- 1Department of Journalism, Media, and Digital Communication, School of Arts, The University of Jordan, Amman, Jordan
- 2Department of Curriculum and Instruction, School of Educational Sciences, The University of Jordan, Amman, Jordan
- 3Department of Social Work, School of Arts, The University of Jordan, Amman, Jordan
- 4Department of Sociology, The University of Jordan, Amman, Jordan
The technology acceptance model (TAM) has garnered considerable attention within higher education in Jordan, particularly during the COVID-19 pandemic. However, we still need to understand how to progress the TAM to enhance educational sustainability. “Perceived enjoyment” (PE) is a significant predictor of perceived ease of use, intention to use technology, use e-learning systems for educational sustainability, and attitudes toward using the TAM. This study aims to reveal the role of sustainable digital communication using PE with the TAM within higher education in Jordan. An online survey was conducted using a random sample of students at the University of Jordan, which reached 576 participants. The results of a subsequent path analysis of the survey data revealed that PE, perceived usefulness, perceived ease of use, and attitudes toward using digital communication strategies were dominant in affecting student intention to use sustainable digital communication. We found that the students would like to use digital communication strategies due to their ease of use and because they can enhance self-education. However, students need to enhance their usage skills, and to better understand digital communication strategies. Moreover, we found that the TAM, PE, perceived ease of use (PEU), and perceived usefulness (PU) immediately and favorably affect attitudes toward usage (ATU) and behavioral intention (BI). PEU negatively affected the intention of students to use technology through ATU, while PE had a significant impact on the PU, PEU, ATU, and BI of using digital communication strategies. Information and communication technologies (ICT) developers in the Jordanian Higher Education environment should create systems that are user-friendly and pertinent to the sustainability of student education, to raise their perceived ease of use. Managers should also encourage students to use ICT.
Introduction
Within the context of the development of information and communication technologies (ICT) over the past 25 years, this paper investigates the continuing transformation and interconnectedness of ICT. This development has been supported in recent times now that sustainable digital communication is available in many contexts and industries. For instance, there is now much readily accessible data provided digitally within Higher Education (Català-Oltra et al., 2023). Many lecturers in higher education are also utilizing technology effectively to modify some or all of their existing course material. Online courses, also known as e-learning, are being offered by an increasing number of universities and other Higher Education organizations worldwide (Arya et al., 2012; Ramesh et al., 2022). According to Sumedrea et al. (2022), the quickening evolution of information technology along with several major challenges that have emerged in our time, the latest of which is the COVID-19 pandemic, have prompted a rise of buy-in to digitization. As a result, education institutions in general and Higher Education in particular have begun to pay great interest about how they present educational options online and through sustainable digital communication.
Our study considers sustainable digital communication in terms of perceived enjoyment (PE). According to Atombo et al. (2017), apart from performance enhancements due to the use of technology, PE is characterized as the level to which the activity of using a particular technology is perceived to be enjoyable. In addition, our study seeks to identify PE of the technology acceptance model (TAM). Charness and Boot (2016) explain that TAM refers to one among the most influential models of technology acceptance, which concentrate on the two essential factors influencing a person’s desire to use new technology: perceived usability and ease of use.
The sustainable digital communication with using PE with TAM in higher education in Jordan has not been studied sufficiently. Therefore, the aim of this research is to understand sustainable digital communication using PE. We also attempt to confirm the role of sustainable digital communication using PE with TAM in higher education. Although integration in PE predicts the use of digital systems in several studies (e.g., Khoa, 2020; Jasin, 2022), interrogating the role of sustainable digital communication using PE is controversial (Shiau and Luo, 2013). The TAM has garnered considerable attention within higher education in Jordan, particularly during the COVID-19 pandemic. However, there is value in understanding the further advancements of the TAM in the context of educational sustainability. We used PE as a significant predictor of perceived ease of use of technology, the intention to use technology, e-learning systems for educational sustainability, and attitudes toward using the TAM (Teo and Noyes, 2011; Almulla, 2021; Alkhwaldi et al., 2022; Rosli et al., 2022; Saleh et al., 2022; Al-Adwan et al., 2023). Due to the importance of function of digital communication within institutions of higher education, as a main tool for information flow and quantitative and cultural exchange. Our study will be of value to educational institutions and higher education in Jordan.
Literature review
Digital communication in higher education
Technological development in general and digital communication aim to meet the basic needs of people and society, and enhancing security and stability, which has become a means that the person resorts to as a communication process with himself or with others to know everything surrounded (Al-Sadiq, 2022). Therefore, people were forced to resort to modern media and various types of communications for information that touched all areas of life. This is because modern media, characterized by digital communication, is characterized by speed and accuracy (Larafi and Zawali, 2021). Asrani and Kar (2022) claim that digital communications, such as emails, phone conversations, online conferences, and various quick, direct messaging applications allow users to move data rapidly. Additionally, digital communication is essential for business because it enables users to communicate discreetly with their clients, who react immediately. Digital communication has many benefits for companies, including timesaving and being highly accessible, affordable, and rapid (Rama et al., 2023). Additionally, communication technologies offer customers individualized service and support. The concept of digital communication refers to those social processes through which a clear and understandable definition of communication takes place between parties if all parties contribute to the development of the digital communication message. This is achieved by taking advantage of the approved digital systems for digital transmission and reception, to achieve various goals and objectives (Stone and Wang, 2019). Lee et al. (2022) described digital communication as a process during which communication takes place at a distance between two or more parties such that they exchange information, and this information is encoded and processed through digital systems, after which transmission and reception occurs using digital communication devices.
Simply put, digital communication refers to an organization’s online communication initiatives. Today, most businesses interact digitally with their current and potential clients, staff members, and other stakeholders via their websites, mobile chat applications, and blogs (Doyle, 2019). The opportunities for communicating a message today are considerably greater and speedier than they were a few decades ago, according to Edward Powers, a lecturer at Northeastern’s Master of Science in Corporate and Organizational Communication, and Bachelor of Science in Digital Communication and Media programs. Experts in digital communication should exercise caution when implementing these new technologies (Doyle, 2019). According to Postelnyak (2023), the effectiveness of a digital communication plan will depend on the digital technologies employed and how they serve the objectives of internal communication. For instance, a quickly moving business that has employees spread across a continent will require reliable, asynchronous communication channels, such as Employee SMS. On the other hand, it will be necessary to improve internal video communications if the top objective is to build a strong business culture and improve team-wide collaboration. Some businesses will wish to mainstream asynchronous cooperation while maintaining culture as the focus. In this situation, adopting the appropriate set of digital communication tools and channels will be required (Postelnyak, 2023). Ratna (2023) indicated that digital communication systems have many basic components, as shown in Figure 1.
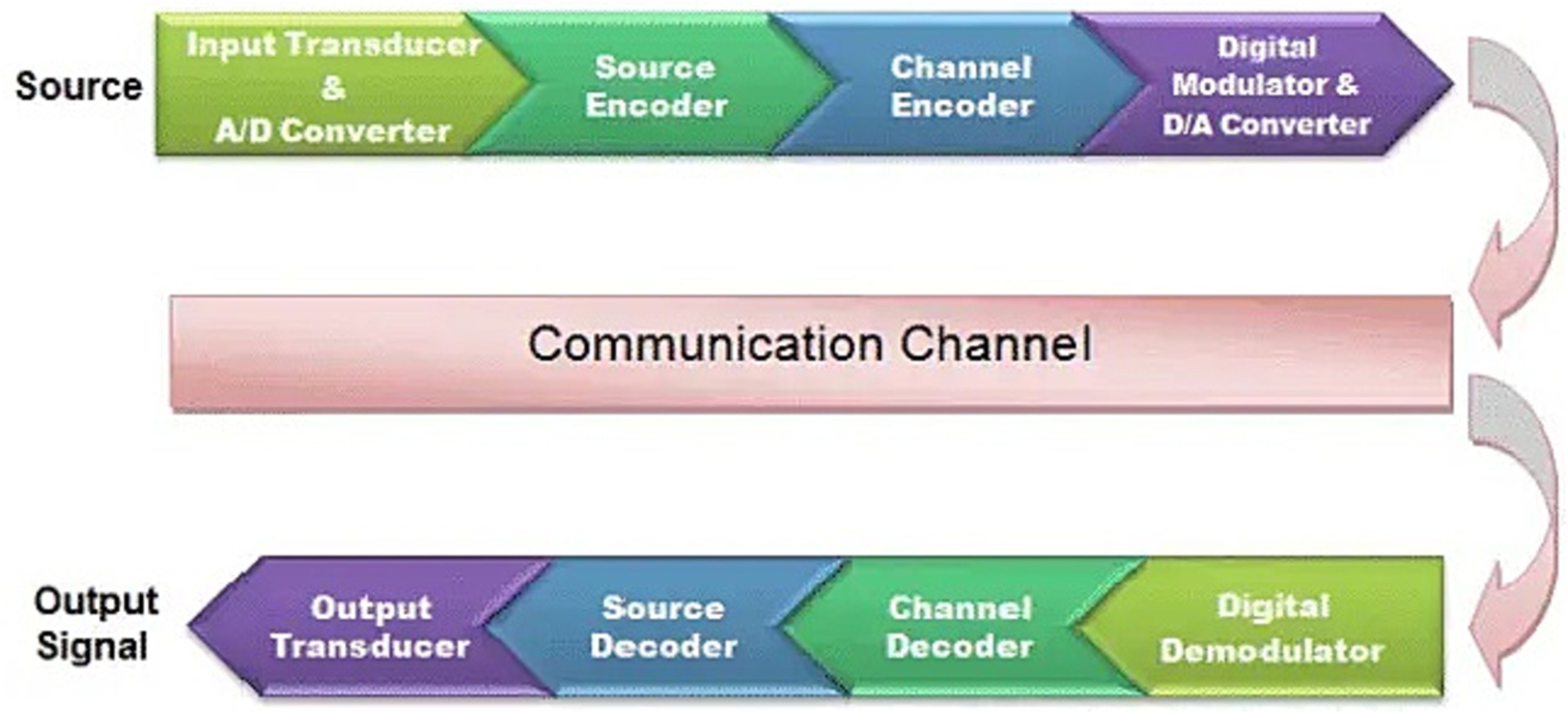
Figure 1. Main digital communication system elements. (a) Source: an analog signal serves as the source, such as a sound signal. (b) input transducer: this component transforms a physical input into an electrical signal, such as occurs in a microphone. (c) Analog to digital converter: used to further process and convert the electrical signal from the input transducer into a digital signal. (d) Source encoder: reduces the number of bits in the data to a minimum. The bandwidth operates more effectively because of this procedure. (e) Channel encoder: coding is done in the channel encoder for error correction. The signal may be corrupted while being transmitted due to the sound in the channel. To avoid this, the channel encoder adds additional bits to the transmitted data, known as error-correcting bits. (f) Digital modulator: a carrier modulates the signal that will be broadcast. The carrier is utilized for efficient long-distance data transmission. (g) Digital to analog converter: after being separated from the carrier, the digital signal is converted back into an analog signal so that it can be transmitted across the channel or medium efficiently. (h) Channel: gives the signal a path and enables analog signals to be transmitted from transmitter ends to receptor ends. (i) Digital demodulator: this is where the data retrieval procedure starts at the receiver end. Before being converted to digital form, the received signal is first demodulated once more. The signal is rebuilt in the digital demodulator. (j) Output signal: the entire process is completed for this product. The audio signal can be heard.
There is no doubt about the importance of digital communication today because it permeates all aspects of our lives – personal, professional, and educational. Digital communication is a method of electronically transmitting information between sources, but why is it significant, and why is digitization required for communication? Grami (2015) explained that for long-distance communications, analog signals were used in conventional communication technologies. Analog transmission incurs numerous losses over long distances, including distortion, interference, and even security breaches. The signals are now digitized using various methods to reduce and overcome these kinds of losses. Digital transmissions are clearer and more accurate with little to no loss (Grami, 2015).
Higher education is the summit of an educational system. There are many names for educational institutions that are affiliated with higher education, such as universities, high schools, academies, institutes, and colleges. Higher education is distinguished by the diversity of its courses and the large number of specializations available. Upon completion of higher education study, a certificate is given qualifying the student to enter the labor market (Chernikova et al., 2020). Educational processes depend on technology partially or completely, and focus on both reducing costs and raising the quality of education, aiming to develop educational processes and the ease of access to them. Higher education realizes the importance of the process of transitioning to digital communication (Protopsaltis and Baum, 2019). Rapid digital development requires educational institutions to reinvent themselves and transform their operations. Modifying institutional culture is among educational institutions priorities as well as the focus on direct needs of students and future market requirements (Saldanha, 2019).
The transformation of digital communication affects all sectors of society, especially the education sector, as new digital technologies such as social networking, mobile, big data, the internet of things, and other breakthroughs, for example blockchain, give the education industry the possibility to drastically modify its business model. Such modifications usually include adjustments to organizational structures as well as fundamental educational processes (Ziyadin et al., 2019). Higher education can move toward embodying sustainable digital communication over several stages, the most important of which are digitizing the library and automating the library reference service desk using chat-bots, linking data to operations, monitoring the student life cycle from admission to graduation, and digitizing payments by providing the basic requirements for sustainable digital communication (Tomej et al., 2022). Nelwamondo and Njenga (2021) argued that the most important requirement for digital communication is cloud computing, which enables higher education to have faster access to its programs, new functions, and updates, as well as data storage, from anywhere and at any time. Because cloud computing enables higher education computing services, students can access cloud data and applications over the internet through remote physical servers, databases, and computers. Commodity information technology enables the higher education sector to direct investment funds and human resources toward information technology and digital communication (Attaran, 2020). Mobile platforms and communication technologies enable communication from anywhere, anytime, including third, fourth, and fifth generation services (El-Sofany and El-Haggar, 2020). Applied machine learning and artificial intelligence (AI) machine learning are subsets of AI that concentrate on building systems which learn from the data they parse to subsequently perform better. Systems or gadgets that mimic human intelligence are included together under the term AI. AI and machine learning are crucial tools and programs for automating complicated tasks, such as safeguarding systems and data, and they give higher education insights enabling it to make strategic decisions more quickly and accurately (Pedro et al., 2020). Digital communication technology has greatly advantaged the process of education. Both the teacher and the student use digital technology to search for information, including when there are few sources and references that talk about a particular topic (Al-Sadiq, 2022). Digital communication has also contributed to the emergence of many features, as higher education has become distinguished through its use e-learning, which has many advantageous features such as those listed below (Al-Mahdi and Maamari, 2019), thus educating many students without restriction: (i) educating many students in a short period, (ii) instant and fast education, (iii) encouraging self-learning, (iv) multiple sources of knowledge, (v) ease of updating informational content, (vi) saving financial costs.
The way that higher education is delivered today has been changed by digital communication. Digital communication is a way for teachers and students to exchange information electronically. Students’ capacity for self-learning is enhanced through digital communication. Remote locations do not hinder communication between teachers and students, while instructors and parents can communicate about a student’s development (Ayman et al., 2020). Using digital communication tools with a (TAM) Model could influence higher educational process sustainability, especially in developing countries (Ayman et al., 2020; Almulla, 2021; Alismaiel et al., 2022; Rosli et al., 2022; Sumedrea et al., 2022). The interest in e-learning at the University of Jordan dates to 1998, through individual initiatives of some faculty members who were using electronic platforms as supportive tools in education, rather than organized and coordinated e-learning, in cooperation with the King Abdullah II School of Information Technology at the University of Jordan. The blended learning initiative started in 2017 as an option among faculty members, exception of the compulsory university requirements, all of which are taught using the blended system. In 2016, the University of Jordan officially approved the blended learning system, and it began to be applied at the beginning of 2017 in university requirements, especially courses that are taught in a theoretical, indoctrination manner that does not encourage discussion. After the establishment of the Center for Open Educational Resources and Blended Learning at the University of Jordan in 2018, the system expanded to 118 courses out of 2,669 courses, or 4%, in 16 different colleges, in the first semester of the academic year 2018/2019. With the issuance of the foundations of blended learning in 2019, the number of courses taught using the blended system increased. In 2021, distance learning and blended learning were officially integrated into some elective and compulsory courses in Jordanian public and private universities (Zaida, 2019; Almamlaka, 2021). During the COVID-19 pandemic, The use of students and teachers in Jordanian universities increased their dependence on digital means of communication due to the success and effectiveness of some of them, such as Microsoft Teams, social media and e-learning, Jordanian universities have begun to adopt some of them as essential means of education and to rely on blended learning (Al-Adwan and Albelbisi, 2020; Amarin, 2022; Alnemrat et al., 2023; Madanat et al., 2023). The Ministry of Education has launched a national e-learning plan through knowledge networks across the country in partnership with the Ministries of Planning and Information and Communication Technology (Alnemrat et al., 2023).
Technology acceptance model (TAM)
The theories of reasoned action and planned behavior gave rise to the TAM. According to Marikyan and Papagiannidis (2023) the lack of empirical insight into users’ responses to the information system, performance was a main stumbling block on the acceptance of personal computing. Therefore, taking care of the importance of the users’ involvement in the design, implementation and development of information systems based on subjective performance perception scales. Due to the weakness of the internal and external validity of these scales, the theory of reasoned action (TRA) was used, which was developed by Ajzen and Fishbein (1980), this theory maintains that volition and intention predict behavior. If Individuals evaluate the suggested behavior as positive and if they think others want them to perform accordingly, this would lead to a higher intent and they are more likely to perform the behavior (Mimiaga et al., 2009). But this theory had several application limitations, to address these limitations, Technology Acceptance Model (TAM) was first proposed by Davis (1989) – in his Doctoral thesis-to predict the use and acceptance of information systems and technology by individual users (Surendran, 2012), and by giving a theoretically supported, universal explanation of the factors that influence computer adoption which can be used to explain user behavior across a wide range of end-user computing technologies and user populations (Burgess and Worthington, 2023). The TAM model confirmed that the behavioral intention in the context of technology utilization is shaped by specific beliefs related to technology use (Marikyan and Papagiannidis, 2023). The TAM maintains that an individual’s intentions to utilize a technology directly influence how that technology is used. The more someone intends to utilize a part of technology, the more likely it is that they will do so. The TAM goes on to state that these intentions are predicted by two factors: (1) a person’s perceived usefulness (PU) for the technology and (2) a person’s perceived ease of use of the technology (PEU). Since, the TAMapplication has been broadened to incorporate several technologies aside from computers, such as the application of telemedicine services (Kamal et al., 2020), digital tools for teachers (Scherer et al., 2019), mobile apps (Min et al., 2019), and student e-learning platforms (Sukendro et al., 2020).
According to the TAM model, emerging technology adoption is determined by four factors: perceived ease of usage (PEOU), perceived utility (PU), attitude toward use (ATU), and behavioral purpose to use (BIU). Perceived usefulness (PU) and Perceived Ease of Use (PEU) are the two cognitive ideas that provide credence to the claim that individual behavioral intentions (BIs) to utilize a system constitute system utilization. A user’s behavioral goals, attitudes, perceptions of the system’s utility, and perceived ease of use of the system are all factors that affect how a technological system is used (Samuel et al., 2018). PEU explains how much a person expects using an information technology system to be effortless (Winarno et al., 2021). Similar to TAM, digital systems or apps that are used more regularly suggest that the system is more comfortable and simpler to use. It is a widely utilized type of digital system, making the program simple to use and beneficial for its consumers (Winarno et al., 2021). In general, the PEU describes the extent that a person thinks a product or modern technology is simple to use and understand. A user is more inclined to continue using a product or new technology if they think it is simple to utilize (Huang, 2021).
However, PU is one of TAMs independent constructs. According to Alsabawy et al. (2016), PU refers to “the degree to which an individual believes that using a particular system would improve his or her job performance.” PU refers to a person’s perception of how beneficial they believe using a particular technology to be. The likelihood of someone using a certain technology rises in direct proportion to how valuable they believe it to be (Burgess and Worthington, 2023).
When anticipating intentions, PU and PEU do not necessarily contribute equally. Sometimes, PEU may have little or no impact on a person’s intents, which may be mostly decided by PU. Sometimes PEU might have a bigger impact. For instance, college students’ PU to use digital education may be significantly influenced by their views that it is valuable; whether they think it to be perceived to use may not have a significant impact on their intentions (Elkaseh et al., 2016). This knowledge is crucial because it can aid those who seek, through creative messaging, to inspire recipients to engage in a particular activity. Measurement of these variables among a group of study participants, along with statistical analysis, are the only ways to ascertain the relative weighting (or importance) of PU and PEU on people’s intentions (Burgess and Worthington, 2023).
With the development of technology and the widening of its fields of application, several changes have been made to TAM model. Taylor and Todd (1995) proposed the combined TAM-TPB model to integrate the Technology acceptance model and theory of planned behavior, which explain that the behavior is influenced by behavioral intention, which, in turn, is influenced by attitude, subjective norm, perceived behavioral control, and perceived usefulness (Surendran, 2012; Al-Mamary et al., 2016). Venkatesh and Davis (2000) proposed a TAM2 model extend TAM based on the idea that the output quality increases the likelihood of a positive perception of technology (Marikyan and Papagiannidis, 2023). TAM2 removed the ATT component from the TAM model and added a theoretical construct involving social influence processes (subjective norm, voluntariness, and image) which help to determine if an individual will adopt or reject a new system. And cognitive instrumental processes of perceived usefulness, which refer to perceived ease of use, output, output quality, and job relevance (Sullivan, 2016). Venkatesh et al. (2003) developed the Unified Theory of Acceptance and Use of Technology (UTAUT) Model to unifying major theories about information technology acceptance and explain the variances in use behavior and IT behavioral intention (Batucan et al., 2022). While Venkatesh and Bala (2008) proposed the TAM3 model that incorporates TAM2 and the model of the perceived ease of use, which include three determinants of customer acceptance of technology, 1) perceived ease of use (PEOU) to measure a user’s effort in using a particular technology, 2) perceived usefulness (PU) to measure the value that the user of this technology will gain, 3) subjective norm (SN) which is “the degree to which an individual perceives that most people who are important to him think he should or should not use the system” (Saravanos et al., 2022).
In a literature review study, Ajibade (2018) explores a range of criticisms of the original TAM model, which include the lack of subjective norms or social influence, it was not designed or modeled for use in evaluating learning in electronic platforms or e-learning systems. Moreover, TAM could not sufficiently predict the acceptance of information communication technology (ICT) and is not able to provide comprehensive precursors to mobile use, or conditions that facilitate behavior. However, the TAM model was more appropriate for individual use and acceptance of technology, easy to comprehend, and demonstrated a high level of predictiveness, and it is a useful framework for explaining course management system usage and satisfaction with the internet as an educational delivery medium, (Arbaugh, 2010). Lai (2017) confirmed that the “TAM model has proved to be a valid and reliable model explaining information system acceptance and use, and can be selected for the novel technology of single platform E-payment, in light that the social norms scales had a poor psychometric standpoint, and might not exert any influence on consumers’ behavior intention, especially when information system application like single platform E-payment System was fairly personal while individual usage was voluntary.” Moreover, TAM2, TAM3 and UTAUT were not favorable to study the novelty technology of single platform E-payment System and did not include direct relations studies. The personal innovativeness, social factors, self-efficacy, computer anxiety, subjective norms, enjoyment, and experience consider as the most used external factors for TAM, and improve students’ learning (Abdullah and Ward, 2016; Chahal and Rani, 2022; Zhou et al., 2022).
Hypotheses development
Many relevant studies have been conducted, for example by Igbaria et al. (1994), who investigated how PU and PE affected the acceptance of microcomputer technology. Igbaria et al. (1994) investigated whether users were driven to adopt a new technology because of its utility or enjoyment. Their results suggested that PU has a greater impact than PE on whether users accept microcomputer technology (Igbaria et al., 1994). Won et al. (2023) conducted a study that extended the TAM and the information system success model within the context of branded sports applications. The study looked at the effects of TAM dimensions and app system success characteristics on the desire of users to use branded sport applications. The research showed that, among the TAM variables, PE had the greatest impact on users’ usage intention, followed by PU and PEU (Won et al., 2023).
Recently, e-learning has become one of the most significant instructional technologies in institutions of higher education, due to the growth of internet technology. At the same time, student acceptance of e-learning systems is a key factor in determining student success (Salloum, 2018). However, in higher education, applying the TAM to examine the adoption of e-learning is not an entirely new subject of study, with various academics having attempted to address it. Additionally, Abdullah and Ward (2016) carried out research to determine the most frequently applied external TAM factors within the field of e-learning adoption and found that “self-efficacy, subjective norm (SN), enjoyment, computer anxiety and experience” are the greatest generally utilized and external TAM factors. The effects of these often-used external elements on the PEU and PU, the two main TAM constructs, have been studied across a range of e-learning technologies. The results show that self-efficacy is the best predictor of students’ PEU of e-learning systems, followed by enjoyment, experience, computer anxiety, and SN. The top four factors that determine a student’s PU in e-learning systems are, in order, enjoyment, SN, self-efficacy, and experience. The paper recommends a generic extended TAM for e-learning that makes use of these outside factors and their effects on PEU and PU (Abdullah and Ward, 2016). Al-Rahmi et al. (2020) examined the use of ICT by students and how satisfied the students were with ICT, with the TAM used as the study’s research domain. The results indicated that (PE) (including PEU and PU), SN, and computer self-efficacy were correlated. Student intentions to use ICT (SIU) and student satisfaction (SS) were influenced by PEU, PU, and position toward computer use (ACU) (Al-Rahmi et al., 2020). Sayaf et al. (2021) found that ICT has a tremendous effect on educational activities (And there is a relationship between PE, computer anxiety, and self-efficacy (SE) – factors that were all influential in determining PU and PEU). Students’ continuous intention to use and satisfaction were also influenced by PU and PEU (Sayaf et al., 2021).
Digital education technologies have emerged because of ICT revolutionizing education methods. Krouska et al. (2022) identified the external TAM components most frequently used in online learning. Regardless of the digital education technology studied, SE, PE, and system quality are the three main elements that determine user attitude, followed by SN, system accessibility, and enabling factors. However, Bagdi and Bulsara (2023) conducted a study using an expanded technology acceptance paradigm to examine how students feel about participating in digital learning while attending higher education institutions. To address each student’s unique needs outside of the traditional classroom, the study examined the variables that affected students’ choices to use digital education. The study’s findings support the notion that PE has a meaningful association with BI in utilizing digital learning, while SE and system accessibility (ACC) had only a negligible effect (Bagdi and Bulsara, 2023).
Moreover, Teo and Zhou (2014) identified the elements that affect HE students’ intentions to use technology. Utilizing an extended TAM as a study structure, their findings showed that PEU negatively impacted the intention to use technology through ATU, while PU and ATU were major predictors of that intention. In general, the focus of studies has historically been on how much teachers use technology in their instruction. There are many theories explaining the mechanics and influencing aspects of technology use in classrooms, while the TAM and its variations have dominated the subject. Scherer et al. (2019) showed that, generally, the TAM explains technological acceptance effectively, although the importance of external variables and the role of some key elements run counter to some preconceived notions about the TAM (Scherer et al., 2019). By combining a TAM with PE to investigate higher education students’ attitudes toward adopting sustainable digital communication, hypotheses were developed according to previous research reviews.
PE and PU are important variables that may either directly or indirectly affect intentions. According to Igbaria et al. (1994), PU has a greater impact than PE when users are deciding whether to accept or reject technology. Moreover, PE significantly affects PEU and PU (e.g., Abdullah and Ward, 2016; Bagdi and Bulsara (2023); and Cabada et al., 2017). Abdullah and Ward (2016) examined several studies of students at universities utilizing e-learning platforms and found that over 70% of those students revealed a significant positive relationship between PE and PEU regarding e-learning. Strong evidence from previous studies linking PE with PU and PEU in HE students’ utilization of digital communication implies that students are more apt to utilize digital communication when it is pleasurable and appealing.
PEU and PU have a direct and considerable impact on ATU, according to previous research that utilized subjects with various learners (Abdullah and Ward, 2016; Lopez and López-Bonilla, 2016), and showed that the impact of PEU on BI was mediated by PU (Venkatesh et al., 2003; Moreno et al., 2016). PU and PEU have significant effects on university students’ intentions to use e-learning as well as learners’ opinions about the adoption of learning systems as tools (Liu and Huang, 2015; Ifinedo et al., 2017). Additionally, ATU is thought to have a favorable and significant influence on students’ intentions to use technology (Liu and Huang, 2015; Abdullah and Ward, 2016). Students will exhibit a favorable attitude and even greater desire to embrace technology if they perceive it to be useful. Given this, we tested the following hypotheses (see Figure 2):
H1: PE has a significant effect on the PU of the use of digital communication tools.
H2: PE has a significant effect on the PEOU of digital communication tools.
H3: PEOU has a significant effect on the PE of the use of digital communication tools.
H4: PE has a significant effect on the ATU of digital communication tools.
H5: PU has a significant effect on the ATU of digital communication tools.
H6: PEOU has a significant effect on the ATU of digital communication tools.
H7: PE has a significant effect on the BI to use digital communication tools.
H8: PU has a significant effect on the BI to use digital communication tools.
H9: PEOU has a significant effect on the BI to use digital communication tool.
Methodology
Participants and data collection
Data were collected through an online survey distributed to 3,005 students at the University of Jordan, aged 18–24 years. One thousand and fifty-seven students consented to participate in this study. These students used digital communication tools in study, such as email, text message, apps, social media “WhatsApp, Facebook,” Skype, Google Classroom, emails, e-learning, Zoom, Google Meet, ClassDojo, ClassTag, Microsoft Teams, and Google Drive. A convenience sample of 576 undergraduate students (340 female, 236 male) was selected after removing missing data. The survey was conducted through an online anonymous survey platform via Google Forms, between Jan and April 2023, during the 2022–2023 academic year. The data were collected anonymously. Students who wished to respond were invited via social media sites, such as Facebook, WhatsApp, and Microsoft Teams to access the survey through an anonymous link. There are several pre-existing groups on Facebook that allow students to exchange survey links. Each participant was advised that their participation was voluntary and there would be no consequences if they chose to withdraw from the survey. All participants provided informed consent when they agreed to fill out the questionnaire. Participants who consented to participate in the survey clicked “yes” on the first question in the survey, which was “Do you consent to participate in this study?” Once students had selected this option, they were directed to complete the self-administered questionnaire, which typically took 15 min to complete. Students known to the researchers were assured anonymity and were informed that the data would be treated in strict confidence. We took into consideration the Pew Research Center’s (2021) instructions and recommendations for electronic surveys. Ethical approval for this study was granted by the Scientific Committee of the Department of Sociology at the University of Jordan.
Measurement
The intention of students at the University of Jordan regarding their use of digital communication tools was ascertained by questionnaire was designed based on Al-Rahmi et al. (2019), Buabeng-Andoh (2018), and Davis (1989) measures. The final questionnaire included two sections: 1) Demographic data of student (sex, age, type of digital communication tool used). 2) Questionnaire consisting of 23 items measuring Perceived Usefulness (PU) (5 Items), Perceived Ease of Use (PEU) (4 items), Perceived Enjoyment (PE) (6 items), Attitude Toward Use (ATU) (4 items), and Behavioral intention (BI) (4 items) (Appendix 1). The items were rated on a five-point Likert-type scale: 1 = Strongly disagree, 2 = Disagree, 3 = Neutral, 4 = Agree, and 5 = Strongly agree. Five-point scales were used in earlier research, and it was shown that their dependability was higher than that of other measures (Cornalba and Giudici, 2004). As given in Table 1, the values of the arithmetic means were interpreted as follows with regards to the quintuple gradation: 3.67 and above was high, 2.34–3.67 was medium, and 2.33 and below was low, in accordance with the following equation
Data analysis
To perform the statistical analysis, we used IBM SPSS-22. We calculated Cronbach’s alpha coefficient to assess the reliability of our questionnaire, using composite reliability. Frequency distributions and descriptive statistics were calculated. To test the hypotheses, we used Smart-PLS and two models were examined: a measurement model to check the validity and reliability of the construct using PLS-Algorithm, and a structural model to test the hypotheses using a bootstrapping technique. All analyzes were two-tailed with a 0.05 threshold for statistical significance. Also, the standardized root means square residual (SRMR) and the normed fit index (NFI) were calculated, as two widely used parameters for evaluating a model’s fit.
Results and data analysis
As shown in Table 2, the mean values for items that measure PE ranged between 2.99 and 3.13. This indicates that using digital communication strategies would keep the students happy but would not be a pleasant experience for a large percentage of them. The mean values for PEOU items ranged from 3.28 to 3.37, with a medium level of agreement for all items, indicating that using digital communication strategies would be easy for the students but for a large percentage of students not clear and understandable. The total mean for the PU variable was 3.26 and the mean values for the items ranged from 3.16 to 3.32, indicating that the students believed using digital communication tools would enhance self-education. For their attitude toward using (ATU) digital communication strategies, the mean values ranged from 3.14 to 3.36 and all items mediumly agree by the respondents. This indicates that the students would like to use digital communication strategies and that doing so would be a pleasure for them. The mean values for the items that measured BI to use different digital communication tools ranged from 3.10 to 3.32 and the overall mean value was 3.24.
Starting with the measurement model (outer model), the PLS-SEM model was examined in terms of the validity and reliability of the latent variables. We began by examining the factor loading, which measured the association between each item and its accompanying construct. Any items with a factor loading of less than 0.4 were deleted, and items with a factor loading of between 0.4 and 0.7 were retained only if they were of significant value (Hair et al., 2021). From Table 3, all factor loading values were more than 0.7 except PE1, PE2, and ATU3 which had factor loadings of more than 0.4.
Utilizing Cronbach’s alpha and composite reliability (CR), the internal consistency reliability was evaluated. The values for these metrics fall between 0 and 1, and should be greater than 0.7 (Sarstedt et al., 2017). In Table 3, every Cronbach’s alpha and CR value was greater than (0.7). Convergent validity, or the degree to which the indicators positively correlate to measure the same idea [average variance extracted (AVE)], is assessed using the AVE. The AVE value must be greater than 0.5 and ranges from 0 to 1 (Sarstedt et al., 2017). Also, in Table 3, the AVE values were greater than 0.5 for every construct. The evaluation findings for the measurement model are also shown in Figure 3.
Discriminant validity was calculated to ensure the uniqueness of constructs compared to other latent constructs. Two methods were adopted to check this, the first being the Fornell-Larcker criterion (Table 4). The square root of the AVE for one construct should be more than the correlation with the other constructs. Table 4 shows that AVE2 for one construct is higher than all the correlations between it and the other constructs. For example, the AVE2 for ATU was 0.844, which is more than its correlation with the other constructs (0.755, 0.750, 0.677, and 0.756). Thus, there was no problem with discriminant validity. The second method was Heterotrait-Monotrait (HTMT). The HTMT results had respectable ranges of more than 0.90 (Kline, 2023), satisfying the criteria for the investigation of the discriminant validity of HTMT and the validity of the research constructs (See Table 5).
The coefficients of determination (R2 values) of the endogenous variables were 0.925, 0.935, 0.723, and 0.966, indicating that the independent variables explained these proportions of the variance. The effect size (ƒ2) is the change in the R2 value when one indicator is eliminated from the model. f2 values less than 0.02 indicate no effect and values between 0.02 and 0.15 indicate a small effect, such as shown in PEOU- > ATU, PE- > ATU, and PU- > ATU. The f2 values more than 0.15 indicate a large effect, such as shown in ATU- > BI, PE- > BI, PE- > PEOU, PEOU- > PU, and PE- > PU (Hair et al., 2017). Finally, predictive relevance (Q2) was obtained using the blindfolding procedure. The Q2 value for all endogenous variables is above 0, which indicates that the independent variables have predictive relevance of some quality for dependent variables (See Table 6 and Table 7).
The completion of the evaluation of the relationships in the structural model was enabled by the satisfying findings for the measurement model analysis in terms of validity and reliability. The bootstrapping procedure was run with 5,000 subsamples and a significant level was set at 0.05 for a two-tailed test. All hypotheses were supported, as shown in Table 8 by assessment of the path coefficient (β), t-value, and value of p. PE had a significant positive impact on ATU (β = 0.875, t = 60.901; p < 0.05), BI (β = 0.907, t = 80.781; p < 0.05), PEOU (β = 0.850, t = 45.007; p < 0.05), and PU (β = 0.891, t = 59.068; p < 0.05), and also on ATU (β = 0.698, t = 19.730; p < 0.05), PEOU (β = 0.523, t = 15.689; p < 0.05), PE(β = 0.907, t = 80.781; p < 0.05), and PU (β = 0.306, t = 3.135; p < 0.05). Therefore, the results of the path analysis showed that PE, PU, perceived ease of use, and ATU were dominant in affecting student intention toward using digital communication strategies.
The final step was testing the model fit. The SRMR and the NFI are two widely used parameters for evaluating a model’s fit. A value of less than 0.08 is regarded as a satisfactory fit for the SRMR, which measures the discrepancy between the observed correlation and the model implied correlation matrix (Hair et al., 2017). In this study’s model, the SRMR was 0.073 (0.08). The second fit index, known as NFI, is an incremental fit measure that computes the proposed model’s value of chi-square and compares it to relevant standards. NFI values near 1 imply improved fit, and in this model it was 0.716 indicating that the data fit the model well (See Table 9). Figure 4 presents the results of the structural model.
Discussion
The current study is among the first adopters of the TAM to investigate the intention of higher education students in Jordan to use sustainable digital communications, using PE. The results showed that the mean values for the items that measured PE ranged between 2.99 and 3.13, where using digital communication strategies would keep the student happy and joyless in a large percentage. In addition, the mean values for PEOU items ranged from 3.28 to 3.37 with a medium level of agreement – using digital communication strategies would be easy for the students but not clear and understandable to a large proportion of them. The total mean for the PU variable was 3.26 and the range of the item means was 3.16 to 3.32, indicating that the students believed using digital communication tools would enhance self-education. For their ATU, the mean values ranged from 3.14 to 3.36 and all item mediums agreed by the respondents, which means that the respondents would like to use the digital communication strategies and it would be a pleasure for them to do so.
The outcomes of the final model showed that PE, PEOU, PU, ATU, and BI significantly predicted the attitude of higher education students in Jordan to sustainable digital communication. The results supported all suggested theories. The study results afford a deeper understanding of the application of sustainable digital communication, as well as PE, PU, PEU, and the TAM model, among higher education students in Jordan. According to the results, several variables, including PE, PEU, PU, and usefulness, had favorable and noticeable connections among University of Jordan students (ATU and BI) with sustainable digital communication use. Previous studies support the current study results about the significant enhancements of PE, PEU, PU, ATU, and BI with sustainable digital communication. Thus, it follows that before choosing to use digital communication, students should consider its applicability to the sustainability of their education and its capacity to meet their academic needs. When students realize that digital communication may fulfill these needs, they are more inclined to think that the technology is beneficial (e.g., Igbaria et al., 1994; Abdullah and Ward, 2016; Cabada et al., 2017; Bagdi and Bulsara, 2023).
Undoubtedly, students are already accustomed to digitization, and digital communications are widely employed in higher education sustainability. Our results showed that PE significantly positively impacted on ATU, BI, PEOU, and PU. As shown by the findings in Table 6, all assumptions were supported. (e.g., Liu and Huang, 2015; Abdullah and Ward, 2016; Ifinedo et al., 2017). Some of the results of assumptions were in contradiction to those previously reported, such as the study of Teo and Zhou (2014), which reported that PEU negatively affected intention to use technology through ATU. While Almulla (2021) found that the undergraduate student’s intentions to use an e-learning system for educational sustainability were influenced by PEU, PU, and attitudes toward use. These results show that more studies in this field are required to identify how the variables are inter-related. In summary, the findings of path analysis showed that PE, PU, PEU, ATU were dominant in affecting student intention toward using digital communication strategies. Salloum et al. (2019) tried to pinpoint TAMs most popular external elements that affected the acceptability of e-learning. They concluded that the effectiveness of the system, computer self-efficacy, have an important impact on the PEU of an e-learning system. Moreover, information quality, PE, and accessibility had positive effects on the PEU and PU of e-learning systems (Salloum et al., 2019). While Murari and Rai (2022) suggest that perceived enjoyment and system quality have a significant impact on the perceived usefulness and perceived ease of use of online learning system. On the other hand, computer self-efficacy, computer playfulness among individual characteristics; and content quality & information quality characteristics of online learning system do not significantly affect the perceived use and perceived ease of use of online learning system, and the content quality does not affect the attitude and intentions of using an online learning system (Tables 7–9).
According to the TAM, PE, PEU, and PU immediately and favorably affect ATU and BI. This was demonstrated in the current study by the fact that participants believed that greater digital communication use, which in turn increased ATU and BI, was influenced by higher PU and PEU. The research of Venkatesh and Davis (2000) validated the findings of this study of a positive and immediate connection between PEU and PU. Another study (Al-Rahmi et al., 2020) confirmed that students perceived ICT as easy to use if it helped them with their education. If students get the chance to utilize ICT, they are more likely to perceive it as simple to use. As a result, ICT developers should create systems that are user-friendly and pertinent to the sustainability of student education so that their ease of use is increased. Managers should also encourage students to use ICT.
The current study has returned several results as follows: a) PE has a significant impact on the PU, PEU, ATU, and BI of the use of digital communication strategies, b) PEU has a significant impact on the PU, ATU, and BI of the use of digital communication strategies, c) PU has a significant impact on the ATU and BI of the use of digital communication strategies. Al-Rahmi et al. (2020) found that the constructs of TAM model succeeded in explaining usage intentions towards ICT among university students and their satisfaction from this usage. Where significant relationship was found between computer self-efficacy (CSE), subjective norms (SN), and perceived enjoyment (PE), which were significant determinants of perceived ease of use (PEU) and perceived usefulness (PU). Furthermore, PEU, PU, and attitudes towards computer use (ACU) influenced students’ intentions to use (SIU) ICT and students’ satisfaction (SS). The results also significantly advance the TAM in the field of higher education sustainability (e.g., Abdullah and Ward, 2016; Salloum et al., 2019; Al-Rahmi et al., 2020). The results of Murari and Rai (2022) study revealed that perceptions of ease of use and usefulness had a substantial impact on undergraduate and post-graduate student’s attitudes toward utilizing online learning (ATU) and behavioral intentions (BI) of acceptance of it. Additionally, the best predictor of ATU and BI was PU. The growing use of digital communication as a teaching medium, teaching and learning processes are changing and undoubtedly will continue to change (Babu and Ma, 2018). Additionally, the modern world offers a variety of digital communication tools that can be utilized to produce and share knowledge (Agrawal and Mittal, 2018). Utilizing available digital communication is helpful for offering global connectedness and enhancing competition, resolving questions from teachers and students, and supporting learning about current events (Dave, 2019).
The study’s overall contributions are: a) The inclusion of digital communication in learning strategies should increase student use of ATU and BI. Additionally, encouragement from instructors and mentors can encourage students to embrace digital communication because it removes confusion, imparts knowledge, and offers information to enhance learning, performance, and research abilities and b) institutions of higher education are encouraged to accept students who are accustomed to using digital communication for studying, because educational institutions must include digital communication elements and tools in all aspects of the learning process. Future research should examine the impact of perceived enjoyment on teachers’ intention to use digital Communication, and to reappraising the motivations and role of attitude toward use in the Technology Acceptance Model among undergraduate students in Jordan.
Conclusion
Today, digitalization and ICT play a significant role for students in higher education and schools in improving their research and learning. Even so, no study has previously assessed students’ intentions to use digital communication and their attitudes toward its use. This study was effective in understanding Sustainable Digital Communication using PE. The study confirms the role of Sustainable Digital Communication using PE with the TAM within higher education in Jordan. Our research found that the TAM is sufficient to produce results according to the study subject, i.e., “a sustainable digital communication using PE with the TAM within higher education in Jordan.” The significant contribution of the present study is in highlighting the prominent role of communication within higher education institutions because communication is the main tool for information flow and quantitative and cultural exchange. Furthermore, given the lack of research on the current topic, our study will be useful for educational institutions and higher education in Jordan. Future research could conduct further investigations into the connection between study dimensions. Additionally, we advise that future researchers look at these subjects using this model and cross-validate them by incorporating cultural considerations.
Data availability statement
The original contributions presented in the study are included in the article/Supplementary material, further inquiries can be directed to the corresponding author.
Ethics statement
The study involving human participants was approved by the Scientific Committee of the Department of Sociology at the University of Jordan. Digital informed consent for participation in the study was provided by the participants.
Author contributions
HD and NH: conceptualization. HD, NH, MNA, and RA: methodology and writing – review and editing. HD, NH, MNA, RA, AA and MA-S: resources. HD, NH, MNA, RA, AA and MA-S: writing – original draft preparation. HD, NH, and RA: project administration. All authors contributed to the article and approved the submitted version.
Conflict of interest
The authors declare that the research was conducted in the absence of any commercial or financial relationships that could be construed as a potential conflict of interest.
Publisher’s note
All claims expressed in this article are solely those of the authors and do not necessarily represent those of their affiliated organizations, or those of the publisher, the editors and the reviewers. Any product that may be evaluated in this article, or claim that may be made by its manufacturer, is not guaranteed or endorsed by the publisher.
Supplementary material
The Supplementary material for this article can be found online at: https://www.frontiersin.org/articles/10.3389/feduc.2023.1226718/full#supplementary-material
References
Abdullah, F., and Ward, R. (2016). Developing a general extended technology acceptance model for E-learning (GETAMEL) by analyzing commonly used external factors. Comput. Hum. Behav. 56, 238–256. doi: 10.1016/j.chb.2015.11.036
Agrawal, A. K., and Mittal, G. K. (2018). “The role of ict in higher education,” In multidisciplinary higher education, research, dynamics and concepts. Perth, Australia: Swaranjali Publication.
Ajzen, I., and Fishbein, M. (1980). Understanding attitudes and predicting social behavior. Englewood Cliffs, NJ: Prentice-Hall.
Ajibade, P. (2018).Technology acceptance model limitations and criticisms: exploring the practical applications and use in technology-related studies, mixed-method, and qualitative researches. Library Philosophy and Practice (e-journal). 1941. Available at: http://digitalcommons.unl.edu/libphilprac/1941
Al-Adwan, A. S., Li, N., Al-Adwan, A., Abbasi, G. A., Albelbisi, N. A., and Habibi, A. (2023). Extending the technology acceptance model (TAM) to predict university students’ intentions to use Metaverse-based learning platforms. Educ. Inf. Technol. 1–33. doi: 10.1007/s10639-023-11816-3
Al-Adwan, A., and Albelbisi, N. (2020). Investigating the impact of social media use on Student’s perception of academic performance in higher education: evidence from Jordan. J. Inf. Technol. Educ.:Res. 19, 953–975. doi: 10.28945/4661
Alismaiel, O. A., Cifuentes-Faura, J., and Al-Rahmi, W. M. (2022). Social media technologies used for education: an empirical study on TAM model during the COVID-19 pandemic. Front. Educ. 7:882831. doi: 10.3389/feduc.2022.882831
Alkhwaldi, F., Abeer, T., and Al-Ajaleen, R. (2022). Toward a conceptual model for citizens’ adoption of smart Mobile government services during the COVID-19 pandemic in Jordan. Inf. Sci. Lett. 11, 1–13. doi: 10.18576/isl/110225
Al-Mahdi, M. S., and Maamari, M. (2019). Digital communication in higher education institutions. Adrar University: Algeria.
Al-Mamary, Y. H., Al-nashmi, M., Abdul, Y., Hassan, G., and Shamsuddin, A. (2016). A critical review of models and theories in field of individual acceptance of technology. Int. J. Hybrid Inf. Technol. 9, 143–158. doi: 10.14257/ijhit.2016.9.6.13
Almamlaka (2021). Approval of the system for integrating e-learning into higher education institutions, electronic article. Available at: https://www.almamlakatv.com/news/68812-%D8%A5%D9%82%D8%B1%D8%A7%D8%B1-%D9%86%D8%B8%D8%A7%D9%85-%D8%A5%D8%AF%D9%85%D8%A7%D8%AC-%D8%A7%D9%84%D8%AA%D8%B9%D9%84%D9%85-%D8%A7%D9%84%D8%A5%D9%84%D9%83%D8%AA%D8%B1%D9%88%D9%86%D9%8A-%D9%81%D9%8A-%D9%85%D8%A4%D8%B3%D8%B3%D8%A7%D8%AA-%D8%A7%D9%84%D8%AA%D8%B9%D9%84%D9%8A%D9%85-%D8%A7%D9%84%D8%B9%D8%A7%D9%84%D9%8A
Almulla, M. A. (2021). Technology acceptance model (TAM) and E-learning system use for education sustainability. Acad. Strategic Manag. J. 20, 1–13.
Alnemrat, A., Aldamen, H., Al-Deaibes, M., and Alsharefeen, R. (2023). E-learning in a Jordanian higher education institution. Front. Psychol. 14:1136142. doi: 10.3389/fpsyg.2023.1136142
Al-Rahmi, W. M., Alzahrani, A. I., Yahaya, N., Alalwan, N., and Kamin, Y. B. (2020). Digital communication: information and communication technology (ICT) usage for education sustainability. Sustainability 12:5052. doi: 10.3390/su12125052
Al-Rahmi, W. M., Yahaya, N., Alamri, M. M., Alyoussef, I. Y., Al-Rahmi, A. M., and Kamin, Y. B. (2019). Integrating innovation diffusion theory with technology acceptance model: supporting students’ attitude towards using a massive open online course (MOOCs) systems. Interactive Learning Environ. 29, 1–13. doi: 10.1080/10494820.2019.1629599
Alsabawy, A. Y., Cater-Steel, A., and Soar, J. (2016). Determinants of perceived usefulness of e-learning systems. Comput. Hum. Behav. 64, 843–858. doi: 10.1016/j.chb.2016.07.065
Al-Sadiq, K. M. (2022). The role of digital communication in improving job performance in university institutions in Algeria. Constantine University: Algeria.
Amarin, N. (2022). The future of blended learning in Jordanian universities post-Covid 19. Cypriot J. Educ. Sci. 17, 4114–4125. doi: 10.18844/cjes.v17i11.7572
Arbaugh, J. B. (2010). Multi-disciplinary and program-level research in online business education. In: Online and blended business education for the 21st century, Woodhead Publishing Limited. Sawston
Arya, R., Antonisamy, B., and Kumar, S. (2012). Sample size estimation in prevalence studies. Indian J. Pediatrics 79, 1482–1488. doi: 10.1007/s12098-012-0763-3
Asrani, C., and Kar, A. (2022). Diffusion and adoption of digital communications services in India. Inf. Technol. Dev. 28, 488–510. doi: 10.1080/02681102.2022.2046536
Atombo, C., Wu, C., Zhang, H., and Wemegah, T. D. (2017). Perceived enjoyment, concentration, intention, and speed violation behavior: using flow theory and theory of planned behavior. Traffic Inj. Prev. 18, 694–702. doi: 10.1080/15389588.2017.1307969
Attaran, M. (2020). Digital technology enablers and their implications for supply chain management. Supply Chain Forum. 21, 158–172. doi: 10.1080/16258312.2020.1751568
Ayman, U., Kaya, A. K., and Kuruç, Ü. K. (2020). The impact of digital communication and PR models on the sustainability of higher education during crises. Sustainability 12:8295. doi: 10.3390/su12208295
Babu, G. S., and Ma, K. S. (2018). Role of information and communication technology (ICT) in higher education: a study. Int. J. Multidisciplinary Ed. and Res. 3, 103–109.
Bagdi, H., and Bulsara, H. P. (2023). Understanding the role of perceived enjoyment, self-efficacy and system accessibility: digital natives' online learning intentions. J. App. Res. Higher Ed. doi: 10.1108/JARHE-09-2022-0302 [Epub ahead-of-print].
Batucan, G. B., Gonzales, G. G., Balbuena, M. G., Pasaol, K. R. B., Seno, D. N., and Gonzales, R. R. (2022). An extended UTAUT model to explain factors affecting online learning system amidst COVID-19 pandemic: the case of a developing economy. Front. Artif. Intell. 5:768831. doi: 10.3389/frai.2022.768831
Buabeng-Andoh, C. (2018). Predicting students’ intention to adopt mobile learning: a combination of theory of reasoned action and technology acceptance model. J. Res. Innov. Teach. Learn. 11, 178–191. doi: 10.1108/JRIT-03-2017-0004
Burgess, G. L., and Worthington, A. K. (2023). Technology acceptance model. Available at: https://ua.pressbooks.pub/persuasiontheoryinaction/chapter/technology-acceptance-model/
Cabada, R. Z., Estrada, M. L. B., Hernández, F. G., Bustillos, R. O., and Reyes-García, C. A. (2017). An affective and web 3.0-based learning environment for a programming language. Telemat. Inform. 35, 611–628. doi: 10.1016/j.tele.2017.03.005
Català-Oltra, L., Martínez-Gras, R., and Penalva-Verdú, C. (2023). The use of languages in digital communication at European universities in multilingual settings. Int. J. Soc Culture Lang. 11, 1–15. doi: 10.22034/ijscl.2022.563470.2794
Chahal, J., and Rani, N. (2022). Exploring the acceptance for e-learning among higher education students in India: combining technology acceptance model with external variables. J. Comput. High. Educ. 34, 844–867. doi: 10.1007/s12528-022-09327-0
Charness, N., and Boot, W. R. (2016). Technology, gaming, and social networking, in handbook of the psychology of aging. Elsevier. p. 389–407. Amsterdam.
Chernikova, O., Heitzmann, N., Stadler, M., Holzberger, D., Seidel, T., and Fischer, F. (2020). Simulation-based learning in higher education: a Meta-analysis. Rev. Ed. Res. 90, 499–541. doi: 10.3102/0034654320933544
Cornalba, C., and Giudici, P. (2004). Statistical models for operational risk management. Physica A: Stat. Mech. App. 338, 166–172. doi: 10.1016/j.physa.2004.02.039
Davis, F. D. (1989). Perceived usefulness, perceived ease of use, and user acceptance of information technology. MIS Q. 13, 319–340. doi: 10.2307/249008
Doyle, L. (2019). What is digital communication?|in-demand skills and careers. Available at: https://bachelors-completion.northeastern.edu/news/what-is-digital-communication/
Elkaseh, A., Wai Wong, K., and Fung, C. (2016). Perceived ease of use and perceived usefulness of social media for e-learning in Libyan higher education: a structural equation modeling analysis. Int. J. Inf. Ed. Tech. 6, 192–199. doi: 10.7763/IJIET.2016.V6.683
El-Sofany, H. F., and El-Haggar, N. (2020). The effectiveness of using Mobile learning techniques to improve learning outcomes in higher education. Int. J. Interactive Mobile Tech. (iJIM) 14, 4–18. doi: 10.3991/ijim.v14i08.13125
Hair, J., Hult, G., Ringle, C., and Sarstedt, M. (2021). A primer on partial least squares structural equation modeling (PLS-SEM). Sage Publications. Thousand Oaks, CA
Hair, J., Sarstedt, M., Ringle, C., and Gudergan, S. (2017). Advanced issues in partial least squares structural equation modeling. 1st Sage Publications. Thousand Oaks, CA
Huang, C. (2021). Exploring the continuous usage intention of online learning platforms from the perspective of social capital. Information (Switzerland) 12:141. doi: 10.3390/info12040141
Ifinedo, P., Pyke, J., and Anwar, A. (2017). Business undergraduates’ perceived use outcomes of Moodle in a blended learning environment: the roles of usability factors and external support. Telematics Inform. 35, 93–102. doi: 10.1016/j.tele.2017.10.001
Igbaria, M., Schiffman, S. J., and Wieckowski, T. J. (1994). The respective roles of perceived usefulness and perceived fun in the acceptance of microcomputer technology. Behav. Inf. Technol. 13, 349–361. doi: 10.1080/01449299408914616
Jasin, M. (2022). The effect of perceived ease of use on behavior intention through perceived enjoyment as an intervening variable on digital payment in the digital era. J. Industrial Eng. Manag. Res. 3, 127–133. doi: 10.7777/jiemar.v3i5.414
Kamal, S. A., Shafiq, M. I., Shafiq, M. I., and Kakria, P. (2020). Investigating acceptance of telemedicine services through an extended technology acceptance model (TAM). Technol. Soc. 60:101212. doi: 10.1016/j.techsoc.2019.101212
Khoa, B. T. (2020). The perceived enjoyment of the online courses in digital transformation age: the uses-gratification theory approach. 2020 sixth international conference on e-learning (econf), Sakheer, Bahrain 2020.
Kline, R. B. (2023). Principles and practice of structural equation modeling. Guilford publications. New York City
Krouska, A., Troussas, C., and Sgouropoulou, C. (2022). Extended technology acceptance models for digital learning: review of external factors. In Novel & Intelligent Digital Systems: Proceedings of the 2nd international conference.
Lai, P. C. (2017). The literature review of technology adoption models and theories for the novelty technology. J. Inf. Syst. Technol. Manag. 14, 21–38. doi: 10.4301/s1807-17752017000100002
Larafi, I., and Zawali, K. (2021). Digital communication and its role in the achievement of media and sports communication students in M’sila, M’sila University. Algeria
Lee, F. L. F., Liang, H., Cheng, E. W., Tang, G. K. Y., and Yuen, S. (2022). Affordances, movement dynamics, and a centralized digital communication platform in a networked movement. Information. Commun. Soc. 25, 1699–1716. doi: 10.1080/1369118X.2021.1877772
Liu, C. H., and Huang, Y. M. (2015). An empirical investigation of computer simulation technology acceptance to explore the factors that affect user intention. Univ. Access Inf. Soc. 14, 449–457. doi: 10.1007/s10209-015-0402-7
Lopez, L., and López-Bonilla, J. (2016). Explaining the discrepancy in the mediating role of attitude in the TAM. Brit. J. Ed. Tech. 48, 940–949. doi: 10.1111/bjet.12465
Madanat, H., Tarawnah, M., and Khzouz, A. (2023). “Adapting to the new Normal: asynchronous learning as a promising solution for post-Covid-19 challenges in Jordanian universities - a case study of the American University of Madaba (AUM)” in Future trends in education post COVID-19. SHJEDU 2022. eds. H. M. K. Al Naimiy, M. Bettayeb, H. M. Elmehdi, and I. Shehadi (Singapore: Springer).
Marikyan, D., and Papagiannidis, S. (2023). “Technology acceptance model: a review” in Theory hub book. ed. S. Papagiannidis Available at: http://open.ncl.ac.uk/
Mimiaga, M., Reisner, S., Reilly, L., Soroudi, N., and Safren, S. (2009). “Individual interventions” in HIV prevention: A comprehensive approach. eds. K. H. Mayer and H. F. Pizer (Cambridge, MA: Academi c Press), 203–239.
Min, S., So, K. K. F., and Jeong, M. (2019). Consumer adoption of the Uber mobile application: insights from diffusion of innovation theory and technology acceptance model. J. Travel Tourism Marketing 36, 770–783. doi: 10.1080/10548408.2018.1507866
Moreno, V., Cavazotte, F., and Alves, I. (2016). Explaining university students’ effective use of e-learning platforms. Br. J. Educ. Technol. 48, 995–1009. doi: 10.1111/bjet.12469
Murari, K., and Rai, S. (2022). University Students' attitudes and intentions to use online learning system in higher education: examining the role of individual and system characteristics. Int. J. Distance Educ. e-Learn. 10, 399–416.
Nelwamondo, M., and Njenga, J. K. (2021). Approaches for enhancing information sharing between government and communities in Western cape. S. African J. Info. Manag. 23, 1–9. doi: 10.4102/sajim.v23i1.1414
Pedro, E. D. M., Leitão, J., and Alves, H. (2020). Stakeholders’ perceptions of sustainable development of higher education institutions: an intellectual capital approach. Int. J. Sustainability Higher Ed. 21, 911–942. doi: 10.1108/IJSHE-01-2020-0030
Postelnyak, M. (2023). What is digital communication in the workplace. Available at: https://www.contactmonkey.com/blog/workplace-digital-communication
Protopsaltis, S., and Baum, S. (2019). Does online education live up to its promise? A look at the evidence and implications for federal policy center for educational policy evaluation. Washington, D.C., USA: Urban Institute.
Rama, M., Selvabaskar, S., Guhana, R., and Rajarathic, K. (2023). The effect of digital communication technologies in retail supply chain management: evidence from small Indian retailers. J. Future Sustainability 3, 125–132. doi: 10.5267/j.jfs.2023.1.002
Ramesh, P., Manickam, R., and Chinnasamy, S. (2022). An empirical study on effectiveness of E-learning over conventional class room learning – a case study with respect to online degree Programmes in higher education. Recent Trends Manag. Commerce 3, 25–33. doi: 10.46632/rmc/3/1/5
Ratna, A., (2023). Digital communication– Introduction, basic components, how signal process works, and advantages, in electrical Funda blog. Available at: https://electricalfundablog.com/digital-communication-introduction-basic-components-how-signal-process-works-and-advantages/
Rosli, M. S., Saleh, N. S., Azlah, M., Ali, S. A. B., and Tahir, L. M. (2022). A systematic review of the technology acceptance model for the sustainability of higher education during the COVID-19 pandemic and identified research gaps. Sustainability 14:11389. doi: 10.3390/su141811389
Saldanha, T. (2019). Why digital transformations fail: The surprising disciplines of how to take off and stay ahead. Berrett-Koehler Publishers. Oakland, CA.
Saleh, S. S., Nat, M., and Aqel, M. (2022). Sustainable adoption of E-learning from the TAM perspective. Sustainability 14:3690. doi: 10.3390/su14063690
Salloum, S. A. (2018). Investigating students' acceptance of e-learning system in higher educational environments in the UAE: applying the extended technology acceptance model (TAM). Dissertations for informatics, The British University in Dubai. Available at: http://bspace.buid.ac.ae/handle/1234/1150
Salloum, S. A., Alhamad, Q., Al-Emran, M., Abdel Monem, A., and Shaalan, K. F. (2019). Exploring students’ acceptance of E-learning through the development of a comprehensive technology acceptance model. IEEE Access 7, 128445–128462. doi: 10.1109/ACCESS.2019.2939467
Samuel, N. S., Onasanya, S. A., and Olumorin, C. O. (2018). Perceived usefulness, ease of use and adequacy of use of Mobile technologies by Nigerian university lecturers. Int. J. Ed. Develop. Inf. Comm. Tech. 14, 5–16.
Saravanos, A., Zervoudakis, S., and Zheng, D. (2022). Extending the technology acceptance model 3 to incorporate the phenomenon of warm-glow. Information 13:429. doi: 10.3390/info13090429
Sarstedt, M., Ringle, C. M., and Hair, J. F. (2017). “Partial least squares structural equation modeling” in Handbook of market research. eds. C. Homburg, M. Klarmann, and A. Vomberg (Cham: Springer)
Sayaf, A. M., Alamri, M. M., Alqahtani, M. A., and Al-Rahmi, W. M. (2021). Information and communications technology used in higher education: an empirical study on digital learning as sustainability. Sustainability 13:7074. doi: 10.3390/su13137074
Scherer, R., Siddiq, F., and Tondeur, J. (2019). The technology acceptance model (TAM): a meta-analytic structural equation modeling approach to explaining teachers' adoption of digital technology in education. Comput. Educ. 128, 13–35. doi: 10.1016/j.compedu.2018.09.009
Shiau, W., and Luo, M. M. (2013). Continuance intention of blog users: the impact of perceived enjoyment, habit, user involvement and blogging time. Behav. Inf. Tech. 32, 570–583. doi: 10.1080/0144929X.2012.671851
Stone, C. B., and Wang, Q. (2019). From conversations to digital communication: the mnemonic consequences of consuming and producing information via social media. Topics Cog. Sci. 11, 774–793. doi: 10.1111/tops.12369
Sukendro, S., Habibi, A., Khaeruddin, K., Indrayana, B., Syahruddin, S., Makadada, F. A., et al. (2020). Using an extended technology acceptance model to understand students' use of e-learning during Covid-19: Indonesian sport science education context. Heliyon 6:e05410. doi: 10.1016/j.heliyon.2020.e05410
Sullivan, M. (2016). Extended technology acceptance model (TAM2) [personality & TKMS series]. Knowledge management (KM). Available at: https://realkm.com/2016/08/24/extended-technology-acceptance-model-tam2-personality-tkms-series/
Sumedrea, S., Maican, C. I., Chițu, I. B., Nichifor, E., Tecău, A. S., Lixăndroiu, R. C., et al. (2022). Sustainable digital communication in higher education—a checklist for page loading speed optimisation. Sustainability 14:10135. doi: 10.3390/su141610135
Surendran, P. (2012). Technology acceptance model: a survey of literature. Int. J. Bus. Soc. Res. 2, 175–178. doi: 10.18533/ijbsr.v2i4.161
Taylor, S., and Todd, P. (1995). Assessing it usage: the role of prior experience, MIS quarterly. pp. 561–570.
Teo, T., and Noyes, J. (2011). An assessment of the influence of perceived enjoyment and attitude on the intention to use technology among pre-service teachers: a structural equation modeling approach. Comp. Ed. 57, 1645–1653. doi: 10.1016/j.compedu.2011.03.002
Teo, T., and Zhou, M. (2014). Explaining the intention to use technology among university students: a structural equation modeling approach. J. Comp. Higher Ed. 26, 124–142. doi: 10.1007/s12528-014-9080-3
Tomej, K., Liburd, J., Blichfeldt, B. S., and Hjalager, A. M. (2022). Blended and (not so) splendid teaching and learning: higher education insights from university teachers during the Covid-19 pandemic. Int. J. Ed. Res. Open 3:100144. doi: 10.1016/j.ijedro.2022.100144
Venkatesh, V., and Bala, H. (2008). Technology acceptance model 3 and a research agenda on interventions. Decis. Sci. 39, 273–315.
Venkatesh, V., and Davis, F. (2000). A theoretical extension of the technology acceptance model: four longitudinal field studies. Manag. Sci. 46, 186–204. doi: 10.1287/mnsc.46.2.186.11926
Venkatesh, V., Morris, M., Davis, G., and Davis, F. (2003). User acceptance of information technology: toward a unified view. MIS Q. 27, 425–478. doi: 10.2307/30036540
Winarno, W. A., Mas’ud, I., and Palupi, T. W. (2021). Perceived enjoyment, application self-efficacy, and subjective norms as determinants of behavior intention in using OVO applications. J. Asian Finance Econom. Bus. 8, 1189–1200. doi: 10.13106/jafeb.2021
Won, D., Chiu, W., and Byun, H. (2023). Factors influencing consumer use of a sport-branded app: the technology acceptance model integrating app quality and perceived enjoyment. Asia Pacific J. Marketing and Logistics 35, 1112–1133. doi: 10.1108/APJML-09-2021-0709
Zaida, S. (2019). Blended learning at the university of jordan: will the experiment succeed? Electronic article. Available at: https://www.7iber.com/society/%D8%A7%D9%84%D8%AA%D8%B9%D9%84%D9%85-%D8%A7%D9%84%D9%85%D8%AF%D9%85%D8%AC-%D9%81%D9%8A-%D8%A7%D9%84%D8%A3%D8%B1%D8%AF%D9%86%D9%8A%D8%A9/
Zhou, L., Xue, S., and Li, R. (2022). Extending the technology acceptance model to explore students’ intention to use an online education platform at a University in China. SAGE Open 12:215824402210852. doi: 10.1177/21582440221085259
Keywords: sustainable digital communication, perceived enjoyment, technology acceptance model, higher education, Jordan
Citation: AlDreabi H, Halalsheh N, Alrawashdeh MN, Alnajdawi AM, Alsawalqa RO and Al-Shboul M (2023) Sustainable digital communication using perceived enjoyment with a technology acceptance model within higher education, in Jordan. Front. Educ. 8:1226718. doi: 10.3389/feduc.2023.1226718
Edited by:
Terrell Lamont Strayhorn, Virginia Union University, United StatesReviewed by:
Antonio Víctor Martín-García, University of Salamanca, SpainAloysius H. Sequeira, National Institute of Technology, Karnataka, India
Copyright © 2023 AlDreabi, Halalsheh, Alrawashdeh, Alnajdawi, Alsawalqa and Al-Shboul. This is an open-access article distributed under the terms of the Creative Commons Attribution License (CC BY). The use, distribution or reproduction in other forums is permitted, provided the original author(s) and the copyright owner(s) are credited and that the original publication in this journal is cited, in accordance with accepted academic practice. No use, distribution or reproduction is permitted which does not comply with these terms.
*Correspondence: Rula Odeh Alsawalqa, Ui5zYXdhbGthQGp1LmVkdS5qbw==
†ORCID: Maissa N. Alrawashdeh, https://orcid.org/0000-0001-7940-5016
Rula Odeh Alsawalqa, https://orcid.org/0000-0002-5605-5444