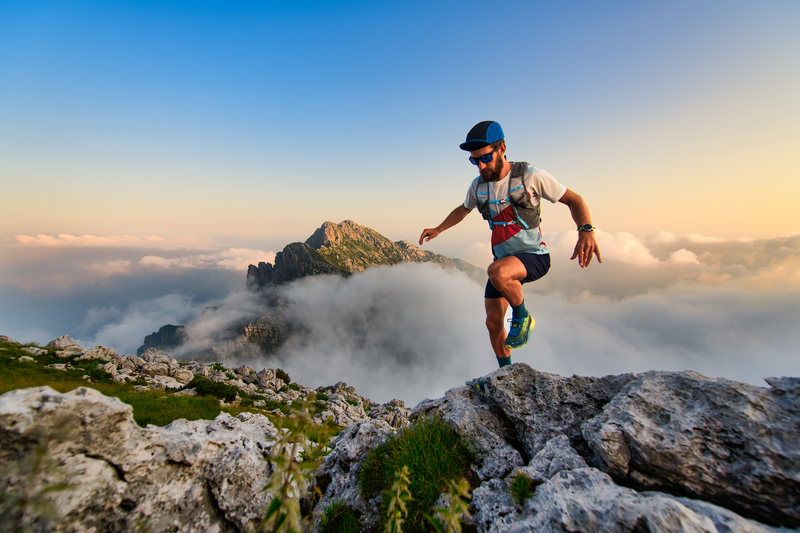
95% of researchers rate our articles as excellent or good
Learn more about the work of our research integrity team to safeguard the quality of each article we publish.
Find out more
ORIGINAL RESEARCH article
Front. Educ. , 09 November 2023
Sec. Digital Education
Volume 8 - 2023 | https://doi.org/10.3389/feduc.2023.1264868
This article is part of the Research Topic Instructional Communication Competence and Instructor Social Presence: Enhancing Teaching and Learning in the Online Environment View all 14 articles
Objective: As changes to higher education following the rapid transition to online learning resulting from the COVID-19 pandemic impacted students and their perceptions of what is possible in scheduling their daily lives around school, this study investigates trends in student access to online asynchronous courses.
Methods: This study utilized course reports of student access from the learning management system for thirty-one sections of eleven different online asynchronous communication courses taught by ten different faculty members over the fall and spring semesters at a large research university in the southwestern United States. A total sample size of 1,201 students were involved in the study.
Results: Profile Analyses indicate clear curvilinear trends for time of day and day of the week in student course access. Repeated Measures ANOVA results indicate those trends vary significantly from a no effect condition, suggesting that students: (a) schedule their course activities around personal schedules, and (b) that time bound synchronous course may not work for them.
Conclusion: Recommendations for class management by instructors relating to student time poverty need regardless of teaching modality, and future directions for research on time poverty in higher education, are provided.
In recent years, researchers have increased interest in student outcomes related to online learning. Although the fields of education, communication and psychology (primarily) as well as scholars in other fields engaged in exploring the characteristic of their teaching strategies have been investigating online education over the past few decades, the rapid transition to online learning due to the COVID-19 pandemic increased interests in this area of study substantially. Within this field of inquiry, scholars have begun investigating student participation and access to asynchronous online courses.
A review of the literature on the subtopic of student online access reveals a large body of research (see Granic, 2022; Stone, 2022). However, very little of this research has focused on time of day or day of the week access to asynchronous online courses given the changes in higher education (see Hachey et al., 2022). More specifically, what has not been so widely studied are the external time constraints which may influence student participation in online learning. This study seeks to initiate and broaden the interests among scholars of education on the time-based characteristics of student online access to higher education.
A systematic search of the literature on online student access through the Web of Sciences database, with no time frame using the search terms “student online access” found 12,610 articles. To reduce this number to a more manageable search, within Web of Sciences, the Highly Cited Papers filters for all years applied to only the fields of education and educational research, social psychology and communication resulted in a total list of 15 articles, the earliest published in 2012. Given the recent changes to online education due to the rapid transition to online learning, the fields and highly cited filters were removed, and the years were restricted to 2022 and 2023 only. This search resulted in 3,648 articles. These articles were reviewed for relevance specific to the purpose of this study, resulting in 21 articles meeting the criteria. Of these articles, six utilized some type of systematic literature search to report on characteristics of student online access, and 17 were empirical studies using student or teacher self-report surveys and/or interviews or public data. These 21 articles covered “student online access” in three mean areas: Online learning (in general), the COVID-19 response, and “time poverty.”
Further expansion on the topic of time poverty involved a second search for literature in Web of Sciences using the keywords “time AND poverty,” with no time frame restriction, resulting in a total of 209 articles, of which an additional fourteen articles were relevant for the specific purpose of this study.
To be sure, student learning requires a commitment of time to the enterprise by students and teachers alike. However, the specific question related to barriers of time for study has up to this point been lightly researched. In a recent study, Hachey et al. (2022) conducted a systematic review of empirical research on ERIC, Education Full Text, and PsychINFO between 2010 and 2021 and found almost no research on the relationship between increased work and family commitments and student outcomes in online and face-to-face courses. The changes in the lives of students (and teachers) during the COVID-19 pandemic brought the issue of time commitment into focus. Many students may have experienced strains to their time for school-work over the last several years due to these changes. Such strains on the time needed to accomplish various goals, including going to school has been termed “time poverty.”
Vickery (1977) first defined time as a second dimension, along with the known dimension of income, that influences poverty. Over the next several decades, researchers have studied both the characteristics and effects of time-poverty. Recently, Giurge et al. (2020) defined time poverty as a persistent sense of lack of time to accomplish needed tasks. According to Mullens and Glorieux (2023), multiple temporal dimensions influence the degree of time wealth and time poverty including total duration of leisure time, share of leisure time during the weekend, fragmentation of leisure time, and subjective time pressure.
Merz and Rathjen (2014) measured interdependent multidimensional poverty (IMD) among the German population which considered the interactional effects of income and time poverty. According to Merz and Rathjen, “families have an increasing IMD poverty risk with an increasing number of children, for single parents in particular and for couples” (Merz and Rathjen, 2014, p. 474). Chatzitheochari and Arber (2012) argued that the construct of time poverty should consider the difference between weekday time and weekend time for typical full-time workers. However, even this differentiation may break down in light of part-time workers or workers with more than one job who may also be working nights and weekends. Regardless, Chatzitheochari and Arber (2012) found that working women have more constraints on their time compared to working men.
These time constraints limit the amount of time available to individuals to pursue long-term goals. Such freely disposable time may be set aside to meet short-term needs. According to Hobbes et al. (2011), freely disposable time may be the best measure for determining the amount of time left over for adults to pursue engagements for investment in the future, such as higher education, after meeting basic needs for themselves and their dependents.
For example, individuals from households with children are more likely to be time-poor than individuals from households without children (Kalenkoski et al., 2011). According to Burchardt, single parents in the UK have considerably more constraints on free time than dual-earner couples, and “those with low educational qualifications, or who are disabled, face particularly pressing constraints” (Burchardt, 2010, p. 339). Additionally, employed mothers experience time pressure due to insufficient time for discretionary activities, need to multitask between work and home responsibilities, and emotional and organizational work (Rose, 2017). In Canada, this time deficit is highest among working single parents (Harvey and Mukhopadhyay, 2007).
Leisure, in and of itself, is not the only potential lost resource due to time poverty. Giurge et al. (2020) claimed that time poverty is associated with health-related consequences including cognitive overload. For example, time-poor individuals spend less time per day on exercise than non-time-poor individuals (Kalenkoski and Hamrick, 2013). According to Zheng et al. (2022), perceived time poverty is strongly and positively associated with physical, mental, and emotional fatigue.
Early in the research on time-poverty, researchers were recommending new technologies to reduce time spent out of the home for travel to work as a mechanism for reducing time poverty. For example, Turner and Grieco (2000) recommended tele-strategies to reduce time poverty for single mothers. These new technologies have provided opportunities for online learning. The advancement of digital learning technologies has occurred alongside the growth of online learning. However, as noted by Hachey et al. (2022), very little research has tied this growth with the construct of time poverty.
In a recent study, Xavier et al. (2022) interviewed students who decided to withdraw from online programs and discovered that time poverty and time-related conflicts were the major contributors to their decision. In many cases, time-related conflicts were due to unrealistic time-management expectations. However, circumstances involving work and family commitments, as well as personal health concerns were the most important contributors.
According to Wladis et al. (2018), students with preschool-aged children have significantly less time for college-related work, and have less quality free time than students with no children. In the US, parents with children under the age of thirteen had significantly less discretionary time and spent more time simultaneously studying and caring for children compared to students without children under the age of thirteen (Conway et al., 2021).
In another study, Wladis et al. (2022) found that students who enrolled in at least one online course had higher rates of time poverty compared to face-to-face only students. However, the authors also found that these online students were also more likely to complete their online courses (Wladis et al., 2022). Additionally, based upon a survey of 120 Australian students, Burston (2017) found a negative correlation between time spent working and semester weighted (grade) averages. The relationship between online enrollment and college credit accumulation is directly mediated by degree of time poverty. These findings suggest that time-management by the online students may be key to their success.
Online learning has transformed over the years from distance learning models such as correspondence courses and remote lectures via closed-circuit television to internet-based asynchronous courses (Kentnor, 2015). In more recent years, the development of communication technology has presented opportunities for changes in course delivery in classroom settings as well as remote settings. Education institutions have embraced multiple delivery modes, even within the same programs, from face-to-face, fully-online synchronous (meeting online at a specified time and day), fully-online asynchronous (no set time and day meeting online), or some hybrid combination which may include some set meeting time and day including face-to-face. Considerable growth in online teaching and learning preceded and continued beyond the COVID-19 pandemic. Current research in student learning outcomes has found focus in the development of online learning (Ulfa and Fatawi, 2021). For example, in a recent study, Smith et al. (2022) found moderate correlations between first access to learning materials and success on exams in online asynchronous courses.
Recently, Granic (2022) conducted a systematic review of articles from Web of Science between 1996 and 2022 regarding student online participation and found that student participation was enhanced through online technologies including discussion forums, blogs, chats, and personalized communication. As previously stated, online learning may refer to different modalities, including hybrid or blended models. According to Dziuban et al. (2018), blended learning which incorporates qualities of traditional face-to-face instruction with advanced information communication technologies increases student perception of learning while increasing access.
Regardless, the development of online learning has engendered new questions about student participation and the consequences to learning outcomes. For example, Stone (2022) argued that evidence from the literature demonstrates that online learning enhances student equity and increases access to higher education. However, such trends may be influenced by teaching strategies within the online environment. According to Barrot and Fernando (2023), teachers’ misuse of teaching strategies can have a significant impact on students’ ability to navigate online courses. Indeed, instructors can have a profound effect on learning outcomes by utilizing student-centered techniques.
Such focus by instructors on student-centered techniques may also influence student participation. Panigrahi et al. (2018) argued that virtual learning communities can increase student engagement in online learning with resulting positive learning outcomes. These results for students may also be positive for teachers. Often, teacher self-efficacy in online environments correlates with student participation, and in the online environment that participation equates with internet access (Mustafa et al., 2022).
Many factors are thought to influence positive student learning outcomes. According to Lu et al. (2023), cognitive engagement is the only factor which influences intentionality toward continuous usage of asynchronous online courses, where intrinsic and extrinsic motivation and perception of multiple sources had little to no direct effect. However, barriers to student learning may have the opposite effect. Demir Kaymak and Horzum (2022) applied the learning barriers identified by Muilenburg and Berge (2001) to online learning environments and found that time and support for studies, among other barriers, most strongly predicted perceived learning by students. They also found that both gender and job status influenced academic achievement and perceived learning.
Instructors’ approaches to these barriers may also have negative consequences. Barrot and Fernando (2023) found that students’ time management issues were exacerbated by instructors who used the same course management for assessment in their online classes as they did in their face-to-face classes. Technology-based strategies, for example, can mitigate these trends. For example, utilizing a self-paced online learning orientation module increases student intent to keep up to date with course materials in an online asynchronous course (Mshayisa and Ivala, 2022).
Other barriers to student participation and success may be outside the instructor’s control. Roessger et al. (2022) using county-level data from 1999 through 2018 demonstrated that proximity to a university is positively correlated with adult participation in higher education, although this relationship was not influenced by a growth in online learning opportunities. Time management may be one of the most important skills for students in online courses (Cox et al., 2022). Time management may be a function of social and environmental conditions outside of the online course. For example, Hachey et al. (2022) found ten factors that influence student participation in online learning including non-traditional status, family responsibilities, employment, and socio-economic status. These particular factors may contribute to pressures on time management for class participation.
The COVID-19 pandemic of 2020–2022 is an extreme example of an environmental condition outside of the control of students and faculty that created barriers to student learning. According to Adedoyin and Soykan (2020), emergency remote teaching due to the COVID-19 pandemic is distinct from planned online learning. Technological advances in course delivery leading to online delivery methods had been occurring well before the rapid transition brought on by the worldwide COVID-19 response. However, that response illuminated shortcomings of online delivery for students of lower socio-economic status who did not have ready access to the internet (Adedoyin and Soykan, 2020). Similarly, Asanov et al. (2021) found that a significant percentage of (Ecuadorian high school) students did not have both internet access and a computer at home during the pandemic.
Response to the environmental conditions of the pandemic also had consequential effects on student learning. Tang et al. (2020) found that students were generally dissatisfied with online learning in the context of the rapid transition to online delivery modes during the COVID-19 pandemic. The authors also found that students were highly dissatisfied with the communication modes provided by instructors during the pandemic (Tang et al., 2020).
Yet some students and faculty were prepared, having previously experienced and participated in online learning prior to the pandemic. In particular, Wang et al. (2022) found that readiness to participate in online learning was highly associated with academic success during the COVID-19 pandemic. Interestingly, both students and faculty used similar strategies to overcome the challenges they faced with the rapid transition to online learning in the midst of the COVID-19 pandemic (Barrot and Fernando, 2023).
During the COVID-19 pandemic students perceived more free time and more flexibility in their course work due to online learning, while at the same time reporting financial problems due to unemployment and the uncertainty about the future as stressors (Kohls et al., 2021). In one study, Asanov et al. (2021) surveyed students about their time-use and found that many students developed a daily routine to do school-work during the pandemic.
Given the current research on student time-related access to online asynchronous courses, the following research questions are proposed:
H1: There is a statistically significant difference between trends in time of the day for student access to online asynchronous courses compared to a no time effect for student access.
H2: There is a statistically significant difference between trends in day of the week for student access to online asynchronous courses compared to a no day of the week effect for student access.
The study procedures were reviewed by the local Institutional Review Board in January 2023 (IRB FY22-23-158) and determined the study did not meet requirements for federally regulated research, was exempt from human subjects’ protections and required no further IRB oversight.
The participants were 1,201 students in 31 distinct sections of 11 different Communication courses from 1000-level (n = 30 courses, N = 1181 students) through 5000-level (n = 1 course, N = 20 students) and their instructors (n = 10) at a large research extensive university in the southwestern United States. The average class size was 38.74 students (sd = 20.75, minimum = 4, maximum = 79). Courses included sections taught Fall 2021 through Spring 2023. All sections of courses were taught utilizing an asynchronous online modality.
All instructors within the department who taught online asynchronous courses were requested via email to participate in the study. Instructors participating in the study were asked to supply student access data from the Learning Management System (LMS) Blackboard (see Ulfa and Fatawi, 2021; Smith et al., 2022). Specifically, faculty were asked to produce reports from the LMS which showed student access to the course by time and day for an eight-week period of the semester (end of the Fall, or beginning of the Spring), to de-identify the students, and submit the reports as Excel files for each individual course section. The LMS course report requested was the “Overall Summary of User Activity.” The time-frame for the report was limited to an eight-week period to account for the difference between the residential program (16-week semester) and the online program (8-week quarter) course sections.
Data for each section includes the time-of-day access rounded to the nearest hour on a twenty-four-hour basis and day of the week access for each student in each section, bounded by 12:00 am (midnight) U.S. Central Standard Time. The data may include, though is not identified by the LMS, multiple “hits” by a single student within a given time-frame due to logging out and logging back in within the time boundaries. The data from each section were parsed and transposed within Excel, then combined with as a row (case) with all other sections to create the dataset for use in the analysis of overall trends of time and day access among all sections of asynchronous online Communication courses.
To determine if significant differences could be attributed to level of student (undergraduate versus graduate), several diagnostic tests were conducted. First, a Kolmogorov–Smirnov (K-S) test was conducted on each subgroup to determine if the normality of distribution criteria was met. For hour of the day data, the K-S score for undergraduate students was 0.150 (p < 0.001, Lilliefors corrected), and the K-S score for graduates was 0.152 (p = 0.160, Lilliefors corrected). For day of the week data, the K-S score for undergraduate students was 0.066 (p = 0.028, Lilliefors corrected), and the K-S score for graduates was 0.232 (p = 0.200, Lilliefors corrected). Given the small subsample size and lack of normality for graduate students in both datasets, a Welch t-Test was conducted between groups for each hour and each day independently (see Welch, 1947). The results are presented in Tables 1 2. To account for differences in class sizes for both hour and day datasets, all access hit data were log-transformed (see West, 2022).
Table 1 demonstrates that for each hour in which a significant difference was found between undergraduate students’ and graduate students’ access hits, undergraduates consistently accessed online course materials more often than graduate students. A test for homogeneity of regression slopes revealed that the regression slopes for undergraduate and graduate per hour access hits did not intersect [F(1, 740) = 0.198, p = 0.657]. Therefore, the undergraduate and graduate student access hit by hour data were analyzed together.
Table 2 demonstrates that for each day in which a significant difference was found between undergraduate students’ and graduate students’ access hits, undergraduates consistently accessed online course materials more often than graduate students. A test for homogeneity of regression slopes revealed that the regression slopes for undergraduate and graduate per hour access hits did not intersect [F(1, 213) = 0.775, p = 0.380]. Therefore, the undergraduate and graduate student access hit by day data were analyzed together.
Data were analyzed using Repeated Measures Analysis of Variance in SPSS 28, following the guidelines for Profile Analysis as described by Cengiz et al. (2021). In Profile Analysis, the three criteria to be met to determine trends in longitudinal data include: (1) Parallelism of trend lines, (2) group equality, and (3) profile flatness. Prior to hypothesis testing, to visualize potential trends in the hit counts of student access in the LMS by hour of the day and day of the week as differing from no effect (demonstrated by a horizontal line), plots were generated by treating hit count as the dependent variable, and either hour of day (H1) or day of the week (H2) as the independent variable. Figure 1 shows the trend between student access and hour of the day for H1.
This plot shows a (non-flat) curvilinear trend with peak access times occurring in the late evening with a peak in the hour between 8 pm and 10 pm. This omnibus trend carries across all sections of all courses measured. Figure 1 indicates a possible variance from no effect, justifying a test of Hypothesis 1. A regression was utilized to determine the strength of the prediction between the hour of day and access hits, assuming a curvilinear relationship (see Robitzsch, 2020). Results indicate that the quadratic (curvilinear) regression model is more predictive of student’s access by day of the week, R2 = 0.807, F(2, 22) = 84.40, p < 0.001, B = 2588.55, compared to the linear model, R2 = 0.793, F(2, 21) = 43.99, p < 0.001, B = 3985.1, although the difference between the linear and quadratic regression lines may not be significant (see Figure 2).
To test H1, a Repeated measures ANOVA model was constructed between the student access hits as the experimental (H1) variable and a horizontal intercept as the control (H1) both in a longitudinal structure over an hour of the day time series (non-parallel) for each hour over a 24 h period, for the eight-week study time-frame. Repeated measures ANOVA is appropriate for testing differences between groups of complex timed responses (Kumar et al., 2013; Macey et al., 2016). A horizontal intercept can be view as a stationarity with statistical characteristics which are invariant over time (see Nemec, 1996). The horizontal line (y = k) was constructed by using the mean and standard deviation of the experimental (H1) variable (M = 32911.25, sd = 20551.33) across all 24 h in the day.
The hits by hour (experimental H1) variable was slightly skewed (−0.373), and a one sample Kolmogorov–Smirnov test revealed the distribution to be non-normal (0.200, Lilliefors corrected, p = 0.014, see Lilliefors, 1967). To account for this, both the experimental and control variables were log transformed (see West, 2022). Repeated measures ANOVA was conducted between the log transformed experimental and control variables. Between-subjects tests revealed a significant difference between student course access hits by hour of the day and the no time-effect control, F(1, 23) = 5609.21, p < 0.001, partial η2 = 0.996. These results support the first hypothesis.
For H2, the number of student access hits was plotted against day of the week (see Figure 3). This plot also shows a curvilinear trend with peak access times occurring on Sunday with a smaller secondary peak occurring on Thursday. This omnibus trend carries across all sections of all courses measured.
Figure 3 indicates a possible variance from no effect, justifying a test of Hypothesis 2. A regression was utilized to determine the strength of the prediction between the day of the week and student access hits, assuming a curvilinear relationship. Results indicate that the quadratic (curvilinear) regression model is more predictive of student’s access by day of the week, R2 = 0.822, F(2, 4) = 9.26, p = 0.032, B = −49817.27, compared to the linear model, R2 = 0.684, F(1, 5) = 10.83, p = 0.022, B = −16195.61, although the difference between the linear and quadratic regression lines may not be significant (see Figure 4).
To test H2, a Repeated Measures ANOVA model also was constructed between the hits by day of the week as the second experimental (H2) variable and a horizontal line as the control (H2) for each day over a seven-day period, for the eight-week study time-frame. The hits by day (experimental H2) variable was highly skewed (−1.927), and a one sample Shapiro–Wilk test revealed the distribution to be non-normal (0.781, p = 0.026, see Shapiro et al., 1968).
The horizontal line (y = k) was constructed by using the mean and standard deviation of this experimental variable (M = 112838.57, sd = 42297.86) across all 7 days of the week, then each variable was log transformed. Between-subjects tests revealed a significant difference between student course access by day and the no day-effect control, F(1, 6) = 10483.96, p < 0.001, partial η2 = 0.999. These results support the second hypothesis.
In general, a pattern emerges for student online access to asynchronous Communication courses by both day and time. Given the number of discreet sections (n = 31), unique courses (n = 11), unique instructors (n = 10), and number of students (N = 1,201), evidence suggests that time of course access by students is a factor by both day and time. The ratio of graduate students to undergraduate students in the study was 3.39%. In comparing hours of the day accessed by graduate students to hours of the day accessed by undergraduate students, no clear pattern of difference emerged. For access hits based on hour of the day, the ratio of access hits between graduate students and undergraduate students varied little from a minimum of 0.13% at 6 am to a maximum of 2.56% at 6 pm (M = 1.30%, sd > 0.01). For access hits based on day of the week, the ratio of access hits between graduate students and undergraduate students varied little from a minimum of 0.78% on Friday to a maximum of 2.99% on Thursday (M = 1.55%, sd > 0.01).
More interestingly, although patterns that emerged did demonstrate that particular days and times were more active, students accessed these courses at all hours of the day across all 7 days of the week. None of the 961 cells in the student access data were empty. As revealed in the analysis, peak times fell on certain days of the week and hours of the day. Follow-up with faculty who provided the data uncovered a trend to require assignments to be turned in at 11:59 pm on Sundays for 4 faculty members in 15 class sections, and at 11:59 pm on Mondays for 1 faculty member in one class section. Other faculty members did not report back or reported requiring assignments to be turned in over multiple days of the week (1 faculty member for six class sections). Regardless, these patterns seem to suggest that students enrolled in asynchronous online courses take advantage of time flexibility that may not be available to them in a residential face-to-face course, synchronous online course, or hybrid course where some aspect of the course happens at a set time. These findings comport with previous research on time poverty in higher education (see Wladis et al., 2018, 2022; Conway et al., 2021; Xavier et al., 2022).
Given emerging research interests in student time-management in the current climate, these findings provide urgency to considerations of educators and education scholars regarding issues of time-poverty among college students. The current need for research on the topic of time-poverty among college students was demonstrated in this article, it is important to note that the approach to data gathering for this study is currently unique in the research literature. Other studies found and reported here utilized self-report through survey or interview rather than actual student access “hit” data. The existence of such “hit” data in the Learning Management Systems used to manage college courses, including non-asynchronous courses, can be used to measure and verify statistical trends across large populations of students.
This study is not without limitations. Although this study involved multiple sections of differing level courses, these courses were still within a single department. Additionally, the current study did not control for distinctions between residential students in an online class and online program students. These populations may have different characteristics not measured here. For example, it could be that the residential students are taking a one-off online course to balance their schedule, whereas online program students take all of their classes online, and may have completed a more robust online program orientation.
Additionally, the study utilized LMS course access data. The LMS does not collect demographic data in its reports, only access records by student name. Further, to meet IRB requirements, the data had to be de-identified eliminated any possibility of cross-checking access record by student against student academic records which may include demographic data. As such, this study may not provide an opportunity to generalize asynchronous online course access by student demographic characteristics.
Other limitations to the methodology include not testing whether a given instructor specified a particular day of the week, or time of the day, as a deadline for submitting assignments. Given the course and instructor sample sizes, such analyses may not have been appropriate. It is important to note that of the ten instructors who participated in the data collection from their courses, only six (60%) specified which day of the week they requested for assignments to be submitted, with four requesting Sunday (only) submission, one requesting Monday (only) submission, and one allowing submission multiple days of the week depending on the assignment. Faculty who attended prior training in best practices for online asynchronous may have selected strategies that differed from those who did not, but these data were not collected for the study.
Additionally, because the data were collected for two semesters and between eight-week and sixteen-week courses (although all were tested over an eight-week period), the start and end dates may have influenced the outcome of the second hypothesis test. The data indicate that the start day for data collection, based upon the course start day could have been one of 5 days with the most common start day being Monday (41.9%) and the least common being Friday (6.5%, mean percentage = 20.0%). The end days of data collection were based upon the last day students accessed to course between all of the sections, which included all 7 days of the week. The peaks in the trend lines may be accounted for by these types of instructor strategies or variances in the data.
Despite these limitations, the current study points a direction for future study of the effects of time-poverty on student access to online courses. The current study also demonstrates the necessity for educators to consider the lives of their students outside of their classes when designing the course.
The original data and analysis code presented in the study are included in the article/Supplementary material, further inquiries can be directed to the corresponding author.
The studies involving human data were approved by Shannon Marquess, Senior Research Compliance Coordinator of The University of Texas at San Antonio [P: (210) 458-4793, E: U2hhbm5vbi5NYXJxdWVzc0B1dHNhLmVkdQ==]. The studies were conducted in accordance with the local legislation and institutional requirements. Written informed consent for participation was not required from the participants or the participants’ legal guardians/next of kin in accordance with the national legislation and institutional requirements.
HPL: Conceptualization, Data curation, Formal analysis, Investigation, Methodology, Project administration, Writing – original draft, Writing – review & editing.
The author declares that no financial support was received for the research, authorship, and/or publication of this article.
I wish to thank Anuradha Roy of the Department of Management Science and Statistics at the University of Texas at San Antonio for her helpful recommendations regarding the analysis of the data for this study. I also wish to acknowledge the faculty in the Department of Communication at the University of Texas at San Antonio who provided data from their classes for this study. An earlier version of this paper with preliminary data was presented at the 34th annual conference of the International Academy of Business Disciplines, New Orleans, LA, March 30, 2023.
The author declares that the research was conducted in the absence of any commercial or financial relationships that could be construed as a potential conflict of interest.
All claims expressed in this article are solely those of the authors and do not necessarily represent those of their affiliated organizations, or those of the publisher, the editors and the reviewers. Any product that may be evaluated in this article, or claim that may be made by its manufacturer, is not guaranteed or endorsed by the publisher.
The Supplementary material for this article can be found online at: https://www.frontiersin.org/articles/10.3389/feduc.2023.1264868/full#supplementary-material
Adedoyin, O. B., and Soykan, E. (2020). COVID-19 pandemic and online learning: the challenges and opportunities. Interact. Learn. Environ. 31, 863–875. doi: 10.1080/10494820.2020.1813180
Asanov, I., Flores, F., McKenzie, D., Mensmann, M., and Schulte, M. (2021). Remote-learning, time-use, and mental health of Ecuadorian high-school students during the COVID-19 quarantine. World Dev. 138:105225. doi: 10.1016/j.worlddev.2020.105225
Barrot, J. S., and Fernando, A. R. R. (2023). Unpacking engineering students' challenges and strategies in a fully online learning space: the mediating role of teachers. Educ. Inform. Technol. 28, 9803–9825. doi: 10.1007/s10639-023-11598-8
Burchardt, T. (2010). Time, income and substantive freedom: a capability approach. Time Soc. 19, 318–344. doi: 10.1177/0961463x10369754
Burston, M. A. (2017). I work and don't have time for that theory stuff: time poverty and higher education. J. Furth. High. Educ. 41, 516–529. doi: 10.1080/0309877x.2015.1135885
Cengiz, C., von Rosen, D., and Singull, M. (2021). Profile analysis in high dimensions. J. Stat. Theory Pract. 15, 1–14. doi: 10.1007/s42519-020-00154-z
Chatzitheochari, S., and Arber, S. (2012). Class, gender and time poverty: a time-use analysis of British workers' free time resources. Br. J. Sociol. 63, 451–471. doi: 10.1111/j.1468-4446.2012.01419.x
Conway, K. M., Wladis, C., and Hachey, A. C. (2021). Time poverty and parenthood: who has time for college? AERA Open 7:233285842110116. doi: 10.1177/23328584211011608
Cox, W., Alexander, A., West, H., Abzhaparova, A., and Hill, J. (2022). How to thrive when studying online. J. Geogr. High. Educ. 47, 502–511. doi: 10.1080/03098265.2022.2087214
Demir Kaymak, Z., and Horzum, M. B. (2022). Student barriers to online learning as predictors of perceived learning and academic achievement. Turk. Online J. Distance Educ. 23, 97–106. doi: 10.17718/tojde.1096250
Dziuban, C., Graham, C. R., Moskal, P. D., Norberg, A., and Sicilia, N. (2018). Blended learning: the new normal and emerging technologies. Int. J. Educ. Technol. High. Educ. 15:16. doi: 10.1186/s41239-017-0087-5
Giurge, L. M., Whillans, A. V., and West, C. (2020). Why time poverty matters for individuals, organisations and nations. Nat. Hum. Behav. 4, 993–1003. doi: 10.1038/s41562-020-0920-z
Granic, A. (2022). Enhancing online participation in education: quarter century of research. J. Comput. Educ. 10, 663–687. doi: 10.1007/s40692-022-00238-8
Hachey, A. C., Conway, K. M., Wladis, C., and Karim, S. (2022). Post-secondary online learning in the US: an integrative review of the literature on undergraduate student characteristics. J. Comput. High. Educ. 34, 708–768. doi: 10.1007/s12528-022-09319-0
Harvey, A. S., and Mukhopadhyay, A. K. (2007). When twenty-four hours is not enough: time poverty of working parents. Soc. Indic. Res. 82, 57–77. doi: 10.1007/s11205-006-9002-5
Hobbes, M., de Groot, W. T., van der Voet, E., and Sarkhel, S. (2011). Freely disposable time: a time and money integrated measure of poverty and freedom. World Dev. 39, 2055–2068. doi: 10.1016/j.worlddev.2011.04.005
Kalenkoski, C. M., and Hamrick, K. S. (2013). How does time poverty affect behavior? A look at eating and physical activity. Appl. Econ. Perspect. Policy 35, 89–105. doi: 10.1093/aepp/pps034
Kalenkoski, C. M., Hamrick, K. S., and Andrews, M. (2011). Time poverty thresholds and rates for the US population. Soc. Indic. Res. 104, 129–155. doi: 10.1007/s11205-010-9732-2
Kentnor, H. E. (2015). Distance education and the evolution of online learning in the United States [Report]. Curric. Teach. Dial. 17, 21–34. Available at: https://digitalcommons.du.edu/cgi/viewcontent.cgi?referer=&httpsredir=1&article=1026&context=law_facpub
Kohls, E., Baldofski, S., Moeller, R., Klemm, S. L., and Rummel-Kluge, C. (2021). Mental health, social and emotional well-being, and perceived burdens of university students during COVID-19 pandemic lockdown in Germany. Front. Psych. 12:11. doi: 10.3389/fpsyt.2021.643957
Kumar, V., Mehta, P., and Shukla, G. (2013). Multivariate analysis of repeated measures data. J. Reliab. Statist. Stud. 6, 133–148. Available at: https://journals.riverpublishers.com/index.php/JRSS/article/view/21901
Lilliefors, H. W. (1967). On the Kolmogorov-Smirnov test of normality with means and variance unknown. J. Am. Stat. Assoc. 62, 399–402. doi: 10.1080/01621459.1967.10482916
Lu, K. L., Pang, F., and Shadiev, R. (2023). Understanding college students' continuous usage intention of asynchronous online courses through extended technology acceptance model. Educ. Inf. Technol. 19, 1–19. doi: 10.1007/s10639-023-11591-1
Macey, P. M., Schluter, P. J., Macey, K. E., and Harper, R. M. (2016). Detecting variable responses in time-series using repeated measures ANOVA: application to physiologic challenges [version 2; peer review: 2 approved]. F1000Research 5:563. doi: 10.12688/f1000research.8252.2
Merz, J., and Rathjen, T. (2014). Time and income poverty: an interdependent multidimensional poverty approach with German time use diary data. Rev. Income Wealth 60, 450–479. doi: 10.1111/roiw.12117
Mshayisa, V. V., and Ivala, E. N. (2022). No student left behind: students' experiences of a self-paced online learning orientation in undergraduate studies during COVID-19 pandemic. Educ. Sci. 12:15. doi: 10.3390/educsci12060386
Muilenburg, L., and Berge, Z. L. (2001). Barriers to distance education: a factor-analytic study. Am. J. Distance Educ. 15, 7–22. doi: 10.1080/08923640109527081
Mullens, F., and Glorieux, I. (2023). Not enough time? Leisure and multiple dimensions of time wealth. Leis. Sci. 45, 178–198. doi: 10.1080/01490400.2020.1805656
Mustafa, F., Weda, S., and Masykar, T. (2022). Correlation between student internet access and teachers' self-efficacy in teaching online classes. Int. J. Emerg. Technol. Learn. 17, 99–119. doi: 10.3991/ijet.v17i10.25819
Nemec, A. F. L. (1996). “Analysis of repeated measures and time series: an introduction with forestry examples” in Biometrics information handbook no. 6 (Victoria, BC: British Columbia Ministry of Forests). Available at: https://www.for.gov.bc.ca/hfd/pubs/docs/wp/wp15.htm
Panigrahi, R., Srivastava, P. R., and Sharma, D. (2018). Online learning: adoption, continuance, and learning outcome-a review of literature. Int. J. Inf. Manag. 43, 1–14. doi: 10.1016/j.ijinfomgt.2018.05.005
Robitzsch, A. (2020). Why ordinal variables can (almost) always be treated as continuous variables: clarifying assumptions of robust continuous and ordinal factor analysis estimation methods. Front. Educ. 5:589965. doi: 10.3389/feduc.2020.589965
Roessger, K. M., Weese, J., Parker, D. A., and Hevel, M. S. (2022). Bridging the distance? How proximity and online learning shape communities' adult participation in public universities. J. Contin. High. Educ. 71, 199–214. doi: 10.1080/07377363.2021.2024001
Rose, J. (2017). “Never enough hours in the day”: employed mothers' perceptions of time pressure. Aust. J. Soc. Iss. 52, 116–130. doi: 10.1002/ajs4.2
Shapiro, S. S., Wilk, M. B., and Chen, H. J. (1968). A comparative study of various test of normality. J. Am. Stat. Assoc. 63, 1343–1372. doi: 10.1080/01621459.1968.10480932
Smith, D., Pasieka, A., Becker, R., and Perdikoulas, C. (2022). Student success in asynchronous STEM education: measuring and identifying contributors to learner outcomes. 2022 IEEE Global Engineering Education Conference (EDUCON), Tunis, Tunisia 473–479
Stone, C. (2022). From the margins to the mainstream: the online learning rethink and its implications for enhancing student equity. Australas. J. Educ. Technol. 38, 139–149. doi: 10.14742/ajet.8136
Tang, T., Abuhmaid, A. M., Olaimat, M., Oudat, D. M., Aldhaeebi, M., and Bamanger, E. (2020). Efficiency of flipped classroom with online-based teaching under COVID-19. Interact. Learn. Environ. 31, 1077–1088. doi: 10.1080/10494820.2020.1817761
Turner, J., and Grieco, M. (2000). Gender and time poverty: the neglected social policy implications of gendered time, transport and travel. Time Soc. 9, 129–136. doi: 10.1177/0961463x00009001007
Ulfa, S., and Fatawi, I. (2021). Predicting factors that influence student’s learning outcomes using learning analytics in online learning environment. Int. J. Emerg. Technol. Learn. 16, 4–17. doi: 10.3991/ijet.v16i01.16325
Vickery, C. (1977). The time-poor: a new look at poverty. J. Hum. Resour. 12, 27–48. doi: 10.2307/145597
Wang, Y. R., Xia, M. Y., Guo, W. J., Xu, F. J., and Zhao, Y. D. (2022). Academic performance under COVID-19: the role of online learning readiness and emotional competence. Curr. Psychol. 14, 1–14. doi: 10.1007/s12144-022-02699-7
Welch, B. L. (1947). The generalization of Student’s problem when several different population variances are involved. Biometrika 34, 28–35. doi: 10.1093/biomet/34.1-2.28
West, R. M. (2022). Best practices in statistics: the use of log transformation. Ann. Clin. Biochem. 59, 162–165. doi: 10.1177/00045632211050531
Wladis, C., Hachey, A. C., and Conway, K. (2018). No time for college? An investigation of time poverty and parenthood. J. High. Educ. 89, 807–831. doi: 10.1080/00221546.2018.1442983
Wladis, C., Hachey, A. C., and Conway, K. (2022). Time poverty: a hidden factor connecting online enrollment and college outcomes? J. High. Educ. 94, 609–637. doi: 10.1080/00221546.2022.2138385
Xavier, M., Meneses, J., and Fiuza, P. J. (2022). Dropout, stopout, and time challenges in open online higher education: a qualitative study of the first-year student experience. Open Learn. 17, 1–17. doi: 10.1080/02680513.2022.2160236
Keywords: teaching modalities, student access, course management, learning management systems, time poverty
Citation: LeBlanc HP III (2023) Time and day: trends in student access to online asynchronous courses in communication demonstrate time poverty in action. Front. Educ. 8:1264868. doi: 10.3389/feduc.2023.1264868
Received: 24 July 2023; Accepted: 17 October 2023;
Published: 09 November 2023.
Edited by:
Patrick R. Lowenthal, Boise State University, United StatesReviewed by:
Kleopatra Nikolopoulou, National and Kapodistrian University of Athens, GreeceCopyright © 2023 LeBlanc. This is an open-access article distributed under the terms of the Creative Commons Attribution License (CC BY). The use, distribution or reproduction in other forums is permitted, provided the original author(s) and the copyright owner(s) are credited and that the original publication in this journal is cited, in accordance with accepted academic practice. No use, distribution or reproduction is permitted which does not comply with these terms.
*Correspondence: H. Paul LeBlanc, III cGF1bC5sZWJsYW5jQHV0c2EuZWR1
Disclaimer: All claims expressed in this article are solely those of the authors and do not necessarily represent those of their affiliated organizations, or those of the publisher, the editors and the reviewers. Any product that may be evaluated in this article or claim that may be made by its manufacturer is not guaranteed or endorsed by the publisher.
Research integrity at Frontiers
Learn more about the work of our research integrity team to safeguard the quality of each article we publish.