- 1Social Psychology Research Lab, Middlesex University Dubai, Dubai, United Arab Emirates
- 2VRX Lab, Middlesex University Dubai, Dubai, United Arab Emirates
Introduction: Student evaluations of teachers (SETs) carry significance for academics' career progression, but evidence suggests that these are influenced by students' expectations and biases. Previous research has shown that female lecturers are viewed less favorably compared to male lecturers. Racial bias has also been observed in higher education. For example, few studies administered in the U.S. found that African American lecturers received lower ratings than White lecturers. Current research investigates whether biases based on instructors' gender (male, female) and race (White and South Asian) influence university students' perception of instructors in an online teaching environment in the UAE.
Methods: Using a between group design, 318 participants viewed one of the four videos (male-South Asian, female-South Asian, male-White, female-White) of a virtual instructor teaching social psychology and then responded to teaching evaluation questions.
Results: Factorial ANOVAs were administered. Biases showcased consistent preference for male and South Asian lecturers. Male lecturers were perceived to be treating students with more respect, speaking in a more appropriate manner, displaying more enthusiasm for the subject, and more approachable than female lecturers. South Asian lecturers were perceived to be more sensitive toward students' feelings, displaying more enthusiasm for the subject, and more approachable than White lecturers. Overall, students expressed wanting to study more from male and South Asian lecturers compared to female and White lecturers.
Discussion: Biases emerged in interpersonal variables, such as approachability, sensitivity, enthusiasm for subject, and respect, and not in the domains of knowledge, presentation skills, and stimulating thinking. Findings reinforce that relying on teaching evaluations may not be accurate, and highlight how certain unconscious biases could impact professional growth of academics.
1 Introduction
One of the most extensively used methods of evaluating instructors in higher education, and their quality of teaching is via student evaluations of teachers (SETs). These scores carry a lot of significance for the instructors' careers, since the results of these evaluations are often taken into account when promotion and tenure are being considered. While institutions do not typically rely solely on these ratings, they do play a substantial part when discussing academic progression, teaching allocations, setting salaries, as well as motivating faculty through special recognition and faculty awards.
However, there is substantial evidence that points toward student evaluations of teachers being heavily influenced by several factors, including the students' own expectations and biases. The theory of discrimination could provide insights into how students' teaching evaluations might be influenced by various factors, including biases and stereotypes. Discrimination theory, in this context, refers to the differential treatment or evaluation of individuals based on characteristics such as gender, race, age, or other personal attributes. Discrimination theory suggests that gender bias may play a role in how students evaluate their instructors (Ullman, 2020; Kreitzer and Sweet-Cushman, 2022). Research has shown that students may hold gender-based stereotypes about teaching abilities. For example, there may be stereotypes that associate women with nurturing and men with authority, which can influence how students perceive and evaluate their instructors. Female instructors may be evaluated less favorably if they do not conform to traditional gender roles, while male instructors may be evaluated more positively if they exhibit traits associated with authority (Renström et al., 2021). Similar to gender bias, racial and ethnic bias can affect students' evaluations of their instructors. Students may hold biases or stereotypes related to race and ethnicity that can influence their perceptions of teaching effectiveness. Instructors from minority racial or ethnic groups may face unfair or less favorable evaluations due to these biases (Chávez and Mitchell, 2020).
In a detailed review, Wachtel (1998) discusses several variables, not directly related to the actual learning, performance, and course content, which potentially influence instructor ratings, such as class size, subject matter, and the instructor's physical appearance and educational qualifications, among others. Whether or not the student evaluations accurately represent an instructor's performance and ability to teach is unclear. A student's own motivation and willingness to pay attention to a specific instructor, based either on preference or unconscious bias, could have an impact on their learning. In a recent review of the literature, Kreitzer and Sweet-Cushman (2022) found that women and faculty belonging to marginalized groups or races are frequently at a disadvantage, regardless of methodology used or type of data collected. While the use of SETs has been criticized specifically for the reason that results could be heavily influenced by factors unrelated to the actual teaching or learning (Zabaleta, 2007; Spooren et al., 2013), institutions still rely on them in order to assess student experience.
In particular, gender bias in this regard has been studied extensively. Throughout the paper and in referenced literature, the term “gender” is used as a binary label. We acknowledge the complexity of the term as well as the possibility that binarization may not be accurate. However, for the purposes of this study we have used the term in the same way as used in the literature, without any other meaning attached. Several studies have reported a clear gender bias in student evaluation where male instructors receive significantly higher ratings as compared to female instructors (Basow, 1995; Centra and Gaubatz, 2000), even if objectively measured student learning is the same (Boring, 2017). Men are often assumed to have more command over their subject matter while women are expected to prove themselves first. Studies have showcased imbalances, where male instructors called “professors” and female instructors are referred to as “teachers” (Miller and Chamberlin, 2000), showcasing lesser perceived stature of female academics compared to male academics.
Sinclair and Kunda (2000) found in their study that female instructors were viewed as less competent if the feedback they had provided to students was negative, while the impact of the student feedback was not as influential for their male counterparts. A meta-analysis (Boring et al., 2016) showed that the bias affects how students rate even putatively objective aspects of teaching, such as how promptly assignments are graded. It is not possible to adjust for the bias, because it depends on so many factors. A few studies, however, have reported no differences in evaluations based on gender (Basow, 1995), while other studies reveal certain factors that impact both male and female lecturers, such as their perceived beauty (Hamermesh and Parker, 2005).
When discussing racial bias in student evaluations, Smith (2007) found that black lecturers tended to receive lower ratings than white lecturers. Although various studies report interaction effects between student and teacher characteristics, they are not always consistent. While studies show a strong in-group bias toward gender, where male students tend to give higher scores to male lecturers, this isn't always the case. In terms of racial bias, Chisadza et al. (2019) reported that black students gave lower scores to black lecturers as compared to white lecturers.
The existence of racial bias against faculty can also be seen through the perspective of spoken accent as well, as seen amongst Asian instructors (Subtirelu, 2015). As is often the case with these findings, discourse amongst students is usually subtle rather than overtly discriminatory. This negative impact is not just limited to student evaluations, but also permeates through regular interactions and delivery of teaching. According to the accounts provided by 14 African American faculty members on a campus mainly comprising White students, there are indications of micro-insults in their interactions (Pittman, 2012). Harlow (2003) discusses that the devalued racial status of black professors as compared to their White colleagues leads to much more complex emotion management in the classroom. The consistency of these findings clearly warrants further attention, as diversity in academia has been repeatedly shown to have an overall positive impact on various aspects of education, including employment of a broader range of pedagogical techniques and more frequent interactions with students (Umbach, 2006).
However, in general, when studying differences in race and ethnicity, the literature is mainly focused on Caucasian, African American, and Hispanic instructors. There is little related information from South Asia or the Middle East. The United Arab Emirates (UAE) in particular has a diverse mix of cultures and populations. Out of 88% of expatriates, 59% have a South Asian descent in the UAE.1 Given the large South Asian expatriate population, not only are there a large number of South Asian instructors, but also a significant number of students from South Asian countries as well. This provides a great opportunity to investigate the existence of biases with this community while still being situated in an ethnically diverse location.
The typical design when addressing bias in student evaluations tends to follow one of two approaches—Using actual student evaluations during the course of a programme, or a simulated design. Although there is merit in using real student evaluations of their instructors, it does make it tricky to isolate the individual variables of interest. In order to specifically look at a particular variable such as gender, race, age, personality, or teaching style, a simulated design shows more potential. As an illustration of this methodology, MacNell et al. (2015) developed an online simulated learning environment to study gender biases by only manipulating the name of the instructor, independent of the instructor's actual gender. Similarly, Chisadza et al. (2019) used videos of various instructors delivering the exact same content in order to focus specifically on the race and gender of the instructor.
Further expanding on the methodologies mentioned earlier, it is important to consider their respective benefits and limitations. Using actual student evaluations of instructors in an observational setting could provide a more holistic perspective, encapsulating students' perceptions not limited to instructors' characteristics in terms of race or gender, but also to their pedagogical techniques, classroom environment, and interpersonal dynamics. However, the multifaceted nature of these evaluations, though rich in context, presents challenges in pinpointing specific biases. While the ecological validity is strong in this case, it isn't an effective approach when trying to isolate specific variables. On the other hand, simulated designs allows a much higher degree of control. By manipulating specific attributes, such as race or gender, and keeping all other factors constant, these designs aim to provide clearer insights into the presence and magnitude of specific biases. The primary concern here, though, is the potential compromise in external validity. While these simulations control for confounding variables, they might not encapsulate the full breadth and depth of actual classroom interactions over an extended period of time. This is important to note as the experimental setup in this case is not a natural representation of a real-world classroom setting. Thus, while in the observational studies, results could be attributed to several aspects including context, subject matter and underlying bias, the simulated design can be used to isolate and measure a specific type of bias. Given the lack of context in simulated design, it might not be considered appropriate to generalize the results to real-world settings. However, its strength lies in pinpointing and providing clear evidence of biases that might otherwise be obscured in observational data. This evidence could act as a foundational basis, allowing researchers to infer and further investigate such biases in more natural environments.
Upon further examination of existing studies, it becomes evident that they often have been non-experimental in nature. These studies typically fall into two categories: those that compared course evaluations with student demographic variables (as seen in Marsh, 1980), and those that delved into the emotional aspect of student assessments of teaching, identifying crucial domains for teaching effectiveness (Dziuban and Moskal, 2011). Few studies have looked into post-course evaluations to compare ratings between male and female instructors. Those that did primarily focused on how the stereotypes present in student responses correlated with actual knowledge and exam results (Boring, 2017).
However, it should be noted that the research was carried out in face-to-face classes. Consequently, even when there was a random assignment of students to different instructors, the level of experimental control over variables such as lecture content, the physical attributes and attire of the lecturer, non-verbal cues, and basic attention was insufficient. In our study, we aimed to minimize the influence of as many potentially confounding variables as possible. To achieve this, we conducted our research using online avatars, which allowed for extensive experimental control over these factors.
In terms of simulated design, extensive research has been carried out on developing and understanding the use of “pedagogical agents”, which are virtual avatars designed to “facilitate learning in computer mediated learning environments” (Baylor and Kim, 2005). In a series of studies, several factors such as ethnicity (Caucasian vs. African American) (Baylor, 2005; Kim and Wei, 2011), gender, avatar realism, and delivery style (Baylor and Kim, 2004) were tested using these agents, mainly from the perspective of understanding student preference and improving learning outcomes (see Kim and Baylor, 2016 for review). The typical results seemed to show student preference toward instructors belonging to the same ethnicity and/or gender as themselves. However, the focus of these studies was also toward developing agents and understanding student preference, in addition to exploring various implicit biases students may have when evaluating an instructor's teaching. Furthermore, the role of pedagogical agents has been typically viewed as a support and supplement for student learning outside the classroom, rather than replacing the instructor. Regardless, these results inform us about the biases that might be expected when exploring these biases in an online setting. Avatars have been used extensively when carrying out social psychology research, especially ones that involve observing or mitigating bias. Virtual Reality (VR) has been used to immerse participants in realistic scenarios where the type of avatar that they “embody” and the type of avatar they interact with, can have a significant impact on their cognition, bias, perception as well as decision-making. Particularly in terms of bias, several studies have shown that exposure to avatars belonging to a specific race can illustrate the existence of such biases, as well as lead to mitigation, depending on the scenario depicted (Banakou et al., 2016, 2020; Hasler et al., 2017).
In addition, in the last few years online education has gained immense popularity. Especially in recent times due to the COVID-19 pandemic, several universities had to shift from physical classes to remote delivery, with various tools being utilized to emulate the “classroom experience” as closely as possible (Rapanta et al., 2020). One of the methods for online education that is gaining momentum is using virtual environments, where the teacher and students are represented by virtual avatars. Petrakou (2010) used the software “Second Life” to deliver an online course and reported enhanced interactivity between all participants. Furthermore, since head-mounted displays (HMDs) have become more affordable, Virtual Reality (VR) is also being utilized to conduct classes and carry out collaborative activities, with varying degrees of success (Freina and Ott, 2015). Furthermore, given the increasing popularity of the “Metaverse”, the trend toward online virtual interactions using avatars is predicted to become increasingly commonplace (Altundas and Karaarslan, 2023). Keeping this trend in mind, it is important to understand how avatars as instructors are perceived by students, and if these well-documented biases carry over to virtual characters as well.
In this particular study, we explore discrepancies in student evaluations of virtual instructors in an online setting, based on the instructor's race (South Asian or White) and gender (Female or Male). Although the design follows a simulation-based approach similar to Chisadza et al. (2019), instead of recording videos of instructors, the study uses customized 3D virtual characters, or avatars, to deliver the teaching material. As mentioned earlier, selecting this approach has several benefits, the most significant being the ability to have a high level of control over the teacher's appearance. This enables us to isolate specific elements while keeping all other variables consistent across different situations. Given the lack of literature with respect to these biases among South Asian faculty, in addition to evaluating their teaching, it is also crucial to understand how the instructors are perceived in terms of their personality.
2 Methodology
2.1 Participants
We conducted a power analysis to determine our sample, which suggested that we required a minimum of 125 participants to reduce type II error, have 0.80 power, effect size of at least 0.15, and a p-value less than 0.05. A sample consisting of 331 participants was recruited in October–May of 2021–2022 through convenience sampling, using social media platforms such as Reddit, Telegram, and Instagram, and in University seminars of the branch campus of a British university in Dubai. The survey was created using Qualtrics. Since forced responses were used, we did not lose a lot of data and only 13 participants were excluded from the final sample as they were High School students, did not qualify due to duplicate cases, or simply did not consent to the study. No one failed the attention test, providing the correct answers to at least two questions out of four. The overall sample comprised of 318 participants (Mage = 20.37, SDage = 3.01, age range = 17–43). Of these, 77.04% were female (n = 245), 21.38% were male (n = 68) and 1.58% (n = 5) preferred not to reveal their gender. The majority of participants reside in the United Arab Emirates (76.42%, n = 243) and are South Asians (65.72%, n = 209), whereas only 8 participants (2.5%) identified as Caucasians and 11 (3.5%) as having a mixed background.
The participants had diverse levels of education, consisting of individuals with a Doctoral-Level Qualification (0.31%), Postgraduate qualification (3.14%), Undergraduate qualification (52.52%), and current Undergraduate students with a High School Diploma (44.03%). 99.06% of participants were in a classroom setting within the last 5 years, and 84.90% were currently enrolled as students. 33.96% of participants were psychology majors (n = 108), 11.95% studied computer science (n = 38), 9.75% studied business administration (n = 31), and the rest (44.34%, n = 141) were majoring in courses such as engineering, medicine, law, media, tourism, and finance.
2.2 Procedure and design
The study featured a 2 × 2 between-subjects design with the factors: gender of a teacher (male, female) and ethnicity of a teacher (White and South Asian). To observe differences in student evaluation, the researchers created four videos where a virtual character (an animated avatar) delivered a 5-min introduction to a social psychology lecture. All videos were identical in terms of the script that the characters articulated, and the environment, including the background of the room and their clothes. The researchers were careful not to prime participants with information about these characters except for two manipulated factors (gender and ethnicity). Thus, all participants were randomly assigned to one of four videos with either White or South Asian, male, or female teachers. The study received an approval from the University's Ethics Committee.
2.3 Materials
The avatars for the virtual instructors were created using the software Character Creator 3 by Reallusion.2 The focus of the study was to observe differences in student evaluation and perceived learning when teaching was carried out via video, by a virtual character, with certain specific characteristics. By manipulating one factor at a time (gender and race) and keeping everything else consistent, the aim was to explore discrepancies in the ratings based on the participants' experience. Thus, four avatars were created, one for each experimental group—Male White (MW), Male South Asian (MSA), Female White (FW), Female South Asian (FSA). In order to ensure consistency, the facial features of each avatar were kept as neutral as possible. Both avatars from the same race had the exact same skin, hair, and eye color. Avatars of the same gender had the exact same hairstyle, facial structure, and features, while the skin tone and eye color modified to match the race. Subtle modifications were applied to other features in order to represent the avatar's race and gender more accurately. Figure 1 shows the four avatars that were used for creating the videos.
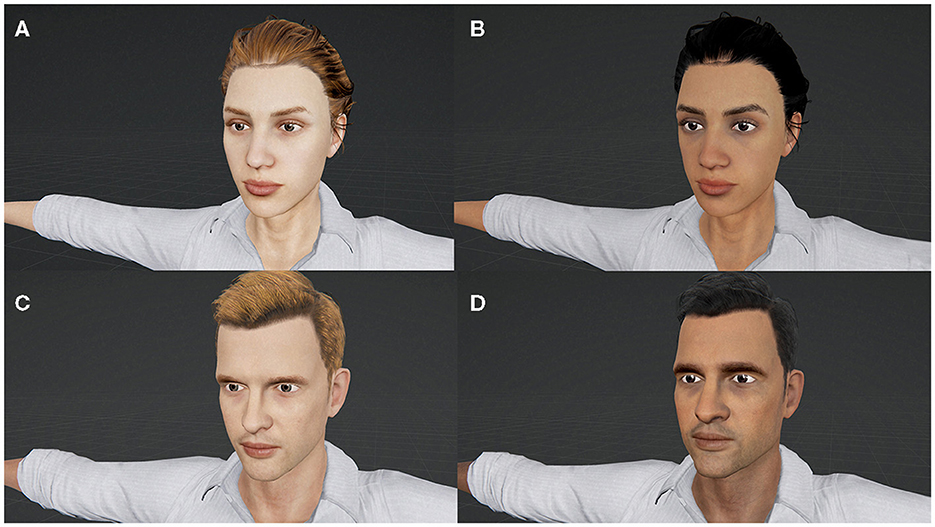
Figure 1. Screenshots of avatars used for creating online teaching videos: (A) White-Female, (B) South Asian-Female, (C) White-Male, (D) South Asian-Male.
The script for the lecture was developed in line with the actual social psychology module taught in the undergraduate psychology programme. Three audio versions of the script were recorded for each instructor, with the voice matching the gender and race. Two independent coders, South Asian and White, were asked to rate each voice for each condition with the following question: “Please rate the voice you just heard on a scale from 1 to 7, where 1 denotes least likely and 7 denotes most likely to sound like an instructor teaching in the UAE”. Individual ratings for each voice can be found in the Supplementary File. Once the audio version of the script was finalized, it was connected to the avatar and the real-time lip-syncing SDK developed by Oculus3 was used to give the illusion that the avatar was speaking the dialogue. The entire scenario with the avatar and the 3D environment was developed in Unity3D.4 Once recorded, the video was processed in Adobe Premiere,5 with the online interface added and the slides from the material integrated into the final version. A Supplementary Video demonstrating the 4 videos that were created, along with a brief explanation of the methodology and results can be found here.6 Specifically, the video showcases the actual delivery of the content by the various avatars and the online environment that was overlaid, which would allow researchers to replicate the study by following a similar approach. The video development platform is part of a larger project, currently being used for a follow-up study.
After watching a video, a manipulation check was administered through a brief attention test to ensure that the participant had watched the video carefully. The questions were designed to ensure that the questions included generic details and did not prime the participants in any way. The following questions were asked, “According to the lecture, is social psychology considered a science?”, “Which research method did the lecturer NOT mention?”, “What was the color of the shirt that the lecturer was wearing?” and “Did the lecturer have a middle name?”. Data from the participants who would not be able answer at least two of the four questions correctly would be excluded from the analysis. After the attention test, participants were provided different questions for teaching evaluation.
To measure different aspects of teaching evaluation, researchers adapted the questionnaire developed by Basow (1995). The original questionnaire contained 14 questions related to teacher behavior (including an overall teaching rating) (e.g., “Instructor treats students with respect”) and five questions relating to the course (e.g., “Exams and papers appropriate”). For the purposes of this study, questions related to the course were not included since we felt that they were not applicable. In the original paper, since each question has been analyzed as an independent factor; thus, we only items relevant to the study were included. That resulted in 8 questions being chosen from the original 14. Items which were either unmeasurable in this particular study or did not match the context of the data collection (brief online video delivered by an avatar), such as “Instructor gives good feedback on writing” or “Instructor is fair and impartial”, were eliminated. Essentially, we removed all the items where there was an implied two-way interaction with the student or the class. More detailed rationale for each of the statements that have been included and excluded are provided in the Supplementary File. Questions were rated on a 5-point Likert scale ranging from completely disagree (1) to completely agree (5).
Participants were also asked to evaluate how approachable the instructor was (“Instructor appears approachable”) on a 7-point Likert scale from poor (1) to excellent (7). This question was adopted from Ryu and Baylor (2005) Pedagogical Agent Persona. In addition to that, participants were asked to evaluate their willingness to study more from this lecture (“I would have liked to study more from this lecturer”) on a 5-point Likert scale ranging from completely disagree (1) to completely agree (5).
The assumption of the design is that people would rely on stereotypes more upon brief interactions compared to a long term contact. Therefore, it is possible that evaluations based on a short video is equally relevant, and in some cases, a more effective design to study biases compared to assessing students' perspectives at the end of the term. However, it is important to note that Basow (1995) had administered the student evaluation questionnaire at the end of term. We adapted it to a simulated design which included watching a brief video of a lecturer.
2.4 Analytical approach
A series of 2 (Male, Female) × 2 (South Asian, White) independent group design Factorial ANOVAs were administered to examine whether gender and race had a significant impact on students' evaluation of lecturers based on an online teaching video. Data was screened for outliers. Levene's test was reported based on median scores to avoid the influence of extreme scores. This test was preferred over others due to its familiarity and robustness. The non-parametric Mann–Whitney test was administered to compare independent groups when Levene's test was significant. All the analysis was re-administered with the gender of the participant as a covariate, and findings related to it have been reported separately. Given the exploratory nature of the study, each item was explored separately. As mentioned before, this approach is inspired by a few research designs of previous literature (Basow, 1995; Boring et al., 2016). In order to control for the risk of inflated error rate due to multiple analysis, Benjamini–Hochberg correction was administered and findings are reported in the Supplementary File. The project is available at Open Science Framework and can be accessed here: https://osf.io/fcx67/?view_only=a4fd9739a91647ca8cd41739f64aab5d.
3 Results
We administered ten factorial ANOVAs to explore whether instructors' gender, ethnicity, and its interaction had a significant impact on students' perception of the lecturers when the lecture was delivered online by a virtual agent. Table 1 provides a summary of the various results, each of which are discussed in detail below.
3.1 Instructor appeared sensitive to students' feelings
While instructor's gender was not found to be significant, participants perceived South Asian instructors were significantly more sensitive to their feelings [F(1, 314) = 4.71, p = 0.03, η2 = 0.01] compared to white instructors. Given Levene's test was significant (p = 0.024), significant results were re-analyzed using non-parametric tests. Independent sample Mann–Whitney U-test confirmed the findings (p = 0.037). Figure 2 shows the boxplot of the responses for this statement, factored by race.
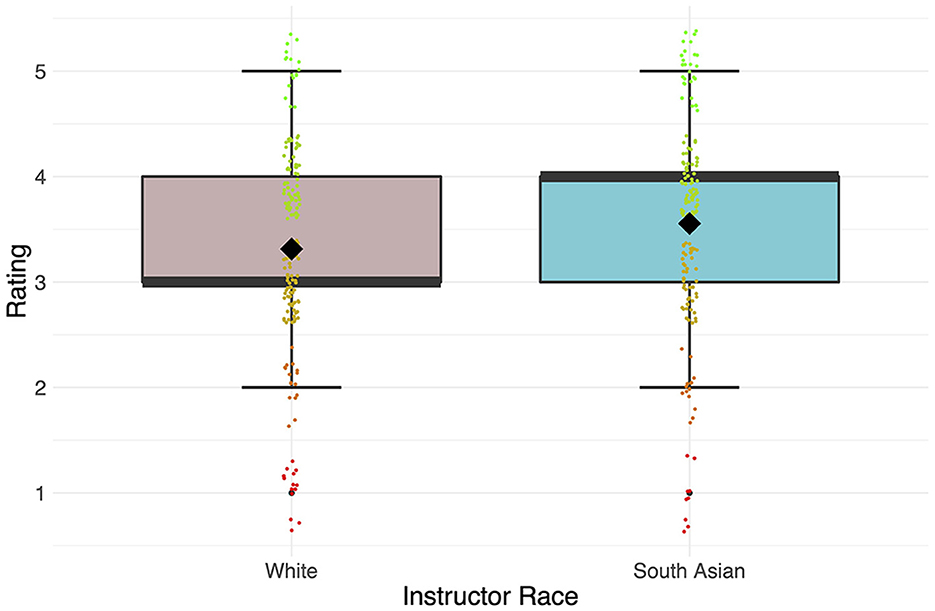
Figure 2. Box-and-whisker plot for “sensitivity toward students' feelings” for White and South Asian instructors. Ratings were on a Likert scale from 1 (completely disagree) to 5 (completely agree).
3.2 Instructor treats students with respect
Second factorial ANOVA showed that ethnicity did not significantly contribute to participants' perception of whether instructor treated the students with respect. However, participants perceived male instructors as showing more respect toward the students compared to female instructors [F(1, 314) = 4.36, p = 0.03, η2 = 0.01]. Levene's test was not significant (p = 0.06). Figure 3 shows the boxplot for the responses received for this statement, factored by gender.
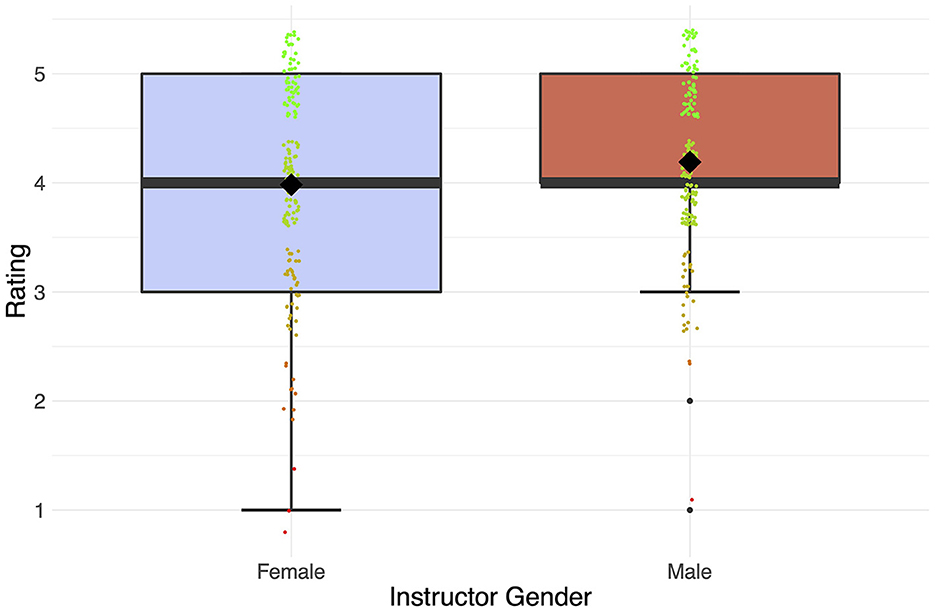
Figure 3. Box-and-whisker plot for “treating students with respect” for female and male instructors. Ratings were on a Likert scale from 1 (completely disagree) to 5 (completely agree).
3.3 Instructor speaks in appropriate manner
Ethnicity also did not significantly contribute to participants' perception of whether the instructor speaks in an appropriate manner. Participants, however perceived male instructors speaking significantly more appropriately than female instructors [F(1, 314) = 4.68, p = 0.03, η2 = 0.01]. Levene's test was not significant (p = 0.06). Figure 4 shows the boxplot for the responses to this statement, factored by gender.
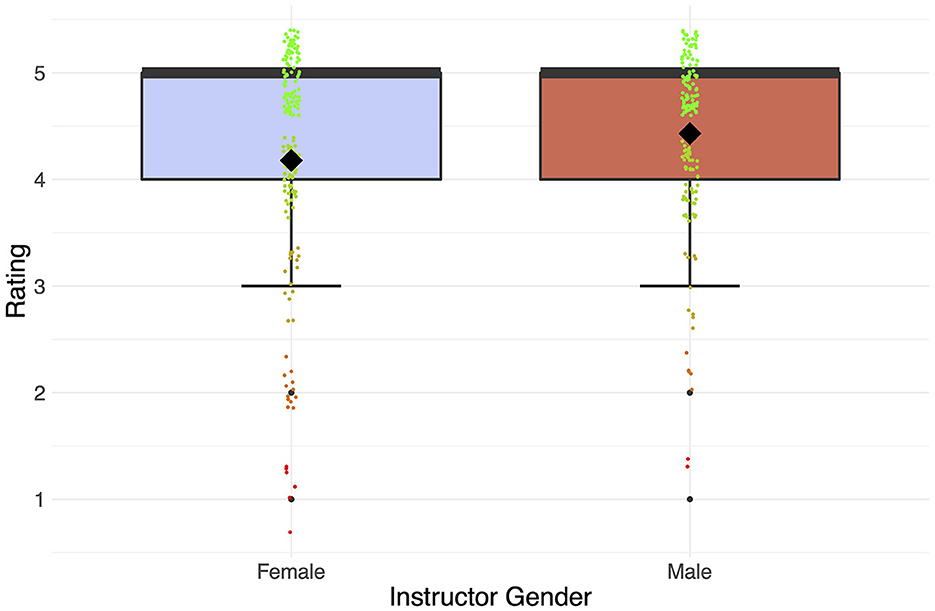
Figure 4. Box-and-whisker plot for “speaking in an appropriate manner” for female and male instructors. Ratings were on a Likert scale from 1 (completely disagree) to 5 (completely agree).
3.4 Instructor displays enthusiasm for subject
In terms of enthusiasm for the subject, male instructors scored significantly higher than female instructors [F(1, 314) = 6.40, p = 0.01, η2 = 0.02]. In addition, South Asian instructors displayed significantly more enthusiasm for the subject than white instructors [F(1, 314) = 6.26, p = 0.01, η2 = 0.02]. Levene's test was significant (p = 0.003), however the non-parametric test confirmed findings for both gender (p = 0.006) and ethnicity (p = 0.01). Figures 5, 6 show the boxplot of the responses to this statement, factored by gender and race, respectively.
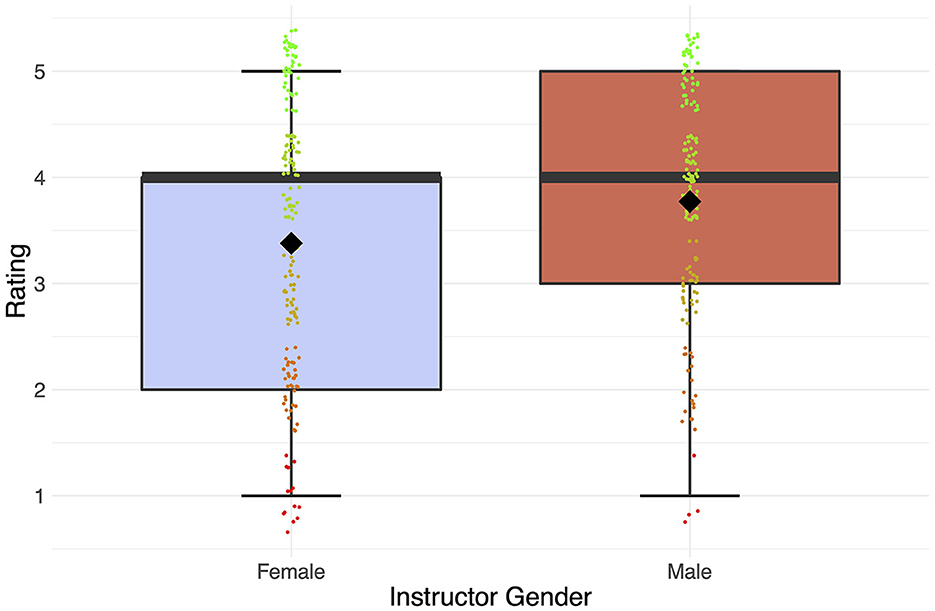
Figure 5. Box-and-whisker plot for “instructor enthusiasm” for male and female instructors. Ratings were on a Likert scale from 1 (completely disagree) to 5 (completely agree).
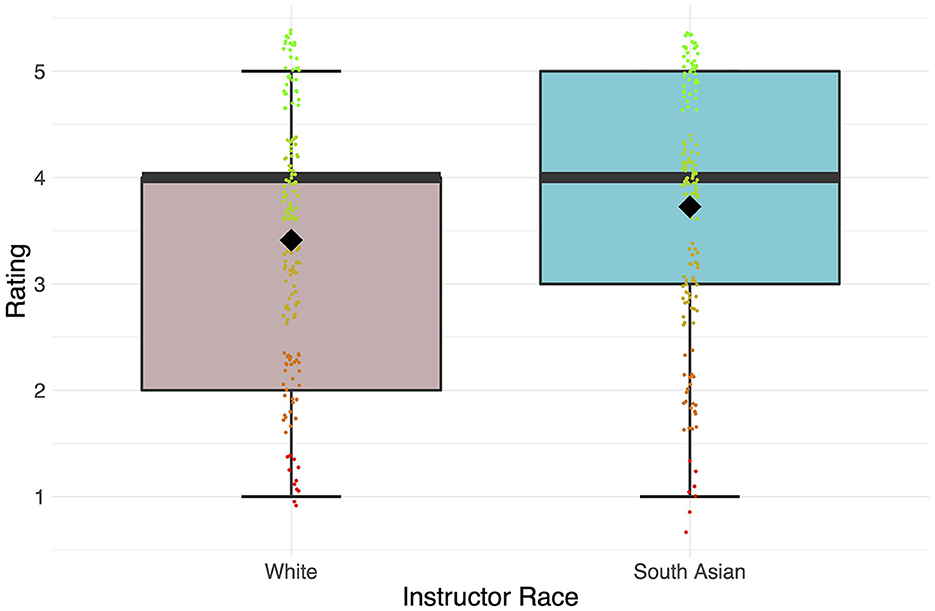
Figure 6. Box-and-whisker plot for “instructor enthusiasm” for White and South Asian instructors. Ratings were on a Likert scale from 1 (completely disagree) to 5 (completely agree).
3.5 Instructor stimulates your thinking, organizes the presentation well, and demonstrates knowledge of the subject
Race and gender were not significantly associated with participants' perception of whether the instructor stimulates their thinking, whether the instructor organizes the presentation well, and whether instructor demonstrates knowledge of the subject.
3.6 Overall instructor rating
While gender did not significantly contribute to participants' overall rating of the instructor, a non-significant trend suggested that South Asian instructors received higher overall ratings compared to white instructors [F(1, 327) = 3.32, p = 0.07, η2 = 0.01]. Levene's test was not significant (p = 0.23).
3.7 Approachability
The next factorial model showed that male and South Asian instructors were perceived to be significantly more approachable compared to female and white instructors, respectively, [F(1, 314) = 5.27, p = 0.02, η2 = 0.02, F(1, 314) = 13.33, p < 0.001, η2 = 0.00]. Levene's test was also not significant (p = 0.21). Figure 7 shows the boxplot for the responses factored by gender.
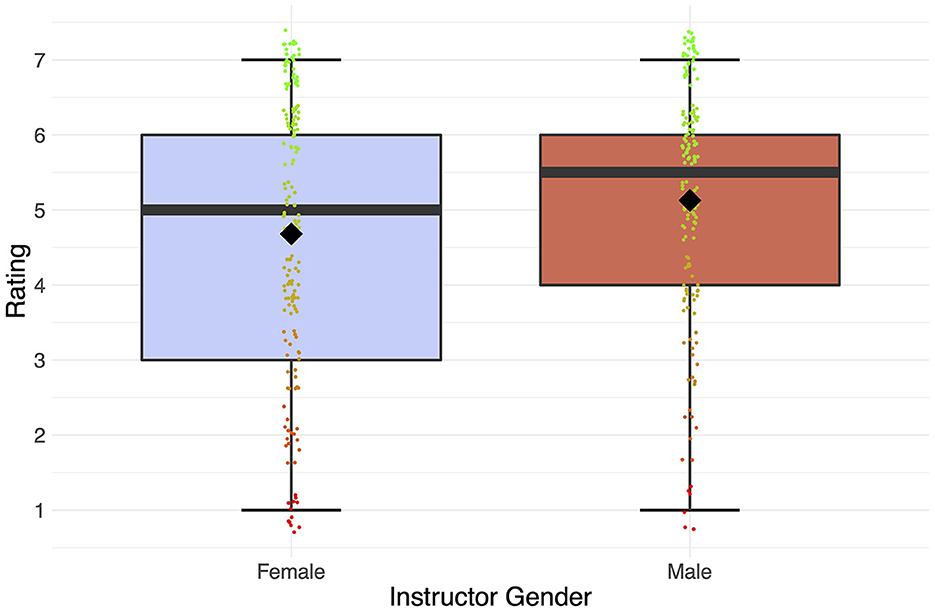
Figure 7. Box-and-whisker plot for “Approachability” for male and female instructors. Ratings were on a Likert scale from 1 (poor) to 7 (excellent).
3.8 Study more
Finally, it was found that students would have liked to study more from male instructors compared to female instructors [F(1, 314) = 4.53, p = 0.03, η2 = 0.01] and from South Asian instructors compared to white instructors [F(1, 314) = 5.77, p = 0.01, η2 = 0.01]. Levene's test was not significant (p = 0.053). Figures 8, 9 show the boxplot of the responses factored by gender and race, respectively.
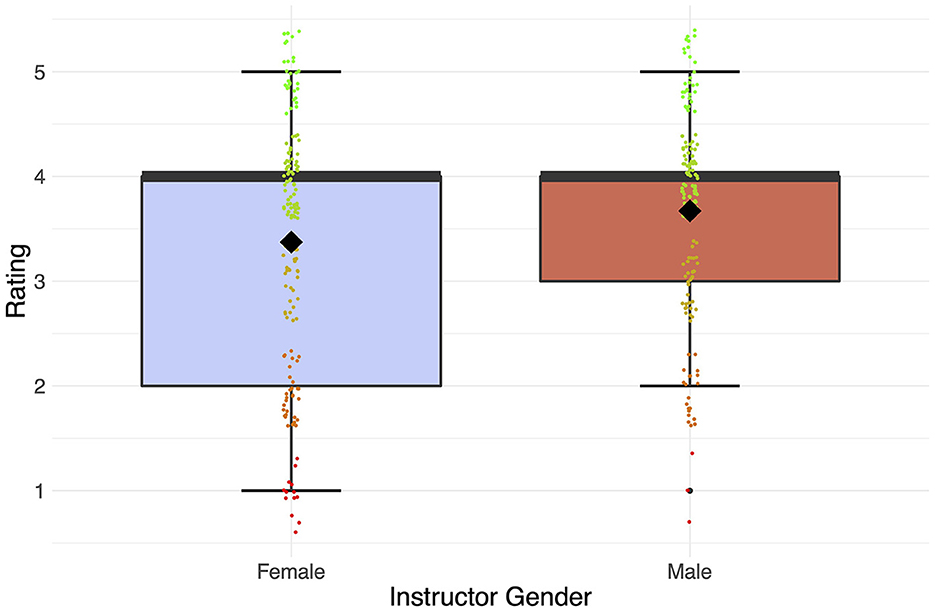
Figure 8. Box-and-whisker plot for “I would like to study more” from male and female instructors. Ratings were on a Likert scale from 1 (completely disagree) to 5 (completely agree).
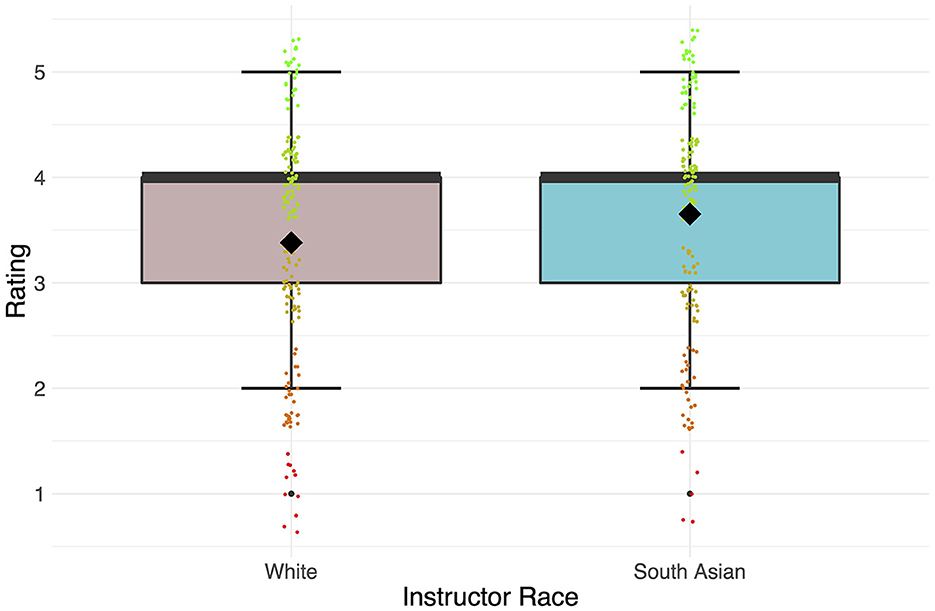
Figure 9. Box-and-whisker plot for “I would like to study more” from White and South Asian instructors. Ratings were on a Likert scale from 1 (completely disagree) to 5 (completely agree).
All the ANOVA models were re-administered with the gender of the participant as the control variable. Other demographic characteristics such as race and field of study were broad categorical variables and were not statistically appropriate to be added as control variables. We found that adding gender of the participant as a control variable only changed one finding: Gender of the lecturer no longer significantly impacted whether they were perceived to be more respectful toward the students (p = 0.06). When avatar gender was female, male students (M = 3.68, SD = 1.10) perceived that instructors treated students with less respect compared to female students (M = 4.07; SD = 0.90), but when avatar gender was male, both male (M = 4.16, SD = 0.73); and female (M = 4.18, SD = 0.78) students did not show much difference in their perception of level of respect the lecturer showed toward students.
In order to control for the potential inflated error rate, we also administered Benjamini–Hochberg method to adjust the p-values and control for false positives. Given we are exploring the biases in a new context, we chose a relatively lenient false discovery rate of 0.2. The tables for gender and race, with Benjamini–Hochberg correction, are provided in the Supplementary File. The findings are consistent with original results.
4 Discussion
Using a between group design, the current study examined if unconscious biases about instructors' race and gender impact participants' perception of instructors. Participants viewed a video of an avatar (Gender: Male or Female, Race: South Asian or White) teaching social psychology on an online teaching platform. It was found that both gender and race tend to have an impact on students' evaluation of their instructors. In particular, in the context of the UAE, participants showed a stronger bias toward male and South Asian instructors compared to female and White instructors. These differences were largely found in variables which tapped into instructor's personality traits or perceived interaction such as, being approachable and showing more enthusiasm toward the subject, instead of their knowledge or teaching skills, such as organizing presentation well and demonstrating knowledge of the subject.
In terms of gender, it was found that male instructors treated students with more respect and spoke in a more appropriate manner compared to female instructors. These findings seem to contradict Basow (1995) observations, who showed that female instructors were perceived as showing more respect and speaking more appropriately, compared to male instructors. However, there are several reasons to consider when making this comparison. Most importantly, while Basow (1995) used an observational design to measure bias by looking at end-of-semester student evaluations over a period of 4 years, we have employed a simulated approach. Although both methods have their merit, the results of these studies cannot be directly compared since there is a fundamental difference in the way bias is being measured. In observed scenarios, the results could be influenced by several factors, including interpersonal relationships, behaviors over a period of time, assessments, feedback, and complexity of the subject matter itself, which are impossible to account for. Whereas, in a simulated approach we can isolate and directly measure the impact of a specific factor, such as race or gender, on the evaluations. Furthermore, the difference could also be attributed to the fact that the two studies are separated by more than 25 years, and have been carried out in vastly different geographical locations and cultures.
However, findings are in line with relatively recent research administered in the U.S. (MacNell et al., 2015), whereby college aged participants perceived male instructors treating students with more respect compared to their female counterparts. Perhaps the respect perceived by the students could be a projection of one's emotions onto the instructor as previous research shows that typically students tend to give more respect to male instructors, referring to them as “professors” as compared to “teachers” for female instructors (Miller and Chamberlin, 2000). However, when gender of the participant was added as a control variable, the finding was no longer significant, and it was found that male students perceived female lecturers to be less respectful compared to male lecturers. Given respect for students is one of the most important dimensions of teacher's perceived effectiveness (Feldman, 1976; Patrick and Smart, 1998) and is one of the highest-ranked dimensions in terms of importance for students (Feldman, 1976), these findings require consideration, especially for female academics.
Basow et al. (2006) have previously shown that students most often described their “best professors” as more approachable, knowledgeable, and enthusiastic. The current findings show a significant bias toward male instructors being viewed as more approachable and enthusiastic about the subject than female instructors. However, gender-based bias did not emerge in the domains of knowledge, presentation skills, and stimulating thinking; indicating that differences exist more in interpersonal variables.
While Basow (2000) has previously shown that male participants may view female instructors as more approachable, the trend of viewing lecturers of opposite sex more approachable did not emerge with female participants. Therefore, the current research, with a greater proportion of female participants, is not in line with those findings. These findings also contradict the common notion that attitudes toward females are dependent on internalized gender schemas (Bennett, 1982; Zikhali and Maphosa, 2012). It could also be assumed that the online teaching format does not allow teachers to fulfill students' gendered expectations (Ayllón, 2022). Perhaps online teaching does not allow lecturers to be as supportive and personable as in face-to-face classes, which leads to even greater burden for female instructors because students have higher interpersonal expectations of them.
Given enthusiasm is seen as a component of high-quality instruction, which seems to have a positive effect on learners' engagement, motivation, and willingness to learn (Turner et al., 1998; Witcher and Onwuegbuzie, 1999), it may lead to significant differences not only in students' learning experience but also in their eagerness to opt for a particular course, making their choice subconsciously based on instructor's gender. This was further indirectly confirmed by students' rating of their willingness to study more with male instructors compared to female instructors.
Overall, the findings are in line with previous research (Arrona-Palacios et al., 2020) that found that students tend to favor male academics over female academics. Given that many universities offer optional modules, consistent student disinterest could lead to reconsideration of these offerings, which may, in turn, impact female professors assigned to these courses.
Given the importance of race for teaching evaluation highlighted by previous research (Harlow, 2003; Pittman, 2012; Chisadza et al., 2019), this study evaluated biases related to instructor's race. No differences were found in several domains including knowledge and presentations skills, which is discussed below. However, in the domains where differences in the evaluation were observed, students displayed a strong preference toward South Asian instructors compared to White instructors in the UAE. Students viewed South Asian instructors as more sensitive to their feelings, enthusiastic for the subject, approachable, and showed more willingness to study more from, compared to White lecturers. Again, greater differences were observed in perceived interpersonal interaction. While race as a variable has been explored less in the context of teaching evaluation and implicit biases in comparison to gender, largely the findings of this research contradicts previous research which suggests that students are more biased against lecturers of color, especially when they are of the same race, evident in the research findings from South Africa on bias against black lecturers compared to white lecturers (Chisadza et al., 2019).
It was found that in some domains, gender and ethnicity did not influence teaching evaluation. Avatars were relatively similarly rated despite their ethnicity or gender when participants evaluated the instructor's knowledge, the extent to which instructors stimulated their thinking; how well they organized presentations; and overall instructor rating. There seems to be something common in all these aspects of teaching evaluation, and they could be described as the instructor's level of professionalism or knowledge. These results confirm findings from previous studies (Boyd and Grant, 2005; Zikhali and Maphosa, 2012), suggesting that competence was not determined by gender and ethnicity.
Observed differences cannot be attributed to differences in performance because each avatar had the exact same script, nor can it be attributed to the differences in personal presentations, dressing, body language, or facial characteristics, as it was matched across avatars. Moreover, although objective evaluation students' learning was not logical since they only watched a short video, in the studies where students' learning was compared, no differences were observed between female and male lecturers (Boring, 2017). Thus, it seems to be a plausible conclusion that the emerged differences could be explained by the participants' implicit biases.
This study provides several contributions to the emerging literature about online teaching and gender and race biases that may impact teaching evaluation. First, differences in terms of gender and race tend to be strong when students evaluate interpersonal interaction or personality. Based on demographic characteristics, largely it can be argued that in-group preferences sustained for race but not for gender. Finally, it is also important to note that wherever there were significant differences, biases were consistently in favor of male and South Asians compared to female and White instructors respectively.
The convenience sampling method might be a limitation of this study, as it limits generalizability, leading to potentially biased results. However, it is also important to note the demographic landscape of the UAE. As mentioned earlier, the UAE comprises 88% expatriates, of which 59% are South Asian. Most participants in this study were from South Asian cultural backgrounds. Therefore, it could be assumed that a greater rating for South Asian instructors might be attributed to in-group favoritism (Chisadza et al., 2021). Furthermore, the representation of different ethnic groups in the university workforce can make a difference in students' evaluation (Fan et al., 2019). As for the greater representation of female students, considering most students were majoring in psychology, it represents the gender composition of psychology graduate programs. However, this also limits the study's generalizability since psychology students would be the only ones that would have had some pre-existing knowledge about the subject, whereas participants belonging to other majors (computer science, media, and so on) might not have found the course content as relevant.
Another aspect to consider when discussing generalizability is the fact that this study employed a simulated design. As discussed earlier, while simulated designs provide great control over the environment and variables being measured, they come at a loss of real-world context. Additionally, while the observed design typically relies on a longer term interaction between students and instructors, simulated designs, such as ours, have a much shorter exposure time. Our goal with this type of research is to understand, observe and develop ways to mitigate these biases in the real-world. When discussing the results of this study, it is important to state that although we cannot generalize these results to the population, it does provide us with insight on the factors that might cause similar results in observational studies as well.
Future research could add stricter attention questions and measure objective learning. Greater sample size would facilitate greater generalizability and stronger effect sizes. It could also aim for a more balanced representation of participants, in terms of gender, educational background, and race. Furthermore, while we designed avatars to provide a natural representation of the gender or race they belonged to, we did not explicitly ask participants whether they were able to successfully recognize the factor. This could be important when studying more subtle differences, such as facial features or educational qualifications. Studies could also compare findings based on live teaching in the region. Another pertinent factor that should be investigated is that of age. The current study controlled for age by having relatively young avatars across all four instructors, which should be addressed by future research comparing teaching evaluations for older and younger instructors. Having an experimental design with avatars, unique cultural context which is different from majority of the previous literature, and an online platform are important strengths of the study.
The findings also suggest that relying on teaching evaluation may not be effective, especially when lecturers are being compared across gender and race, as this process could be discriminatory. Perhaps universities should consider ways to reduce such biases, by having a better gender and ethnicity representation (Fan et al., 2019) or by discussing such biases openly with students, making them consciously aware of it, and helping them to identify strategies that can help overcome the bias (Paluck et al., 2021).
Data availability statement
The raw data supporting the conclusions of this article will be made available by the authors, without undue reservation.
Ethics statement
The studies involving humans were approved by Ethics Committee at Middlesex University Dubai. The studies were conducted in accordance with the local legislation and institutional requirements. The participants provided their written informed consent to participate in this study.
Author contributions
NL, SK, and OK contributed to conception and design of the study. NL performed the statistical analysis. SK created online videos with virtual avatars for data collection. All authors contributed toward the data collection, manuscript revision, read, and approved the submitted version.
Acknowledgments
The authors would like to thank Eleanor Pinto Abigail and Robyn Leigh Morillo Capio for their assistance with data collection. The authors would also like to thank Kanaka Raghavan, Eleanor Theodore Roosevelt, Tanmay Dhall, and Stuart Maggs for recording the final versions of the audio for the avatars.
Conflict of interest
The authors declare that the research was conducted in the absence of any commercial or financial relationships that could be construed as a potential conflict of interest.
Publisher's note
All claims expressed in this article are solely those of the authors and do not necessarily represent those of their affiliated organizations, or those of the publisher, the editors and the reviewers. Any product that may be evaluated in this article, or claim that may be made by its manufacturer, is not guaranteed or endorsed by the publisher.
Supplementary material
The Supplementary Material for this article can be found online at: https://www.frontiersin.org/articles/10.3389/feduc.2023.1237672/full#supplementary-material
Footnotes
1. ^https://www.un.org/development/desa/en/news/population/international-migrant-stock-2019.html
2. ^https://www.reallusion.com/character-creator/
3. ^https://developer.oculus.com/documentation/unity/audio-ovrlipsync-unity/
References
Altundas, S., and Karaarslan, E. (2023). “Cross-platform and personalized avatars in the metaverse: ready player me case,” in Digital Twin Driven Intelligent Systems and Emerging Metaverse, eds E. Karaarslan, Ö. Aydin, Ü. Cali, and M. Challenger (Singapore: Springer Nature Singapore), 317–330.
Arrona-Palacios, A., Okoye, K., Camacho-Zuñiga, C., Hammout, N., Luttmann-Nakamura, E., Hosseini, S., et al. (2020). Does professors' gender impact how students evaluate their teaching and the recommendations for the best professor? Heliyon 6, e05313. doi: 10.1016/j.heliyon.2020.e05313
Ayllón, S. (2022). Online teaching and gender bias. Econ. Educ. Rev. 89, 102280. doi: 10.1016/j.econedurev.2022.102280
Banakou, D., Beacco, A., Neyret, S., Blasco-Oliver, M., Seinfeld, S., and Slater, M. (2020). Virtual body ownership and its consequences for implicit racial bias are dependent on social context. R. Soc. Open Sci. 7, 201848. doi: 10.1098/rsos.201848
Banakou, D., Hanumanthu, P. D., and Slater, M. (2016). Virtual embodiment of white people in a black virtual body leads to a sustained reduction in their implicit racial bias. Front. Hum. Neurosci. 10, 601. doi: 10.3389/fnhum.2016.00601
Basow, S. A. (1995). Student evaluations of college professors: when gender matters. J. Educ. Psychol. 87, 656–665.
Basow, S. A. (2000). Best and worst professors: gender patterns in students' choices. Sex Roles 43, 407–417. doi: 10.1023/A:1026655528055
Basow, S. A., Phelan, J. E., and Capotosto, L. (2006). Gender patterns in college students' choices of their best and worst professors. Psychol. Women Q. 30, 25–35. doi: 10.1111/j.1471-6402.2006.00259.x
Baylor, A. L. (2005). “The impact of pedagogical agent image on affective outcomes,” in International Conference on Intelligent User Interfaces (San Diego, CA), 29.
Baylor, A. L., and Kim, Y. (2004). “Pedagogical agent design: the impact of agent realism, gender, ethnicity, and instructional role,” in Intelligent Tutoring Systems, eds J. C. Lester, R. M. Vicari, and F. Paraguaçu (Berlin; Heidelberg: Springer), 592–603. doi: 10.1007/978-3-540-30139-4_56
Baylor, A. L., and Kim, Y. (2005). Simulating instructional roles through pedagogical agents. Int. J. Artif. Intell. Educ. 15, 95–115.
Bennett, S. K. (1982). Student perceptions of and expectations for male and female instructors: evidence relating to the question of gender bias in teaching evaluation. J. Educ. Psychol. 74, 170.
Boring, A. (2017). Gender biases in student evaluations of teaching. J. Public Econ. 145, 27–41. doi: 10.1016/j.jpubeco.2016.11.006
Boring, A., Ottoboni, K., and Stark, P. B. (2016). Student evaluations of teaching (mostly) do not measure teaching effectiveness. ScienceOpen Res. doi: 10.14293/S2199-1006.1.SOR-EDU.AETBZC.v1
Boyd, E., and Grant, T. (2005). Is gender a factor in perceived prison officer competence? Male prisoners' perceptions in an English dispersal prison. Crim. Behav. Mental Health 15, 65–74. doi: 10.1002/cbm.37
Centra, J. A., and Gaubatz, N. B. (2000). Is there gender bias in student evaluations of teaching? J. Higher Educ. 71, 17–33. doi: 10.2307/2649280
Chávez, K., and Mitchell, K. M. W. (2020). Exploring Bias in student evaluations: gender, race, and ethnicity. Polit. Sci. Polit. 53, 270–274. doi: 10.1017/S1049096519001744
Chisadza, C., Nicholls, N., and Yitbarek, E. (2019). Race and gender biases in student evaluations of teachers. Econ. Lett. 179, 66–71. doi: 10.1016/j.econlet.2019.03.022
Chisadza, C., Nicholls, N., and Yitbarek, E. (2021). Group identity in fairness decisions: discrimination or inequality aversion? J. Behav. Exp. Econ. 93, 101722. doi: 10.1016/j.socec.2021.101722
Dziuban, C., and Moskal, P. (2011). A course is a course is a course: factor invariance in student evaluation of online, blended and face-to-face learning environments. Internet Higher Educ. 14, 236–241. doi: 10.1016/j.iheduc.2011.05.003
Fan, Y., Shepherd, L. J., Slavich, E., Waters, D. D., Waters, D., Waters, D., et al. (2019). Gender and cultural bias in student evaluations: why representation matters. PLoS ONE 14, e0209749. doi: 10.1371/journal.pone.0209749
Feldman, K. A. (1976). The superior college teacher from the students' view. Res. Higher Educ. 5, 243–288.
Freina, L., and Ott, M. (2015). “A literature review on immersive virtual reality in education: state of the art and perspectives,” in Proceedings of eLearning and Software for Education (eLSE) (Bucharest), 8.
Hamermesh, D. S., and Parker, A. M. (2005). Beauty in the classroom: instructors' pulchritude and putative pedagogical productivity. Econ. Educ. Rev. 24, 369–376. doi: 10.1016/j.econedurev.2004.07.013
Harlow, R. (2003). “Race doesn't matter, but...”: the effect of race on professors' experiences and emotion management in the undergraduate college classroom. Soc. Psychol. Q. 66, 348–363. doi: 10.2307/1519834
Hasler, B. S., Spanlang, B., and Slater, M. (2017). Virtual race transformation reverses racial in-group bias. PLoS ONE 12, e0174965. doi: 10.1371/journal.pone.0174965
Kim, Y., and Baylor, A. L. (2016). Research-based design of pedagogical agent roles: a review, progress, and recommendations. Int. J. Artif. Intell. Educ. 26, 160–169. doi: 10.1007/s40593-015-0055-y
Kim, Y., and Wei, Q. (2011). The impact of learner attributes and learner choice in an agent-based environment. Comput. Educ. 56, 505–514. doi: 10.1016/j.compedu.2010.09.016
Kreitzer, R. J., and Sweet-Cushman, J. (2022). Evaluating student evaluations of teaching: a review of measurement and equity bias in SETs and recommendations for ethical reform. J. Acad. Eth. 20, 73–84. doi: 10.1007/s10805-021-09400-w
MacNell, L., Driscoll, A., and Hunt, A. N. (2015). What's in a name: exposing gender bias in student ratings of teaching. Innovat. Higher Educ. 40, 291–303. doi: 10.1007/s10755-014-9313-4
Marsh, H. W. (1980). The influence of student, course, and instructor characteristics in evaluations of university teaching. Am. Educ. Res. J. 17, 219–237.
Miller, J., and Chamberlin, M. (2000). Women are teachers, men are professors: a study of student perceptions. Teach. Sociol. 28, 283–298. doi: 10.2307/1318580
Paluck, E. L., Porat, R., Clark, C. S., and Green, D. P. (2021). Prejudice reduction: progress and challenges. Annu. Rev. Psychol. 72, 533–560. doi: 10.1146/annurev-psych-071620-030619
Patrick, J., and Smart, R. M. (1998). An empirical evaluation of teacher effectiveness: the emergence of three critical factors. Assess. Eval. Higher Educ. 23, 165–178.
Petrakou, A. (2010). Interacting through avatars: virtual worlds as a context for online education. Comput. Educ. 54, 1020–1027. doi: 10.1016/j.compedu.2009.10.007
Pittman, C. T. (2012). Racial microaggressions: the narratives of African American faculty at a predominantly white university. J. Negro Educ. 81, 82–92. doi: 10.7709/jnegroeducation.81.1.0082
Rapanta, C., Botturi, L., Goodyear, P., Guàrdia, L., and Koole, M. (2020). Online university teaching during and after the COVID-19 crisis: refocusing teacher presence and learning activity. Postdigit. Sci. Educ. 2, 923–945. doi: 10.1007/s42438-020-00155-y
Renström, E. A., Gustafsson Sendén, M., and Lindqvist, A. (2021). Gender stereotypes in student evaluations of teaching. Front. Educ. 5, 571287. doi: 10.3389/feduc.2020.571287
Ryu, J., and Baylor, A. L. (2005). The psychometric structure of pedagogical agent persona. Technol. Instruct. Cogn. Learn. 2, 291.
Sinclair, L., and Kunda, Z. (2000). Motivated stereotyping of women: she's fine if she praised me but incompetent if she criticized me. Pers. Soc. Psychol. Bull. 26, 1329–1342. doi: 10.1177/0146167200263002
Smith, B. P. (2007). Student ratings of teaching effectiveness: an analysis of end-of-course faculty evaluations. Coll. Stud. J. 41, 788–800.
Spooren, P., Brockx, B., and Mortelmans, D. (2013). On the validity of student evaluation of teaching: the state of the art. Rev. Educ. Res. 83, 598–642. doi: 10.3102/0034654313496870
Subtirelu, N. (2015). “She does have an accent but…”: race and language ideology in students' evaluations of mathematics instructors on RateMyProfessors.com. Lang. Soc. 44, 35–62. doi: 10.1017/S0047404514000736
Turner, J. C., Meyer, D. K., Cox, K. E., Logan, C., DiCintio, M., and Thomas, C. T. (1998). Creating contexts for involvement in mathematics. J. Educ. Psychol. 90, 730–745. doi: 10.1037/0022-0663.90.4.730
Ullman, J. (2020). Present, yet not welcomed: gender diverse teachers' experiences of discrimination. Teach. Educ. 31, 67–83. doi: 10.1080/10476210.2019.1708315
Umbach, P. D. (2006). The contribution of faculty of color to undergraduate education. Res. Higher Educ. 47, 317–345. doi: 10.1007/s11162-005-9391-3
Wachtel, H. K. (1998). Student evaluation of college teaching effectiveness: a brief review. Assess. Eval. Higher Educ. 23, 191–212.
Witcher, A., and Onwuegbuzie, A. J. (1999). Characteristics of effective teachers: perceptions of preservice teachers. Res. Schl. 8, 45–57.
Zabaleta, F. (2007). The use and misuse of student evaluations of teaching. Teach. Higher Educ. 12, 55–76. doi: 10.1080/13562510601102131
Keywords: teaching perception, online teaching, unconscious biases, instructor evaluation, south asian instructors, higher education
Citation: Lamba N, Kishore S and Khokhlova O (2023) Examining racial and gender biases in teaching evaluations of instructors by students on an online platform in the UAE. Front. Educ. 8:1237672. doi: 10.3389/feduc.2023.1237672
Received: 09 June 2023; Accepted: 27 November 2023;
Published: 14 December 2023.
Edited by:
Jihea Maddamsetti, Old Dominion University, United StatesReviewed by:
Seema Rivera, Clarkson University, United StatesSebastian E. Wenz, GESIS Leibniz Institute for the Social Sciences, Germany
Kerstin Hoenig, German Institute for Adult Education (LG), Germany
Copyright © 2023 Lamba, Kishore and Khokhlova. This is an open-access article distributed under the terms of the Creative Commons Attribution License (CC BY). The use, distribution or reproduction in other forums is permitted, provided the original author(s) and the copyright owner(s) are credited and that the original publication in this journal is cited, in accordance with accepted academic practice. No use, distribution or reproduction is permitted which does not comply with these terms.
*Correspondence: Nishtha Lamba, N.Lamba@mdx.ac.ae
†These authors have contributed equally to this work and share first authorship