- Department of Journalism and Electronic Media, University of Tennessee, Knoxville, TN, United States
YouTube is widely adopted in educational settings because it can support a variety of learning practices. However, unlike traditional learning resources, YouTube videos lack a standardized quality assurance process, posing a significant concern for educational users. The responsibility of identifying suitable educational content falls on the individual user as they navigate the site and select videos. Despite its importance, the multi-step process of video selection remains poorly understood among educational users. While it is established that most users begin with a keyword search for their topic of interest, there is limited empirical evidence on how users deliberate between returned video options, considering features such as view count and comment section sentiment, to make an informed video selection. To address this knowledge gap, this study surveyed college (1) instructors (N = 61) and (2) students (N = 300) to compare their prioritization of ten YouTube video features in relation to video selection. The results revealed fundamental similarities in their prioritization of key aspects such as accuracy, content creators’ expertise, video duration, and style. However, the analyses also suggest that instructors and students may value differing platform affordances across YouTube’s features.
1 Introduction
YouTube is a freemium social media platform that supports the viewing and sharing of video content. Although YouTube is not specifically designed to support educational uses, the versatility and diversity of its User Generated Content have led to its widespread adoption in educational settings (Allgaier, 2019). However, the emergence of YouTube in education is fraught with concerns about content quality, which are tied to the notion that user generated content is largely unregulated and not subject to the same quality assurances that are commonplace across traditional learning resources (Curran et al., 2020). While some user generated content holds legitimate educational utility, other user generated content can include inaccurate, misleading, and inappropriate material (Shoufan and Mohamed, 2022). Despite these pitfalls, little evidence-based research has sought to identify the best practices for the application of YouTube, particularly as it relates to mitigating concerns about content quality.
While the use of videos in education is a well-established practice, YouTube differs from traditional video resources because it presents users with a vast library of content (Chintalapati and Daruri, 2017). To navigate YouTube’s library, users typically conduct a keyword search related to their topic of interest (Fyfield et al., 2021; Mohamed and Shoufan, 2022). However, given that YouTube’s library of content is extremely large, keyword searches often return an unmanageable number of video options. Fyfield et al. (2021) describe that after a keyword search, significant pedagogical labor remains to sort search results and identify videos of suitable quality. Emerging research suggests that such pedagogical labor is tied to the evaluation of YouTube’s site-specific video features, such as a video’s view count, comment section feedback sentiment, and number of channel subscribers (Savitrie, 2012; Pokharel, 2014; Mohamed and Shoufan, 2022). In other words, when deliberating between multiple video options, users apply their knowledge of video features to infer a video’s quality and its educational suitability. This process is useful to users because it eliminates or drastically reduces the need to watch multiple videos to find a desirable option.
Within the extant literature, research has not yet established the features prioritized by users during the video selection process. This prioritization likely stems from an individual’s platform affordances, defined by Ronzhyn et al. (2023) as “the perceived properties of social media that emerge from technological, social, and contextual interactions, enabling and constraining specific platform uses.” Consequently, affordances mirror the value users derive from platform features, as noted by Oz et al. (2023). Moreover, it is important to recognize that educational YouTube users are not homogeneous but rather identifiable as the two distinct groups of (1) instructors and (2) students, which each deploy YouTube for different functions (Shoufan and Mohamed, 2022). With distinct roles, responsibilities, and perspectives within the educational landscape, instructors and students are likely to value differing platform features across YouTube, with implications for their video selection practices. Instructors, leveraging their expertise, are likely to prioritize features that align with pedagogical objectives, content quality, and instructional coherence. Their focus may be on functionalities that facilitate effective communication, assessment, and overall curriculum delivery. On the other hand, students are likely to prioritize features that enhance engagement, accessibility, and ease of comprehension. Their concerns may extend to the navigability of platforms, user-friendly interfaces, and interactive elements that foster a more dynamic learning experience. However, to date, little research has considered how platform affordances may relate to how educational users select videos on YouTube.
To address this knowledge gap, this study aims to understand how educational users prioritize video features in the YouTube video selection process. To achieve this, this study deploys an online survey of college instructors (N = 61) and college students (N = 300) and assesses their respective perceptions of ten distinct platform features. The analysis compares instructor and student perceptions and makes conceptual linkages to platform affordances. This study represents an essential first step in seeking best practices for the use of YouTube in education.
2 YouTube in education
YouTube’s role in education spans many instructional niches, including information-based, engagement-based, and communication-based activities (Jia, 2019). It provides functionalities such as the summarization of course content, clarification of complex concepts, explanation of domain-specific jargon, illustration of abstract phenomena, and presentation of historical footage (Jackman, 2019). Similarly, YouTube offers educational utility for students outside of traditional classroom environments in areas of distance learning (Subhi et al., 2020), homework activities (Asogwa et al., 2021), and pre-lecture preparation (Yim et al., 2019). As a tool that can be accessed remotely, YouTube was praised as a valuable supplementary learning device during the COVID-19 pandemic, which limited in-person education (Temban et al., 2021; Trabelsi et al., 2022).
Attempts to quantitatively assess the impacts of YouTube on student learning have, in general, revealed YouTube’s positive influence. A scoping review of YouTube in education, which spanned 647 publications, found that in most studies, YouTube had a positive influence on student skills, competencies, interest, motivation, engagement levels, and test performances (Shoufan and Mohamed, 2022). In the context of language learning, YouTube use was associated with lower student anxiety, improved speed of learning, increased student interest (Albahlal, 2019), and supported vocabulary learning (Maziriri et al., 2020). Similarly, in medical education, YouTube can support student understanding, memorization, and recall of anatomical information (Mustafa et al., 2020). In mathematical education, YouTube can improve problem-solving abilities (Nugroho et al., 2019) and support the understanding of mathematical concepts (Insorio and Macandog, 2022).
However, despite the potential benefits of YouTube, there are increasing concerns regarding content quality on YouTube (Shoufan and Mohamed, 2022). One possible reason for this heightening concern may be tied to algorithmic factors of video recommendation, which can work to suppress educational content in favor of content intended to entertain (Fyfield et al., 2021). Noting that effective educational videos do not always satisfy the algorithmic requirements for high levels of exposure, Fyfield et al. (2021) suggest that creators of educational content are at constant tension between producing high-quality educational content and seeking maximum exposure. This is a notion exacerbated by the reality that some YouTube content creators rely on video exposure as a source of income (Kopf, 2020).
In the context of content quality and algorithmic suppression, it is critical to understand how instructors and students navigate, evaluate, and ultimately select YouTube videos. Foundational research suggests that both instructors (Fyfield et al., 2021; Pattier, 2021) and students (Rashid and Asghar, 2016; Aldallal et al., 2019) often begin the process of video selection using a keyword search relating to their topic of interest. This technique, which is termed ‘search and scroll’ is defined as “entering a small number of keywords into YouTube’s search bar and then scrolling through the result list to find a video” (Fyfield et al., 2021). However, the search and scroll practice does not explain the precise process of video selection, which takes place among the list of returned video options. For instructors, following a keyword search, there remains significant pedagogical labor in sifting through content before arriving at a selection (Fyfield et al., 2021). This challenging and time-consuming process is further exacerbated by the notion that keyword searches often fail to return videos from known and trusted content creators, meaning that instructors often overlook their preferred sources (Fyfield et al., 2021).
Unlike instructors, emerging evidence suggests that students may avoid this laborious process by simply selecting videos from the top of returned keyword search lists (Mohamed and Shoufan, 2022). However, other seemingly contradictory findings suggest that students are accustomed to assessing YouTube video features, including view count (Savitrie, 2012) and viewer feedback (like/dislike ratio and comment section sentiment) (Pokharel, 2014) in the video selection process. An explanation for this apparent contradiction may be explained by the notion that such features correlate with YouTube’s algorithmic recommendations, meaning that videos with higher view counts and engagement rates are displayed at the top of returned lists (Mohamed and Shoufan, 2022). As such, it remains somewhat unclear as to whether students tend to select top-returned videos based on their return ranking alone or due to the assessment of other correlated features.
Perhaps indicative of the inherent challenges associated with the search and scroll strategy, there exist a growing number of online ranking lists for the top educational YouTube channels. As a strategy for video selection, both instructors and students can use these lists to identify high-quality content from reputable channels, thus drastically reducing the number of videos they must choose from. Using the Google search terms ‘top educational channels YouTube’ and ‘best educational channels YouTube’, Tadbier and Shoufan (2021) were able to identify 193 ranked lists across 101 websites. However, the authors note that while ranking lists may speed up the process of video selection, their use is problematic because many do not describe their ranking criteria, are not customized to specific educational fields, and do not specify a target audience, thus limiting their practical utility (Tadbier and Shoufan, 2021). Also, the authors explain that owing to the already large and growing number of online ranking lists, users are forced to choose between many list options, with little grounds for this decision. Similarly, while the lists often suggest a specific YouTube channel, they fail to identify specific videos, which is a particular issue among channels that house a great number of videos and span a variety of topics (Tadbier and Shoufan, 2021). As such, it seems unlikely that ranking lists will replace the predominant search and scroll strategy.
While the above literature begins to identify initial strategies deployed by instructors and students, it remains unclear how users may combine the search and scroll strategy with consideration for other site-specific features in order to satisfactorily explain the video selection process. This is striking because a holistic understanding of this process is necessary to identify best practices for the use of YouTube in education, particularly as it relates to alleviating growing concerns about content quality (Shoufan and Mohamed, 2022). Additionally, recognizing that instructors and students are likely to value varying platform affordances based on their differing characteristics and roles within the educational landscape, it is important to understand how instructors and students may navigate this process differently. In this context, we seek to understand whether instructors and students value different affordances in the process of video selection. So we asked the following research question: In the context of video selection, do instructors and students place different values on various platform features?
3 Methods
To investigate instructor and student perceptions and usage strategies regarding YouTube in education, an online survey of college instructors (N = 61) and students (N = 300) was deployed between April 1, 2022, and October 9, 2022. Below the instructor and student samples, the survey tool and data analyses are described.
3.1 Instructor sample
Data were collected from a voluntary response sample of educators employed at a large southeastern university in the United States. A recruitment email was distributed via faculty and staff email lists across each college. The recruitment email invited all instructors of record of at least one college-level class to complete the survey. Data collection lasted for 1 month, from April 1, 2022, to May 1, 2022. After 1 month, we concluded the data collection process; we obtained 67 respondents. However, as some individuals did not meet the inclusion criteria or provided incomplete data, a number of respondents were eliminated. After these data were removed, 61 complete responses were recorded.
Of the instructor sample, 24 self-identified as a professor (39.34%), 15 as an associate professor (24.59%), 20 as an assistant professor (32.79%), and two as teaching staff (3.28%). Participants reported an average of 15.53 years of teaching at the college level (SD = 11.64), an average of 7.38 teaching hours per week during the academic semester in the last year (SD = 7.21), and their primary area of teaching to be art (n = 8, 13.11%), humanities (n = 14, 22.95%), mathematics (n = 2, 3.28%), natural science (n = 8, 13.11%), and social science (n = 29, 47.54%).
3.2 Student sample
Data was collected through an online survey of college students. The survey was hosted on the platform Qualtrics, and the recruitment of participants was facilitated by the online survey company, Prolific. As an online subject pool, Prolific has demonstrated that it provides data quality that is not significantly different than that of the industry leading MTurk, while also providing a more diverse population in terms of geographical location and ethnicity (Peer et al., 2017; Palan and Schitter, 2018). Using Prolific’s pre-screening, only participants who self-identified as college students were invited to participate in the survey. Both full-time and part-time college students were invited to participate. Data collection commenced on October 3, 2022, and concluded on October 9, 2022. As only completed surveys were recorded by Prolific software, no incomplete surveys were recorded or removed from the data. As such, a total of 300 completed responses were recorded.
Participants reported their university class rank to be freshman (n = 21, 7.00%), sophomore (n = 38, 12.67%), junior (n = 65, 21.67%), senior (n = 81, 27.00%), and graduate (n = 95, 31.67%). The participant’s area of study was art (n = 14, 4.67%), humanities (n = 31, 10.33%), mathematics (n = 20, 6.67%), natural science (n = 26, 68.67%), social science (n = 206, 68.67%), and undefined/general studies (n = 3, 1.00%).
3.3 Survey tool
Participants completed an online survey that was hosted by the platform Qualtrics and distributed by the platform Prolific. Potential participants were informed that participation was voluntary, anonymous, and would take approximately 15 min to complete. The survey tool deployed predominately multiple-choice and Likert scale questions and included one open-ended question.
Initially, the survey collected essential demographic information, encompassing students’ university class and major, as well as the instructors’ job positions (e.g., assistant, associate, etc.), years of teaching experience at the university level, and their primary subject of instruction. Equally, both groups were asked to respond to the question ‘How familiar are you with the social media platform YouTube?’ Responses were measured on a five-point scale from 1 (not important at all) to 5 (extremely familiar).
To assess perceptions of ten specific video selection features, participants were asked the question: “As a student/instructor, how important are the following features when assessing the strengths and weaknesses of a YouTube video as an educational resource?” To provide context, respondents were supplied with a definition of an ‘educational resource’ as a tool facilitating and enhancing student comprehension, education, and engagement on a particular topic. Responses were captured on a five-point scale, ranging from 1 (least important) to 5 (most important). Participants evaluated the following features: content creator expertise, channel subscriber count, comment section engagement, video like/dislike ratio, entertainment value, content accuracy and validity, video age, style type (e.g., monologue, animation), duration, and total view count.
Finally, participants were asked the question: “Would you benefit from support or training regarding how to optimize your educational application of YouTube?” Responses were limited to ‘yes’ and ‘no’, with those responding ‘yes’, prompted to provide a written specification.
3.4 Data analyses
Data analysis was performed in SPSS (IBM Corporation, 2023). Predominantly, independent-samples t-tests were used to analyze Likert scale data. Additionally, qualitative data derived from open-ended textual survey responses were thematically assessed using inductive codes that emerged from the data (Braun and Clarke, 2023). This process was used to identify patterns among responses in the textual data and draw meaningful conclusions (Rouder et al., 2021).
4 Results
Foundationally, the results of this study indicate that YouTube is commonplace in college education, with 88.52% of instructors and 94.67% of students reporting the use of YouTube in their respective instruction and education. We found students to be significantly more familiar with YouTube (M = 4.76, SD = 0.52) when compared to instructors (M = 4.11, SD = 0.78); t (71.22) = 6.25, p = <0.001. Both instructors (M = 3.96, SD = 0.49) and students (M = 4.10, SD = 0.68) reported YouTube to have a positive effect on the quality of college course instruction, which was measured on a five-point Likert scale (1, Overall Negative Effect to 5, Overall Positive Effect). An independent-samples t-test revealed no significant differences between the two groups; t (89.40) = 1.74, p = 0.09.
To assess the perceived importance of ten platform features in the identification of suitable educational YouTube content, instructors and students were asked to report the relative importance of each feature on a five-point Likert scale (1, least important to 5 most important). Using the ranked mean score, we found that instructors and students have largely similar perceptions of platform features.
Notably, both instructors and students reported Accuracy and Validity, Content Creators Expertise, Video Duration, and Video Style Type, to be among the most important features, while Comment section feedback, Total View Count, and Channel Subscriber Count to be among the least important features. An independent-samples t-test revealed significant differences between instructor and student perceptions for five of the ten features evaluated at the 95% confidence level.
For the feature Channel Subscriber Count, students (M = 1.92, SD = 1.01) reported a significantly higher score than instructors (M = 1.29, SD = 0.61); t (103.94) = 6.04, p = <0.001. For the feature Comment Section Feedback and Engagement, students reported a significantly higher score (M = 2.59, SD = 1.32) than instructors (M = 1.35, SD = 0.66); t (131.96) = 10.35, p = <0.001. For the feature Video Like to Dislike Ratio, students (M = 2.86, SD = 1.35) reported a significantly higher score than instructors (M = 1.39, SD = 0.80); t (106.20) = 10.72, p = <0.001. For the feature Video Age, students (M = 3.01, SD = 1.13) reported a significantly higher score than educators (M = 2.27, SD = 1.17); t (347) = 4.27, p = <0.001. For the feature Video Total View Count, students (M = 2.21, SD = 1.13) reported a significantly higher score than instructors (M = 1.29, SD = 0.61); t (119.73) = 8.54, p = <0.001. For the remaining features, which include Video Entertainment, Video Accuracy and Validity, Video Style, Video Duration, and Content Creator Expertise, the independent-samples t-test identified no significant differences between the two groups at the 95% confidence level.
When asked if they would benefit from support or training on how to optimize their use of YouTube in education, 27.87% of instructors responded ‘yes’, compared to just 16.67% of students. Additionally, individuals that responded ‘yes’ were promoted to provide an open-ended textual response regarding the type of support or training they felt would be helpful. Thematic analysis of this data identifies three emergent themes, which are described in Table 1.
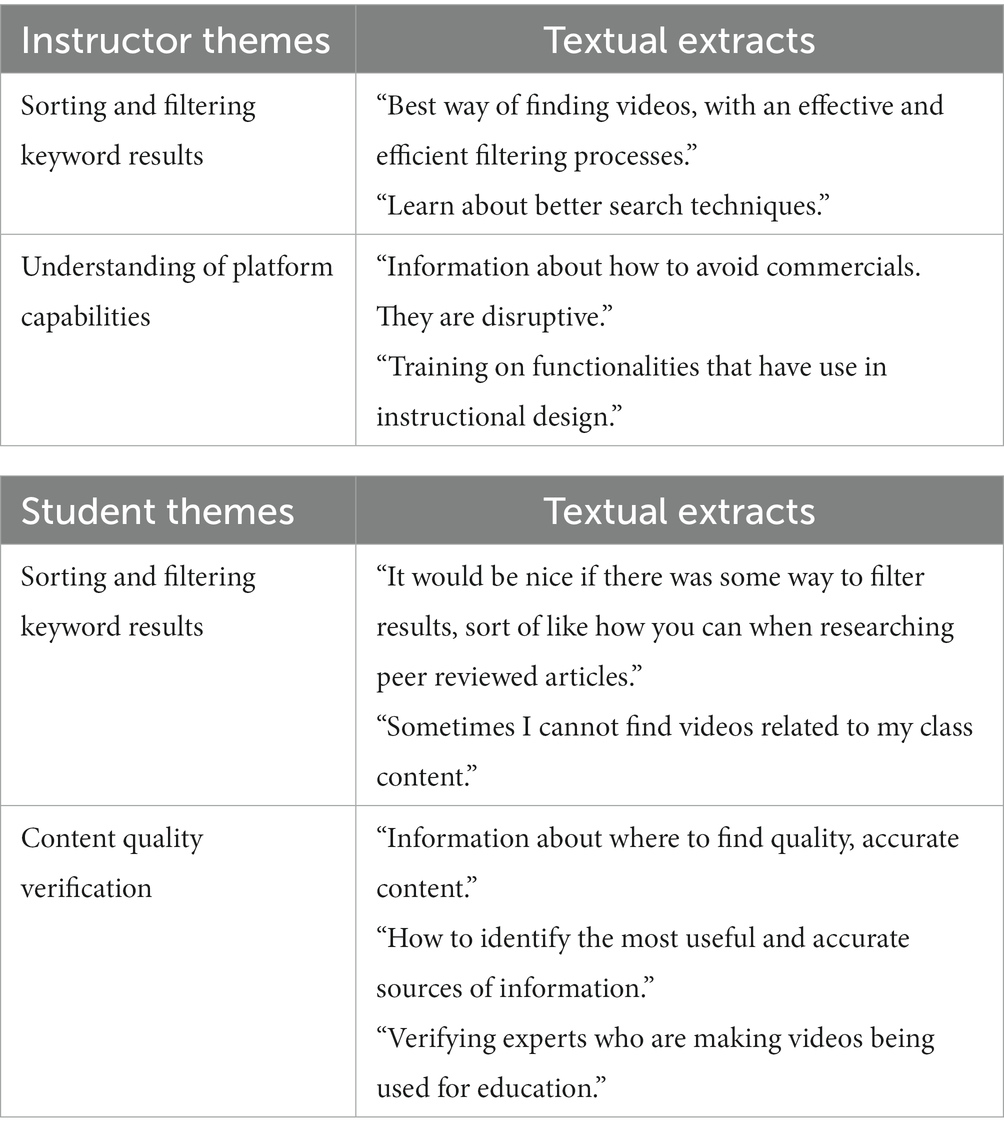
Table 1. Qualitative themes from open-ended textual responses regarding the type of support or training desired to improve YouTube’s application in education.
The first theme, which relates to the desire for training on the sorting and filtering of keyword search results, was identified across the responses provided by instructors and students. Respondents from both groups indicated a desire to be more efficient in their refinement of the returned search results. The second theme, which relates to the desire to become more familiar with YouTube’s platform capabilities, was identified in the instructors’ responses. The instructors indicated that training regarding YouTube’s features may support their instructional use of the platform. The third theme, which relates to the desire for training on content quality verification, was identified in the students’ responses. Students indicated a need for support regarding how to identify high-quality content and verify the expertise of content creators.
5 Discussion
Overall, our results suggest that YouTube is an essential resource in college education. While students demonstrate greater familiarity with the platform, both educators and students perceive the platform’s positive impact on the instructions. Students and educators shared similar perceptions in regard to the importance of some platform features, such as expertise, video duration, and video style. On the other hand, we found differences between educators and instructors regarding how they perceive some features of YouTube. For example, students reported a significantly higher score for Channel Subscriber Count than instructors. Finally, both groups emphasized the importance of training for finding relevant educational content on YouTube while focusing on different aspects of YouTube.
The contemporary literature regarding the use of YouTube in education is lacking evidence-based research. Specifically, little research seeks to understand how educational users assess platform-specific features when deliberating between multiple video options. While foundational studies suggest that most users practice a form of keyword search known as ‘search and scroll’, this understanding does not work to describe how users choose between multiple video options that are returned by a keyword search (Fyfield et al., 2021). In simple terms, we poorly understand the secondary step in the YouTube video selection process, which occurs subsequent to a keyword search. Although emerging evidence indicates that educational users may consider YouTube video features, including view count (Savitrie, 2012) and viewer feedback (like/dislike ratio and comment section sentiment) (Pokharel, 2014), it is unclear how users prioritize such features in video selection.
In this study, we hypothesized that, owing to different platform affordances, instructors and students will hold differing perceptions of YouTube video features as they relate to supporting educational video selection. However, our findings present a nuanced picture, partially contradicting this hypothesis. When assessed by importance, varying from the most to the least important, the instructor and student groups demonstrate comparable rankings of video features. Specifically, we found that both groups place the highest priority on video accuracy, content creators’ expertise, video duration, and video style, while assigning a lower priority to channel subscriber count and video view count, as outlined in Table 2. However, in interpreting these results, it is important to highlight that while the instructor and student groups exhibit similar ranking priorities, the student group reported significantly higher importance scores across five of the ten features evaluated. This supports, in part, the initial hypothesis. Specifically, we found that for the features channel subscriber count, comment section feedback and engagement, video like to dislike ratio, video age, and video view count, the students reported a significantly higher important score than the instructors.
One possible explanation for this result is tied to our related finding that students are significantly more familiar with YouTube than instructors. Given their younger demographic, students are not only more likely to be familiar with YouTube but also more accustomed to diverse social media platforms, using them more frequently than their older counterparts (Pew Research Center, 2019). This is significant because younger users can easily draw parallels in terms of site navigation, characteristics, and functionality between different social media sites, potentially influencing their perceptions of YouTube (Bucher and Helmond, 2018). For example, the desirability of large Instagram followings may be reflected in YouTube’s channel subscriber count, indicating the user’s network size. While this study does not explicitly discuss the relationship’s directionality, evidence suggests that a higher channel subscriber count on YouTube correlates with social status and prestige (Christin and Lewis, 2021), reinforcing parallels with Instagram. Therefore, students’ greater familiarity with YouTube and their likely increased use of other social media platforms may explain why they give more importance to features directly connected to platform features.
Aligned with the limitations identified in the ‘search and scroll strategy’ (Fyfield et al., 2021), this study reveals a shared need among both educators and students for training and assistance in utilizing keyword-search sorting and filtering practices on social media platforms. Our analysis of open-ended responses underscored common challenges faced by both groups in selecting videos from search results. While both instructors and students encountered similar difficulties, educators expressed a heightened desire to understand YouTube’s platform capabilities, likely influenced by their lower familiarity. Conversely, students highlighted challenges in verifying content quality, indicating a struggle to assess video quality even after engaging in preliminary viewing. Overall, these results highlighted the need for training and support using YouTube’s search and filtering features and evaluating video quality for instructors and students. Our results also emphasized the importance of media literacy in education (Jackman, 2019).
While this study focused on college instructors, future research should explore the generalizability of these findings to diverse educational contexts, including K–12 education and alternative learning methods like online education. Understanding contextual differences is crucial for optimizing learning outcomes. Future studies should establish direct links between the identified features in this study and the broader literature on platform-specific features on YouTube. The evolving functionalities of YouTube’s platform necessitate ongoing research to understand shifting affordances. Notably, changes like the removal of dislike counts since the inception of this study underscore the need for continued investigation into how YouTube can best support evolving educational needs in the future. Overall, the findings of this study underscore the need for a pedagogical approach that considers the unique features and challenges of digital platforms. Theoretical discussions within education should explore the development of pedagogical strategies that integrate platform-specific skills, recognizing the varying needs of educators and students.
Data availability statement
The raw data supporting the conclusions of this article will be made available by the authors, without undue reservation.
Ethics statement
The studies involving humans were approved by University of Tennessee, Human Research Protection Program. The studies were conducted in accordance with the local legislation and institutional requirements. The participants provided their written informed consent to participate in this study.
Author contributions
SG: Conceptualization, Data curation, Formal analysis, Funding acquisition, Methodology, Writing – original draft, Writing – review & editing. MO: Formal analysis, Funding acquisition, Writing – review & editing.
Funding
The author(s) declare financial support was received for the research, authorship, and/or publication of this article. This project was supported by an internal research grant from the University of Tennessee, Graduate School, as part of a Student Faculty Research Award.
Conflict of interest
The authors declare that the research was conducted in the absence of any commercial or financial relationships that could be construed as a potential conflict of interest.
Publisher’s note
All claims expressed in this article are solely those of the authors and do not necessarily represent those of their affiliated organizations, or those of the publisher, the editors and the reviewers. Any product that may be evaluated in this article, or claim that may be made by its manufacturer, is not guaranteed or endorsed by the publisher.
References
Albahlal, F. S. (2019). The impact of YouTube on improving secondary school students’ speaking skills: English language teachers’ perspectives. J. Appl. Linguist. Lang. Res. 9, 13–17. doi: 10.7575/aiac.ijalel.v.9n.6p.13
Aldallal, S., Yates, J., and Ajrash, M. (2019). Use of YouTube™ as a self-directed learning resource in oral surgery among undergraduate dental students: a cross-sectional descriptive study. Br. J. Oral Maxillofac. Surg. 57, 1049–1052. doi: 10.1016/j.bjoms.2019.09.010
Allgaier, J. (2019). Science and environmental communication on YouTube: strategically distorted communications in online videos on climate change and climate engineering. Front. Commun. 4:36. doi: 10.3389/fcomm.2019.00036
Asogwa, U., Duckett, T. R., Mentzer, G., and Liberatore, M. W. (2021). Impact of YouTube homework problems on students’ learning attitudes. Chem. Eng. Educ. 55, 175–182. doi: 10.18260/2-1-370.660-126292
Braun, V., and Clarke, V. (2023). Toward good practice in thematic analysis: avoiding common problems and be(com)ing a knowing researcher. Int. J. Transgend. Health 24, 1–6. doi: 10.1080/26895269.2022.2129597
Bucher, T., and Helmond, A. (2018). The affordances of social media platforms. The SAGE handbook of social media. 1, 233–253.
Chintalapati, N., and Daruri, V. S. K. (2017). Examining the use of YouTube as a learning resource in higher education: scale development and validation of TAM model. Telematics Inform. 34, 853–860. doi: 10.1016/j.tele.2016.08.008
Christin, A., and Lewis, R. (2021). The drama of metrics: Status, spectacle, and resistance among YouTube drama creators. Soc. Media Soc. 7:2056305121999660. doi: 10.1177/2056305121999660
Curran, V., Simmons, K., Matthews, L., Fleet, L., Gustafson, D. L., Fairbridge, N. A., et al. (2020). YouTube as an educational resource in medical education: a scoping review. Med. Sci. Educ. 30, 1775–1782. doi: 10.1007/s40670-020-01016-w
Fyfield, M., Henderson, M., and Phillips, M. (2021). Navigating four billion videos: teacher search strategies and the YouTube algorithm. Learn. Media Technol. 46, 47–59. doi: 10.1080/17439884.2020.1781890
IBM Corporation. (2023). IBM SPSS statistics for windows, version 29.0. Armonk, NY: IBM Corporation.
Insorio, A. O., and Macandog, D. M. (2022). Video lessons via YouTube channel as mathematics interventions in modular distance learning. Contemp. Math. Sci. Educ. 3:ep22001. doi: 10.30935/conmaths/11468
Jackman, W. M. (2019). YouTube usage in the university classroom: an argument for its pedagogical benefits. Int. J. Emerg. Technol. Learn. 14:157. doi: 10.3991/ijet.v14i09.10475
Jia, S. (2019). Literature review of YouTube in teaching activities Association for Information System, (PACIS 2019 Proceedings Available at: https://aisel.aisnet.org/pacis2019/228?utm_source=aisel.aisnet.org%2Fpacis2019%2F228&utm_medium=PDF&utm_campaign=PDFCoverPages.
Kopf, S. (2020). “Rewarding good creators”: corporate social media discourse on monetization schemes for content creators. Soc.l Media+ Soc. 6:2056305120969877. doi: 10.1177/2056305120969877
Maziriri, E. T., Gapa, P., and Chuchu, T. (2020). Student perceptions towards the use of YouTube as an educational tool for learning and tutorials. Int. J. Instr. 13, 119–138. doi: 10.29333/iji.2020.1329a
Mohamed, F., and Shoufan, A. (2022). Choosing YouTube videos for self-directed learning. IEEE Access 10, 51155–51166. doi: 10.1109/ACCESS.2022.3174368
Mustafa, A. G., Taha, N. R., Alshboul, O. A., Alsalem, M., and Malki, M. I. (2020). Using YouTube to learn anatomy: perspectives of Jordanian medical students. Biomed. Res. Int. 2020, 1–8. doi: 10.1155/2020/6861416
Nugroho, K. U. Z., Widada, W., and Herawaty, D. (2019). The ability to solve mathematical problems through youtube based ethnomathematics learning. Int. J. Sci. Technol. Res. 8, 1232–1237. doi: 10.1088/1742-6596/1731/1/012050
Oz, M., Shahin, S., and Greeves, S. B. (2023). Platform affordances and spiral of silence: how perceived differences between Facebook and twitter influence opinion expression online. Technol. Soc. 76:102431. doi: 10.1016/j.techsoc.2023.102431
Palan, S., and Schitter, C. (2018). Prolific.Ac—a subject pool for online experiments. J. Behav. Exp. Financ. 17, 22–27. doi: 10.1016/j.jbef.2017.12.004
Pattier, D. (2021). ‘Teachers and YouTube: the use of video as an educational resource’, Ricerche di Pedagogia e Didattica. J. Theor. Res. Educ. 16, 59–77. doi: 10.6092/issn.1970-2221/11584
Peer, E., Brandimarte, L., Samat, S., and Acquisti, A. (2017). Beyond the Turk: alternative platforms for crowdsourcing behavioral research. J. Exp. Soc. Psychol. 70, 153–163. doi: 10.1016/j.jesp.2017.01.006
Pew Research Center. (2019). Millennials stand out for their technology use, but older generations also embrace digital life. Available at: https://www.pewresearch.org/short-reads/2019/09/09/us-generations-technology-use/ (Accessed February 11, 2023).
Pokharel, O. (2014). Impact of User-Generated Contents on End-Users: Self-Directed Learning using YouTube University of Alberta Available at: https://era.library.ualberta.ca/items/713275c0-744d-4e25-a927-39c7b6568a2d/view/c9274f33-b0ee-43d3-b159-5e13d4795e3f/Pokharel.pdf.
Rashid, T., and Asghar, H. M. (2016). Technology use, self-directed learning, student engagement and academic performance: examining the interrelations. Comput. Hum. Behav. 63, 604–612. doi: 10.1016/j.chb.2016.05.084
Ronzhyn, A., Cardenal, A. S., and Batlle Rubio, A. (2023). Defining affordances in social media research: a literature review. New Media Soc. 25, 3165–3188. doi: 10.1177/14614448221135187
Rouder, J., Saucier, O., Kinder, R., and Jans, M. (2021). What to do with all those open-ended responses? Data visualization techniques for survey researchers. Survey Pract. doi: 10.29115/SP-2021-0008
Savitrie, P. (2012). The use of authentic videos taken from youtube in the teaching of english to the ninth graders of SMP Negeri Repository of Univeristy of Negeri Malang Available at: http://repository.um.ac.id/id/eprint/11113.
Shoufan, A., and Mohamed, F. (2022). YouTube and education: a scoping review. IEEE Access 10, 125576–125599. doi: 10.1109/ACCESS.2022.3225419
Subhi, M. A., Nurjanah, N., Kosasih, U., and Rahman, S. A. (2020) ‘Design of distance lectures in mathematics education with the utilization of the integration of zoom and YouTube application’, in. Journal of Physics: Conference Series, IOP Publishing, p. 012058.
Tadbier, A. W., and Shoufan, A. (2021). Ranking educational channels on YouTube: aspects and issues. Educ. Inf. Technol. 26, 3077–3096. doi: 10.1007/s10639-020-10414-x
Temban, M. M., Hua, T. K., and Said, N. E. M. (2021). Exploring informal learning opportunities via youtube kids among children during COVID-19. AJIS 10:272. doi: 10.36941/ajis-2021-0083
Trabelsi, O., Souissi, M. A., Scharenberg, S., Mrayeh, M., and Gharbi, A. (2022). YouTube as a complementary learning tool in times of COVID-19: self-reports from sports science students. Trends Neurosci. Educ. 29:100186. doi: 10.1016/j.tine.2022.100186
Keywords: YouTube, education, pedagogy, student, instructor, affordances
Citation: Greeves S and Oz M (2024) YouTube in higher education: comparing student and instructor perceptions and practices. Front. Educ. 8:1330405. doi: 10.3389/feduc.2023.1330405
Edited by:
Jean-Pierre Thibaut, Université de Bourgogne, FranceReviewed by:
Layla Hasan, University of Technology Malaysia, MalaysiaFatimah Alhashem, Gulf University for Science and Technology, Kuwait
Katarzyna Anna Bobrowicz, University of Luxembourg, Luxembourg
Copyright © 2024 Greeves and Oz. This is an open-access article distributed under the terms of the Creative Commons Attribution License (CC BY). The use, distribution or reproduction in other forums is permitted, provided the original author(s) and the copyright owner(s) are credited and that the original publication in this journal is cited, in accordance with accepted academic practice. No use, distribution or reproduction is permitted which does not comply with these terms.
*Correspondence: Scott Greeves, c2dyZWV2ZXNAdm9scy51dGsuZWR1