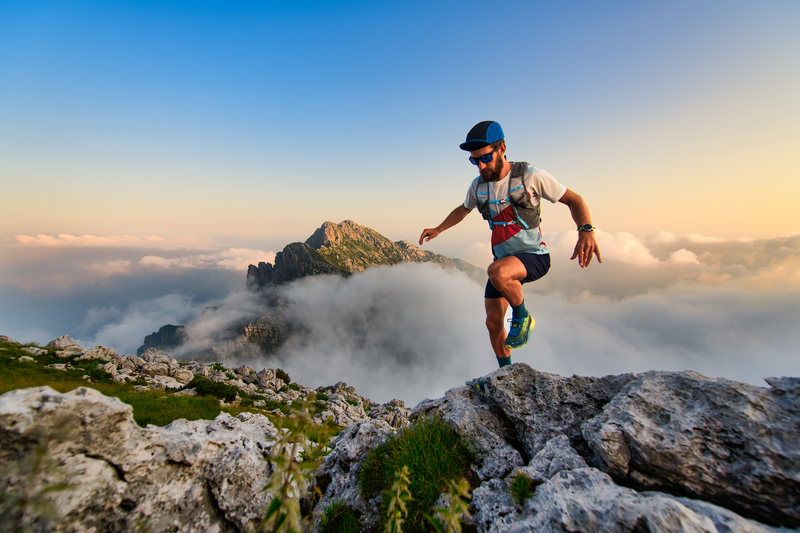
94% of researchers rate our articles as excellent or good
Learn more about the work of our research integrity team to safeguard the quality of each article we publish.
Find out more
ORIGINAL RESEARCH article
Front. Educ. , 08 January 2024
Sec. Digital Education
Volume 8 - 2023 | https://doi.org/10.3389/feduc.2023.1302701
Although electronic reading of fiction has become mainstream, little is known about how electronic texts are read, and whether this behavior is connected to readers’ motivation or experience reading electronically. To address this gap, sixty undergraduate students’ reading behavior was tracked while reading a 15-page short story. A novel method was used to study participants’ frequency of task-switching, their reading speed, and navigational patterns unobtrusively, outside of the lab. Reading behavior was analyzed by two multilevel models to assess (1) whether variance in behavior could be predicted by the task context, such as location in text or timing of reading sessions, and (2) whether behavior was connected to participants’ situational motivation to read the short story, their contextual motivation toward reading as an activity, or their task-relevant electronic experience. Our results showed that highly experienced and avid readers reacted to text difficulty more adaptively, indicating that motivation and electronic experience may have a key role in supporting comprehension. In contrast, situational motivation was not associated with reading behavior, contrary to our expectations. These findings provide a significant contribution to our understanding of e-reading, which can be used as a foundation to support recreational reading engagement on digital devices.
Frequent recreational reading has been recognized as an important predictor of reading ability (Mol and Bus, 2011; McGeown et al., 2015; Torppa et al., 2020). In particular, reading of narrative, fictional texts such as novels is key for strong reading skills (Schiefele et al., 2012). Despite its importance, many adults do not read for pleasure (Department for Culture, Media, and Sport, 2015; Gelles-Watnick and Perrin, 2021).
To address barriers to reading, it is important to understand reading engagement within the context of adults’ daily lives. Readers do not only differ in how frequently they engage in reading, but for how long, in what conditions they read, and how they navigate a text (Everatt et al., 1998; Merga, 2017). Reading behavior is likely to be connected to the readers’ motivation toward a text and reading as an activity, considering that motivation has been found to play a key role in reading engagement (Guthrie and Wigfield, 2000).
Furthermore, technological advancements have influenced our reading behavior. A total of 30% of adults now read electronic books (Faverio and Perrin, 2022), most often on a multipurpose device, such as a smartphone or a tablet (Anderson, 2015; Maloney, 2015). Considering that most adults own a smartphone (Atske and Perrin, 2021; Lee and Stanton, 2022), they can read electronically wherever they go (D’Ambra et al., 2019). However, previous research has shown that reading on these devices is distractible and fragmented (Mangen et al., 2019; Baron and Mangen, 2021), potentially due to the lack of sufficient task-relevant experience in reading electronically (Yoo and Roh, 2019). Enhancing our understanding of how adults read electronically, and whether behavior is connected to task-relevant experience or motivation can help us support adults in unlocking the positive potential of reading on digital devices.
Previous research has been hampered by limited methodologies. Whereas lab-based approaches fail to capture natural reading behavior (Kingstone et al., 2008; Mckay et al., 2021), self-reports can be biased by retrospective recall and the tendency to report socially desirable behavior (Smith and Stahl, 1999). The current study uses a novel method to address these limitations. Reading behavior was tracked outside of the lab using a bespoke e-reader system. The e-reader was designed to imitate popular e-book reading applications that work on mainstream digital devices, such as smartphones, tablets, and laptops. Embedded tracking functions allowed us to unobtrusively collect rich information about the participants’ e-reading behavior over 14 days, without bias from self-reports. The goal was not to study or compare e-reading of narrative texts to their print equivalents or to web reading. Instead, we sought to study the connection between observable reading behavior, motivation, and task-specific electronic reading experience, without comparison between reading formats.
Motivation drives engagement in effortful activities such as reading (Schiefele et al., 2012). According to one of the most dominant theories of motivation, the self-determination theory (SDT) by Deci and Ryan (2000), readers’ motivation can be divided into “autonomous” and “controlled” types, or the complete lack of motivation – “amotivation” (see Figure 1). Autonomous motivation describes voluntary engagement in an activity that an individual finds interesting, and so they may pick up a book because they identify themselves as a “reader,” because they gain something valuable from reading, or simply because they enjoy it as an activity (Levine et al., 2022). In contrast, controlled motivation is driven by an external force or attempts to align with social pressure, for example in the case of required reading done for school or reading of socially acclaimed texts to avoid shame (Deci and Ryan, 2008a). This distinction is important as only autonomous motivation has been connected to sustained, positive outcomes of reading (De Naeghel et al., 2012; Howard et al., 2021). Indeed, autonomously motivated readers read more frequently and in higher volume than their peers (De Naeghel et al., 2012; Levine et al., 2022). This high reading engagement contributes to autonomously motivated readers’ comprehension skills (De Naeghel et al., 2012), whereas no connection has been found between controlled motivation and reading ability (Van Ammel et al., 2021). Indeed, reinforcing adults’ autonomous motivation is essential for increasing their voluntary reading engagement (Levine et al., 2022). SDT suggests that it can be nurtured by fulfillment of three basic needs: readers need autonomy in selecting their own reading materials, relatedness via social encouragement to read, and sufficient competence to read their selected texts (Deci and Ryan, 2008b; see Figure 1).
Figure 1. Motivation theory according to self-determination theory and hierarchical model of motivation. The diagram is inspired by Deci and Ryan (2000) and Vallerand (2000), and it is based on Self-determination theory and Hierarchical model of motivation. Motivation occurs on three hierarchical levels, global, contextual, and situational, and motivation type is mediated by the basic needs of motivation. Motivation varies by the extent to which action is self-determined, and it is categorized in three types–amotivation (the lack of drive), controlled motivation (which compasses externally regulated extrinsic motivation), and autonomous motivation (which reflects autonomous extrinsic motivation and intrinsic motivation).
Self-determination theory provides a powerful framework to study situational motivation, such as the drive to read a particular text. However, motivation is a multifaceted concept that is not only connected to situational drive, but also a “set of an individual’s beliefs about, attitudes toward, and goals for reading” (Conradi et al., 2014, p.154). To capture this complexity, Vallerand (2000) expanded SDT with three hierarchical levels of motivation - situational, contextual, and global–which describe increasingly high-level motivation (see Figure 1). The former two, contextual and situational levels of motivation, are particularly important for reading engagement. Whereas situational motivation tells us about the readers’ experiences with a text, contextual motivation indicates how individuals approach reading as an activity (Vallerand, 2000). For example, avid readers are likely to have autonomous motivation on the contextual level, but nevertheless, their drive toward individual texts can shift situationally (Vallerand, 2000). To account for the multifaceted nature of motivation, we adopt SDT with the extension of Vallerand (2000)’s hierarchical levels (see Figure 1). Therefore, motivation to read was measured on both the contextual and the situational levels.
Previously, “reading behavior” has been used non-specifically to describe habits and cognitive processes associated with reading. We define reading behavior as behavior that can be observed or measured quantitatively, such as for how long or how linearly a text is read. We focus on three measures of reading behavior: task-switching, reading speed, and linearity of reading.
Task-switching refers to the alternation between two or more tasks, and it is common during activities such as reading or studying (Rosen et al., 2013). Previous research has connected task-switching to controlled motivation, as feelings of boredom and fatigue can make the reader gravitate toward other activities, and thus task-switch frequently (Tulis and Fulmer, 2013; Ralph et al., 2021). In contrast, autonomously motivated readers are likely to be absorbed in reading, resulting in longer continuous engagements (List et al., 2019; Levine et al., 2022). Indeed, a keen interest can cause a reader to disregard all distractions as sufficiently challenging and engaging texts can induce “flow” which is characterized by a complete immersion in a task (McQuillan and Conde, 1996; Csikszentmihalyi, 2014).
Similarly, reading speed and linearity of reading may be connected to readers’ motivation to read a text. Previous research has shown a robust link between speed, linearity, and comprehension (e.g., Rayner, 1998; Schotter et al., 2014). When the text is difficult to understand readers slow down and reread sections (Rayner et al., 2016), whereas an easy text can be read chronologically, using a faster reading speed (Brysbaert, 2019). High reading fluency allows readers to decode words automatically at a fast rate, which frees their attention for comprehending meaning in the text (Pikulski and Chard, 2005). In contrast, low fluency is associated with a slower reading speed and frequent regressions to reread text (Stanovich, 1989; Pikulski and Chard, 2005). Previous research has demonstrated that high reading fluency is reciprocally connected to motivation: high reading frequency facilitates reading competence, which in turn encourages the individual to read more often (Stanovich, 1989; Mol and Bus, 2011). Similarly, it would be plausible to expect that competence and motivation influence reading behavior with individual texts: readers with low situational motivation may not wish to spend additional time and cognitive resources on a difficult task, and so they may not react to text difficulty in the same way as motivated readers. Indeed, unmotivated and uninterested readers have described themselves speeding through books and skipping parts of the text (Rosenthal, 1995; Garces-bacsal and Yeo, 2017), whereas autonomous motivation can encourage careful reading (Worthy et al., 2001; Ronimus et al., 2022).
Previous research has studied linearity on the word or sentence level (Jarodzka and Brand-Gruwel, 2017), whereas we measure linearity on the page-level. Although lab-based approaches have indicated that long-ranging nonlinear movements may be rare (Weger and Inhoff, 2007), it is possible that individuals make use of them in their natural environments. Indeed, reluctant readers in a study by Garces-bacsal and Yeo (2017) reported that they often skip uninteresting and difficult sections in a text. Our approach provides the first opportunity to inspect nonlinearity quantitatively in an ecologically valid context.
Electronic texts can take various forms, from linear, mono-modal texts that are similar to their print versions, to multimodal text presentation designed for nonlinear, fragmented reading (D’Ambra et al., 2019). Although the former is intended for deep reading engagement, similarly to print books, many feel distracted during electronic reading and prefer reading narrative fiction in print format (Liu, 2022). Baron (2017) and Latini et al. (2020) argue that distractibility during electronic reading is due to the immateriality of the reading format, which promotes shallow reading habits and frequent task-switching. Similarly, findings by Mangen et al. (2019) indicated that the fluidity of electronic texts undermines readers’ understanding of their location in an electronic text, suggesting that the materiality of print texts is important for accurate story recall.
However, few studies have accounted for readers’ electronic experience. Adults tend to have more experience with print texts (Vernon, 2006), which have different affordances than their electronic equivalents. These differences can result in frustration if the reader’s existing strategies are not compatible with the reading format (Chau and Hu, 2001; Vernon, 2006). It is plausible to expect that readers with little experience reading electronic texts are unaware of features that could support their reading engagement. Findings by Chen et al. (2021) showed that this can have a considerable impact on comprehension, as university students who failed to reread text and make use of electronic features, such as bookmarking or annotating text, when studying lecture slides received a lower grade for their course. Whereas print-reliant individuals may struggle with digital reading, electronically experienced readers are unlikely to face difficulties. Findings by Yoo and Roh (2019) showed that readers with experience reading electronically had a mental schema of electronic reading strategies that was consistent with the reading medium, and so they found electronic books easy to use. Similarly, electronically experienced readers in a study by Kosch et al. (2021) reported that they can become equally immersed in fictional texts, regardless of the reading format.
Different electronic texts and digital devices provide different affordances and challenges, and accordingly, it is likely that a reader needs task-relevant electronic reading experience (TR-EEXP) to effectively engage in e-reading. Experience with a reading device may allow the reader to neglect any notifications and interactive elements they do not want to engage with, whereas an uncustomed user may feel overwhelmed by them (Hess and Detweiler, 1994; Kuzmicova et al., 2020). However, experience using the device is unlikely to be sufficient without experience in reading long-form texts. If the reader is only experienced in using the device for rapidly changing media, they may not be accustomed to focusing on a text for extended periods of time (Baron, 2021). Therefore, electronic experience should be task-relevant both in relation to the text being read and the digital device used. This TR-EEXP may play a key role in supporting electronic reading engagement and navigation. Indeed, the importance of electronic reading experience has been recognized for web reading (e.g., Leu et al., 2015; Kiili and Leu, 2019), although it has been largely overlooked in research on reading of linear, electronic texts.
Previous studies have used a variety of methods to study how individuals engage in reading. Eye-tracking research has studied reading of mostly short passages of text, focusing on low-level measures such as fixation duration (Jarodzka and Brand-Gruwel, 2017). Self-report studies, on the other hand, have studied reading habits such as frequency of reading and reading preferences (e.g., Garces-bacsal and Yeo, 2017). The current study provides a middle ground between these two approaches: our aim was to study electronic reading behavior in detail by unintrusive observation. To do so, we created an e-reader web application, which allows us to gather rich data on reading behavior on the page-level in the participants’ natural environments.
Participants were asked to read an approximately 15-page short story on the e-reader web application. To influence participants’ situational motivation to read the story, we manipulated their autonomy in text selection. According to SDT, autonomy is one of the basic needs of autonomous motivation (Deci and Ryan, 2008b). Thwarting it should therefore result in controlled motivation, whereas participants who are given autonomy in text selection should be more likely to experience situational autonomous motivation. As a result, we expected that (H1) participants in the high-autonomy condition would experience more autonomous situational motivation compared to participants in the low-autonomy group.
We aimed to address two research questions:
RQ1: Is electronic reading behavior connected to situational or contextual motivation?
RQ2: Is electronic reading behavior connected to task-relevant electronic reading experience?
Previous studies have suggested that autonomous motivation and TR-EEXP can support reading engagement (e.g., Yoo and Roh, 2019; Ralph et al., 2021). Accordingly, we expected autonomously motivated and electronically experienced readers to task-switch infrequently (H2a-c). Furthermore, motivation and TR-EEXP have been connected to the ways in which readers react to text difficulty (Worthy et al., 2001; Garces-bacsal and Yeo, 2017). As a result, we expected autonomous motivation and TR-EEXP to be connected to slower reading speeds (H3a-c) and frequent nonlinear reading patterns (H4a-c) when text difficulty is high, as an indication of efforts to enhance comprehension. See Table 1 for an overview of the hypotheses.
Sixty undergraduate students from a Scottish university (35 women, 24 men), aged 19–30 (M = 22, SD = 2), were recruited by advertisements in a student recruitment website. 28% of participants were non-native English speakers. Informed consent was obtained from all participants. Participants were reimbursed 20GBP for their time.
Participants were asked to respond to three questionnaires, one at the beginning of the study and two at the end. The questionnaires were used to measure situational and contextual motivation, demographics, and TR-EEXP.
Participants first responded to Intrinsic Motivation Inventory on Reading (IMI-R), developed by Frijters (2004) to measure contextual reading motivation in children. IMI-R is an adjusted version of a situational motivation questionnaire, Intrinsic Motivation Inventory (IMI) by Deci and Ryan (1990). IMI-R includes 20 items asking participants to rate their interest toward reading as an activity, their reading ability, and effort put in reading. Only the interest and competence subcomponents were used in analyses. The ratings are made on a 7-point Likert-scale from “not at all true of me” to “very true of me.” Frijters (2004) found a high internal consistency (α=0.89) for IMI-R. The wording in IMI-R was modified to allow use with adults. In all items, “reading stories” was changed into “recreational reading” (e.g., I like reading stories was changed into I like recreational reading), and item 11 “I think I read stories well, compared to other kids” was changed into “I think I read well, in comparison to others.” Items in each subcomponent were summed to capture scores. The full scale and the subcomponents had good internal consistency, α=0.80−0.90.
Participants responded to IMI after reading the short story. IMI is a widely used questionnaire inspired by SDT, and its usage has been tested extensively (Leng et al., 2010). The full questionnaire comprises 45 items measured on a 7-point Likert-scale ranging from “not at all true of me” to “very true of me,” however, the questionnaire is rarely used in full (Markland and Hardy, 1997). We used 21 items from IMI to measure participants’ situational interest, perceived competence, and autonomy. Whereas autonomy and competence refer to the basic needs of autonomous motivation, the subcomponent of interest is thought to represent intrinsic motivation, the most internalized type of autonomous motivation, and as such it is considered to represent participants’ situational motivation (Center for Self-determination Theory, 2021). Each item in IMI was modified by specifying the activity as “reading the story” (for example, “I thought this activity was quite enjoyable.” was changed into “I thought that reading this story was quite enjoyable”). Similarly to IMI-R, the full scale and the subcomponents had good internal consistency, α=0.87−0.95.
Task-relevant electronic reading experience was measured by a survey developed for this study. It included 21 items measuring participants’ frequency of using digital devices for reading (5-point Likert-scale from “Never” to “Every day,” see the full questionnaire in Supplementary Information). TR-EEXP was assessed by two measures: (1) frequency of reading task-relevant text types on any electronic devices, and (2) frequency of using task-relevant digital devices for any type of recreational reading. Task-relevant text types included two types of narrative texts, books and short stories, and task-relevant digital devices included smartphones, laptops, PCs, and tablet computers. A combination of these two measures was expected to be indicative of TR-EEXP.
Nine short stories in English were used as reading material. The stories were obtained from public domain and Creative Commons repositories.1 The stories covered a wide range of genres to cater for the participants’ interests. On average, the stories were 9,333 words long (approximately 15–24 A4 pages, see Supplementary Tables 1, 2).
In the text selection procedure, participants were asked to give ratings to short story summaries. The summaries were constructed by two annotators who after reading the stories extracted sentences from them that were descriptive of the content without giving away the plot. The extracted sentences were compared, and 3–4 were selected and arranged into summaries. Summaries were then minimally edited to improve readability. The stories and summaries were assessed by five external annotators, and all were reported to reflect the story content accurately.
An e-reader web application was developed in collaboration with a professional web developer. The e-reader was accessed as a website via a browser on computers or mobile devices. In addition to the e-reader, the website included the consent form, a baseline reading speed test, questionnaires, and an information sheet.
Similarly to popular e-readers, our system displays text on horizontal page-views on which pages are turned instead of vertically scrolled. The text on screen is dynamically organized depending on the device: if the screen width is less than 1,192 pixels, the text is shown in one column, whereas wider screens show text organized into two columns (see Figure 2). Pages could be turned backwards or forwards using a keyboard, or by tapping or clicking on the side of the page. The text could also be navigated by interacting with a progress bar at the bottom of the screen.
Figure 2. Screenshots from the e-reader system. (A) Engaged reading view on a large device, such as a laptop, with two columns of text. (B) Engaged reading view on a small device, such as a smartphone, with one column of text. (C) Masked text view on a small device. The text is masked when the user unfocuses the browser or after 5 min of inactivity. (D) Open menu view on a small device. Opening the menu masks the text.
The text was shown on a light gray background with a black, Times New Roman font at size 13.2pt. The background and text color combination were used based on findings by Huang et al. (2019) which showed that it was the most comfortable reading layout for readers in a variety of lighting conditions. E-readers often provide options to alter the reading layout, however, no customizations were included to reduce noise in the data.
Text masking was used to differentiate when the participants were engaged or disengaged from the text. Opening a menu in the e-reader, unfocusing the browser window (e.g., by clicking outside the browser window), or more than 5 min of inactivity caused the e-reader to mask the text (see Figure 2). Clicking or tapping on the e-reader reactivated it, removing the mask.
The functionality and usability of the e-reader was tested in a pilot study with five participants. All pilot participants reported that the e-reader was comfortable to use and it functioned well on various devices.
The e-reader has embedded, unobtrusive tracking functions to collect data on reading behavior. Data are collected in the client-side by creating a tracking event when the participant logs in or out of the website, uses menus, turns the page, or disengages from the reading material. The events create a continuous record of participants’ reading behavior when the e-reader is in use. Therefore, event durations could be calculated by subtracting an event’s start time from the next.
Participants were assumed to be reading the story and thus they were “engaged” if the text was visible for longer than 15 s continuously. This threshold was used in accordance with findings by Iqbal and Horvitz (2007) which showed that resuming attention in a task following a disruption took individuals approximately 15 s. Events were grouped into reading sessions. A session began when the e-reader was opened, and ended when the e-reader was closed, or after 60 min of inactivity. Any reading sessions that lasted for less than a minute or which consisted of less than 20% engagement were removed.
Reading speed was captured in words per minute (wpm) by comparing the time spent on a page-view to the number of words on each page. We were primarily interested in “deep reading” speeds which reflect a speed with which the text can be comprehended in full. Deep reading was defined based on participants’ baseline speed measured at the beginning of the study to account for individual variation. Previous research has indicated that texts are rarely read slower than 100 wpm in English (Chung, 2002; Brysbaert, 2019), and so this value was used as a lower threshold of deep reading. The upper limit, on the other hand, was set based on findings by Dyson and Haselgrove (2001) which showed that an individual with a baseline reading speed of 250 wpm can comprehend a text in full with a speed up to 600 wpm. Considering that 250 wpm * 2.4 = 600 wpm, deep reading was expected to be at most 2.4× faster than baseline reading speed.
In the baseline reading speed test, participants were asked to read a 200-word segment of a narrative text. If the participant advanced from the reading speed test too quickly, indicating a reading speed of over 900 wpm, they were asked to repeat the test with a different 200-word segment of the story. Due to a mistake, no lower threshold was included in the test, and indeed, six participants’ baseline reading speed was slower than deep reading (48.5–86.4 wpm). Despite the low baseline, these participants did not use lower reading speeds during the study compared to the rest of the sample, t(8559) = 53.864, p > 0.999. These participants’ baseline was adjusted by taking an average from recorded reading speeds that fell in between 100–900 wpm. After these adjustments, the average baseline reading speed in the sample was 280.4 wpm (SD = 127.0 wpm).
To classify navigation as nonlinear or linear, we compared the location of each event to the next. Any events that showed movement backwards in the text were identified as regressions, whereas events that showed movement forwards to a position further than the next page were categorized as forward leaps. Any other navigation was identified as “linear.”
More information on the tracking data and its interpretations are available in the code repository, see https://github.com/PauliinaV/Short_Story_Reading_Behaviour_Public.
To participate, students contacted the experimenter and registered to use the e-reader with a personalized code. The participants were made aware that the study includes a speed test, questionnaires, and reading a short story on the e-reader within 14 days. They were told that the story would be selected according to their interest ratings for summaries.
After registration, participants completed the consent form, the baseline reading speed test, and IMI-R. Participants were then presented with the titles, authors, and summaries of nine short stories, and they were asked to rate how interested they were in reading each story in full (5-point Likert-scale ranging from “very interested” to “not at all interested”). The ratings were used to allocate participants in two conditions: in the high-autonomy condition, participants were given the story that they ranked as most interesting (n = 30), whereas in the low-autonomy condition, they were given the least interesting story (n = 30). After text selection, participants could access the e-reader. A pop-up informed participants which story was selected for them, but no information was included on why they received this text. Automatic reminder emails to complete the study were sent 7 days, 2 days, and 24 h before the end of the reading phase.
Participants responded to IMI and the electronic experience questionnaire once the 14 days had passed, or earlier if they indicated from the e-reader menu that they had finished the reading task. Once the participant had responded to the questionnaires, they were thanked, compensated, and debriefed.
This study had a between-subjects design. The independent variables included the autonomy condition, contextual reading motivation, and TR-EEXP. The dependent variables were measures of reading behavior; task-switching, reading speed, and linearity of reading. See Figure 3 for information on the reading behavior measures.
Figure 3. Illustrations of reading behavior measures. The measures are shown for illustrative purposes only, not based on the tracking data. (A) Continuous engagement duration was used as a measure of task-switching. The gray arrows indicate the length of continuous engagements during a reading session. (B) Reading speed was measured on each page-view by dividing tracked speed with participants’ baseline speed. Only “deep reading” speeds were used. (C) Frequency of initiating nonlinearity was used as a measure of linearity of reading, shown as orange circles in the illustration.
Reading behavior was analyzed by multilevel models to capture variation between participants and short stories. We used two models, one to address our hypotheses (a model of “reader characteristics”), and another to explore how reading behavior varied in the context of the reading task (a model of “event properties,” see Supplementary Table 3). The former was conducted for all reading behavior measures, whereas the latter was only applied to page-level measures (linearity of reading and reading speed).
Depending on the outcome variable distribution, we used either linear mixed models or generalized linear mixed models with R and the lme4 package (Bates et al., 2015), and p-values were estimated with the lmerTest package (Kuznetsova et al., 2017). The models were tested for multicollinearity, influential observations, heteroscedasticity, and normality of random effects (see analysis code for more information2). All categorical variable contrasts were set with Helmert coding, whereas continuous variables were centered around the mean and scaled. See Supplementary Tables 4A–C for additional information on each model.
A theoretically motivated maximal interactive model structure was pre-specified for the reader characteristics models. The key variables in the model included measures of situational and contextual motivation, text difficulty, and TR-EEXP. Situational motivation was measured by autonomy condition, whereas contextual “interest” subcomponent from the IMI-R was used as an indicator of contextual motivation. Participants’ score in the situational “competence” subcomponent from the IMI was used as a measure of text difficulty. Finally, TR-EEXP was measured by two variables to assess frequency of reading task-relevant text types and usage of task-relevant digital devices.
In addition to these key variables, the reader characteristics models included control variables to account for possible confounds. Reading ability was controlled by contextual competence score, whereas demographic information on participants’ gender and native language was included to control for between-subjects effects. A measure of “window width” was included to account for variation in device size and text layout, and the number of days left to read the story was used to account for the possibility that behavior may have been affected by increasing pressure to finish the story in time.
The model included nested random intercepts for participant and story indicator. Explicit nesting was used so that random slopes could be specified separately for the two random effects. In accordance to suggestions by Barr et al. (2013), we included random slopes for variables of theoretical interest. However, the slopes could only be included in the random effect of story because the variables of interest (motivation, situational competence and TR-EEXP) were measured only once for each participant.
The maximal interactive model structure had the following form, where predictors in italics were only included in models for speed and linearity:
Reading Behavior = Window width + Days until reading deadline + Whether native language is English or not + Condition + Situational competence + Contextual motivation + Contextual competence + Electronic device types + Electronic text types + Electronic device types x Electronic text types + Condition x Situational competence + Contextual interest x Situational competence + Electronic device types x Electronic text types x Situational competence + (1 + Condition x Situational competence + Contextual motivation x Situational competence + Electronic device types x Electronic text types x Situational competence | Story indicator) + (1 | Participant indicator).
The event properties model was used for explorative purposes to study variance in linearity and reading speed. First, an additive intercepts-only model was specified, which included predictors describing the event timing (reading session indicator and time in a reading session), location in text, and previous events. The last was used to assess the connection between the outcome variable (reading behavior at event k) and reading behavior at events k-1 and k-2. Additionally, the model included days until reading deadline and window width as control variables, similarly to the reader characteristics model. Nested random intercepts of participant and story indicator were included to account for dependencies in the data.
The additive intercepts-only structure had the following form:
Reading behavior = Window width + Days until reading deadline + Eventk–1 + Eventk–2 + Reading session number + Time in the reading session + Location in text + (1 | Story indicator / Participant indicator).
Random slopes were added to the model with the “best-path” algorithm described by Barr et al. (2013). In this data-driven selection method, addition of each predictor as a random slope was tested against the intercepts-only model using a liberal alpha-level of 0.20. If multiple predictors contributed to the model, the slope with the lowest p-value was added to it. This selection was then repeated for the remaining slopes.
Once the additive random slopes model was selected, it was simplified by the backward stepwise method until convergence. Two-way interactions were then added between predictors remaining in the model, and in case of non-convergence, the model was again selected backward stepwise. To avoid multicollinearity, the two predictors indicating of previous events (Eventk–1 and Eventk–2) were not included in interactions with any predictors apart from each other.
Participants varied in how many sessions they read the short story, with 39 of the 60 participants (65%) reading it in a single session, whereas the rest read the story in 2–5 sessions. In total, the participants spent 13.2–126.3 min reading the story during the study (M = 50.5 min, SD = 28.3 min). All but two participants finished their story.
Findings from the “interest” subcomponent of IMI-R showed that participants were on average somewhat interested in reading as an activity (see Table 2). Contextual motivation was significantly correlated with participants’ recreational reading frequency, r = 0.415, p = 0.001, and how many books were read for leisure in the past year, r = 0.567, p < 0.0001. Therefore, participants with more autonomous contextual motivation read more frequently for leisure. In contrast, no connection was found between contextual motivation and frequency of reading as part of work or study, r = −0.034, p = 0.794.
Most frequently, participants read print books for pleasure (M = 3.3, SD = 1.2), whereas laptops (M = 2.6, SD = 1.3) and smartphones (M = 3.0, SD = 1.4) were used for recreational reading on average “a few times a month.” Narrative texts were read digitally only a “few times a year” (Mbooks = 2.0, SDbooks = 1.0, Mstories = 1.9, SDstories = 1.0), and the most frequently read electronic text types included newspaper articles (M = 3.7, SD = 1.3), academic journals (M = 3.6, SD = 0.9), and textbooks (M = 3.2, SD = 0.9). Indeed, while the participants were somewhat experienced in using digital devices for reading expository texts, few had TR-EEXP from e-reading narrative texts on task-relevant digital devices (see Table 2).
Autonomy in text selection was manipulated to influence participants’ situational motivation. In accordance with H1, participants in the high-autonomy condition reported more autonomous motivation in comparison to the low-autonomy group, t(49.66) = 2.225, p = 0.031 (see Table 2). These differences were not due to participants’ contextual motivation as participants in the two conditions did not differ in contextual interest scores, t(55.14) = 1.181, p = 0.243.
Task-switching frequency was expected to be connected to motivation and TR-EEXP (H2a-c). It was measured by continuous engagement duration which reflects the time that participants engaged in reading continuously before disengaging from the text (see Figure 3). Therefore, longer continuous engagements reflect infrequent task-switching, whereas short continuous engagement durations indicate the opposite. In total, the participants had 1–21 continuous engagement blocks during the study (M = 4.2, SD = 3.7), and on average, continuous engagement lasted only for 11.6 min (see Table 2).
Participants in the high-autonomy condition were expected to task-switch infrequently compared to the low-autonomy group (H2a). However, we failed to reject the null hypothesis as autonomy condition was not a significant predictor in the model (see Table 3). In contrast, contextual motivation, indicating participants’ interest in reading as an activity, was a significant, positive predictor of continuous engagement duration, supporting our hypothesis H2b (see Table 3). The finding showed that participants with more autonomous contextual motivation task-switched less often compared to participants with more controlled contextual motivation.
Table 3. Results on reader characteristics models of task-switching frequency, reading speed, and linearity of reading.
Similarly, TR-EEXP was expected to be connected to task-switching frequency (H2c). However, contrary to our expectations, an interaction between the two electronic experience measures was not a significant predictor of continuous engagement duration (see Table 3), indicating that task-relevant experience did not influence participants’ task-switching frequency.
Finally, participants’ task-switching frequency was found to be significantly connected to contextual and situational competence (see Table 3). The results showed that participants who found the story easier to read task-switched less often than participants who found the task more difficult. In contrast, contextual competence, indicating participants’ perception of their reading ability, was negatively associated with continuous engagement duration: participants who reported a higher reading ability task-switched more often compared to participants with lower competence scores.
Participants showed considerable variance in their deep reading speeds (see Figure 4). This variance was measured by reading rate, which indicated how speed varied on each page view relative to baseline speed (speed/baseline speed, see Figure 3). Therefore, the measure shows the magnitude and the direction of variance in deep reading as a proportion of each participants’ baseline speed.
Figure 4. Six participants’ variance in deep reading across the text. Each plot shows one participant’s tracked deep reading rate at different parts of the text. The horizontal black line represents baseline speed.
A model of reader characteristics was used to assess the connection between participants’ reading speed, motivation, and TR-EEXP. Participants in the high-autonomy condition were expected to use baseline-level and slower reading speeds when the task was perceived to be difficult (H3a). However, the null hypothesis was not rejected as an interaction between condition and situational competence was not a significant predictor of reading speed (see Table 3). Furthermore, autonomous contextual motivation was expected to be connected to slower reading speeds when the text was difficult (H3b). The findings supported this hypothesis as an interaction between situational competence and contextual motivation was a significant predictor of reading speed (see Table 3). The result showed that more autonomously contextually motivated readers used slower reading speeds when the text was difficult to read, and faster speeds when the text was perceived to be easy (see Figure 5). In contrast, participants with a lower score in contextual motivation used higher than baseline-level reading speeds when the text was perceived to be difficult to read, and slower reading speeds when the text was reported to be easy.
Figure 5. The effect of contextual motivation and situational competence on reading speed. The points represent observed values whereas the lines show the model fit. Contextual motivation is measured by contextual interest from IMI-R, and reading speed is measured by reading rate which indicates reading speed on each page-view divided by baseline speed. The predictors were centered around the mean and scaled.
Similarly, TR-EEXP was expected to be associated with slower reading speeds when the text was difficult to read (H3c). Indeed, an interaction between the two electronic reading experience measures and situational competence was a significant predictor in the model (see Table 3). The effect showed that participants with task-relevant experience used slower reading speeds when the text was difficult to comprehend, and faster reading speeds when the text was perceived to be easy. In contrast, a mismatch in electronic experience, due to a high level of either type of experience and a low level of the other, was connected to the opposite pattern: difficult texts were read at a higher speed than stories which were perceived to be easier to comprehend (see Figure 6). Interestingly, a low score in both electronic reading experience measures was connected to a pattern that was similar to high TR-EEXP (see Figure 6).
Figure 6. The effect of TR-EEXP and situational competence on reading speed. The lines show the model fit and the shaded areas show 95% confidence interval. TR-EEXP (Task-relevant Electronic Reading Experience) is measured by two measures: Electronic Experience 1 shows frequency of reading task-relevant text types electronically, and Electronic Experience 2 shows frequency of using task-relevant digital devices for recreational reading. Reading speed is measured by reading rate which indicates reading speed on each page-view divided by baseline speed. The predictors were centered around the mean and scaled.
Furthermore, reading speed was predicted by contextual competence (see Table 3). The finding showed that participants who perceived themselves to be highly proficient readers were more likely to use faster reading speeds than participants who reported a lower reading skill.
Another model was used to assess how reading speed varied within the context of the reading task. The findings from this event properties model showed that reading speed could be predicted by behavior at the previous events (eventk–1 and eventk–2), and location in text (see Table 4). Connection to previous events showed that a fast reading speed at eventk (the outcome variable) was likely to follow from a similarly high reading speed at eventk–1 and eventk–2. Therefore, consecutive pages were likely to be read at a similar speed. Furthermore, reading speed was also connected to location in text: participants tended to increase their reading speed toward the end of the document (see Table 4).
Participants varied in how linearly they read the short story (see Figure 7). On average, participants made 8.8 regressions (SD = 8.8) and 1.2 forward leaps (SD = 1.9) while reading the text. This nonlinearity was measured by a binary variable indicating whether nonlinear navigation was initiated on each page-view (see Figure 3).
Figure 7. Reading process of participants who read The Adventures of Sherlock Holmes: The Boscombe Valley Mystery by Arthur Conan Doyle. The black, dashed line shows what a chronological reading process would have looked like at a steady speed of 260 wpm. The colored lines indicate individual participants’ reading process.
First, nonlinearity was modeled by reader characteristics. We expected an interaction between situational competence and the two motivation measures, condition and contextual interest, to be connected to frequency of initiating nonlinearity (H4a-b). However, the null hypotheses could not be rejected as the interaction effects were not significant predictors of nonlinearity (see Table 3). This indicates that motivation was not connected to nonlinear navigation via text difficulty, contrary to our expectations.
An interaction between the TR-EEXP measures and situational competence was similarly expected to be connected to nonlinear navigation patterns (H4c). The three-way interaction effect was not significant, and thus our hypothesis was not supported. Interestingly, a two-way interaction between the electronic reading experience measures was a significant predictor of linearity (see Table 3). The finding showed that a high score in the TR-EEXP measures was associated with a lower likelihood of nonlinear navigation. In contrast, a mismatch in the measures – indicated by a low score in either measure and a high level of the other - was associated with frequent nonlinear navigation. A low score in both TR-EEXP measures was connected to low likelihood of nonlinear navigation, similarly to high levels of TR-EEXP. Overall, this indicates that electronic experience was connected to participants’ linearity of reading, although not via situational competence like we expected.
Second, linearity of reading was modelled by event properties to study how linearity varied across the reading task. The results showed that nonlinearity was connected to the previous navigation event, timing in a reading session, and an interaction between time in a reading session and location in text. The first finding showed that nonlinearity could be predicted by the previous navigation event (see Table 4). Participants were more likely to initiate nonlinear navigation if the previous event (eventk–1) did not initiate nonlinearity. In other words, participants were unlikely to move between different types of nonlinear navigation (e.g., from a regression to a forward leap) in consecutive navigation events. Unlike findings on reading speed, eventk–2 was not connected to the odds of nonlinear navigation at eventk.
A main effect of time since the beginning of the reading session showed that the odds of nonlinear navigation were highest at the beginning of reading sessions (see Table 4). This indicates that nonlinearity was used primarily at the beginning of the reading sessions. The main effect was qualified by a significant interaction between time in a reading session and location in text. The finding showed that nonlinear navigation was most likely when the participants were at the beginning of the story at the beginning of a reading session. Therefore, nonlinear navigation may have been most likely at the beginning of the reading task.
The aim of this study was to enhance our understanding of the ways in which adults read electronically. Reading behavior was tracked on participants’ own digital devices to assess their task-switching frequency, reading speed, and frequency of nonlinear navigation. Situational motivation to read the story was manipulated by giving half of the participants a sense of autonomy over text selection, whereas the other half were asked to read a short story that they would not have selected for themselves. In response to our research questions, we studied the connection between situational and contextual motivation, and TR-EEXP (task-relevant electronic reading experience). Furthermore, we assessed how reading behavior varied in the context of the reading task.
Contrary to our expectations, the motivation condition was not a significant predictor of reading behavior. This was surprising as previous studies have linked situational motivation to lower distractibility (Ralph et al., 2021), persistent engagement with the text (List et al., 2019), and more linear reading patterns (Garces-bacsal and Yeo, 2017). The non-significant findings could be due to the limited impact of the autonomy manipulation. Indeed, participants in the two conditions differed only by 0.84 points (on a 7-point scale) in their situational motivation. The small difference may have not resulted in noticeable variation in reading behavior.
In contrast, contextual motivation was connected to reading behavior. The results showed that participants who enjoy reading as an activity engaged continuously for longer at a time, and thus task-switched less. Similar results have been reported previously in self-report studies, indicating that contextual motivation can support engagement in an activity (Levine et al., 2022) and result in immersive flow experiences which are characterized by extended attention to a task (McQuillan and Conde, 1996).
Furthermore, contextual motivation was linked to reading speed via an interaction with situational competence. Participants who were motivated to read for pleasure used slower reading speeds when the text was perceived to be difficult, and faster speeds when the text was easy. Previous studies have shown that this alternation is important for comprehending difficult texts (e.g., Brysbaert, 2019). Interestingly, participants with lower contextual motivation showed the opposite pattern: they read difficult texts at a fast speed and slowed down when reading easier texts. One plausible explanation for this difference is that contextual motivation modulates readers’ response to task demands. Motivated readers’ interest in the activity may have encouraged them to read the text more carefully, whereas lower levels of contextual motivation could have caused the readers to neglect the importance of reading difficult texts slowly. Previous studies have indicated that difficult and uninteresting tasks are seen as unrewarding and resource-depleting, which can cause readers to devote less cognitive resources to them (Soemer and Schiefele, 2019). Motivated readers may be more likely to struggle through difficult texts, a finding that was echoed in an interview study by Worthy et al. (2001).
In addition to alternations in reading speed, varying one’s linearity of reading is important for comprehension (Schotter et al., 2014). Previous studies have made this connection by eye-tracking during reading of short passages; however, our findings indicate that frequency of nonlinear navigation on the page-level may be similarly connected to text difficulty. High text difficulty was associated with frequent nonlinear navigation, whereas easier texts were read more chronologically.
We expected participants’ contextual motivation to be connected to their frequency of nonlinear navigation via an interaction with text difficulty. This hypothesis was not supported, which was surprising considering that variance in reading speed could be partly explained by text difficulty and contextual motivation. It is possible that nonlinear navigation is more automatic and reactive compared to alternations in reading speed, and so it may not be dependent on participants’ motivation to understand the text. In eye-tracking research, regressions backward in text have been found to be largely automatic (Rayner et al., 2016), however it is unclear to what extent nonlinear navigation on the page-level is consciously controlled behavior. Alternatively, the lack of an effect could have been due to our measure of nonlinearity. The e-reader system allowed us to track both regressions and forward leaps, but they were not differentiated in the measure of nonlinearity. However, the two types may have been used for different purposes - whereas regressions are frequently used for enhancing comprehension (Rayner et al., 2016), forward leaps may be driven by motivation. Indeed, findings from an interview study by Garces-bacsal and Yeo (2017) showed that unmotivated readers occasionally skip parts of the text by jumping ahead to finish the text faster. Therefore, studying regressions and forward leaps separately could have provided different results.
In addition to motivation, we studied the connection between reading behavior and participants’ experience with electronic reading. The findings showed that participants with a high level of task-relevant experience read the story slowly if the text was difficult, and at a faster pace if the text was easy. In contrast, a mismatch in the two TR-EEXP measures, indicating a high level of one type of task-relevant experience and a low level of the other, was connected to fast reading speeds when the text was difficult to read, and a slower reading rate when the text was easy. This pattern of results resembles our finding on contextual motivation and reading speed, and indeed, it is possible that TR-EEXP supports readers in reacting to task-demands, similarly to motivation.
Interestingly, participants’ linearity of reading was connected to TR-EEXP independently from text difficulty. The results showed that participants with task-relevant experience read the text more linearly compared to the less experienced participants, regardless of how difficult the text was. This indicates that page-level nonlinear navigation may not tell us about text difficulty, whereas a robust effect between the two has been found in eye-tracking research during reading of short passages of text (Schotter et al., 2014). Instead, TR-EEXP may guide readers’ navigation patterns across multiple pages.
In addition to motivation and TR-EEXP, reading behavior was assessed in relation to the task context. The findings showed that reading speed was positively connected to location in text, and therefore, participants increased their speed toward the end of the story. A similar finding has been reported in eye-tracking research; for example, Demberg and Keller (2008) and Kaakinen et al. (2018) found reading speed to increase toward later parts of a text. This could indicate the readers’ anticipation of finishing the reading task, or their increasing familiarity with the writing style. Similarly, linearity of reading was connected to location in text: participants were most likely to initiate nonlinearity when they were at the beginning of the text in the beginning of a reading session. This behavior could be similar to what Milne (2021) described as a habit of “good readers”–exploration of the text and its structure by nonlinear navigation before committing to reading it.
Lastly, speed and linearity of reading were connected to the ways in which previous pages had been read. Reading speed at eventsk–1 and eventk–2 were positively associated with speed at eventk, indicating that consecutive pages were read at constant speed. Similarly, linearity of reading was connected to the previous event. The finding showed that alternation between forward leaps and regressions was uncommon, and instead, participants were likely to move between linear and nonlinear navigation rather than different types of nonlinearity. However, the event preceding the previous, eventk–2, could not be used to predict linearity at eventk. This indicates that nonlinearity may have been used for a variety of different purposes during the study. Some participants may have used nonlinearity primarily to enhance their comprehension by moving backwards in text to reread the previous page. Others may have varied their linearity frequently to relieve feelings of boredom, for example, by browsing the text. These differences would have made it difficult to predict linearity by events preceding the previous.
The current study provides rich descriptions of adults’ fiction e-reading behavior using a novel method. Our findings can provide a foundation for future research; however, the study also has some limitations.
Participants’ situational motivation may have been biased by the monetary compensation. Deci et al. (1999) argue that rewards influence motivation, and they can even turn autonomous motivation into controlled motivation. Indeed, the compensation may have undermined our manipulation, which aimed to influence participants’ situational motivation by their autonomy in text selection. Monetary compensation was primarily used to access a wider participant pool: we expected that only avid readers would be attracted to the study in the absence of compensation, and therefore it was decided that a reward would be favorable to a biased sample. However, future research should pursue to use compensation that does not bias situational motivation.
Short stories were used to due to time constraints in data collection. However, the reading task may have not been sufficiently long to observe reading behavior comprehensively. In adults’ everyday life, recreational reading materials are likely to consist of full-length novels which are read in multiple reading sessions, in a variety of locations (Merga, 2017). Behavior may vary across these reading sessions, as a reflection of the reader’s mood and responsibilities. Future studies should pursue to study behavior with longer texts to capture adults’ natural reading behavior.
Finally, participants’ knowledge of the tracking functions in the e-reader system may have influenced their reading behavior. Risko and Kingstone (2011) argue that the simple knowledge of being observed can cause individuals to engage in socially desirable behavior. Therefore, participants may have read the text more linearly at a steady speed, task-switching infrequently.
Future research is needed to identify how task-switching, linearity, and speed vary at different parts of a text and for what reasons. For example, text characteristics and events in the narrative text are likely to have an impact on the ways in which the text is read. To develop efficient reading promotions and encourage reading engagement in adults, reading and text selection should be studied further in ecologically valid environments via unobtrusive methods.
To support electronic recreational reading engagement, it is important to study how digital devices are used for reading in adults’ everyday life, and whether this behavior is connected to motivation or task-relevant electronic reading experience. We used a novel method that allows us to track reading behavior unobtrusively on participants’ own devices. The results showed that behavior could be predicted by the task context, such as location in text. Furthermore, behavior was associated with readers’ contextual motivation and task-relevant electronic reading experience, suggesting that avid and highly experienced readers react to text difficulty adaptively, slowing down when the text is difficult and speeding up when it is easy. Our findings contribute a much-needed middle-ground between lab-based and self-report approaches in studying reading behavior.
The datasets presented in this study can be found in online repositories. The names of the repository/repositories and accession number(s) can be found below: https://github.com/PauliinaV/Short_Story_Reading_Behaviour_Public.
The studies involving humans were approved by the University of Edinburgh, School of Informatics Ethics Committee. The studies were conducted in accordance with the local legislation and institutional requirements. The participants provided their written informed consent to participate in this study.
PV: Conceptualization, Formal analysis, Investigation, Methodology, Visualization, Writing—original draft. BT: Supervision, Writing—review and editing. FK: Supervision, Writing—review and editing.
The author(s) declare financial support was received for the research, authorship, and/or publication of this article. This research was funded by a doctoral studentship grant from the Economic and Social Research Council (ESRC) managed by the Scottish Graduate School of Social Science (SGSSS), and with additional funding from School of Informatics, University of Edinburgh.
We would like to thank Mikko Vuorinen for his work on the e-reader web application, and ESRC SGSSS and School of Informatics for funding this research project.
The authors declare that the research was conducted in the absence of any commercial or financial relationships that could be construed as a potential conflict of interest.
All claims expressed in this article are solely those of the authors and do not necessarily represent those of their affiliated organizations, or those of the publisher, the editors and the reviewers. Any product that may be evaluated in this article, or claim that may be made by its manufacturer, is not guaranteed or endorsed by the publisher.
The Supplementary Material for this article can be found online at: https://www.frontiersin.org/articles/10.3389/feduc.2023.1302701/full#supplementary-material
Atske, S., and Perrin, A. (2021). Home broadband adoption, computer ownership vary by race, ethnicity in the U.S. Washington, DC: Pew Research Centre.
Baron, N. (2017). Reading in a digital age. Phi Delta Kappan 99, 15–20. doi: 10.1177/0031721717734184
Baron, N. (2021). Know what? How digital technologies undermine learning and remembering. J. Pragmat. 175, 27–37. doi: 10.1016/j.pragma.2021.01.011
Baron, N., and Mangen, A. (2021). Doing the reading: The decline of long long-form reading in higher education. Poetics Today 42, 253–279. doi: 10.1215/03335372-8883248
Barr, D. J., Levy, R., Scheepers, C., and Tily, H. J. (2013). Random effects structure for confirmatory hypothesis testing: Keep it maximal. J. Mem. Lang. 68, 255–278. doi: 10.1016/j.jml.2012.11.001
Bates, D., Mächler, M., Bolker, B., and Walker, S. (2015). Fitting linear mixed-effects models using lme4. J. Stat. Softw. 67, 1–48. doi: 10.18637/jss.v067.i01
Brysbaert, M. (2019). How many words do we read per minute? A review and meta-analysis of reading rate. J. Mem. Lang. 109, 104047. doi: 10.1016/j.jml.2019.104047
Center for Self-determination Theory (2021). Intrinsic Motivation Inventory (IMI). Available online at: self-determinationtheory.org (accessed July 8, 2021).
Chau, P. Y. K., and Hu, P. J.-H. (2001). Information technology acceptance by individual professionals: A model comparison approach. Decis. Sci. 32, 699–719. doi: 10.1111/j.1540-5915.2001.tb00978.x
Chen, C., Yang, S. J. H., Weng, J., Ogata, H., and Su, C. (2021). Predicting at-risk university students based on their e-book reading behaviours by using machine learning classifiers. Austral. J. Educ. Technol. 37, 130–144. doi: 10.14742/ajet.6116
Chung, S. T. L. (2002). The effect of letter-stroke boldness on reading speed in central and peripheral vision. Investig. Ophthalmol. Vis. Sci. 43, 33–42. doi: 10.1016/j.visres.2013.03.005
Conradi, K., Jang, B. G., and McKenna, M. C. (2014). Motivation terminology in reading research: A conceptual review. Educ. Psychol. Rev. 26, 127–164. doi: 10.1007/s10648-013-9245-z
Csikszentmihalyi, M. (2014). Flow and the foundations of positive psychology: The collected works of Mihaly Csikszentmihalyi. Claremont: Springer.
D’Ambra, J., Wilson, C. S., and Akter, S. (2019). Affordance theory and e-books: evaluating the e-reading experience using netnography. Pers. Ubiquitous Comput. 23, 873–892. doi: 10.1007/s00779-017-1086-1
De Naeghel, J., Van Keer, H., Vansteenkiste, M., and Rosseel, Y. (2012). The relation between elementary students’ recreational and academic reading motivation, reading frequency, engagement, and comprehension: A self-determination theory perspective. J. Educ. Psychol. 104, 1006–1021. doi: 10.1037/a0027800
Deci, E. L., Koestner, R., and Ryan, R. M. (1999). A meta-analytic review of experiments examining the effects of extrinsic rewards on intrinsic motivation. Psychol. Bull. 125, 627–668. doi: 10.1037/0033-2909.125.6.627
Deci, E. L., and Ryan, R. M. (1990). A motivational approach to self: Integration in personality. Nebraska Symp. Motiv. 1990, 237–288.
Deci, E. L., and Ryan, R. M. (2000). The ‘what’ and ‘why’ of goal pursuits: Human needs and the self-determination of behaviour. Psychol. Inquiry 11, 37–41. doi: 10.1207/S15327965PLI1104
Deci, E. L., and Ryan, R. M. (2008a). Facilitating optimal motivation and psychological well-being across life’s domains. Can. Psychol. 49, 14–23. doi: 10.1037/0708-5591.49.1.14
Deci, E. L., and Ryan, R. M. (2008b). Self-determination theory: A macrotheory of human motivation, development, and health. Can. Psychol. 49, 182–185. doi: 10.1037/a0012801
Demberg, V., and Keller, F. (2008). Data from eye-tracking corpora as evidence for theories of syntactic processing complexity. Cognition 109, 193–210. doi: 10.1016/j.cognition.2008.07.008
Department for Culture, Media, and Sport (2015). Taking part 2014/15, focus on: Free time activities. London: Department for Culture, Media, and Sport.
Dyson, M. C., and Haselgrove, M. (2001). The influence of reading speed and line length on the effectiveness of reading from screen. Int. J. Hum.-Comp. Stud. 54, 585–612. doi: 10.1006/ijhc.2001.0458
Everatt, J., Bradshaw, M. F., and Hibbard, P. B. (1998). “Individual differences in reading and eye movement control,” in Eye Guidance in Reading and Scene Perception, ed. G. Underwood (Amsterdam: Elsevier).
Faverio, M., and Perrin, A. (2022). Three-in-ten Americans now read e-books. Washington, DC: Pew Research Centre.
Frijters, J. C. (2004). Conative Determinants of Reading Development and Reading-disabled Children’s Response to Remediation. Ph.D. thesis. Guelph: University of Guelph.
Garces-bacsal, R. M., and Yeo, S. D. (2017). Why and what they read when they don’t have to: Factors influencing the recreational reading habits of gifted students in Singapore. J. Educ. Gifted 40, 247–265. doi: 10.1177/0162353217717035
Gelles-Watnick, R., and Perrin, A. (2021). Who doesn’t read books in America?. Washington, DC: Pew Research Center.
Guthrie, J. T., and Wigfield, A. (2000). “Engagement and motivation in reading,” in Handbook of Reading Research, eds M. L. Kamil, P. B. Mosenthal, P. D. Pearson, and R. Barr (Mahwah, NJ: Lawrence Erlbaum Associates), 403–422.
Hess, S. M., and Detweiler, M. C. (1994). Training to reduce the disruptive effects of interruptions. Proc. Hum. Fact. Ergon. Soc. Annu. Meet. 38, 1173–1177. doi: 10.1177/154193129403801806
Howard, J. L., Bureau, J., Guay, F., Chong, J. X. Y., and Ryan, R. M. (2021). Student motivation and associated outcomes: A meta-analysis from self-determination theory. Persp. Psychol. Sci. 16, 1300–1323. doi: 10.1177/1745691620966789
Huang, H. P., Wei, M., and Ou, L. C. (2019). Effect of text-background lightness combination on visual comfort for reading on a tablet display under different surrounds. Color Res. Applic. 44, 54–64. doi: 10.1002/col.22259
Iqbal, S. T., and Horvitz, E. (2007). “Disruption and recovery of computing tasks: Field study, analysis, and directions,” in Conference on Human Factors in Computing Systems, San Jose, CA, doi: 10.1145/1240624.1240730
Jarodzka, H., and Brand-Gruwel, S. (2017). Tracking the reading eye: Towards a model of real-world reading. J. Comput. Assist. Learn. 33, 193–201. doi: 10.1111/jcal.12189
Kaakinen, J. K., Ballenghein, U., and Tissier, G. (2018). Fluctuation in cognitive engagement during reading: Evidence from concurrent recordings of postural and eye movements. J. Exp. Psychol. 44, 1671–1677. doi: 10.1037/xlm0000539
Kiili, C., and Leu, D. J. (2019). Exploring the collaborative synthesis of information during online reading. Comput. Hum. Behav. 95, 146–157. doi: 10.1016/j.chb.2019.01.033
Kingstone, A., Smilek, D., and Eastwood, J. D. (2008). Cognitive ethology: A new approach for studying human cognition. Br. J. Psychol. 99, 317–340. doi: 10.1348/000712607X251243
Kosch, L., Gunther, S., Schwabe, A., and Boomgaarden, H. G. (2021). Reading fiction with an e-book or in print: Purposes, pragmatics and practices. A focus group study. Sci. Study Literat. 11, 196–222. doi: 10.1075/ssol.21012.kos
Kuzmicova, A., Schilhab, T., and Burke, M. (2020). m-Reading: Fiction reading from mobile phones. Convergence 26, 333–349. doi: 10.1177/1354856518770987
Kuznetsova, A., Brockhoff, P. B., and Christensen, R. H. B. (2017). lmerTest package: Tests in linear mixed effects models. J. Stat. Softw. 82, 1–26. doi: 10.18637/jss.v082.i13
Latini, N., Bråten, I., and Salmerón, L. (2020). Does reading medium affect processing and integration of textual and pictorial information? A multimedia eye-tracking study. Contemp. Educ. Psychol. 62, 101870. doi: 10.1016/j.cedpsych.2020.101870
Leng, E. Y., Wan Ali, W. Z. B., Baki, R., and Mahmud, R. (2010). Stability of the intrinsic motivation inventory (IMI) for the use of Malaysian form one students in ICT literacy class. Eurasia J. Math. Sci. Technol. Educ. 6, 215–226. doi: 10.12973/ejmste/75242
Leu, D., Forzani, E., Timbrell, N., and Maykel, C. (2015). Seeing the forest, not the trees. Read. Teach. 69, 139–145. doi: 10.1002/trtr.1406
Levine, S. L., Cherrier, S., Holding, A. C., and Koestner, R. (2022). For the love of reading: Recreational reading reduces psychological distress in college students and autonomous motivation is the key. J. Am. College Health 70, 158–164. doi: 10.1080/07448481.2020.1728280
List, A., Stephens, L. A., and Alexander, P. A. (2019). Examining interest throughout multiple text use. Read. Writ. 32, 307–333. doi: 10.1007/s11145-018-9863-4
Liu, Z. (2022). Reading in the age of digital distraction. J. Docum. 78, 1201–1212. doi: 10.1108/JD-07-2021-0130
Maloney, J. (2015). It’s not the e-reader that will drive future book sales, it’s the phone: How publishers are rethinking books for the small screen. Wall Street Journal. Available online at: https://www.wsj.com/articles/the-rise-of-phone-reading-1439398395 (accessed September 15, 2021).
Mangen, A., Olivier, G., and Velay, J. (2019). Comparing comprehension of a long text read in print book and on kindle: Where in the text and when in the story? Front. Psychol. 10:38. doi: 10.3389/fpsyg.2019.00038
Markland, D., and Hardy, L. (1997). On the factorial and construct validity of the intrinsic motivation inventory: Conceptual and operational concerns. Res. Q. Exerc. Sport 68, 20–32. doi: 10.1080/02701367.1997.10608863
McGeown, S. P., Duncan, L. G., Griffiths, Y. M., and Stothard, S. E. (2015). Exploring the relationship between adolescent’s reading skills, reading motivation and reading habits. Read. Writ. 28, 545–569. doi: 10.1007/s11145-014-9537-9
Mckay, D., Twidale, M. B., and Buchanan, G. (2021). “Lady Chatterley’s library: Books and reading as public performance and private act,” in Proceedings of the 2021 Conference on Human Information Interaction and Retrieval, Canberra, doi: 10.1145/3406522.3446032
McQuillan, J., and Conde, G. (1996). The conditions of flow in reading: Two studies of optimal experience. Read. Psychol. 17, 109–135. doi: 10.1080/0270271960170201
Merga, M. K. (2017). Meeting the needs of avid book readers: Access, space, concentration support and barrier mitigation. J. Library Adm. 57, 49–68. doi: 10.1080/01930826.2016.1185854
Milne, J. (2021). Individual reading styles: A narrative approach to understanding reading behaviour. SN Soc. Sci. 1, 1–21. doi: 10.1007/s43545-020-00054-w
Mol, S. E., and Bus, A. G. (2011). To read or not to read: A meta-analysis of print exposure from infancy to early adulthood. Psychol. Bull. 137, 267–296. doi: 10.1037/a0021890
Pikulski, J. J., and Chard, D. J. (2005). Fluency: Bridge between decoding and reading comprehension. Read. Teach. 58, 510–519.
Ralph, B. C. W., Smith, A. C., Seli, P., and Smilek, D. (2021). The relation between task-unrelated media multitasking and task-related motivation. Psychol. Res. 85, 408–422. doi: 10.1007/s00426-019-01246-7
Rayner, K. (1998). Eye movements in reading and information processing: 20 years of research. Psychol. Bull. 124, 372–422. doi: 10.1037/0033-2909.124.3.372
Rayner, K., Schotter, E. R., Masson, M. E. J., Potter, M. C., and Treiman, R. (2016). So much to read, so little time: How do we read, and can speed reading help? Psychol. Sci. Public Interest 17, 4–34. doi: 10.1177/1529100615623267
Risko, E. F., and Kingstone, A. (2011). Eyes wide shut: Implied social presence, eye tracking and attention. Attent. Percept. Psychophys. 73, 291–296. doi: 10.3758/s13414-010-0042-1
Ronimus, M., Tolvanen, A., and Hautala, J. (2022). The roles of motivation and engagement in computer-based assessment of children’s reading comprehension. Learn. Individ. Diff. 98, 102197. doi: 10.1016/j.lindif.2022.102197
Rosen, L. D., Mark Carrier, L., and Cheever, N. A. (2013). Facebook and texting made me do it: Media-induced task-switching while studying. Comput. Hum. Behav. 29, 948–958. doi: 10.1016/j.chb.2012.12.001
Schiefele, U., Schaffner, E., Möller, J., Wigfield, A., Nolen, S., and Baker, L. (2012). Dimensions of reading motivation and their relation to reading behavior and competence. Read. Res. Q. 47, 427–463. doi: 10.1002/RRQ.030
Schotter, E. R., Tran, R., and Rayner, K. (2014). Don’t believe what you read (only once): Comprehension is supported by regressions during reading. Psychol. Sci. 25, 1218–1226. doi: 10.1177/0956797614531148
Smith, M. C., and Stahl, N. A. (1999). Adults reading practices and activities: Age, educational and occupational effects. New Delhi: Department of Education.
Soemer, A., and Schiefele, U. (2019). Text difficulty, topic interest, and mind wandering during reading. Learn. Instruct. 61, 12–22. doi: 10.1016/j.learninstruc.2018.12.006
Stanovich, K. E. (1989). Matthew effects in reading: Some consequences of individual differences in the acquisition of literacy. Read. Res. Q. 21, 360–407. doi: 10.1177/0022057409189001-204
Torppa, M., Niemi, P., Vasalampi, K., Lerkkanen, M.-K., Tolvanen, A., and Poikkeus, A.-M. (2020). Leisure reading (but not any kind) and reading comprehension support each other longitudinal study across grades 1 and 9. Child Dev. 91, 876–900. doi: 10.1111/cdev.13241
Tulis, M., and Fulmer, S. M. (2013). Students’ motivational and emotional experiences and their relationship to persistence during academic challenge in mathematics and reading. Learn. Individ. Diff. 27, 35–46. doi: 10.1016/j.lindif.2013.06.003
Vallerand, R. J. (2000). Deci and Ryan’s Self-Determination theory: A view from the hierarchical model of intrinsic and extrinsic motivation. Psychol. Inquiry 11, 312–318.
Van Ammel, K., Aesaert, K., De Smedt, F., and Van Keer, H. (2021). Skill or will? The respective contribution of motivational and behavioural characteristics to secondary school students’ reading comprehension. J. Res. Read. 44, 574–596. doi: 10.1111/1467-9817.12356
Vernon, R. F. (2006). Paper or pixels? An Inquiry into how students adapt to online textbooks. J. Soc. Work Educ. 42, 417–427. doi: 10.5175/JSWE.2006.200404104
Weger, U. W., and Inhoff, A. W. (2007). Long-range regressions to previously read words are guided by spatial and verbal memory. Mem. Cogn. 35, 1293–1306. doi: 10.3758/BF03193602
Worthy, J., Patterson, E., Salas, R., Prater, S., and Turner, M. (2001). “More than just reading”: The human factor in reaching resistant readers. Read. Res. Instruct. 41, 177–201. doi: 10.1080/19388070209558364
Keywords: electronic reading, reading behavior, motivation, digital literacy, electronic experience
Citation: Vuorinen PTE, Tatler BW and Keller F (2024) Tracking e-reading behavior: uncovering the effects of task context, electronic experience, and motivation. Front. Educ. 8:1302701. doi: 10.3389/feduc.2023.1302701
Received: 03 October 2023; Accepted: 11 December 2023;
Published: 08 January 2024.
Edited by:
Tom Crick, Swansea University, United KingdomReviewed by:
Mojgan Rashtchi, Islamic Azad University North Tehran Branch, IranCopyright © 2024 Vuorinen, Tatler and Keller. This is an open-access article distributed under the terms of the Creative Commons Attribution License (CC BY). The use, distribution or reproduction in other forums is permitted, provided the original author(s) and the copyright owner(s) are credited and that the original publication in this journal is cited, in accordance with accepted academic practice. No use, distribution or reproduction is permitted which does not comply with these terms.
*Correspondence: Pauliina Tea Eleonoora Vuorinen, cC50LmUudnVvcmluZW5Ac21zLmVkLmFjLnVr
Disclaimer: All claims expressed in this article are solely those of the authors and do not necessarily represent those of their affiliated organizations, or those of the publisher, the editors and the reviewers. Any product that may be evaluated in this article or claim that may be made by its manufacturer is not guaranteed or endorsed by the publisher.
Research integrity at Frontiers
Learn more about the work of our research integrity team to safeguard the quality of each article we publish.