- 1Department of Psychological and Brain Sciences, University of Louisville, Louisville, KY, United States
- 2Department of Engineering Fundamentals, University of Louisville, Louisville, KY, United States
- 3Department of Information Systems, Analytics and Operations, University of Louisville, Louisville, KY, United States
STEM undergraduate instructors teaching remote courses often use traditional lecture-based instruction, despite evidence that active learning methods improve student engagement and learning outcomes. One simple way to use active learning online is to incorporate exploratory learning. In exploratory learning, students explore a novel activity (e.g., problem solving) before a lecture on the underlying concepts and procedures. This method has been shown to improve learning outcomes during in-person courses, without requiring the entire course to be restructured. The current study examined whether the benefits of exploratory learning extend to a remote undergraduate physics lesson, taught synchronously online. Undergraduate physics students (N = 78) completed a physics problem-solving activity either before instruction (explore-first condition) or after (instruct-first condition). Students then completed a learning assessment of the problem-solving procedures and underlying concepts. Despite lower accuracy on the learning activity, students in the explore-first condition demonstrated better understanding on the assessment, compared to students in the instruct-first condition. This finding suggests that exploratory learning can serve as productive failure in online courses, challenging students but improving learning, compared to the more widely-used lecture-then-practice method.
Introduction
Use of online learning formats has increased in recent years, in part due to better technology, convenience, and extenuating circumstances (e.g., the COVID-19 pandemic). However, the predominant instructional method used in online courses is lecture—either delivered synchronously (with students attending via live videoconference) or asynchronously (with students watching prerecorded lectures) (Sandrone and Schneider, 2020). Although learning from lecture has been shown to be equivalent between in-person and online lecture formats (e.g., Vaccani et al., 2016; Brockfeld et al., 2018; Chirikov et al., 2020; Olsen et al., 2020; Musunuru et al., 2021), students report less engagement in online courses (Vaccani et al., 2016; Olsen et al., 2020). Moreover, lecture-based methods result in more superficial attention and lower learning outcomes, compared to methods that incorporate active learning (Wegner, 1998; Prince, 2004; Freeman et al., 2014). The current research examines how one method to improve active learning during a live online lecture (i.e., exploratory learning), impacts students’ learning of the lesson material.
Active learning
Active learning includes any meaningful instructional activity that requires students to actively engage and respond during class, and to think about what they are doing (Prince, 2004; Felder and Brent, 2009). Rather than passively viewing a lecture, active learning engages students in constructing or applying knowledge for themselves. Commonly-described active learning activities range from collaborative or cooperative learning, personal response systems (e.g., clicker questions), answering questions in a think-pair-share format, and problem-solving activities (Felder and Brent, 2009; Freeman et al., 2014).
Active learning can help students engage in constructive or interactive levels of engagement known to be associated with greater learning (Chi and Wiley, 2014). Active learning methods can also support social-psychological needs, such as self-efficacy and perceptions of belonging (Ballen et al., 2017). Courses using these methods have been shown to reduce or eliminate gaps in learning outcomes between minoritized and majority students otherwise found in traditional lecture-based courses (Haak et al., 2011; Ballen et al., 2017; Theobald et al., 2020). Despite these important benefits, active learning methods are less frequently used in online instruction. Moreover, there are few studies comparing learning outcomes between online course sessions that use active learning methods vs. more traditional lecture-only instruction (cf., McClellan et al., 2023).
Instructors often resist using active learning methods, because they assume use of these methods will require a great deal of time and effort (Mogavi et al., 2021). However, certain active learning methods can be implemented online without an entire course overhaul. Even interspersing well-designed learning activities among shorter lectures can be beneficial (Sandrone et al., 2021). Exploratory learning activities provide one such approach. In exploratory learning, students complete a novel learning activity (e.g., solve problems) before receiving instruction on the procedure and concepts (DeCaro and Rittle-Johnson, 2012). This overarching term describes the two-phase sequence used across studies from the literatures on productive failure (e.g., Kapur, 2008), preparation for future learning (e.g., Schwartz et al., 2009), and problem-solving-instruction methods (PS-I; e.g., Loibl and Rummel, 2014).
Multiple studies across these literatures, using exploratory learning during in-person class or lab settings, have shown that exploring before instruction can improve learning (Loibl et al., 2017; Darabi et al., 2018; Sinha and Kapur, 2021). However, relatively few studies have implemented exploratory learning in undergraduate STEM courses (e.g., Weaver et al., 2018; Chowrira et al., 2019; Bego et al., 2022; DeCaro et al., 2022). Even fewer studies have compared the use of exploratory learning before instruction in an online format to a more traditional online lecture-then-practice approach (e.g., Hieb et al., 2021). Given the importance of active learning methods for learning and persistence in STEM disciplines, exploratory learning provides a promising, relatively simple, method to accompany lecture in an online course.
Exploratory learning
In traditional lecture-based instruction, students often experience cognitive fluency, in which they process the information superficially and think they understand the information better than they actually do (Bjork, 1994; Felder and Brent, 2009; DeCaro and Rittle-Johnson, 2012). When engaging in exploratory learning before lecture, students are given a novel problem or activity that is relevant to the target topic. Students can begin to attempt this activity using prior knowledge, but they have not necessarily encountered the problem before (Kapur, 2016). This process better enables students to integrate the new information with their existing knowledge, creating more connected schemas in long-term memory (Schwartz et al., 2009; Kapur, 2016; Chen and Kalyuga, 2020).
However, the activity is novel, and students often solve the problem incorrectly. Through this process, students can become more aware of the gaps in their knowledge (Loibl and Rummel, 2014; Glogger-Frey et al., 2015). Students might become more curious (Glogger-Frey et al., 2015; Lamnina and Chase, 2019), and desire to make sense of the content, leading them to pay greater attention during the subsequent lecture (Wise and O’Neill, 2009). This exploratory process has therefore been described as productive failure (Kapur, 2016).
During the exploration process, students also test hypotheses, and begin to discern which features of the problem are relevant or useful for a solution, and which elements are less important (DeCaro and Rittle-Johnson, 2012). Some of these elements may include the relationships between individual features in a more complex, to-be-learned formula (Alfieri et al., 2013; Chin et al., 2016). These cognitive, metacognitive, and motivational processes can help to deepen students’ understanding (Loibl et al., 2017).
The benefits of exploring before instruction are generally found on measures of conceptual understanding (Loibl et al., 2017), although some studies find benefits for procedural knowledge as well (e.g., Kapur, 2010, 2011; Kapur and Bielaczyc, 2012). Conceptual knowledge consists of underlying principles and relationships between connected ideas, whereas procedural knowledge includes the memorization or use of a series of actions to solve problems (Rittle-Johnson et al., 2001).
For example, Bego et al. (2022, Experiment 1) examined the causal benefits of exploratory learning during an in-person undergraduate physics lesson on gravitational field. Bego et al. randomly assigned half of the class to a traditional lecture-then-practice condition (instruct-first condition). The other half were assigned to an exploratory learning condition (explore-first condition), in which they instead completed the same learning activity prior to instruction. On a subsequent posttest, students in the explore-first condition showed equal procedural knowledge as those in the instruct-first condition, but higher conceptual knowledge. Thus, students learned and applied the formulas they were taught equally well between conditions, but understood the relational principles better after having explored the topic for themselves first.
Current study
The current experiment examined whether the benefits of exploratory learning extend to a synchronous, online learning environment, during which the class meets live via videoconference. We adapted Bego et al.’s (2022) materials and procedures for an online physics lesson on gravitational field, and students participated during their regular class time. The course instructor was the same as in Bego et al.’s study. Students were randomly assigned to either an explore-first or instruct-first condition, and participated on separate class days. Students in the instruct-first condition completed the traditional instructional order (lecture then activity). Students in the explore-first condition completed the same materials in reverse order (activity then lecture). The activity included a series of contrasting cases, examples that vary in ways that help students encounter important features of the problems (e.g., Schwartz et al., 2011; Roll et al., 2012; Roelle and Berthold, 2015). Students completed a posttest assessing their procedural and conceptual knowledge of gravitational field. By randomly assigning students to condition, and providing the exact same materials in reverse order, this experimental research design allowed us to examine the causal effect of exploring before instruction in an online setting.
Although we used the same basic materials as Bego et al. (2022), we made several general modifications to the procedure, due to time constraints. The class period in our study was shorter (50 min vs. 75 min in Bego et al.). To account for this decreased time, first we focused only on the gravitational field lesson, and did not include other measures that Bego et al. used (i.e., learning transfer or survey). Second, students completed the posttest on their own, outside of class time. Also, as is commonly needed with online courses, we allowed more time during class for transitions (Mogavi et al., 2021; Venton and Pompano, 2021). We allowed for more class time for students to locate and download the learning materials and transition in and out of breakout groups.
Our specific research questions included the following:
1. Does exploratory learning before instruction improve learning outcomes compared to an instruct-then-practice order, when used in an online, undergraduate STEM class?
2. Do the benefits of exploring before instruction depend on the type of knowledge assessed (i.e., procedural vs. conceptual knowledge)?
3. Do our findings resemble prior research using these materials in an in-person classroom setting (i.e., Bego et al., 2022)?
We expected that the modality of teaching would not change the cognitive, metacognitive, or motivational learning benefits of exploring, even in an online setting. We hypothesized that students in the explore-first condition would score higher on the posttest, especially on the conceptual knowledge subscale. Thus, we also hypothesized that our results would resemble those of Bego et al. (2022), extending those findings to an online, synchronous learning setting.
Method
Participants
Participants (N = 78) included all students who attended an online, synchronous, introductory undergraduate physics course (Fundamentals of Physics I) on the dates of the experiment and submitted all learning materials. Participants were primarily upper-level undergraduate students working toward degrees with a pre-professional health science focus. Additional participants were excluded from analyses for experiencing an internet connection issue during class that lasted more than 1 min during a critical time period (n = 3) or for scoring a 0 on the procedural knowledge subscale of the posttest (n = 1), indicating lack of attention or effort.
Materials
Learning activity and pedagogy
Students completed the same gravitational field learning materials as used by Bego et al. (2022, Experiment 1), with some modifications for online administration (Figure 1). The materials provided a set of contrasting cases, including ten scenarios (points PA – PJ), within three figures, designed to differ along critical problem features (distance, mass, relative position, number of objects). Values for the masses and distances were given in each figure, and a table listed gravitational field vectors with magnitude and direction. Students were asked to determine which points in space corresponded to each vector.
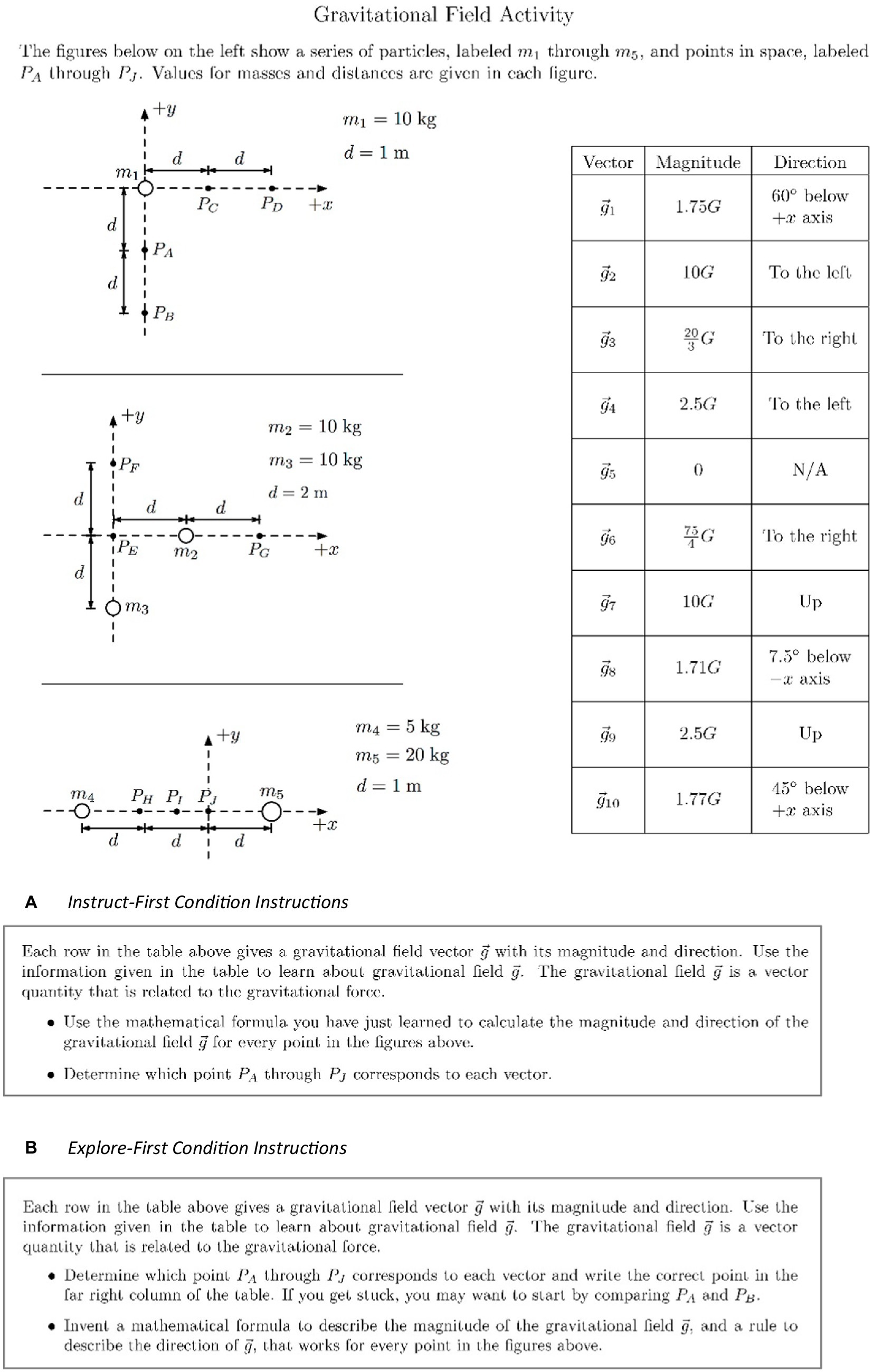
Figure 1. Learning activity, including instructions for the (A) instruct-first condition and (B) explore-first condition (adapted from Bego et al., 2022).
Students in the explore-first condition were asked to invent a mathematical equation that describes the magnitude of the gravitational field, and a rule to describe the direction of the gravitational field that works for every point in space. Students had not yet learned how to solve these problems in lecture. Because the activity included contrasting cases that varied by masses, distances, and number of objects in the space, it was possible for students to identify the problem features and potentially invent the correct solution (Schwartz et al., 2011; Chin et al., 2016). Students in the instruct-first condition were asked to use the mathematical equation they had learned in the instruction to calculate the magnitude and direction of the gravitational field for each point in space. Students in both conditions were given a table on a separate page, with the 10 vectors listed in the left column, and a blank “Point” column to the right, on which to enter their answers. The rest of the page was blank, for students to write their work. Scores on the activity were calculated by summing the total number of correct “points” written, out of 10 possible.
Instruction
The course instructor lectured on gravitational field using a slideshow presentation. The lecture emphasized the concept of gravitational field and the formula to compute magnitude of this field. The instructor connected this topic to gravitational force, which had been taught in the previous class period. Then, he outlined how to use vector addition (with which the students were familiar) to do the calculations. Finally, the instructor completed an example problem on the screen.
Posttest
The posttest was adapted from Bego et al. (2022) and assessed students’ procedural and conceptual knowledge (Supplementary appendix). Procedural knowledge items (7 items, α = 0.79) asked students to find the magnitude and direction of gravitational field at various points in space. Students were given sets of masses, points in space, and distances between them. They were provided with the equation of gravitational field to support their work. Conceptual knowledge items (10 items, α = 0.30) included true/false questions about gravitational force and field, distance, mass, and direction, including several common misconceptions. These questions were designed to target students’ relational understanding across a range of different concepts, such that higher scores across the range of items indicated greater conceptual understanding.
Procedure
Students from one Fundamentals of Physics I course section were randomly assigned to explore-first (n = 37) or instruct-first (n = 41) conditions, and attended class on the day of their assigned condition. Students participated online during one 50-min class period through Blackboard Collaborate, the learning management system (LMS) used all semester.
The session included four phases: instruction, activity, activity review, and posttest. The only difference between conditions was the order of the phases. In the explore-first condition, students completed the learning activity first. The instructor provided “tips for effective exploration” on a slide, explaining that correct answers were less important than the process of working through the activity and trying to explain ideas. Students accessed the activity through a link on the LMS page and worked in breakout groups (13 min). Then, students rejoined the whole class and were given the lecture-based instruction (24 min), followed by a brief review of the learning activity and the answers (5 min). Students had the opportunity to ask questions throughout. Students in the instruct-first condition followed these same procedures, except they began with the lecture-based instruction, followed by the learning activity, then activity review.
At the end of class, all students were directed to the posttest link on their LMS, referred to as a “Gravitational Field Practice Assignment.” Students were advised that the assignment should take around 15 min to complete, to work individually, and that they would be graded for effort, not accuracy. Students were asked to submit their learning activities and posttests before the next class period.
All procedures were approved by the university Institutional Review Board. Students were debriefed about the study in a letter emailed at the end of the semester and given the opportunity to withdraw their data.
Data analysis method
Learning activity scores were analyzed using a between-subjects ANOVA as a function of condition (explore-first, instruct-first). Posttest scores for each subscale were transformed into percentages and analyzed using a 2 (condition: instruct-first, explore-first) × 2 (subscale: procedural, conceptual) mixed-factorial ANOVA, with condition between-subjects and subscale within-subjects.
Results
Learning activity
As expected, students in the explore-first condition had lower scores on the learning activity than students in the instruct-first condition, F(1, 76) = 11.12, p = 0.001, η2 = 0.13 (Table 1).
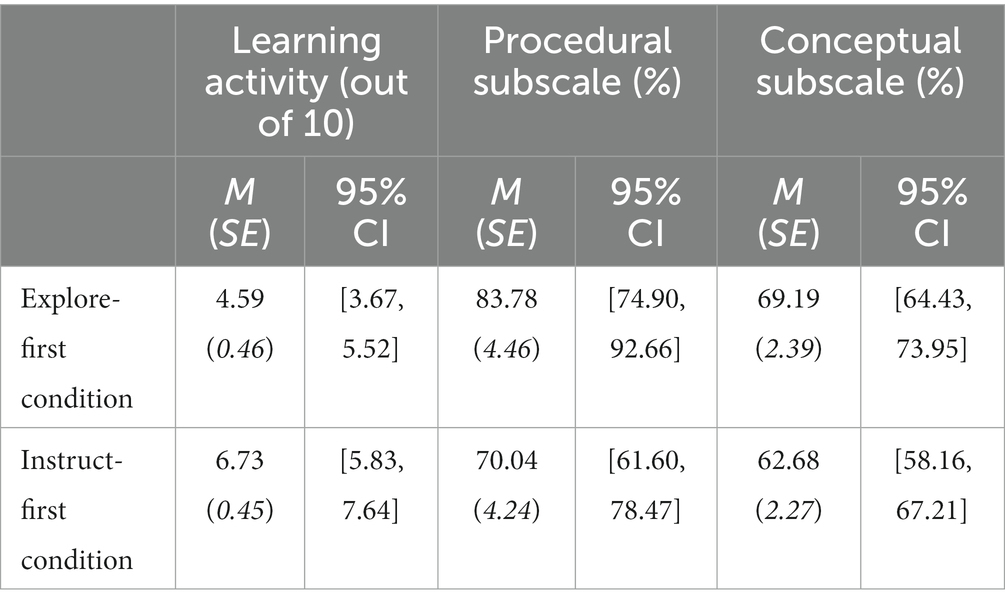
Table 1. Descriptive statistics for the learning activity and posttest scores on the conceptual and procedural knowledge subscales as a function of condition.
Posttest scores
As shown in Figure 2, there was a main effect of condition, in which students in the explore-first condition (M = 76.49%, SE = 3.05, 95% CI[70.41, 82.56]) had higher overall posttest scores than students in the instruct-first condition (M = 66.36%, SE = 2.90, 95% CI [60.59, 72.13]), F(1, 76) = 5.79, p = 0.019, ηp2 = 0.07. There was also a main effect of subscale, in which students scored higher on the procedural items (M = 76.91%, SE = 3.08, 95% CI [70.79, 83.03]) than the conceptual items (M = 65.94%, SE = 1.65, 95% CI [62.65, 69.22]), F(1, 76) = 18.15, p < 0.001, ηp2 = 0.19. No interaction was found, F(1, 76) = 1.98, p = 0.164, ηp2 = 0.03, suggesting that the effects of condition occurred similarly across both procedural and conceptual subscales. This observation was confirmed by examining 95% confidence intervals. As shown in Table 1, students in the explore-first condition scored higher than those in the instruct-first condition on both the procedural subscale and conceptual subscale.
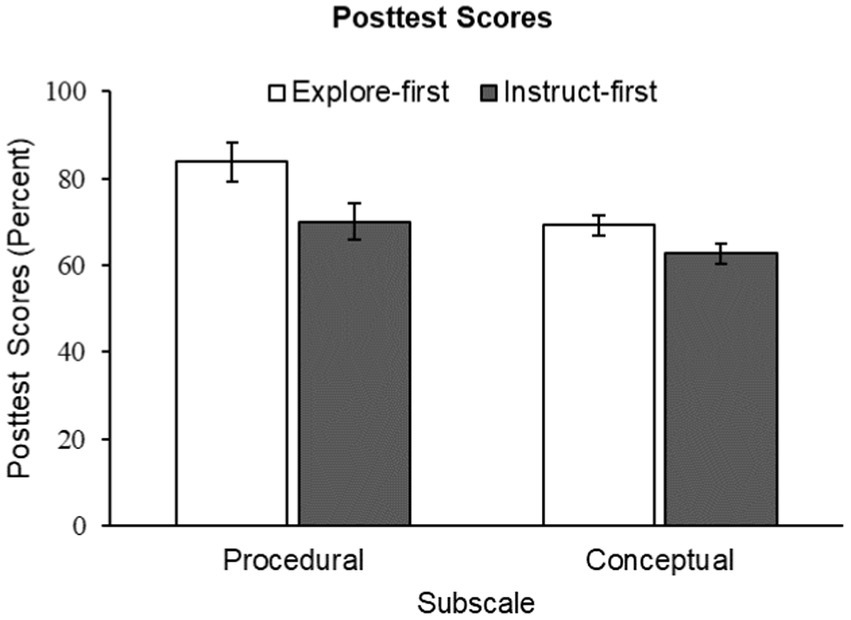
Figure 2. Posttest scores on the conceptual and procedural knowledge subscales as a function of condition. Error bars = ±1 SEM.
Discussion
Consistent with research on productive failure (Kapur, 2008), students who explored prior to instruction were less accurate on the learning activity, but scored higher on the posttest, than students who had lecture before completing the activity. This effect on the posttest occurred across both procedural and conceptual knowledge assessments. Thus, the benefits of exploring before instruction extended to an online undergraduate physics lesson, taught during a live videoconference.
Prior research demonstrates that students learn equally well from lectures given in-person vs. online (Sandrone et al., 2021). The current study demonstrates that, when an active learning activity is added online, the order of instruction matters. Giving an online lecture before a practice activity leads to lower learning outcomes than exploring the activity before lecture. These results mirror other exploratory learning studies conducted during in-person classes (Loibl et al., 2017), demonstrating that the benefits of exploring extend to online formats as well.
We specifically replicated Bego et al.’s (2022) general benefits of exploratory learning, using the same learning materials, instructor, and assessment. However, Bego et al. found selective benefits of exploration on conceptual, but not procedural, knowledge. We found that these benefits occurred across both posttest subscales. This difference seems more likely due to other changes made between studies, rather than the online format itself. Specifically, students in Bego et al.’s study worked on the posttest immediately after learning, without notes, and within a limited time frame. Students in the current study were not constrained in how long they worked on the posttest, or in what materials they used to help them. The open-ended nature of the assessment could have limited our ability to detect any differences between conditions. However, the higher scores in the explore-first condition suggest that these students may have had conceptual understanding that improved both their use of the provided formulas as well as understanding of the conceptual items (Rittle-Johnson et al., 2001). It is also possible that students in this condition simply tried harder on the posttest, for example due to greater interest or engagement in the material (e.g., Glogger-Frey et al., 2015). Importantly, in both studies, the posttest was described as a practice activity, and grades recorded for the assignment in the course were given based on effort, rather than accuracy. Thus, students might have felt less pressure to use external aids to help them with the assignment, improving our ability to detect differences in learning between conditions.
Limitations and future research
Although these findings provide promising support for the use of exploratory learning activities in online courses, there are potential limitations both in using online active-learning methods and in experimentally studying their use. First, because the study took place online, we cannot be certain that students worked on the learning activity when they were told to (e.g., before, rather than after, instruction). Given that students who were asked to explore before instruction on average scored lower on the learning activity, it seems likely that they followed these procedures in our study. However, instructors are less able to monitor what students do while working online. For similar reasons, controlling how students approach assessments is more difficult when done online vs. in person. Although we gave students ample time to work on the posttest, results might have looked different if students were asked to complete the posttest immediately and given time constraints (e.g., using an exam function in their LMS). However, our results generally mirror those of Bego et al. (2022), who used these same materials during an in-person class session. If control during assessment was an issue, it would more likely have diminished differences between conditions. That we found similar effects as Bego et al. suggests that control did not seem to be a factor in our study.
We also focused on only one outcome measure—posttest results for procedural and conceptual knowledge. Our results would be strengthened by additional survey and outcome measures, such as the transfer scale used in Bego et al.’s (2022) study.
Online learning in this study occurred in a live, synchronous, virtual classroom. More research is needed to determine if exploratory learning can be implemented, and benefit learning outcomes, in an online course conducted asynchronously. LMSs provide the tools to implement this method. For example, students can be required to submit an exploration activity (graded for effort, not accuracy) prior to being granted access to the video lecture on the topic. Similar methods can also be used in hybrid courses such as flipped classrooms. In such courses, instructors typically ask students to view a lecture outside of class, and spend class time using active learning methods such as cooperative problem solving (Lage et al., 2010). Rather than (or in addition to) asking students to solve problems after viewing the lecture, students could be given exploratory learning activities in class, prior to watching the assigned video lecture on the topic outside of class (Kapur et al., 2022). Like the current study, such methods have the potential to increase learning outcomes, and potentially other aspects of engagement as well.
Conclusion
In concert with the increasing capacity and demand for online instruction comes a need for use of evidence-based instruction in these courses. Instructors need guidance as to which methods to employ, and the research literature needs more studies to extend this evidence to online formats. The current study adds to this evidence, demonstrating that simply adding a novel activity before lecture may support students’ learning, even from afar.
Data availability statement
The datasets presented in this study can be found in online repositories. The names of the repository/repositories and accession number(s) can be found at: https://osf.io/pc6j8/?view_only=181a5a1f28114864a24a942b0433e74c.
Ethics statement
This research was approved by the University of Louisville Institutional Review Board. The studies were conducted in accordance with the local legislation and institutional requirements. Students were debriefed about the study in a letter emailed at the end of the semester and given the opportunity to withdraw their data.
Author contributions
MD, CB, and RC contributed to conception and design of the study. MD organized the database and performed the statistical analysis. MD and RI wrote the first draft of the manuscript. All authors contributed to the article and approved the submitted version.
Funding
This work was supported by the National Science Foundation Division for Undergraduate Education under grant number DUE-2012342. Any opinions, findings, conclusions or recommendations expressed in this material are those of the authors and do not necessarily reflect the views of the National Science Foundation or the University of Louisville.
Conflict of interest
The authors declare that the research was conducted in the absence of any commercial or financial relationships that could be construed as a potential conflict of interest.
Publisher’s note
All claims expressed in this article are solely those of the authors and do not necessarily represent those of their affiliated organizations, or those of the publisher, the editors and the reviewers. Any product that may be evaluated in this article, or claim that may be made by its manufacturer, is not guaranteed or endorsed by the publisher.
Supplementary material
The Supplementary material for this article can be found online at: https://www.frontiersin.org/articles/10.3389/feduc.2023.1215975/full#supplementary-material
References
Alfieri, L., Nokes-Malach, T. J., and Schunn, C. D. (2013). Learning through case comparisons: a meta-analytic review. Educ. Psychol. 48, 87–113. doi: 10.1080/00461520.2013.775712
Ballen, C. J., Wieman, C., Salehi, S., Searle, J. B., and Zamudio, K. R. (2017). Enhancing diversity in undergraduate science: self-efficacy drives performance gains with active learning. CBE—Life Sci. Educ. 16:ar56. doi: 10.1187/cbe.16-12-0344
Bego, C. B., Chastain, R. J., and DeCaro, M. S. (2022). Designing novel activities before instruction: use of contrasting cases and a rich dataset. Br. J. Educ. Psychol. 292, 299–317. doi: 10.1111/bjep.12555
Bjork, R. A. (1994). “Memory and metamemory considerations in the training of human beings” in Metacognition: Knowing about knowing. eds. J. Metcalfe and A. P. Shimamura (Cambridge MA, London, England: The MIT Press), 185–205.
Brockfeld, T., Muller, B., and de Laffolie, J. (2018). Video versus live lecture courses: a comparative evaluation of lecture types and results. Med. Educ. Online 23:1555434. doi: 10.1080/10872981.2018.1555434
Chen, O., and Kalyuga, S. (2020). Exploring factors influencing the effectiveness of explicit instruction first and problem-solving first approaches. Eur. J. Psychol. Educ. 35, 607–624. doi: 10.1007/s10212-019-00445-5
Chi, M., and Wiley, R. (2014). The ICAP framework: linking cognitive engagement to active learning outcomes. Educ. Psychol. 49, 219–243. doi: 10.1080/00461520.2014.965823
Chin, D. B., Chi, M., and Schwartz, D. L. (2016). A comparison of two methods of active learning in physics: inventing a general solution versus compare and contrast. Instr. Sci. 44, 177–195. doi: 10.1007/s11251-016-9374-0
Chirikov, I., Semenova, T., Maloshonok, N., Bettinger, E., and Kizilcec, R. F. (2020). Online education platforms scale college STEM instruction with equivalent learning outcomes at lower cost. Science. Advances 6:eaay5324. doi: 10.1126/sciadv.aay5324
Chowrira, S. G., Smith, K. M., Dubois, P. J., and Roll, I. (2019). DIY productive failure: boosting performance in a large undergraduate biology course. Npj Sci. Learn. 4:1. doi: 10.1038/s41539-019-0040-6
Darabi, A., Arrington, T. L., and Sayilir, E. (2018). Learning from failure: a meta-analysis of the empirical studies. Educ. Technol. Res. Dev. 66, 1–18. doi: 10.1007/s11423-018-9579-9
DeCaro, M. S., McClellan, D. K., Powe, A., Franco, D., Chastain, R. J., Hieb, J. L., et al. (2022). Exploring an online simulation before lecture improves undergraduate chemistry learning. Proceedings of the International Society of the Learning Sciences. International Society of the Learning Sciences.
DeCaro, M. S., and Rittle-Johnson, B. (2012). Exploring mathematics problems prepares children to learn from instruction. J. Exp. Child Psychol. 113, 552–568. doi: 10.1016/j.jecp.2012.06.009
Felder, R. M., and Brent, R. (2009). Active learning: an introduction. ASQ High. Educ. Brief 2, 1–5.
Freeman, S., Eddy, S. L., McDonough, M., Smith, M. K., Okoroafor, N., Jordt, H., et al. (2014). Active learning increases student performance in science, engineering, and mathematics. Proc. Natl. Acad. Sci., 8410–8415. doi: 10.1017/pnas.1319030111
Glogger-Frey, I., Fleischer, C., Grüny, L., Kappich, J., and Renkl, A. (2015). Inventing a solution and studying a worked solution prepare differently for learning from direct instruction. Learn. Instr. 39, 72–87. doi: 10.1016/j.learninstruc.2015.05.001
Haak, D. C., HilleRisLambers, J., Pitre, E., and Freeman, S. (2011). Increased structure and active learning reduce the achievement gap in introductory biology. Science 332, 1213–1216. doi: 10.1126/science.1204820
Hieb, J., DeCaro, M. S., and Chastain, R. J. (2021). “Work in Progress: Exploring before instruction using an online Geogebra™ activity in introductory engineering calculus” in Proceedings of the American Society for Engineering Education. American Society for Engineering Education.
Kapur, M. (2010). Productive failure in mathematical problem solving. Instr. Sci. 38, 523–550. doi: 10.1007/s11251-009-9093-x
Kapur, M. (2011). A further study of productive failure in mathematical problem solving: unpacking the design components. Instr. Sci. 39, 561–579. doi: 10.1007/s11251-010-9144-3
Kapur, M. (2016). Examining productive failure, productive success, unproductive failure, and unproductive success in learning. Educ. Psychol. 51, 289–299. doi: 10.1080/00461520.2016.1155457
Kapur, M., and Bielaczyc, K. (2012). Designing for productive failure. J. Learn. Sci. 21, 45–83. doi: 10.1080/10508406.2011.591717
Kapur, M., Hattie, J., Grossman, I., and Sinha, T. (2022). Fail, flip, fix, and feed–rethinking flipped learning: a review of meta-analyses and a subsequent meta-analysis. Front. Educ. 7:956416. doi: 10.3389/feduc.2022.956416
Lage, M. J., Platt, G. J., and Treglia, M. (2010). Inverting the classroom: a gateway to creating an inclusive learning environment. J. Econ. Educ. 31, 30–43. doi: 10.1080/00220480009596759
Lamnina, M., and Chase, C. C. (2019). Developing a thirst for knowledge: how uncertainty in the classroom influences curiosity, affect, learning, and transfer. Contemp. Educ. Psychol. 59:101785. doi: 10.1016/j.cedpsych.2019.101785
Loibl, K., Roll, I., and Rummel, N. (2017). Towards a theory of when and how problem solving followed by instruction supports learning. Educ. Psychol. Rev. 29, 693–715. doi: 10.1007/s10648-016-9379-x
Loibl, K., and Rummel, N. (2014). Knowing what you don’t know makes failure productive. Learn. Instr. 34, 74–85. doi: 10.1016/j.learninstruc.2014.08.004
McClellan, D. K., Chastain, R. J., and DeCaro, M. S. (2023). Enhancing learning from online video lectures: The impact of embedded learning prompts in an undergraduate physics lesson. Comput. Educ. 1–23. doi: 10.1007/s12528-023-09379-w
Mogavi, R. H., Zhao, Y., Haq, E. U., Hui, P., and Ma, X. (2021). “Student barriers to active learning in synchronous online classes: Characterization, reflections, and suggestions [paper presentation]” in Proceedings of the 8th ACM virtual conference on learning @ scale, Germany. Association for Computing Machinery.
Musunuru, K., Machanda, Z. P., Qiao, L., and Anderson, W. J. (2021). Randomized controlled studies comparing traditional lectures versus online modules. bio Rxiv. doi: 10.1101/2021.01.18.427113
Olsen, J. K., Faucon, L., and Dillenbourg, P. (2020). Transferring interactive activities in large lectures from face-to-face to online settings. Inform. Learn. Sci. 121, 559–567. doi: 10.1108/ILS-04-2020-0109
Prince, M. (2004). Does active learning work? A review of the research. J. Eng. Educ. 93, 223–231. doi: 10.1002/j.2168-9830.2004.tb00809.x
Rittle-Johnson, B., Siegler, R. S., and Alibali, M. W. (2001). Developing conceptual understanding and procedural skill in mathematics: an iterative process. J. Educ. Psychol. 93, 346–362. doi: 10.1037/0022-0663.93.2.346
Roelle, J., and Berthold, K. (2015). Effects of comparing contrasting cases on learning from subsequent explanations. Cogn. Instr. 33, 199–225. doi: 10.1080/07370008.2015.1063636
Roll, I., Holmes, N. G., Day, J., and Bonn, D. (2012). Evaluating metacognitive scaffolding in guided invention activities. Instr. Sci. 40, 691–710. doi: 10.1007/s11251-012-9208-7
Sandrone, S., and Schneider, L. D. (2020). Active and distance learning in neuroscience education. Neuron 106, 895–898. doi: 10.1016/j.neuron.2020.06.001
Sandrone, S., Scott, G., Anderson, W. J., and Musunuru, K. (2021). Active learning-based education for in-person and online learning. Cell Press 184, 1409–1414. doi: 10.1016/j.cell.2021.01.045
Schwartz, D. L., Chase, C. C., Oppezzo, M. A., and Chin, D. B. (2011). Practicing versus inventing with contrasting cases: the effects of telling first on learning and transfer. J. Educ. Psychol. 103, 759–775. doi: 10.1037/a0025140
Schwartz, D. L., Lindgren, R., and Lewis, S. (2009). “Constructivism in an age of non-constructivist assessments” in Constructivist instruction: Success or failure. eds. S. Tobias and T. M. Duffy (New York, NY: Routledge/Taylor & Francis Group), 34–61.
Sinha, T., and Kapur, M. (2021). When problem solving followed by instruction works: evidence for productive failure. Rev. Educ. Res. 91, 761–798. doi: 10.3102/00346543211019
Theobald, E. J., Hill, M. J., Tran, E., Agrawal, S., Nicole Arroyo, E., Behling, S., et al. (2020). Active learning narrows achievement gaps for underrepresented students in undergraduate science, technology, engineering, and math. Proc. Natl. Acad. Sci. U. S. A. 117, 6476–6483. doi: 10.1073/pnas.1916903117
Vaccani, J. P., Javidnia, H., and Humphrey-Murto, S. (2016). The effectiveness of webcast compared to live lectures as a teaching tool in medical school. Med. Teach. 38, 59–63. doi: 10.3109/0142159X.2014.970990
Venton, J. B., and Pompano, R. R. (2021). Strategies for enhancing remote student engagement through active learning. Anal. Bioanal. Chem. 413, 1507–1512. doi: 10.1007/s00216-021-03519-0
Weaver, J. P., Chastain, R. J., DeCaro, D. A., and DeCaro, M. S. (2018). Reverse the routine: problem solving before instruction improves conceptual knowledge in undergraduate physics. Contemp. Educ. Psychol. 52, 36–47. doi: 10.1016/j.cedpsych.2017.12.003
Wegner, E. (1998). Communities of practice: Learning, meaning, and identity. Cambridge, MA: Cambridge University Press.
Keywords: exploratory learning, productive failure, active learning, online learning, physics
Citation: DeCaro MS, Isaacs RA, Bego CR and Chastain RJ (2023) Bringing exploratory learning online: problem-solving before instruction improves remote undergraduate physics learning. Front. Educ. 8:1215975. doi: 10.3389/feduc.2023.1215975
Edited by:
Mary Ellen Wiltrout, Massachusetts Institute of Technology, United StatesReviewed by:
Milan Kubiatko, J. E. Purkyne University, CzechiaDaner Sun, The Education University of Hong Kong, Hong Kong SAR, China
Copyright © 2023 DeCaro, Isaacs, Bego and Chastain. This is an open-access article distributed under the terms of the Creative Commons Attribution License (CC BY). The use, distribution or reproduction in other forums is permitted, provided the original author(s) and the copyright owner(s) are credited and that the original publication in this journal is cited, in accordance with accepted academic practice. No use, distribution or reproduction is permitted which does not comply with these terms.
*Correspondence: Marci S. DeCaro, marci.decaro@louisville.edu