in reading speed. Based on these results, we argue that engagement with written materials is at least one factor that explains why some students can continue to make greater gains in literacy development, while others do not. Simply put, more frequent exposure to English texts provides more opportunities to practice reading and improve lexical quality (Perfetti, 2007), which in turn strengthens decoding and word identification abilities (Share, 1995).
The results of Analysis 2 show that greater exposure to reading materials during the bridging program boosts reading speed gains. This pattern of results is consistent with the premise of the ‘reciprocal causation’ account of reading development (Mol and Bus, 2011), the idea that as time spent reading increases, so does the development of reading efficiency, which in turn stimulates greater motivation to spend more time reading (see also, Aunola et al., 2002; Pfost et al., 2014). It is important to clarify that the results presented here do not directly speak to the prediction of the reciprocal causation account that more reading experience leads to greater subsequent increases in leisure time that is spent reading. However, the data support the expected outcomes of the ‘virtuous circle of reading’, that (i) greater reading experience leads to greater increases in reading outcomes, i.e., reading efficiency, and that (ii) reading experience leads to growing inter-individual differences in reading speed over time, such that the “rich get richer” while “the poor get poorer,” i.e., The Matthew Effect (Stanovich, 1986).
A limitation of this study is that the estimates of reading speed are based on self-reported data. Future studies should attempt to incorporate laboratory measures of reading speed in a more systematic and detailed fashion. For example, eye-tracking could provide a detailed temporal record of reading behavior, enabling researchers to separately examine the effects of print experience on change in (i) initial stages of text reading such as word recognition processes (e.g., first fixation duration), and (ii) later stages of passage reading that reflect meaning integration processes (e.g., re-reading time). This would provide further nuance to the interpretation of results, allowing us to pinpoint whether reading experience contributes to growth in specific measures of reading behavior or the entire reading time course (Kuperman et al., 2018).
Another limitation is that our method is not able to detect mind-wandering episodes during reading, which is characterized by a loss of attentional focus midway through a reading activity, disrupting text processing (D’Mello and Mills, 2021). As per recommendation by D’Mello and Mills (2021), a self-report question which asks participants if they noticed zoning out during reading may be the most viable method of accounting for mind-wandering effects in future studies.
In addition, it would be desirable to achieve greater precision in the estimate of reading experience. We measured reading volume by asking participants to report how many pages of each text they read, yet it is important to note that the term “page” may differ in various contexts, such as in digital or traditional print media. Differences in the amount of text on a page may vary as a function of font size, spacing, and other formatting details. We therefore acknowledge that the reported number of pages is a rough approximation of the amount of text material a student read. Of course, this issue could be resolved by providing the same texts to all students. Unfortunately, a scenario in which all students read from the same text options would not make it possible to capture the range of non-required readings that learners may engage with. In addition, while we acknowledge that minutes per page is a crude and coarse-grained approximation of reading speed, we feel it is unlikely that deliberate misreporting of reading habits could systematically explain the observed pattern of results. A dishonest goal of appearing to be a faster reader would mean that the participants would need to have known the objective of the reading habits study a priori, which they did not. Specifically, they would have had to know that we use two separate pieces of self-reported information (number of pages and time spent reading) to compute the critical outcome measure of Analysis 1 (to be used also for Analysis 2).
Prior longitudinal eye-movement research (Schmidtke and Moro, 2021) has shown that vocabulary growth during a bridging program impacts word reading time, particularly measures. Future studies on reading habits therefore might wish to examine the link between vocabulary knowledge growth with both reading speed and reading experience. Unfortunately, the final part of this study was conducted online due to COVID-19. For this reason, we were unable to collect in-person follow-up data to estimate change in vocabulary size.
A highly practical and straightforward implication of the present study is that focus should be directed at encouraging students to read in English as much as possible. Based on our results, we recommend that students in academic bridging programs read a minimum of eight pages of English text per week. Support should be offered to students who read fewer texts: these students should be encouraged to read broadly, i.e., beyond required readings, to set in motion an upward spiral of reading speed development. A strength of the present paper is that we were able to focus on reading speed development within a very homogenous sample of L2 learners of English enrolled in a bridging program. That is, the present students occupy a narrow range of English ability and share a similar L2 background (Cantonese and Mandarin). Because of this focus, our study provides a novel and applied contribution to the literature by showing that additional reading experience during an academic bridging program provides an additional boost to reading speed gains. However, future studies may wish to compare the effects of reading habits on L2 readers who learned a logographic system (morpho-syllabic) prior to learning English (i.e., the present cohort), with a sample of L2 English readers whose L1 is also alphabetic (e.g., German). This comparison would allow researchers to examine the contribution of orthographic script differences to L2 reading habits and L2 reading speed development.
We know that efficient and effective reading is essential for academic success, particularly for students who aspire to operate in a non-native language while at university. We also know that it is crucially important for EALs both to receive explicit reading instruction and to accumulate reading experience more generally (cf. Grabe, 2009, 2017). In this study, we found a within-participant increase in reading speed across a 26-week bridging program and that individual differences in change in reading speed can be explained by the amount of reading experience acquired within that same period. With this study, we have outlined an approach for further understanding and quantifying the role of reading experience on reading development among pre-university EALs. It is our hope that a study of reading habits among bridging program students can also help to motivate learners to dedicate adequate time and attention to their reading development.
The data that support the findings of this study are not available due to restrictions in the ethics protocol. R code used for analysis is available upon request.
The studies involving humans were approved by McMaster Research Ethics Board. The studies were conducted in accordance with the local legislation and institutional requirements. The participants provided their informed consent to participate in this study.
DS: Conceptualization, Formal analysis, Investigation, Methodology, Project administration, Software, Visualization, Writing – original draft. SR: Data curation, Investigation, Project administration, Software, Writing – original draft. AM: Conceptualization, Investigation, Project administration, Resources, Supervision, Writing – review & editing.
The author(s) declare that no financial support was received for the research, authorship, and/or publication of this article.
We are thankful to Kirandeep Matharu, Avneet Sandhu, Theodore Saratsiotis, and Jennifer Thammasouk for data entry. We are grateful to the Dean’s Office, Faculty of Humanities at McMaster University, for critical infrastructure support provided to the MELD Bilingualism Lab in the Centre for Advanced Research in Experimental and Applied Linguistics.
The authors declare that the research was conducted in the absence of any commercial or financial relationships that could be construed as a potential conflict of interest.
All claims expressed in this article are solely those of the authors and do not necessarily represent those of their affiliated organizations, or those of the publisher, the editors and the reviewers. Any product that may be evaluated in this article, or claim that may be made by its manufacturer, is not guaranteed or endorsed by the publisher.
The Supplementary material for this article can be found online at: https://www.frontiersin.org/articles/10.3389/feduc.2024.1286132/full#supplementary-material
1. ^Reading week is a break in the academic term, approximately midway through each term. No classes are scheduled during this time.
2. ^The length of website pages are less restricted compared to physical reading material, so we completed Analysis 1 and Analysis 2 after removing website readings. We also assessed the results after removing participants for whom website materials made up greater than 50% of the share of their total reading consumption (fewer than 10% of participants). The results of both analyses did not change when either of these factors were considered.
Acheson, D. J., Wells, J. B., and MacDonald, M. C. (2008). New and updated tests of print exposure and reading abilities in college students. Behav. Res. Methods 40, 278–289. doi: 10.3758/BRM.40.1.278
Aunola, K., Leskinen, E., Onatsu-Arvilommi, T., and Nurmi, J. E. (2002). Three methods for studying developmental change: a case of reading skills and self-concept. Br. J. Educ. Psychol. 72, 343–364. doi: 10.1348/000709902320634447
Baayen, R. H., Davidson, D. J., and Bates, D. M. (2008). Mixed-effects modeling with crossed random effects for subjects and items. J. Mem. Lang. 59, 390–412. doi: 10.1016/j.jml.2007.12.005
Bates, D., Mächler, M., Bolker, B., and Walker, S. (2015). Fitting linear mixed-effects models using lme4. J. Stat. Softw. 67. doi: 10.18637/jss.v067.i01
Beech, J. R. (2002). Individual differences in mature readers in reading, spelling, and grapheme-phoneme conversion. Curr. Psychol. 21, 121–132. doi: 10.1007/s12144-002-1008-9
Beglar, D., and Hunt, A. (2014). Pleasure reading and reading rate gains. Read. Foreign Lang. 26, 29–48.
Cain, K., and Oakhill, J. (2014). Reading comprehension and vocabulary: is vocabulary more important for some aspects of comprehension? Annee Psychol. 114, 647–662. doi: 10.4074/S0003503314004035
Castro-Schilo, L., and Grimm, K. J. (2018). Using residualized change versus difference scores for longitudinal research. J. Soc. Pers. Relat. 35, 32–58. doi: 10.1177/0265407517718387
Coxhead, A., Nation, P., and Sim, D. (2014). Creating and trialling six versions of the vocabulary size test. TESOLANZ J. 22, 13–27.
Coxhead, A., Nation, P., and Sim, D. (2015). Measuring the vocabulary size of native speakers of English in New Zealand secondary schools. N. Z. J. Educ. Stud. 50, 121–135. doi: 10.1007/s40841-015-0002-3
Crossley, S. A., Yang, H. S., and McNamara, D. S. (2014). What’s so simple about simplified texts? A computational and psycholinguistic investigation of text comprehension and text processing. Read. Foreign Lang. 26, 92–113.
Daller, M. H., and Phelan, D. (2013). Predicting international student study success. Appl. Linguist. Rev. 4, 173–193. doi: 10.1515/applirev-2013-0008
D’Mello, S. K., and Mills, C. S. (2021). Mind wandering during reading: an interdisciplinary and integrative review of psychological, computing, and intervention research and theory. Lang. Linguist. Compass 15:e12412. doi: 10.1111/lnc3.12412
Dong, Y., Tang, Y., Chow, B. W. Y., Wang, W., and Dong, W. Y. (2020). Contribution of vocabulary knowledge to reading comprehension among Chinese students: a meta-analysis. Front. Psychol. 11:525369. doi: 10.3389/fpsyg.2020.525369
Duff, D., Tomblin, J. B., and Catts, H. (2015). The influence of Reading on vocabulary growth: a case for a Matthew effect. J. Speech Lang. Hear. Res. 58, 853–864. doi: 10.1044/2015_JSLHR-L-13-0310
Ehri, L. C. (1995). “Phases of development in learning to read words by sight. Journal of research in Reading” in Reading development and the teaching of reading: a psychological perspective. eds. J. Oakhill and R. Beard (Oxford, UK: Blackwell Publishers), 79–108.
Fitzmaurice, G. M., Laird, N. M., and Ware, J. H. (2011). Applied longitudinal analysis. Hoboken, NJ: John Wiley & Sons.
Fox, J., and Weisberg, S. (2011). Multivariate linear models in R. An R companion to applied regression. Los Angeles: Thousand Oaks.
Grabe, W. (2017). “L2 Reading comprehension and development” in Handbook of research in second language teaching and learning. ed. E. Hinkel, vol. III (New York: Routledge), 299–311.
Gunn, B., Smolkowski, K., Biglan, A., and Black, C. (2002). Supplemental instruction in decoding skills for Hispanic and non-Hispanic students in early elementary school: a follow-up. J. Spec. Educ. 36, 69–79. doi: 10.1177/00224669020360020201
Huffman, J. (2014). Reading rate gains during a one-semester extensive reading course. Read. Foreign Lang. 26, 17–33.
Hu, M., and Nation, I. S. P. (2000). Vocabulary density and reading comprehension. Reading in a Foreign Language 13, 403–430.
Jorm, A. F., and Share, D. L. (1983). An invited article: phonological recoding and reading acquisition. Appl. Psycholinguist. 4, 103–147. doi: 10.1017/S0142716400004380
Juffs, A. (2020). Aspects of language development in an intensive English program. New York NY: Routledge.
Kaushanskaya, M., Blumenfeld, H. K., and Marian, V. (2020). The language experience and proficiency questionnaire (LEAP-Q): ten years later. Biling. Lang. Congn. 23, 945–950. doi: 10.1017/S1366728919000038
Kim, M., Crossley, S. A., and Skalicky, S. (2018). Effects of lexical features, textual properties, and individual differences on word processing times during second language reading comprehension. Read. Writ. 31, 1155–1180. doi: 10.1007/s11145-018-9833-x
Kuperman, V., Matsuki, K., and Van Dyke, J. A. (2018). Contributions of reader-and text-level characteristics to eye-movement patterns during passage reading. J. Exp. Psychol. Learn. Mem. Cogn. 44, 1687–1713. doi: 10.1037/xlm0000547
Kuznetsova, A., Brockhoff, P. B., and Christensen, R. H. B. (2017). lmerTest package: tests in linear mixed effects models. J. Stat. Softw. 82, 1–26. doi: 10.18637/jss.v082.i13
Linck, J. A. (2016). “Analyzing individual differences in second language research: the benefits of mixed effects models” in Cognitive individual differences in second language processing and acquisition. eds. G. Granena, D. O. Jackson, and Y. Yilmaz (Amsterdam, The Netherlands: John Benjamins), 105–128.
Locher, F., and Pfost, M. (2020). The relation between time spent reading and reading comprehension throughout the life course. J. Res. Read. 43, 57–77. doi: 10.1111/1467-9817.12289
Long, J. D. (2012). Longitudinal data analysis for the behavioral sciences using R. Thousand Oaks, California: Sage.
Martin-Chang, S. L., and Gould, O. N. (2008). Revisiting print exposure: exploring differential links to vocabulary, comprehension and reading rate. J. Res. Read. 31, 273–284. doi: 10.1111/j.1467-9817.2008.00371.x
Masrai, A., and Milton, J. (2017). Recognition vocabulary knowledge and intelligence as predictors of academic achievement in EFL context. TESOL Int. J. 12, 128–142. Retrieved from: https://eric.ed.gov/?id=EJ1247860
McLean, S., and Rouault, G. (2017). The effectiveness and efficiency of extensive Reading at developing reading rates. System 70, 92–106. doi: 10.1016/j.system.2017.09.003
Mol, S. E., and Bus, A. G. (2011). To read or not to read: a meta-analysis of print exposure from infancy to early adulthood. Psychol. Bull. 137, 267–296. doi: 10.1037/a0021890
Moore, M., and Gordon, P. C. (2015). Reading ability and print exposure: item response theory analysis of the author recognition test. Behav. Res. Methods 47, 1095–1109. doi: 10.3758/s13428-014-0534-3
Nagy, W. E., Anderson, R. C., and Herman, P. A. (1987). Learning word meanings from context during normal reading. Am. Educ. Res. J. 24, 237–270. doi: 10.3102/00028312024002237
O’Connor, M., Geva, E., and Koh, P. W. (2019). Examining reading comprehension profiles of grade 5 monolinguals and English language learners through the lexical quality hypothesis lens. J. Learn. Disabil. 52, 232–246. doi: 10.1177/0022219418815646
Oliver, R., Vanderford, S., and Grote, E. (2012). Evidence of English language proficiency and academic achievement of non-English-speaking background students. Higher Educ. Res. Develop. 31, 541–555. doi: 10.1080/07294360.2011.653958
Perfetti, C. A. (2007). Reading ability: lexical quality to comprehension. Sci. Stud. Read. 11, 357–383. doi: 10.1080/10888430701530730
Perfetti, C. A., and Hart, L. (2002). The lexical quality hypothesis. Precursors Funct Literacy 11, 189–213. doi: 10.1075/swll.11.14per
Pfost, M., Hattie, J., Dörfler, T., and Artelt, C. (2014). Individual differences in reading development: a review of 25 years of empirical research on Matthew effects in reading. Rev. Educ. Res. 84, 203–244. doi: 10.3102/0034654313509492
Project Atlas. (2023). Canadian higher education. [data set]. Available at: https://iie.widen.net/s/tnfgptjrsg/canada.
R Core Team (2021). R: A language and environment for statistical computing. R Foundation for Statistical Computing, Vienna, Austria
Raudszus, H., Segers, E., and Verhoeven, L. (2018). Lexical quality and executive control predict children’s first and second language reading comprehension. Read. Writ. 31, 405–424. doi: 10.1007/s11145-017-9791-8
Robinson, G. K. (1991). That BLUP is a good thing: the estimation of random effects. Stat. Sci. 6, 15–32.
Rogosa, D. (1988). “Myths about longitudinal research” in Methodological issues in aging research. eds. K. W. Schaie, R. T. Campbell, W. Meredith, and S. C. Rawlings (New York, NY: Springer Publishing Company), 171–209.
RStudio Team. (2021). RStudio: Integrated development environment for r [computer software manual]. Available at: http://www.rstudio.com/
Sakurai, N. (2015). The influence of translation on Reading amount, proficiency, and speed in extensive Reading. Read. Foreign Lang. 27, 96–112. doi: 10.10125/66702
Schiefele, U., Schaffner, E., Möller, J., and Wigfield, A. (2012). Dimensions of Reading motivation and their relation to Reading behavior and competence. Read. Res. Q. 47, 427–463. doi: 10.1002/RRQ.030
Schmidtke, D., and Moro, A. L. (2021). Determinants of word-reading development in English learner university students: a longitudinal eye movement study. Read. Res. Q. 56, 819–854. doi: 10.1002/rrq.362
Schmidtke, D., Rahmanian, S., and Moro, A. L. (2023). Tracking reading development in an English language university-level bridging program: evidence from eye-movements during passage reading. Biling. Lang. Congn. 26, 356–370. doi: 10.1017/S1366728922000542
Schramm, P., and Rouder, J. N. (2019). Are reaction time transformations really beneficial? PsyArXiv
Share, D. L. (2004). Orthographic learning at a glance: On the time course and developmental onset of self-teaching. J. Exp. Child Psychol. 87, 267–298. doi: 10.1016/j.jecp.2004.01.001
Share, D. L. (1995). Phonological recoding and self-teaching: sine qua non of reading acquisition. Cognition 55, 151–218. doi: 10.1016/0010-0277(94)00645-2
Stanovich, K. E. (1986). Matthew effects in Reading: some consequences of individual differences in the acquisition of literacy. Read. Res. Q. 21, 360–407. doi: 10.1598/RRQ.21.4.1
Stanovich, K. E., and Cunningham, A. E. (1992). Studying the consequences of literacy within a literate society: the cognitive correlates of print exposure. Mem. Cogn. 20, 51–68. doi: 10.3758/BF03208254
Stanovich, K. E., and West, R. F. (1989). Exposure to print and orthographic processing. Read. Res. Q. 24, 402–433. doi: 10.2307/747605
Stanovich, K. E., West, R. F., and Harrison, M. R. (1995). Knowledge growth and maintenance across the life span: the role of print exposure. Dev. Psychol. 31, 811–826. doi: 10.1037/0012-1649.31.5.811
Sternberg, R. J. (2014). “Most vocabulary is learned from context” in The nature of vocabulary acquisition. eds. M. G. McKeown and M. E. Curtis (New York, NY: Psychology Press), 89–105.
Thomas, D. R., and Zumbo, B. D. (2012). Difference scores from the point of view of reliability and repeated-measures ANOVA: in defense of difference scores for data analysis. Educ. Psychol. Meas. 72, 37–43. doi: 10.1177/0013164411409929
Thorpe, A., Snell, M., Davey-Evans, S., and Talman, R. (2017). Improving the academic performance of non-native English-speaking students: the contribution of pre-sessional English language Programmes. High. Educ. Q. 71, 5–32. doi: 10.1111/hequ.12109
Trenkic, D., and Warmington, M. (2019). Language and literacy skills of home and international university students: how different are they, and does it matter? Biling. Lang. Congn. 22, 349–365. doi: 10.1017/S136672891700075X
Welten, M., de Kroon, M. L., Renders, C. M., Steyerberg, E. W., Raat, H., Twisk, J. W., et al. (2018). Repeatedly measured predictors: a comparison of methods for prediction modeling. Diagnostic Prognostic Res. 2, 1–10. doi: 10.1186/s41512-018-0024-7
Whitford, V., and Titone, D. (2012). Second-language experience modulates first-and second-language word frequency effects: evidence from eye movement measures of natural paragraph reading. Psychon. Bull. Rev. 19, 73–80. doi: 10.3758/s13423-011-0179-5
Whitford, V., and Titone, D. (2015). Second-language experience modulates eye movements during first-and second-language sentence reading: evidence from a gaze-contingent moving window paradigm. J. Exp. Psychol. Learn. Mem. Cogn. 41, 1118–1129. doi: 10.1037/xlm0000093
Wolf, M., and Katzir-Cohen, T. (2001). Reading fluency and its intervention. Sci. Stud. Read. 5, 211–239. doi: 10.1207/S1532799XSSR0503_2
Keywords: reading development, international students, language and literacy skills, reading experience, L2 reading, vocabulary knowledge
Citation: Schmidtke D, Rahmanian S and Moro AL (2024) Reading experience drives L2 reading speed development: a longitudinal study of EAL reading habits. Front. Educ. 9:1286132. doi: 10.3389/feduc.2024.1286132
Received: 30 August 2023; Accepted: 10 January 2024;
Published: 31 January 2024.
Edited by:
Juhani Järvikivi, University of Alberta, CanadaReviewed by:
Claudia Marzi, Antionio Zampolli Institute of Computational Linguistics National Research Council, ItalyCopyright © 2024 Schmidtke, Rahmanian and Moro. This is an open-access article distributed under the terms of the Creative Commons Attribution License (CC BY). The use, distribution or reproduction in other forums is permitted, provided the original author(s) and the copyright owner(s) are credited and that the original publication in this journal is cited, in accordance with accepted academic practice. No use, distribution or reproduction is permitted which does not comply with these terms.
*Correspondence: Daniel Schmidtke, c2NobWlkZGZAbWNtYXN0ZXIuY2E=
Disclaimer: All claims expressed in this article are solely those of the authors and do not necessarily represent those of their affiliated organizations, or those of the publisher, the editors and the reviewers. Any product that may be evaluated in this article or claim that may be made by its manufacturer is not guaranteed or endorsed by the publisher.
Research integrity at Frontiers
Learn more about the work of our research integrity team to safeguard the quality of each article we publish.