- 1Department of Mathematics, Natural Sciences, and Social Sciences Applied to Education, Faculty of Teaching and Education Sciences, Universidad Católica de Valencia San Vicente Mártir, Valencia, Spain
- 2Department of General Didactics, Theory of Education and Technological Innovation, Faculty of Teaching and Education Sciences, Universidad Católica de Valencia San Vicente Mártir, Valencia, Spain
Currently, coexistence is an essential factor that influences educational quality. Issues relating to school violence affect a large number of students, affecting their personal, academic, and professional futures. For this reason, this study aimed to present a different study tool that would allow the optimization of the factors that influence classroom climate, establish mechanisms to detect and predict how the modification of these factors can positively or negatively affect it, and determine the most appropriate intervention before it occurs. Dynamic mathematical models are complex tools that allow us to study a given problem or reality in real-time and develop predictive instruments through the analysis of mathematical relationships, allowing us to adapt the answers in a customized manner. In this study, an example is provided in measuring classroom climate throughout an academic course in a simulated situation. It consists of a dynamic mathematical model developed with Stella 10.0 software using only some of the variables that affect classroom climate, which are distributed in two sections—a psychological one that includes Rabies Level and Isolation Level due to loss of self-esteem and a physical one that includes the number of aggressive students and the total number of physically assaulted students. Since the classroom climate is very complex, other variables or a greater number of them could have been used in this hypothetical case; however, the example explains how this kind of model works and shows the great utility that it can have in this type of study. Regarding the most significant benefits that this tool can offer, some stand out. On the one hand, we can adapt the instrument to the specific characteristics of a class group, introducing or eliminating the variables depending on their relevance to the problem at hand. On the other hand, this versatile tool not only adapts to the reality of the classroom but also to the specific moment in which the students are. The greatest limitation of a Dynamic Mathematical Model as a tool is the large quantity of information required to adjust the model to reality; the more information we have, the more adjusted the model can be. Furthermore, gathering the type of information needed to develop the model is complicated.
1. Introduction
In the current times, the quality of education is significantly impacted by coexistence (Dobarro et al., 2013; Montero and Cervelló, 2019). Not in vain, in the well-known “Learning: the treasure within” report of the International Commission on Education for the 21st century to UNESCO (Delors, 1996), “Learning to live together or with others” is established as one of the four fundamental pillars of 21st century education. Similarly, Sustainable Development Goal 4 (Quality of Education) calls for a culture of peace and non-violence and for building and upgrading education facilities that provide safe, non-violent, inclusive, and effective learning environments for all by 2030 (United Nations, 2015).
If we pay attention to the literalness of SDG 4 established by the UN for sustainable development, the creation of safe, non-violent, and inclusive learning environments for all is marked as a priority for implementation in 21st-century schools. This reality, which should be guaranteed for all students in schools, is breached more often than desired. News and testimonies from students or alumni tell us about the bullying situations experienced at school. Bullying refers to a situation in which someone is exposed, repeatedly and over time, to negative actions on the part of one or more persons (Olweus, 2006), which instills in the victim what Seligman coined in the late 60s a feeling of “learned helplessness.” As defined by Olweus (2006), bullying has several characteristics. It is harassment with a clear intent to harm, hurt, or belittle by the victim (physically, morally, verbally, or psychologically); it must also be repeated harassment over time and not sporadic aggression; and finally, there must be an imbalance of real power or, at least, an imbalance felt by the victim.
This leads us to consider the best way to combat possible cases of bullying in schools. We understand that the most effective and appropriate way is to implement measures and actions within the teaching-learning process that can prevent bullying behaviors. Therefore, favoring measures and mechanisms for adequate coexistence in schools and classrooms can be a good solution.
One of the key measures within school coexistence that can contribute to preventing violence or bullying is the management of classroom climate, which can be defined as the social environment and emotional and physical aspects of the classroom (Sieberer-Nagler, 2016; Riekie et al., 2017; Aldridge et al., 2018; Montero and Cervelló, 2019). This is an important factor in learning development (Hattie, 2017). Hattie (2017) conducted a macro-study that collected over than 800 meta-analyses from 52,637 studies involving a sample of 240 million students. The present study aimed to determine the impact and effectiveness of various programs, educational policies, and teacher innovations designed to improve academic achievement. The study covered not only early childhood and primary education but also university education. Some of the factors related to classroom climate are among the 50 measures that, according to Hattie (2017), have the greatest influence on educational achievement. For example, teacher-student relationships (impact 0.72, position 12 of 150, high influence), classroom behavior (impact 0.68, position 16, high influence), and class management (impact 0.52, position 42, high influence) significantly affect learning. The quality of the interpersonal, social, and pedagogical relationships established in the classroom determines not only intellectual learning but also the emotional, moral, and social development of students (Dobarro et al., 2013; Codina, 2015).
Since positive classroom climate acts as a protective factor against problems related to school violence (Aldridge et al., 2016; Wang and Degol, 2016; Seray and Zúniga, 2021) and, conversely, poor classroom climate management acts as a risk factor, teachers need the pedagogical ability or technical resources (Remache-Bunci et al., 2022) to identify and act quickly in the face of potential problems [and] be aware of what is happening in the class (Hattie, 2017). Consequently, it would be possible to prevent and intervene in the problems of school violence that occur or can occur, analyze the possible responses and their effectiveness, and enhance the learning of all students. The results of the TALIS program in 2009, 2013 and 2018 Teaching and Learning International Surveys (OECD, 2010, 2013, 2019, respectively) clearly show that teachers' concern regarding things that can affect classroom climate is increasing (Table 1). When teachers were asked if the students' wellbeing was important for learning and personal, social, and moral development, the majority of surveyed teachers said yes. Similarly, the teachers affirmed that they were interested in what the students shared in this context. Specifically, Spanish teachers confirmed that they really helped their students when they needed something.
One of the first classroom climate questionnaires to analyze the classroom environment was developed by Walberg in 1966 (Anderson and Walberg, 1967). In 1973, Trickett and Moos (1973) developed a scale to measure classroom climate (CES Classroom Environment Scale). Since then, this scale, along with many different surveys and other methods of gathering information as direct observation, has been widely used. Many previous studies have focused on issues related to school life (Cava and Musitu, 2009; Peiró, 2012; Luengo, 2018) and classroom climate (Cohen, 2013; Murillo, 2015) or analyzed the problems arising from inadequate management of coexistence or classroom climate, such as school violence (Fernández, 2001; Ortega, 2015; Luengo, 2017), bullying (Bisquerra, 2014; Hinduja and Patchin, 2017; Navarro et al., 2018), or, more recently, cyberbullying (Ortega et al., 2016; Machimbarrena and Garaigordobil, 2018; Rey et al., 2018).
1.1. Violence in school models
Many studies have attempted to develop prevention and intervention models for violence in schools. These models have different perspectives and aim to minimize violent behavior among students. Ortega Ruiz et al. (2013) analyzed the behavior of 7,037 secondary students using the Escala Convivencia Escolar (ECE). Structural equations were created, and the results obtained were used to optimize the school climate. Montero and Cervelló (2019) studied the relationship between resilience and moral identity, bullying, and victimization using the questionnaire, “What is Happening in this School?” (see Figure 1).
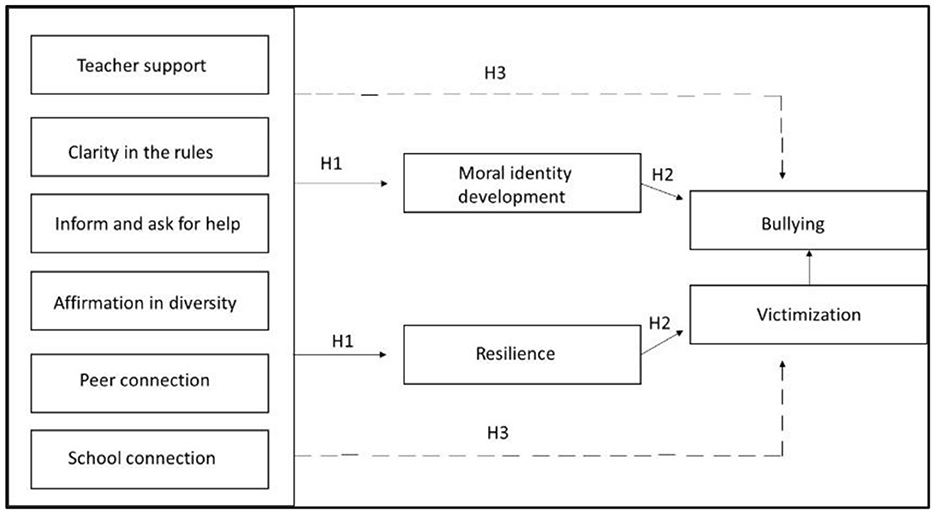
Figure 1. Prediction model of school violence. Reproduced from Montero and Cervelló (2019, p. 142), licensed under CC BY-NC-ND.
Parkman (2018) attempted to establish measures against school violence before the problem occurred, using all possible tools, such as social networks. Villarreal et al. (2010) presented the model depicted in Figure 2 to explain violent behavior.
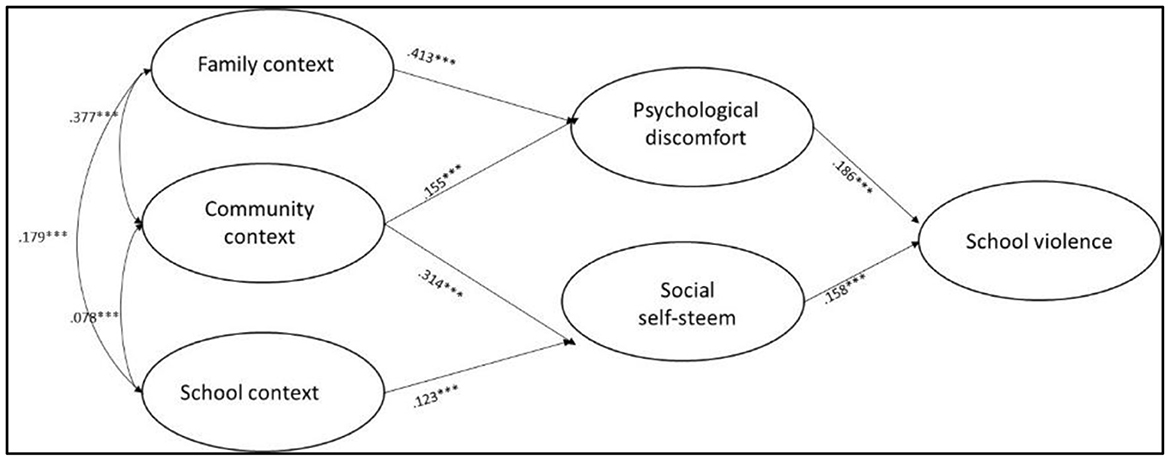
Figure 2. Violent behavior model, from Villarreal et al. (2010, p. 417). ***p < 0.001.
All these methodologies offer a static view of the classroom climate at the time the study was conducted, which may or may not result in a subsequent intervention. However, by themselves, these methodologies do not allow, neither orient nor predict, such intervention. However, it is important to consider that rapid action, in one way or another, can pose significant risks. This is especially true in social sciences, where studies are carried out on groups of people, making it impossible to test certain aspects in a laboratory setting.
Nevertheless, changes over time can be achieved by constructing a dynamic model. Modeling enables us to study and understand the multifaceted and interrelated phenomena that occur worldwide by identifying the variables that affect these phenomena and the relationships between them. There are different types of models. While one model may represent an event at a particular point in time, there are others that represent the event in real or simulated time, helping us understand the dynamics of the processes (Hannon and Ruth, 2001).
Dynamic mathematical models have been described in detail by Professor John D. Sterman of the Massachusetts Institute of Technology in his book Business Dynamics: Systems Thinking and Modeling for a Complex World, published in 2000. System dynamics, as a method, enhances learning in complex systems (Sterman, 2000). According to Sterman, every element of a system is interconnected, and their actions provoke feedback. However, the effects are not usually proportional to the causes, which can affect any region of the system; thus, systems are non-linear. Systems are also dynamic because they change frequently, are adaptive, self-organizing, history-dependent, and more importantly, “The complexity of systems in which we are embedded overwhelms our ability to understand them. The result: many seemingly obvious solutions to problems fail or actually worsen the situation” (Sterman, 2000, p. 22).
Because mental models are usually inappropriate for understanding these types of systems, the development of formal models is essential. The powerful software that we chose to use for modeling dynamic systems was Stella Architect Professional Software (2018). The basic principles are explained below.
The model adopted in this study is a non-closed dynamic mathematical model, developed by Stella, that can be adapted according to the different changes produced in the reality of the classroom. Using this method, a more robust database can be created, which allows us not only to have a real-time view of what is happening in the classroom but also to develop prediction and improvement instruments to determine the necessary and appropriate prevention or intervention measures.
Many studies in various fields have used dynamic mathematical models as predictive tools, for instance, in economics (Pradas et al., 2009), electronics (Rivas et al., 1994), psychology (Mazar, 2006; Verdière et al., 2014), and education (Bakwai and Yisa, 2014; Van Geert, 2014).
Several studies have shown that victimization is one of the factors that increase the incidence of bullying (Ramos, 2008; Barboza et al., 2009). High levels of negative emotions, such as rage, generate instances of harassment (Avilés, 2009) that increase the likelihood of students adopting strategies that do not favor problem-solving (Sánchez et al., 2012). Jiménez et al. (2008) found that, when adolescents perceive a lack of support and friendship from their peers, the risk of manifesting violent behavior increases, which worsens their relationship with teachers and leads to lower acceptance by their peers.
Analyzing the results of investigations on the relationship between self-esteem and aggressiveness using multidimensional measures, it was evident that the group of individuals who were both aggressors and victims did not present high levels of self-esteem, unlike the group of aggressors who have never suffered harassment. Similarly, in a study of 180 students who were both victims and aggressors, low social acceptance and self-esteem were observed (Andreou, 2000). Similar results were obtained in another study involving more than 900 students. The individuals who were both aggressors and victims had the lowest values of self-esteem in the family, school, and emotional and social dimensions (Cava and Musitu, 2002).
Consequently, the aggressiveness shown by some students in the classroom is sometimes the result of harassment situations, such as humiliation or other verbal or physical aggression. Attacked students tend to isolate themselves from their classmates, and their school performances decrease because of the loss of self-esteem (Ruiz Coronel and Padilla, 2012). When this situation continues over time, the likelihood of aggressive behavior increases, which can negatively affect the classroom climate.
In this study, we asked ourselves the following question: can the dynamic mathematical model be an effective tool to combat cases of bullying and help teachers and counselors to prevent, detect, and act correctly in cases of bullying?
2. Materials and methods
The theoretical model developed in this study allows for the simulation of the classroom climate throughout an academic year. It consists of state variables that indicate the stage of the system at all times and are represented graphically by rectangles. These variables typically represent the accumulation or reserves of physical elements or emotional states.
The situation in which the state variables are found may change over time. This change is represented graphically by arrows and the so-called information flows that represent activities or actions, physical or emotional, and whose speed can be modified by convertors, which are represented graphically by circles. A graphical representation of the model is shown in Figure 3.
Accordingly, the developed model has a psychological and a physical part. In total, it consists of four state variables or accumulators of material, individuals, or emotions. Two of them would be in the psychological module and two in the physical. The present study aimed to present a different study tool that would allow the optimization of the factors that influence classroom climate, establish mechanisms to detect and predict how the modification of these factors can positively or negatively affect it, and determine the most appropriate way to intervene before it occurs.
2.1. Psychological module
This module includes two state variables—Rabies Level and Isolation Level by Self-Esteem Loss. Both state variables have a range of values from 0 to 100%.
2.2. Physical module
This module includes two state variables—the Number of Aggressive Students and the Total Number of Students who have been physically assaulted and present evidence of minor injuries or some type of blow or bruise. For these two state variables, the units are individuals.
As previously mentioned, the variations observed in these state variables throughout the academic year are marked by the entry and exit of flows in the state variables.
Mathematically, all information that flows between state variables follows the format described below. Assuming that the state variable Rabies Level is represented by R, the value that R will have at any time (t) is defined by the difference in flow between the input and the output.
Stella Architect Professional Software (2018) was used to develop and execute the model. A classroom group with 100 students was simulated for a duration of 40 weeks. The passage time was 0.25, and the Runge-Kutta4 integration method was used. A detailed description of the model is presented in Figures 4, 5.
3. Results
Table 2 presents the results where a classroom of 100 students was simulated for 40 weeks. The scenarios ranged from one to three humiliation activities each week. How emotional and physical situations evolved at 20 and 40 weeks of class were evaluated.
For example, when there was one act of humiliation per week, the percentage of isolation at the end of the course was ~21%, and the level of rage was 3%. In this situation, one aggressive student can assault two classmates. This situation is controllable, although the model does not include correction measures. However, if nothing is done, humiliation activities per week increase. In the case of three humiliation activities per week, at the end of the course, the percentage of estimated isolation was more than double that in the first case (70%), and the Rabies Level also increased exponentially from 3 to 28%. Consequently, if there was one aggressive student, there would have been 10 students in the classroom who may have attacked 31 classmates by the end of the course (see Table 2).
4. Discussion
Dynamic mathematical models can be very useful when working on the problem of school violence, although they have possible limitations. Predicting the future behavior of humans remains a highly complex mission. However, despite this, these predictions can help guide future actions with a degree of certainty regarding expected outcomes. As Ruiz Coronel and Padilla (2012, p. 122) state, the mathematical model “does not ensure the prophetic forecast of events, but it certainly allows for a better-informed decision making and the contemplation of at least a good number of affected variables.”
Regarding the most significant benefits that this tool can bring us, some stand out. On the one hand, we can adapt the instrument to the specific characteristics of the class group by introducing or eliminating variables depending on their relevance to the problem at hand. In this way, it is a customizable tool that responds to our reality such that the results and intervention proposals derived from it will respond to our real problem. On the other hand, this versatile tool not only adapts to the reality of the classroom but also to the specific moment in which the students are. One of the advantages of the dynamic system is that it is open, and all the information we consider relevant can be incorporated at any time and the more information we have, the more customized the model can be. However, gathering the type of information needed to develop the model is complicated.
Although a theoretical model based on classroom experience has been constructed, its evaluation using real data is yet to be conducted. However, it has been observed that, in the absence of measures to control harassment, aggression increases exponentially (see Figure 4) due to the feedback nature of the situation.
Moreover, the model allows the incorporation of new factors related to violence in classrooms as well as measures and activities to reduce aggressiveness and abuse. This tool could be efficient not only for detecting classroom climate but also for the prediction and prevention of school violence.
Data availability statement
The original contributions presented in the study are included in the article/supplementary material, further inquiries can be directed to the corresponding author.
Author contributions
IH: Conceptualization, Data curation, Formal analysis, Investigation, Methodology, Supervision, Writing—original draft, Writing—review and editing. RS: Conceptualization, Data curation, Formal analysis, Investigation, Methodology, Supervision, Writing—original draft, Writing—review and editing. EM-L: Conceptualization, Data curation, Methodology, Writing—original draft. All authors contributed to the article and approved the submitted version.
Funding
The author(s) declare that no financial support was received for the research, authorship, and/or publication of this article.
Conflict of interest
The authors declare that the research was conducted in the absence of any commercial or financial relationships that could be construed as a potential conflict of interest.
Publisher's note
All claims expressed in this article are solely those of the authors and do not necessarily represent those of their affiliated organizations, or those of the publisher, the editors and the reviewers. Any product that may be evaluated in this article, or claim that may be made by its manufacturer, is not guaranteed or endorsed by the publisher.
References
Aldridge, J. M., Fraser, B. J., Fozdar, F., Ala'i, K., Earnest, J., and Afari, E. (2016). Students' perceptions of school climate as determinants of wellbeing, resilience and identity. Improv. Sch. 19, 5–26. doi: 10.1177/1365480215612616
Aldridge, J. M., McChesney, K., and Afari, E. (2018). Relationships between school climate, bullying, and delinquent behavior's. Learn. Environ. Res. 21, 153–172. doi: 10.1007/s10984-017-9249-6
Anderson, G. J., and Walberg, H. J. (1967). Classroom climate and group learning. J. Educ. Psychol. 59, 414. doi: 10.1037/h0026490
Andreou, E. (2000). Bully/victim problems and their association with psychological constructs in 8-to 12-year-old Greek schoolchildren. Aggress. Behav. 26, 49–56. doi: 10.1002/(SICI)1098-2337(2000)26:1<49::AID-AB4>3.0.CO;2-M
Avilés, J. M. (2009). Victimización percibida y bullying. Factores esenciales entre víctimas. Bol. Psicol. 95, 7–28.
Bakwai, B., and Yisa, H. M. (2014). Mathematical modelling: a scientific tool in educational management. Al-hikma J. Educ. 1, 38–46.
Barboza, G. E., Schiamberg, L. B., Oehmeke, J., Korzeniewski, S. J., Post, L. A., and Heraux, C. G. (2009). Individual characteristics and the multiple contexts of adolescent bullying. An ecological perspective. J. Youth Adolesc. 38, 101–121. doi: 10.1007/s10964-008-9271-1
Codina, Mª. J. (2015). Neuroeducación en Virtudes Cordiales. Cómo Reconciliar lo que Decimos con lo que Hacemos. Barcelona: Octaedro.
Cohen, J. (2013). “Creating a positive school climate: a foundation for resilience,” in Handbook of Resilience in Children, eds S. Goldstein, and T. B. Brooks (New York, NY: Springer), 411–423. doi: 10.1007/978-1-4614-3661-4_24
Dobarro, A., García Fernández, T., and Álvarez, D. (2013). ¿Qué medidas para la mejora de la convivencia se están desarrollando en los centros educativos?: una perspectiva desde dentro. Eur. J. Investig. Health Psychol. Educ. 3, 207–213. doi: 10.30552/ejihpe.v3i3.50
Fernández, I. (2001). Prevención de la Violencia y Resolución de Conflictos. El Clima Escolar como Factor de Calidad. Madrid: Narcea.
Hannon, B., and Ruth, M. (2001). Dynamic Modeling. New-York, NY: Springer-Verlag. doi: 10.1007/978-1-4613-0211-7
Hattie, J. (2017). “Aprendizaje visible” Para los Profesores. Maximizando el Impacto en el Aprendizaje. Madrid: Paraninfo.
Hinduja, S., and Patchin, J. W. (2017). Cultivating youth resilience to prevent bullying and cyberbullying victimization. Child Abuse Neglect 73, 51–62. doi: 10.1016/j.chiabu.2017.09.010
Jiménez, T. I., Moreno, D., Murgui, S., and Musitu, G. (2008). Factores psicosociales relacionados con el estatus social del alumno en el aula. El rol de la reputación social, la amistad, la conducta violenta y la relación con el profesor. Int. J. Psychol. Psychol. Ther. 8, 227–236.
Luengo, J. A. (2017). “Repensar la convivencia en los centros educativos: hoy más necesario que nunca,” [Entrada en Blog] in EDUForics. Anticipando la Educación del Futuro. El Futuro de la Educación. Available online at: http://www.eduforics.com/es/repensar-la-convivencia-los-centroseducativos-hoy-mas-necesario-nunca/ (accessed September 13, 2023).
Luengo, J. A. (2018). La Elaboración y Revisión del Plan de Convivencia de los Centros Educativos. (Documento de Apoyo a la supervisión del Plan de Convivencia). Madrid: Consejería de Educación e Investigación de la Comunidad de Madrid.
Machimbarrena, J. M., and Garaigordobil, M. (2018). Acoso y ciberacoso en Educación Primaria. Behav. Psychol. 26, 263–280.
Mazar, J. E. (2006). Mathematical Models and the Experimental Analysis of Behavior. Journal of the Experimental Analysis of Behavior. (2006), 85, 275–291. doi: 10.1901/jeab.2006.65-05
Montero, C., and Cervelló, E. M. (2019). Estudio de un modelo predictivo del clima escolar sobre el desarrollo del carácter y las conductas de bullying. Estud. Sobre Educ. 37, 135–157. doi: 10.15581/004.37.135-157
Murillo, O. (2015). Análisis existencial del rol docente en el manejo de la disciplina en el aula y en el centro educativo. Actual. Investig. Educ. 15, 1–21.
Navarro, R., Yubero, S., and Larranaga, E. (2018). Cyberbullying victimization and fatalism in adolescence: resilience as a moderator. Child. Youth Serv. Rev. 84, 215–221. doi: 10.1016/j.childyouth.2017.12.011
OECD (2013). Teaching and Learning International Survey TALIS 2013. Conceptual Framework. Paris: TALIS; OECD Publishing.
OECD (2019). Teaching and Learning International Survey TALIS 2018. Research Report. Paris: TALIS; OECD Publishing.
Ortega Ruiz, R., Rey Alamillo, R. D., and Casas Bolaños, J. A. (2013). La convivencia escolar: clave en la predicción del bullying. Rev. Iberoam. Eval. Educ. 6, 91–102.
Ortega, R., del Rey, R., and Casas, J. A. (2016). Evaluar el bullying y el ciberbullying: validación española del EBIP-Q y del ECIP-Q. Rev. Psicol. Educ. 22, 71–79. doi: 10.1016/j.pse.2016.01.004
Parkman, B. J. (2018). Using Predictive Analytics to Address School Violence: Creating Safer Campuses for Students. Fayettvielle, NC: The Safe Campus.
Peiró, S. (2012). Convivencia en Educación: Problemas y Soluciones. Perspectiva Europea y Latinoamericana. Alicante: Universidad de Alicante.
Pradas, R., Antoñanzas, F., and Mar, J. (2009). Modelos matemáticos para la evaluación económica: los modelos dinámicos basados en ecuaciones diferenciales. Gac. Sanit. 23, 473–478. doi: 10.1016/j.gaceta.2008.12.007
Ramos, M. (2008). Violencia y Victimización en Adolescentes Escolares [Tesis doctoral]. Sevilla: Universidad Pablo de Olavide.
Remache-Bunci, M. G., Rosero, N. V., and Cóndor, B. H. (2022). Estrategias educativas en la solución de conflictos escolares en adolescentes. Rev. Complut. Educ. 34, 701–716. doi: 10.5209/rced.80036
Rey, R., Mora, J. A., Casas, J. A., Ortega, R., and Elipe, P. (2018). Programa “Asegúrate”: efectos en ciberagresión y sus factores de riesgo. Comunicar XXVI, 39–48.
Riekie, H., Aldridge, J. M., and Afari, E. (2017). The role of the school climate in high school student's mental health and identity formation: a South Australian study. Br. Educ. Res. J., 43, 95–123. doi: 10.1002/berj.3254
Rivas, R., Herranz, J., Llanes, O., and Cartaya, L. (1994). Modelo Matemático dinámico de generadores de vapor. Ing. Electrón. Autom. Comun. XV, 45–53.
Ruiz Coronel, A., and Padilla, P. (2012). Los modelos matemáticos en las ciencias sociales. Pensar Epistemol. Polít. Cienc. Soc. 7, 115–126.
Sánchez, V., Ortega, R., and Menesini, R. (2012). La competencia emocional de agresores y víctimas de bullying. An. Psicol. 28, 71–82.
Seray, D., and Zúniga, P. (2021). La convivencia escolar post COVID 19: una propuesta didáctica desde el coaching educativo. Int. J. Educ. Res. Innov. 15, 143–161. doi: 10.46661/ijeri.5005
Sieberer-Nagler, K. (2016). Effective classroom-management and positive teaching. Engl. Lang. Teach. 9, 163–172. doi: 10.5539/elt.v9n1p163
Stella Architect Professional Software (2018). High Performance System Version 1, 9.4. Hanover, NH: ISEE Systems Incorporation.
Sterman, J. D. (2000). Business Dinamics: Systems Thinking and Modeling for a Complex World. Boston, MA: McGraw Hill Higher Education.
Trickett, E. J., and Moos, R. H. (1973). Social environment of junior high and high school classrooms. J. Educ. Psychol. 65, 93–102. doi: 10.1037/h0034823
United Nations (2015). Transforming our World: The 2030 Agenda for Sustainable Development. New York, NY: United Nations.
Van Geert, P. (2014). Dynamic modeling for development an education: from concepts to numbers. Mind Brain Educ. 8, 57–73. doi: 10.1111/mbe.12046
Verdière, N., Lanza, V., Charrier, R., Provitolo, D., Dubos-Paillard, E., Bertelle, C., et al. (2014). “Mathematical modeling of human behaviors during catastrophic events,” in 4th International Conference on Complex System and Applications, 67–74.
Villarreal, Mª. E., Sánchez-Sosa, J. C., and Musitu, G. (2010). Un modelo predictivo de la violencia escolar en adolescentes. Ciencia UANL XIII, 413–420.
Keywords: dynamic mathematical model, classroom climate, classroom management, detection of school violence, prevention of school violence
Citation: Hernando I, Sanz R and Moreno-Latorre E (2023) Dynamic mathematical model as a tool for detecting and preventing school violence: a case report. Front. Educ. 8:1254926. doi: 10.3389/feduc.2023.1254926
Received: 27 July 2023; Accepted: 01 September 2023;
Published: 27 September 2023.
Edited by:
Alfredo Jiménez Eguizabal, University of Burgos, SpainReviewed by:
Carmen Palmero, University of Burgos, SpainÁngela Martín Gutiérrez, International University of La Rioja, Spain
Copyright © 2023 Hernando, Sanz and Moreno-Latorre. This is an open-access article distributed under the terms of the Creative Commons Attribution License (CC BY). The use, distribution or reproduction in other forums is permitted, provided the original author(s) and the copyright owner(s) are credited and that the original publication in this journal is cited, in accordance with accepted academic practice. No use, distribution or reproduction is permitted which does not comply with these terms.
*Correspondence: Roberto Sanz, Roberto.sanz@ucv.es