- 1Department of Psychology, Institute of Psychiatry, Psychology and Neuroscience, King’s College London, London, United Kingdom
- 2Centre for Technology Enhanced Learning, King’s College London, London, United Kingdom
The prevalence of mental distress among young adults, including those at university, has increased. In this context, learning analytics, students’ digital trace data, are increasingly being used to understand student mental health. In line with calls for more research on learning analytics from student perspectives, as part of a broader focus group study, 44 undergraduate students from three United Kingdom universities were invited to consider how they felt about having a digital footprint on their virtual learning environment (VLE). Two main themes were constructed using reflexive thematic analysis. First, students’ responses depended on the perceived threat to their privacy and identity. Some students were indifferent if no threat was perceived, but expressed unease if there was. Second, some students expressed personal preference for autonomy over use of their VLE data. Two uses identified were for non-judgmental personalized support, and using aggregated data to improve student learning. These themes suggest how the use of educational digital data can, under some circumstances, impact wellbeing negatively. The students’ perspectives garnered from the focus groups could have implications for policy and practice concerning privacy and surveillance, the possibility for misuse or misinterpretation of data, and informed consent. This small study supports the importance of partnering with students to develop and implement guidance for how VLE learning analytics data are used and interpreted by students and staff, including lecturers, to protect and enhance student mental wellbeing.
1 Introduction
Over the past decade the prevalence of mental distress among young adults in the UK, including those at university, has increased (Tabor et al., 2021; Hansard, 2023). Universities are adopting a university-wide approach to support student mental health (Hughes and Spanner, 2019). Such an approach involves considering how all aspects of the university environment impact student wellbeing. In this context, data analytics are increasingly being used to understand student mental health (e.g., Peck, 2023). However, we do not have a clear understanding of how the use of data analytics itself impacts student mental health and wellbeing.
Using data analytics within education is often termed learning analytics, which includes collecting, analyzing and reporting students’ digital trace data to understand and improve students’ learning environments, and the experience and outcomes of students’ learning (Siemens, 2013; Francis et al., 2020). There are many sources of data that an institution may draw upon, including access to physical spaces on campus, requests for academic extensions or additional support, and enrolments in clubs and societies. In this context, the Virtual Learning Environment (VLE), provides a rich data and accessible source. The VLE is a web-based learning management system that provides students with remote access to course-related content (e.g., lecture slides, reading lists, assessment feedback) along with synchronous and asynchronous communication between peers and teaching staff. Any VLE-use creates a digital footprint, including records of content viewed, downloaded, and added to discussion boards. A VLE digital footprint also logs student identity and timestamps for their VLE activity. While these data can be used for learning analytics, there are significant ethical issues around data ownership and autonomy (Slade and Prinsloo, 2013; O’Donoghue, 2023). Using these data risks disempowering students (Broughan and Prinsloo, 2020) and prompting concerns of being judged and labelled based on their digital footprint (Slade and Prinsloo, 2014). Therefore, how VLE learning analytics are used could impact on student mental wellbeing.
Studies that have investigated the use of learning analytics from student perspectives suggest that students see potential value in learning analytics (Jones et al., 2020). If given the opportunity to view their own data, students prefer to customize their dashboard, including for goal-setting and comparing with peers (Bennett and Folley, 2019). However, students do not necessarily trust the accuracy of the analytics, the predictions made from the data, or find the predicted grades motivating (Joseph-Richard et al., 2021). Students wish to retain control over how their data are used (Slade and Prinsloo, 2014), but are not always aware of the consequences for giving consent for their data to be used (Tsai et al., 2020). While these studies provide insight into students’ views of learning analytics, Foster and Francis (2020) concluded from their review of 34 studies of the effectiveness of using learning analytics for academic outcomes that more qualitative research involving the “student voice” was needed to understand the impact of learning analytics. To our knowledge, the intersect between learning analytics and mental wellbeing is one aspect of this that has not been explored from students’ perspectives. In a study that contributes to addressing this gap in the literature, we conducted focus groups with undergraduate students to understand their perspectives on their VLE digital footprint.
2 Method
2.1 Design and recruitment
The study used an inductive qualitative approach involving focus groups designed to hear perspectives from a range of students. Participants were purposively recruited, via university-wide recruitment circulars, from different academic disciplines, year groups, and universities using different VLEs (Table 1). In 2020, twelve focus groups were conducted online and in-person. Research ethics approval was granted by the Institutional Ethical Review Committee. Participants received a £10.00 voucher.
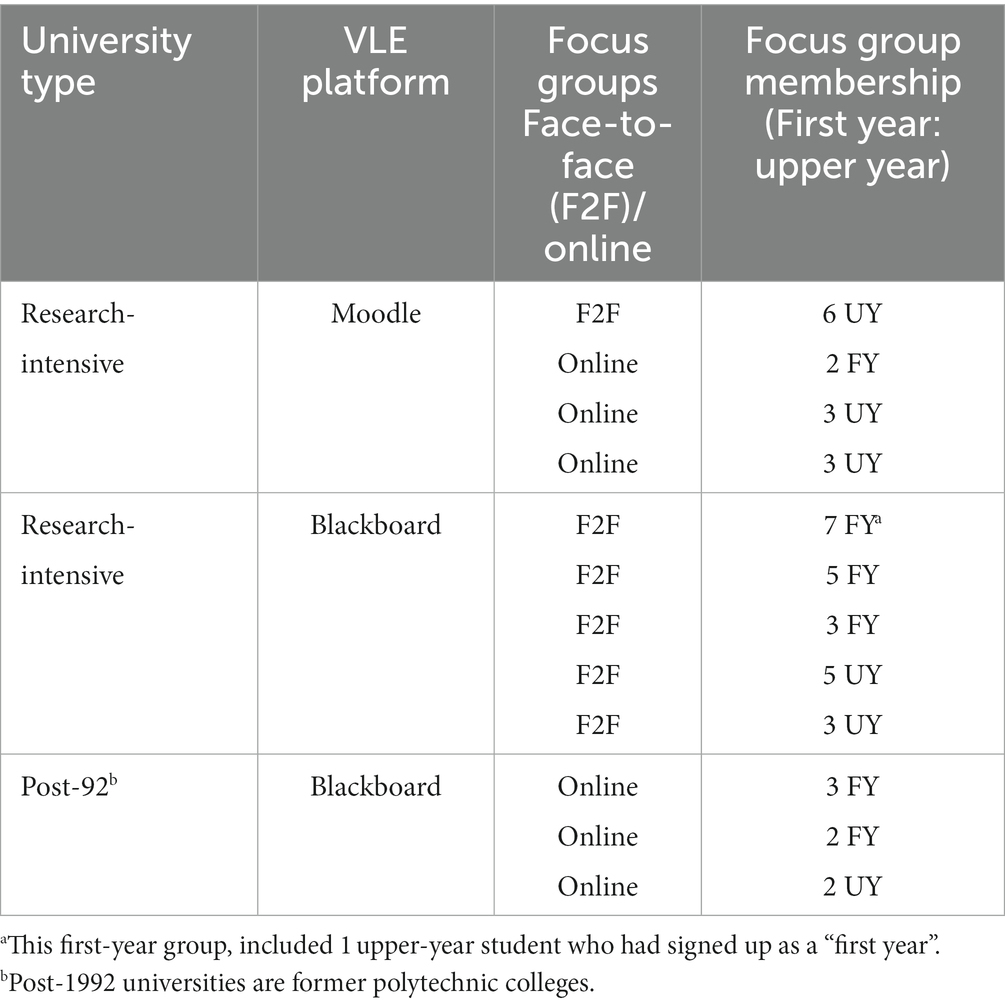
Table 1. Focus group characteristics: university-type, VLE, year group, number of participants, and mode of data collection (n = 44).
2.1.1 Participants
Participants were full-time undergraduate students enrolled on campus-based degree courses, in which a VLE was used alongside face-to-face teaching and learning.
2.2 Procedures
During each focus group (approximately 75 min), participants were prompted to describe their experience of using their VLE, including their pattern of use, and encouraged to explore these experiences in relation to their mental wellbeing (see Supplementary Materials for Topic Guide). Within each group, participants were also asked how they felt about having a “digital footprint” on the VLE, for example, “So how do you feel about your module leader having access to your activity on Moodle/Blackboard?”
2.3 Data analysis
Focus groups were transcribed, anonymized and analyzed, assisted by NVivo12 software and paper and pen. First-year and upper-year participants were denoted FY and UY, respectively. Reflexive thematic analysis with an inductive approach was used to code and generate themes, defined as “patterns of shared meaning” with reference to the research question (Braun and Clarke, 2019, 592). This iterative process involved: familiarization with data, coding, generating initial themes, reviewing themes, naming themes, and writing the report (Braun and Clarke, 2006, 2019). Codes were generated independently by two coders (KER & RJU) and discussed to explore different possible interpretations (Terry et al., 2017). Following a critical realist approach, semantic and latent themes were generated (Braun and Clarke, 2006). This paper reports the themes generated from students’ responses to the focus group question about their digital footprint (Supplementary Materials, Topic Guide, question 10). The other themes from the focus group examine students’ experiences of using their VLE to study. Those themes are reported in Rakow et al. (2023), and their names are reproduced in this paper’s Supplementary Materials. The themes regarding students’ attitudes towards the digital footprint have been reported separately, here in this paper, because these themes related closely to learning analytics.
3 Results
Two themes, each with two sub-themes, were constructed: (1) Responses depend on perceived threat to privacy and identity; (2) Personal preference for autonomy over use of their VLE data.
3.1 Responses depend on perceived threat to privacy and identity
Participants’ reactions varied in response to the suggestion that lecturers knew how their students were using the VLE. Several participants had not contemplated having a digital footprint on the VLE, and raising the topic during the focus groups triggered alarmed responses such as “They do? Wait? What? Really?” (FY20), “what, how, how, far does it go? What, what can they see?” (FY18), or simply, “I did not know that was a thing” (UY30), or “Is it anonymized?” (FY18). Overall, students’ reactions varied from indifference to anxiety. These reactions depended on the level of perceived threat to their privacy and identity.
3.1.1 Indifference if no perceived threat
Some participants expressed indifference about lecturers having access to these data. Although the participants’ explanations varied, their indifference seemed to be underpinned by a belief that their lecturers would not use information about their students’ VLE activity to harm them. These students did not believe that lecturers’ judgement based on the data could undermine their sense of worth or expose students to data misuse. One student noted that they “would not really feel judged” by lecturers for their online activity, because some lecturers “send emails at like 12 o’clock at night” (UY22). For this student, knowing that some lecturers are “as bad as we are (…) everyone’s constantly on there” assuaged any concerns about having a digital footprint on the VLE (UY22). In the same focus group, another student “could not really care less” (UY21), while a third student commented “I do not really think about it when I’m on social media or stuff anyway, (…) even less so on Blackboard” (UY23). In a different focus group, one student did “not see any problem with it” because “it’s not like there is anything sensitive,” equating their VLE activity with being seen “rummaging around (…) lecture notes” (FY36). Another student was “not bothered” by having a digital footprint because they were confident that the information that their lecturers could access was limited, and that made it difficult for lecturers to infer very much from their VLE data trail (UY8).
Some students’ indifference was associated with lack of evidence that their lecturers had used their VLE digital footprint. One student did not believe that lecturers would use the data: “They’ve got a thousand things to do. I do not think they are particularly interested (. …) their role is, their relationship with you is, what you learn rather than necessarily how you learn” (UY26). Another student thought it was “just not realistic” for lecturers to follow up students based on which lectures they watched or attended (UY8). Other students felt “indifferent” (FY1, FY7) about their digital footprint, because their lecturer did not “really pay attention” to their VLE engagement (FY1) or “never asked” (FY7) or were “not asking questions about it” (FY1).
Some students expressed ambivalence or uncertainty about lecturers accessing their VLE data. A few students seemed to be weighing up the risks, “I have mixed feelings (…) it’s a bit frustrating for someone to be keeping track of what I’m doing but at the same time, I mean, I’m not too bothered by it if I know what I’m doing and I’m getting things done” (FY37).
3.1.2 Concern about surveillance, lack of control over privacy and being misjudged
Privacy mattered to students. Several students felt that having teaching staff monitoring their individual VLE activity, beyond “tracking” data usage for trends, was an “invasion of privacy” (UY30). The idea of a lecturer observing them studying was uncomfortable, “I mean, it’s my business” (UY34). A few students found it “scary” (FY16, UY31) or “intimidating” (FY16) that lecturers might “watch” (UY33) or “monitor” (UY31, UY33) their VLE activity. This concept made one student feel “anxious (…) like there’s like a presence over me” (UY33). Other students were concerned about the potential for misuse arising from surveillance, asserting that it would not be appropriate for lecturers to “monitor” (FY16) or “penalize” (FY19) students based on whether they have used the VLE. Other students highlighted the potential for harming relationships between lecturers and students:
Your personal tutor is there is to help with you mentally and help you get the best of your degree (…). But at the same time if they are doing this (…) I think that will be a breach of trust between me and them and I’d feel a bit betrayed that they were allowed to go and look at my data and (…) judge me as an individual based on that (FY6).
Students wanted to be judged on their output, not their process of learning. Students were concerned about being “judge[d]” (FY20) based on when they opened their documents (UY31) or what they looked at or downloaded (FY6), “you want your final work to be what is judged, not anything before that” (UY32). Another student reflected, “if they were to, I think I would feel a bit more stressed especially if they could see my submission times and stuff” (FY7).
Students were concerned that their VLE digital footprint left an inaccurate representation of the effort they were investing in their academic studies. For instance, if students preferred to download materials and work offline (UY10), or study materials that were not sourced from the VLE (FY6, UY10), they may appear to be less diligent; “some people they can just go on Blackboard and that’s brilliant for them but like that’s not how everyone will learn best and how they’ll succeed” (FY2). Conversely, some students kept their VLE “constantly (…) just open in the background on another tab anyway, so it would not be very reflective of what I’m actually doing” (FY5).
Privacy about their VLE use also mattered because it could represent personal pain. One student recounted how they “got told off” publicly in front of peers about their VLE use by their personal tutor, “UY4 has not looked at this” (UY4). Their personal tutor’s behavior felt like an invasion of privacy when being asked to justify their inactivity on the VLE. This was particularly challenging when this student did not wish to discuss personal reasons for their VLE activity. Insensitive discussion of students’ VLE data by lecturers can impact students negatively. This impact on students can be worsened if data are misunderstood or misinterpreted, and if students are challenged publicly.
3.2 Personal preferences for autonomy over use of their VLE data
The variety of reactions to, and preferences for, the ways in which lecturers could and should use students’ digital footprint highlighted the complexity and emotions associated with it. The importance of students controlling how their data are used was reflected in suggestions for either an “opt-in (…) or maybe opt-out” system so that lecturers “cannot really use [student VLE data] to (…) identify you and say “oh, you are not doing this” (FY18). Students volunteered potential conditions under which lecturer use of their VLE data would be appropriate: (1) for personalized support, and (2) when data are aggregated.
3.2.1 Non-judgmental personalized support
A few students stipulated that if lecturers were to use their data individually, it should be used for non-judgmental tailored support. For instance, one student who “had a different view on [their] personal data” wanted their lecturers to use their VLE digital footprint to notice them as individuals, rather than within a mass of 400 students in a lecture theatre:
I would love for them to use it even more (…) I think that (…) they can see “Oh, like they did not submit this assignment whereas usually they do and they have good grades. Something might be wrong, and we can go and help them.” So, because otherwise it really feels like we are just cattle, that we are just a big group of people, a bunch of numbers (UY24).
Other students also thought that their VLE footprint could be used to notice individual students and offer them non-judgmental support, “I expect like there’s a certain level of tracking, for example statistics or, just checking “oh, have all of my students opened the worksheet yet? If they have not, is there a reason? Are they okay?” (UY30).
3.2.2 Aggregated data to improve student learning
A few students suggested that instead of using individual-level data, a more appropriate use of student VLE data would be to aggregate data to enable lecturers to improve course delivery (FY18). These students would be comfortable with lecturers using their data for “research purposes (…) if they have [the] purpose of saying, “okay we want to (…) see how we can (…) really improve our course”” (FY20), including reading lists and resources (UY12, UY29). One student surmised that the purpose of the VLE digital footprint might be for lecturers to ascertain whether students could find resources “properly,” but the student countered “but if I cannot find something, I’ll ask, so. I mean, I would not have a problem with it, but I do not see the use in it” (FY36). Therefore, reliance on these VLE data instead of communicating directly with students could limit lecturers’ insight into ways to improve students’ learning.
4 Discussion
Responses to our question about the digital footprint suggested that student reactions to learning analytics varied. The participants’ responses can be grouped into three areas: first, privacy and surveillance; second, the possibility for misuse or misinterpretation of data; and third, informed consent. Students had concerns about privacy and inappropriate judgement arising from poorly understood data. Students could feel threatened and disempowered if they perceived lecturers would not evaluate their VLE digital footprint fairly. However, when these perceived threats were absent, students were indifferent about having a digital footprint and a few students saw the potential for lecturers using these data to provide non-judgmental support. Some students valued autonomy over what their lecturers can know about their VLE use, and the conditions under which their lecturers could access and use these data.
4.1 Privacy and surveillance, managing perceived threat and wellbeing
Some participants perceived surveillance by lecturers as threating and anxiety-provoking, because they did not want to be evaluated based on their VLE activity. Likening the use of learning analytics to Foucault’s Panopticon, “where structural design allows a central authority to oversee all activity,” Slade and Prinsloo (2013, 1511) highlight a power imbalance, when a student cannot access their own data that their lecturer can. Some of our participants’ discussions support the concern that using learning analytics for surveillance could contribute to student performativity on the VLE (Gourlay, 2017; Macfarlane and Tomlinson, 2017). Indeed, Wintrup (2017) argues that surveillance could incentivize students to engage in behaviors that might not necessarily contribute to their knowledge or understanding. The JISC Code of Learning Analytics advises HEIs to “ensure that knowledge that their activity is being monitored does not lead to (…) negative impacts on their academic progress or wellbeing” (Sclater and Bailey, 2015). Responses from some participants exemplify a kind of negative effect on student wellbeing that the JISC Code of Practice warns against.
Several participants explicitly stated that they did not want data that made them identifiable to be shared with others, although some suggested that aggregated VLE data could be used constructively by teaching staff to improve the curriculum. In line with these participants’ views, 77% of UK HE students surveyed were “fine” with their anonymous grade and course data being used to predict students’ academic performance or retention (Hewitt and Natzler, 2019). According to data protection legislation, only ‘personal’ data captured on the VLE are categorized as ‘sensitive’ or ‘special category’ data (Sclater and Bailey, 2015). However, our participants’ responses suggest that some students consider their academic data to be sensitive. JISC warns that students’ data and analytics should not be shared with third parties without students’ explicit consent and that measures should be taken to protect students from being identifiable within aggregated data (Sclater and Bailey, 2015). However, the JISC code of ethical practice is referring to third parties beyond the university. Our participants’ discussions indicate that some students have strong feelings about limiting who within the university can access their data, including, lecturers, personal tutors, academic advisers, or specialist university-wide support staff. This suggests that students should be treated as “data owners,” not “data objects” and so contribute as “equal partners” (Broughan and Prinsloo, 2020, 618–619) to develop guidance about protecting privacy of their data within each HEI.
4.2 Data misinterpretation
Some participants were concerned that their VLE use could be misinterpreted by their lecturers. Some concerns stemmed from a potential mismatch between students’ effort invested into their academic work and what is captured via the VLE. VLE ‘click’ data do not necessarily reflect academic effort or engagement in learning, because students study offline (Maltby and Mackie, 2009) or with peers (Wintrup, 2017). Students’ concerns are valid because VLE activity data can have little predictive power for academic performance (Tempelaar et al., 2015). Our participants’ responses support concerns that using students’ VLE digital footprint as a proxy for academic engagement could result in student behavior being misinterpreted as apathy or disengagement (Macfarlane and Tomlinson, 2017; Wintrup, 2017).
The JISC Code of Practice for Learning Analytics recognizes that some uses of learning analytics can harm students (Sclater and Bailey, 2015). Our participants’ discussions emphasize that such misunderstandings could harm student-staff relationships, for example if students feel judged and interrogated based on inaccurate assumptions. In contrast, positive staff-student relationships can promote learning and wellbeing (Ryan and Deci, 2017). For example, students associate their lecturers’ empathy and communication skills with their sense of wellbeing (Baik et al., 2019). How academics use student VLE activity data and discuss the data with their students may impact staff-student relationships and consequently student wellbeing.
4.3 Consent – transparency about how student VLE data are collected and used
Transparency with students about how their learning analytics data will be used has been emphasized as a vital ethical matter (e.g., Slade and Prinsloo, 2013; Slade and Prinsloo, 2014; Ferguson, 2019). However, very few of our participants seemed to know whether their VLE digital footprint was being used by their lecturers, and if so, how. Moreover, many participants did not seem to know what their digital footprint on the VLE consisted of. This suggests that any consent students have given is unlikely to have been fully informed. Research on university-held student data find relatively low levels of awareness among students for how their data are used (Roberts et al., 2016; Tsai et al., 2020). While policies may specify that data use should be transparent and that students should have the opportunity to give informed consent (Sclater and Bailey, 2015), our participants’ experiences indicate a disconnect between policy and practice.
Transparency through fully informed consent should mean that, before giving consent, students know and understand which of their VLE data will be used, how and with whom. Our participants valued transparency about how their data are used, or preferred their VLE data were aggregated before being shared. Some students wanted their data to be used to provide personalized timely non-judgmental support, aligning with findings from other student focus groups (Tsai et al., 2020). Our participants’ range of perspectives on how their data could be used productively suggests that universities may need to enable students to opt-in or opt-out for how their VLE data are used at both individual and aggregate level (Slade and Prinsloo, 2013, 2014; Roberts et al., 2016; Selwyn, 2019). Broughan and Prinsloo (2020) endorse the University of California’s policy of the university being stewards of student data, over which students maintain ownership and control. Nonetheless, if students do not understand how their VLE data can be used for their benefit, students who want personalized support via their VLE data might opt-out of their data being used, paradoxically undermining their autonomy and reinforcing the power-imbalance (O’Donoghue, 2023). Therefore, together these insights indicate that further work is needed to ensure students are genuinely equipped to give informed consent (Roberts et al., 2016; Wintrup, 2017; Tsai et al., 2020).
4.4 Student digital footprint, autonomy, relationships, and mental wellbeing
Student autonomy over their VLE data trail and how this might affect student-lecturer relationships are two threads running through the findings and the ethical issues discussed above. According to Basic Psychological Needs Theory, autonomy and relatedness are two basic needs that are essential for flourishing and mental well-being (Ryan and Deci, 2017). In contrast, when these needs are thwarted, psychological ill-being is likely to be experienced. Autonomy is feeling “willingness,” “volition” and “ownership” over one’s actions, so that one’s actions are self-endorsed rather than a result of coercion or pressure (Ryan and Deci, 2017, 86). Relatedness includes “experiencing others as responsive and sensitive (…) and having a sense of belonging” (Ryan and Deci, 2017, 86) and is “satisfied by connecting to and feeling significant to others” (Vansteenkiste et al., 2020, 3). Educators can play a role in supporting the satisfaction of these psychological needs (Vansteenkiste et al., 2020).
Ideally, educators create autonomy-supportive environments by enabling students to make informed choices, without coercion or controlling methods, and exploring their students’ perspectives about learning instead of making assumptions based on superficial indicators (Black and Deci, 2000; Sheldon and Krieger, 2007). Findings from our study suggest that facilitating student autonomy over the use of their VLE digital footprint could impact students’ mental wellbeing positively and reduce the risk of perceived threat or harm. Therefore, HEIs should consider whether and how their VLE policies, systems and training for VLE-use for staff and students make it possible to create autonomy-supportive environments.
4.5 Strengths, limitations, and future directions
There have been calls for more qualitative research conducted on learning analytics from the student perspective (Foster and Francis, 2020). Recruiting a diverse group of participants and using reflexive thematic analysis enabled us to explore a range of student perspectives. However, our study has several limitations. Our study did not set out to evaluate the effectiveness of using VLE analytics per se, but used an inductive approach to understand the students’ perspective of using these types of analytics. We asked one question about the digital footprint within the VLE use. Future research would benefit from exploring students’ attitudes to VLE use alongside objective insights into how the students’ lecturers are using the students’ VLE digital footprint. We did not define the term ‘digital footprint’ for students. This decision enabled us to identify student knowledge gaps. Further investigation here would be beneficial to confirm whether students are receiving adequate information from their HEI about digital footprint and how they understand this guidance.
Our focus group students raised issues associated with privacy, trust, misunderstanding and consent. Further research is needed to establish the generality of university students’ experiences of and attitudes toward these issues. Future research would benefit from investigating what students know about their digital footprint within different university data systems and in different jurisdictions for data regulation. Further research exploring student perceptions of the use of VLE data in the context of other student data (e.g., library, sports, activities access) is also recommended. It would be important to explore which data students want to be used, how they want it to be used by themselves and university staff, and for what purposes. Exploring how trust could be built, maintained, or undermined in the use of student digital footprint could also be explored.
5 Conclusion
Reactions from focus group students suggest that despite policies, training and practice, students can feel vulnerable and exposed if their digital data are used inappropriately, even if those data might not technically be categorized as sensitive. Current policies on using learning analytics refer to sharing data with third parties outside each student’s HEI. However, focus group students were concerned about data-sharing within their university. Consequently, our findings point to the importance of student autonomy over who accesses their VLE data and how these data are used and interpreted. More work is needed to ensure lecturer use of student VLE data does not undermine the privacy of students or misinterpret their data. Together, these can undermine autonomy and relatedness, both of which are important conditions for mental wellbeing. These findings support Broughan and Prinsloo’s (2020, 625) recommendation for students to be involved as “equal partners” to develop and implement guidance for students and staff for how VLE student data are used for learning analytics.
Data availability statement
The raw data supporting the conclusions of this article will be made available by the authors, without undue reservation.
Ethics statement
The studies involving humans were approved by the Institutional Ethics Committee of King’s College London (LRS-18/19–8,512 on 6 November 2018; RESCM-19/20–8,512 on 16 March 2020). The studies were conducted in accordance with the local legislation and institutional requirements. The participants provided their written informed consent to participate in this study.
Author contributions
KR, ED, and NB contributed to the study design and material preparation. KR collected, analyzed the data, and wrote the first draft of the manuscript. RU contributed to the analysis. All authors contributed to manuscript revision, read, and approved the submitted version.
Funding
The author(s) declare financial support was received for the research, authorship, and/or publication of this article. This research was funded by the Economic and Social Research Council [Grant Reference Numbers: ES/P000703/1 (KER LISS-DTP PhD studentship) and ES/S00324X/1 (NB & ED)].
Acknowledgments
Thanks to students who participated in the focus groups.
Conflict of interest
The authors declare that the research was conducted in the absence of any commercial or financial relationships that could be construed as a potential conflict of interest.
Publisher’s note
All claims expressed in this article are solely those of the authors and do not necessarily represent those of their affiliated organizations, or those of the publisher, the editors and the reviewers. Any product that may be evaluated in this article, or claim that may be made by its manufacturer, is not guaranteed or endorsed by the publisher.
Supplementary material
The Supplementary material for this article can be found online at: https://www.frontiersin.org/articles/10.3389/feduc.2023.1208671/full#supplementary-material
References
Baik, C., Larcombe, W., and Brooker, A. (2019). How universities can enhance student mental wellbeing: the student perspective. High. Educ. Res. Dev. 38, 674–687. doi: 10.1080/07294360.2019.1576596
Bennett, L., and Folley, S. (2019). Four design principles for learner dashboards that support student agency and empowerment. J. App. Res. High. Educ. 12, 15–26. doi: 10.1108/jarhe-11-2018-0251
Black, A. E., and Deci, E. L. (2000). The effects of instructors’ autonomy support and students’ autonomous motivation on learning organic chemistry: a self-determination theory perspective. Sci. Educ. 84, 740–756. doi: 10.1002/1098-237X(200011)84:6<740::AID-SCE4>3.0.CO;2-3
Braun, V., and Clarke, V. (2006). Using thematic analysis in psychology. Qual. Res. Psychol. 3, 77–101. doi: 10.1191/1478088706qp063oa
Braun, V., and Clarke, V. (2019). Reflecting on reflexive thematic analysis. Qual. Res. Sport Exer. Health 11, 589–597. doi: 10.1080/2159676x.2019.1628806
Broughan, C., and Prinsloo, P. (2020). (Re)centring students in learning analytics: in conversation with Paulo Freire. Assess. Eval. High. Educ. 45, 617–628. doi: 10.1080/02602938.2019.1679716
Ferguson, R. (2019). Ethical challenges for learning analytics. J. Learn. Analyt. 6, 25–30. doi: 10.18608/jla.2019.63.5
Foster, C., and Francis, P. (2020). A systematic review on the deployment and effectiveness of data analytics in higher education to improve student outcomes. Assess. Eval. High. Educ. 45, 822–841. doi: 10.1080/02602938.2019.1696945
Francis, P., Broughan, C., Foster, C., and Wilson, C. (2020). Thinking critically about learning analytics, student outcomes, and equity of attainment. Assess. Eval. High. Educ. 45, 811–821. doi: 10.1080/02602938.2019.1691975
Gourlay, L. (2017). Student engagement, ‘Learnification’ and the Sociomaterial: critical perspectives on higher education policy. High Educ. Pol. 30, 23–34. doi: 10.1057/s41307-016-0037-1
Hansard. (2023). Higher Education Students: statutory duty of care [Hansard]. (vol. 733). Available at: https://hansard.parliament.uk/commons/2023-06-05/debates/9BA59E93-4342-4AD6-BA94-379DCA6A24E0/HigherEducationStudentsStatutoryDutyOfCare
Hewitt, Rachel, and Natzler, Michael. (2019). Students or data subjects? What students think about university data security. Oxford: HEPI.
Hughes, Gareth, and Spanner, Leigh. (2019). The university mental health charter. Leeds: Student Minds.
Jones, K. M. L., Asher, A., Goben, A., Perry, M. R., Salo, D., Briney, K. A., et al. (2020). “We’re being tracked at all times”: student perspectives of their privacy in relation to learning analytics in higher education. J. Assoc. Inf. Sci. Technol. 71, 1044–1059. doi: 10.1002/asi.24358
Joseph-Richard, P., Uhomoibhi, J., and Jaffrey, A. (2021). Predictive learning analytics and the creation of emotionally adaptive learning environments in higher education institutions: a study of students’ affect responses. Int. J. Inf. Learn. Technol. 38, 243–257. doi: 10.1108/IJILT-05-2020-0077
Macfarlane, B., and Tomlinson, M. (2017). Critiques of Student Engagement. High Educ. Pol. 30, 5–21. doi: 10.1057/s41307-016-0027-3
Maltby, A., and Mackie, S. (2009). Virtual learning environments – help or hindrance for the ‘disengaged’ student? ALT-J 17, 49–62. doi: 10.1080/09687760802657577
O’Donoghue, K. (2023). Learning analytics within higher education: autonomy, beneficence and non-maleficence. J. Acad. Ethics 21, 125–137. doi: 10.1007/s10805-021-09444-y
Peck, Edward. (2023). Student analytics: a core specification for engagement and wellbeing analytics report. Bristol: JISC.
Rakow, K., Upsher, R., Foster, J., Byrom, N., and Dommett, E. (2023). “It Ain’t what you use, It’s the way that you use it”: how virtual learning environments may impact student mental wellbeing. Educ. Sci. 13, 1–20. doi: 10.3390/educsci13070749
Roberts, L. D., Howell, J. A., Seaman, K., and Gibson, D. C. (2016). Student attitudes toward learning analytics in higher education: “the Fitbit version of the learning world”. Front. Psychol. 7:1959. doi: 10.3389/fpsyg.2016.01959
Ryan, Richard M., and Deci, Edward L.. (2017). Self-determination theory: basic psychological needs in motivation, development, and wellness. United Kingdom: The Guilford Press.
Sclater, N., and Bailey, P. (2015). Code of practice for learning analytics. Bristol: JISC. https://www.jisc.ac.uk/guides/code-of-practice-for-learning-analytics
Selwyn, N. (2019). What’s the problem with learning analytics? J. Learn. Anal. 6, 11–19. doi: 10.18608/jla.2019.63.3
Sheldon, K. M., and Krieger, L. S. (2007). Understanding the negative effects of legal education on law students: a longitudinal test of self-determination theory. Personal. Soc. Psychol. Bull. 33, 883–897. doi: 10.1177/0146167207301014
Siemens, G. (2013). Learning analytics: the emergence of a discipline. Am. Behav. Sci. 57, 1380–1400. doi: 10.1177/0002764213498851
Slade, S., and Prinsloo, P. (2013). Learning analytics ethical issues and dilemmas. Am. Behav. Sci. 57, 1510–1529. doi: 10.1177/0002764213479366
Slade, S., and Prinsloo, P. (2014). “Student perspectives on the use of their data: between intrusion, surveillance and care” in Challenges for research into Open & Distance Learning: doing things better – Doing better things, 291–300. Available at: https://oro.open.ac.uk/41229/
Tabor, E., Patalay, P., and Bann, D. (2021). Mental health in higher education students and non-students: evidence from a nationally representative panel study. Soc. Psychiatry Psychiatr. Epidemiol. 56, 879–882. doi: 10.1007/s00127-021-02032-w
Tempelaar, D. T., Rienties, B., and Giesbers, B. (2015). In search for the most informative data for feedback generation: learning analytics in a data-rich context. Comput. Hum. Behav. 47, 157–167. doi: 10.1016/j.chb.2014.05.038
Terry, Gareth, Hayfield, Nikki, Clarke, Victoria, and Braun, Virginia. (2017). “Thematic analysis.” In The SAGE handbook of qualitative research in psychology. SAGE Publications Ltd. 17–36.
Tsai, Y.-S., Perrotta, C., and Gašević, D. (2020). Empowering learners with personalised learning approaches? Agency, equity and transparency in the context of learning analytics. Assess. Eval. High. Educ. 45, 554–567. doi: 10.1080/02602938.2019.1676396
Vansteenkiste, M., Ryan, R. M., and Soenens, B. (2020). Basic psychological need theory: advancements, critical themes, and future directions. Motiv. Emot. 44, 1–31. doi: 10.1007/s11031-019-09818-1
Keywords: learning analytics, student voice, wellbeing, privacy, surveillance, consent
Citation: Rakow KE, Upsher RJ, Foster JLH, Byrom NC and Dommett EJ (2023) Student perspectives on their digital footprint in virtual learning environments. Front. Educ. 8:1208671. doi: 10.3389/feduc.2023.1208671
Edited by:
Alan F. Smeaton, Dublin City University, IrelandReviewed by:
Daniel García-Pérez, Complutense University of Madrid, SpainSusana Henriques, Universidade Aberta (UAb), Portugal
Copyright © 2023 Rakow, Upsher, Foster, Byrom and Dommett. This is an open-access article distributed under the terms of the Creative Commons Attribution License (CC BY). The use, distribution or reproduction in other forums is permitted, provided the original author(s) and the copyright owner(s) are credited and that the original publication in this journal is cited, in accordance with accepted academic practice. No use, distribution or reproduction is permitted which does not comply with these terms.
*Correspondence: Katie E. Rakow, Katie.Rakow@kcl.ac.uk