- 1Department of Educational Psychology, University of Minnesota, St. Paul, MN, United States
- 2Department of Educational Psychology, South Valley University, Qena, Egypt
Creative self-efficacy (CSE) has recently received much attention due to its association with student learning and creativity. To that end, a CSE scale was developed for undergraduates and sources of validity evidence based on scale content, response processes, and internal structure were collected. Score reliability, using categorical omega based on the categorical confirmatory factor analysis model and marginal reliability for response pattern scores based on item response theory (IRT), were estimated. After various revision iterations of the initial 28-item pool by 10 subject matter experts and 18 undergraduates, some items were revised, four items were dropped, and ultimately 24 items were field tested for measuring two hypothesized dimensions of CSE among 602 undergraduates. Categorical confirmatory factor analysis results indicated that the two-dimensional model had better fit. Similarly, between the two competing multidimensional IRT models, the two-dimensional graded response model had the best fit. Categorical omega coefficients and marginal reliability for response pattern scores were, respectively, 0.88 and 0.81 for the two underlying dimensions.
1 Introduction
Self-efficacy theory of behavioral change has been one of the most widely studied topics in the modern social-cognitive sciences. Self-efficacy has been defined as individuals’ beliefs in their ability to exert efforts necessary to reach a specific level of performance and attainments (Bandura, 1977, 1997). Bandura (1977, 2000) argued that people’s perceived self-efficacy affects their aspirations, choice of activities, behavioral settings, goals, outcome expectations, quality of functioning, the amount of effort exerted, and level of persistence while facing challenges and obstacles to reach the required level of performance. Self-efficacy has therefore received much attention specifically in the field of social learning in various domains and populations (Betz and Hackett, 1983; Gist et al., 1989; Sanna, 1992; Pajares, 2009; Köseoğlu, 2015; Wilde and Hsu, 2019; Cheng et al., 2020).
Based on Bandura’s concept of general self-efficacy, researchers have identified domain-specific concepts such as academic self-efficacy (Schunk and Pajares, 2002), emotional self-efficacy (Kirk et al., 2008), and creative self-efficacy (CSE; Tierney and Farmer, 2002). Before diving into the CSE concept, the Four-C model of creativity is discussed (Kaufman and Beghetto, 2009), given its association with individuals’ potential to be creative. Kaufman and Beghetto (2009) distinguished among four dimensions of creativity: (a) Big-C, (b) Pro-C, (c) Little-C, and (d) Mini-C. First, Big-C, also known as eminent creativity, refers to high level original ideas and is demonstrated by great artists and brilliant scientists. Second, Pro-C designates professional-level expertise in any creativity area (e.g., art). Third, Little-C, also called everyday creativity, refers to creativity embedded in daily life activities, innovations, and experiences. Fourth, Mini-C indicates the types of creativity inherent in the learning process (i.e., transformative learning). Bereczki and Kárpáti (2018) noted that the Four-C model highlights the potential for every individual to be creative, which speaks directly to CSE.
Given the significance of developing creative performance and its antecedents, a great deal of research has been conducted on CSE. The roots of CSE go back to Schack (1989) who argued that general self-efficacy could be studied within the context of creative performance. Bandura (1997) also argued that individuals’ self-efficacy was important to seek new knowledge and produce creative solutions. Based on these views, Tierney and Farmer (2002) officially proposed the CSE construct and tried to link people’s beliefs and thoughts about their creative abilities on the one hand with their creative performance on the other hand.
CSE is, therefore, a relatively recent concept of domain specific self-efficacy that has received much attention in the contexts of achievement and creativity. In the sections that follow, there is a detailed discussion on its significance, conceptualization, and measurement with special emphasis on its dimensionality and the need to identify students with low CSE. Additionally, CSE previous unidimensional and multidimensional measures were reviewed, illustrating their limitations and the need to develop a new scale with advanced measurement models such as categorical confirmatory factor analysis (CCFA) with the weighted least squares mean and variance (WLSMV) adjusted estimator and item response theory (IRT).
1.1 CSE significance
Similar to the role of general self-efficacy in enhancing individuals’ beliefs about their ability to perform well in various contexts, CSE has been found to support individuals’ performance in academic and creative contexts. For instance, Beghetto (2006) investigated the association between CSE and motivational beliefs among middle and high school students. He found that students’ with higher levels of CSE had positive beliefs about their academic abilities. Tan et al. (2008) pointed out that individuals should trust themselves to perform creatively, since CSE represented the latent psychological process that affected their self-confidence to come up with novel ideas. Michael et al. (2011) argued that CSE improved learners’ motivation, cognitive abilities, and achievement strategies specifically with challenging tasks that required generating novel ideas and solutions. Michael et al.’s (2011) argument has been partially supported, since achievement may also lead to higher levels of CSE among learners. CSE also mediated the association between various positive psychological variables such as ability, cognitive motivation, learning orientations, personality, and self-confidence on one hand, and creative performance on the other hand (Choi, 2004; Tan et al., 2007; Gong et al., 2009; Mathisen and Bronnick, 2009; Tierney and Farmer, 2011; Jaussi and Randel, 2014; Wang et al., 2014; Alzoubi et al., 2016; Yang et al., 2020). Karwowski et al. (2013) found that openness to experience, extraversion, and conscientiousness were positively correlated with CSE in a nationwide sample of Poles.
With regard to creativity prediction, development, and improvement, CSE has been empirically found to moderate and/or be a strong predictor of creative performance within various populations (Tierney and Farmer, 2002; Lemons, 2010; Karwowski, 2011, 2014; Putwain et al., 2012; Richter et al., 2012; Simmons et al., 2014). Relatedly, students with higher levels of CSE have attempted to find real situations to demonstrate their creative performance, have positive emotions and psychological well-being, and be aware of their points of strength and weakness (Yu, 2013a). Additionally, some authors have recently argued that CSE has been an essential psychological attribute to understand and improve creative personal identity and creative performance based on different creativity measures (e.g., Karwowski, 2016; Haase et al., 2018; Shaw et al., 2021). Collectively, CSE has appeared to be an important attribute for predicting, developing, and improving creative performance.
Due to its significance in various contexts, some researchers have highlighted the necessity of developing students’ CSE through creativity training and other related intervention programs (Mathisen and Bronnick, 2009; Vally et al., 2019). Karwowski (2012) also emphasized the need to investigate the psychological variables needed to develop CSE. Puente-Diaz and Cavazos-Arroyo (2016) found that task/self-approach goals and trait curiosity had positive influence on CSE and could be antecedents that should be enhanced to improve individuals’ CSE. Kong et al. (2019) recommended enhancing CSE through goal orientation and team learning behavior.
1.2 CSE conceptualization
One of the earliest definitions of CSE lies in individuals’ beliefs about their ability to think and perform creatively (Tierney and Farmer, 2002). Beghetto (2006) defined CSE as “students’ beliefs about their ability to generate novel and useful ideas” (p. 450). In their study among 279 high school students in Singapore, Tan et al. (2008) defined CSE in terms of four components, namely cognitive style, working style, personal traits, and domain-relevant skill. Abbott (2010) conceptualized CSE as individuals’ self-beliefs to express their creative thinking and performance in various contexts. He argued that there were two main dimensions for CSE: (a) creative thinking self-efficacy (CTSE) and (b) creative performance self-efficacy (CPSE). In delineating Tierney and Farmer’s (2002) definition, Abbott (2010) provided a theoretical framework for viewing CSE as a two-dimensional construct. Beghetto et al. (2011) defined it as individuals’ self-judgment in terms of their ability to generate creative ideas and solutions characterized by novelty. Aqdas et al. (2016) conceptualized CSE in terms of individuals’ beliefs about their creative performance. Farmer and Tierney (2017) defined it as individuals’ beliefs about their ability to produce creative outcomes. When synthesizing various definitions of CSE, two common themes have emerged in terms of individuals’ beliefs about their ability to: (a) generate novel ideas and (b) perform creatively.
1.3 Measuring CSE
There have been two research streams related to developing instruments to measure CSE. Some researchers have viewed CSE as a unidimensional construct, whereas others have viewed it as a multidimensional construct. Both views are introduced in the next two subsections. In addition, limitations of previous measures are also discussed, which serve the basis for developing the CSE scale in the present study.
1.3.1 Unidimensional measures of CSE
When proposing the CSE construct, Tierney and Farmer (2002) introduced the first instrument with an initial item pool containing 13 items. After field testing among 233 employees and conducting exploratory factor analysis (EFA), they obtained a three-item instrument rated on a seven-point scale (1, very strongly disagree; 7, very strongly agree). It demonstrated a good level of score reliability with two college of business departments (manufacturing, α = 0.83; operations, α = 0.87). Choi (2004) developed a four-item scale to measure CSE among 386 undergraduates in a classroom context. The author reported only a score reliability coefficient (α = 0.71) without any indication to validity evidence, which might limit its utility. Beghetto (2006) developed a three-item scale to measure middle and high school students’ CSE in a sample of 1,322 students. Similar to Choi (2004), the author reported only a score reliability coefficient (α = 0.86) without any reference to validity evidence that might invalidate score-based inferences. Yang and Cheng (2009) developed a 13-item scale administered to 94 system developers in Taiwan. EFA results yielded a one-factor solution that explained 57% of the total variance with 0.94 coefficient alpha for score reliability.
Brockhus et al. (2014) created a 10-item scale to measure CSE among 49 undergraduates in the Netherlands. Unfortunately, the authors did not report any psychometric evidence about validity or score reliability of the measure; instead, they referenced information from two related existing scales. Sangsuk and Siriparp (2015) utilized a sample of 105 undergraduates in Thailand to examine the five-factor model of CSE: (a) idea generation, (b) concentration, (c) tolerance of ambiguity, (d) independence, and (e) working style. Based on EFA and CFA results, the authors confirmed a single latent factor structure with adequate model-data fit [χ2(4) = 5.98, p = 0.21, CFI = 0.99, TLI = 0.98, RMSEA = 0.07, and RMR = 0.03]. However, the authors did not conduct item-level CFA, which has been a recommended psychometric practice to collect validity evidence-based on internal structure. Rather, they conducted CFA on the subdomains level, which assumes measures of the subdomains (sums/means) are free from error – an assumption unlikely to hold in real data.
In a recent endeavor, Karwowski et al. (2018) developed a short scale to measure CSE and creative personal identity using six and five items, respectively. Using five different samples from Poland, the authors confirmed the factor structure of the scale, estimated its score reliability, and demonstrated its validity evidence based on relations with other variables (e.g., divergent thinking, emotional intelligence, intrinsic motivation, self-esteem). In a follow up study, Shaw et al. (2021) used the graded response model (GRM) to investigate the psychometric properties of the six-item CSE subscale. Administering the scale to 173 ethnically diverse US college students, the authors confirmed CSE unidimensionality. They also reported adequate measurement precision (marginal reliability = 0.82) and correlation with openness to experience (r = 0.23, p < 0.01). They also found none of the six items functioned differently across gender subgroups using differential item functioning analysis.
1.3.2 Multidimensional measures of CSE
Turning to the multidimensional view of CSE, Abbott (2010) used a mixed method approach to measure CSE among 297 undergraduates in a US Midwestern research university. He proposed a two-dimensional factor structure to measure CSE: (a) CTSE (fluency, flexibility, elaboration, and originality) and (b) CPSE (domain, field, and personality) with four items for each of the seven subscales, totaling 28 items. The author also conducted interviews to understand how the four cohorts of individuals (high in CTSE, low in CTSE, high in CPSE, low in CPSE) viewed CSE and creativity. CFA results with robust maximum likelihood supported the revised model with three items for each subscale and 21 items total [χ2(178) = 295.571, p < 0.01, CFI = 0.95, RMSEA = 0.05, SRMR = 0.06, and AIC = 52758.669]. In a sample of 545 secondary schools students in Shanghai, Tan et al. (2011) investigated the factor structure of a multidimensional CSE scale. It consisted of 29 items with five subscales: (a) idea generation (seven items), (b) concentration (six items), (c) tolerance of ambiguity (three items), (d) independence (six items), and (e) working style (seven items). CFA results supported the model-data fit [χ2(308) = 893.47, p < 0.01, CFI = 0.93, RMSEA = 0.06].
Yu (2013a) adapted Tierney and Farmer’s (2002) scale and created a new nine-item measure by generating more items to assess students’ self-beliefs about their talent and expertise in life as well as willingness to take risks by trying out new ideas. Responses from 158 undergraduates were factor analyzed by means of EFA with Varimax rotation. Results yielded a two-factor solution that explained 68% of the total variance. The first factor had four items and was named creative intention, whereas the second had five items and was named creative behavior. In a study among 135 undergraduates in China, Yu (2013b) investigated the factorial structure of a 12-item CSE measure testing two competing three and four-factor models. Using maximum likelihood, CFA results supported the proposed four-factor model-data fit: (a) fluency self-efficacy, (b) flexibility self-efficacy, (c) originality self-efficacy, and (d) elaboration self-efficacy [χ2(76) = 191.4, p < 0.01, CFI = 0.91, TLI = 0.81, IFI = 0.91, SRMR = 0.08].
Alotaib (2016) examined the psychometric properties of the Arabic version of Abbott’s. CSE inventory among 320 distinguished undergraduate students in Saudi Arabia. The author used two analytic techniques: (a) principal component analysis and (b) CFA. For the first, the author used Varimax rotation and results yielded a two-factor solution that explained 77% of the total variance, where the first and second factors accounted for 43 and 34%, respectively. For the second, the author utilized CFA with maximum likelihood to compare the one- vs. the two-factor model data fit. The author concluded that the two-factor model had adequate fit [χ2(56) = 356.61, p < 0.01, CFI = 0.93, IFI = 0.99, RMSEA = 0.04, 90% CI = 0.02–0.07]. Taken together, results of exploratory and confirmatory techniques supported the two-dimensional factor structure hypothesized by Abbott (2010).
Hung (2018) used the rating scale model (RSM) to validate a CSE scale developed by Huang and Hung (2009), which consisted of 12 items distributed evenly over three subscales: (a) efficacy of creative thinking, (b) efficacy of creative production, and (c) persistence of efficacy in the face of negative feedback. A total of 1,416 Taiwanese students (759 university students, 235 high school students and 422 junior high school students) responded to the five-point rating scale items. The author confirmed the three-factor model via the deviance test results of three competing models. Marginal reliability based on the IRT scores ranged from 0.80 to 0.82. To conclude, the development of unidimensional and multidimensional measures to assess CSE among different populations has emphasized the importance of CSE in individuals’ creative performance and other attributes as illustrated earlier in the manuscript.
1.4 Limitations of previous measures
To conclude, there have been two main research streams related to CSE dimensionality. Some researchers have viewed CSE as a unidimensional construct (Tierney and Farmer, 2002; Choi, 2004; Beghetto, 2006; Yang and Cheng, 2009; Brockhus et al., 2014; Sangsuk and Siriparp, 2015; Karwowski et al., 2018; Shaw et al., 2021), whereas others have viewed it as a multidimensional construct (Abbott, 2010; Tan et al., 2011; Yu, 2013a,b; Alotaib, 2016; Hung, 2018).
Based on a thorough review of previous literature conducted to construct new measures for CSE or gather validity evidence for existing measures, there were some limitations with psychometric methodology serving as a key component. First, some measures consisted only of three, four, or six items (Tierney and Farmer, 2002; Choi, 2004; Beghetto, 2006; Karwowski et al., 2018) that might cause construct underrepresentation as a major threat to validity evidence based on the scale content, and consequently invalidate score interpretations related to CSE content coverage (American Educational Research Association, American Psychological Association, and National Council on Measurement in Education, 2014). Second, some researchers utilized EFA or principal component analysis with Varimax rotation ignoring the intercorrelations among the resulting factors (Yu, 2013a; Alotaib, 2016). Ignoring factor intercorrelations have yielded biased factor loadings (e.g., cross-loadings; Zopluoglu and Davenport, 2017). Third, most CFA studies utilized maximum likelihood that assumes multivariate normality, which is not a property of ordinal data, leading to biased parameter estimates. With this in mind, other robust estimators should be utilized to fit ordinal data (more details are provided in the Data Analysis section below).
Fourth, most authors relied essentially on fit indices to accept or reject hypothesized factor structures when using CFA (Tan et al., 2007; Yu, 2013b; Sangsuk and Siriparp, 2015; Alotaib, 2016). However, from a psychometric perspective, it has been recommended in the structural equation modeling literature not to depend solely on fit indices to judge the model-data fit, since they have been descriptive indices for the lack of fit (e.g., Brown, 2015). In more detail, it has been sometimes the case where a researcher generally has found good fit indices indicating model-data fit, but still some items with low standardized loadings, which have contributed much error to the model, and consequently should be investigated and revised (McNeish et al., 2018). Other parameter estimates should accordingly be examined such as standardized estimates (i.e., loadings), error variance, and latent factors correlations (Kline, 2011).
Although Abbott (2010) developed a 21-item instrument for measuring CSE, some limitations may threaten validity of score-based inferences. First, the author used robust maximum likelihood, which tends to underestimate factor loadings with ordinal data (Li, 2016). Second, the inventory consisted of seven sub-dimensions with three items each, which may not be appropriate to fully represent the sub-constructs being assessed (i.e., construct underrepresentation; American Educational Research Association, American Psychological Association, and National Council on Measurement in Education, 2014). Third, the sample size (n = 297) utilized in the study may not be large enough to guarantee cross-validation of results across other samples (i.e., produce stable parameter estimates). Hung (2018) utilized the RSM, which does not provide discrimination parameters to assess the contribution of individual items to the scale, however. Additionally, the author depended solely on the deviance test to select the model that best fits the data. Accordingly, additional criteria should be used in selecting the model that best fits the data (more details are provided in the Data Analysis section below). Despite using an IRT framework, the sample size utilized in Shaw et al. (2021) analysis is likely a concern for producing stable parameter estimates, given the recommendation to use a minimum of 500 participants with the GRM (de Ayala, 2009, p. 223).
Regarding score reliability, only alpha coefficient was reported in most studies despite its strict assumptions of the essentially-tau equivalent measurement model (Graham, 2006). Alternatively, categorical omega should be used particularly with ordinal data, which has yielded unbiased score reliability estimates (more information is provided in section 2.4). All the above-mentioned methodological and psychometric limitations may invalidate score-based inferences and consequently influence utility of the existing measures.
1.5 Present study
Despite the significance of CSE, some related major research questions have remained unanswered (Chang and Yang, 2012; Alotaib, 2016; Liu et al., 2016). One of these major research questions has been how to best construct an instrument for measuring CSE and validate its intended score interpretations for proposed uses using advanced psychometric models such as CCFA and IRT. Prior research was extended by utilizing these two advanced measurement models to construct a new measure for CSE among Egyptian undergraduates, given that all existing measures were developed and validated in other populations, and had several methodological and/or psychometric limitations as illustrated above. Thus, there were three research questions under investigation: (a) which model fits the data better: the unidimensional or two-dimensional CCFA?; (b) which IRT model fits the data better: the two-dimensional RSM or the two-dimensional GRM assuming that CCFA yielded a two-dimensional structure consistent with the theoretically adopted dimensionality of CSE?; and (c) how comparable are the categorical omega coefficients based on factor analytic models and marginal reliability for response pattern scores based on IRT?
2 Methods
2.1 Participants
Participants were 602 undergraduates (212 males, 390 females) enrolled in a large public university in Upper Egypt (Age range: 20–22 years, M = 20.92, SD = 0.40). They were recruited during an undergraduate cognitive psychology class. In particular, the author invited students to participate explaining the objectives of the study through face-to-face interaction. The class included students from humanities (e.g., history), literary (e.g., English studies), and scientific (e.g., biology) fields of study. The study sample also included students from various levels of socioeconomic status, since this region of Egypt is typically resided by high, middle, and low class families. Participants’ diversity with regard to their social and economic status as well as major and gender groups has increased the likelihood of generalizing the study results.
2.2 Development of the CSE scale
The first step in developing a measurement instrument (e.g., scale) is to specify intended score interpretations and uses (American Educational Research Association, American Psychological Association, and National Council on Measurement in Education, 2014; Sireci and Faulkner-Bond, 2014). In the present study, the main intended score interpretation was that scores reflected undergraduates’ level of both self-beliefs about their abilities to think and perform creatively as two hypothesized dimensions of CSE. The scale is intended to be used in creativity programs where undergraduates with low CSE in the two hypothesized dimensions should be directed to receive intervention. The interpretation/use argument (IU argument) outlined by Kane (2013) was utilized in the present study. The principle underlying IU argument was outlining score interpretation and collecting validity evidence that supported the intended score interpretations and uses. Given the intended score interpretations and uses specified above, validity evidence based on scale content, response processes, and internal structure was collected consistent with the IU argument and the recommendations of the American Educational Research Association, American Psychological Association, and National Council on Measurement in Education (2014). A more detailed description of the scale development process with emphasis on collecting the three sources of validity evidence is below.
2.2.1 Validity evidence based on scale content
When writing scale items, the CSE scale content went through various steps to ensure the appropriateness of the scale development procedures (Sireci, 1998; American Educational Research Association, American Psychological Association, and National Council on Measurement in Education, 2014; Sireci and Faulkner-Bond, 2014). First, a comprehensive and thorough literature review was conducted to identify existing studies and measures of CSE validated within different populations and contexts. This step was paramount to ensure rigorous understanding of the construct and its dimensionality. Second, CSE was operationally defined as “undergraduates’ self-beliefs about their ability to generate creative ideas and perform creatively in challenging tasks such as problem solving requiring novel solutions” (i.e., the domain definition). Thus, undergraduates’ self-beliefs about their ability to generate creative ideas and perform creatively were hypothesized as two dimensions for CSE. Third, an item pool of 28 items was drafted (15 items for the first dimension, 13 items for the second dimension) based on the domain definition and comprehensive and thorough literature review. Then, domain representation and relevance were assessed.
2.2.1.1 Domain representation
A total of 10 subject matter experts (SMEs) reviewed the item pool to ensure domain representation. To that end, they were provided with the construct operational definitions and its two hypothesized dimensions to determine if the item pool fully and sufficiently represented the CSE domain. To do that, they were asked to provide a rating about the degree to which each item was important to represent the CSE domain using a five-point scale: (1) not at all important, (2) slightly important, (3) important, (4) fairly important, and (5) very important. To be considered representative of the domain, eight SMEs (80%) should rate an item as important or above, and consequently could be retained in the scale.
2.2.1.2 Domain relevance
The main objective of this step was to ensure that the item pool did not include irrelevant content, redundant items, or any other source of construct-irrelevant variance, which is a major threat to validity of score-based inferences. Similar to domain representation, SMEs were asked to rate each item’s relevance to the CSE domain using a five-point scale: (1) not at all relevant, (2) slightly relevant, (3) relevant, (4) fairly relevant, and (5) very relevant. To be considered relevant to the domain, eight SMEs (80%) should rate an item as relevant or above. In addition, while providing their ratings, SMEs were asked to suggest language edits, if any, to improve item clarity and consequently its readability.
Four items received low ratings (e.g., less than 80%) with regard to both their domain representation and relevance. Specifically, two items were redundant. For instance, “I have the ability to generate novel ideas” shares similar content with the first item “I think I can come up with novel ideas.” Two other items were not relevant to the CSE domain (e.g., I enjoy drawing creative images), which assesses creative ability in a specific creativity domain rather than general self-beliefs about thinking or performing creatively. As a result, the four items were removed. Additionally, SMEs also made language revisions for some items to increase their clarity and readability and consequently their accessibility. Editing and revising items were important procedures to remove construct-irrelevant variance.
As illustrated, collecting validity evidence based on scale content followed the steps emphasized in related literature. A second important source of validity evidence for intended score interpretations is related to response processes (American Educational Research Association, American Psychological Association, and National Council on Measurement in Education, 2014; Padilla and Benítez, 2014), which was mostly ignored in all existing measures discussed above. To fill the gap and support validity of score interpretations, validity evidence based on response processes was collected.
2.2.2 Validity evidence based on response processes
To collect validity evidence based on response processes, cognitive interviews (CIs) were conducted (Padilla and Leighton, 2017; Peterson et al., 2017). CIs had two analytic types: (a) reparative and (b) descriptive (Willis, 2015). The former was used to identify problematic items and revise them, whereas the latter was used to describe the response processes underlying scale items, which were crucial for validity of score-based inferences (American Educational Research Association, American Psychological Association, and National Council on Measurement in Education, 2014).
To conduct CIs, previous researchers recommended interviewing 10 to 30 participants (Padilla and Leighton, 2017). In line with this recommendation, 18 undergraduates were interviewed for reparative and descriptive purposes. To that end, participants were asked to read each item, repeat it in their language (i.e., to assess comprehension), and find the response option that best captured their preferred response among the five presented response options. To avoid potential confusion for participants when field testing scale items, a relatively strict quantitative cutoff was adopted to submit an item for more revision. Based on this process, an item was deemed confusing if four participants (20%) repeated it differently in their own language. Fortunately, only two items were subject to more revision, since more than four participants provided different interpretations. For instance, participants interpreted item 10 differently “I have fun when I like to think creatively.” In more detail, some participants interpreted it as having fun is the result of thinking creatively, whereas others interpreted it the vice versa (i.e., fun leads to creative thinking). This item was revised to be “Thinking of creative ideas is an exciting activity,” to make the focus on thinking creatively rather than having fun, which increased its clarity. It is worth noting that the potential rationale underlying the fewer items deemed confusing was following item writing guidelines when developing the item pool, and considering SMEs’ suggestions to revise some items when collecting validity evidence based on scale content.
With regard to the descriptive approach, participants were asked to describe their thought processes required to provide a response or more technically their underlying response processes. These responses were qualitatively analyzed by classifying them into schemes such as imaging a context where an undergraduate was being able to: (a) provide creative ideas and (b) perform creatively, given that these were the two dimensions on which inferences were to be made. To conclude, collecting validity evidence based on response processes was important to ascertain that scale items were clear (i.e., removing sources of construct-irrelevant variance that might affect participants’ responses) and appropriately assessed the hypothesized underlying response processes, which are critical to validity of score-based inferences.
After collecting validity evidence based on scale content and response processes, the final version of the CSE scale consisted of 24 items with two subscales: (a) self-beliefs about creative ideas (13 items, e.g., “I think I can come up with novel ideas”) and (b) self-beliefs about creative performance (11 items, e.g., “When I encounter a difficult problem, I feel I can solve it creatively”). All items were rated on a five-point rating scale (1 = totally inapplicable to me to 5 = totally applicable to me). The scale was piloted after various revision iterations as outlined above.
2.3 Procedures
Egyptian administrative authorities, which are the national equivalent to the Institutional Review Board in the United States, were contacted to obtain permission for administering the instrument. Participants were informed that their participation was voluntary. After their consent, teaching assistants distributed the scale to participants in their classes. They were told briefly but clearly the purpose of the study and how to respond to the scale based on instructions provided. They completed the scale in 10–15 min. All ethical guidelines of research on human participants were followed prior and during the scale administration (see section 8 “Research and Publication” of the “Ethical Principles of Psychologists and Code of Conduct; American Psychological Association, 2017). Finally, responses were collected and later scored.
2.4 Data analysis
Prior to conducting data analyses, data were screened for extreme response patterns (i.e., most responses were selected in the upper or lower response category) or response sets (i.e., most responses under a specific response category). Accordingly, 23 participants (approximately 4%) were removed due to similar response patterns or response sets on all items, which is considered an indication of careless responding (Meade and Craig, 2012). Thus, the final sample size consisted of 579 participants, who provided complete responses to all items in the scale. Given the small percentage of problematic responses (i.e., <5%), removing them was not expected to bias obtained results.
To establish validity evidence based on internal structure of the measure, it has been recommended to fit rival plausible models to find which had best model-data fit (American Educational Research Association, American Psychological Association, and National Council on Measurement in Education, 2014). In addition, selecting the best fitting model should be consistent with the theoretical framework of the construct being assessed. Data were analyzed and reported consistent with the order of the research questions outlined above
2.4.1 Items response frequencies
Item response frequencies were examined to identify ceiling and floor effects prior to conducting data analyses, since such effects have been found to increase measurement error and lead to range restriction, which in turn has reduced variance and biased parameter estimates (Allen, 2017).
2.4.2 CCFA for ordinal data
To answer the first research question and assess the dimensionality of CSE or establish its validity evidence based on the scale internal structure, two competing models were fitted to the data using CCFA for ordinal data (D’Urso et al., 2022) with WLSMV estimator, which has been appropriate for estimating model parameters with ordinal data collected by means of rating scales (Beauducel and Herzberg, 2006). One- and two-factor generalized linear models were compared to find which had the best model-data fit, and consequently assess the scale dimensionality, as an important step for choosing the unidimensional vs. the multidimensional IRT framework.
2.4.2.1 Assessing model-data fit
In the present study, various goodness-of-fit indices were utilized to assess model-data fit including the scaled chi-square test statistic used with the WLSMV estimator to evaluate the overall model fit (Satorra and Bentler, 1994, 2010), the comparative fit index (CFI), Tucker-Lewis index (TLI), and root mean square error of approximation (RMSEA) with its 90% confidence intervals. The scaled chi-square test statistic measures the degree of discrepancy between the observed and the model-implied covariance matrices. It tests the null hypothesis that “there is no difference between the two matrices.” Unlike hypothesis testing in statistics, the researcher here prefers to retain the null hypothesis to conclude the non-significant difference between the two matrices and consequently accepts the hypothesized model (i.e., accept-support test). However, it is well documented in the structural equation modeling literature that the chi-square test statistic is sensitive to sample size, since the power of the test increases as sample size increases (e.g., Kline, 2011). For instance, in case of a large sample size, the test may become significant leading to rejection of the null hypothesis even in case of a minor discrepancy between the observed and the model-implied covariance matrices.
The CFI and TLI are incremental or comparative fit indices that assess the relative improvement in the fit of the hypothesized model compared to the null or baseline model (i.e., the model without correlations or the worst fitting model). Values ≥ 0.90 for both CFI and TLI indicate good fit (Hoe, 2008; Finch and French, 2015). On the other hand, the RMSEA is an absolute fit index that measures the discrepancy between the hypothesized model, with optimally chosen parameter estimates, and the population covariance matrix. Kline (2011) provided the following criteria for using RMSEA for model fit: RMSEA ≤ 0.05 (good fit), 0.05 < RMSEA ≤ 0.08 (adequate fit), and RMSEA > 0.08 is poor. Researchers have recommended reporting both absolute and comparative fit indices (see Brown, 2015).
2.4.2.2 Comparing nested models
When comparing nested models, additional statistical tests such as the chi-square difference test (χ2diff.) should be used. It is important to highlight that the “DIFFTEST” option available in Mplus (Muthén and Muthén, 1998) should be used for chi-square difference testing when using the WLSMV estimator, with degrees of freedom equals the difference in the number of parameters estimated between the two models. This is an important procedure, since the typically obtained chi-square value cannot be used for chi-square difference testing in the regular way and “cannot generally be interpreted as a statistic that tests the equal-fit hypothesis (Kline, 2011, p. 216).”
From a descriptive perspective, the Akaike information criterion (AIC) and Bayesian information criterion (BIC) can be used to compare nested models fitted by maximum likelihood, since the AIC and BIC cannot be obtained when the WLMSV estimator is utilized. Note that the unidimensional and two dimensional models were refitted by maximum likelihood to obtain AIC and BIC as other pieces of evidence supporting the model that fits the data better. AIC is used as an efficiency criterion (i.e., favors the most parsimonious model), whereas BIC is viewed as a consistency criterion, which increases the probability of selecting the correct model as sample size increases (Yang, 2005). Overall, the smaller the value of both AIC and BIC, the better the fit. Burnham and Anderson (2002, p. 70) provided some useful rules of thumbs for comparing nested models based on the change in AIC (∆AIC): (a) models with 0–2 points have substantial support, (b) models falling in 4–7 points range have less support, and (c) models having >10 points difference have typically no support. AIC weights were also recommended, which can be directly interpreted as conditional probabilities for each model to quantify uncertainty in model selection (Burnham and Anderson, 2002, 2004; Wagenmakers and Farrell, 2004). Put simply, a weighted AIC value can be interpreted as the probability that a specific model is the best, given the data and a set of candidate models. Thus, probabilities for all compared models should sum to one. In addition, the relative fit of nested models, fitted to the same data, can be descriptively compared by each model’s set of fit statistics such as RMSEA (Kline, 2011).
2.4.3 IRT analysis
Given its advantages over the classical test theory, IRT is recommended when developing new assessments, since it is a sample- and test-invariant framework, meaning persons’ ability estimates are independent of the set of items they respond to, and items’ properties are independent from the sample used in calibration, if model assumptions hold (de Ayala, 2009; Bandalos, 2018). The reason underlying the use of IRT in addition to CCFA was to ensure the stability of the final selected model to represent CSE regardless of the psychometric model used. Most importantly, CFA and IRT have been recommended as complementary approaches for scale development and validation (for more information, see Bean and Bowen, 2021).
To answer the second research question, two rival polytomous IRT models were fitted to the data: (a) a two-dimensional RSM and (b) a two-dimensional GRM. Samejima (1969) introduced the GRM as a two-parameter model for polytomous data (i.e., partial credit or rating scale), where the discrimination parameter is allowed to vary across items, which may help researchers pick items with higher discrimination (a measurement perspective). The step parameters are also allowed to vary across items. In addition, the GRM model has commonly been used with rating scale data (Ferero and Maydeu-Olivares, 2009). Andrich (1978) introduced the RSM, as a Rasch framework, for polytomous ordinal data to be used with instruments that had the same number of response categories across all items. In the RSM, the discrimination parameter is fixed to one and step parameters are also fixed to be equal across all items. To fit both models, IRTPRO software was utilized (Cai et al., 2011). In addition, RMSEA values were obtained using the MIRT package in R (Chalmers, 2012). The overall evaluation of model-data fit was assessed by AIC, BIC, and RMSEA as described above. On the other hand, item fit was assessed by the item level diagnostic statistics (Kang and Chen, 2008), with a null hypothesis “item data fits the model,” which should be retained to conclude that the item fits the model and consequently is appropriate and informative for the construct being assessed. Regarding item discrimination, de Ayala (2009) argued “reasonably good values of α range from approximately 0.8 to about 2.5” (p. 101).
2.4.4 Categorical omega and IRT-based marginal reliability of response pattern scores
When estimating observed score reliability based on factor analytic models, a non-linear approach has been recommended, as it has been more robust for ordinal data (Green and Yang, 2009). One of the most highly recommended score reliability coefficients with ordinal data, but unfortunately rarely used in practice compared to alpha, has been categorical omega, which can be computed using the MBESS package in R (Kelley, 2007). Omega is an index of the proportion of variance in observed scores attributable to the model or the latent construct being measured. A major distinction between score reliability estimation based on factor analytic models and IRT is that the former is an index of the amount of random error in raw scores, whereas the latter is associated with score precision across the scale score. Put simply, score reliability does not theoretically exist in IRT, since score precision is a function of item information, conditional on theta, and is assessed by the conditional standard error of measurement. With that said, reliability changes across the score scale in IRT, given the test information function.
3 Results
In the following sub-sections, results are reported as outlined above in the “Data Analysis” section.
3.1 Item response frequencies
Percentages of item response frequencies were obtained as shown in Table 1. As emphasized, it has been useful to screen item response frequencies for identifying floor and ceiling effects. Based on the frequencies presented in Table 1, no clear evidence of either the ceiling or floor effect was observed.
3.2 CCFA for ordinal data
Table 2 shows goodness-of-fit indices and model comparison for the two competing unidimensional and two-dimensional CSE models.
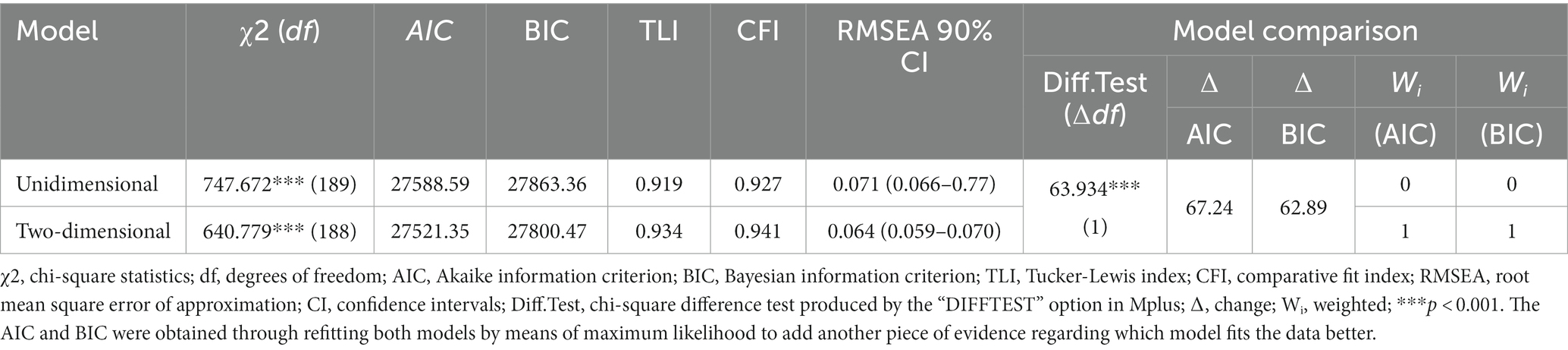
Table 2. Goodness-of-fit indices and model comparison for the unidimensional and two-dimensional CSE models (N = 579).
As shown in Table 2, chi-square tests for both models were significant. However, such significant chi-square test statistic should not solely drive the decision making process for accepting/rejecting hypothesized models, given its sensitivity to the large sample size as illustrated in the Data Analysis section. Compared to the unidimensional model, the two-dimensional model had lower AIC, BIC, and RMSEA, and higher CFI and TLI values, indicating its better fit to the data. Additionally, the two models were compared descriptively and inferentially, given the unidimensional model was nested in the two-dimensional model. For the former, changes in AIC and BIC values were, respectively, 67.24 and 62.89 exceeding 10, favoring the two-dimensional model. Additionally, the weighted AIC and BIC values were one for the two-dimensional model, meaning that the probability of the two-dimensional model to best fit the data is one, whereas zero for its unidimensional counterpart. Inferentially, the chi-square difference test statistic was significant, χ2(1) = 63.934, p < 0.001, meaning that the two-dimensional model had statistically better fit than its unidimensional counterpart. Collectively, descriptive and inferential sources of evidence favored the two dimensional model, or more technically, there was almost no inferential or descriptive support for the unidimensional model. The factor correlation of the two-dimensional model was 0.471, a relatively medium correlation indicating two distinct and consequently meaningful factors. Consistent with the theory underlying the scale development, the two factors were named “creative ideas self-efficacy (CISE)” and “creative performance self-efficacy (CPSE).”
Table 3 contains standardized item loadings with their associated standard error and test statistic for the unidimensional and two-dimensional models.
As shown in Table 3, the magnitude of standardized loadings for the two-dimensional model were all relatively larger than those for the unidimensional model, which likely aligned with the previous conclusion related to superiority of the two-dimensional model. It is worth noting that three items (8, 20, 23) had low standardized factor loadings (0.20, 0.158, 0.252). These items also had low discrimination based on the two-dimensional GRM as will be illustrated in more detail below.
3.3 IRT analysis
To answer the second research question, two rival polytomous IRT models were fitted to the data: (a) a two-dimensional RSM and (b) a two-dimensional GRM. Based on the two-dimensional GRM results, items 8, 20, and 23 had low discrimination parameters (α < 0.8), which was not consistent with the recommended guidelines and consequently were eliminated. The same items did not have high standardized loadings based on CCFA results as illustrated earlier. This suggested that the removal of the three items did not depend solely on one model fitted to the data. Stated differently, using multiple pieces of evidence to decide when to remove items increases the accuracy and appropriateness of the decision.
Table 4 contains the −2log likelihood, AIC, BIC, RMSEA, change in AIC and BIC, and number of parameters estimated for the two rival fitted models.
As shown in Table 4, AIC, BIC, and RMSEA values were smaller for the two-dimensional GRM. Specifically, RMSEA value for the two-dimensional GRM indicated good fit. Additionally, the change in AIC and BIC values were greater than 10. Taken together, the two-dimensional GRM had better model-data fit compared to the two-dimensional RSM.
From a measurement perspective, the two-dimensional GRM had good discrimination parameters ranging from 0.85 to 2.08 as shown in Table 5. Based on item diagnostic statistics, the null hypothesis was not retained for three items in case of the two-dimensional GRM, indicating that item fit to the model or lack of local misfit for most CSE items (see Table 5). On the contrary, the null hypothesis was rejected for nine items in the two-dimensional RSM indicating the nine items did not fit the model (see Appendix A). To sum up, the two-dimensional GRM had the best model-data fit both descriptively and psychometrically, and consequently it is the adopted model between the two competing IRT models. To conclude, results of CCFA and IRT had better fit for the CSE scale two-dimensional factor model as hypothesized. Appendix B has the final 21-item version of the CSE scale.
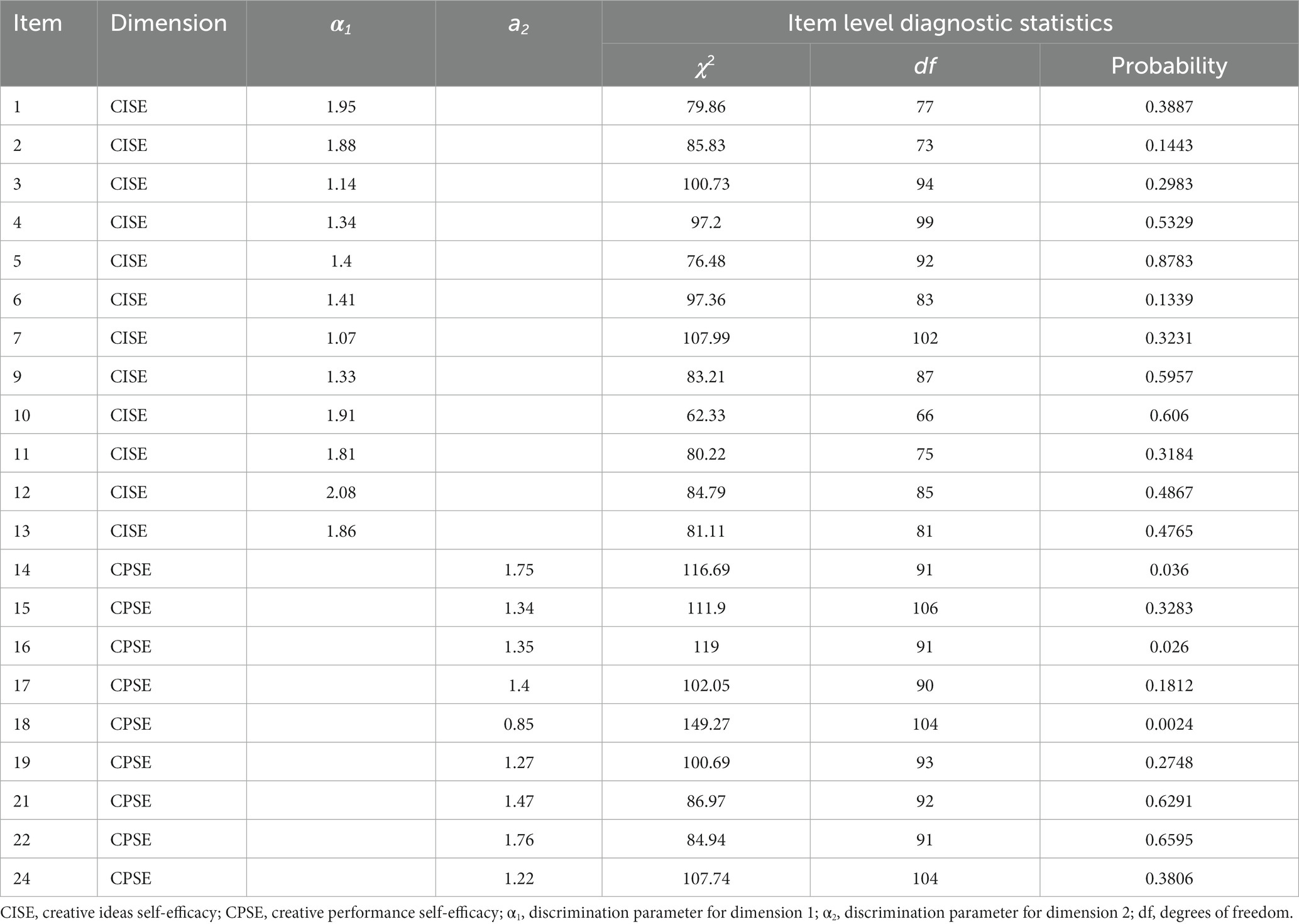
Table 5. Discrimination parameters and item level diagnostic statistics for the two-dimensional GRM model (N = 579).
3.4 Categorical omega and marginal reliability of response pattern scores
After results of both CCFA and IRT provided empirical evidence that the two-dimensional model had the best model-data fit compared to its unidimensional counterpart, score reliability was estimated based on the two analytic frameworks. For the former, categorical omega coefficients were 0.876 and 0.810 for the first and second dimensions, respectively. This indicated, respectively, that approximately 88% and 81% of the observed-score variance in undergraduates’ self-beliefs about their creative ideas and creative performance was true-score variance. Second, marginal reliabilities of response pattern scores, based on the two-dimensional GRM, were also 0.88 and 0.81 for the first and second dimensions, respectively. The similarity of score reliability estimates based on factor analytic and IRT models supported the consistency of score reliability estimates across the two psychometric models.
4 Discussion
CSE is one of the most recent concepts of domain-specific self-efficacy. Since Tierney and Farmer (2002) have introduced CSE, it has received much attention in various fields such as business, education, management, and psychology. Previous research has also provided strong empirical evidence that CSE has been an antecedent to creative performance. Given its significance, various researchers have attempted to develop scales for measuring individuals’ CSE, but some have engaged in limited validation studies, as detailed throughout the manuscript. Furthermore, most of the reviewed measures had some methodological flaws that might invalidate score-based inferences.
Overall, the main objectives of this study were to develop a CSE scale and collect its validity evidence based on scale content, response processes, and internal structure as well as estimate its score reliability using categorical omega and IRT-based marginal reliability. Specifically, the present study objectives were three-fold. First, assessing CSE dimensionality was a major objective, since there was a debate about its underlying factor structure. The present study utilized an advanced psychometric model such as CCFA with ordinal data to assess CSE dimensionality. Utilizing appropriate psychometric models with robust estimators for ordinal data yielded unbiased estimates and consequently valid scores interpretation (DiStefano et al., 2019). CCFA results based on descriptive and inferential evidence supported the two-dimensional model compared to its unidimensional counterpart, which were consistent with previous studies (Abbott, 2010; Tan et al., 2011; Yu, 2013a,b; Alotaib, 2016; Hung, 2018).
Second, two competing polytomous IRT models were fitted to the data: (a) a two-dimensional RSM and (b) a two-dimensional GRM. Results supported the two-dimensional GRM. These results were in agreement with Hung (2018) who used the RSM and concluded that CSE is a multidimensional construct. The two-dimensional CSE factor structure was also consistent with the theoretical framework according to which the scale was developed in the present study. To conclude, evidence from both CCFA and IRT supported the two-dimensional factor structure of the CSE scale. However, there should be some caution in interpreting a multidimensional factor structure underlying an educational or psychological construct. This is of a particular concern if the factors were correlated, which is mostly the case with educational and psychological variables. In saying that, interpretation of the two-dimensional factor structure of CSE relies heavily on its underlying conceptual model and goes beyond merely the statistical evidence. Put simply, when interpreting a factor structure of a measurement instrument, the conceptual or theoretical framework as well as intended score interpretations should be heavily considered, compared to relying only on the statistical evidence in selecting a specific model. When results obtained from empirical data align with the conceptual model or theory used to develop a measurement instrument, this validity evidence supports intended score interpretations for proposed uses (American Educational Research Association, American Psychological Association, and National Council on Measurement in Education, 2014).
Third, score reliability was estimated in the present study by means of categorical omega based on the two-dimensional CCFA model and marginal reliability of response pattern scores using the two-dimensional GRM. The two analytic approaches produced approximately similar estimates for the first and second dimensions, which provided empirical evidence about the amount of random error and score precision across scale scores, respectively. Specifically, using a score reliability estimation method such as categorical omega is appropriate for obtaining unbiased score reliability estimates for dimensions composed of rating scale items, which increases the utility of the scale (Kelley and Pornprasertmanit, 2016).
In the present study, the necessity of establishing internal structure of measurement instruments was emphasized prior to collecting validity evidence based on relations with other variables. Benson (1998) noted that validity evidence based on relations to other variables should come after testing the internal structure of the instrument, since it is not reasonable to investigate whether the construct is associated with external variables of interest, if it is not internally consistent. To summarize, an example of developing and initially validating the intended interpretations of a CSE scale for undergraduates was provided by collecting and reporting three sources of validity evidence: (a) validity evidence based on scale content, (b) response processes, and (c) internal structure. Score reliability was also established by more robust methods (e.g., categorical omega and IRT-based marginal reliability) than those used in previous measures contributing to the methodological rigor of the present study compared to previously published studies. To conclude, the overall objective was to overcome the methodological and psychometric limitations noted through the literature review by developing a two-dimensional scale for measuring undergraduates’ CSE, and initially validating its intended score interpretations using advanced psychometric models. Results supported the hypothesized two-dimensional factor structure with relatively high score reliability estimates.
4.1 Educational implications
The CSE scale utility also stems from the importance of developing individuals’ creative abilities in various domains. Thus, the current scale can be used in some contexts in higher education institutions. For instance, creativity programs can utilize the scale as an index for assessing undergraduates’ beliefs about their ability to generate creative ideas and perform creatively. An individual’s CSE is composed of self-beliefs about the ability to generate creative ideas and perform creatively (Beghetto et al., 2011).
Given the validity evidence collected and reported in the present study, undergraduates with higher levels of CSE have strong beliefs in their abilities to think and perform creatively. To that end, the scale can be used for diagnostic purposes to identify undergraduates with low beliefs about their abilities to think and perform creatively. Undergraduates with low CSE profiles may be more likely to suffer in study tasks that require them to think of themselves as creative thinkers and performers. Intervention programs can accordingly be planned and implemented for undergraduates with low CSE profiles in terms of self-beliefs about creative thinking and performance. Such intervention programs may include educational activities (e.g., I can be a creative person) that help undergraduates have positive beliefs about their abilities to think and perform creatively. The potential consequences of intervening may include improving undergraduates’ CSE beliefs associated with generating creative ideas and performing creatively. On the other hand, the potential consequences of not intervening may include low creative performance by those who have low CSE profiles, since CSE is an antecedent to creative performance.
4.2 Limitations and future research directions
Although three major research questions were addressed to fill the gap identified through a rigorous literature review, there were some limitations. First, participants were undergraduates, which likely limits generalizability of results beyond this population. According to the American Society for Cell Biology (2015, as cited in Jitendra et al., 2019), there are three types of replication studies: (a) analytic, (b) direct, and (c) systematic. Most importantly in the context of the present study is direct replication studies, where other researchers replicate the study with its methodology but with samples from different populations. Put differently, there is a substantial need to cross-validate the current CSE scale among other populations.
Given the importance of CSE in creative performance in various domains, much research needs to be conducted to collect other sources of validity evidence. There is still a need to collect validity evidence based on relations with other variables to better understand how the two dimensions are associated with concurrent (convergent and discriminant) and predictive evidence. Additionally, validity evidence based on consequences of testing needs to be collected and reported when the scale is used in high-stakes creativity programs. Additionally, testing measurement invariance is recommend to ascertain that the scale functions equally across subgroups (e.g., males/females), which is a critical procedure for drawing valid mean score comparisons (Putnick and Bornstein, 2016; Abulela and Davenport, 2020). In short, researchers can still use the scale for other purposes conditioned on collecting sources of validity evidence needed for the new score interpretations and uses.
Data availability statement
The datasets presented in this article are not readily available because consent for sharing was not obtained from the participants. Queries regarding the datasets may be directed to the author.
Ethics statement
The studies involving humans were approved by IRB/South Valley University, Egypt. The studies were conducted in accordance with the local legislation and institutional requirements. A written consent from participants was not needed, since there were no individual risks associated with participation in the study.
Author contributions
MA: Conceptualization, Formal analysis, Investigation, Methodology, Software, Validation, Writing – original draft, Writing – review & editing.
Funding
The author(s) declare that no financial support was received for the research, authorship, and/or publication of this article.
Acknowledgments
The author would like to deeply thank Michael C. Rodriguez, Mark L. Davison, and Michael R. Harwell, Professors of Educational Measurement, University of Minnesota, for their thoughtful comments on an earlier draft of the manuscript. I would like also to deeply thank Rabea A. A. Rashwan, Professor of Educational Psychology, Qassim University, as well as other subject matter experts, for providing feedback on an Arabic version of the scale. Last, my a special thank-you goes to Amaniel P. Mrutu, a former graduate student at the University of Minnesota, for providing critical feedback on an earlier draft of the manuscript. Last, my deep thank-you for the 18 undergraduate students who participated in the cognitive interview conducted to collect validity evidence based on response processes. Relatedly, the author would like to deeply thank undergraduate students who voluntarily participated in the study.
Conflict of interest
The author declares that the research was conducted in the absence of any commercial or financial relationships that could be construed as a potential conflict of interest.
Publisher’s note
All claims expressed in this article are solely those of the authors and do not necessarily represent those of their affiliated organizations, or those of the publisher, the editors and the reviewers. Any product that may be evaluated in this article, or claim that may be made by its manufacturer, is not guaranteed or endorsed by the publisher.
Supplementary material
The Supplementary material for this article can be found online at: https://www.frontiersin.org/articles/10.3389/feduc.2023.1306532/full#supplementary-material
References
Abbott, D. H. (2010). Constructing creative self-efficacy inventory: A mixed methods inquiry, unpublished doctoral dissertation. University of Nebraska, Lincoln.
Abulela, M. A. A., and Davenport, E. C. (2020). Measurement invariance of the learning and study strategies inventory-(LASSI-II) across gender and discipline in Egyptian college students. Educ. Sci.: Theory Pract. 20, 32–49. doi: 10.12738/jestp.2020.2.003
Allen, M. (2017). “Errors of measurement: ceiling and floor effects” in The sage encyclopedia of communication research methods. ed. M. Allen. Thousand Oaks, CA: Sage.
Alotaib, K. N. (2016). Psychometric properties of creative self-efficacy inventory among distinguished students in Saudi Arabian universities. Psychol. Rep. 118, 902–917. doi: 10.1177/0033294116646021
Alzoubi, A. M., Alqudah, M. F., Albursan, I. S., Bakhiet, S. F., and Abduljabbar, A. S. (2016). The effect of creative thinking education in enhancing creative self-efficacy and cognitive motivation. Educ. Dev. Psychol. 6, 117–130. doi: 10.5539/jedp.v6n1p117
American Educational Research Association, American Psychological Association, and National Council on Measurement in Education (2014). Standards for educational and psychological testing. Washington, DC: American Educational Research Association.
American Psychological Association. (2017). Ethical principles of psychologists and code of conduct (2002, amended effective June 1, 2010, and January 1, 2017). Available at: https://www.apa.org/ethics/code/
Andrich, D. (1978). A rating formulation for ordered response categories. Psychometrika 43, 561–573. doi: 10.1007/BF02293814
Aqdas, R., Bilal, A., Abbas, A., and Zirwa, F. (2016). Impact of resistance to change and creative self-efficacy on enhancing creative performance. GBSE. 2, 150–161.
Bandalos, D. L. (2018). Measurement theory and applications for the social sciences. New York, NY: The Guilford Press.
Bandura, A. (1977). Self-efficacy: toward a unifying theory of behavioral change. Psychol. Rev. 84, 191–215. doi: 10.1037/0033-295X.84.2.191
Bandura, A. (2000). Exercise of human agency through collective efficacy. Curr. Dir. Psychol. Sci. 9, 75–78. doi: 10.1111/1467-8721.00064
Bean, G. J., and Bowen, N. K. (2021). Item response theory and confirmatory factor analysis: complementary approaches for scale development. J. Evid. Based Soc. Work 18, 597–618. doi: 10.1080/26408066.2021.1906813
Beauducel, A., and Herzberg, P. Y. (2006). On the performance of maximum likelihood versus means and variance adjusted weighted least squares estimation in CFA. Struct. Equ. Modeling 13, 186–203. doi: 10.1207/s15328007sem1302_2
Beghetto, R. A. (2006). Creative self-efficacy: correlates in middle and secondary students. Creat. Res. J. 18, 447–457. doi: 10.1207/s15326934crj1804_4
Beghetto, R. A., Kaufman, J. C., and Baxter, J. (2011). Answering the unexpected questions: exploring the relationship between students’ creative self-efficacy and teacher ratings of creativity. Psychol. Aesthet. Creat. Arts 5, 342–349. doi: 10.1037/a0022834
Benson, J. (1998). Developing a strong program of construct validation: a test anxiety example. Educ. Meas. 17, 10–17. doi: 10.1111/j.1745-3992.1998.tb00616.x
Bereczki, E. O., and Kárpáti, A. (2018). Teachers’ beliefs about creativity and its nurture: a systematic review of the recent research literature. Educ. Res. Rev. 23, 25–56. doi: 10.1016/j.edurev.2017.10.003
Betz, N. E., and Hackett, G. (1983). The relationship of mathematics self-efficacy expectations to the selection of science-based college majors. J. Vocat. Behav. 23, 329–345. doi: 10.1016/0001-8791(83)90046-5
Brockhus, S., Kolk, T. E. C., Koeman, B., and Badk-Schaub, P. G. (2014). The influence of creative self-efficacy on creative performance [paper presentation]. In: The 13th International Design Conference, Dubrovnik, Croatia.
Brown, T. A. (2015). Confirmatory factor analysis for applied research. 2nd Edn New York, NY: The Guilford Press.
Burnham, K. P., and Anderson, D. R. (2002). Model selection and multimodel inference: A practical information –theoretic approach. 2nd Edn. Fort Collins, CO: Springer.
Burnham, K. P., and Anderson, D. R. (2004). Multimodel inference: understanding AIC and BIC in model selection. Sociol. Methods Res. 33, 261–304. doi: 10.1177/0049124104268644
Cai, L., Thissen, D., and du Toit, S. (2011). IRTPRO: Flexible, multidimensional, multiple categorical IRT modeling [computer software]. Skokie, IL: Scientific Software International, Inc.
Chalmers, R. P. (2012). Mirt: a multidimensional item response theory package for the R environment. J. Stat. Softw. 48, 1–29. doi: 10.18637/jss.v048.i06
Chang, J., and Yang, Y. (2012). The effect of organization’s innovational climate on student’s creative self-efficacy innovative behavior. Bus Entrepreneursh J 1, 1–5.
Cheng, L., Cui, Y., Chen, Q., Ye, Y., Liu, Y., Zhang, F., et al. (2020). Pediatric nurses’ general self-efficacy, perceived organizational support and perceived professional benefits from class A tertiary hospitals in Jilin province of China: the mediating effect of nursing practice environment. BMC Health Serv. Res. 20, 1–9. doi: 10.1186/s12913-019-4878-3
Choi, J. N. (2004). Individual contextual predictors of creative performance: the mediating role of psychological processes. Creat. Res. J. 16, 187–199. doi: 10.1080/10400419.2004.9651452
D’Urso, E. D., De Roover, K., Vermunt, J. K., and Tijmstra, J. (2022). Scale length does matter: recommendations for measurement invariance testing with categorical factor analysis and item response theory approaches. Behav. Res. Methods 54, 2114–2145. doi: 10.3758/s13428-021-01690-7
de Ayala, R. J. (2009). The theory and practice of item response theory. New York, NY: Guilford Press.
DiStefano, C., McDaniel, H. L., Zhang, L., Shi, D., and Jiang, Z. (2019). Fitting large factor analysis models with ordinal data. Educ. Psychol. Meas. 79, 417–436. doi: 10.1177/0013164418818242
Farmer, S. M., and Tierney, P. (2017). “Considering creative self-efficacy: its current state and ideas for future inquiry” in Explorations in creativity research. The creative self: Effect of beliefs, self-efficacy, mindset, and identity. eds. M. Karwowski and J. C. Kaufman (Elsevier Academic Press), 23–47. doi: 10.1016/B978-0-12-809790-8.00002-9
Ferero, C. G., and Maydeu-Olivares, A. (2009). Estimation of IRT graded response models: limited versus full information methods. Psychol. Methods 14, 275–299. doi: 10.1037/a0015825
Gist, M. E., Schwoerer, C., and Rosen, B. (1989). Effects of alternative training methods on self-efficacy and performance in computer software training. J. Appl. Psychol. 74, 884–891. doi: 10.1037/0021-9010.74.6.884
Gong, Y., Huang, J., and Farh, J. (2009). Employee learning orientation, transformational leadership, and employee creativity: the mediating role of employee self-efficacy. Acad. Manage. J. 52, 765–778. doi: 10.5465/amj.2009.43670890
Graham, J. M. (2006). Congeneric and (essentially) tau-equivalent estimates of score reliability: what they are and how to use them? Educ. Psychol. Meas. 66, 930–944. doi: 10.1177/0013164406288165
Green, S. B., and Yang, Y. (2009). Reliability of summed item scores using structural equation modeling: An alternative to coefficient alpha. Psychometrika 74, 155–167. doi: 10.1007/s11336-008-9099-3
Haase, J., Hoff, E. V., Hanel, P. H. P., and Innes-Ker, Å. (2018). A meta-analysis of the relation between creative self-efficacy and different creativity measurements. Creat. Res. J. 30, 1–16. doi: 10.1080/10400419.2018.1411436
Hoe, S. L. (2008). İssues and procedures in adopting structural equation modeling technique. J Appl Quant Methods 3, 76–83.
Huang, H.-Y., and Hung, S. P. (2009). A study of examining construct validity on the scale of creative self-efficacy for students through both linear and nonlinear approaches. J Pingtung Univer Educ 33, 489–514.
Hung, S. -P. (2018). Validating the creative self-efficacy student scale with a Taiwanese sample: An item response theory-based investigation. Think. Skills Creat. 27, 190–203. doi: 10.1016/j.tsc.2018.02.006
Jaussi, K. S., and Randel, A. E. (2014). Where to look? Creative self-efficacy, knowledge retrieval, and incremental radical creativity. Creat. Res. J. 26, 400–410. doi: 10.1080/10400419.2014.961772
Jitendra, A. K., Harwell, M. R., Im, S. H., Karl, S. R., and Slater, S. C. (2019). Improving student learning of ratio, proportion, and percent: a replication study of schema-based instruction. J. Educ. Psychol. 111, 1045–1062. doi: 10.1037/edu0000335
Kane, M. (2013). Validating the interpretations and uses of test scores [special issue]. J. Educ. Meas. 50, 1–73. doi: 10.1111/jedm.12000
Kang, T., and Chen, T. T. (2008). Performance of the generalized S-χ2 item fit index for polytomous IRT models. J. Educ. Meas. 45, 391–406. doi: 10.1111/j.1745-3984.2008.00071.x
Karwowski, M. (2011). It doesn’t hurt to ask. But sometimes it hurts to believe: polish students’ creative self-efficacy and its predictors. Psychol. Aesthet. Creat. Arts 5, 154–164. doi: 10.1037/a0021427
Karwowski, M. (2012). Did curiosity kill the cat? Relationship between trait curiosity, creative self-efficacy and creative personal identity. Eur. J. Psychol. 8, 547–558. doi: 10.5964/ejop.v8i4.513
Karwowski, M. (2014). Creative mindsets: measurement, correlates, consequences. Psychol. Aesthet. Creat. Arts 8, 62–70. doi: 10.1037/a0034898
Karwowski, M. (2016). The dynamics of creative self-concept: changes and reciprocal relations between creative self-efficacy and creative personal identity. Creat. Res. J. 28, 99–104. doi: 10.1080/10400419.2016.1125254
Karwowski, M., Lebuda, I., and Wiśniewska, E. (2018). Measuring creative self-efficacy and creative personal identity. Int J Creat Probl Solving 28, 45–57.
Karwowski, M., Lebuda, I., Wiśniewska, E., and Gralewski, J. (2013). Big five personality traits as the predictors of creative self-efficacy and creative personal identity: does gender matter? J. Creat. Behav. 47, 215–232. doi: 10.1002/jocb.32
Kaufman, J. C., and Beghetto, R. A. (2009). Beyond big and little: the four C model of creativity. Rev. Gen. Psychol. 13, 1–12. doi: 10.1037/a0013688
Kelley, K. (2007). Methods for the behavioral, educational, and social sciences: An R package. Behav. Res. Methods 39, 979–984. doi: 10.3758/BF03192993
Kelley, K., and Pornprasertmanit, S. (2016). Confidence intervals for population reliability coefficients: evaluation of methods, recommendations, and software for composite measures. Psychol. Methods 21, 69–92. doi: 10.1037/a0040086
Kirk, B. A., Schutte, N. S., and Hine, D. W. (2008). Development and preliminary validation of an emotional self-efficacy scale. Pers. Individ. Differ. 45, 432–436. doi: 10.1016/j.paid.2008.06.010
Kline, R. B. (2011). Principles and practice of structural equation modeling. 3rd Edn. New York, NY: Guilford Press.
Kong, H., Chiu, W. C., and Leung, H. K. (2019). Building creative self-efficacy via learning goal orientation, creativity job requirement, and team learning behavior: the key to employee creativity. Aust. J. Manag. 44, 443–461. doi: 10.1177/0312896218792957
Köseoğlu, Y. (2015). Self-efficacy and academic achievement – a case from Turkey. J. Educ. Pract. 6, 131–141.
Lemons, G. (2010). Bar drinks, rugas, and gay pride parades: is creative behavior a function of creative self-efficacy? Creat. Res. J. 22, 151–161. doi: 10.1080/10400419.2010.481502
Li, C. (2016). Confirmatory factor analysis with ordinal data: comparing robust maximum likelihood and diagonally weighted least squares. Behav. Res. Methods 48, 936–949. doi: 10.3758/s13428-015-0619-7
Liu, C., Lu, K., Wu, L. Y., and Tsai, C. (2016). The impact of peer review on creative self-efficacy and learning performance in web 2.0 learning activities. Educ. Technol. Soc. 19, 286–297.
Mathisen, G. E., and Bronnick, K. S. (2009). Creative self-efficacy: An intervention study. Nt. J. Educ. Res. 48, 21–29. doi: 10.1016/j.ijer.2009.02.009
McNeish, D., An, J., and Hancock, G. R. (2018). The thorny relation between measurement quality and fit index cutoffs in latent variable models. J. Pers. Assess. 100, 43–52. doi: 10.1080/00223891.2017.1281286
Meade, A. W., and Craig, S. B. (2012). Identifying careless responses in survey data. Psychol. Methods 17, 437–455. doi: 10.1037/a0028085
Michael, L. A., Hou, S. T., and Fan, H. (2011). Creative self-efficacy and innovative behavior in a service setting: optimism as a moderator. J. Creat. Behav. 45, 258–272. doi: 10.1002/j.2162-6057.2011.tb01430.x
Muthén, L. K., and Muthén, B. O. (1998). Mplus user’s guide. 8th Edn. Los Angeles, CA: Muthén & Muthén.
Padilla, J., and Benítez, I. (2014). Validity evidence based on response processes. Psicothema 26, 136–144. doi: 10.7334/psicothema2013.259
Padilla, J., and Leighton, J. P. (2017). “Cognitive interviewing and thinking aloud methods” in Understanding and investigating response processes in validation research. eds. B. D. Zumbo and A. M. Hubley. 211–228. New York, NY: Springer.
Pajares, F. (2009). “Toward a positive psychology of academic motivation: the role of self-efficacy beliefs” in Handbook of positive psychology in schools. eds. R. Gilman, E. S. Huebner, and M. J. Furlong. 149–160. New York, NY: Routledge.
Peterson, C. H., Peterson, N. A., and Powell, K. G. (2017). Cognitive interviewing for item development: validity evidence based on content and response processes. Meas. Eval. Couns. Dev. 50, 217–223. doi: 10.1080/07481756.2017.1339564
Puente-Diaz, R., and Cavazos-Arroyo, J. (2016). An exploration of some antecedents and consequences of creative self-efficacy among college students. J. Creat. Behav. 52, 256–266. doi: 10.1002/jocb.149
Putnick, D. L., and Bornstein, M. H. (2016). Measurement invariance conventions and reporting: the state of the art and future directions for psychological research. Dev. Rev. 41, 71–90. doi: 10.1016/j.dr.2016.06.004
Putwain, D. W., Kearsley, R., and Symes, W. (2012). Do creativity self-beliefs predict literacy achievement and motivation? Learn. Individ. Differ. 22, 370–374. doi: 10.1016/j.lindif.2011.12.001
Richter, A. W., Hirst, G., Knippenberg, D., and Baer, M. (2012). Creative self-efficacy and individual creativity in team contexts: cross-level interactions with team informational resources. J. Appl. Psychol. 97, 1282–1290. doi: 10.1037/a0029359
Samejima, F. (1969). Estimation of latent ability using a response pattern of graded scores. Psychometrika Monogr Suppl 34:100.
Sangsuk, P., and Siriparp, T. (2015). Confirmatory factor analysis of a scale measuring creative self-efficacy of undergraduate students. Procedia. Soc. Behav. Sci. 171, 1340–1344. doi: 10.1016/j.sbspro2015.01.251
Sanna, L. J. (1992). Self-efficacy theory: implications for social facilitation and social loafing. Pers. Soc. Psychol. 62, 774–786. doi: 10.1037/0022-3514.62.5.774
Satorra, A., and Bentler, P. M. (1994). Corrections to test statistics and standard errors in covariance structure analysis. In A. EyeVon and C. C. Clogg (Eds.), Latent variables analysis: Applications for developmental research (pp. 399–419). Thousand Oaks, CA: Sage.
Satorra, A., and Bentler, P. M. (2010). Ensuring positiveness of the scaled difference chi-square test statistic. Psychometrika 75, 243–248. doi: 10.1007/s11336-009-9135-y
Schack, G. D. (1989). Self-efficacy as a mediator in the creative productivity of gifted children. J. Educ. Gift. 12, 231–249. doi: 10.1177/016235328901200306
Schunk, D. H., and Pajares, F. (2002). “The development of academic self-efficacy” in A Vol. in the educational psychology series. Development of achievement motivation. eds. A. Wigfield and J. S. Eccles. 15–31. San Diego, CA: Academic Press.
Shaw, A., Kapnek, M., and Morelli, N. A. (2021). Measuring creative self-efficacy: An item response theory analysis of the creative self-efficacy scale. Front. Psychol. 12. doi: 10.3389/fpsyg.2021.678033
Simmons, A. L., Payne, S. C., and Pariyothorn, M. M. (2014). The role of means efficacy when predicting creative performance. Creat. Res. J. 26, 53–61. doi: 10.1080/10400419.2014.873667
Sireci, S. G. (1998). The construct of content validity. Soc. Indic. Res. 45, 83–117. doi: 10.1023/A:1006985528729
Sireci, S., and Faulkner-Bond, M. (2014). Validity evidence based on test content. Psicothema 26, 100–107. doi: 10.7334/psicothema2013.256
Tan, A., Ho, V., Ho, E., and Ow, S. (2008). High school students’ perceived creativity self-efficacy and emotions in a service learning context. Int J Creat Probl Solv 18, 115–126.
Tan, A., Ho, V., and Yong, L. (2007). Singapore high school students’ creativity efficacy. New Horizons Educ 55, 96–106.
Tan, A., Li, J., and Rotgans, J. (2011). Creativity self-efficacy scale as a predictor for classroom behavior in a Chinese student context. Open Educ J 4, 90–94. doi: 10.2174/1874920801104010090
Tierney, P., and Farmer, S. M. (2002). Creative self-efficacy: its potential antecedents and relationship to creative performance. Acad. Manage. J. 45, 1137–1148. doi: 10.2307/3069429
Tierney, P., and Farmer, S. M. (2011). Creative self-efficacy development and creative performance over time. J. Appl. Psychol. 96, 277–293. doi: 10.1037/a0020952
Vally, Z., Salloum, L., AlQedra, D., El Shazly, S., Albloshi, M., Alsheraifi, S., et al. (2019). Examining the effects of creativity training on creative production, creative self-efficacy, and neuro-executive functioning. Think. Skills Creat. 31, 70–78. doi: 10.1016/j.tsc.2018.11.003
Wagenmakers, E. J., and Farrell, S. (2004). AIC model selection using Akaike weights. Psychon. Bull. Rev. 11, 192–196. doi: 10.3758/BF03206482
Wang, C., Tsai, H., and Tsai, M. (2014). Linking transformational leadership and employee creativity in the hospitality industry: the influences of creative role identity, creative self-efficacy, and job complexity. Tour. Manag. 40, 79–89. doi: 10.1016/j.tourman.2013.05.008
Wilde, N., and Hsu, A. (2019). The influence of general self-efficacy on the interpretation of vicarious experience information within online learning. Int. J. Educ. Technol. 16, 1–20. doi: 10.1186/s41239-019-0158-x
Willis, G. B. (2015). Analysis of the cognitive interview in questionnaire design. Oxford: Oxford University Press.
Yang, Y. (2005). Can the strengths of AIC and BIC be shared? A conflict between model indentification and regression estimation. Biometrika 92, 937–950. doi: 10.1093/biomet/92.4.937
Yang, H., and Cheng, H. (2009). Creative self-efficacy and its factors: An empirical study of information system analyst’s programmers. Comput. Hum. Behav. 25, 429–438. doi: 10.1016/j.chb.2008.10.005
Yang, Y., Xu, X., Liu, W., and Pang, W. (2020). Hope and creative self-efficacy as sequential mediators in the relationship between family socioeconomic status and creativity. Front. Psychol. 11:438. doi: 10.3389/fpsyg.2020.00438
Yu, C. (2013a). The relationship between undergraduate students’ creative self-efficacy, creative ability and career self-management. Int. J. Acad. Res. Progress. Educ. Dev. 2, 181–193.
Yu, C. (2013b). An empirical examination of a four-component of creative self-efficacy among undergraduate students. J. Appl. Sci. 13, 4092–4095. doi: 10.3923/jas.2013.4092.4095
Keywords: self-efficacy, creative self-efficacy, validity evidence, categorical confirmatory factor analysis, item response theory
Citation: Abulela MAA (2024) Development and initial validation of a creative self-efficacy scale for undergraduates: categorical confirmatory factor analysis and multidimensional item response theory. Front. Educ. 8:1306532. doi: 10.3389/feduc.2023.1306532
Edited by:
Yi-Hsin Chen, University of South Florida, United StatesReviewed by:
Claudio Bustos, University of Concepcion, ChileAmery D. Wu, University of British Columbia, Canada
Copyright © 2024 Abulela. This is an open-access article distributed under the terms of the Creative Commons Attribution License (CC BY). The use, distribution or reproduction in other forums is permitted, provided the original author(s) and the copyright owner(s) are credited and that the original publication in this journal is cited, in accordance with accepted academic practice. No use, distribution or reproduction is permitted which does not comply with these terms.
*Correspondence: Mohammed A. A. Abulela, bWhhZHkwMDFAdW1uLmVkdQ==
†ORCID: Mohammed A. A. Abulela, https://orcid.org/0000-0002-5397-8817