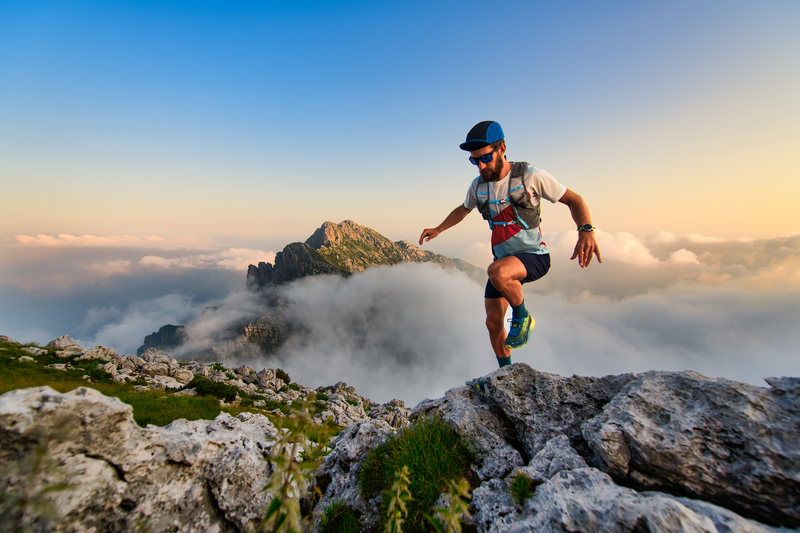
95% of researchers rate our articles as excellent or good
Learn more about the work of our research integrity team to safeguard the quality of each article we publish.
Find out more
HYPOTHESIS AND THEORY article
Front. Educ. , 01 August 2023
Sec. Educational Psychology
Volume 8 - 2023 | https://doi.org/10.3389/feduc.2023.1215510
This article is part of the Research Topic Enhanced Learning and Teaching via Neuroscience View all 10 articles
Recent advances in pedagogical research have called attention to the dynamic nature of the teaching and learning process in which the actors mutually influence one another. The understanding of how this works in the brain—the specialized neural networks related to this process—is often limited to neuroscientists but are slowly becoming available to other learning scientists, including teachers. A transdisciplinary approach combining the best information about observable teaching-learning processes from education with newer information from the neurosciences may aid in resolving fundamental questions in the learning process. Teachers’ professional formation and development is often structured in segmented topical ways (e.g., pedagogy, evaluation, planning, classroom management, social–emotional learning), to identify important content knowledge (e.g., art, reading, mathematics, STEM), or to appreciate life skills (e.g., collaboration, critical thinking, social–emotional learning). While important, knowledge about the brain, the organ responsible for learning, is typically absent from teacher education. This paper reexamines the evidence from neuroconstructivism and the hierarchy of learning trajectories and combines it with evidence from psychology and the ways humans interact during the teaching-learning process to suggest radical neuroconstructivism as a framework within which to organize teachers’ professional development. The radical neuroconstructivism framework may contribute to making the content knowledge of teachers’ continual professional development more visible.
The quality of education hinges on the quality of teachers (Barber and Mourshed, 2007; Engelbrecht and Ankiewicz, 2016; Boeren, 2019; Organisation for Economic Cooperation and Development, 2020). Teachers’ continual professional development (TCPD) covers a wide range of topics (e.g., pedagogy, evaluation, planning, classroom management, social–emotional learning), subject areas (e.g., art, reading, mathematics, STEM), life skills appreciation (e.g., collaboration, critical thinking, social–emotional learning), and should exists throughout one’s professional career (Sancar et al., 2021). There are few if any opportunities, however, for teachers to learn how these topics, subject areas, and life skills are supported by neural networks in the brain (Dubinsky et al., 2022), and fewer still about how to improve them (Peters et al., 2020). Understanding the neural underpinnings of knowledge building in the brain—or neuroconstructivism—may create useable knowledge for teachers.
Placing TCPD within the “messiness” of classrooms (Tokuhama-Espinosa, 2014) and the relevance of cultural contexts (Hammond, 2014) may also contribute to improved learning outcomes as it acknowledges the ways one’s learning is influenced by other actors. This dynamic exchange between students-to-students and students-to-teachers and vice versa influences what a learner takes from teaching and, consequently, changes what is learned (Bevilacqua et al., 2019). Recent research shows how individuals co-construct learning experiences (Vieluf and Klieme, 2023), which elevates the role of “others” in individual learning to the extent of deserving the label “radical” (Von Glasersfeld, 1995, 2013). Radical constructivism suggests that an individual’s ability to learn is changed by context. To unite neuroconstructivism with the dynamic exchange of learning between actors, we propose a new theory of radical neurocontructivism.
The transdisciplinary field of Mind, Brain, and Education is uniquely positioned to support research into the theory of radical neuroconstructivism as it encompasses micro-level research at the level of neurons, to consideration of individual genetic and epigenetics traits, to the individual in within classroom dynamics, and all the way to macro-level research that consider social and cultural influences on learning (see Figure 1).
Figure 1. Mind, brain, and education research on multiple data collection levels (Tokuhama-Espinosa, 2017).
Despite the growth of the International Mind, Brain, and Education Society founded in 2007, and the Neuroscience and Education Special Interest Group of the European Association for Research on Learning and Instruction founded in 2010, advancements in designing a curriculum for teachers’ professional development around concepts from neuroscience have been few and far between. To explore the potential contributions of radical neuroconstructivism theory to benefit teacher education, the first part of this paper defines and uses holonic thinking, a Greek word meaning something that is once a part and a whole. Holonic thinking is a newer conceptual framework to explain the relationships between the many elements in the educational process. This is followed by a brief historical overview of teachers’ professional development between the 1980s and today, which shows radical neuroconstructivism as a natural outgrowth of past advances. The second part of the paper offers an example of early childhood education in math and language using studies from neuroscience that are the puzzle pieces of learning trajectories in these two domains. The paper concludes by summarizing how the how (pedagogy), what (curriculum), and why (Mind, Brain, and Education science) of radical neuroconstructivism may improve teacher education (Figure 2).
Figure 2. The educator as a learning scientist (Tokuhama-Espinosa, 2021a,b). Used with permission of the author.
In one of the most famous philosophical psychological undertakings, Arthur Koestler’s The Ghost in the Machine (Koestler, 1967) observes that all things are both parts and wholes, which he labeled holons. “A holon, as Koestler devised the term, is an identifiable part of a system that has a unique identity yet is made up of sub-ordinate parts and in turn is part of a larger whole” (Edwards, 2003, para. 19). Later, Ken Wilber used holons to explain his All Quadrants, All Levels framework (AQAL), which showed the hierarchical nature of each part and whole (Wilber, 2001). This allowed Gallifa (2019, p. 15) to describe “integral thinking” using a holonic approach to explain the complex nature of all learning through relationships build on hierarchies.
Based on Koestler’s definition, everything in the natural world is a holon. A child, for example, is a holon as he is a whole on his own, but he is also a part of a family. A leaf is a holon because it is a whole unto itself, but it can also be a part of a tree. A person’s brain is a holon as it is a whole entity on its own, but also part of the person’s body. Holonic thinking embraces the idea that not only can everything be a part of something bigger, but each holon can be broken down into smaller parts as well. The child is made up of body parts, which in turn are made of flesh, blood, bones, and muscles (that can themselves also be broken down into ever smaller parts). The leaf can also be broken down into fibers and chemicals, which in turn can also be broken down further. A person’s brain can also be broken down into different types of cells, proteins, and so on. In short, everything is a holon, a whole on its own and a part of bigger things that can also be broken down into smaller elements.
Holonic thinking offers a new lens through which to view challenges in teaching and learning. Education is a holon. It can be considered a part of the Learning Sciences, as well as an academic field on its own, and can be broken down into smaller elements. Teacher education is also a holon. It is part of Education, but can also be broken down into elements, such as how to teach [pedagogy and didactics (methodology, activities and strategies)] and what to teach (content, curriculum, learning how to learn). The ability to evaluate and give good feedback; how to use technology appropriately; what is needed to create inclusive classrooms; how to differentiate; the cultivation of social–emotional skills to nurture oneself and others, among other elements, are all holons and sub-elements of teachers’ continual education. Both pedagogy and curriculum are parts and wholes, as are all of the other topics and skills that contribute to good teaching and successful learning.
Merging the how (e.g., pedagogy) with the what (e.g., curriculum) of the teaching-learning dynamic yields teachers’ pedagogical content knowledge (TPCK) (Gess-Newsome, 2013). In contrast to general best practice teaching, TPCK displays a more nuanced understanding of the interventions that are most appropriate given a specific subject matter and age group. For example, the specialized knowledge teachers must have to anticipate the errors and knowledge of how to correct them differs when teaching math to 3rd graders versus teaching English to high school students. Shulman’s (1987) seminal work in this field elevated the mechanistic approach of teaching from a simple delivery system of facts to include a more subtle and precise knowledge base required of teachers both of their subject matter and for the correct pedagogical interventions that can be used to reach educational objectives.
Technology was added to TPCK around 2004 and yielded what many now call the TPACK (Technology, Pedagogy, and Content Knowledge) Model (Mishra and Koehler, 2006). Technology, and specifically educational technology tools, both aid traditional learning as in the correction of objective assessments (e.g., multiple-choice question quizzes), for instance, as well as force society to rethink the role of traditional educational design. If the only goal of schooling is to gain knowledge and knowledge can be learned using mobile devices, then why go to school at all?, one might ask. Such reflections help elevate the expectations of schooling and also change the expectations of what teachers’ roles are within those schools. For example, the existence of technology that provides content knowledge in a subject area like math reduces the expectation that teachers use time in class reciting math facts (knowledge) (Donahoe et al., 2019) and elevates expectations that they now use their time applying the use of that information (skills), or how to cultivate values around the information, such as learning how to think like a mathematician (attitudes) (Seufert et al., 2021). Some argue that the role of school was never about transmitting factual knowledge, but rather to nurturing of the whole child (Perkins, 2009) by considering his context, likes and dislikes, and particular learning needs (Moon et al., 2020), and that technology can give teachers more time to personalize the teaching-learning experience (Schmid et al., 2022).
Technology has also introduced Artificial Intelligence and machine learning into educational processes (e.g., ChatGPT) which has forced teachers to pivot in ways that will likely change the teaching-learning dynamic forever. Whereas in the past students were judged by their ability to produce answers on standardized tests (Cunningham, 2018), large language models like ChatGPT will force students to come up with better questions relevant to their unique contexts (Lund et al., 2023). Technology can serve to make some types of learning more personalized and tailored to individual needs, enhancing learning outcomes. To learn to leverage these new technologies and to participate in their design, teachers will necessarily also need to learn more about the ways that both artificial intelligence and real human intelligence work. This new need has catalyzed a renewed interest in the learning sciences, specifically Mind, Brain, and Education (MBE) science.
In viewing the teacher as a learning scientist one can combine pedagogy, content, technology and MBE to suggest a new approach to teacher formation (Tokuhama-Espinosa, 2021a,b). Mind, Brain, and Education science adds a why to the how and what of teaching as it explains the reasoning behind certain teaching interventions (pedagogy: how) and content (curriculum: what).
As the newest addition to teachers’ basic skills, Mind, Brain, and Education science and the International Mind, Brain, and Education Society (IMBES) were founded in 2007 to help practitioners understand how the brain learns in order to verify the best teaching methods to reach the most students (Tokuhama-Espinosa, 2010). MBE has proven implications for pedagogical interventions (Tokuhama-Espinosa, 2014, 2021a,b; Wilson and Conyers, 2020) as well as has made inroads in curriculum (Larrison, 2013). According to Dubinsky et al. (2022, p. 267), “the foundational contributions from neuroscience regarding how learning occurs in the brain reside within one of Shulman’s seven components of teacher knowledge (Shulman, 1987, p. 8), Knowledge of Students… teachers must also (and increasingly) know what happens inside students’ brains.” It has been suggested that “knowledge of learners and their characteristics” (Shulman, 1987, p. 8) should now include clarity about how the brain understands concepts in domain-specific areas (e.g., Hawes and Ansari, 2020), leverages emotions for better cognition (e.g., Li et al., 2020), and co-constructs meaning making in group situations (Immordino-Yang et al., 2019).
Holonic thinking can be used to reframe the way we view teacher education and our understanding about the teaching and learning process. Both philosophers (e.g., Procter, 2011) and neuroscientists (e.g., Lamme, 2006) believe learning is based on fundamental building blocks of knowledge, which permit the construction of increasingly complex thinking (Hernández Armenta et al., 2019). Thinking is a holon that is part of the learning process itself, and it can be broken down into smaller and smaller parts. Disaggregating this thinking process into its smaller parts allows for a more precise understanding of all of the elements that contribute to how people learn both in classroom settings and in the world more broadly. To construct new learning, people build on previous knowledge using the foundation of what have been called core notions (Bada, 2015). Some of the building blocks of learning are explained in neuroscientific studies, but few teachers benefit from this information in their initial teacher training or in continual professional development (Deans for Impact, 2015). Furthermore, the majority of the contributions from neuroscience to education are related to pedagogy, not curriculum. In a review of all the articles from the Mind, Brain, and Education journal 2007–2018, just 24 of 312, less than 1%, related to curriculum (Nouri et al., 2022, pp. 58–59). This confirms Dubinsky and colleagues’ belief that “Neuroscience professional development provides neuroscience principles that teachers can learn and apply to distinguish among pedagogical choices, plan lessons, guide in-the-moment classroom decisions, and inform the views of students. Neuroscience does not directly invent new pedagogies. Rather, knowledge of neuroscience guides teachers in choosing appropriate pedagogies, pragmatically informing teaching” (Dubinsky et al., 2022, p. 267).
One potential way to extend MBE into Education both pedagogically and through curriculum is through radical neuroconstructivism. Radical neuroconstructivism is an as-of-yet untested theoretical framework for understanding the teaching-learning dynamic. It is difficult to prove as it rests against the backdrop of a student’s prior experiences and contexts which vary greatly. It also depends, however, on universal building blocks, meaning some generalizations relating to all humans can be posited.
Constructivism has been used successfully as a framework to explain the way the mind orders the hierarchy of learning concepts, beginning with an approach from developmental psychology and spilling into education (Piaget, 1923). To construct new learning, people build on previous knowledge using the foundation of these core notions (Solis-Stovall, 2020). The individual does not live in the world alone, however, so many researchers, especially those in social learning theory (e.g., Bandura, 1977), raise the importance of constructivism within environments and social contexts. When the environment and the role of others is also incorporated into the constructivist learning model, this is called radical constructivism (Von Glasersfeld, 1995, 2013). Ernst Von Glaserfeld used the constructivist ideas suggested by Vico (1710), Ceccato (1964/1966), and Piaget (1968) to which he added on the cyclical, iterative processes that occurs as people try “to order the as such amorphous flow of experience by establishing repeatable experiences and relatively reliable relations between them,” (Von Glasersfeld, 1984, p. 5). Von Glaserfeld’s ideas were firmly grounded in strong philosophical roots but they carried over naturally into the teaching and learning environment as the means by which societies devise formal education. Von Glasersfeld (1984, p. 20) uses “radical” to emphasize the relationship of a person to reality and explains that rather than a “picture-like (iconic) correspondence or match, radical constructivism sees it as an adaptation in the functional sense.” This means that the very contact with others or with new information would change a person’s understanding of it. An idea in one’s head about how to approach a problem, a work of art, or a piece of literature, is changed by the simple act of articulating it out loud for another person. While words facilitate thinking, they are not the same thing as thinking. One’s understanding of one’s own ideas require no explanation to one’s self; once put “out in the world” one must modify the choice of words to meet others’ levels of understanding. Furthermore, teachers must modify this language use to meet a variety of learners’ needs in the same setting. This means that what is thought cannot always be articulated clearly to others, resulting in the voice in our heads being different than the one we hear as we speak (LaValley, 2022).
A second aspect of the “radical” nature of thinking and learning relates to the individual themselves in time. As all new learning passes through the filter of prior experience (Tokuhama-Espinosa, 2008), and the older we get the more experiences we have, our interpretation of the world becomes more colored by what we already know. For example, reading The Diary of Anne Frank at age 13 is different at age 18 or 30 or 50, not because the book is different, but you and your context are different.
Radical constructivism emphasizes the role that others can play in influencing how an individual thinks about information. A person may have one kind of idea in their mind, but when they articulate this in words to another person, the idea changes Hitchcock, (2018). And by listening to the response of the other to the idea, the idea is again changed. This dynamic process of idea transformation is what turns constructivism within the individual into a social exchange in the world (De Soto, 2022). Teachers know that classroom exchanges between students and with themselves modify the way they think about information.
After radical constructivism, a newer concept from neuroscience, neuroconstructivism, took hold. Neuroconstructivism, like its predecessor constructivism, requires that lower or base level concepts be learned before more complex ideas can be built upon them and that this occurs in a neurophysiological way structuring primary networks before secondary ones can be scaffolded upon them. Dekker and Karmiloff-Smith (2011) were some of the first to suggest that the combination of behavioral studies, neuroimaging, and genetics research in both typical and atypical populations pointed to the existence of neuroconstructivism. By Karmiloff-Smith et al. (2018) were able to formulate a new theory of human development based on neuroconstructivism.
“Neuroconstructivism” is a term used to explain the physical scaffolding of core notions and conceptual knowledge (Broadbent and Mareschal, 2019) “that influence the emergence of mental representations in postnatal development,” (Westermann et al., 2007, p. 75). The brain makes basic neural connections, then successively more complex ones based on experiences which are unique to the individual (Mareschal et al., 2007; Westermann et al., 2007, 2011; Karmiloff-Smith et al., 2018). As Westermann and colleagues pointed out in their seminal article Neuroconstructivism (Westermann et al., 2007, p. 75), “Cognitive development is explained as emerging from the experience-dependent development of neural structures supporting mental representations.” The scaffolding of conceptual understanding permits the construction of neural networks that eventually become the learning manifested in observable behavior, such as the ability to read a story or to do a math problem. Earlier studies in neuroconstructivism showed that when certain fundamental networks were missing, a child was unable to perform certain tasks and future tasks that relied on that initial task. For example, a child can scaffold a new understanding of subtraction upon the basis of addition. If the child knows how to add well, then learning to subtract takes relatively few steps to master. However, many children have gaps in core notions in mathematics and because they have missing conceptual knowledge in addition, they are unable to easily learn subtraction. This is not only true for math but for every other subject taught in school or experienced in the real world.
In 2019, Tokuhama-Espinosa suggested that this promising new idea could be merged with Von Glaserfeld’s thinking and coined the term radical neuroconstructivism. Building off both the dynamic, iterative exchange of an individual with his or her surroundings, and the constant co-construction of neural networks of the brain’s design and on a natural hierarchy of conceptual knowledge, this paper suggests that radical neuroconstructivism can potentially create the framework to explain how people learn.
This paper suggests that the radical aspect of radical neuroconstructivism involves “meaning making,” made popular thanks to Neil Postman and Charles Weingartner’s chapter in Teaching as a Subversive Activity (Postman and Weingartner, 1969). In their work they point out that “meaning making also forces us to focus on the individuality and the uniqueness of the meaning maker,” (Postman and Weingartner, 1969, p. 91). This was a shift from prior teaching and learning models in which school subjects (math, language, art, history, and so on) were meant to be learned by all individuals in the same way without much consideration for the variability among students. Postman and Weingatner valued that the way people understand their world and make meaning depends to a great extent on what they already know and how they have already habituated responses to certain contexts and stimuli. When one begins with the meaning makers (students) in mind, and the many differences they each have, it becomes clear why the learning processes in school do not always go to plan; the individuality of the learner changes the outcomes.
To make meaning of one’s world, an individual first perceives the environment through the senses, as Aristotle suggested 2,500 years ago (Caston, 2020). This sense perception is perceived and interpreted in the brain by comparing what is known from prior experiences to the incoming information from the outside world (Tokuhama-Espinosa, 2008). The prior experiences a person can have are grounded in both formal and informal learning, as well as based on life experience. Life experiences and a person’s environment also includes one’s culture, which like all social environments, influences learning (Gay, 2018). People then construct meaning by taking the new information that is being perceived in the brain and comparing it with what they already know from prior experiences including their cultures and contexts. Contexts and cultures include contact with other people in settings like schools and with teachers and students.
Complementary to Postman and Weingartner (1969) work is a newer interpretation of meaning making from Mary Helen Immordino-Yang’s lab. She suggests knowing how others feel (empathy) and think (mentalizing), then comparing that to one’s own thinking and feeling, helps derive meaning (Immordino-Yang and Knecht, 2020). That is, seeing how others react in situations and comparing that to how one would act themselves in the same situation helps people make meaning out of the world: “Radical neuroconstructivism changes based on the student-teacher and student–student dynamics, and other human exchanges converging with what the student already knew about the information mediated by the pedagogical choices of the instructor,” (Nouri et al., 2022, p. 115). The interaction with the outside world, compared with internal knowledge and memories, is modified by other students and by the teacher, making it “radical” as compared to static (Nouri et al., 2022). Radical neuroconstructivism explains why different students react differently to teaching strategies and activities. The unique reaction of each student to what the teacher and other students do in the classroom changes the way the student thinks about the information, and ultimately how he or she learns.
Students come to class with their past experiences, their cultures, and their genetic profiles which then interacts with exchanges they have with other students, with their teacher, and the teacher’s choice of pedagogies. The intricate interaction between this large number of complex variables results in learning. As shared in Crossing Mind, Brain, and Education Boundaries we contend:
Rather than a simple “Teach A-Learn A” scenario, MBE (Mind, Brain, and Education) teachers appreciate that learning is complex, and influenced by multiple factors. MBE practitioners understand that:
(a) students come to class on an uneven playing field due to genetic inheritance;
(b) students do not share the same prior experiences;
(c) what the student already knows influences how they learn;
(d) knowledge, skills, and attitudes influence learning;
(e) the student’s relationship with the other learners influences learning;
(f) the student’s relationship with the teacher influences learning;
(g) the teacher’s execution of the methodology, strategy, or activity influences learning;
(h) the learner’s self-perception in the class context/environment influences learning;
(i) what else is vying for the student’s attention can influence learning.
These different actors, actions, reactions, and interactions can all influence learning outcomes (Nouri et al., 2022, pp. 115–116).
Taken as a whole, radical constructivism suggests that the individual’s conceptual knowledge of the world is shaped by what he or she already knows, and how, when, why, and by and with whom the stimuli occurs.
The concept of core notions has been posited in philosophy (e.g., Schaffer et al., 2009), demonstrated in cognitive psychology (e.g., Tuominen and Kallio, 2020), and imaged in neuroscience (e.g., Skerry and Saxe, 2016). Core notions are pre-requisite knowledge at each stage of the learning process. Furthermore, each progressive level of knowledge has its own core notions and depends on those that proceed them (Sporns, 2022); counting has different core notions than calculus, for example, and calculus depends on counting. Similarly, higher order language depends on the lower notions that sustain them; the core notions within word choice are different from higher order language notions such as metaphorical thinking, for example. Metaphorical thinking, in turn, depends on word choice (Black, 1962). In the best-case scenario, the curriculum or order of subjects a child learns, should first introduce fundamental core notions and once mastered, advance to subsequently more complex notions.
Countries around the world use the evaluation of math and language as proxies for intelligence (Tokuhama-Espinosa, 2019) often in combination with more complex tools that depend on them, such as reasoning (Flanagan and McDonough, 2018). Both math and language are comprised of “core notions” or fundamental building blocks of knowledge, which permit the construction of learning and progressively more complex thinking (Hernández Armenta et al., 2019; Solis-Stovall, 2020). Examples of “core notions” include any fundamental or pre-requisite knowledge needed to complete a higher order task and are characterized by thinking states rather than process memorization. For example, zero (“0”) is a complex notion, which, if misunderstood, can lead to problems with understanding “ones” and “tens” and eventually decimals, negative integers, and other key notions in mathematics (Hansen et al., 2020). In a second example, the core notion of a mental number line can be used to see addition or subtraction problems inside one’s head (Dehaene, 2003; Haman and Lipowska, 2021). Problems like “1 + 2 = 3” are visualized in the mind’s eye and such visualization is vital to developing efficient, accurate and speedy arithmetic skills (Sari and Olkun, 2020). An understanding of zero combined with a mental number line permits a visualization and understanding of negative numbers, and eventually the addition and subtraction of both positive and negative integers (Vest and Alibali, 2021). If zero or the mental number line are not learned by children, they will be unsuccessful in early math, and consequently higher math. Missing core notions in children are a primary reason kids “hate math” (Liu, 2016); the inability of teachers to identify these gaps is exacerbated by the fact that teachers themselves often have missing core notions (Ball, 2017). Missing core notions in teacher knowledge are also responsible for poor math and language learning by their students (Loch et al., 2015), signifying a systemic problem.
Unfortunately, many students advance through the education system with progressively complex missing core notions (Rist, 2017) for which teachers are unprepared. Bartelet et al. (2014, p. 657) noted that learning difficulties in math can spring from at least six different origins: “(a) a weak mental number line group, (b) weak ANS (Approximate Number System) group, (c) spatial difficulties group, (d) access deficit group, (e) no numerical cognitive deficit group and (f) a garden-variety group,” suggesting that a more nuanced look at both gaps in mathematical instruction as well as diagnosis of mathematical sub-types of errors is necessary to help students achieve. If teachers do not know about core notions or their hierarchy in brains, they cannot easily identify the types of errors being committed by students. This is an example of what Dubinsky and colleagues meant by improving teacher Knowledge of Students “and what happens inside their brains,” (Dubinsky et al., 2022, p. 267).
Research into language has also identified many core notions which can go unattended in early childhood education. One area that has received a lot of attention is vocabulary. Educational research has demonstrated for years that rich, age-appropriate vocabulary lays the foundation for complex thinking (Hirsh-Pasek and Golinkoff, 2003) and that poor vocabulary is correlated with academic failure (Baker, 1995). Hart and Risley (2003) “The Early Catastrophe,” showed a “30 million word gap by the age of 3” for children from lower social economic status homes due to less exposure to rich conversational exchanges, fewer books in the home, and parental knowledge of language development (Johnson et al., 2017). Researchers warn that “denying the existence of the 30-million-word gap” suffered by underserved children “has serious consequences” (Golinkoff et al., 2019, p. 985). Therefore, explicit vocabulary instruction is a part of several early childhood training programs, but not all. Despite neuroscientific evidence showing that “children’s conversational exposure is associated with language related brain function,” (Romeo et al., 2018, p. 700) that do not exist without human conversation, other researchers have pointed out that “talk alone will not close the 30-million word gap” (Wasik and Hindman, 2015, p. 50) and that meaningful interactions with language use in varied contexts are necessary to fill in the gap. Appropriate word use in the right context with increasingly complex patterns is fundamental to language development but not all early childhood education programs emphasize this and not all teachers know vocabulary is a fundamental building block in learning.
Other core notions in language development relate to normal speech patterns, including the grammar and syntax that is acceptable in local cultural contexts. While all humans learn to speak from an innate language sense (Chomsky, 2000; Pinker, 2009), the parameters of acceptable speech differs by country (e.g., British to American), region (e.g., Hawaii to Texas), district (e.g., English in the Bronx versus English in upper Manhattan), and even neighborhood (e.g., East Los Angeles versus West). Furthermore, the way humans speak differs greatly from how they write, especially from informal to academic contexts (Chafe, 1985). This puts children whose core notions of grammar and syntax that differ from standard English in school at an academic disadvantage from the start (Au, 2009). When the school’s standard English differs greatly from the home language, students first need to learn the “foreign” language of school before they can be successful in other subjects. This sets up many for failure. Many find learning the school language a burden and decide they are not “cut out for school,” and/or “hate reading,” (Hale and Crowe, 2001), and too many drop out (Rumberger and Lim, 2008) for this reason. This paper proposes that teaching core notions in language in a more orderly trajectory may change students’ negative attitudes toward education as the neuroconstruction of core notions in an orderly way may ease the path by creating a more solid early learning foundation.
In this paper it is suggested that a deeper and better understanding of the radical neuroconstructivist building blocks of cognition may permit a more precise and orderly introduction of skills that would be coherent with the brain’s natural progression from lower-to-higher-level knowledge. This would improve the design of the curriculum, allowing more children to succeed.
While psychology has contributed to educational best practice for over 100 years (Berliner and Calfee, 2014), contributions from neuroscience have only recently been regularly incorporated into teacher professional development (Deans for Impact, 2015). Thanks to neuroscientific insights, there have been improvements in pedagogy, didactics, strategies, activities, and methodologies for learning at all levels of education (K-16). This is especially true of new knowledge about the dynamic exchange between cognition and affect (e.g., Immordino-Yang, 2015), meaning making (e.g., Zittoun and Brinkmann, 2012) at the crossroads of culture and cognition (Rawlings and Childress, 2021), and the importance of student-teacher relationships (e.g., Hattie and Zierer, 2017).
While how to teach has benefitted greatly from neuroscientific insights, what to teach has received less attention; the promise of neuroscientific insights into shaping the design of curriculum, such as in early literacy or math learning, is an underexplored area for educators. A key impediment to the use of neuroscientific knowledge in education is that the puzzle as a whole has not been constructed using all of the parts that are available.
The second part of this paper uses the examples of early years language and math to explain the holonic thinking from Neuroscience, Psychology and Education that can lead to a better neuroconstructivist curriculum design for schools. Education and Psychology have helped construct a relatively orderly curriculum (Tokuhama-Espinosa, 2019), which takes into consideration human variability (e.g., Mezirow, 2018). It is possible that additional evidence from Neuroscience can bring a more nuanced understanding of typical gaps in notions that children may experience. We propose that the ability to diagnose missing core notions earlier will allow more timely and accurate interventions in early childhood education.
Learning depends on the quantity, quality, and timing of exposure to learning objectives (Paolini, 2015). The literature suggests the earlier an academic competency is introduced to a learner, and the stronger its subsequent constructivist development, the more likely a positive learning outcome in that competency (Bakken et al., 2017) due to the quantity and quality of exposure. The literature also suggests that quality experiences at pre-school benefits both kids who had enriching home experiences and those who did not (Fuson et al., 2015). Klein and Starkey’s research showed that a broad socioeconomic gap in informational mathematical knowledge was present at the beginning of the pre-kindergarten year. This gap included not just numerical concepts and arithmetic reasoning, but also spatial concepts and geometric reasoning, knowledge of patterns, and nonstandard measurement (Klein and Starkey, 2004). One theory for this occurrence relates to the kinds of play experienced by different socio-economic groups (Missall et al., 2015). This means some kids arrive at school with missing core notions as compared with their peers due to the contexts in which they were raised. Quantity, quality, and timing are aided by exposure to core notions in a logical order which strengthens neural pathways for future learning (Karmiloff-Smith, 2009; Galván, 2010). Nowhere is this more evident than in research on early language development and early math.
The stimulation of language development (i.e., vocabulary, correct word order, social cues for interaction, and so on) begins in the home with the family and is generally developed further in regular school settings by trained educational professionals. Similarly, pre-numeracy skills (ordinality as parents count a child’s fingers and toes out loud; magnitude as he is given “more” or “less” of an object; symbolic numeric representation as he blows out the candles on a birthday cake, and so on) aid in the development of a child’s number sense (Dehaene, 2011) and are cultivated in a similar pattern (Campbell, 2014) through adult-child interaction. High-quality early childhood education can play an important role in the effective development of early academic skills development (Campbell et al., 2012) but requires a home (parent)-school (teacher) partnership with a shared plan (Missall et al., 2015). We suggest that by disaggregating math and language into sub-skills in a neuroconstructivist trajectory for mastery, we may potentially improve the diagnosis of problems and aid in the selection of more accurate remediating activities with the goal of ensuring all children have a successful start to school.
It is only since the turn of the century that neuroimaging studies have offered definitive proof of the changes in the brain during infant learning. This includes cognitive development such as typical growth rate, myelination, top-down modulation, and changes in cortical hubs, (Deoni et al., 2011; Fransson et al., 2011; Holland et al., 2014; Dempsey et al., 2015; Emberson et al., 2015). While teachers and parents generally understand that infants learn at an astounding rate, few are aware that infants have a preverbal early number sense that permits them to estimate quantities, gauge relative size and judge spatial orientation (Dehaene, 2011). One way to make these ideas clearer to parents and teachers is by showing neuroconstructivist studies alongside more familiar learning trajectories shared by pediatricians (Morris et al., 2020). To do this, it is important to update basic professional knowledge in the learning sciences for both math and language.
Understanding the neural networks of learning requires holonic thinking in which the smallest of parts are placed in context with their larger wholes. Between 2013 and 2023 we reviewed over 1,000 studies on early math and literacy and sought to create a taxonomy of early learning using their content. Initially, research was limited to domain-specific studies that looked at language and mathematical networks in the brain in young children. Domain-specific networks overlap significantly between language and math (Caravolas et al., 2012), and include pathways for symbols, patterns, order, relationships, and categories (Tokuhama-Espinosa, 2019). It soon became apparent, however, that while learning to read or do math involves domain-specific areas, learning also depends heavily on the general cognitive abilities of memory, attention, and executive functions (specifically inhibitory control, cognitive flexibility, and working memory), and in fact, without well-functioning general cognition, it was all but impossible to have domain specific instruction.
In addition to domain-specific networks and general cognitive networks, the literature also identified numerous studies related to the context in which a person learns. A learner’s relationships with others in the class and with the teacher influence learning (Frey et al., 2019), as does the student’s self-esteem and belief in him or herself to learn (Agir, 2019). Motivation is also part and parcel of the learner context (Ahn et al., 2019), and one’s awareness of the impact their social contexts, including culture, has an important influence on one’s ability to learn in a given classroom setting (Osher et al., 2020). The literature clearly shows the uneven playing fields upon which different children begin their lives. The risk and protective factors of family (parents’ education, SES, marital status), homes (homelessness, proximity to parks and libraries, daycare options), as well as the impact of culture and social contagion on well-being influence learning.
Finally, there were also several studies concerned with the physiological sensory networks related to the senses, specifically hearing, vision, and touch. Well-functioning sensory systems influence learning. While there were fewer studies about the role of gustatory and taste influence on learning, a student’s ability to see, hear, and learn through touch or haptic knowledge (Connolly, 2019) was vital to every learning encounter. Vision and hearing tests are standard procedure in many early childhood education programs, but not in all (Oosthuizen et al., 2023). This suggests 16 neural networks divided into four categories explained below (Figure 3).
The four categories have a total of 16 distinct neural networks within them, and those networks sub-divide into numerous pathways, which we define as core notions in domain areas such as math (Table 1) and language (Table 2). For the purposes of this paper, the neural pathways are considered “distinct” if one or more brain areas is different. For example, auditory working memory and visual working memory differ in the sensory input but not in memory areas and are treated as distinct networks (Figure 3).
Table 1. Examples of differences in the math literature between educational curriculum and neuroconstructivist design.
Table 2. Examples of differences in the language literature between educational curriculum and neuroconstructivist design.
The concept of “learning trajectories” (Gorard, 2006) is based on research into “hierarchies of skills” (Kuhn et al., 2000) and the general concept of constructivism proposed in the mid-1900s in which basic concepts are established before higher-order thinking occurs (Piaget, 1967). We generally presume that the curriculum structure of a country, state, or district should order the information we consider valuable to teach into the right trajectory so that students can logically advance from one concept to another. Curriculum structures around the world are surprisingly similar in terms of subject area content. The same subjects are taught all around the world at roughly the same time (Tokuhama-Espinosa, 2019), which allows for international comparative studies like TIMMS (Trends in International Mathematics and Science Study) and PIRLS (Progress in International Reading Literacy Study). Independent of country values, social economic status, culture, public-private-parochial status, political inclination, age group, and rural–urban status, all school systems, large and small, teach math and language. Language and mathematics are cornerstones of all educational programs worldwide (Pinar, 2013), and are vital to both an individual’s success as well as country competitiveness (Organisation for Economic Cooperation and Development, 2021). Among countries that conduct national exams, these are the only two universally tested subject areas due to the foundations they lay for other academic fields (Martin and Mullis, 2013), including history, art, the natural, social, computer, and hard sciences. Despite their importance, even within-country studies show there is no consensus on the best ways to teach core subjects such as math and language.
Constructivism can explain why some learning goals are not met. As mentioned earlier, a child cannot learn subtraction (learning goal) if he does not understand addition (pre-requisite knowledge). To be successful in basic arithmetic, he will first need to understand everything underpinning the concept of addition, and then make his way to the higher-order skill of subtraction, which involves dozens of core notions. If any one of the pre-requisite skills laid out in the hierarchy is not developed properly, the child will not be unable to master the new knowledge upon which it is based (Vergnaud, 1982). It is important to acknowledge that some children will learn to mechanically identify the pattern of subtraction questions and appear to dominate that skill, but in reality, they will simply be using extended working memory and knowledge of patterns to feign knowledge (Ball, 2017). True understanding means the learner can comprehend, identify, explain, use, and transfer knowledge as evidenced by creating their own problems correctly (Ringel and Springer, 1980).
Math, like all subject area, has four categories of networks were sub-divided by 16 neural networks. In math, we have further divided the networks into smaller parts—core notions—or neural pathways. In our review of the literature on the neural correlates of math, we have identified over 130 distinct pathways (Table 1), which can be observed in over 180 behaviors related to mathematical development. For example, the observable, visible behavior of counting can be observed in a classroom as the student counts on his fingers, counts objects, sings a song about counting, labels number symbols on a number line, among dozens of other activities. The invisible neural pathways involved in counting include decoding, discrimination and enumeration; distance and congruity; finger counting; inhibitory control and visual processing; number versus non-number symbols, among others (Table 1). In both math and language there are more observable behaviors than invisible networks, suggesting that the same networks sub-serve more than one behavior.
The main ways neuroscience can contribute to educational practices is by (a) assuring all neural pathways are stimulated through a variety of activities so that (b) all sub-skills and prerequisite knowledge are learned. This can be done if (c) core notions are approached in an orderly, hierarchical way. Additional benefits include the ability to (d) identify missing core notions early, therefore (e) making teaching interventions more precise, which would prevent children from school failure.
Table 1 is not exhaustive, and offers just a sampling of possible core notions, some of which have additional sub-elements.
Other evidence shows how the brain learns to code mathematical symbols and to distinguish between “3,” “three,” and “***” in a triple code (Dehaene, 1992; Dehaene and Cohen, 1995; Dehaene et al., 2003; Schmithorst and Brown, 2004; Klein et al., 2014), and estimate magnitude (i.e., Lourenco and Longo, 2011; Notebaert et al., 2011; Linsen et al., 2015; Lyons and Ansari, 2015). The brain also rotates shapes (i.e., Harris and Miniussi, 2003; Frick et al., 2013; Thompson et al., 2013; Bruce and Hawes, 2015), and understands the role and meaning of place value (i.e., Butterworth et al., 2011; Ferguson, 2015; Lambert and Moeller, 2019).
Yet other research clarifies the neural networks related to the role of fixed sequence (Grafton et al., 1995; Orban et al., 2011; Kidd et al., 2012; Pariyadath et al., 2012), and how the brain determines a general sense of numerosity (i.e., Piazza et al., 2004, 2006; Xu et al., 2005; Domahs et al., 2010; Anobile et al., 2013). These overlap but are distinct from neural networks related to approximations, estimations (Gilmore et al., 2014; Kibbe and Feigenson, 2015), and equivalencies (Mix, 1999; Hunt, 2011; Price et al., 2013; Chesney et al., 2014). There is also extensive work describing the brain and how it comprehends arithmetic, including division (i.e., LeFevre and Morris, 1999; Fehr et al., 2007; Grabner et al., 2009; Ischebeck et al., 2009; Andres et al., 2011; Rosenberg-Lee et al., 2011; Venneri and Semenza, 2011; Bugden et al., 2012), and grasps proportions (i.e., Sophian, 2000; Jacob et al., 2012).
Teachers can turn this list of neural pathways for math into useable knowledge in three ways. First and foremost, teachers can embrace the complexity of the brain and the sheer number of pathways involved in learning and resisting simplistic formulas for teaching and learning. Second, teachers can learn how observable behavior maps onto different types of neural networks which will help them better diagnose learning problems or gaps in student knowledge. And third, by understanding that different neural pathways are stimulated by different classroom and life experiences, they can select more efficient and effective learning interventions.
Learning trajectories in language are similar to those found in math. In language, the four categories of networks were sub-divided by 16 neural networks that sub-divided into over 90 neural pathways. When matched with the educational literature, there were over 171 observable behaviors related to early language development.
To devise elements for the educational curriculum (left column in Table 2) studies from public policy, pediatrics, and literacy were combined. These studies span from the role of parents in pre-literacy development as correlated with social-economic status (Fernald et al., 2013), racial disparity (Hoff, 2013), current practices in nursery schools around the world (Halden et al., 2011); and the ways that literacy parallels other milestones in growth (Hoff, 2009). Furthermore, there is documentation of the natural ordering of language skills in children 0–6 (Luinge et al., 2006) described as a natural hierarchy of pre-literacy skills. Literacy understanding from the contributions made by research from second language learners (e.g., Kuhl, 2011) as well as that from language learning delays caused by congenital defects and in cases of autism (McDuffie and Haebig, 2013) have also highlighted the core notions underpinning successful language acquisition. As with Tables 1, 2 right-hand column is comprised of a representative sampling of the various sub-skills or core notions needed to achieve the educational curriculum indicated in the left column.
This sampling of the many pathways found within the networks makes the precision of their activation more targeted than general guidelines found in education. For example, educators often talk about “language problems,” whereas a neuroscientist might speak about the precise problem of semantic memory, non-letter symbols used in reading, or the way that prosody influences meaning. Educators can learn from neuroscientists as the more precise the diagnosis, the better, more accurate the cure. That is, if a teacher know the “language problem” is one of symbol-to-sound (phoneme) difficulties, they will use a different intervention than if the problem is one of semantic retrieval.
The domain-specific areas of math and language were subdivided into (a) innate sense (i.e., innate number sense; innate language sense), (b) symbols, (c) patterns, (d) order and (e) categories, and (f) relationships.
One way to use the terminology from Education and Neuroscience together is in Figure 4.
To display a transdisciplinary understanding of early math and pre-literacy, it is necessary to travel from visible behavior to invisible neural networks, as seen in Figure 5.
The domain specific neural networks for math and language are important when students encounter problems limited to those subject areas. If the student has both math and language problems, however, it is more likely than not that the student has a general cognitive network problem (Figure 6).
All domain specific learning in math and language also depend on general cognition as well. General cognition is founded on two core pillars of learning: well-functioning (a) memory systems and well-functioning (b) attention systems (Tokuhama-Espinosa et al., 2020). Based on evidence from neuroimaging, both memory and attention make up (c) Executive Functions. Memory is sub-divided into complex (1) long-term memory, which in turn is divided into (i) non-declarative and (ii) declarative, (2) working memory, and (3) short-term memory. Executive Functions are sub-divided into (1) working memory, (2) cognitive flexibility, and (3) inhibitory control. Attention is sub-divided into (1) executive or sustained attention, (2) alerting, and (3) orienting systems (Fan and Posner, 2004). Just as each network in the domain specific areas of math and language sub-divide into numerous neural pathways (core notions), so do general cognitive networks. For example, long-term declarative memory networks can be further divided into semantic, autobiographical and episodic memory pathways, and there are likely many more.
It is now commonly accepted that the context within which one learns influences the learning itself (National Academies of Sciences, Engineering, and Medicine, 2018). The literature review revealed studies of learning context related to the role of (a) social contagion in learning, how (b) relationships with caregivers influenced learning, the role of (c) self-esteem in learning, and how (d) motivation impacts learning (Figure 7). These pathways, in turn, were sub-divided even further. For example, social contagion (a) was viewed differently in studies related to (1) cultural awareness and context as compared with (2) theory of mind research.
All learning occurs through the senses, as Aristotle pointed out over 2,500 years ago. Without sensory perception no learning is possible, let alone math and language. Hearing, Sight, and Touch studies were included in the review. Smell and taste were less prevalent in both the neuroscientific and educational literature and were therefore not included though future studies should consider their possible roles in learning math and language (Figures 8–10).
There are 10 identifiable pathways that emerge from the Hearing network, including distinct pathways for (a) pitch, (b) tempo, (c) tone, (d) prosody, and (e) loudness. Related to orientation within hearing were two distinct pathways related to echolocation or sounds that come from the (f) left versus right and from (g) foreground versus background sounds. Other pathways relate to the integration of sight and hearing through the interpretation and use of (h) hand gestures to support auditory compression. It was also found that the brain perceives and interprets (i) human voices distinctly from other sounds. Finally, there were multiple studies on (j) auditory processing, which combined sensory, motor, memory, and attention sub-systems.
Within Vision, it was found that there are 11 distinct pathways including those for (a) color, (b) luminance, (c) size, and (d) proximity. Visual pathways also distinguish (e) perception vs. action, (f) motion, and (g) spatial–temporal contrast. It was also found that as the most studied human sense, (h) visual crowding, occupies a distinct neural pathway from (i) spatial frequency, which is also distinct from the brain’s ability to search and determine (j) saliency in its surroundings. Finally, after 40 years of debate, it appears clear that the brain distinguished (k) human faces from other objects (Burns and Bukach, 2019).
The sense of Touch involves at least seven different neural pathways, but as the least studied of the senses, it is likely that additional research may extend these findings related to haptics and perception. There are distinct neural pathways for (a) visual motor integration, (b) scribbling, (c) fine motor tracing, and the (d) tactile recognition of shapes. Additionally, (e) writing—distinct from scribbling—(f) drawing, and the understanding of the (g) variant expressions of writing (such as capital versus small letters and cursive versus print, as well as different font forms) are also in distinct neural networks.
Of the four types of neural networks (domain specific, general cognitive, context, and sensory), sensory networks have the most research and the longest history. The sensory networks are based on perception from outside stimuli and memories of stimuli. Sensory networks were the gateways into the other three categories of networks. All four network categories are vital for learning to occur and should become part of teachers’ knowledge. We suggest that sharing these 16 neural networks in teacher training can potentially improve teacher diagnosis of learning problems by increasing their nuanced understanding of “language problems” or “math problems” and relating them to the core notions of these subjects.
On the basis of evidence from the learning sciences, we present a novel theory called Radical Neuroconstructivism, which is supported by extensive research from psychology, neuroscience, and education (Von Glasersfeld, 1984, 1995, 2013; Westermann et al., 2007, 2011; Dekker and Karmiloff-Smith, 2011; Hitchcock, 2018; Karmiloff-Smith et al., 2018; Broadbent and Mareschal, 2019; Tokuhama-Espinosa, 2019; De Soto, 2022). To further explain Radical Neuroconstructivism, we incorporate the concept of Meaning Making (Postman and Weingartner, 1969; Gay, 2018; Immordino-Yang and Knecht, 2020; Nouri et al., 2022) and Core Notions as the fundamental building blocks of cognition (Skerry and Saxe, 2016; Rist, 2017; Hernández Armenta et al., 2019; Solis-Stovall, 2020; Tuominen and Kallio, 2020; Sporns, 2022). We also provide examples of Math and Language learning trajectories that can be designed using neuroconstructivist principles, with more than 100 sources supporting each trajectory. Each of these studies holds individual significance and, when synthesized, we consider them to establish a powerful foundation for the proposed theory. We propose that the theory of Radical Neuroconstructivism offers a new framework for teacher education.
We suggest that teacher education can be seen as a holon, a complex system that consists of different parts that are interrelated and interdependent. However, not all parts of this system have received equal attention from academic disciplines such as psychology and education. While the question of how to teach has been widely researched, followed by the question of what to teach, the why of teaching has been less explored. This is where Mind, Brain, and Education (MBE) science can offer valuable insights. In Figure 11, in the first panel (Figure 5 “From Invisible Core Notions to the Visible Educational Curriculum”), the child learns the core notions in their own brain, but that same child (second panel) interacts with other children and the teacher. This dynamic exchange in the classroom is combined with the genetics, social-economic status and cultural context of the learner (third panel).
Evidence from MBE science can integrate the different subparts of teacher education by providing a better understanding of the why, which has been often neglected in traditional educational and psychological approaches. Radical neuroconstructivism is one framework that can inform teachers’ professional development and complete the holonic perspective of teaching.
In relation to the how of teaching, radical neurosconstrutivism suggests that teachers should encourage active exploration and discovery in their students, rather than transmitting information passively. This approach allows students to engage with the material in a meaningful way, and to construct their own representations based on prior knowledge and experience. Evidence as to why this is important suggests that active exploration can improve students’ motivation, curiosity, creativity, and memory retention.
Regarding the what, radical neuroconstructivism suggests that teachers should be aware of students’ developmental trajectories and individual differences, and tailor instruction accordingly. Teachers should identify difficulties and provide appropriate scaffolding and support to help students overcome them. Moreover, teachers should integrate different domains of learning in their curriculum to facilitate the formation of more abstract and generalizable representations, as well as the transfer of skills and knowledge across contexts.
To extend this perspective to the why, teachers need a solid understanding of core notions and the trajectories through which neural networks are constructed. This approach improves the order of skill acquisition by using a neuroconstructivist hierarchy, which may help create a more orderly curriculum built on insights from MBE science. Compared with MBE advancements from 2007 to present, by integrating research from neuroscience, psychology, and education, this new idea has the potential to inform the design of curriculum and instructional strategies that not only consider the what and how of teaching, but also the why, ensuring alignment with the brain’s natural learning processes.
In conclusion, MBE science offers a hol(on)istic perspective on teacher education that takes into account the what, how, and why of teaching and learning. Radical neuroconstructivism is a useful framework for organizing teachers” professional development and applying insights from MBE science into curriculum design and instructional strategies. By using MBE science to inform teaching practices, teachers can create a more effective and engaging learning environment for students.
The original contributions presented in the study are included in the article/Supplementary material, further inquiries can be directed to the corresponding author.
All authors listed have made a substantial, direct, and intellectual contribution to the work and approved it for publication.
The authors wish to thank people who have offered feedback on various versions of this manuscript between 2013 and 2023, especially Mariana Rivera who assisted in the original research in 2013.
Authors TT-E and CB provide consultancy services via the Conexiones platform. Author CB is employed by The Decision Lab.
All claims expressed in this article are solely those of the authors and do not necessarily represent those of their affiliated organizations, or those of the publisher, the editors and the reviewers. Any product that may be evaluated in this article, or claim that may be made by its manufacturer, is not guaranteed or endorsed by the publisher.
Abreu-Mendoza, R. A., Zarabozo-Hurtado, D., Chamorro, Y., Vazquez, P., Matute, E., and Fandakova, Y. (2020). The neural correlates of the core number systems contribute to mathematical ability in adolescence (preprint). PsyArXiv. doi: 10.31234/osf.io/96tuy
Agir, M. S. (2019). The effect of perceived teacher behaviors on students’ self-esteem and attitudes towards learning. J. Educ. Learn. 8, 203–218.
Ahn, I., Patrick, H., Chiu, M. M., and Levesque-Bristol, C. (2019). Measuring teacher practices that support student motivation: examining the factor structure of the teacher as social context questionnaire using multilevel factor analyses. J. Psychoeduc. Assess. 37, 743–756. doi: 10.1177/0734282918791655
Andres, M., Pelgrims, B., Michaux, N., Olivier, E., and Pesenti, M. (2011). Role of distinct parietal areas in arithmetic: an fMRI-guided TMS study. NeuroImage 54, 3048–3056. doi: 10.1016/j.neuroimage.2010.11.009
Anguera, J. A., Reuter-Lorenz, P. A., Willingham, D. T., and Seidler, R. D. (2010). Contributions of spatial working memory to visuomotor learning. J. Cogn. Neurosci. 22, 1917–1930. doi: 10.1162/jocn.2009.21351
Anobile, G., Stievano, P., and Burr, D. C. (2013). Visual sustained attention and numerosity sensitivity correlate with math achievement in children. J. Exp. Child Psychol. 116, 380–391. doi: 10.1016/j.jecp.2013.06.006
Ansari, D., Garcia, N., Lucas, E., Hamon, K., and Dhital, B. (2005). Neural correlates of symbolic number processing in children and adults. Neuroreport 16, 1769–1773. doi: 10.1097/01.wnr.0000183905.23396.f1
Artemenko, C., Soltanlou, M., Ehlis, A.-C., Nuerk, H.-C., and Dresler, T. (2018). The neural correlates of mental arithmetic in adolescents: a longitudinal fNIRS study. Behav. Brain Funct. 14:5. doi: 10.1186/s12993-018-0137-8
Athanasopoulos, P., and Casaponsa, A. (2020). Aristotle on the Transmission of Information: Receiving Form Without the Matter. Philosophical Problems in Sense Perception: Testing the Limits of Aristotelianism, 15–55.
Attout, L., Fias, W., Salmon, E., and Majerus, S. (2014). Common neural substrates for ordinal representation in short-term memory, numerical and alphabetical cognition. PLoS One 9:e92049. doi: 10.1371/journal.pone.0092049
Au, W. W. (2009). High-stakes testing and discursive control: the triple bind for non-standard student identities. Multicult. Perspect. 11, 65–71. doi: 10.1080/15210960903028727
Augustine, E., Jones, S. S., Smith, L. B., and Longfield, E. (2015). Relations among early object recognition skills: objects and letters. J. Cogn. Dev. 16, 221–235. doi: 10.1080/15248372.2013.815620
Axelrod, V., Schwarzkopf, D. S., Gilaie-Dotan, S., and Rees, G. (2017). Perceptual similarity and the neural correlates of geometrical illusions in human brain structure. Sci. Rep. 7:39968. doi: 10.1038/srep39968
Bada, S. O. (2015). Constructivism learning theory: a paradigm for teaching and learning. J. Res. Method Educ. 5, 66–70. doi: 10.9790/7388-05616670
Baker, S. K. (1995). Vocabulary acquisition: synthesis of the research. Technical report no. 13. National Center to Improve the Tools of Educators, Eugene, OR. Available at: https://eric.ed.gov/?id=ED386860
Bakken, L., Brown, N., and Downing, B. (2017). Early childhood education: the long-term benefits. J. Res. Child. 31, 255–269. doi: 10.1080/02568543.2016.1273285
Baldo, J. V., and Dronkers, N. F. (2007). Neural correlates of arithmetic and language comprehension: a common substrate? Neuropsychologia 45, 229–235. doi: 10.1016/j.neuropsychologia.2006.07.014
Ball, D. L. (2017). “Uncovering the special mathematical work of teaching” in Proceedings of the 13th International Congress on Mathematical Education: ICME-13. ed. G. Keiser (Germany: Hamburg), 11–34.
Bandura, A. (1977). Self-efficacy: toward a unifying theory of behavioral change. Psychol. Rev. 84, 191–215. doi: 10.1037/0033-295X.84.2.191
Barber, M., and Mourshed, M. (2007). How the World’s best-performing school systems come out on top. London: McKinsey & Co.
Bartelet, D., Ansari, D., Vaessen, A., and Blomert, L. (2014). Cognitive subtypes of mathematics learning difficulties in primary education. Res. Dev. Disabil. 35, 657–670. doi: 10.1016/j.ridd.2013.12.010
Bates, E., Thal, D., and Janowsky, J. S. (1992). “Early language development and its neural correlates” in Handbook of neuropsychology. eds. I. Rapin and S. Segalowitz (Amsterdam: Elsevier), 69–110.
Baumann, A., Tödt, I., Knutzen, A., Gless, C. A., Granert, O., Wolff, S., et al. (2022). Neural correlates of executed compared to imagined writing and drawing movements: a functional magnetic resonance imaging study. Front. Hum. Neurosci. 16:829576. doi: 10.3389/fnhum.2022.829576
Benavides-Varela, S., and Gervain, J. (2017). Learning word order at birth: a NIRS study. Dev. Cogn. Neurosci. 25, 198–208. doi: 10.1016/j.dcn.2017.03.003
Benischek, A. M. (2018). Early language abilities and the underlying neural functional reading network in preschoolers [Master’s thesis, University of Calgary]. Available at: https://prism.ucalgary.ca/items/c92fe4af-0d9e-4e45-b508-454b7bc2275a
Benischek, A., Long, X., Rohr, C. S., Bray, S., Dewey, D., and Lebel, C. (2020). Pre-reading language abilities and the brain’s functional reading network in young children. Neuroimage 217:116903. doi: 10.1016/j.neuroimage.2020.116903
Berch, D. B., Geary, D. C., and Koepke, K. M. (Eds.) (2016). Development of mathematical cognition: neural substrates and genetic influences, mathematical cognition and learning. Boston, MA: Elsevier/Academic Press.
Bergeson, T. R., and Trehub, S. E. (2006). Infants perception of rhythmic patterns. Music. Percept. 23, 345–360. doi: 10.1525/mp.2006.23.4.345
Berliner, D. C., and Calfee, R. C. (2014). Handbook of educational psychology. 2nd Ed. New York, NY: Routledge.
Bernbaum, M., Goodnow, J., and Lehman, E. (1974). Relationships among perceptual-motor tasks: tracing and copying. J. Educ. Psychol. 66, 731–735. doi: 10.1037/h0037430
Bers, M. U., and Cassell, J. (2000). “Children as designers of interactive storytellers: ‘let me tell you a story about myself…” in Human cognition and social agent technology. ed. K. Dautenhahn (Netherlands: John Benjamins Publisher), 61–83.
Bevilacqua, D., Davidesco, I., Wan, L., Chaloner, K., Rowland, J., Ding, M., et al. (2019). Brain-to-brain synchrony and learning outcomes vary by student–teacher dynamics: evidence from a real-world classroom electroencephalography study. J. Cogn. Neurosci. 31, 401–411. doi: 10.1162/jocn_a_01274
Biederman, I. (2013). “Psychophysical and neural correlates of the phenomenology of shape” in Handbook of experimental phenomenology. ed. L. Albertazzi (Chichester: John Wiley & Sons, Ltd), 415–436.
Black, M. (1962). Models and metaphors: Studies in language and philosophy. Ithaca, NY: Cornell University Press.
Bloechle, J., Huber, S., Klein, E., Bahnmueller, J., Moeller, K., and Rennig, J. (2018). Neuro-cognitive mechanisms of global gestalt perception in visual quantification. Neuroimage 181, 359–369. doi: 10.1016/j.neuroimage.2018.07.026
Boeren, E. (2019). Understanding sustainable development goal (SDG) 4 on “quality education” from micro, meso and macro perspectives. Int. Rev. Educ. 65, 277–294. doi: 10.1007/s11159-019-09772-7
Booth, J. R., Cho, S., Burman, D. D., and Bitan, T. (2007). Neural correlates of mapping from phonology to orthography in children performing an auditory spelling task. Dev. Sci. 10, 441–451. doi: 10.1111/j.1467-7687.2007.00598.x
Braadbaart, L., Waiter, G. D., and Williams, J. H. G. (2012). Neural correlates of individual differences in manual imitation fidelity. Front. Integr. Neurosci. 6:91. doi: 10.3389/fnint.2012.00091
Broadbent, H., and Mareschal, D. (2019). “Neuroconstructivism” in The Wiley Encyclopedia of child and adolescent development. eds. S. Hupp and J. D. Jewell (New Jersey, NY: John Wiley & Sons), 1–11.
Bruce, C. D., and Hawes, Z. (2015). The role of 2D and 3D mental rotation in mathematics for young children: what is it? Why does it matter? And what can we do about it? ZDM - int. J. Math. Educ. 47, 331–343. doi: 10.1007/s11858-014-0637-4
Bugden, S., Price, G. R., McLean, D. A., and Ansari, D. (2012). The role of the left intraparietal sulcus in the relationship between symbolic number processing and children’s arithmetic competence. Dev. Cogn. Neurosci. 2, 448–457. doi: 10.1016/j.dcn.2012.04.001
Burns, E. J., and Bukach, C. (2019). Meta-analyses support the expertise hypothesis of the right fusiform face area. J. Vis. 19:115a. doi: 10.1167/19.10.115a
Burr, D. C., Turi, M., and Anobile, G. (2010). Subitizing but not estimation of numerosity requires attentional resources. J. Vis. 10, 1–10. doi: 10.1167/10.6.20
Butterworth, B., Varma, S., and Laurillard, D. (2011). Dyscalculia: from brain to education. Science 332, 1049–1053. doi: 10.1126/science.1201536
Calmels, C. (2020). Neural correlates of motor expertise: extensive motor training and cortical changes. Brain Res. 1739:146323. doi: 10.1016/j.brainres.2019.146323
Campbell, J. I. (Ed.). (2014). Handbook of mathematical cognition. New York, NY: Taylor and Francis Group.
Campbell, F. A., Pungello, E. P., Burchinal, M., Kainz, K., Pan, Y., Wasik, B. H., et al. (2012). Adult outcomes as a function of an early childhood educational program: an abecedarian project follow-up. Dev. Psychol. 48, 1033–1043. doi: 10.1037/a0026644
Cantlon, J. F., Pinel, P., Dehaene, S., and Pelphrey, K. A. (2011). Cortical representations of symbols, objects, and faces are pruned back during early childhood. Cerebellum 21, 191–199. doi: 10.1093/cercor/bhq078
Cao, F., Khalid, K., Zaveri, R., Bolger, D. J., Bitan, T., and Booth, J. R. (2010). Neural correlates of priming effects in children during spoken word processing with orthographic demands. Brain Lang. 114, 80–89. doi: 10.1016/j.bandl.2009.07.005
Caravolas, M., Lervåg, A., Mousikou, P., Efrim, C., Litavský, M., Onochie-Quintanilla, E., et al. (2012). Common patterns of prediction of literacy development in different alphabetic orthographies. Psychol. Sci. 23, 678–686. doi: 10.1177/0956797611434536
Carreiras, M., Quiñones, I., Hernández-Cabrera, J. A., and Duñabeitia, J. A. (2015). Orthographic coding: brain activation for letters, symbols, and digits. Cerebellum 25, 4748–4760. doi: 10.1093/cercor/bhu163
Caston, V. (2020). Aristotle on the Transmission of Information: Receiving Form Without the Matter. In: Philosophical Problems in Sense Perception: Testing the Limits of Aristotelianism. Studies in the History of Philosophy of Mind, vol 26. eds. D. Bennett and J. Toivanen, Cham: Springer.
Chafe, W. L. (1985). “Linguistic differences produced by differences between speaking and writing” in Literacy, language, and learning. eds. D. R. Olson, N. Torrance, and A. Hildyard (Camebridge: CUP), 9–50.
Chen, F., Hu, Z., Zhao, X., Wang, R., Yang, Z., Wang, X., et al. (2006). Neural correlates of serial abacus mental calculation in children: a functional MRI study. Neurosci. Lett. 403, 46–51. doi: 10.1016/j.neulet.2006.04.041
Chen, L., Iuculano, T., Mistry, P., Nicholas, J., Zhang, Y., and Menon, V. (2021). Linear and nonlinear profiles of weak behavioral and neural differentiation between numerical operations in children with math learning difficulties. Neuropsychologia 160:107977. doi: 10.1016/j.neuropsychologia.2021.107977
Cheng, K., Huttenlocher, J., and Newcombe, N. S. (2013). 25 years of research on the use of geometry in spatial reorientation: a current theoretical perspective. Psychon. Bull. Rev. 20, 1033–1054. doi: 10.3758/s13423-013-0416-1
Cherodath, S., Rao, C., Midha, R., Sumathi, T. A., and Singh, N. C. (2017). A role for putamen in phonological processing in children. Bilingualism 20, 318–326. doi: 10.1017/S1366728916000614
Chesney, D. L., McNeil, N. M., Matthews, P. G., Byrd, C. E., Petersen, L. A., Wheeler, M. C., et al. (2014). Organization matters: mental organization of addition knowledge relates to understanding math equivalence in symbolic form. Cogn. Dev. 30, 30–46. doi: 10.1016/j.cogdev.2014.01.001
Cho, S., Ryali, S., Geary, D. C., and Menon, V. (2011). How does a child solve 7 + 8? Decoding brain activity patterns associated with counting and retrieval strategies: dissociating arithmetic strategy use in children. Dev. Sci. 14, 989–1001. doi: 10.1111/j.1467-7687.2011.01055.x
Chomsky, N. (2000). “Recent contributions to the theory of innate ideas” in Minds, brains and computers the foundation of cognitive science, an anthology. eds. R. M. Harnish and D. D. Cummins (Malden, MA: Blackwell), 452–457.
Christodoulou, J. A. (2010). Identifying the neural correlates of fluent reading. [doctoral dissertation, Harvard University]. Available at: https://www.proquest.com/openview/d17847c7df24fb45d83c9f50597d803b/1?pq-origsite=gscholar&cbl=18750
Clements, D. H., Banse, H., Sarama, J., Tatsuoka, C., Joswick, C., Hudyma, A., et al. (2022). Young children’s actions on length measurement tasks: strategies and cognitive attributes. Math. Think. Learn. 24, 181–202. doi: 10.1080/10986065.2020.1843231
Connolly, K. (2019). Perceptual learning: the flexibility of the senses. New York, NY: Oxford University Press.
Costa, L. J. C., Spencer, S. V., and Hooper, S. R. (2022). Emergent neuroimaging findings for written expression in children: a scoping review. Brain Sci. 12:406. doi: 10.3390/brainsci12030406
Cristia, A., Minagawa-Kawai, Y., Egorova, N., Gervain, J., Filippin, L., Cabrol, D., et al. (2014). Neural correlates of infant accent discrimination: an fNIRS study. Dev. Sci. 17, 628–635. doi: 10.1111/desc.12160
Cui, J., Yu, X., Yang, H., Chen, C., Liang, P., and Zhou, X. (2013). Neural correlates of quantity processing of numeral classifiers. Neuropsychology 27, 583–594. doi: 10.1037/a0033630
Cunningham, J. (2018). Missing the mark: standardized testing as epistemological erasure in U.S. schooling. Power Educ. 11, 111–120. doi: 10.1177/1757743818812093
Cutini, S., Scatturin, P., Basso Moro, S., and Zorzi, M. (2014). Are the neural correlates of subitizing and estimation dissociable? An fNIRS investigation. Neuroimage 85, 391–399. doi: 10.1016/j.neuroimage.2013.08.027
D’Angiulli, A., Griffiths, G., and Marmolejo-Ramos, F. (2015). Neural correlates of visualizations of concrete and abstract words in preschool children: a developmental embodied approach. Front. Psychol. 6:856. doi: 10.3389/fpsyg.2015.00856
Daly, I., Bourgaize, J., and Vernitski, A. (2019). Mathematical mindsets increase student motivation: evidence from the EEG. Trends Neurosci. Educ. 15, 18–28. doi: 10.1016/j.tine.2019.02.005
Damasio, H., Grabowski, T. J., Tranel, D., Ponto, L. L. B., Hichwa, R. D., and Damasio, A. R. (2001). Neural correlates of naming actions and of naming spatial relations. Neuroimage 13, 1053–1064. doi: 10.1006/nimg.2001.0775
Davis, N., Cannistraci, C. J., Rogers, B. P., Gatenby, J. C., Fuchs, L. S., Anderson, A. W., et al. (2009). The neural correlates of calculation ability in children: an fMRI study. JMRI 27, 1187–1197. doi: 10.1016/j.mri.2009.05.010
De Marco, D., De Stefani, E., and Vecchiato, G. (2022). Embodying language through gestures: residuals of motor memories modulate motor cortex excitability during abstract words comprehension. Sensors 22:7734. doi: 10.3390/s22207734
De Smedt, B., and Gilmore, C. K. (2011). Defective number module or impaired access? Numerical magnitude processing in first graders with mathematical difficulties. J. Exp. Child Psychol. 108, 278–292. doi: 10.1016/j.jecp.2010.09.003
De Soto, J. A. (2022). The constructivism of social discourse: toward a contemporaneous understanding of knowledge. Sci. Res. J. 12, 376–396. doi: 10.4236/ojpp.2022.123025
Deans for Impact. (2015). The science of learning. Austin, TX: Author. Available at: http://www.deansforimpact.org/wp-content/uploads/2016/12/The_Science_of_Learning.pdf
Deetsch, M., Glass, R., Jankowski, R., Mylander, E., Roth, P., and Wharton, E. (2018). Visual literacy and its impact on pre-literacy development. J. Mus. Educ. 43, 148–158. doi: 10.1080/10598650.2018.1426332
Dehaene, S. (1992). Varieties of numerical abilities. Cognition 44, 1–42. doi: 10.1016/0010-0277(92)90049-N
Dehaene, S. (2003). The neural basis of the Weber–Fechner law: a logarithmic mental number line. TiCS 7, 145–147. doi: 10.1016/S1364-6613(03)00055-X
Dehaene, S. (2011). The number sense: how the mind creates mathematics. New York, NY: Oxford University Press.
Dehaene, S., and Cohen, L. (1995). Towards an anatomical and functional model of number processing. Math. Cogn. 1, 83–120.
Dehaene, S., and Cohen, L. (2010). “Neural coding of written words in the visual word form area” in The neural basis of reading. eds. P. Cornelissen, P. Hensan, M. Kringelbach, and K. Pugh (New York, NY: Oxford University Press), 111–146.
Dehaene, S., Meyniel, F., Wacongne, C., Wang, L., and Pallier, C. (2015). The neural representation of sequences: from transition probabilities to algebraic patterns and linguistic trees. Neuron 88, 2–19. doi: 10.1016/j.neuron.2015.09.019
Dehaene, S., Molko, N., Cohen, L., and Wilson, A. J. (2004). Arithmetic and the brain. Curr. Opin. Neurobiol. 14, 218–224. doi: 10.1016/j.conb.2004.03.008
Dehaene, S., Nakamura, K., Jobert, A., Kuroki, C., Ogawa, S., and Cohen, L. (2010). Why do children make mirror errors in reading? Neural correlates of mirror invariance in the visual word form area. Neuroimage 49, 1837–1848. doi: 10.1016/j.neuroimage.2009.09.024
Dehaene, S., Piazza, M., Pinel, P., and Cohen, L. (2003). Three parietal circuits for number processing. Cogn. Neuropsychol. 20, 487–506. doi: 10.1080/02643290244000239
Dehaene-Lambertz, G., Pallier, C., Serniclaes, W., Sprenger-Charolles, L., Jobert, A., and Dehaene, S. (2005). Neural correlates of switching from auditory to speech perception. Neuroimage 24, 21–33. doi: 10.1016/j.neuroimage.2004.09.039
Dekker, T. M., and Karmiloff-Smith, A. (2011). The dynamics of ontogeny: a neuroconstructivist perspective on genes, brains, cognition and behavior. Prog. Brain Res. 189, 23–33. doi: 10.1016/B978-0-444-53884-0.00016-6
Demeyere, N., Rotshtein, P., and Humphreys, G. W. (2012). The neuroanatomy of visual enumeration: differentiating necessary neural correlates for subitizing versus counting in a neuropsychological voxel-based morphometry study. J. Cogn. Neurosci. 24, 948–964. doi: 10.1162/jocn_a_00188
Dempsey, L. A., Cooper, R. J., Powell, S., Edwards, A., Lee, C.-W., Brigadoi, S., et al. (2015). “Whole-head functional brain imaging of neonates at cot-side using time-resolved diffuse optical tomography” in Diffuse Optical Imaging V. Presented at the European Conference on Biomedical Optics (Munich: OSA)
den Ouden, D.-B., Fix, S., Parrish, T. B., and Thompson, C. K. (2009). Argument structure effects in action verb naming in static and dynamic conditions. J. Neurolinguistics 22, 196–215. doi: 10.1016/j.jneuroling.2008.10.004
Deoni, S. C. L., Mercure, E., Blasi, A., Gasston, D., Thomson, A., Johnson, M., et al. (2011). Mapping infant brain myelination with JMRI. J. Neurosci. 31, 784–791. doi: 10.1523/JNEUROSCI.2106-10.2011
DiCarlo, J. J., Zoccolan, D., and Rust, N. C. (2012). How does the brain solve visual object recognition? Neuron 73, 415–434. doi: 10.1016/j.neuron.2012.01.010
Dillon, M. R. (2017). Becoming Euclid: connecting core cognition, spatial symbols, and the abstract concepts of formal geometry. [doctoral dissertation, Harvard University]. Available at: https://www.proquest.com/openview/11a2ef086df7ab38c3d74d418e76dfc8/1?pq-origsite=gscholar&cbl=18750&diss=y (Accessed June 3, 2023)
Domahs, F., Moeller, K., Huber, S., Willmes, K., and Nuerk, H.-C. (2010). Embodied numerosity: implicit hand-based representations influence symbolic number processing across cultures. Cognition 116, 251–266. doi: 10.1016/j.cognition.2010.05.007
Donahoe, B., Rickard, D., Holden, H., Blackwell, K., and Caukin, N. (2019). Using EdTech to enhance learning. Int. J. Whole Child 4, 57–63.
Donovan, A. M., and Fyfe, E. (2019). Connecting concrete objects and abstract symbols promotes children’s mathematics learning (preprint). PsyArXiv. doi: 10.31234/osf.io/ye2j6
Du, C., Miyazaki, Y., Cook, M., Papadopoulos, J., and Hao, Y. (2018). Relational language improves preschool children’s performance of analogical reasoning. Int. J. Psychol. Stud. 10, 91–101. doi: 10.5539/ijps.v10n2p91
Dubinsky, J. M., Roehrig, G., and Varma, S. (2022). A place for neuroscience in teacher knowledge and education. Mind Brain Educ. 16, 267–276. doi: 10.1111/mbe.12334
Dunagan, D., Zhang, S., Li, J., Bhattasali, S., Pallier, C., Whitman, J., et al. (2022). Neural correlates of semantic number: a cross-linguistic investigation. Brain Lang. 229:105110. doi: 10.1016/j.bandl.2022.105110
Edwards, M. (2003). A brief history of holons. Unpublished essay. Available at: https://spiraldynamicsintegral.nl/wp-content/uploads/2013/09/Edwards-Mark-A-Brief-History-of-Holons.pdf (Accessed: 30 April 2023)
Ejima, Y., Takahashi, S., Yamamoto, H., and Goda, N. (2007). “Visual perception of contextual effect and its neural correlates” in Representation and brain. ed. S. Funahashi (Tokyo: Springer), 3–20.
Ellis, A. (2015). The developing math brain: an fNIRS study. [doctoral dissertation, University of Michigan]. Available at: https://deepblue.lib.umich.edu/handle/2027.42/111890
Emberson, L. L., Richards, J. E., and Aslin, R. N. (2015). Top-down modulation in the infant brain: learning-induced expectations rapidly affect the sensory cortex at 6 months. Proc. Natl. Acad. Sci. U. S. A. 112, 9585–9590. doi: 10.1073/pnas.1510343112
Emerson, R. W., and Cantlon, J. F. (2012). Early math achievement and functional connectivity in the fronto-parietal network. Dev. Cogn. Neurosci. 2, S139–S151. doi: 10.1016/j.dcn.2011.11.003
Engelbrecht, W., and Ankiewicz, P. (2016). Criteria for continuing professional development of technology teachers’ professional knowledge: a theoretical perspective. Int. J. Technol. Des. Educ. 26, 259–284. doi: 10.1007/s10798-015-9309-0
Fabiani, E., Velay, J. L., Younes, C., Anton, J. L., Nazarian, B., Sein, J., et al. (2023). Writing letters in two graphic systems: behavioral and neural correlates in French/Arabic biscripters. Neuropsychologia 185:108567. doi: 10.1016/j.neuropsychologia.2023.108567
Fan, L.-Y., Gau, S. S.-F., and Chou, T.-L. (2014). Neural correlates of inhibitory control and visual processing in youths with attention deficit hyperactivity disorder: a counting Stroop functional MRI study. Psychol. Med. 44, 2661–2671. doi: 10.1017/S0033291714000038
Fan, J., and Posner, M. (2004). Human attentional networks. Psychiatr. Prax. 31, 210–214. doi: 10.1055/s-2004-828484
Fayol, M., Alamargot, D., and Berninger, V. W. (Eds.). (2012). Translation of thought to written text while composing: advancing theory, knowledge, research methods, tools, and applications. New York, NY: Psychology Press/Taylor & Francis Group.
Fecteau, J., and Enns, J. (2005). Visual letter matching: hemispheric functioning or scanning biases? Neuropsychologia 43, 1412–1428. doi: 10.1016/j.neuropsychologia.2005.01.006
Fehr, T., Code, C., and Herrmann, M. (2007). Common brain regions underlying different arithmetic operations as revealed by conjunct fMRI–BOLD activation. Brain Res. 1172, 93–102. doi: 10.1016/j.brainres.2007.07.043
Feng, X., Altarelli, I., Monzalvo, K., Ding, G., Ramus, F., Shu, H., et al. (2020). A universal reading network and its modulation by writing system and reading ability in French and Chinese children. Elife 9:e54591. doi: 10.7554/eLife.5459
Fernald, A., Marchman, V. A., and Weisleder, A. (2013). SES differences in language processing skill and vocabulary are evident at 18 months. Dev. Sci. 16, 234–248. doi: 10.1111/desc.12019
Ferreira, R. A., Göbel, S. M., Hymers, M., and Ellis, A. W. (2015). The neural correlates of semantic richness: evidence from an fMRI study of word learning. Brain Lang. 143, 69–80. doi: 10.1016/j.bandl.2015.02.005
Fish, M. (2020). Exploring the spatiotemporal dynamics of on-line sentence comprehension in 5-year-olds: the role of semantic context in syntactic processing and behavioral correlates of MEG-recorded brain activity. [doctoral dissertation, University of Washington]. Available at: https://digital.lib.washington.edu/researchworks/handle/1773/45547
Flanagan, D. P., and McDonough, E. M. (eds.) (2018). Contemporary intellectual assessment: theories, tests, and issues. New York, NY: Guilford Press.
Fockert, J., Rees, G., Frith, C., and Lavie, N. (2004). Neural correlates of attentional capture in visual search. J. Cogn. Neurosci. 16, 751–759. doi: 10.1162/089892904970762
Forkstam, C., Hagoort, P., Fernandez, G., Ingvar, M., and Petersson, K. M. (2006). Neural correlates of artificial syntactic structure classification. Neuroimage 32, 956–967. doi: 10.1016/j.neuroimage.2006.03.057
Fragaszy, D. M., Kuroshima, H., and Stone, B. W. (2015). “Vision for action” in Young children aligning multi-featured objects: development and comparison with nonhuman primates. PLoS One 10:e0140033. doi: 10.1371/journal.pone.0140033
Frank, S. L., Bod, R., and Christiansen, M. H. (2012). How hierarchical is language use? Proc. R. Soc. B 279, 4522–4531. doi: 10.1098/rspb.2012.1741
Fransson, P., Åden, U., Blennow, M., and Lagercrantz, H. (2011). The functional architecture of the infant brain as revealed by resting-state fMRI. Cerebellum 21, 145–154. doi: 10.1093/cercor/bhq071
Frey, N., Fisher, D., and Smith, D. (2019). All learning is social and emotional: helping students develop essential skills for the classroom and beyond. Alexandria, VA: ASCD.
Frick, A., Hansen, M. A., and Newcombe, N. S. (2013). Development of mental rotation in 3- to 5-year-old children. Cogn. Dev. 28, 386–399. doi: 10.1016/j.cogdev.2013.06.002
Friederici, A. D. (2005). Neurophysiological markers of early language acquisition: from syllables to sentences. TiCS 9, 481–488. doi: 10.1016/j.tics.2005.08.008
Friederici, A. D., Chomsky, N., Berwick, R. C., Moro, A., and Bolhuis, J. J. (2017). Language, mind and brain. Nat. Hum. Behav. 1, 713–722. doi: 10.1038/s41562-017-0184-4
Friederici, A. D., and Männel, C. (2013). “Neural correlates of the development of speech perception and comprehension” in The Oxford handbook of cognitive neuroscience. eds. K. Ochsner and S. M. Kosslyn, vol. 1 (Oxford: Oxford University Press), 1–36.
Fudickar, J. (2018). Elementary students use voice-to-text to write. [doctoral dissertation, University of Oklahoma]. Available at: https://shareok.org/handle/11244/299859
Fuson, K. C., Clements, D. H., and Sarama, J. (2015). Making early math education work for all children. Phi Delta Kappan 97, 63–68. doi: 10.1177/0031721715614831
Gallace, A., and Spence, C. (2008). The cognitive and neural correlates of “tactile consciousness”: a multisensory perspective. Conscious. Cogn. 17, 370–407. doi: 10.1016/j.concog.2007.01.005
Gallace, A., and Spence, C. (2009). The cognitive and neural correlates of tactile memory. Psychol. Bull. 135, 380–406. doi: 10.1037/a0015325
Gallifa, J. (2019). Integral thinking and its application to integral education. J. Int. Educ. Pract. 2:15. doi: 10.30564/jiep.v2i1.603
Galván, A. (2010). Neural plasticity of development and learning. Hum. Brain Mapp. 31, 879–890. doi: 10.1002/hbm.21029
Gandini, D., Lemaire, P., Anton, J.-L., and Nazarian, B. (2008). Neural correlates of approximate quantification strategies in young and older adults: an fMRI study. Brain Res. 1246, 144–157. doi: 10.1016/j.brainres.2008.09.096
Gansler, D. A., Moore, D. W., Susmaras, T. M., Jerram, M. W., Sousa, J., and Heilman, K. M. (2011). Cortical morphology of visual creativity. Neuropsychologia 49, 2527–2532. doi: 10.1016/j.neuropsychologia.2011.05.001
Gao, F., Hua, L., He, Y., Xu, J., Li, D., Zhang, J., et al. (2023). Word structure tunes electrophysiological and hemodynamic responses in the frontal cortex. Bioengineering 10:288. doi: 10.3390/bioengineering10030288
Garnier, M., Lamalle, L., and Sato, M. (2013). Neural correlates of phonetic convergence and speech imitation. Front. Psychol. 4:600. doi: 10.3389/fpsyg.2013.00600
Gay, G. (2018). Culturally responsive teaching: theory, research, and practice. New York, NY: Teachers College Press.
Geangu, E., Quadrelli, E., Lewis, J. W., Macchi Cassia, V., and Turati, C. (2015). By the sound of it. An ERP investigation of human action sound processing in 7-month-old infants. Dev. Cogn. Neurosci. 12, 134–144. doi: 10.1016/j.dcn.2015.01.005
Gebauer, D., Enzinger, C., Kronbichler, M., Schurz, M., Reishofer, G., Koschutnig, K., et al. (2012). Distinct patterns of brain function in children with isolated spelling impairment: new insights. Neuropsychologia 50, 1353–1361. doi: 10.1016/j.neuropsychologia.2012.02.020
Gervain, J., Werker, J. F., Black, A., and Geffen, M. N. (2016). The neural correlates of processing scale-invariant environmental sounds at birth. Neuroimage 133, 144–150. doi: 10.1016/j.neuroimage.2016.03.001
Gerván, P. (2012). Cortical structural and functional components of visual perceptual learning. [doctoral dissertation, Budapest University of Technology and Economics]. Available at: http://www.cogsci.bme.hu/~ktkuser/PHD_iskola/dissertations/20121217_Gervan_Patricia/Thesis%20booklet_GP2012.pdf
Gess-Newsome, J. (2013). “Pedagogical content knowledge” in International guide to student achievement. eds. J. Hattie and E. M. Anderman (New York, NY: Routledge), 267–269.
Ghio, M. V. (2013). A cognitive and pragmatic approach to meaning. Behavioral and neural correlates of concept processing [doctoral dissertation, Scuola Normale Superiore]. Available at: https://ricerca.sns.it/retrieve/e3aacdfd-ef22-4c98-e053-3705fe0acb7e/GHIO-Marta-PhD-2013-Lettere.pdf
Gilet, E., Diard, J., and Bessière, P. (2011). Bayesian action–perception computational model: interaction of production and recognition of cursive letters. PLoS One 6:e20387. doi: 10.1371/journal.pone.0020387
Gilmore, C., Attridge, N., De Smedt, B., and Inglis, M. (2014). Measuring the approximate number system in children: exploring the relationships among different tasks. Learn. Individ. Differ. 29, 50–58. doi: 10.1016/j.lindif.2013.10.004
Goffin, C. (2019). How does the brain represent digits? Investigating the neural correlates of symbolic number representation using fMRI-adaptation [doctoral dissertation, the University of Western Ontario (Canada)]. Available at: https://www.proquest.com/openview/a8761e80795554487ad3180b5d4fa12c/1?pq-origsite=gscholar&cbl=18750&diss=y
Goffin, C., and Ansari, D. (2016). Beyond magnitude: judging ordinality of symbolic number is unrelated to magnitude comparison and independently relates to individual differences in arithmetic. Cognition 150, 68–76. doi: 10.1016/j.cognition.2016.01.018
Golinkoff, R. M., Hoff, E., Rowe, M. L., Tamis-LeMonda, C. S., and Hirsh-Pasek, K. (2019). Language matters: denying the existence of the 30-million-word gap has serious consequences. Child Dev. 90, 985–992. doi: 10.1111/cdev.13128
Gorard, S. (2006). “Learning trajectories” in Human learning: an holistic approach. eds. P. Jarvis and S. Parker (Oxon: Routledge), 195–209.
Gordon, R., and Ramani, G. B. (2021). Integrating embodied cognition and information processing: a combined model of the role of gesture in Children’s mathematical environments. Front. Psychol. 12:650286. doi: 10.3389/fpsyg.2021.650286
Grabner, R. H., Ansari, D., Koschutnig, K., Reishofer, G., Ebner, F., and Neuper, C. (2009). To retrieve or to calculate? Left angular gyrus mediates the retrieval of arithmetic facts during problem solving. Neuropsychologia 47, 604–608. doi: 10.1016/j.neuropsychologia.2008.10.013
Grafton, S. T., Hazeltine, E., and Ivry, R. (1995). Functional mapping of sequence learning in normal humans. J. Cogn. Neurosci. 7, 497–510. doi: 10.1162/jocn.1995.7.4.497
Gredebäck, G., Melinder, A., and Daum, M. (2010). The development and neural basis of pointing comprehension. Soc. Neurosci. 5, 441–450. doi: 10.1080/17470910903523327
Grotheer, M., Ambrus, G. G., and Kovács, G. (2016). Causal evidence of the involvement of the number form area in the visual detection of numbers and letters. Neuroimage 132, 314–319. doi: 10.1016/j.neuroimage.2016.02.069
Gruber, O. (2001). Dissociating neural correlates of cognitive components in mental calculation. Cerebellum 11, 350–359. doi: 10.1093/cercor/11.4.350
Gunderson, E. A., and Hildebrand, L. (2021). Relations among spatial skills, number line estimation, and exact and approximate calculation in young children. J. Exp. Child Psychol. 212:105251. doi: 10.1016/j.jecp.2021.105251
Hahn, N., Jansen, P., and Heil, M. (2010). Preschoolers’ mental rotation: sex differences in hemispheric asymmetry. J. Cogn. Neurosci. 22, 1244–1250. doi: 10.1162/jocn.2009.21236
Halden, A., Clark, C., and Lewis, F. (2011). National Literacy Trust Survey in partnership with nursery world: investigating communication, language and literacy development in the early years sector National Literacy Trust Available at: https://eric.ed.gov/?id=ED521659.
Hale, L. A., and Crowe, C. (2001). ‘I hate reading if I don’t have to’: results from a longitudinal study of high school students’ reading interest. Virginia Tech University Libraries. Available at: https://scholar.lib.vt.edu/ejournals/ALAN/v28n3/hale.html (Accessed April 27, 2023).
Hallowell, D. A., Okamoto, Y., Romo, L. F., and La Joy, J. R. (2015). First-graders’ spatial-mathematical reasoning about plane and solid shapes and their representations. Int. J. Math. Educ. 47, 363–375. doi: 10.1007/s11858-015-0664-9
Haman, M., and Lipowska, K. (2021). Moving attention along the mental number line in preschool age: study of the operational momentum in 3- to 5-year-old children’s non-symbolic arithmetic. Dev. Sci. 24:e13007. doi: 10.1111/desc.13007
Hammond, Z. (2014). Culturally responsive teaching and the brain: promoting authentic engagement and rigor among culturally and linguistically diverse students. Thousand Oaks, CA, USA: Corwin Press.
Hanakawa, T., Honda, M., Okada, T., Fukuyama, H., and Shibasaki, H. (2003). Neural correlates underlying mental calculation in abacus experts: a functional JMRI study. Neuroimage 19, 296–307. doi: 10.1016/S1053-8119(03)00050-8
Hannula-Sormunen, M. M. (2015). “Spontaneous focusing on numerosity and its relation to counting and arithmetic” in The Oxford handbook of numerical cognition. eds. R. C. Kadosh and A. Dowker (New York, NY: Oxford University Press), 275–290.
Hannula-Sormunen, M. M., McMullen, J., Räsänen, P., Lepola, J., and Lehtinen, E. (2016). Is the study about spontaneous attention to exact quantity based on studies of spontaneous focusing on numerosity? Eur. J. Dev. Psychol. 13, 115–120. doi: 10.1080/17405629.2015.1071252
Hansen, A., Drews, D., Dudgeon, J., Lawton, F., and Surtees, L. (2020). Children′ s errors in mathematics. Thousand Oaks, CA: Sage.
Harris, I. M., and Miniussi, C. (2003). Parietal lobe contribution to mental rotation demonstrated with rTMS. J. Cogn. Neurosci. 15, 315–323. doi: 10.1162/089892903321593054
Hart, B., and Risley, T. R. (2003). The early catastrophe: the 30 million word gap by age 3. Am. Educ. 27, 4–9.
Hasegawa, C., Takahashi, T., Ikeda, T., Yoshimura, Y., Hiraishi, H., Nobukawa, S., et al. (2021). Effects of familiarity on child brain networks when listening to a storybook reading: a magneto-encephalographic study. Neuroimage 241:118389. doi: 10.1016/j.neuroimage.2021.118389
Hattie, J., and Zierer, K. (2017). 10 mindframes for visible learning: teaching for success. New York, NY: Routledge.
Hawes, Z., and Ansari, D. (2020). What explains the relationship between spatial and mathematical skills? A review of evidence from brain and behavior. Psychon. Bull. Rev. 27, 465–482. doi: 10.3758/s13423-019-01694-7
Hedenius, M., Persson, J., Alm, P. A., Ullman, M. T., Howard, J. H., Howard, D. V., et al. (2013). Impaired implicit sequence learning in children with developmental dyslexia. Res. Dev. Disabil. 34, 3924–3935. doi: 10.1016/j.ridd.2013.08.014
Hernández Armenta, I., de la Garza Becerra, J., and Dominguez, A. (2019). “Towards a full integration of physics and math concepts: words versus meanings” in Presented at the 2019 ASEE Annual Conference & Exposition, ASEE Conferences (Tampa, FL)
Hertanti, A., Retnawati, H., and Wutsqa, D. U. (2019). The role of spatial experience in mental rotation. J. Phys. Conf. Ser. 1320:012043. doi: 10.1088/1742-6596/1320/1/012043
Hinton, E. C., Dymond, S., von Hecker, U., and Evans, C. J. (2010). Neural correlates of relational reasoning and the symbolic distance effect: involvement of parietal cortex. Neuroscience 168, 138–148. doi: 10.1016/j.neuroscience.2010.03.052
Hirsh-Pasek, K., and Golinkoff, R. M. (2003). Einstein never used flash cards. New York, NY: Rodale Books.
Hitchcock, D. (2018). Critical thinking. Stanford Encyclopedia of Philosophy. https://plato.stanford.edu/entries/critical-thinking/?fbclid=IwAR3qb0fbDRba0y17zj7xEfO79o1erD-h9a-VHDebal73R1avtCQCNrFDwK8
Hoff, E. (2009). “Language development at an early age: learning mechanisms and outcomes from birth to five years” in Encyclopedia on early childhood development. eds. R. E. Tremblay, M. Boivin, R. D. V. Peters, and S. Rvachew (Montreal: Centre of Excellence for Early Childhood Development and Strategic Knowledge Cluster on Early Child Development), 1–5.
Hoff, E. (2013). Interpreting the early language trajectories of children from low-SES and language minority homes: implications for closing achievement gaps. Dev. Psychol. 49, 4–14. doi: 10.1037/a0027238
Holland, D., Chang, L., Ernst, T. M., Curran, M., Buchthal, S. D., Alicata, D., et al. (2014). Structural growth trajectories and rates of change in the first 3 months of infant brain development. JAMA Neurol. 71:1266. doi: 10.1001/jamaneurol.2014.1638
Holloway, I. D., Battista, C., Vogel, S. E., and Ansari, D. (2013). Semantic and perceptual processing of number symbols: evidence from a cross-linguistic fMRI adaptation study. J. Cogn. Neurosci. 25, 388–400. doi: 10.1162/jocn_a_00323
Hou, C., Pettet, M. W., Vildavski, V. Y., and Norcia, A. M. (2006). Neural correlates of shape-from-shading. Vis. Res. 46, 1080–1090. doi: 10.1016/j.visres.2005.10.017
Hubbard, E. M., Piazza, M., Pinel, P., and Dehaene, S. (2009). “Numerical and spatial intuitions: a role for posterior parietal cortex?” in Cognitive biology: Evolutionary and developmental perspectives on mind, brain, and behavior. eds. L. Tommasi, M. A. Peterson, and L. Nadel (Cambridge: MIT Press), 221–246.
Hulme, C., Monk, A., and Ives, S. (1987). Some experimental studies of multi-sensory teaching: the effects of manual tracing on children’s paired-associate learning. Br. J. Dev. Psychol. 5, 299–307. doi: 10.1111/j.2044-835X.1987.tb01066.x
Hung, Y.-H., Pallier, C., Dehaene, S., Lin, Y.-C., Chang, A., Tzeng, O. J.-L., et al. (2015). Neural correlates of merging number words. Neuroimage 122, 33–43. doi: 10.1016/j.neuroimage.2015.07.045
Hunt, J. H. (2011). The effects of a ratio-based teaching sequence on performance in fraction equivalency for students with mathematics disabilities [doctoral dissertation, University of Central Florida, Orlando]. Available at: https://stars.library.ucf.edu/etd/1941/
Hupp, J. M., and Jungers, M. K. (2013). Beyond words: comprehension and production of pragmatic prosody in adults and children. J. Exp. Child Psychol. 115, 536–551. doi: 10.1016/j.jecp.2012.12.012
Hurschler, M. A. (2015). Neural correlates of sentence-level rhyme processing. [doctoral dissertation, University of Zurich]. Available at: https://www.zora.uzh.ch/id/eprint/164489/
Hutton, J. S., Dudley, J., Horowitz-Kraus, T., DeWitt, T., and Holland, S. K. (2020). Differences in functional brain network connectivity during stories presented in audio, illustrated, and animated format in preschool-age children. Brain Imaging Behav. 14, 130–141. doi: 10.1007/s11682-018-9985-y
Immordino-Yang, M. H. (2015). Emotions, learning, and the brain: exploring the educational implications of affective neuroscience (the Norton series on the social neuroscience of education). New York, NY: W. W. Norton & Company.
Immordino-Yang, M. H., Darling-Hammond, L., and Krone, C. R. (2019). Nurturing nature: how brain development is inherently social and emotional, and what this means for education. Educ. Psychol. 54, 185–204. doi: 10.1080/00461520.2019.1633924
Immordino-Yang, M. H., and Knecht, D. R. (2020). Building meaning builds teens’ brains. Educ. Leadersh. 77, 36–43.
Ischebeck, A., Zamarian, L., Schocke, M., and Delazer, M. (2009). Flexible transfer of knowledge in mental arithmetic—an fMRI study. Neuroimage 44, 1103–1112. doi: 10.1016/j.neuroimage.2008.10.025
Jacob, S. N., Vallentin, D., and Nieder, A. (2012). Relating magnitudes: the brain’s code for proportions. TiCS 16, 157–166. doi: 10.1016/j.tics.2012.02.002
Jansen, P., and Heil, M. (2010). The relation between motor development and mental rotation ability in 5-to 6-year-old children. Int. J. Dev. Sci. 4, 67–75. doi: 10.3233/DEV-2010-4105
Jia, X., Liang, P., Lu, J., Yang, Y., Zhong, N., and Li, K. (2011). Common and dissociable neural correlates associated with component processes of inductive reasoning. Neuroimage 56, 2292–2299. doi: 10.1016/j.neuroimage.2011.03.020
Johnson, E. J., Avineri, N., and Johnson, D. C. (2017). Exposing gaps in/between discourses of linguistic deficits. Int. Multiling. Res. J. 11, 5–22. doi: 10.1080/19313152.2016.1258185
Johnson, J. D., McDuff, S. G. R., Rugg, M. D., and Norman, K. A. (2009). Recollection, familiarity, and cortical reinstatement: a multivoxel pattern analysis. Neuron 63, 697–708. doi: 10.1016/j.neuron.2009.08.011
Judd, N., and Klingberg, T. (2021). Training spatial cognition enhances mathematical learning in a randomized study of 17,000 children. Nat. Hum. Behav. 5, 1548–1554. doi: 10.1038/s41562-021-01118-4
Jung, S., Halm, K., Huber, W., Willmes, K., and Klein, E. (2015). What letters can “learn” from Arabic digits – fMRI-controlled single case therapy study of peripheral agraphia. Brain Lang. 149, 13–26. doi: 10.1016/j.bandl.2015.06.003
Kalenine, S., Pinet, L., and Gentaz, E. (2011). The visual and visuo-haptic exploration of geometrical shapes increases their recognition in preschoolers. Int. J. Behav. 35, 18–26. doi: 10.1177/0165025410367443
Kansaku, K., Johnson, A., Grillon, M.-L., Garraux, G., Sadato, N., and Hallett, M. (2006). Neural correlates of counting of sequential sensory and motor events in the human brain. Neuroimage 31, 649–660. doi: 10.1016/j.neuroimage.2005.12.023
Karmiloff-Smith, A. (2009). Preaching to the converted? From constructivism to neuroconstructivism. Child Dev. Perspect. 3, 99–102. doi: 10.1111/j.1750-8606.2009.00086.x
Karmiloff-Smith, A., Thomas, M. S., and Johnson, M. H. (2018). Thinking developmentally from constructivism to neoconstructivism: the selected works of Annette Karmiloff-Smith. New York: Routledge.
Kartalkanat, H., and Göksun, T. (2020). The effects of observing different gestures during storytelling on the recall of path and event information in 5-year-olds and adults. J. Exp. Child Psychol. 189:104725. doi: 10.1016/j.jecp.2019.104725
Katzir, T., Misra, M., and Poldrack, R. A. (2005). Imaging phonology without print: assessing the neural correlates of phonemic awareness using fMRI. Neuroimage 27, 106–115. doi: 10.1016/j.neuroimage.2005.04.013
Kaufmann, L., Koppelstaetter, F., Delazer, M., Siedentopf, C., Rhomberg, P., Golaszewski, S., et al. (2005). Neural correlates of distance and congruity effects in a numerical Stroop task: an event-related fMRI study. Neuroimage 25, 888–898. doi: 10.1016/j.neuroimage.2004.12.041
Kaufmann, L., Koppelstaetter, F., Siedentopf, C., Haala, I., Haberlandt, E., Zimmerhackl, L.-B., et al. (2006). Neural correlates of the number-size interference task in children. Neuroreport 17, 587–591. doi: 10.1097/00001756-200604240-00007
Kaufmann, L., Vogel, S. E., Starke, M., Kremser, C., and Schocke, M. (2009). Numerical and non-numerical ordinality processing in children with and without developmental dyscalculia: evidence from fMRI. Cogn. Dev. 24, 486–494. doi: 10.1016/j.cogdev.2009.09.001
Kemény, F., Banfi, C., Gangl, M., Perchtold, C. M., Papousek, I., Moll, K., et al. (2018). Print-, sublexical and lexical processing in children with reading and/or spelling deficits: an ERP study. J. Psychophysiol. 130, 53–62. doi: 10.1016/j.ijpsycho.2018.05.009
Khandaker, S. (2015). Neural correlates of verbal communication using infant directed speech in language acquisition: an fNIRS investigation. [doctoral dissertation, University of Michigan]. Available at: https://deepblue.lib.umich.edu/bitstream/handle/2027.42/112155/shaimakr.pdf?sequence=1
Kibbe, M. M., and Feigenson, L. (2015). Young children ‘solve for x’ using the approximate number system. Dev. Sci. 18, 38–49. doi: 10.1111/desc.12177
Kidd, C., Piantadosi, S. T., and Aslin, R. N. (2012). The goldilocks effect: human infants allocate attention to visual sequences that are neither too simple nor too complex. PLoS One 7:e36399. doi: 10.1371/journal.pone.0036399
Klein, C. C., Berger, P., Goucha, T., Friederici, A. D., and Grosse Wiesmann, C. (2022). Children’s syntax is supported by the maturation of BA44 at 4 years, but of the posterior STS at 3 years of age. Cereb. Cortex 2022:bhac430. doi: 10.1093/cercor/bhac430
Klein, A., and Starkey, P. (2004). “Fostering preschool children’s mathematical knowledge: findings from the Berkeley math readiness project” in Engaging young children in mathematics: standards for early childhood mathematics education. eds. D. H. Clements and J. Sarama (New Jersey, NY: Lawrence Erlbaum Associates Publishers), 343–360.
Klein, E., Suchan, J., Moeller, K., Karnath, H.-O., Knops, A., Wood, G., et al. (2014). Considering structural connectivity in the triple code model of numerical cognition: differential connectivity for magnitude processing and arithmetic facts. Brain Struct. Funct. 221, 979–995. doi: 10.1007/s00429-014-0951-1
Knops, A. (2006). On the structure and neural correlates of the numerical magnitude representation and its influence in the assessment of verbal working memory [doctoral dissertation, Aquisgrán, Technical University]. Available at: http://publications.rwth-aachen.de/record/52100/files/Knops_Andre.pdf
Knops, A. (2017). Probing the neural correlates of number processing. Neuroscientist 23, 264–274. doi: 10.1177/1073858416650153
Knops, A., and Willmes, K. (2014). Numerical ordering and symbolic arithmetic share frontal and parietal circuits in the right hemisphere. Neuroimage 84, 786–795. doi: 10.1016/j.neuroimage.2013.09.037
Knouse, L. (2006). fMRI activation during mental rotation. ADHD Rep. 14:12. doi: 10.1521/adhd.2006.14.6.12
Kokkinaki, T., and Vitalaki, E. (2013). Exploring spontaneous imitation in infancy: a three generation inter-familial study. EJOP 9, 259–275. doi: 10.5964/ejop.v9i2.506
Kozhevnikov, M., and Blazhenkova, O. (2013). “Individual differences in object versus spatial imagery: from neural correlates to real-world applications” in Multisensory imagery. eds. S. Lacey and R. Lawson (New York, NY: Springer), 299–318.
Kraut, C., and Pixner, S. (2023). Language does arithmetic: linguistic differences in children’s place-value processing. Psychol. Res. 87, 152–160. doi: 10.1007/s00426-022-01653-3
Kroeger, L. (2012). Neural correlates of error detection in math facts [doctoral dissertation, University of Cincinnati]. Available at: https://www.proquest.com/openview/6c07742ec2279075fdb25fbaf43e5506/1?pq-origsite=gscholar&cbl=18750
Kucian, K., von Aster, M., Loenneker, T., Dietrich, T., Mast, F. W., and Martin, E. (2007). Brain activation during mental rotation in school children and adults. J. Neural Transm. 114, 675–686. doi: 10.1007/s00702-006-0604-5
Kuhl, P. K. (2011). Early language learning and literacy: neuroscience implications for education. MBE 5, 128–142. doi: 10.1111/j.1751-228X.2011.01121.x
Kuhn, D., Black, J., Keselman, A., and Kaplan, D. (2000). The development of cognitive skills to support inquiry learning. Cogn. Instr. 18, 495–523. doi: 10.1207/S1532690XCI1804_3
Lambert, K., and Moeller, K. (2019). Place-value computation in children with mathematics difficulties. J. Exp. Child Psychol. 178, 214–225. doi: 10.1016/j.jecp.2018.09.008
Lamme, V. A. F. (2006). Towards a true neural stance on consciousness. TiCS 10, 494–501. doi: 10.1016/j.tics.2006.09.001
Larrison, A. L. (2013). Mind, brain and education as a framework for curricular reform [doctoral dissertation, University of California, San Diego]. Available at: https://www.proquest.com/openview/5a609eca9d24561bd7c21926fb7571c8/1?pq-origsite=gscholar&cbl=18750
LaValley, D. (2022). Equivocating on unconsciousness. Theor. Psychol 32, 521–534. doi: 10.1177/09593543221092708
Lee, K., Lim, Z. Y., Yeong, S. H. M., Ng, S. F., Venkatraman, V., and Chee, M. W. L. (2007). Strategic differences in algebraic problem solving: neuroanatomical correlates. Brain Res. 1155, 163–171. doi: 10.1016/j.brainres.2007.04.040
LeFevre, J.-A., and Morris, J. (1999). More on the relation between division and multiplication in simple arithmetic: evidence for mediation of division solutions via multiplication. Mem. Cogn. 27, 803–812. doi: 10.3758/BF03198533
Lehne, M., Engel, P., Rohrmeier, M., Menninghaus, W., Jacobs, A. M., and Koelsch, S. (2015). Reading a suspenseful literary text activates brain areas related to social cognition and predictive inference. PLoS One 10:e0124550. doi: 10.1371/journal.pone.0124550
Li, L., Gow, A. D. I., and Zhou, J. (2020). The role of positive emotions in education: a neuroscience perspective. MBE 14, 220–234. doi: 10.1111/mbe.12244
Li, H., Wu, D., Yang, J., Xie, S., Luo, J., and Chang, C. (2021a). A functional near-infrared spectroscopy examination of the neural correlates of cognitive shifting in dimensional change card sort task. Front. Hum. Neurosci. 14:561223. doi: 10.3389/fnhum.2020.561223
Li, J., Yang, Y., Viñas-Guasch, N., Yang, Y., and Bi, H. Y. (2023). Differences in brain functional networks for audiovisual integration during reading between children and adults. Ann. N. Y. Acad. Sci. 1520, 127–139. doi: 10.1111/nyas.14943
Li, H., Zhang, J., and Ding, G. (2021b). Reading across writing systems: a meta-analysis of the neural correlates for first and second language reading. BLC 24, 537–548. doi: 10.1017/S136672892000070X
Liang, B., and Du, Y. (2018). The functional neuroanatomy of lexical tone perception: an activation likelihood estimation Meta-analysis. Front. Neurosci. 12:495. doi: 10.3389/fnins.2018.00495
Lin, C.-L., Jung, M., Wu, Y. C., She, H.-C., and Jung, T.-P. (2015). Neural correlates of mathematical problem solving. Int. J. Neur. Syst. 25:1550004. doi: 10.1142/S0129065715500045
Lin, J., Wen, X., Cui, X., Xiang, Y., Xie, J., Chen, Y., et al. (2021). Common and specific neural correlates underlying insight and ordinary problem solving. Brain Imaging Behav. 15, 1374–1387. doi: 10.1007/s11682-020-00337-z
Linsen, S., Verschaffel, L., Reynvoet, B., and De Smedt, B. (2015). The association between numerical magnitude processing and mental versus algorithmic multi-digit subtraction in children. Learn. Instruct. 35, 42–50. doi: 10.1016/j.learninstruc.2014.09.003
List, S. M. (2019). The sound of meaning: physical, perceptual, and neural correlates of sound to shape mapping in natural language. [doctoral dissertation, Emory University]. Available at: https://www.proquest.com/openview/0b7e12d64f09e0314a01a269ed1e13e8/1?pq-origsite=gscholar&cbl=18750&diss=y
Liu, F. (2016). Anxiety towards teaching mathematics and science: correlation, prevalence, and intensity. J. Math. Educ. 9, 29–46.
Loch, C., Lindmeier, A. M., and Heinze, A. (2015). “The missing link? – School-related content knowledge of pre-service mathematics teachers” in Proceedings of the 39th Conference of the International Group for the Psychology of Mathematics Education. eds. K. Beswick, T. Muir, and J. Fielding-Wells (Hobart: PME), 209–216.
Longcamp, M., Tanskanen, T., and Hari, R. (2006). The imprint of action: motor cortex involvement in visual perception of handwritten letters. Neuroimage 33, 681–688. doi: 10.1016/j.neuroimage.2006.06.042
Louleli, N., Hämäläinen, J. A., Nieminen, L., Parviainen, T., and Leppänen, P. H. (2022). Neural correlates of morphological processing and its development from pre-school to the first grade in children with and without familial risk for dyslexia. J. Neurolinguistics 61:101037. doi: 10.1016/j.jneuroling.2021.101037
Lourenco, S. F., and Longo, M. R. (2011). “Origins and development of generalized magnitude representation” in Space, time and number in the brain: searching for the foundations of mathematical thought. eds. S. Dehaene and E. M. Brannon (London: Elsevier), 225–244.
Luinge, M. R., Post, W. J., Wit, H. P., and Goorhuis-Brouwer, S. M. (2006). The ordering of milestones in language development for children from 1 to 6 years of age. J. Speech Lang. Hear. Res. 49, 923–940. doi: 10.1044/1092-4388(2006/067)
Lund, B. D., Wang, T., Mannuru, N. R., Nie, B., Shimray, S., and Wang, Z. (2023). CHATGPT and a new academic reality: artificial intelligence-written research papers and the ethics of the large language models in scholarly publishing. JASIST 74, 24750. doi: 10.1002/asi.24750
Lyons, I. M., and Ansari, D. (2015). Foundations of children’s numerical and mathematical skills: the roles of symbolic and nonsymbolic representations of numerical magnitude. Adv. Child Dev. Behav. 48, 93–116. doi: 10.1016/bs.acdb.2014.11.003
Lyons, I. M., and Beilock, S. L. (2013). Ordinality and the nature of symbolic numbers. J. Neurosci. 33, 17052–17061. doi: 10.1523/JNEUROSCI.1775-13.2013
Lyons, I. M., Vogel, S., and Ansari, D. (2016). On the ordinality of numbers: a review of neural and behavioral studies. Prog. Brain Res. 227, 187–221. doi: 10.1016/bs.pbr.2016.04.010
Marchand, E., and Barner, D. (2018). “Analogical mapping in numerical development” in Language and culture in mathematical cognition. eds. D. C. Geary, K. M. Koepke, and D. B. Berch (London, UK: Academic Press), 31–47.
Mareschal, D., Johnson, M. H., Sirois, S., Spratling, M., Thomas, M. S., and Westermann, G. (2007). Neuroconstructivism-I: how the brain constructs cognition. New York, NY: Oxford University Press.
Marshall, P. J., Young, T., and Meltzoff, A. N. (2011). Neural correlates of action observation and execution in 14-month-old infants: an event-related EEG desynchronization study: infant EEG desynchronization. Dev. Sci. 14, 474–480. doi: 10.1111/j.1467-7687.2010.00991.x
Martin, M. O., and Mullis, I. V. S. (Eds.). (2013). TIMSS and PIRLS international study center. Chestnut Hill, MA: Boston College.
Masataka, N., Ohnishi, T., Imabayashi, E., Hirakata, M., and Matsuda, H. (2006). Neural correlates for numerical processing in the manual mode. JDSDE 11, 144–152. doi: 10.1093/deafed/enj017
Masataka, N., Ohnishi, T., Imabayashi, E., Hirakata, M., and Matsuda, H. (2007). Neural correlates for learning to read Roman numerals. Brain Lang. 100, 276–282. doi: 10.1016/j.bandl.2006.11.011
Mathur, A., Schultz, D., and Wang, Y. (2020). Neural bases of phonological and semantic processing in early childhood. Brain Connect. 10, 212–223. doi: 10.1089/brain.2019.0728
Mayer, C., Wallner, S., Budde-Spengler, N., Braunert, S., Arndt, P. A., and Kiefer, M. (2020). Literacy training of kindergarten children with pencil, keyboard or tablet stylus: the influence of the writing tool on reading and writing performance at the letter and word level. Front. Psychol. 10:3054. doi: 10.3389/fpsyg.2019.03054
Mazza, V., and Caramazza, A. (2015). Multiple object individuation and subitizing in enumeration: a view from electrophysiology. Front. Hum. Neurosci. 9:162. doi: 10.3389/fnhum.2015.00162
McDuffie, A., and Haebig, E. (2013). “Language development survey” in Encyclopedia of autism Spectrum disorders. ed. F. R. Volkmar (Berlin: Springer), 478.
McLaughlin, E. (2000). Haptic to visual cross-modal shape perception in preschoolers [doctoral dissertation, Rutgers the State University of new Jersey and University of medicine and dentistry of New Jersey]. Available at: https://www.proquest.com/openview/7ab9ba1bbf89be70c1d9aab7a4a60aba/1?pq-origsite=gscholar&cbl=18750&diss=y
Melinder, A. M. D., Konijnenberg, C., Hermansen, T., Daum, M. M., and Gredebäck, G. (2015). The developmental trajectory of pointing perception in the first year of life. Exp. Brain Res. 233, 641–647. doi: 10.1007/s00221-014-4143-2
Meng, X., and Moriguchi, Y. (2021). Neural basis for egalitarian sharing in five-to six-year-old children. Neuropsychologia 154:107787. doi: 10.1016/j.neuropsychologia.2021.107787
Mestres Missé, A. (2007). Neural correlates of word learning and meaning acquisition [doctoral dissertation, Universitat de Barcelona]. Available at: https://diposit.ub.edu/dspace/handle/2445/42699
Metcalfe, A. W. S., Ashkenazi, S., Rosenberg-Lee, M., and Menon, V. (2013). Fractionating the neural correlates of individual working memory components underlying arithmetic problem solving skills in children. Dev. Cogn. Neurosci. 6, 162–175. doi: 10.1016/j.dcn.2013.10.001
Mezirow, J. (2018). “Transformative learning theory” in Contemporary theories of learning. ed. K. Illeris (London: Routledge), 114–128.
Miller, E. K., Nieder, A., Freedman, D. J., and Wallis, J. D. (2003). Neural correlates of categories and concepts. Curr. Opin. Neurobiol. 13, 198–203. doi: 10.1016/S0959-4388(03)00037-0
Mishra, P., and Koehler, M. J. (2006). Technological pedagogical content knowledge: a framework for teacher knowledge. Teach. Coll. Rec. 108, 1017–1054. doi: 10.1111/j.1467-9620.2006.00684.x
Misra, M., Katzir, T., Wolf, M., and Poldrack, R. A. (2004). Neural systems for rapid automatized naming in skilled readers: unraveling the RAN-reading relationship. Sci. Stud. Read. 8, 241–256. doi: 10.1207/s1532799xssr0803_4
Missall, K., Hojnoski, R. L., Caskie, G. I., and Repasky, P. (2015). Home numeracy environments of preschoolers: examining relations among mathematical activities, parent mathematical beliefs, and early mathematical skills. Early Educ. Dev. 26, 356–376. doi: 10.1080/10409289.2015.968243
Misyak, J. B., Christiansen, M. H., and Bruce Tomblin, J. (2010). Sequential expectations: the role of prediction-based learning in language. Top. Cogn. Sci. 2, 138–153. doi: 10.1111/j.1756-8765.2009.01072.x
Mix, K. S. (1999). Similarity and numerical equivalence. Cogn. Dev. 14, 269–297. doi: 10.1016/S0885-2014(99)00005-2
Möller, K. (2010). The influence of the place-value structure of the Arabic number system on two-digit number processing-Representational, developmental, neuropsychological and computational aspects (Doctoral dissertation, Universität Tübingen).
Monaghan, P., and Pollmann, S. (2003). Division of labor between the hemispheres for complex but not simple tasks: an implemented connectionist model. J. Exp. Psychol. 132, 379–399. doi: 10.1037/0096-3445.132.3.379
Moon, T. R., Brighton, C. M., and Tomlinson, C. A. (2020). Using differentiated classroom assessment to enhance student learning. New York, NY: Routledge.
Morrill, T. H., McAuley, J. D., Dilley, L. C., and Hambrick, D. Z. (2015). Individual differences in the perception of melodic contours and pitch-accent timing in speech: support for domain-generality of pitch processing. J. Exp. Psychol. 144, 730–736. doi: 10.1037/xge0000081
Morris, A. S., Jespersen, J. E., Cosgrove, K. T., Ratliff, E. L., and Kerr, K. L. (2020). Parent education: what we know and moving forward for greatest impact. Fam. Relat. 69, 520–542. doi: 10.1111/fare.12442
Mussolin, C., De Volder, A., Grandin, C., Schlögel, X., Nassogne, M.-C., and Noël, M.-P. (2010). Neural correlates of symbolic number comparison in developmental dyscalculia. J. Cogn. Neurosci. 22, 860–874. doi: 10.1162/jocn.2009.21237
Mussolin, C., Noël, M.-P., Pesenti, M., Grandin, C., and De Volder, A. G. (2013). Neural correlates of the numerical distance effect in children. Front. Psychol. 4:663. doi: 10.3389/fpsyg.2013.00663
Nakamura, K., Kuo, W.-J., Pegado, F., Cohen, L., Tzeng, O. J. L., and Dehaene, S. (2012). Universal brain systems for recognizing word shapes and handwriting gestures during reading. Proc. Natl. Acad. Sci. U. S. A. 109, 20762–20767. doi: 10.1073/pnas.1217749109
Nan, Y., Knösche, T. R., and Luo, Y.-J. (2006). Counting in everyday life: discrimination and enumeration. Neuropsychologia 44, 1103–1113. doi: 10.1016/j.neuropsychologia.2005.10.020
National Academies of Sciences, Engineering, and Medicine (2018). How people learn II: learners, contexts, and cultures. Washington, DC: National Academies Press.
Neubauer, A. C., Bergner, S., and Schatz, M. (2010). Two-vs. three-dimensional presentation of mental rotation tasks: sex differences and effects of training on performance and brain activation. Intelligence 38, 529–539. doi: 10.1016/j.intell.2010.06.001
Newcombe, N. S., Uttal, D. H., and Sauter, M. (2013). “Spatial development” in The Oxford handbook of developmental psychology (vol. 1): body and mind. ed. P. D. Zalazo (New York, NY: Oxford University Press), 564–590.
Nieder, A. (2005). Counting on neurons: the neurobiology of numerical competence. Nat. Rev. Neurosci. 6, 177–190. doi: 10.1038/nrn1626
Norton, E. S. (2012). Using cognitive neuroscience to examine the brain basis of pre-reading skills in kindergarten children and subtypes of risk for dyslexia: toward MRI and EEG prediction of reading outcomes. [doctoral dissertation, Tufts University]. Available at: https://www.proquest.com/openview/6ba7a71068ddc1a016e3cb4fe3fbb70c/1?pq-origsite=gscholar&cbl=18750
Notebaert, K., Nelis, S., and Reynvoet, B. (2011). The magnitude representation of small and large symbolic numbers in the left and right hemisphere: an event-related fMRI study. J. Cogn. Neurosci. 23, 622–630. doi: 10.1162/jocn.2010.21445
Nouri, A., Tokuhama-Espinosa, T. N., and Borja, C. (2022). Crossing mind, brain, and education boundaries. UK: Cambridge Scholars Publishing.
Oberecker, R., Friedrich, M., and Friederici, A. D. (2005). Neural correlates of syntactic processing in two-year-olds. J. Cogn. Neurosci. 17, 1667–1678. doi: 10.1162/089892905774597236
Olkun, S., Altun, A., Göçer Şahin, S., and Akkurt Denizli, Z. (2015). Deficits in basic number competencies may cause low numeracy in primary school children. Educ. Sci. 40, 141–159. doi: 10.15390/EB.2015.3287
Ongchoco, J. D. K., and Scholl, B. J. (2019). How to create objects with your mind: from object-based attention to attention-based objects. Psychol. Sci. 30, 1648–1655. doi: 10.1177/0956797619863072
Ons, B., and Wagemans, J. (2012). Generalization of visual shapes by flexible and simple rules. Seeing Perceiving 25, 237–261. doi: 10.1163/187847511X571519
Oosthuizen, I., Frisby, C., Chadha, S., Manchaiah, V., and Swanepoel, D. W. (2023). Combined hearing and vision screening programs: a scoping review. Front. Public Health 11:1119851. doi: 10.3389/fpubh.2023.1119851
Orban, P., Peigneux, P., Lungu, O., Debas, K., Barakat, M., Bellec, P., et al. (2011). Functional neuroanatomy associated with the expression of distinct movement kinematics in motor sequence learning. Neuroscience 179, 94–103. doi: 10.1016/j.neuroscience.2011.01.040
Orechwa, A. Z. (2009). The neural correlates of skilled reading: an MRI investigation of phonological processing. [doctoral dissertation, University of Southern California]. Available at: https://www.proquest.com/openview/b58ba0f808d962613cac42d4c95c444a/1?pq-origsite=gscholar&cbl=18750
Organisation for Economic Cooperation and Development. (2020). TALIS 2018 results (volume II): teachers and school leaders as valued professionals. Paris: OECD Publishing.
Organisation for Economic Cooperation and Development. (2021). Education at a glance, 2021. Paris: Author.
Osher, D., Cantor, P., Berg, J., Steyer, L., and Rose, T. (2020). Drivers of human development: how relationships and context shape learning and development1. Appl. Dev. Sci. 24, 6–36. doi: 10.1080/10888691.2017.1398650
Palmis, S., Danna, J., Velay, J.-L., and Longcamp, M. (2017). Motor control of handwriting in the developing brain: a review. Cogn. Neuropsychol. 34, 187–204. doi: 10.1080/02643294.2017.1367654
Paolini, A. (2015). Enhancing teaching effectiveness and student learning outcomes. J. Effect. Teach. 15, 20–33.
Pariyadath, V., Plitt, M. H., Churchill, S. J., and Eagleman, D. M. (2012). Why overlearned sequences are special: distinct neural networks for ordinal sequences. Front. Hum. Neurosci. 6:328. doi: 10.3389/fnhum.2012.00328
Park, Y. (2020). Effects of age, spatial relation and object type on Young Children’s analogical transfer of spatial relations. Korean J. Child Stud. 41, 81–93. doi: 10.5723/kjcs.2020.41.3.81
Pedott, P. R., Cáceres-Assenço, A. M., and Befi-Lopes, D. M. (2017). Habilidades de aliteração e rima em crianças com distúrbio específico de linguagem. CoDAS 29:1. doi: 10.1590/2317-1782/20172016017
Peeters, D., Snijders, T. M., Hagoort, P., and Özyürek, A. (2017). Linking language to the visual world: neural correlates of comprehending verbal reference to objects through pointing and visual cues. Neuropsychologia 95, 21–29. doi: 10.1016/j.neuropsychologia.2016.12.004
Perkins, D. N. (2009). Making learning whole: how seven principles of teaching can transform education. San Francisco, CA: Jossey-Bass.
Peters, S., van Atteveldt, N., Massonnié, J., and Vogel, S. E. (Eds.). (2020). Everything you and your teachers need to know about the learning brain. SA: Frontiers Media.
Peters, L. (2016). What counts in the brain? The neural correlates of arithmetic in adults and children with and without learning disorders. [doctoral dissertation, University Ku Leuven]. Available at: https://lirias.kuleuven.be/1788148?limo=0
Petitto, L. A., Berens, M. S., Kovelman, I., Dubins, M. H., Jasinska, K., and Shalinsky, M. (2012). The “perceptual wedge hypothesis” as the basis for bilingual babies’ phonetic processing advantage: new insights from fNIRS brain imaging. Brain Lang. 121, 130–143. doi: 10.1016/j.bandl.2011.05.003
Piaget, J. (1968). Le Point De Vue De Piaget. Int. J. Psychol. 3, 281–299. doi: 10.1080/00207596808246651
Piazza, M., Giacomini, E., Le Bihan, D., and Dehaene, S. (2003). Single-trial classification of parallel pre-attentive and serial attentive processes using functional JMRI. Proc. R. Soc. Lond. B 270, 1237–1245. doi: 10.1098/rspb.2003.2356
Piazza, M., Izard, V., Pinel, P., Le Bihan, D., and Dehaene, S. (2004). Tuning curves for approximate numerosity in the human intraparietal sulcus. Neuron 44, 547–555. doi: 10.1016/j.neuron.2004.10.014
Piazza, M., Mechelli, A., Price, C. J., and Butterworth, B. (2006). Exact and approximate judgements of visual and auditory numerosity: an fMRI study. Brain Res. 1106, 177–188. doi: 10.1016/j.brainres.2006.05.104
Pinker, S. (2009). Language learnability and language development: with new commentary by the author. Cambridge: Harvard University Press.
Pishnamazi, M., Nojaba, Y., Ganjgahi, H., Amousoltani, A., and Oghabian, M. A. (2016). Neural correlates of audiotactile phonetic processing in early-blind readers: an fMRI study. Exp. Brain Res. 234, 1263–1277. doi: 10.1007/s00221-015-4515-2
Pollen, D. A. (1999). On the neural correlates of visual perception. Cerebellum 9, 4–19. doi: 10.1093/cercor/9.1.4
Portnoy, S., Rosenberg, L., Alazraki, T., Elyakim, E., and Friedman, J. (2015). Differences in muscle activity patterns and graphical product quality in children copying and tracing activities on horizontal or vertical surfaces. J. Electromyogr. Kinesiol. 25, 540–547. doi: 10.1016/j.jelekin.2015.01.011
Postman, N., and Weingartner, C. (1969). “Meaning making” in Teaching as a subversive activity (New York, NY: Delacorte Press), 82–97.
Powers, S. J., Wang, Y., Beach, S. D., Sideridis, G. D., and Gaab, N. (2016). Examining the relationship between home literacy environment and neural correlates of phonological processing in beginning readers with and without a familial risk for dyslexia: an fMRI study. Ann. Dyslexia 66, 337–360. doi: 10.1007/s11881-016-0134-2
Prado, J., Lu, J., Liu, L., Dong, Q., Zhou, X., and Booth, J. R. (2013). The neural bases of the multiplication problem-size effect across countries. Front. Hum. Neurosci. 7:189. doi: 10.3389/fnhum.2013.00189
Prado, J., Mutreja, R., and Booth, J. R. (2014). Developmental dissociation in the neural responses to simple multiplication and subtraction problems. Dev. Sci. 17, 537–552. doi: 10.1111/desc.12140
Price, G. R., Mazzocco, M. M. M., and Ansari, D. (2013). Why mental arithmetic counts: brain activation during single digit arithmetic predict high school math scores. J. Neurosci. 33, 156–163. doi: 10.1523/JNEUROSCI.2936-12.2013
Procter, H. G. (2011). “The roots of Kellian notions in philosophy: the categorial philosophers—Kant, Hegel, and Peirce” in Personal construct psychology in an accelerating world. eds. D. Stojnov, V. Džinović, J. Pavlović, and M. Frances (Bellagrade: Serbian Constructivist Association), 29–46.
Purcell, J. J., Napoliello, E. M., and Eden, G. F. (2011a). A combined fMRI study of typed spelling and reading. Neuroimage 55, 750–762. doi: 10.1016/j.neuroimage.2010.11.042
Purcell, J. J., Turkeltaub, P. E., Eden, G. F., and Rapp, B. (2011b). Examining the central and peripheral processes of written word production through meta-analysis. Front. Psychol. 2:239. doi: 10.3389/fpsyg.2011.00239
Rawlings, C. M., and Childress, C. (2021). Schemas, interactions, and objects in meaning making 1. Sociol. Forum 36, 1446–1477. doi: 10.1111/socf.12759
Richards, T. L., Berninger, V. W., and Fayol, M. (2012). “The writing brain of normal child writers and children with writing disabilities: generating ideas and transcribing them through the orthographic loop” in Writing: a mosaic of perspectives and views. eds. E. Grigorenko, E. Mambrino, and D. Preiss (New York, NY: Psychology Press), 85–105.
Ringel, B. A., and Springer, C. J. (1980). On knowing how well one is remembering: the persistence of strategy use during transfer. J. Exp. Child Psychol. 29, 322–333. doi: 10.1016/0022-0965(80)90023-5
Romanovska, L., Janssen, R., and Bonte, M. (2021). Cortical responses to letters and ambiguous speech vary with reading skills in dyslexic and typically reading children. Neuroimage 30:102588. doi: 10.1016/j.nicl.2021.102588
Romeo, R. R., Leonard, J. A., Robinson, S. T., West, M. R., Mackey, A. P., Rowe, M. L., et al. (2018). Beyond the 30-million-word gap: children’s conversational exposure is associated with language-related brain function. Psychol. Sci. 29, 700–710. doi: 10.1177/0956797617742725
Rosenberg-Lee, M., Ashkenazi, S., Chen, T., Young, C. B., Geary, D. C., and Menon, V. (2015). Brain hyper-connectivity and operation-specific deficits during arithmetic problem solving in children with developmental dyscalculia. Dev. Sci. 18, 351–372. doi: 10.1111/desc.12216
Rosenberg-Lee, M., Barth, M., and Menon, V. (2011). What difference does a year of schooling make? Neuroimage 57, 796–808. doi: 10.1016/j.neuroimage.2011.05.013
Rosenberg-Lee, M., Lovett, M. C., and Anderson, J. R. (2009). Neural correlates of arithmetic calculation strategies. Cogn. Affect. Behav. Neurosci. 9, 270–285. doi: 10.3758/CABN.9.3.270
Roux, F.-E., Niare, M., van Ierschot, F. C., Durand, J.-B., Miceli, G., and Demonet, J.-F. (2021). “Handwriting” in Intraoperative mapping of cognitive networks. eds. E. Mandonnet and G. Herbet (Cham: Springer International Publishing, Cham), 127–142.
Rubinsten, O., Dana, S., Lavro, D., and Berger, A. (2013). Processing ordinality and quantity: ERP evidence of separate mechanisms. Brain Cogn. 82, 201–212. doi: 10.1016/j.bandc.2013.04.008
Rumberger, R. W., and Lim, S. A. (2008). Why students drop out of school: a review of 25 years of research. Santa Barbara, CA: University of California.
Saby, J. N., Marshall, P. J., and Meltzoff, A. N. (2012). Neural correlates of being imitated: an EEG study in preverbal infants. Soc. Neurosci. 7, 650–661. doi: 10.1080/17470919.2012.691429
Saccuman, M. C., Cappa, S. F., Bates, E. A., Arevalo, A., Della Rosa, P., Danna, M., et al. (2006). The impact of semantic reference on word class: an fMRI study of action and object naming. Neuroimage 32, 1865–1878. doi: 10.1016/j.neuroimage.2006.04.179
Saletta, M. (2019). Orthography and speech production in children with good or poor reading skills. Appl. Psycholinguist. 40, 905–931. doi: 10.1017/S0142716419000055
Sancar, R., Atal, D., and Deryakulu, D. (2021). A new framework for teachers’ professional development. Teach. Teach. Educ. 101:103305. doi: 10.1016/j.tate.2021.103305
Sari, M. H., and Olkun, S. (2020). Developing number sense in students with mathematics learning disability risk. Int. Online J. Prim. Educ. 9, 228–243.
Schaadt, G. (2015). Visual, auditory, and visual-auditory speech processing in school children with writing difficulties. [doctoral dissertation, max Planck Institute for Human Cognitive and Brain Sciences Leipzig, Germany]. Available at: https://pure.mpg.de/rest/items/item_2241336/component/file_2327130/content
Schaffer, J., Chalmers, D., Manley, D., and Wasserman, R. (2009). “On what grounds what” in Metaphysics. eds. J. Kim, D. Z. Korman, and E. Sosa (New Jersey, NJ: Wiley Blackwell), 73–96.
Scherf, K. S., Behrmann, M., Kimchi, R., and Luna, B. (2009). Emergence of global shape processing continues through adolescence. Child Dev. 80, 162–177. doi: 10.1111/j.1467-8624.2008.01252.x
Schipke, C. S., Knoll, L. J., Friederici, A. D., and Oberecker, R. (2012). Preschool children’s interpretation of object-initial sentences: neural correlates of their behavioral performance: children’s interpretation of object-initial sentences. Dev. Sci. 15, 762–774. doi: 10.1111/j.1467-7687.2012.01167.x
Schlegel, A., Alexander, P., Fogelson, S. V., Li, X., Lu, Z., Kohler, P. J., et al. (2015). The artist emerges: visual art learning alters neural structure and function. Neuroimage 105, 440–451. doi: 10.1016/j.neuroimage.2014.11.014
Schmid, R., Pauli, C., Stebler, R., Reusser, K., and Petko, D. (2022). Implementation of technology-supported personalized learning—its impact on instructional quality. J. Educ. Res. 115, 187–198. doi: 10.1080/00220671.2022.2089086
Schmithorst, V. J., and Brown, R. D. (2004). Empirical validation of the triple-code model of numerical processing for complex math operations using functional MRI and group independent component analysis of the mental addition and subtraction of fractions. Neuroimage 22, 1414–1420. doi: 10.1016/j.neuroimage.2004.03.021
Schneider, W., Lockl, K., and Fernandez, O. (2005). “Interrelationships among theory of mind, executive control, language development, and working memory in young children: a longitudinal analysis” in Young children’s cognitive development: interrelationships among executive functioning, working memory, verbal ability, and theory of mind. eds. W. Schneider, R. Schumann-Hengsteler, and B. Sodian (New York, NY: Psychology Press), 259–284.
Schneider, J. M., and Maguire, M. J. (2019). Developmental differences in the neural correlates supporting semantics and syntax during sentence processing. Dev. Sci. 22:e12782. doi: 10.1111/desc.12782
Schouwenaars, A., Hendriks, P., and Ruigendijk, E. (2018). German children’s processing of morphosyntactic cues in wh -questions. Appl. Psycholinguist. 39, 1279–1318. doi: 10.1017/S0142716418000334
Seufert, S., Guggemos, J., and Sailer, M. (2021). Technology-related knowledge, skills, and attitudes of pre-and in-service teachers: the current situation and emerging trends. CHB 115:106552. doi: 10.1016/j.chb.2020.106552
Shulman, L. (1987). Knowledge and teaching: foundations of the new reform. Harv. Educ. Rev. 57, 1–23. doi: 10.17763/haer.57.1.j463w79r56455411
Siegler, R. S., and Booth, J. L. (2004). Development of numerical estimation in young children. Child Dev. 75, 428–444. doi: 10.1111/j.1467-8624.2004.00684.x
Simiona, A. G. (2016). “The evolution of handwriting in primary school. Comparison between types of handwriting” in The European proceedings of social and behavioral sciences. Future Academy: Offering evxcellence in Social and Behavioral Sciences, 2357–1330.
Simos, P. G., Rezaie, R., Fletcher, J. M., Juranek, J., and Papanicolaou, A. C. (2011). Neural correlates of sentence reading in children with reading difficulties. Neuroreport 22, 674–678. doi: 10.1097/WNR.0b013e328349ecf7
Siok, W. T., and Luke, K. K. (2020). Editorial: reading in the digital age: the impact of using digital devices on children’s reading, writing and thinking skills. Front. Psychol. 11:586118. doi: 10.3389/fpsyg.2020.586118
Skagenholt, M., Träff, U., Västfjäll, D., and Skagerlund, K. (2018). Examining the triple code model in numerical cognition: an fMRI study. PLoS One 13:e0199247. doi: 10.1371/journal.pone.0199247
Skerry, A. E., and Saxe, R. (2016). “What neuroscience can reveal about cognition and its origins” in Core knowledge and conceptual change. eds. D. Barner and A. S. Baron (New York, NY: Oxford University Press), 321–334.
Skoning, S. N., Wegner, T., and Mason-Williams, L. (2017). Teaching vocabulary through movement: what are the outcomes for children? J. Res. Child. 31, 1–8. doi: 10.1080/02568543.2016.1242519
Snapp-Childs, W., Fath, A. J., and Bingham, G. P. (2018). Training children aged 5–10 years in compliance control: tracing smaller figures yields better learning not specific to the scale of drawn figures. Exp. Brain Res. 236, 2589–2601. doi: 10.1007/s00221-018-5319-y
Solis-Stovall, L. A. (2020). An analysis of the higher order thinking requirements of PARCC practice assessments in grades 3 and 4. [doctoral dissertation, seton hall university]. Available at: https://www.proquest.com/openview/4da1af1a02838bafa39b3b06d67e5425/1?pq-origsite=gscholar&cbl=18750&diss=y
Sophian, C. (2000). Perceptions of proportionality in young children: matching spatial ratios. Cognition 75, 145–170. doi: 10.1016/S0010-0277(00)00062-7
Soylu, F., Lester, F. K., and Newman, S. D. (2018). You can count on your fingers: the role of fingers in early mathematical development. J. Numer. Cogn. 4, 107–135. doi: 10.5964/jnc.v4i1.85
Sporns, O. (2022). Structure and function of complex brain networks. Dialogues Clin. Neurosci. 15, 247–262. doi: 10.31887/DCNS.2013.15.3/osporns
Steber, S., and Rossi, S. (2020). So young, yet so mature? Electrophysiological and vascular correlates of phonotactic processing in 18-month-olds. Dev. Cogn. Neurosci. 43:100784. doi: 10.1016/j.dcn.2020.100784
Steinemann, N. A., Moisello, C., Ghilardi, M. F., and Kelly, S. P. (2016). Tracking neural correlates of successful learning over repeated sequence observations. Neuroimage 137, 152–164. doi: 10.1016/j.neuroimage.2016.05.001
Sutton, J. E., Joanisse, M. F., and Newcombe, N. S. (2010). Spinning in the scanner: neural correlates of virtual reorientation. J. Exp. Psychol. Learn. Mem. Cogn. 36, 1097–1107. doi: 10.1037/a0019938
Sylvester, T., Liebig, J., and Jacobs, A. M. (2021). Neural correlates of affective contributions to lexical decisions in children and adults. Sci. Rep. 11:945. doi: 10.1038/s41598-020-80359-1
Szczepek Reed, B. (2020). Reconceptualizing mirroring: sound imitation and rapport in naturally occurring interaction. J. Pragmat. 167, 131–151. doi: 10.1016/j.pragma.2020.05.010
Takashima, A., Bakker-Marshall, I., van Hell, J. G., McQueen, J. M., and Janzen, G. (2019). Neural correlates of word learning in children. Dev. Cogn. Neurosci. 37:100649. doi: 10.1016/j.dcn.2019.100649
Takashima, A., Konopka, A., Meyer, A., Hagoort, P., and Weber, K. (2020). Speaking in the brain: the interaction between words and syntax in sentence production. J. Cogn. Neurosci. 32, 1466–1483. doi: 10.1162/jocn_a_01563
Thompson, J. M., Nuerk, H.-C., Moeller, K., and Cohen Kadosh, R. (2013). The link between mental rotation ability and basic numerical representations. Acta Psychol. 144, 324–331. doi: 10.1016/j.actpsy.2013.05.009
Tokuhama-Espinosa, T. (2008). The scientifically substantiated art of teaching: a study in the development of standards in the new academic field of neuroeducation (mind, brain, and education science). [doctoral dissertation, Capella University]. Available at: https://www.proquest.com/openview/117084b972c7dc99bbaed9aac35b8221/1?pq-origsite=gscholar&cbl=18750
Tokuhama-Espinosa, T. (2010). Mind, brain, and education science: a comprehensive guide to the new brain-based teaching. New York, NY: W. W. Norton & Company.
Tokuhama-Espinosa, T. (2014). Making classrooms better: 50 practical applications of mind, brain, and education science. New York, NY: W. W. Norton & Company.
Tokuhama-Espinosa, T. (2017). Teachers’ new pedagogical content knowledge [power point]. Available at: https://docs.google.com/presentation/d/0B8RaPiQPEZ9ZSzZhY0Z2RWI1UG8/edit?resourcekey=0-Wcyg2cF5JlIDHV9FdFTAQQ#slide=id.p1 (Accessed June 30, 2023).
Tokuhama-Espinosa, T. (2019). Five pillars of the mind: redesigning education to suit the brain. New York, NY: W. W. Norton & Company.
Tokuhama-Espinosa, T. (2021a). Bringing the neuroscience of learning to online teaching: an educator’s handbook. New York, NY: Teachers College Press.
Tokuhama-Espinosa, T. (2021b). Neuromyths. Encycl. Behav. Neurosci. 3, 620–631. doi: 10.1016/B978-0-12-809324-5.24101-1
Tokuhama-Espinosa, T., Nouri, A., and Daniel, D. (2020). 2020 international survey-what has MBE taught us about teaching. Available at: https://www.researchgate.net/publication/341959117_2020_International_Survey-What_has_MBE_Taught_Us_About_Teaching_TOKUHAMA_NOURI_DANIELS_June_5_2020_v4 (Accessed April 30, 2023).
Tuominen, M., and Kallio, E. K. (2020). “Logical contradiction, contrary opposites, and epistemological relativism: critical philosophical reflections on the psychological models of adult cognitive development 1” in Development of adult thinking. ed. E. K. Kallio (London: Routledge), 208–229.
Varma, S., McCandliss, B. D., and Schwartz, D. L. (2008). Scientific and pragmatic challenges for bridging education and neuroscience. Educ. Res. 37, 140–152. doi: 10.3102/0013189X08317687
Vendetti, M. S., Matlen, B. J., Richland, L. E., and Bunge, S. A. (2015). Analogical reasoning in the classroom: insights from cognitive science. MBE 9, 100–106. doi: 10.1111/mbe.12080
Venkatraman, V., Ansari, D., and Chee, M. W. L. (2005). Neural correlates of symbolic and non-symbolic arithmetic. Neuropsychologia 43, 744–753. doi: 10.1016/j.neuropsychologia.2004.08.005
Venneri, A., and Semenza, C. (2011). On the dependency of division on multiplication: selective loss for conceptual knowledge of multiplication. Neuropsychologia 49, 3629–3635. doi: 10.1016/j.neuropsychologia.2011.09.017
Veraksa, A., Bukhalenkova, D., Kartushina, N., and Oshchepkova, E. (2020). The relationship between executive functions and language production in 5–6-year-old children: insights from working memory and storytelling. Behav. Sci. 10:52. doi: 10.3390/bs10020052
Veraksa, A. N., Ochepkova, E. S., Bukhalenkova, D. A., and Kartushina, N. A. (2019). The relationship of executive functions and speech production in senior preschool children: working memory and storytelling. Clin. Psychol. Spec. Educ. 8, 56–84. doi: 10.17759/psyclin.2019080304
Verdine, B. N., Bunger, A., Athanasopoulou, A., Golinkoff, R. M., and Hirsh-Pasek, K. (2017). Shape up: an eye-tracking study of preschoolers’ shape name processing and spatial development. Dev. Psychol. 53, 1869–1880. doi: 10.1037/dev0000384
Vergnaud, G. (1982). “A classification of cognitive tasks and operations of thought involved in addition and subtraction problems” in Addition and subtraction. eds. T. P. Carpenter, J. M. Moser, and T. A. Romberg (London: Routledge), 39–59.
Vest, N., and Alibali, M. W. (2021). The mental representation of integers: further evidence for the negative number line as a reflection of the natural number line. Proc. Annu. Meet. Cogn. Sci. Soc. 43, 1670–1676.
Vieluf, S., and Klieme, E. (2023). “Teaching effectiveness revisited through the lens of practice theories” in Theorizing teaching: current status and open issues. eds. P. Anna-Katharina and C. Y. Charalambous (Cham: Springer International Publishing), 57–95.
Vinci-Booher, S., and James, K. H. (2020). Visual experiences during letter production contribute to the development of the neural systems supporting letter perception. Dev. Sci. 23:e12965. doi: 10.1111/desc.12965
Vinci-Booher, S., and James, K. H. (2021). Protracted neural development of dorsal motor systems during handwriting and the relation to early literacy skills. Front. Psychol. 12:750559. doi: 10.3389/fpsyg.2021.750559
Vingerhoets, G. (2008). Knowing about tools: neural correlates of tool familiarity and experience. Neuroimage 40, 1380–1391. doi: 10.1016/j.neuroimage.2007.12.058
Von Glasersfeld, E. (1984). “An introduction to radical constructivism” in The invented reality. ed. P. Watzlawick (New York, NY: WW Norton), 17–40.
Von Glasersfeld, E. (1995). “A constructivist approach to teaching” in Constructivism in education. eds. L. P. Steffe and J. Gale (New Jersey, NJ: Routledge), 21–34.
Voss, J. L., and Paller, K. A. (2010). Real-time neural signals of perceptual priming with unfamiliar geometric shapes. J. Neurosci. 30, 9181–9188. doi: 10.1523/JNEUROSCI.0403-10.2010
Voss, J. L., Schendan, H. E., and Paller, K. A. (2010). Finding meaning in novel geometric shapes influences electrophysiological correlates of repetition and dissociates perceptual and conceptual priming. Neuroimage 49, 2879–2889. doi: 10.1016/j.neuroimage.2009.09.012
Vuokko, E., Niemivirta, M., and Helenius, P. (2013). Cortical activation patterns during subitizing and counting. Brain Res. 1497, 40–52. doi: 10.1016/j.brainres.2012.12.019
Wagensveld, B., van Alphen, P., Segers, E., Hagoort, P., and Verhoeven, L. (2013). The neural correlates of rhyme awareness in preliterate and literate children. Clin. Neurophysiol. 124, 1336–1345. doi: 10.1016/j.clinph.2013.01.022
Wakefield, E. M., Congdon, E. L., Novack, M. A., Goldin-Meadow, S., and James, K. H. (2019). Learning math by hand: the neural effects of gesture-based instruction in 8-year-old children. Atten. Percept. Psychophys. 81, 2343–2353. doi: 10.3758/s13414-019-01755-y
Wallis, P., Richards, T., Boord, P., Abbott, R., and Berninger, V. (2017). Relationships between translation and transcription processes during fMRI connectivity scanning and coded translation and transcription in writing products after scanning in children with and without transcription disabilities. Creat. Educ. 8, 716–748. doi: 10.4236/ce.2017.85055
Wasik, B. A., and Hindman, A. H. (2015). Talk alone won’t close the 30-million word gap. Phi Delta Kappan 96, 50–54. doi: 10.1177/0031721715575300
Wei, W., Chen, C., Yang, T., Zhang, H., and Zhou, X. (2014). Dissociated neural correlates of quantity processing of quantifiers, numbers, and numerosities: neural correlates of quantity processing. Hum. Brain Mapp. 35, 444–454. doi: 10.1002/hbm.22190
Weiss, Y., and Booth, J. R. (2017). Neural correlates of the lexicality effect in children. Brain Lang. 175, 64–70. doi: 10.1016/j.bandl.2017.09.006
Werker, J. F., and Vouloumanos, A. (2001). “Speech and language processing in infancy: a neurocognitive approach” in Handbook of developmental cognitive neuroscience. eds. C. A. Nelson and M. Luciana (Cambridge: MIT Press), 269–307.
Westermann, G., Mareschal, D., Johnson, M. H., Sirois, S., Spratling, M. W., and Thomas, M. S. C. (2007). Neuroconstructivism. Dev. Sci. 10, 75–83. doi: 10.1111/j.1467-7687.2007.00567.x
Westermann, G., Thomas, M. S. C., and Karmiloff-Smith, A. (2011). “Neuroconstructivism” in The Wiley-Blackwell handbook of childhood cognitive development. ed. U. Goswami (New York, NY: Wiley-Blackwell), 723–747.
Whitney, C., Huber, W., Klann, J., Weis, S., Krach, S., and Kircher, T. (2009). Neural correlates of narrative shifts during auditory story comprehension. Neuroimage 47, 360–366. doi: 10.1016/j.neuroimage.2009.04.037
Widmann, A., Gruber, T., Kujala, T., Tervaniemi, M., and Schroger, E. (2007). Binding symbols and sounds: evidence from event-related oscillatory gamma-band activity. Cerebellum 17, 2696–2702. doi: 10.1093/cercor/bhl178
Wiedenbauer, G., and Jansen-Osmann, P. (2008). Manual training of mental rotation in children. Learn. Instruct. 18, 30–41. doi: 10.1016/j.learninstruc.2006.09.009
Wilber, K. (2001). Sex, ecology, spirituality: the spirit of evolution. Boston, MA: Shambhala Publications.
Williams, J. H. G., Casey, J. M., Braadbaart, L., Culmer, P. R., and Mon-Williams, M. (2014). Kinematic measures of imitation Fidelity in primary school children. J. Cogn. Dev. 15, 345–362. doi: 10.1080/15248372.2013.771265
Wilson, D., and Conyers, M. (2020). Five big ideas for effective teaching: Connecting mind, brain, and education research to classroom practice. New York, NY: Teachers College Press.
Woodward, A. L. (2005). “Infants’ understanding of the actions” in Joint attention: communication and other minds: issues in philosophy and psychology. eds. N. Eilan, C. Hoerl, T. McCormack, and J. Roessler (Oxford: Oxford University Press), 110–128.
Wortha, S. M., Bloechle, J., Ninaus, M., Kiili, K., Lindstedt, A., Bahnmueller, J., et al. (2020). Neurofunctional plasticity in fraction learning: an fMRI training study. Trends Neurosci. Educ. 21:100141. doi: 10.1016/j.tine.2020.100141
Wu, X., Jung, R. E., and Zhang, H. (2016). Neural underpinnings of divergent production of rules in numerical analogical reasoning. Biol. Psychol. 117, 170–178. doi: 10.1016/j.biopsycho.2016.03.011
Xia, Z., Zhang, L., Hoeft, F., Gu, B., Gong, G., and Shu, H. (2018). Neural correlates of oral word reading, silent reading comprehension, and cognitive subcomponents. Int. J. Behav. Dev. 42, 1–15. doi: 10.1177/0165025417727872
Xu, C., and LeFevre, J.-A. (2016). Training young children on sequential relations among numbers and spatial decomposition: differential transfer to number line and mental transformation tasks. Dev. Psychol. 52, 854–866. doi: 10.1037/dev0000124
Xu, F., Spelke, E. S., and Goddard, S. (2005). Number sense in human infants. Devel. Sci. 8, 88–101. doi: 10.1111/j.1467-7687.2005.00395.x
Yamada, Y., Stevens, C., Dow, M., Harn, B. A., Chard, D. J., and Neville, H. J. (2011). Emergence of the neural network for reading in five-year-old beginning readers of different levels of pre-literacy abilities: an fMRI study. Neuroimage 57, 704–713. doi: 10.1016/j.neuroimage.2010.10.057
Yang, T., Gathercole, S. E., and Allen, R. J. (2014). Benefit of enactment over oral repetition of verbal instruction does not require additional working memory during encoding. Psychon. Bull. Rev. 21, 186–192. doi: 10.3758/s13423-013-0471-7
Yu, C., and Ballard, D. H. (2007). A unified model of early word learning: integrating statistical and social cues. Neurocomputing 70, 2149–2165. doi: 10.1016/j.neucom.2006.01.034
Yuan, Y., Major-Girardin, J., and Brown, S. (2018). Storytelling is intrinsically mentalistic: a functional JMRI study of narrative production across modalities. J. Cogn. Neurosci. 30, 1298–1314. doi: 10.1162/jocn_a_01294
Yue-jia, L., Yun, N., and Hong, L. (2004). Difference of neural correlates between subitizing and counting reflected by ERPs. Acta Psychol. Sin. 36, 434–441.
Zago, L., Pesenti, M., Mellet, E., Crivello, F., Mazoyer, B., and Tzourio-Mazoyer, N. (2001). Neural correlates of simple and complex mental calculation. Neuroimage 13, 314–327. doi: 10.1006/nimg.2000.0697
Zago, L., Petit, L., Mellet, E., Joliot, M., Mazoyer, B., and Tzourio-Mazoyer, N. (2010). Neural correlates of counting large numerosity. Int. J. Math. Educ. 42, 569–577. doi: 10.1007/s11858-010-0254-9
Zambrzycka, J., Kotsopoulos, D., Lee, J., and Makosz, S. (2017). In any way, shape, or form? Toddlers’ understanding of shapes. Infant Behav. Dev. 46, 144–157. doi: 10.1016/j.infbeh.2016.12.002
Zhang, H., Chen, C., and Zhou, X. (2012). Neural correlates of numbers and mathematical terms. Neuroimage 60, 230–240. doi: 10.1016/j.neuroimage.2011.12.006
Zhang, L., and Pylkkänen, L. (2018). Composing lexical versus functional adjectives: evidence for uniformity in the left temporal lobe. Psychon. Bull. Rev. 25, 2309–2322. doi: 10.3758/s13423-018-1469-y
Zhang, Y., Wang, K., Yue, C., Mo, N., Wu, D., Wen, X., et al. (2018). The motor features of action verbs: fMRI evidence using picture naming. Brain Lang. 179, 22–32. doi: 10.1016/j.bandl.2018.02.002
Zhao, T. C., Boorom, O., Kuhl, P. K., and Gordon, R. (2021). Infants’ neural speech discrimination predicts individual differences in grammar ability at 6 years of age and their risk of developing speech-language disorders. Dev. Cogn. Neurosci. 48:100949. doi: 10.1016/j.dcn.2021.100949
Keywords: radical neuroconstructivism, mind-brain-education, core notions, learning sciences, learning trajectory, teacher education, holonic thinking, teacher professional development (TPD)
Citation: Tokuhama-Espinosa T and Borja C (2023) Radical neuroconstructivism: a framework to combine the how and what of teaching and learning? Front. Educ. 8:1215510. doi: 10.3389/feduc.2023.1215510
Received: 02 May 2023; Accepted: 23 June 2023;
Published: 01 August 2023.
Edited by:
Lorna Uden, Staffordshire University, United KingdomReviewed by:
Stamatios Papadakis, University of Crete, GreeceCopyright © 2023 Tokuhama-Espinosa and Borja. This is an open-access article distributed under the terms of the Creative Commons Attribution License (CC BY). The use, distribution or reproduction in other forums is permitted, provided the original author(s) and the copyright owner(s) are credited and that the original publication in this journal is cited, in accordance with accepted academic practice. No use, distribution or reproduction is permitted which does not comply with these terms.
*Correspondence: Tracey Tokuhama-Espinosa, dHJhY2V5dG9rdWhhbWFlc3Bpbm9zYUBmYXMuaGFydmFyZC5lZHU=
†These authors have contributed equally to this work and share first authorship
‡ORCID: Tracey Tokuhama-Espinosa, https://orcid.org/0000-0002-0661-4586
Cynthia Borja, https://orcid.org/0000-0002-7245-1359
Disclaimer: All claims expressed in this article are solely those of the authors and do not necessarily represent those of their affiliated organizations, or those of the publisher, the editors and the reviewers. Any product that may be evaluated in this article or claim that may be made by its manufacturer is not guaranteed or endorsed by the publisher.
Research integrity at Frontiers
Learn more about the work of our research integrity team to safeguard the quality of each article we publish.