- 1Department of Community Sustainability, Michigan State University, East Lansing, MI, United States
- 2College of Arts and Sciences, Oberlin College, Oberlin, OH, United States
- 3Center for the Philosophy of Freedom, University of Arizona, Tucson, AZ, United States
Introduction: Disagreements between people on different sides of popular issues in STEM are often rooted in differences in “mental models,” which include both rational and emotional cognitive associations about the issue; especially given these issues are systemic in nature.
Methods: In the research described here, we employ the fuzzy cognitive mapping software MentalModeler (developed by one of the authors)1 as a tool for articulating implicit and explicit assumptions about one’s knowledge of both the environmental and social science and values underpinning complex system related issues. More specifically, we test the assumption that this pedagogical approach will foster certain aspects of perspective taking that can be traced with cognitive development and systems thinking as students not only articulate their own understanding of an issue, but also articulate the view of others.
Results and discussion: Results are discussed with respect to systems thinking that is developed through this type of modeling.
Introduction
The complex topics that the biological sciences grapple with are not merely scientific puzzles; they are also ideologically and emotionally powered issues that affect many research fields and the diverse public in different ways. Such issues require teaching and learning strategies that enable students to solve problems that are often associated with complex biological and social systems. In this paper, we share the outcomes of an undergraduate classroom-based research study that teaches problem- solving and complex system thinking using semi-quantitative and computational modeling with perspective taking. More specifically, we use the MentalModeler software suite and case studies based on emotionally charged issues that serve to both motivate and set the context for student learning. This approach was designed to encourage multiple perspective taking to teach both systems thinking and problem solution/resolution. The latter of which we argue also requires creativity and thinking across temporal and spatial scales.
Background
To develop socio-scientific thinking skills in biology, students need learning materials nested in the context of real-world problems (Zeidler et al., 2009). This necessarily involves thinking in systems and across scale. Further, we argue, learners need to develop, model, and support explanations of complex biological systems (e.g., modeling their understanding), while thinking across perspectives (Jordan and Sorensen, 2021). The focus of the learning intervention described here is to use models as a means for students to engage in different perspectives. In this study, we defined models as simplified abstractions that aid in thinking. Below are the three research literatures that inform our approach: (1) systems thinking, (2) model-based learning for systems thinking, and (3) novel perspective taking.
1. Systems thinking. Systems thinking is increasingly recognized as central to being scientifically literate citizens (e.g., Gray, 2018). While a systems-thinking approach to STEM education has been popular for decades (Stave and Hopper, 2007; Skaza and Stave, 2009), there remain significant gaps in understanding how systems thinking is best scaffolded in the undergraduate STEM classroom and how to assess and measure students’ understanding of systems. Nonetheless, systems thinking generates scientific habits of mind (Kay and Foster, 1999; Steinkuehler and Duncan, 2008) that are useful frameworks for reasoning and abstracting about a range of complex interactions that underlie contemporary societal biological-related problems (Tabacaru et al., 2009).
Although definitions of systems thinking vary, most definitions highlight that systems thinking is an ability to recognize and understand the relationships between the structure and function of complex systems and the ability to qualify this understanding through graphical or semantic representation, definition, and explanation. In this manuscript, we our view of systems thinking is akin to the soft Systems-thinking Methodology (i.e., SSM) defined by Checkland and Poulter (2020). Here, learners are using a purposeful activity (such as generating FCM models; see below) to grapple with real- world, often social, issues that are complex and require the learner to approach the problem from different angles. We stray from SSM in that we do not follow the learning cycle exactly as described by the authors, but we do include a cycle where information is gathered, leverage points are defined, trade-offs are compared, and decision or action is supported.
Systems thinking has been shown to be challenging for learners because of the non-linear, multi- scalar dynamics with often embedded feedbacks, hidden mechanism, and emergent properties (Hmelo-Silver and Azevedo, 2006; Jacobson and Wilensky, 2006; Verhoeff et al., 2008). In natural systems such complexity includes macroscopic and microscopic phenomena (Penner, 2000; Samon and Levy, 2017) that interact with the living and nonliving elements of the environment (Hmelo-Silver et al., 2007; Shepardson et al., 2007; Eilam, 2012). Learners also struggle with how mechanisms and outcomes are interrelated (Covitt et al., 2009). Learners often put undue focus on unidirectional causal chains that then cause the learner to miss the reciprocal relationships between structures and the associated processes (Grotzer and Basca, 2003; Mohan et al., 2009). This oversimplicity in relational understanding is especially exacerbated when thinking about how smaller system elements interact to result in larger scale outcomes (Grotzer et al., 2017). In addition, learners can ascribe forces within a system to have intent and purpose to produce a certain outcome, leading to oversimplified and inaccurate interpretations of causality (Cuzzolino et al., 2019).
Ben-Zvi Assaraf and Orion (2005) report that learners’ systems thinking can be supported through interventions that feature knowledge integration, but in a case study of four students 4 years later, few ideas remained stable: suggesting a need for greater thinking support throughout the curriculum. Some (e.g., York and Orgill, 2020; Jordan and Sorensen, 2021) have suggested approaches to systems thinking scaffolding and assessment, which involve clearly defining the system processes within the context of the curriculum, whether it be chemistry, life sciences, or in the case of Mahaffy et al. (2018), within interdisciplinary studies (e.g., chemistry and socio-environmental systems). How can students’ internal representations of their systems understanding, therefore be determined, and assessed? We suggest that models as external representations of learners’ system understanding can be useful in not only teaching about systems but also in the assessment of systems thinking.
2. Model-based learning for systems thinking. Based on the idea that internal mental models are constructed over time as new information is obtained e.g., (see Dauer and Long, 2015), we argue that iterative model construction is an effective practice from which learners can integrate both evidence and domain-specific knowledge into visualizations (i.e., concept maps) that can be used to measure degrees of systems thinking through model-based reasoning. Indeed, iterative model development provides a manner for learning about systems in domain specific contexts (Jordan and Sorensen, 2021). Below we discuss the value of models in supporting the cognitive offloading of complex ideas.
Here we define models as simplified abstractions or representations that characterize an idea or phenomenon (e.g., Crawford and Jordan, 2013). Models allow cognition to be distributed by offloading parts of difficult tasks into the physical environment, where thinking can be organized and discussed. Furthermore, because models often include a small number of semantic representations, individuals coming from different backgrounds, once familiar with model terms, can communicate in a standardized space. Finally, models provide opportunities for learners to make their ideas visible and open for discussion, negotiation, revision, and extension; supporting constructive discourse, which is associated with positive learning outcomes (Greeno, 1998; Chi et al., 2001).
Learner mental models, as made visible through cognitive mapping software, can also serve as a kind of boundary object (Star and Griesemer, 1989) by providing the means for bridging ideas across disciplines. In this manner, students taking ideas and perspectives from different disciplines are given a common language for discourse. We, therefore use models as a way for students to help the instructors and each other make their thinking visible. Doing so can enable the multiple and complex parts and processes of the system to be identified and elaborated. We argue that providing learners with a specific modeling approach can enable a common language and classroom artifact that can be assessed. In addition, this common language serves as an effective means for assessment. Finally, modeling can also aid students in taking multiple perspectives.
3. Perspective taking. Cabrera (2009), and more recently Taylor et al. (2020), argue that perspective taking is a fundamental part of systems thinking but is underdeveloped in terms of STEM and life science learning. Perspective taking is the ability to make inferences about and represent others’ psychological states—their emotions, thoughts, goals, and intentions (Stietz et al., 2019). We argue that promoting such perspective-taking pedagogy is essential for developing social and scientific problem-solving skills, both because it will enhance learners’ understanding of complex systems, as well as help to overcome cognitive biases elicited by controversial socio-biological problems.
Like the ambiguity of defining, understanding, and measuring systems thinking, perspective taking is often referred to in the literature using various terms including theory of mind, mentalizing, and cognitive empathy (in contrast to emotional empathy, the capacity to share others’ emotions; see Kahn and Zeidler, 2019 for a review). When learners are exposed to a view that is contrary to their own on an issue that matters to them (e.g., climate change, Genetically Modified Organisms), their moral feelings are violated. This triggers emotions that take over thinking, therefore much of the reasoning that follows serves solely to find arguments that support one’s own view on the issue and arm against deviating arguments (Molden and Higgins, 2012; Greene, 2014). The false consensus effect, or the tendency to assume that others see the world as we do, leads people to systematically underestimate the extent to which others’ perspectives diverge from their own (Bergquist et al., 2019). In this manuscript, we focus on several issues that present likely moral dilemmas for the learner, though, we acknowledge that this might vary and therefore use positionality (i.e., whether a student agrees or disagrees and to what extent) on an issue as a consideration in our study.
Research questions
In this study, we wanted to characterize the change in what students modeled (i.e., in terms of model micro-motifs such as causal linkages and feedback loops) when modeling different perspectives of complex issues/systems.
We hypothesized that engagement in this perspective taking intervention will result in an increase in more sophisticated (i.e., greater causal linkages and indirect outcomes) model micro-motifs. We predict that such a change could be related to greater complexity that results from the multiple vantages of perspective taking.
Methods
Study design
We partnered with instructors at a small midwestern liberal arts college for two semesters: including instructors of introductory classes in psychology, economics, and environmental studies from this institution. These data represent a subset of a larger study in progress. The larger study focuses not only on systems thinking and perspective taking, but also is intended to develop tools that allow for rapid conceptual model assessment in large classes. Instructors informed students that course products produced by them would be included in this study and that students had the option of opting out of the study. While the assignments were mandatory, students were able to elect or decline for their materials to be used in the research study and were given the option to withdraw throughout the semester. Student participation involved filling out two surveys and completing three modeling assignments. Research was done with institutional IRB approval (i054387).
Students participated in a survey at the beginning of the semester. This survey sought student agreement with one side or the other of a controversial issue. Three issues were chosen as the focus of this study: one biological (Genetically Modified Crops), one economic (price gouging), and one social (social media use) controversy. These topics were chosen because the authors thought they would likely elicit significant personal investment from students based on prior experience. Throughout the semester, students engaged in the three modeling assignments, each focused on one topic described above. To complete this modeling assignment, students read two contrasting perspectives written as first person narratives by the authors (pro and con). These perspectives were balanced in length and argument strength. Both shared common arguments for each perspective (see Supplementary material). After reading both perspectives, students were asked to make a model using the software, MentalModeler, for each perspective (see Supplementary material for the homework prompts). These models appear as concept maps (again, see Supplementary material) with arrows that have indicated both a direction, weight, which is positive or negative, and strength represented by line thickness. In this way, these models are semi-quantitative and represent drivers, receivers, and ordinary variables within the system. Note: that the direction was used to quantify the type of relationship between the variables, but through an informal inspection of the data saw no reason to include line strength as a variable. Whether the line was positive or negative was used in the micro-motif analysis. Students were given five or six predetermined (by the study authors) components for each model that they were required to put in their models and were told to add up to 10 more. They were told to think about and add as many connections to their models as they saw fit. All students did the assignments in the same order and roughly the same time across classes.
Data collection
Surveys and modeling works were collected online. Students were told to attach their dotmmp (Mental Modeler File type) files. However, many failed to do so, instead attaching different file types, which resulted in a reduction of our sample size because data analysis required the dotmmp file. In addition, throughout the semester, students were instructed to complete and upload six models: one for and against each of the three topics, but some students were missing models altogether. We received a total of 104 out of a possible 225 complete student responses from the first semester, and 67 out of 132 complete student responses from the second semester. Attrition was greatest at the first timepoint.
Data analysis
All data were extracted through download of the model structure metrics using the dotmmp files and MentalModeler. Model structure metrics included component information, which included each component put into the model and the nature of the arrows linking the components (and arrow direction and strength). These data included: Total Components, Total Connections, Density (number of lines), Number of Driver Components (causal), Number of Receiver Components (effect), and Number of Ordinary Components (neutral).
Once these data were extracted, model component counts were averaged across the pro and con for each side. They were also separated into “my side” and “other side” based on which stance the student took at the beginning of the semester and which side the model was on. For example, if a student was against GMOs, the GMO pro model would be “other side” and the GMO con model would be “myside.”
Models averages of component information were analyzed through a type II, unbalanced design Analysis as Variance (ANOVA) across the three assignments with time, course enrolled, and issue side as a within-subjects factors. In this manner, model effects were tested separately.
Using these data, information on micro-motifs was also generated. The micro motifs reflect the patterns associated with the components (represented by boxes in the modeling software) and the links (represented by arrows in the modeling software) students create between the boxes. Table 1 explains each micro-motif and how they were calculated using the model structure data.
Bidirectionality is the simplest type of linkage with multiple causes and effects being the next level in that students are interlinking constructs and relationships. Next are the indirect effects, which are essential to representing non-linear and often dynamic component relations, and then finally feedback loops represent cyclic type mechanisms that play a role in complex system outcomes (see Supplementary material for model motif images). We define sophistication as the presence of indirect effects, causal chains, and feedback loops. The four categories of motifs (see Table 1) represent increasing levels of sophistication with feedbacks/feedback loops being the highest level of understanding in terms of system representation motifs.
These micro-motif data were analyzed like model component data in that each motif was counted and averaged across students and then ANOVA was used to compare within side and across time.
Results
We found no significant difference in models as analyzed with ANOVA in terms of class enrolled or in side taken as a within subject factor. This means that modeling a perspective different from one’s own results in differences in model structure or the micro-motifs. We, however, found differences in models averaged across time. While we looked at both own side and other side, we chose to analyze own side models only. Again, we note that students did all tasks in the same order and own side prior to other side. We provide those data in Tables 2, 3.
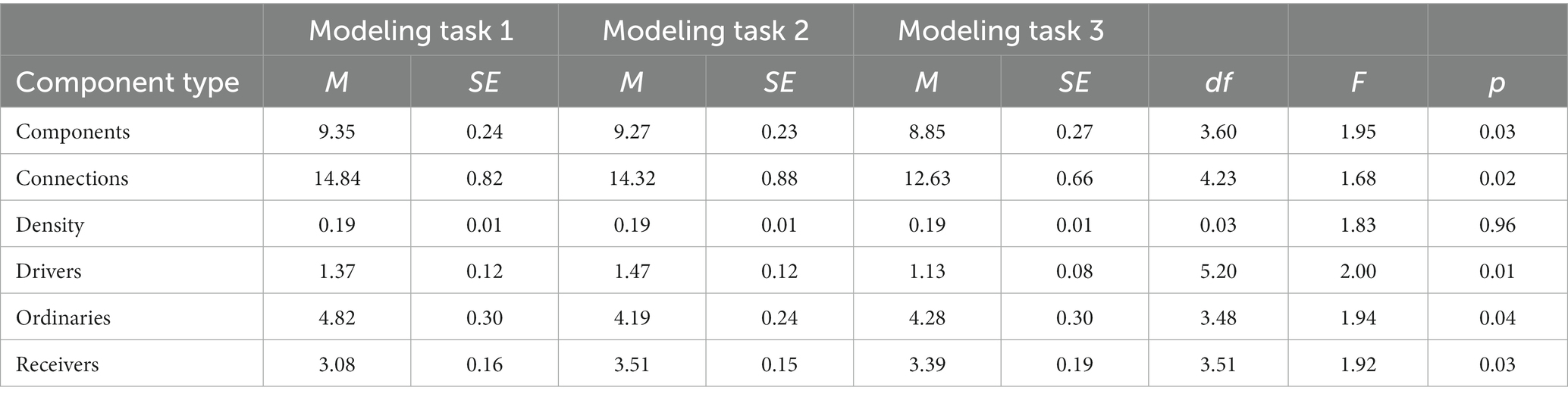
Table 2. This table is representing means, standard errors, and the ANOVA statistics for each model term using the downloaded model structure metrics for one side of the issue (note the overall model was significant).
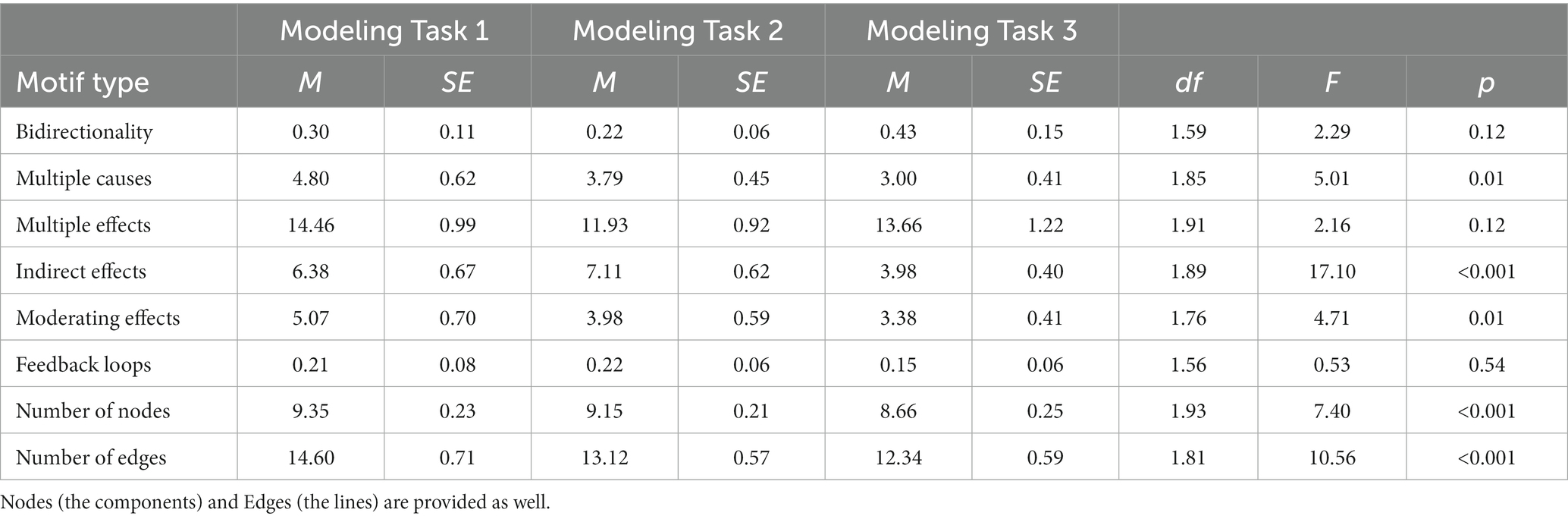
Table 3. This table is representing means, standard errors, and the ANOVA statistics for each model term using the micro-motif metrics for one side of the issue (note the overall model was significant).
The ANOVA indicate several significant statistical model terms. We will discuss these terms and the trends indicated by the means. The number of components and connections went down as time progressed. This indicates that the models were getting relatively simpler. The number of drivers, receivers, and ordinary variables were variable over time with the number of drivers and receivers being less in the final model and ordinary variables being more. This means that with the reduction of model terms, the students changed how they modeled to indicate less components that are affected in all aspects of the system (imagine a wagon wheel with a central component driving several outcomes or being affected by everything depending on arrow direction). Such a change should and did indicate a difference in how components and lines were represented as motifs.
The micro-motif data indicate a decrease in overall number of each type of motif represented. This is not surprising given the decline in model complexity over time. In general students tended to model most the second and third level of sophistication in representations. More specifically, students tended to represent effects (multiple, indirect, and moderating), which mean that students represented outcomes with drivers and receivers that were multiple mostly but also indirect. Bidirectionality, which is the simplest type of relationship and feedback loops, which are the most complex motif, were represented far less. Note that the students stayed at the same model sophistication levels throughout the semester.
Discussion
In summary, we found that model structure changed through time; mostly representing a reduction of components and relationships with time. In addition, we found that students tended to model at mid- level sophistication represented by the micro-motifs and that this did not change with time. Finally, we found no support that perspective-taking changed modeling structure in that there was little difference in that modeled between own side and other side.
These results have given us a guide for future directions. While we have evidence that taking multiple perspectives changes certain habits of mind (e.g., open-mindedness and intellectually humility, etc.) regarding how issues are presented and discussed outside of the modeling practice (forthcoming, authors et al.), it was clear that representing perspective did not change the sophistication level of the model being developed. This may not be surprising because we were measuring model counts as related to systems thinking and not the nature of the issue being represented. Analyzing model content on a more qualitative scale could yield more insight; though in our study, post-hoc inspection of the models did not indicate much variation in model terms being used (i.e., in terms of the up to 10 added components). Our approach (and subsequent analysis) to analyzing large groups of models, however, have given us a target for how the cases might be restructured to encourage thinking about feedback loops, which we argue are critical to student understanding of how complex systems yield indirect, emergent, and often uncertain outcomes. The latter of which is critical for issue decision making, which may present a greater challenge to one’s moral imperatives and therefore, would allow us to measure perspective taking akin to the levels described in Kahn and Zeidler (2019).
Given that feedbacks/feedback loops represent the highest level of system representation, we sought to understand more about how our work fits with data from projects featuring these loops. Feedback loops have long been seen as critical to systems thinking (e.g., Stave and Hopper, 2007). Much like our approach described above, Cabrera et al. (2015) have divided systems thinking as a compendium of distinctions of what is being modeled (i.e., outcome-related elements), and of the parts and wholes (i.e., the components and the mechanisms within) and then the represented relationships, which are associated with actions and reactions. The latter, two we argue, target the critical value of feedback loops and how they might be taught. Certainly feedback loops have been found to be somewhat difficult for learners (e.g., from elementary students; Hokayem, 2012) to undergraduate students (Hokayem et al., 2014). While students were able to represent cycles when prompted (Green, 1997), feedback loops remain difficult (Wellmanns and Schmiemann, 2022). Clearly, more scaffolding in this area is necessary.
While perspective taking, as structured in our case study and assignment, did not result in different model structures in taking side, it remains to be determined if taking perspective could change model practice in a different way. If, perspective taking can result in the reduction of biased information being used and represented and in the increase of creative solutions, then perhaps case studies that provide components beyond normatively accepted elements might result in change of information selection and representation over time? In addition, if the students were subsequently encouraged to represent scenarios (i.e., outcomes related to what is represented in the model but allowing students to change the strength of influence of what is represented), then might student creativity be an outcome that increases with time. Of course, we did not measure creativity in the study described above so this remains speculative.
Future directions include adding scenario building and measuring model relations as sophistication of content (versus structure described above). Additionally, and to truly understand the role of perspective taking, we plan to investigate differences in models from those who did not participate in both sides but rather modeled their own side only. We are confident, however, that our data provide support that our intervention is a viable means by which life science classes can present and measure model building to support systems thinking instruction. More data are necessary, however, to determine the extent to which our intervention can increase sophistication of systems representation beyond what we have shared above.
Data availability statement
The raw data supporting the conclusions of this article will be made available by the authors, without undue reservation.
Ethics statement
The studies involving humans were approved by Michigan State Institutional Review Board. The studies were conducted in accordance with the local legislation and institutional requirements. The participants provided their written informed consent to participate in this study.
Author contributions
RJ and AS: writing. SG: model tool development. AB-P: data analysis. CF and JJ: research tool development. PB, MS, and JP: case study development. All authors contributed to the article and approved the submitted version.
Funding
This work was funded by the Heterodox Foundation and the National Science Foundation (proposal no. 2111406/2111065).
Acknowledgments
The authors acknowledge the many students and instructors who participated in this work.
Conflict of interest
The authors declare that the research was conducted in the absence of any commercial or financial relationships that could be construed as a potential conflict of interest.
Publisher’s note
All claims expressed in this article are solely those of the authors and do not necessarily represent those of their affiliated organizations, or those of the publisher, the editors and the reviewers. Any product that may be evaluated in this article, or claim that may be made by its manufacturer, is not guaranteed or endorsed by the publisher.
Supplementary material
The Supplementary material for this article can be found online at: https://www.frontiersin.org/articles/10.3389/feduc.2023.1215436/full#supplementary-material
Footnotes
References
Ben-Zvi Assaraf, O., and Orion, N. (2005). Development of system thinking skills in the context of earth system education. J. Res. Sci. Teach. 42, 518–560. doi: 10.1002/tea.20061
Bergquist, M., Nilsson, A., and Schultz., W. P. (2019). A meta-analysis of field-experiments using social norms to promote proenvironmental behaviors. Global Environmental Change doi: 10.1016/j.gloenvcha.2019.101941
Cabrera, D. (2009). Systems thinking: four universal patterns of thinking Saarbrücken, Germany: VDM Verlag.
Cabrera, D., Cabrera, L., and Powers, E. (2015). A unifying theory of systems thinking with psychosocial applications. Syst. Res. Behav. Sci. 32, 534–545. doi: 10.1002/sres.2351
Checkland, P., and Poulter, J. (2020). “Soft systems methodology” in Systems approaches to making change: a practical guide. eds. M. Reynolds and S. Holwell (London: Springer)
Chi, M.T.H., Siler, S.A., Jeong, H., Yamauchi, T., and Hausmann, R.G. (2001). Learning from human tutoring Cognitive Science 25, 471–533. doi: 10.1207/s15516709cog2504_1
Covitt, B. A., Gunckel, K. L., and Anderson, C. W. (2009). Students' developing understanding of water in environmental systems. J. Environ. Educ. 40, 37–51. doi: 10.3200/JOEE.40.3.37-51
Crawford, B., and Jordan, R. C. (2013). “Inquiry, models, and complex reasoning to transform learning in environmental education” in Transdisciplinary research in environmental education. eds. M. E. Krasny and J. Dillon (Ithaca: Cornell University Press)
Cuzzolino, M., Grotzer, T., Tutwiler, M., and Torres, E. (2019). An agentive focus may limit learning about complex causality and systems dynamics: a study of seventh graders' explanations of ecosystems. J. Res. Sci. Teach. 56, 1083–1105. doi: 10.1002/tea.21549
Dauer, J., and Long, T. (2015). Long-term conceptual retrieval by college biology majors following model-based instruction. J. Res. Sci. Teach. 52, 1188–1206. doi: 10.1002/tea.21258
Eilam, B. (2012). System thinking and feeding relations: learning with a live ecosystem model. Instr. Sci. 40, 213–239. doi: 10.1007/s11251-011-9175-4
Gray, S. (2018). Measuring systems thinking. Nat Sustainab. 1, 388–389. doi: 10.1038/s41893-018-0121-1
Green, D. W. (1997), Explaining and envisaging an ecological phenomenon. British Journal of Psychology, 88: 199–217. doi: 10.1111/j.2044-8295.1997.tb02630.x
Greene, J. D. (2014). Moral tribes: emotion, reason, and the gap between us and them. Chicago: Penguin.
Greeno, J. G., and Middle School Mathematics through Applications Project Group. (1998). The situativity of knowing, learning, and research. American Psychologist, 53, 5–26. doi: 10.1037/0003-066X.53.1.5
Grotzer, T. A., and Basca, B. B. (2003). How does grasping the underlying causal structures of ecosystems impact students' understanding? J. Biol. Educ. 38, 16–29. doi: 10.1080/00219266.2003.9655891
Grotzer, T., Derbiszewska, K., and Solis, S. L. (2017). Leveraging fourth and sixth graders’ experiences to reveal understanding of the forms and features of distributed causality. Cogn. Instr. 35, 55–87. doi: 10.1080/07370008.2016.1251808
Hmelo-Silver, C., and Azevedo, R. (2006). Understanding complex systems: some core challenges. J. Learn. Sci. 15, 53–61. doi: 10.1207/s15327809jls1501_7
Hmelo-Silver, C. E., Marathe, S., and Liu, L. (2007). Fish swim, rocks sit, and lungs breathe: expert- novice understanding of complex systems. J. Learn. Sci. 16, 307–331. doi: 10.1080/10508400701413401
Hokayem, H. A. (2012). Learning progression of ecological system reasoning for lower elementary (G1-4) students (doctoral dissertation). ProQuest. (MI 48106-1346).
Hokayem, H., Ma, J., and Hui, J. (2014). A learning progression for feedback loop reasoning at lower elementary level. J. Biol. Educ. 49, 246–260. doi: 10.1080/00219266.2014.943789
Jacobson, M. J., and Wilensky, U. (2006). Complex systems in education: scientific and educational importance and implications for the learning sciences. J. Learn. Sci. 15, 11–34. doi: 10.1207/s15327809jls1501_4
Jordan, R. C., and Sorensen, A. E. (2021). Modeling and conceptual representations work together in the undergraduate classroom. J. Multidiscipl. Eng. Sci. Stud. 7:2021.
Kahn, S., and Zeidler, D. (2019). A conceptual analysis of perspective taking in support of socioscientific reasoning. Sci. Educ. 28, 605–638. doi: 10.1007/s11191-019-00044-2
Kay, J. J., and Foster, J. (1999). “About teaching systems thinking” in Proceedings of the HKK Conference, Ontario 165–172.
Mahaffy, P. G., Krief, A., Hopf, H., Mehta, G., and Matlin, S. A. (2018). Reorienting chemistry education through systems thinking. Nat. Rev. Chem. 2:0126. doi: 10.1038/s41570-018-0126
Mohan, L., Chen, J., and Anderson, C. W. (2009). Developing a multi-year learning progression for carbon cycling in socio-ecological systems. Journal of Research in Science Teaching, 46, 675–698. doi: 10.1002/tea.20314
Penner, D. (2000). Explaining systems investigating middle school students' understanding of emergent phenomena. J. Res. Sci. Teach. 37, 784–806. doi: 10.1002/1098-2736(200010)37:8<784::AID-TEA3>3.0.CO;2-E
Samon, S., and Levy, S. T. (2017). Micro–macro compatibility: when does a complex systems approach strongly benefit science learning? Sci. Educ. 101, 985–1014. doi: 10.1002/sce.21301
Shepardson, D. P., Wee, B., Priddy, M., and Harbor, J. (2007). Students' mental models of the environment. J. Res. Sci. Teach. 44, 327–348. doi: 10.1002/tea.20161
Skaza, H., and Stave, K. (2009). A test of the relative effectiveness of using systems simulations to increase student understanding of environmental issues. The 27th International Conference of the Systems Dynamics Society, Albuquerque, New Mexico, July 26-30.
Star, S. L., and Griesemer, J. (1989). Institutional ecology, translations, and boundary objects: amateurs and professionals in Berkeley's Museum of Verterbrate Zoology, 1907-39. Soc. Stud. Sci. 19, 387–420.
Stave, K., and Hopper, M. (2007). What constitutes systems thinking? A proposed taxonomy. The 25th International Conference of the Systems Dynamics Society, Boston, MA, July 29 – August 7.
Steinkuehler, C., and Duncan, S. (2008). Scientific habits of mind in virtual worlds. J. Sci. Educ. Technol. 17, 530–543. doi: 10.1007/s10956-008-9120-8
Stietz, J, Jauk, E, Krach, S, and Kanske, P. Dissociating Empathy From Perspective-Taking: Evidence From Intra- and Inter-Individual Differences Research. Front Psychiatry. 2019 10:126. doi: 10.3389/fpsyt.2019.00126
Tabacaru, M., Kopainsky, K., Stave, K., and Skaza, H. (2009). How can we assess whether our simulation models improve system understanding for the ones interacting with them? The 27th International Conference of the Systems Dynamics Society, Albuquerque, New Mexico, July 26–30.
Taylor, S., Calvo-Amodio, J., and Well, J. (2020). A method for measuring systems thinking learning. Systems 8:11. doi: 10.3390/systems8020011
Verhoeff, R. P., Waarlo, A. J., and Boersma, K. T. (2008). Systems modelling and the development of coherent understanding of cell biology. Int. J. Sci. Educ. 30, 543–568. doi: 10.1080/09500690701237780
Wellmanns, A., and Schmiemann, P. (2022). Feedback loop reasoning in physiological contexts. J. Biol. Educ. 56, 465–485. doi: 10.1080/00219266.2020.1858929
York, S., and Orgill, M. (2020). ChEMIST table: a tool for designing or modifying instruction for a systems thinking approach in chemistry education. J. Chem. Educ. 97, 2114–2129. doi: 10.1021/acs.jchemed.0c00382
Keywords: systems thinking, perspective taking, model based learning, life science, environmental science
Citation: Jordan RC, Gray S, Boyse-Peacor A, Sorensen AE, Frantz CM, Jauernig J, Brehm P, Shammin MR and Petersen J (2023) Promoting systems thinking through perspective taking when using an online modeling tool. Front. Educ. 8:1215436. doi: 10.3389/feduc.2023.1215436
Edited by:
Sara Wyse, Bethel University (Minnesota), United StatesReviewed by:
Caleb Trujillo, University of Washington Bothell, United StatesJoe Dauer, University of Nebraska System, United States
Copyright © 2023 Jordan, Gray, Boyse-Peacor, Sorensen, Frantz, Jauernig, Brehm, Shammin and Petersen. This is an open-access article distributed under the terms of the Creative Commons Attribution License (CC BY). The use, distribution or reproduction in other forums is permitted, provided the original author(s) and the copyright owner(s) are credited and that the original publication in this journal is cited, in accordance with accepted academic practice. No use, distribution or reproduction is permitted which does not comply with these terms.
*Correspondence: Rebecca C. Jordan, jordanre@msu.edu