- 1Sociology Discipline, Social Science School, Khulna University, Khulna, Bangladesh
- 2Department of Urban and Regional Planning, Jahangirnagar University, Dhaka, Bangladesh
Introduction: A persistent gender divide in digital competence is visible empirically in both developed and developing countries. But there is not a single study in the context of Bangladesh, as per the author’s best knowledge. This study, therefore, was designed to find out the gender divide in the digital competence of university students with reference to socioeconomic background.
Methods: This cross-sectional study was carried out in a public university of Bangladesh, where data were collected from 1,059 students using a semi-structured interview schedule, where digital competence was measured by computer application usage (CAU) and computer self-efficacy (CSe), with overall reliability of 0.840 and 0.960, respectively. Data were analyzed using IBM SPSS Statistic v25, and one-way analysis of variance (ANOVA) and t-test were used to determine the differences between students regarding digital competence.
Results: Findings from ANOVA suggested that older students, in terms of age (p < 0.001 for CAU and p < 0.001 for CSe) and levels of education (p < 0.001 for CAU and p < 0.001 for CSe), were more digitally competent. Likewise, students of Management and Business school (p < 0.001 for CAU and p < 0.001 for CSe) and from higher SES (p < 0.001 for CAU and p < 0.001 for CSe) were better off in digital competence. Regarding the gender divide, it is apparent that male students, irrespective of age (p < 0.001 for CAU and p < 0.001 for CSe), levels of education (p < 0.001 for CAU and p < 0.001 for CSe), school (p < 0.001 for CAU and p < 0.001 for CSe), and SES (p < 0.001 for CAU and p < 0.001 for CSe), were more digitally competent than their female counterparts.
Conclusion: It is, therefore, strongly recommended to educators and policymakers to reduce long-established gender stereotypes by implementing gender-specific training and educational guidelines to create a generation of knowledgeable and skillful workforce.
1. Introduction
Digital competence, an ability to efficiently use technical tools for learning, working, and participating in society (Vázquez-Cano et al., 2020), has become an essential component of education system in contemporary world (Höyng, 2022) as no or low digital efficacy negatively affect the learning process (Hasan and Bao, 2020). Following the COVID-19 pandemic, numerous improvements, including integrated online learning platforms, access to digital devices, greater coverage of internet network, have been introduced to enhance digital teaching and learning process, despite some problems (Almaiah et al., 2020; Hossain et al., 2022). The usage of digital resources boosts the development of new skills, such as use of codes and symbols to communicate digital information via communication networks (Gómez and Almenara, 2013), that has a direct impact on academic achievement (Mehrvarz et al., 2021). However, to facilitate successful digital pedagogies for teaching and learning, all students and teachers should be digitally competent (Barboutidis and Stiakakis, 2023), which is subject to various socioeconomic and politico-cultural issues, including human, financial and social capital (Scholes et al., 2022b).
Over the years, there has been a stereotype regarding technology use, and in a gender comparison toward technology, females might have a more unfavorable attitude toward technology use as they are less actively engaged in technical activities, which have contributed to the “technological gender gap” (Cai et al., 2017). There is no denial to the fact that gender divide in digital competence is a major obstacle to the comprehensive development of citizenship in the 21st-century (Gómez-Trigueros, 2023), and in South Asia, women are much less likely than men to use digital devices. In Bangladesh, for example, less than 6% households had a computer and less than 5% had access to the internet, while women were the marginal consumers of computer, internet as well as mobile phone (Bangladesh Bureau of Statistics, 2015; Jeffrie, 2022). Family disapproval was listed as one of the top three obstacles preventing women in Pakistan and Bangladesh from owning access to the digital devices (Cai et al., 2017). Gender inequality also existed in terms of access to and ownership of devices, that hindered the digital fluency and the ability to use technology meaningfully (Mariscal et al., 2019). Gender role views, which hold that women are presumed to be less capable of and interested in technology than males, have been mentioned as a key factor in these inequalities as well (Sobieraj and Krämer, 2020). In fact, it has become widely accepted that male students perform better than their female counterparts because they have more favorable attitudes toward technology and its use and have higher ICT self-efficacy (Jackson et al., 2008).
Gender gaps in digital competence are becoming more pronounced, especially in societies with patriarchal culture; therefore, compelling studies are needed to create appropriate learning environments and pedagogies that would equalize opportunities for all students (Almasri, 2022). Although there are numerous studies in both developed and developing countries examining the gender divide in digital competence with reference to socioeconomic issues; there is none in the context of Bangladesh, as per the author’s best knowledge. In order to comprehend the gender gap in digital competence, the current study was designed to initially assess the digital divide with reference to their socioeconomic backgrounds, and later to examine the role of gender in digital divide among university students. The current study is the first to provide information on the gender gap in digital competence among university students of Bangladesh, which may prove critical for future policymaking and implications.
2. Theoretical framework and literature review
There is no denial of the fact that disparities between gender regarding digital competence are prevalent across the world, both in developed and developing countries (Vázquez-Cano et al., 2020). Studies conducted in different countries outlined numerous factors, including social, economic, structural as well as cultural, that contributed substantially to widening the disparities between gender regarding digital competence. There are, however, a handful of theories that could identify the potential issues, and the “social capital theory” by Coleman (1988) is one of them. For Coleman (1988), none can achieve or attain any skill or excellence without existing capitals within a social structure, and he delineated capital further into human, financial, and social capital. Human capital denotes the “skills and capabilities” of individuals to shape the desired change in actions, while financial capital refers to the “tangible resources” generally measured by wealth or income or socioeconomic status, and social capital includes specific “social elements” that facilitate not only actions and interactions but also produce different behavior and outcomes for individuals (Coleman, 1988; Sumi et al., 2021).
Applying Coleman’s (1988) “social capital theory,” an attempt has been made to comprehend how human (age, level of education, school), financial (SES) and social (gender) capitals are shaping the gender divide in digital competence among university students in the context of Bangladesh (see Figure 1). Studies related to the digital divide indicate that the most important factor affecting the technical competence of individuals is gender. A study, exploring the digital competence of both male and female students, i.e., search engines, web browsers, and digital cartography, found that male students were relatively better than their female counterparts in digital cartography and online presentation (Vázquez-Cano et al., 2017). Another study indicated that male students in vocational schools were more competent in problem-solving, information and data literacy, as well as safety (Wild and Schulze Heuling, 2020). Likewise, Vishnu et al. (2022) observed that male students showed greater digital content creation, safety, and problem-solving skills. On the contrary, Aytekin and Isiksal-Bostan (2019) noted a higher positive attitude among female students toward technology in mathematics learning compared to male students, whereas He and Zhu (2017) found a similar attitude and personal innovativeness toward technical information and learning among male and female students. Shala and Grajcevci (2018) found, however, no significant impact of gender on the digital competence of university students.
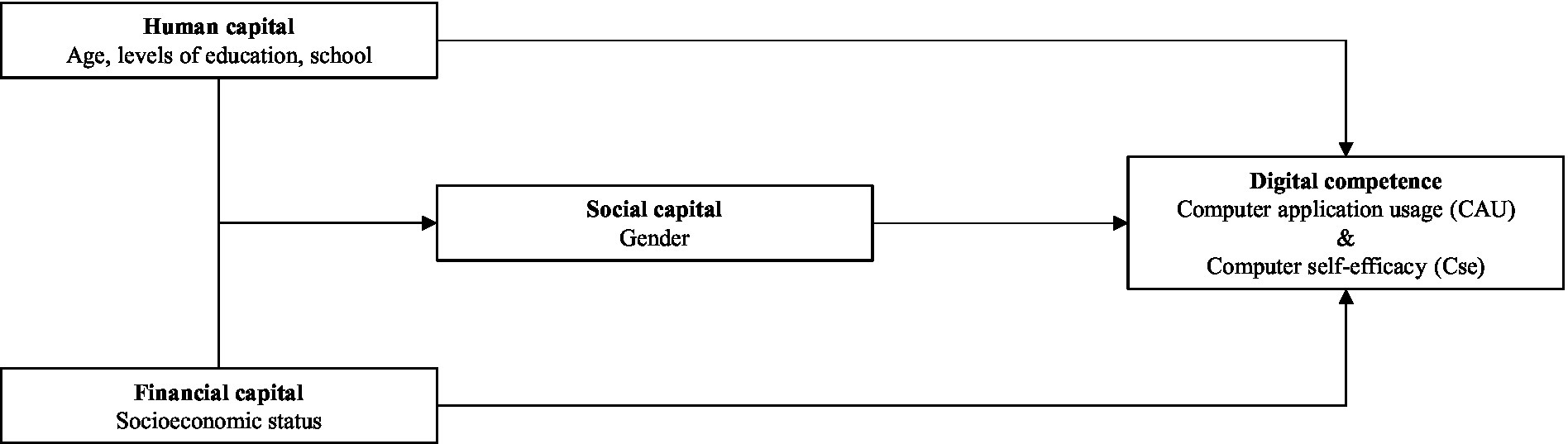
Figure 1. Conceptual framework based on the interpretation of Coleman (1988).
Age is also an important factor that substantially defines the digital divide. Barboutidis and Stiakakis (2023), for example, found that older students in vocational training schools exhibited better digital competence compared to their younger counterparts. Synnott et al. (2020), on the contrary, observed a reduction in digital skills among older students and workers. Like age, education evidently is another factor that determines digital skills. Barboutidis and Stiakakis (2023) and Wild and Schulze Heuling (2020), in their respective studies, found a significant difference between students at the university and vocational training in technology usage. Vishnu et al. (2022) also observed that post-graduate and doctoral students showed relatively higher computer-based application skills than their first-year compatriots. Apart from age and education, socioeconomic status (SES) plays a crucial role in the digital competence of individuals. Yuen et al. (2016) observed that students from higher SES, e.g., parental education, financial conditions, and technology ownership, in Hong Kong have greater access to and use of computer and ICT and exhibited better skills. A study in Kosovo suggested that students from lower SES, struggling financially, showed the lowest IT skills, whereas students belonging to the highest SES with greater economic wellbeing reported greater IT skills (Shala and Grajcevci, 2018). These studies concluded that digital competence is subject to the overall SES of individuals as people from higher SES have more access to advanced technologies than their contemporaries from lower SES, thereby, the former exhibited more technical competence than the latter (Yuen et al., 2016; Vishnu et al., 2022).
3. Materials and methods
3.1. Study settings, participants, and procedure
This cross-sectional study was carried out at a public university in the southwestern region with a reputation of academic excellence in higher education promoting quality and equitable education and research for all years (see Map 1). This university started with only four Disciplines with less than a hundred students in 1991, is now providing education for more than 7,000 students enrolled in undergraduate and post-graduate programs in 29 Disciplines under eight Schools (Bangladesh University Grants Commission, 2021), where the university authority pays considerable attention to enhancing the digital competence of its graduates through technological aids in off-campus and on-campus education. For this study, some specifications were made to identify the participants: i.e., (i) the participant must be a current student in the selected university; (ii) enrolled in a regular undergraduate program; (iii) not a Term or Year repeater; and (iv) must be a Bangladeshi citizen. Based on the criteria mentioned above, the data enumerators – a group of well-trained second-year students of a Discipline with extensive knowledge about the research objectives and the research tools – approached over 1,200 students from all 29 Disciplines under eight Schools and a total of 1,059 students participated voluntarily, and all the responses were retained in this study after scrutiny. The data were collected conveniently by administering a semi-structured interview schedule (SSIS) – an instrument to carry out survey research through telephone or in face-to-face situation (Bryman, 2012; Neuman, 2014), divided into seven mutually exclusive modules, focusing on personal attributes and socioeconomic background as well as assessing digital competence and anxiety among university students for three consecutive weeks in March 2021, starting from 9 March and ending on 25 March, and each interview lasted for 25 min, on average. It is important to note that the interviews were conducted during the lunch break or after class sessions without interrupting the regular academic activities of the participants.
3.2. Ethical issues
The institutional ethical clearance committee approved this study (Reference No. KUECC – KUECC-2023-03-16). The participants responded to the interviews voluntarily by filling out a written informed consent letter in the first section of the SSIS, confirming their anonymity and right to withdraw. The data enumerators initially briefed the participants about the purpose and procedure of participating in the study. The data enumerators started the face-to-face interview following verbal and written consent from the participants. The participants were free to decline the survey at any moment without any prior justification.
3.3. Measures
3.3.1. Background information
Some specific factors, i.e., age (in year; 18–20, 21–23, 24–26), gender (male and female), education (in year; first year, second year, third year, fourth year), school (arts and humanities school [AHS], science, engineering and technology school [SETS], life science school [LSS], social science school [SSS], law school [LS], management and business administration [MBAS], fine arts school [FAS], education school [ES]), and socioeconomic status (lower, middle, higher).
3.3.2. Socioeconomic status
Socioeconomic status (SES) is generally measured by a person’s social and financial position concerning their educational background, professional record, and financial capacity. In educational research, however, some opinions vary among researchers regarding the components of SES. For example, Snyder et al. (2009) suggested a composite of five elements, i.e., father’s education, occupation, mother’s education, family income, and other household items, to explain the SES of an individual. In contrast, Hollingshead (1975) suggested four factors, e.g., education, occupation, sex, and marital status, to refer to the SES of an individual. In this study, the SES was measured by a composite of parental education [“0” = not literate, “1” = primary (Class I – Class V), “2 = secondary (Class VI – Class X),” “3 = higher secondary (Class XI – Class XII),” “4 = honors/degree (Class XIII – Class XVI),” “5 = master (Class XVII – Class XVIII)”] and the father’s occupation (“0 = disable/deceased,” “1 = agricultural/informal/manual workers,” “2 = entrepreneurs/businessmen,” “3 = government/non-government service,” “4 = professionals”) and income (“0 = no income,” “1 = BDT 1–20,000,” “2 = 20,001–40,000,” “3 = ≥40,001”), as suggested by Sumi et al. (2022). The aggregated score generated for SES was later divided into lower (≤6), middle (7–12), and higher (≥13) SES.
3.3.3. Computer application usage
Computer application usage (CAU) was a 16-item index developed by Noiwan et al. (2005) on a five-point Likert scale ranging from “1 = never” to “5 = every day.” In this study, however, the score for the five-point Likert scale was re-assigned, i.e., “0 = never” to “4 = every day,” and the overall reliability of the CAU was Cronbach’s α = 0.840.
3.3.4. Computer self-efficacy
Computer self-efficacy (CSe) was a 19-item index adapted from Ertmer et al. (1994) and Khan et al. (2011) on a five-point Likert scale ranging from “1 = strongly disagree” to “5 = strongly agree.” In this study, however, the score for the five-point Likert scale was re-assigned, i.e., “0 = strongly disagree” to “4 = strongly agree,” and the overall reliability of the CSe was Cronbach’s α = 0.960.
3.4. Analysis
In three consecutive stages, this study analyzed data using IBM SPSS software version 25 for Windows. The descriptive statistics (frequency and percentage) showed the participants’ background information in the first phase. In the second phase, a one-way independent analysis of variance (ANOVA, F-test) was executed to determine statistically significant differences between different age, education, school, and SES groups regarding CAU and CSe. However, when the assumptions of Levene’s test for homogeneity of variance were violated, Welch’s robust test of equality of means (W-test) were reported instead of the F-test to rectify the degree of heterogeneity (Pallant, 2011; Field, 2013; Glantz et al., 2016). For post-hoc analysis, Tukey HSD and Games-Howell were reported. In the final phase, the student’s t-test was executed to determine the differences between male and female university students regarding CAU and CSe concerning their age, education, school, and SES. In case of violating the assumptions of Levene’s test for equality of variances in the t-test, the outcomes of “equal variance not assumed” were reported (Pallant, 2011; Field, 2013). For both the F-test and t-test, the effect size, eta-squared (η2) for the former and Cohen’s d for the latter, was performed and reported as per the recommendation of Cohen (1988).
4. Results
4.1. Background information
Table 1 shows the background information of the participants. It is apparent that more than 60% of the students were between the age bracket of 21–23 years, while around 36% of the participants were second-year students during the survey. Overall, more than a quarter percent of the participants (25.2%) were students of LSS, while 26% of the male students were from SETS and 25.9% of the female students were from LSS. Around half of the total participants (48.7%) belonged to middle SES, whereas more male students (35.6%) reportedly were from lower SES than their female counterparts (18.5%).
4.2. Differential impact of age, education, school, and SES on CAU and CSe
One-way independent ANOVA was conducted to compare the differential impact of age, education, school, and SES in the CAU and CSe (see Table 2). It is apparent that there was a significant impact of age on CAU [F(2, 1,056) = 95.453; p < 0.000; η2 = 0.43] and CSe [W(2, 1,056) = 118.595, p < 0.000; η2 = 0.47]. The data showed that younger students had relatively lower digital competence (M = 30.1, SD = 8.85 [CAU]; M = 27.0, SD = 20.17 [CSe]) compared to the students in the age bracket of 21–23 (M = 36.8, SD = 9.88 [CAU]; M = 45.9, SD = 19.37 [CSe]) and 24–26 (M = 44.5, SD = 10.53 [CAU]; M = 55.1, SD = 16.18 [CSe]), respectively, and the post-hoc analyses, using Tukey HSD for CAU and Games-Howell for CSe, showed that differences between the age groups among the students regarding their digital competence were statistically significant.
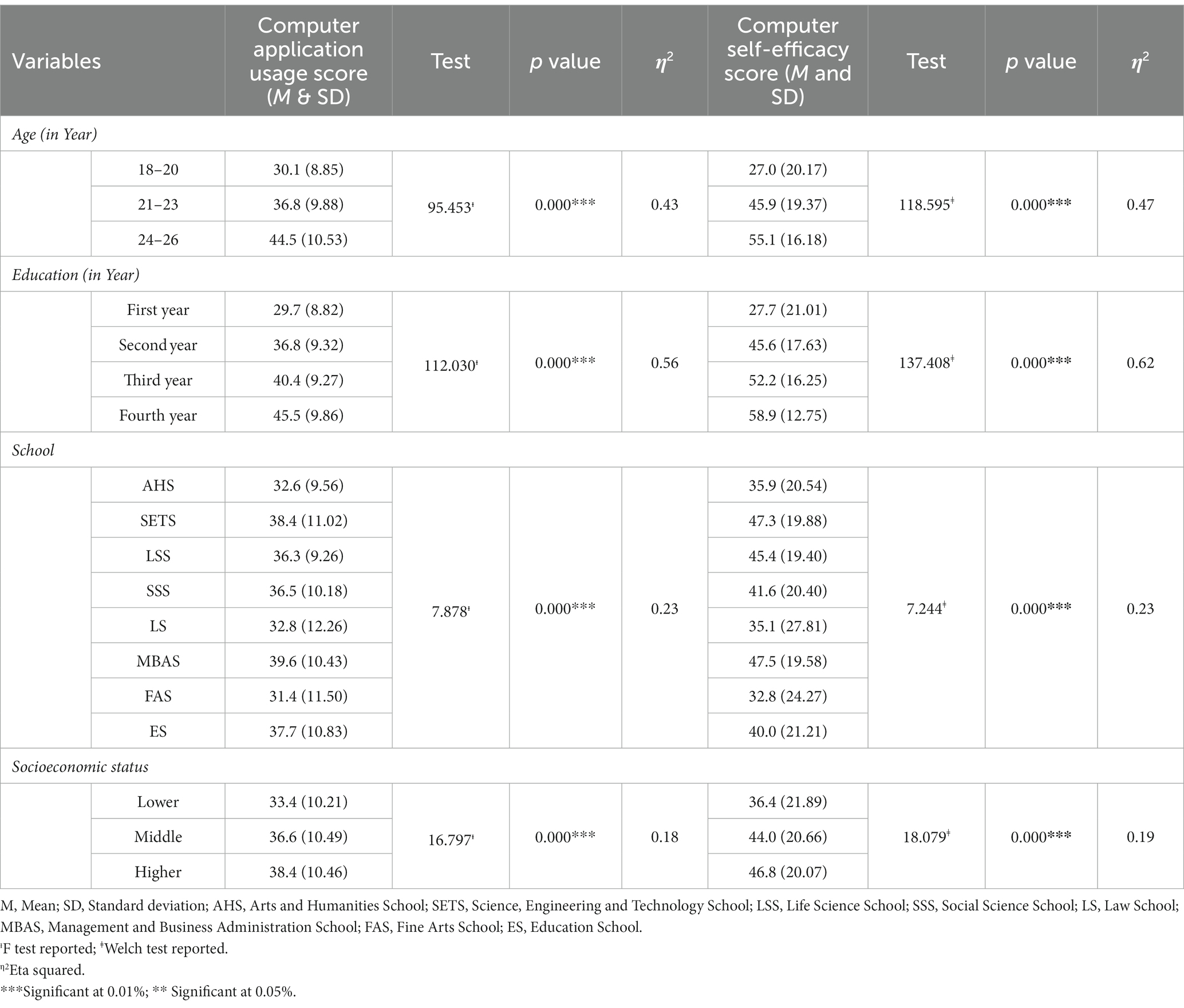
Table 2. Variations in computer application and computer self-efficacy score by age, education, school and socioeconomic status.
Likewise, it is also evident that education had a significant impact on the digital competence of university students, i.e., CAU [F(3, 1,055) = 112.030; p < 0.000; η2 = 0.56] and CSe [W(3, 1,055) = 137.408; p < 0.000; η2 = 0.63]. Findings showed that students in the fourth year were digitally more competent (M = 45.5, SD = 9.86 [CAU]; M = 58.9, SD = 12.75 [CSe]) than their younger counterparts, e.g., third year (M = 40.4, SD = 9.27 [CAU]; M = 52.2, SD = 16.24 [CSe]), second year (M = 36.8, SD = 9.31 [CAU]; M = 45.6, SD = 17.63 [CSe]), and first year (M = 29.7, SD = 8.81 [CAU]; M = 27.7, SD = 21 [CSe]), respectively. The post-hoc analyses, using Tukey HSD for CAU and Games-Howell for CSe, showed that differences between the education levels among the students regarding their digital competence were statistically significant.
Regarding the influence of school on digital competence, it appeared that digital competence among students of different schools varied significantly, i.e., CAU [F(7, 1,051) = 7.787; p < 0.000; η2 = 0.23] and CSe [W(7, 1,051) = 7.244; p < 0.000; η2 = 0.23]. Findings indicate that students of MBAS were digitally more competent (M = 39.6, SD = 10.43 [CAU]; M = 47.5, SD = 19.58 [CSe]) than students of other schools, particularly that of LS (M = 32.8, SD = 12.26 [CAU]; M = 35.1, SD = 27.81 [CSe]) and FAS (M = 31.4, SD = 11.50 [CAU]; M = 32.8, SD = 24.27 [CSe]). The post-hoc analyses of Tukey HSD (CAU) and Games-Howell (CSe) suggest that there are statistically significant differences between the students at various schools regarding their digital competence.
It is also found that the digital competence among students from different SES varied significantly, e.g., CAU [F(2, 1,056) = 16.797; p < 0.000; η2 = 0.18] and CSe [W(2, 1,056) = 18.079; p < 0.000; η2 = 0.19]. It is apparent that students from higher SES (M = 38.4, SD = 10.46 [CAU]; M = 46.8, SD = 20.07 [CSe]) were digitally more competent than students from middle SES (M = 36.6, SD = 10.5 [CAU]; M = 44.0, SD = 20.66 [CSe]) and lower SES (M = 33.4, SD = 10.21 [CAU]; M = 36.4, SD = 21.89 [CSe]). Moreover, the post-hoc analyses, i.e., Tukey HSD for CAU and Games-Howell for CSe, also indicate that the differences among students from different SES regarding CAU and CSe were statistically significant.
4.3. Differential impact of gender on CAU and CSe concerning age, education, school, and SES
Following the one-way independent ANOVA to explore the differential impact of age, education, school, and SES on CAU and CSe, this study attempted to extend analysis further by examining the relationship between gender with CAU and CSe, based on age, education, school and SES, using student’s t-test (see Figures 2, 3). Findings indicate that male students showed higher CAU compared to their female counterparts in all three age categories (see Figure 2A), i.e., 18–20 (M = 31.9, SD = 7.70 for male versus M = 29.1, SD = 9.26 for female, p = 0.023, d = 0.32), 21–23 (M = 39.0, SD = 10.06 for male versus M = 34.6, SD = 9.20 for female, p < 0.000, d = 0.45), and 24–26 (M = 45.8, SD = 10.66 for male versus M = 41.4, SD = 9.66 for female, p = 0.031, d = 0.43). Likewise, it is also found that male students scored higher in CSe compared to female students (see Figure 3A) in all, e.g., 18–20 (M = 30.6, SD = 19.37 for male versus M = 25.2, SD = 20.37 for female, p = 0.045, d = 0.28) and 21–23 (M = 48.6, SD = 20.07 for male versus M = 43.1, SD = 18.23 for female, p < 0.000, d = 0.29), but for the age bracket of 24–26 years (M = 56.4, SD = 15.89 for male versus M = 52.3, SD = 16.70 for female, p > 0.202, d = 0.25).
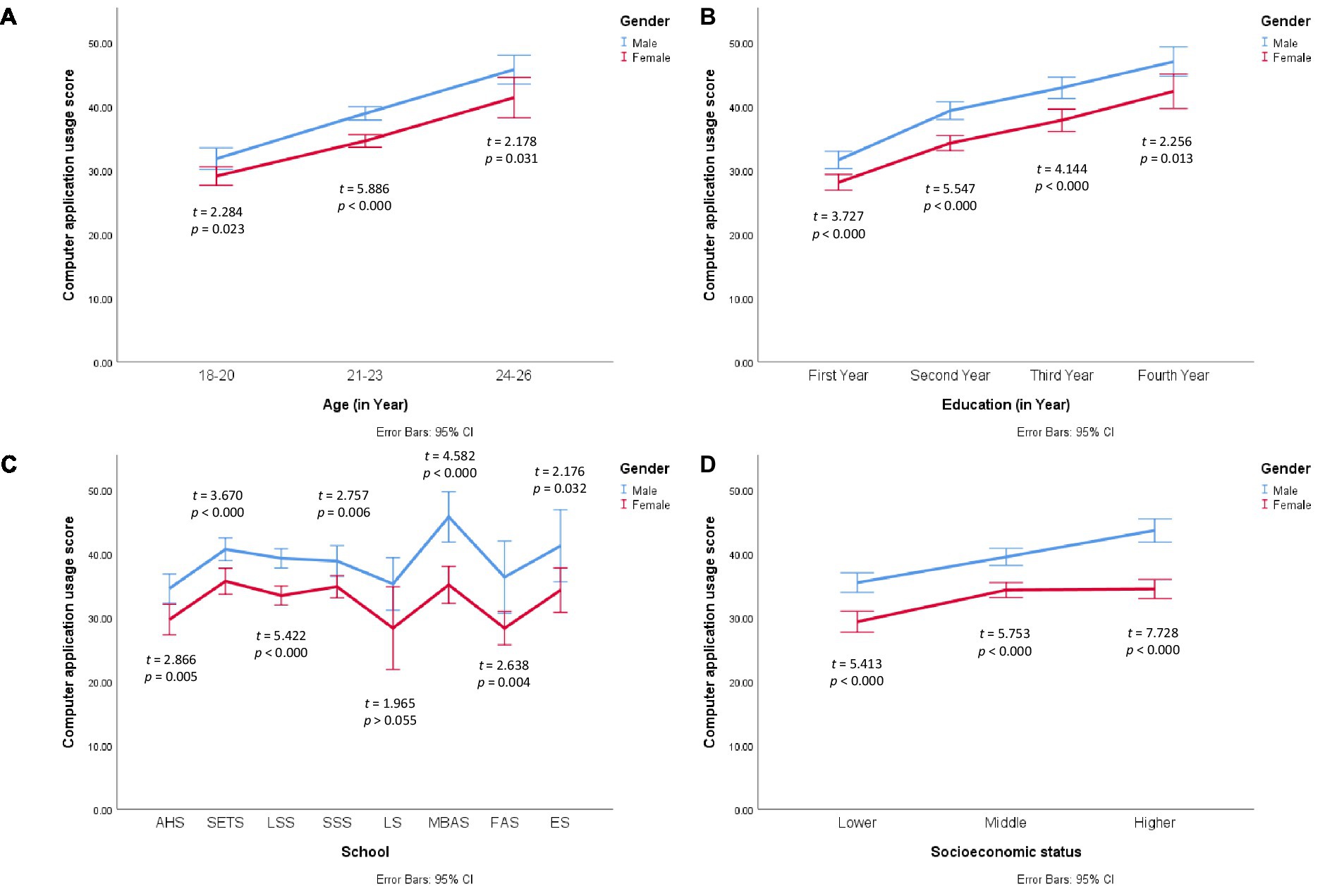
Figure 2. Line plots showing mean and 95% CIs for gender-related differences in computer application usage across (A) age, (B) education, (C) school, and (D) socioeconomic status.
Findings further showed that male students, irrespective of their levels of education, scored higher in CAU and CSe than their female counterparts. For example, male students in the first year (M = 31.7, SD = 8.52), second year (M = 39.4, SD = 9.52), third year (M = 43.0, SD = 8.59), and fourth year (M = 47.1, SD = 10.13) scored higher in CAU compared to their female compatriots in the first year (M = 28.2, SD = 8.76), second year (M = 34.3, SD = 8.42), third year (M = 37.9, SD = 9.25) and fourth year (M = 42.4, SD = 8.65), and the differences between the gender among the levels of education were statistically significant with small to moderate effect size (see Figure 2B), i.e., first year (p < 0.000, d = 0.41), second year (p < 0.000, d = 0.57), third year (p < 0.000, d = 0.57), and fourth year (p = 0.013, d = 0.49). Regarding the CSe score (see Figure 3B), it is also found that male students showed greater self-efficacy than female students across the levels of education, and the differences between gender were statistically significant with small to moderate effect size, e.g., first year (M = 31.2, SD = 21.23 for male versus M = 24.9, SD = 20.46 for female, p = 0.006, d = 0.30), second year (M = 50.0, SD = 17.34 for male versus M = 41.5, SD = 16.91 for female, p < 0.000, d = 0.50), third year (M = 54.9, SD = 16.05 for male versus M = 49.7, SD = 16.11 for female, p = 0.021, d = 0.38), and fourth year (M = 60.7, SD = 12.77 for male versus M = 55.5, SD = 12.14 for female, p = 0.032, d = 0.42).
Regarding the gender differences in CAU and CSe with reference to school (see Figures 2, 3C), it is evident that there were significant differences between male and female students regarding CAU and Cse in all schools, except LS (M = 35.3, SD = 11.21 for male versus M = 28.4, SD = 13.05 for female, p > 0.055, d = 0.59 in CAU, and M = 39.3, SD = 27.58 for male versus M = 27.8, SD = 27.44 for female, p > 0.155, d = 0.42 in CSe). In fact, the gender differences with medium to large effect size to in CAU and CSe were relatively more prevalent among students of MBAS (M = 45.8, SD = 9.75 for male versus M = 35.2, SD = 8.53 for female, p < 0.000, d = 0.93 in CAU, and M = 56.7, SD = 15.21 for male versus M = 40.9, SD = 19.90 for female, p = 0.001, d = 0.89 in CSe), LSS (M = 39.3, SD = 8.60 for male versus M = 33.5, SD = 8.97 for female, p < 0.000, d = 0.67 in CAU, and M = 50.5, SD = 18.28 for male versus M = 40.8, SD = 19.29 for female, p < 0.000, d = 0.51 in CSe), and SETS (M = 40.7, SD = 10.48 for male versus M = 35.7, SD = 11.07 for female, p < 0.000, d = 0.46 in CAU, and M = 50.0, SD = 19.28 for male versus M = 44.0, SD = 20.17 for female, p = 0.018, d = 0.30 in CSe), while a similar scenario was also observed in other schools, including SSS, AHS, FAS and ES; however, the gender difference in CSe for the latter school was not statistically significant (M = 44.8, SD = 22.95 for male versus M = 35.6, SD = 20.00 for female, p > 0.163, d = 0.43).
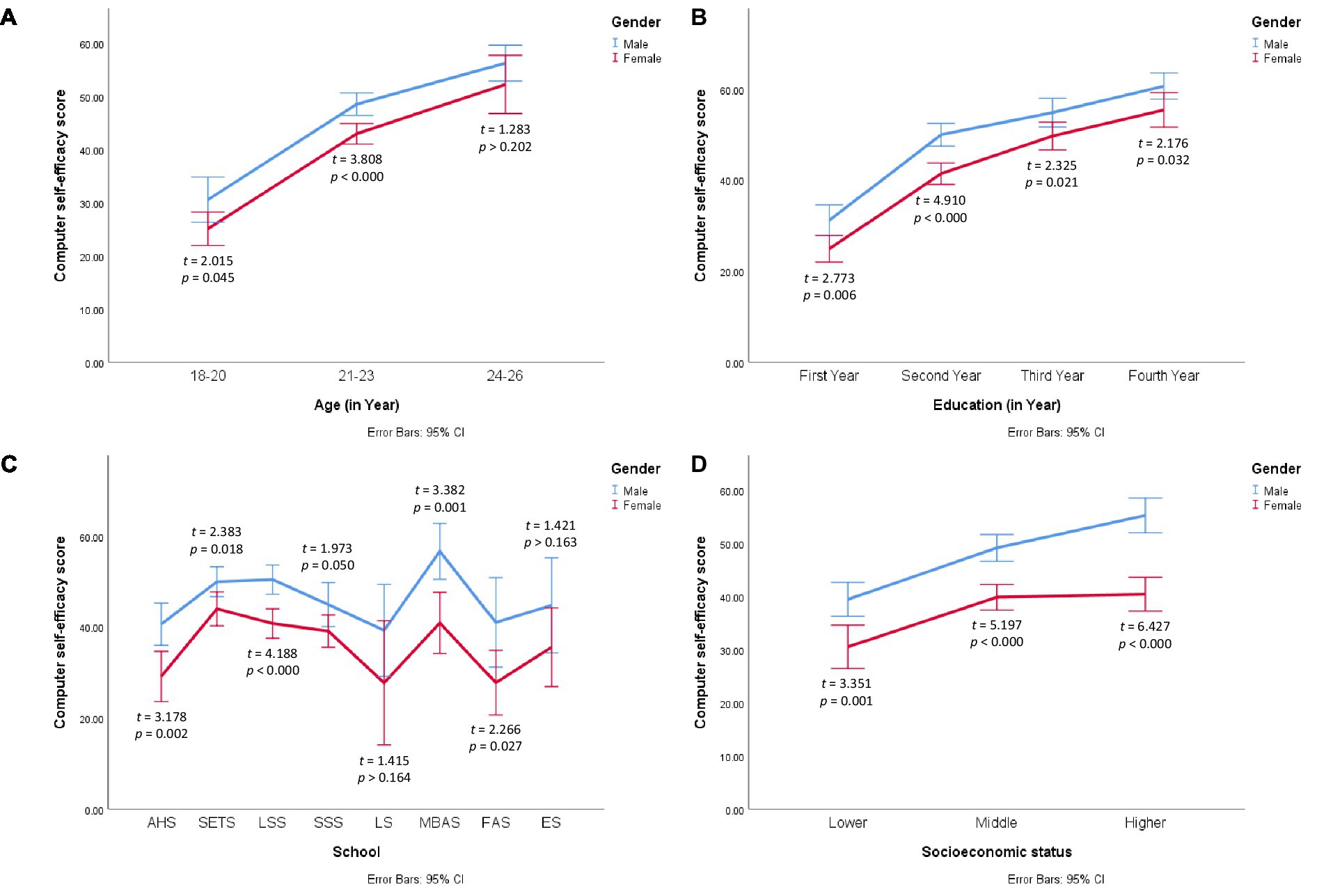
Figure 3. Line plots showing mean and 95% CIs for gender-related differences in computer self-efficacy across (A) age, (B) education, (C) school, and (D) socioeconomic status.
In addition, the results also indicated that significant gender differences in CAU and CSe also existed among SES (see Figures 2D, 3D). For example, male students were more technically competent than females in lower (M = 35.1, SD = 10.52 for male versus M = 29.4, SD = 8.29 for female, p < 0.000, d = 0.65 in CAU, and M = 39.5, SD = 22.01 for male versus M = 30.6, SD = 20.53 for female, p < 0.000, d = 0.42 in CSe), middle (M = 39.6, SD = 10.16 for male versus M = 34.4, SD = 10.19 for female, p < 0.000, d = 0.51 in CAU, and M = 49.2, SD = 19.24 for male versus M = 39.9, SD = 20.84 for female, p < 0.000, d = 0.46 in CSe), and higher (M = 43.7, SD = 9.58 for male versus M = 34.5, SD = 9.33 for female, p < 0.000, d = 0.97 in CAU, and M = 55.4, SD = 17.24 for male versus M = 40.5, SD = 19.72 for female, p < 0.000, d = 0.80 in CSe) SES in CAU and CSe, and the differences were statistically significant with small to large effect size.
5. Discussion
Digital technology has impacted how we live as information and communication technology (ICT) spreads quickly around the globe (Zhang and Zhu, 2016). The internet and computers have replaced traditional teaching and learning instruments as the primary means of production and dissemination of knowledge (Gialamas et al., 2013). Recent studies are paying more attention to informal digital learning in higher education because it is a new trend among university students in the contemporary digital era (Chan et al., 2015; Huang and Oh, 2016). Additionally, in informal learning situations, young people are building digital competence that aligns with crucial cognitive processes of digital learning (He and Zhu, 2017). Thus, the present study, considering the “social capital theory” by Coleman (1988), aimed to assess the digital competence of university students and to explain the gender divide in digital competence along with their socioeconomic backgrounds. Findings of the current study reveal that human (age, level of education, school), and financial (SES) capitals significantly influenced the digital competence, measured by CAU and CSe. Besides, results also depicted that social capital (i.e., gender) also played a decisive role in digital competence as male students had higher CAU and CSe irrespective of their age, level of education, school and SES compared to their female counterparts.
5.1. Differential impact of age, education, school, and SES on CAU and CSe
Consistent with the findings of prior studies (Li and Ranieri, 2010; Martzoukou et al., 2022), the present study reported a significant impact of age on CAU and CSe. The data showed that younger students had relatively lower digital competence than those in the age bracket of 21–23 years and 24–26 years, respectively. This could be illustrated by the fact that higher-grade students are more familiar with using different digital technologies in their educational environment than younger students. Similarly, Appel (2012) reported in his study that higher-grade students possessed better theoretical and practical computer knowledge than younger students. In contrast, a study conducted among Chinese university students and found that young people are developing digital competence that corresponds to important cognitive processes of digital learning (He and Zhu, 2017). However, other studies have not found age to be a significant factor in digital competence (Napal Fraile et al., 2018; Vega-Gea et al., 2021).
Likewise, it is evident that the education level significantly impacted the digital competence of university students, i.e., CAU and CSe. Findings showed that students in the fourth year were digitally more competent than their younger counterparts which confirms the findings of existing literature (Huang and Fang, 2013; Vishnu et al., 2022) that the level of education is a key determinant of the learners’ digital competence. Additionally, prior research discovered that post-graduate and doctorate students had higher mean scores with significant differences than graduate students (Yu, 2021; Vishnu et al., 2022). This could be explained by the fact that students’ prior everyday participation as a digital citizen was connected to several critical academic skills, such as the ability to identify information in different contexts, students’ digital learning and development, their digital abilities to complete academic work, their information, literacy skills and their skills around managing their digital wellbeing and identity (Martzoukou et al., 2022).
Regarding the influence of schools on digital competence, it appeared that digital competence among students at different schools varied significantly. Findings indicate that students of MBAS were digitally more competent than students at other schools, particularly LS and FAS. This might be interpreted that the availability and application of ICT equipment in MBAS fosters students’ digital competence. Previous studies also reported that the availability of ICT equipment in school and the frequent use of ICT in teaching-learning foster students’ digital competence (Fraillon et al., 2014; Martzoukou et al., 2022). Besides, a study conducted in England (Martzoukou et al., 2022) to assess the digital competence of university law students found that law school graduates lack digital skills and suggest that students are more actively engaged with digital technologies.
It was also found that the digital competence among students from different SES varied significantly. It is apparent that students from higher SES were digitally more competent than students from middle SES and lower SES. This finding is congruent with the studies conducted in China (Ren et al., 2022) Kerala, India (Vishnu et al., 2022), and Florida, USA (Hohlfeld et al., 2013; Ritzhaupt et al., 2013). On the contrary, Scholes et al. (2022b) found that SES and digital technology were inversely related, with students in lower-SES categories possessing significantly higher digital skills than those in higher-SES categories.
5.2. Differential impact of gender on CAU and CSe concerning age, education, school, and SES
Gender stereotype regarding digital competence is prevalent both in developed and developing countries which is evident in existing literature. Some studies found that males tended to have higher digital competence compared to female learners (Wild and Schulze Heuling, 2020; Vishnu et al., 2022), whereas other studies found higher levels of digital competence among females than males (Aesaert and van Braak, 2015; Scherer and Siddiq, 2019). However, in this study an attempt has been made to find out which dimension of gender divide in digital competence was prevalent among university students in the context of Bangladesh, and it is found that male students scored higher in CAU and CSe compared to female students and this finding is supported by another study that found males tend to have a greater inclination toward digital technologies compared to females (Kuhlemeier and Hemker, 2007). Additionally, girls’ lower digital self-efficacy is consistent with their self-reported digital competencies, which were found in earlier studies (Laakso et al., 2021; Scholes et al., 2022b). Girls’ lower usage of computer applications could be attributed to the social and cultural environment that promotes gender stereotypes in which girls are discursively set up as subordinate in relation to boys within the digital skills (Fisher and Jenson, 2017; Jenson and de Castell, 2018).
Findings further showed that male students, irrespective of their levels of education and school, scored higher in CAU and CSe than their female counterparts. Similarly, previous studies also reported that male students exhibit higher levels of confidence and self-efficacy in relation to digital technologies compared to female students (Whitton, 2014; Scholes et al., 2022a,b). This phenomenon could be explained by the vicious cycle that reinforces girls’ lack of time, effort, interest, and skill development in digital technologies. The frequency with which girls use digital devices and how they view their digital skills may have an impact on how engaged they remain in digital spaces (Scholes et al., 2022b) and contribute to the digital skills gap.
In addition, the results also indicated that significant gender differences in CAU and CSe also existed among different SES. For example, male students were more technically competent, in CAU and CSe, than females in lower and higher SES. This might be explained by the fact that the gender norms of Bangladesh favor and motivate males to engage more in digital technology usage (Islam and Inan, 2021). Another study also confirmed these gender norms that children as young as six years old support the stereotype that girls are less interested in computing and digital experiences than boys (Master et al., 2021). On the contrary, a previous study found that higher-SES males had the propensity to rate their digital skills below their lower-SES male counterparts. In addition, female students from higher SES were more likely than male students to report lower digital skills (Scholes et al., 2022b).
Overall, findings of the present study supported the long-established gender stereotypes regarding digital competence and showed that male students have higher digital competence than female students at tertiary education in Bangladesh. To reduce the gender divide in digital competence, female students need more drive and confidence to use digital technologies such as computers (Schlebusch, 2018) and a variety of incentive tactics should be taken into account to encourage and motivate female students to become interested in and comfortable using computers (Yoon et al., 2016) or digital technologies in Bangladesh.
6. Strengths and limitations
The study has certain limitations, which the readers might consider when generalizing the findings. For example, the reliance on face-to-face interview to assess the digital competence of university students may lead to response bias due to interference of the interviewers. The use of a non-probability sampling approach, the selection of participants from a single university, and the study’s cross-sectional nature may also constrain the inferences drawn. Yet, the major contribution of this study is reporting the digital divide between gender in the context of university students in Bangladesh using globally standardized and validated tools, which are rarely sampled in such studies. However, more empirical research, both qualitative and quantitative, with nationally representative samples are needed in all four levels of education in Bangladesh, including primary, secondary, higher secondary, and tertiary, to find out the prevalence and determinants of digital competence among students of public and private universities to comply with national and international educational policies to assure quality and equitable education for all.
7. Conclusions and recommendations
The current study intends to assess the gender divide in digital competence among university students in the southwestern region of Bangladesh. Findings reveal that students’ self-reported digital competence was significantly influenced by their human (age, levels of education, school) financial (SES) and social (gender) capitals. It is also apparent that male students reported higher levels of CAU and CSe regardless of their age, level of education, school, and SES than female students. The findings of this study unveiled numerous implications in higher academia that educators and policymakers should address. Firstly, long-established gender stereotypes must be addressed properly to reduce the gender divide in digital technology to achieve quality and equitable education for all. Secondly, gender-specific training and educational guidelines can be developed and implemented to enhance technology-based skills among learners at secondary and tertiary levels to meet the requirements of the 21st-century. Thirdly, administrators should take the initiative to ensure the availability, accessibility, and applicability of digital technologies at universities to enhance students’ digital competence. Finally, as students’ digital competence depends on teachers’ knowledge, skills, and application of digital technologies, thus, digital competence of educators should also be promoted by implementing extensive training programs.
Data availability statement
The raw data supporting the conclusions of this article are made available at Harvard Dataverse (https://doi.org/10.7910/DVN/PBX4U9).
Ethics statement
This study was approved by the Institutional Ethical Clearance Committee of Khulna University (Reference No. KUECC – KUECC-2023-03-16). The participants provided their written informed consent to participate in this study.
Author contributions
MTH: Conceptualization, Data curation, Formal analysis, Investigation, Methodology, Software, Writing – original draft, Writing – review & editing. SA: Writing – original draft. NAN: Writing – original draft. LK: Writing – original draft. TTS: Writing – original draft. NJ: Data curation, Formal analysis, Software, Writing – original draft. MMR: Writing – review & editing. MTK: Investigation, Supervision, Writing – review & editing.
Funding
The author(s) declare financial support was received for the research, authorship, and/or publication of this article. This work was partially supported by High Impact Factor Journal Publication Grants (HIFJPG - 02/2023) of Khulna University for Open Access Publication.
Acknowledgments
The authors are indebted to the participants for their voluntary participation in this study and sharing with us the unexplored aspects of their digital competence without hesitation.
Conflict of interest
The authors declare that the research was conducted in the absence of any commercial or financial relationships that could be construed as a potential conflict of interest.
Publisher’s note
All claims expressed in this article are solely those of the authors and do not necessarily represent those of their affiliated organizations, or those of the publisher, the editors and the reviewers. Any product that may be evaluated in this article, or claim that may be made by its manufacturer, is not guaranteed or endorsed by the publisher.
References
Aesaert, K., and van Braak, J. (2015). Gender and socioeconomic related differences in performance based ICT competences. Comput. Educ. 84, 8–25. doi: 10.1016/j.compedu.2014.12.017
Almaiah, M. A., Al-Khasawneh, A., and Althunibat, A. (2020). Exploring the critical challenges and factors influencing the e-learning system usage during COVID-19 pandemic. Educ. Inf. Technol. 25, 5261–5280. doi: 10.1007/s10639-020-10219-y
Almasri, F. (2022). The impact of e-learning, gender-groupings and learning pedagogies in biology undergraduate female and male students’ attitudes and achievement. Educ. Inf. Technol. 27, 8329–8380. doi: 10.1007/s10639-022-10967-z
Appel, M. (2012). Are heavy users of computer games and social media more computer literate? Comput. Educ. 59, 1339–1349. doi: 10.1016/j.compedu.2012.06.004
Aytekin, E., and Isiksal-Bostan, M. (2019). Middle school students’ attitudes towards the use of technology in mathematics lessons: does gender make a difference? Int. J. Math. Educ. Sci. Technol. 50, 707–727. doi: 10.1080/0020739X2018.1535097
Bangladesh Bureau of Statistics. (2015). ICT use and access by individuals and households: Bangladesh 2013. Dhaka: Bangladesh Bureau of Statistics (BBS), Ministry of Planning.
Bangladesh University Grants Commission. (2021). 48th annual report 2021. Dhaka: Bangladesh University Grants Commission.
Barboutidis, G., and Stiakakis, E. (2023). Identifying the factors to enhance digital competence of students at vocational training institutes. Technol. Knowl. Learn. 28, 613–650. doi: 10.1007/s10758-023-09641-1
Cai, Z., Fan, X., and Du, J. (2017). Gender and attitudes toward technology use: a meta-analysis. Comput. Educ. 105, 1–13. doi: 10.1016/j.compedu.2016.11.003
Chan, N. N., Walker, C., and Gleaves, A. (2015). An exploration of students’ lived experiences of using smartphones in diverse learning contexts using a hermeneutic phenomenological approach. Comput. Educ. 82, 96–106. doi: 10.1016/j.compedu.2014.11.001
Cohen, J. (1988). Statistical power analysis for the behavioral sciences. Hillsdale, New Jersey: Lawrence Erlbaum Associates.
Coleman, J. S. (1988). Social capital in the creation of human capital. Am. J. Sociol. 94, S95–S120. doi: 10.1086/228943
Ertmer, P. A., Evenbeck, E., Cennamo, K. S., and Lehman, J. D. (1994). Enhancing self-efficacy for computer technologies through the use of positive classroom experiences. Educ. Technol. Res. Dev. 42, 45–62. doi: 10.1007/BF02298094
Fisher, S., and Jenson, J. (2017). Producing alternative gender orders: a critical look at girls and gaming. Learn. Media Technol. 42, 87–99. doi: 10.1080/17439884.2016.1132729
Fraillon, J., Ainley, J., Schulz, W., Friedman, T., and Gebhardt, E. (2014). Preparing for life in a digital age: The IEA international computer and information literacy study international report London: Springer Nature.
Gialamas, V., Nikolopoulou, K., and Koutromanos, G. (2013). Student teachers’ perceptions about the impact of internet usage on their learning and jobs. Comput. Educ. 62, 1–7. doi: 10.1016/j.compedu.2012.10.012
Glantz, S.A., Slinker, B.K., and Neilands, T.B. (2016). Primer of applied regression and anaysis of variance (3rd). New York: McGraw-Hill Education.
Gómez, J. I. A., and Almenara, J. (2013). Tecnologías y medios para la educación en la e-sociedad. Madrid: Alianza.
Gómez-Trigueros, I. M. (2023). “Teaching professional ethical knowledge and teaching digital skills in higher education,” in Higher education - reflections from the field. Eds. L. Waller and S. Waller Waller. London: IntechOpen. 1–12.
Hasan, N., and Bao, Y. (2020). Impact of “e-learning crack-up” perception on psychological distress among college students during COVID-19 pandemic: a mediating role of “fear of academic year loss”. Child Youth Serv. Rev. 118:105355. doi: 10.1016/j.childyouth.2020.105355
He, T., and Zhu, C. (2017). Digital informal learning among Chinese university students: the effects of digital competence and personal factors. Int. J. Educ. Technol. High. Educ. 14:44. doi: 10.1186/s41239-017-0082-x
Hohlfeld, T. N., Ritzhaupt, A. D., and Barron, A. E. (2013). Are gender differences in perceived and demonstrated technology literacy significant? It depends on the model. Educ. Technol. Res. Dev. 61, 639–663. doi: 10.1007/s11423-013-9304-7
Hollingshead, A.B. (1975). Four factor index of social status. Connecticut: Department of Sociology, Yale University.
Hossain, M. T., Nishu, N. A., Jahan, N., Saadia, H., Ela, M. Z., Moshrur-Ul-Alam, Q., et al. (2022). “Challenges of participating in online education/distance learning amidst the COVID-19 pandemic in Bangladesh: the response from students and teachers” in Handbook of research on asian perspectives of the educational impact of covid-19. eds. M. R. Islam, B. Santosh Kumar, and N. Lamhot (Hershey, PA, USA: IGI Global)
Höyng, M. (2022). Encouraging gameful experience in digital game-based learning: a double-mediation model of perceived instructional support, group engagement, and flow. Comput. Educ. 179:104408. doi: 10.1016/j.compedu.2021.104408
Huang, S., and Fang, N. (2013). Predicting student academic performance in an engineering dynamics course: a comparison of four types of predictive mathematical models. Comput. Educ. 61, 133–145. doi: 10.1016/j.compedu.2012.08.015
Huang, W.H.D., and Oh, E. (2016). Retaining disciplinary talents as informal learning outcomes in the digital age: an exploratory framework to engage undergraduate students with career decision-making processes handbook of research on learning outcomes and opportunities in the digital age Hershey: IGI Global.
Islam, M. N., and Inan, T. T. (2021). Exploring the fundamental factors of digital inequality in Bangladesh. SAGE Open 11:110214. doi: 10.1177/21582440211021407
Jackson, L. A., Zhao, Y., Kolenic, A. 3rd, Fitzgerald, H. E., Harold, R., and Von Eye, A. (2008). Race, gender, and information technology use: the new digital divide. Cyberpsychol. Behav. 11, 437–442. doi: 10.1089/cpb.2007.0157
Jeffrie, N. (2022). The mobile gender gap in south asia is now widening. Retrieved from https://www.gsma.com/mobilefordevelopment/blog/the-mobile-gender-gap-in-south-asia-is-now-widening/ (Accessed May 20, 2023).
Jenson, J., and de Castell, S. (2018). “The entrepreneurial gamer”: Regendering the order of play. Games Cult. 13, 728–746. doi: 10.1177/1555412018755913
Khan, S. A., Bhatti, R., and Khan, A. A. (2011). Use of ict by students: a survey of faculty of education at iub. Library Philos. Pract. E-Journal 677, 1–12.
Kuhlemeier, H., and Hemker, B. (2007). The impact of computer use at home on students’ internet skills. Computers & Education 49, 460–480. doi: 10.1016/j.compedu.2005.10.004
Laakso, N. L., Korhonen, T. S., and Hakkarainen, K. P. (2021). Developing students’ digital competences through collaborative game design. Comput. Educ. 174:104308. doi: 10.1016/j.compedu.2021.104308
Li, Y., and Ranieri, M. (2010). Are ‘digital natives’ really digitally competent?—a study on Chinese teenagers. Br. J. Educ. Technol. 41, 1029–1042. doi: 10.1111/j.1467-8535.2009.01053.x
Mariscal, J., Mayne, G., Aneja, U., and Sorgner, A. (2019). Bridging the gender digital gap. Economics, 13, 20190009. doi: 10.5018/economics-ejournal.ja.2019-9
Martzoukou, K., Kostagiolas, P., Lavranos, C., Lauterbach, T., and Fulton, C. (2022). A study of university law students’ self-perceived digital competences. J. Librariansh. Inf. Sci. 54, 751–769. doi: 10.1177/09610006211048004
Master, A., Meltzoff, A. N., and Cheryan, S. (2021). Gender stereotypes about interests start early and cause gender disparities in computer science and engineering. Proc. Natl. Acad. Sci. 118:e2100030118. doi: 10.1073/pnas.2100030118
Mehrvarz, M., Heidari, E., Farrokhnia, M., and Noroozi, O. (2021). The mediating role of digital informal learning in the relationship between students’ digital competence and their academic performance. Comput. Educ. 167:104184. doi: 10.1016/j.compedu.2021.104184
Napal Fraile, M., Peñalva-Vélez, A., and Mendióroz Lacambra, A. M. (2018). Development of digital competence in secondary education teachers’ training. Educ. Sci. 8:104. doi: 10.3390/educsci8030104
Neuman, W.L. (2014). Basics of social research: Qualitative & quantitative approaches (3rd). Essex: Pearson Education Limited.
Noiwan, J., Piyawat, T., and Norcio, A.F. (2005). Computer attitude and computer self-efficacy: a case study of Thai undergraduate students. Paper presented at the 11th International Conference on Human-Computer Interaction, Nevada, The United States of America.
Pallant, J.F. (2011). SPSS survival manual: A step by step guide to data analysis using SPSS (4th). New South Wales, Australia: Allen & Unwin.
Ren, W., Zhu, X., and Yang, J. (2022). The ses-based difference of adolescents’ digital skills and usages: an explanation from family cultural capital. Comput. Educ. 177:104382. doi: 10.1016/j.compedu.2021.104382
Ritzhaupt, A. D., Liu, F., Dawson, K., and Barron, A. E. (2013). Differences in student information and communication technology literacy based on socio-economic status, ethnicity, and gender: evidence of a digital divide in Florida schools. J. Res. Technol. Educ. 45, 291–307. doi: 10.1080/15391523.2013.10782607
Scherer, R., and Siddiq, F. (2019). The relation between students’ socioeconomic status and ICT literacy: findings from a meta-analysis. Comput. Educ. 138, 13–32. doi: 10.1016/j.compedu.2019.04.011
Schlebusch, C. L. (2018). Computer anxiety, computer self-efficacy and attitudes towards the internet of first year students at a south African university of technology. Africa Educ. Rev. 15, 72–90. doi: 10.1080/18146627.2017.1341291
Scholes, L., Mills, K. A., and Wallace, E. (2022a). Boys’ gaming identities and opportunities for learning. Learn. Media Technol. 47, 163–178. doi: 10.1080/17439884.2021.1936017
Scholes, L., Rowe, L., Mills, K. A., Gutierrez, A., and Pink, E. (2022b). Video gaming and digital competence among elementary school students. Learn. Media Technol., 1–16. doi: 10.1080/17439884.2022.2156537
Shala, A., and Grajcevci, A. (2018). Digital competencies among student populations in Kosovo: the impact of inclusion, socioeconomic status, ethnicity and type of residence. Educ. Inf. Technol. 23, 1203–1218. doi: 10.1007/s10639-017-9657-3
Snyder, T.D., Dillow, S.A., and Hoffman, C.M. (2009). Digest of education statistics 2008 (NCES 2009–020). Washington, DC: National Center for Education Statistics, Institute of Education Sciences, U.S. Department of Education.
Sobieraj, S., and Krämer, N. C. (2020). Similarities and differences between genders in the usage of computer with different levels of technological complexity. Comput. Hum. Behav. 104:106145. doi: 10.1016/j.chb.2019.09.021
Sumi, S. S., Jahan, N., Rahman, S. T., Seddeque, A., and Hossain, M. T. (2021). Explaining rural-urban differences in the academic achievement of secondary students: an empirical study in Magura district of Bangladesh. Asia Pac. J. Educ. Educ. 36, 195–216. doi: 10.21315/apjee2021.36.1.11
Sumi, S. S., Mondal, B. K., Jahan, N., Seddeque, A., and Hossain, M. T. (2022). Determinants of academic achievement at secondary levels: a study in Magura district of Bangladesh. Malays. Online J. Educ. Manag. 10, 21–44.
Synnott, J., Harkin, M., Horgan, B., McKeown, A., Hamilton, D., McAllister, D., et al. (2020). The digital skills, experiences and attitudes of the Northern Ireland social care workforce toward technology for learning and development: survey study. JMIR Med. Educ. 6:e15936. doi: 10.2196/15936
Vázquez-Cano, E., Meneses, E. L., and García-Garzón, E. (2017). Differences in basic digital competences between male and female university students of social sciences in Spain. Int. J. Educ. Technol. High. Educ. 14:27. doi: 10.1186/s41239-017-0065-y
Vázquez-Cano, E., Urrutia, M. L., Parra-González, M. E., and Meneses, E. L. (2020). Analysis of interpersonal competences in the use of ICT in the Spanish university context. Sustainability 12:476. doi: 10.3390/su12020476
Vega-Gea, E., Calmaestra Villén, J., and Ortega Ruiz, R. (2021). Percepción docente del uso de tic en la educación inclusiva. Revista de Medios Y Educación, 62, 235–268. doi: 10.12795/pixelbit.90323
Vishnu, S., Raghavan Sathyan, A., Susan Sam, A., Radhakrishnan, A., Olaparambil Ragavan, S., Vattam Kandathil, J., et al. (2022). Digital competence of higher education learners in the context of COVID-19 triggered online learning. Soc. Sci. Human. Open 6:100320. doi: 10.1016/j.ssaho.2022.100320
Wild, S., and Schulze Heuling, L. (2020). How do the digital competences of students in vocational schools differ from those of students in cooperative higher education institutions in Germany? Emp. Res. Voc. Educ. Train. 12:5. doi: 10.1186/s40461-020-00091-y
Yoon, H., Jang, Y., and Xie, B. (2016). Computer use and computer anxiety in older Korean Americans. J. Appl. Gerontol. 35, 1000–1010. doi: 10.1177/0733464815570665
Yu, Z. (2021). The effects of gender, educational level, and personality on online learning outcomes during the COVID-19 pandemic. Int. J. Educ. Technol. High. Educ. 18:14. doi: 10.1186/s41239-021-00252-3
Yuen, A. H. K., Lau, W. W. F., Park, J. H., Lau, G. K. K., and Chan, A. K. M. (2016). Digital equity and students’ home computing: a Hong Kong study. Asia Pac. Educ. Res. 25, 509–518. doi: 10.1007/s40299-016-0276-3
Keywords: gender divide, digital competence, social capital, university students, Bangladesh
Citation: Hossain MT, Akter S, Nishu NA, Khan L, Shuha TT, Jahan N, Rahman MM and Khatun MT (2023) The gender divide in digital competence: a cross-sectional study on university students in southwestern Bangladesh. Front. Educ. 8:1258447. doi: 10.3389/feduc.2023.1258447
Edited by:
Raufhon Salahodjaev, Tashkent State Economic University, UzbekistanReviewed by:
Pinaki Chakraborty, Netaji Subhas University of Technology, IndiaDennis Arias-Chávez, Continental University, Peru
Copyright © 2023 Hossain, Akter, Nishu, Khan, Shuha, Jahan, Rahman and Khatun. This is an open-access article distributed under the terms of the Creative Commons Attribution License (CC BY). The use, distribution or reproduction in other forums is permitted, provided the original author(s) and the copyright owner(s) are credited and that the original publication in this journal is cited, in accordance with accepted academic practice. No use, distribution or reproduction is permitted which does not comply with these terms.
*Correspondence: Nusrat Jahan, nusratku05@soc.ku.ac.bd