- Higher Colleges of Technology, Ras Al Khaimah, United Arab Emirates
Introduction: This study explores the effects of Artificial Intelligence (AI) chatbots, with a particular focus on OpenAI’s ChatGPT, on Higher Education Institutions (HEIs). With the rapid advancement of AI, understanding its implications in the educational sector becomes paramount.
Methods: Utilizing databases like PubMed, IEEE Xplore, and Google Scholar, we systematically searched for literature on AI chatbots’ impact on HEIs. Our criteria prioritized peer-reviewed articles, prominent media outlets, and English publications, excluding tangential AI chatbot mentions. After selection, data extraction focused on authors, study design, and primary findings. The analysis combined descriptive and thematic approaches, emphasizing patterns and applications of AI chatbots in HEIs.
Results: The literature review revealed diverse perspectives on ChatGPT’s potential in education. Notable benefits include research support, automated grading, and enhanced human-computer interaction. However, concerns such as online testing security, plagiarism, and broader societal and economic impacts like job displacement, the digital literacy gap, and AI-induced anxiety were identified. The study also underscored the transformative architecture of ChatGPT and its versatile applications in the educational sector. Furthermore, potential advantages like streamlined enrollment, improved student services, teaching enhancements, research aid, and increased student retention were highlighted. Conversely, risks such as privacy breaches, misuse, bias, misinformation, decreased human interaction, and accessibility issues were identified.
Discussion: While AI’s global expansion is undeniable, there is a pressing need for balanced regulation in its application within HEIs. Faculty members are encouraged to utilize AI tools like ChatGPT proactively and ethically to mitigate risks, especially academic fraud. Despite the study’s limitations, including an incomplete representation of AI’s overall effect on education and the absence of concrete integration guidelines, it is evident that AI technologies like ChatGPT present both significant benefits and risks. The study advocates for a thoughtful and responsible integration of such technologies within HEIs.
1. Introduction
On November 30, 2022, the AI based chatbot called ChatGPT (Chat Generative Pre-trained Transformer) was launched as a prototype by OpenAI and rapidly gathered media attention for its comprehensive and articulate responses to questions spanning many domains of technical and professional knowledge (GPT, 2022). ChatGPT is an AI-based natural language processing (NLP) system proficient in mimicking human-like communication with the end user. This virtual assistant enables responding to inquiries and supporting activities like crafting emails, writing essays, generating software code, and so on (Ortiz, 2022). This AI-based tool was initially offered open to the public free of charge because the launched demo and research version GPT-3.5 was intended to allow widespread general experimentation to get reinforcement learning from human feedback to be incorporated in the next version of GPT-4 (Goldman, 2022).
ChatGPT is a conversational AI chatbot engineered by OpenAI, a collective of researchers and technologists focused on constructing AI securely and responsibly. OpenAI was founded in 2015 by a team of tech innovators, and it has received substantial funding from tech giants such as Microsoft, Amazon, and Alphabet. The development of ChatGPT builds upon the tremendous advancements in the field of NLP. The GPT architecture has seen several iterations, with each new version achieving superior language generation, accuracy, and speed performance. The chatbot has been acclaimed as a breakthrough in NLP and used in various contexts, including customer service, education, and healthcare. In the field of learning, ChatGPT has been employed as an educational aid, replying to pupils’ questions, giving feedback, and helping virtual conversations. ChatGPT can also be a writing helper, aiding people create grammatically accurate and logical text.
ChatGPT is a product of the GPT architecture, a leading-edge NLP model conditioned on copious amounts of text information to generate language similar to humans (GPT, 2022). A transformer is a deep learning model proposed by Vaswani et al. (2017), which introduced a self-attention approach that allows for a differential weighting of each input data component.
The revolutionary approach of transformers has been considered the most recent breakthrough in AI. Indeed, Chance (2022) describes transformers as deep learning models that allow expressing inputs in natural language to generate outputs like translations, text summaries, grammar and writing style correction, etc. Bellapu (2021) highlights the singularity of transformers as the amalgamation of convolutional neural networks and recurrent neural networks, with advantages such as better accuracy, faster processing, working with any sequential data, and forecasting.
Since its 2022 launch, AI chatbots like ChatGPT have sparked concerns in education. While risks about students’ independent thinking and language expression skills deteriorating exist, banning the tool from academic institutions should not be the answer (Dwivedi et al., 2023). Teachers and professors are uneasy about potential academic fraud with AI-driven chatbots such as ChatGPT (Meckler and Verma, 2022). The proficiency of ChatGPT spans from assisting in scholarly investigations to finalizing literary compositions for learners (Roose, 2022; Shankland, 2022). However, students may exploit technologies like ChatGPT to shortcut essay completion, endangering the growth of essential competencies (Shrivastava, 2022). Coursera CEO Jeff Maggioncalda believes that ChatGPT’s existence would swiftly change any education using written assessment (Alrawi, 2023).
To gauge the media impact since the launch of ChatGPT on Nov. 30, 2022, we compared Google user search interests using Google Trends. This web service displays the search volume of queries over time in charts across countries and languages – Figure 1 shows ChatGPT’s overwhelming media impact since its November 30, 2022 launch. Interest in the AI-based app exceeded Ukraine’s war, news concerning U.S. President Joe Biden, Bitcoin, and the S&P 500. The data depicted in the chart is in line with Libert (2023) findings, which show that the search interest for ChatGPT soared to 112,740%.
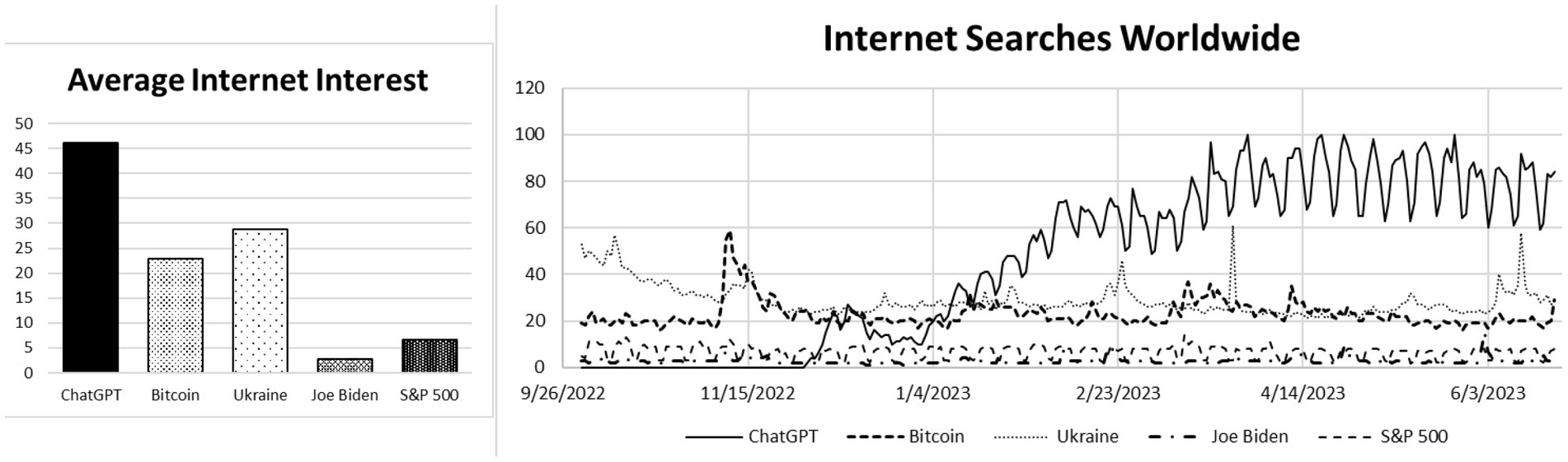
Figure 1. Search interest based on Google trends. The figures indicate search interest compared to the maximum point on the graph for the specified area and duration. A score of 100 signifies the zenith of popularity for the phrase. A rating of 50 implies the word is only half as prevalent. A value of 0 indicates insufficient data for the given term.
As a result of the significant global breakthrough represented by the launch of ChatGPT, thousands of tech leaders and researchers, including Elon Musk, have called for a pause in the development of AI systems more potent than GPT-4 for 6 months or more, during which a set of shared safety protocols should be developed and implemented. An open letter of +50 K signatories emphasizes the need for robust AI governance systems, such as new regulatory authorities, tracking systems, auditing and certification, and liability for AI-caused harm. Finally, they suggest that a pause on AI development is necessary to ensure it is used for the benefit of all and to give society a chance to adapt (Bengio et al., 2020). The call comes as tech companies race to develop and deploy more powerful AI tools in their products, leading to concerns about biased responses, misinformation, privacy, and the impact on professions and relationships with technology.
On December 5, 2022, Altman (2022), the head of OpenAI, announced via Twitter that ChatGPT had garnered over a million users in under a week since its launch. The remarkable success of the Silicon Valley-based OpenAI has allowed it to forecast $200 million in revenue in 2023 and $1 billion by 2024, which placed the company’s valuation at $20 billion in a secondary share sale by the end of 2022 (Dastin et al., 2022). A more evident appreciation of OpenAI’s achievement can be gained by comparing it with those of other successful firms such as Netflix (+177 weeks), Facebook (+43 weeks), and Instagram (+10 weeks) in reaching the 1 million users mark (ColdFusion, 2022). Similarly, Hu (2023) reports that ChatGPT had achieved 100 million monthly active users within 2 months of its unveiling, making it the fastest-growing consumer application in recorded history, according to a UBS analysis.
ChatGPT4 was launched on March 14, 2023, and provides makers, developers, and creators with a powerful tool to generate labels, classify visible features, and analyze images. Compared to GPT-3.5, ChatGPT4 is more dependable, imaginative, and interactive and can tackle longer passages in one request because of the expanded setting length. Moreover, GPT-4 can handle textual and visual prompts and give both back, although the capacity to employ picture input is yet to be made available to the public. Furthermore, GPT-4 is more than 85% accurate in 25 languages, including Mandarin, Polish, and Swahili, and can write code in all major programming languages. Microsoft has brought out its Bing AI chatbot equipped with GPT-4 (Elecrow, 2023).
Recent news already provides information about the subsequent versions of ChatGPT. Indeed, Smith (2023) informs that OpenAI is working on the next major software upgrade for ChatGPT, GPT-5, which is expected to launch in winter 2023. If a report about the GPT-5 abilities is correct, it could bring ChatGPT to the point of AGI, making it indistinguishable from a human. OpenAI expects the intermediate ChatGPT version of GPT-4.5 to be launched in September or October 2023 if GPT-5 cannot be ready at that time (Chen, 2023).
Since its inception on November 30, 2022, ChatGPT has incited a notable amount of research articles. The deluge of scholarly works increases daily, making it unfeasible to offer an updated overview of the papers written regarding ChatGPT without becoming outdated in a few days or weeks. Some examples of such articles include Zhai (2023), who established that ChatGPT could resolve the most challenging issues in science education through automated assessment production, grading, guidance, and material suggestion. Similarly, Lund and Agbaji (2023) find that interest in using ChatGPT to benefit one’s community was associated with information and privacy literacy but not data literacy among four northern Texas county residents.
Similarly, Susnjak (2022) findings suggest that ChatGPT can successfully replicate human-written text, raising doubt about the security of online tests in tertiary education. Likewise, Biswas (2023a) suggests that ChatGPT can be used to help improve the accuracy of climate projections through its ability to generate and analyze different climate scenarios based on a wide range of data inputs, including model parameterization, data analysis and interpretation, scenario generation, and model evaluation. Equally, Biswas (2023b) underlines the power of OpenAI’s language model ChatGPT to advise people and groups in forming prudent judgments concerning their health and probes the potential applications of this chatbot in public health, as well as the upsides and downsides of its implementation. In the same way, Sobania et al. (2023) evaluated ChatGPT’s proficiency at fixing bugs on QuixBugs and concluded that it was equivalent to CoCoNut and Codex - two widely used deep learning approaches – and was superior to typical program repair methods.
Likewise, Pavlik (2023) illustrates the potential and boundaries of ChatGPT by co-creating a paper with it and provides musings on the effects of generative AI on journalism and media education. Jeblick et al. (2022) conducted a probing analysis of 15 radiologists who asked about the quality of radiology reports produced by ChatGPT. Most radiologists agreed that the simplified reports were precise, thorough, and risk-free. Still, a few misstatements, missed medical particulars, and potentially detrimental segments were noticed. Equally, Gao et al. (2022) tested ChatGPT by generating research abstracts from titles and journals in 10 high-impact medical journals (n = 50). AI output detector identified the most generated abstracts (median of 99.98%) with a 0.02% probability of AI-generated output in original abstracts. Human reviewers identified 68% of generated abstracts but mistook 14% of original abstracts for generated.
Additionally, Chavez et al. (2023) suggest a neural network approach to forecast student outcomes without relying on personal data like course attempts, average evaluations, pass rates, or virtual resource utilization. Their method attains 93.81% accuracy, 94.15% precision, 95.13% recall, and 94.64% F1-score, enhancing the educational quality and reducing dropout and underperformance. Likewise, Kasepalu et al. (2022) find that an AI assistant can help teachers raise awareness and provide a data bank of coregulation interventions, likely leading to improved collaboration and self-regulation.
Patel and Lam (2023) discuss the potential use of ChatGPT, an AI-powered chatbot, for generating discharge summaries in healthcare. They report that ChatGPT allows doctors to input specific information and develop a formal discharge summary in seconds. Qin et al. (2023) analyze the ability of ChatGPT to perform zero-shot learning on 20 commonly used NLP datasets across seven categories of tasks. The researchers discovered that while ChatGPT excels in jobs requiring reasoning skills, it encounters difficulties performing specific tasks such as sequence tagging.
Generative Pre-trained Transformers have been used for research purposes in many areas, including climate (Alerskans et al., 2022), stock market (Ramos-Pérez et al., 2021), traffic flow (Reza et al., 2022), and flooding (Castangia et al., 2023). Additional examples of transformers being used for research purposes include predictions of electrical load (L’Heureux et al., 2022), sales (Vallés-Pérez et al., 2022), influenza prevalence (Wu et al., 2020), etcetera. Specifically, Lopez-Lira and Tang (2023) discovered that ChatGPT could accurately forecast stock market returns and surpasses traditional sentiment analysis approaches. They recommend integrating advanced language models into investment decision-making to enhance the accuracy of predictions and optimize quantitative trading strategies.
The fundamental purpose of this study is to deliver a qualitative analysis of the impact of AI chatbots like ChatGPT on HEIs by performing a scoping review of the existing literature. This paper examines whether AI chatbots can be used to enhance learning experiences and their potentially detrimental effect on the education process. Furthermore, this paper explores potential solutions to the prospective issues related to AI chatbots adopted by HEIs. Ultimately, this paper examines the existing literature on the current state of AI chatbot technology and its potential implications for future academic usage.
The novel contribution of this study resides in its comprehensive analysis of the impact of AI chatbots, particularly ChatGPT, on HEIs, synthesized through a detailed scoping review of existing literature. The primary research questions that drive this investigation include:
1. In what ways might AI chatbots like ChatGPT potentially replace humans in academic tasks, and what are the inherent limitations of such replacement?
2. How might AI technology be harnessed to detect and deter academic fraud?
3. What are the potential risks associated with the implementation of AI chatbots in Higher Education Institutions (HEIs)?
4. What academic activities in HEIs could be potentially enhanced with the adoption of AI chatbots like ChatGPT?
5. How might AI chatbots impact the digital literacy of students and their anxiety regarding AI technology?
6. What societal and economic implications might result from the wide-scale adoption of AI chatbots?
These questions guide the study’s objectives, which include conducting a comprehensive review of existing literature to understand the current state of research, identifying trends and gaps in the literature, and informing future directions in the study of AI chatbots in HEIs. Additionally, the article highlights some critical societal and economic implications of AI adoption in HEIs, explores potential approaches to address the challenges and harness the benefits of AI integration, and underscores the need for strategic planning and proactive engagement from educators in leveraging AI technologies. This study uniquely amalgamates varied perspectives on the impact of AI chatbots in higher education, offering a broad, balanced, and nuanced understanding of this complex issue. In doing so, it aims to contribute significantly to the existing knowledge of AI in education and guide future research and policy-making in this rapidly evolving field.
2. Methods
2.1. Search strategy and databases
We used several databases to comprehensively cover the body of literature related to the impact of AI chatbots on higher education institutions. They include PubMed, Web of Science, IEEE Xplore, Scopus, Google Scholar, ACM Digital Library, ScienceDirect, JSTOR, ProQuest, SpringerLink, EBSCOhost, and ERIC. These databases were chosen due to their extensive coverage of scientific and scholarly publications across various disciplines, including technology, computer science, artificial intelligence, and education. Our search string was designed based on recent literature reviews of AI chatbots in HEIs (Okonkwo and Ade-Ibijola, 2021; Rahim et al., 2022). Our search strategy was systematic, combining relevant keywords and Boolean operators. Keywords included “ChatGPT,” “AI chatbot,” “Artificial Intelligence,” “chatbot in education,” “impact of AI chatbots on higher education,” and their variants. Our search strategy was refined to ensure that it yielded the most relevant articles for our scoping review (Peters et al., 2015).
2.2. Inclusion and exclusion criteria
To streamline the process and maintain the quality and relevance of the study, we set out explicit inclusion and exclusion criteria. Our inclusion criteria included: (I) Published peer-reviewed articles that focus on the impact of AI chatbots, precisely like ChatGPT, on higher education institutions (HEIs). (II) Articles published in top media news outlets like the Washington Post, Forbes, The Economist, The Wall Street Journal, etc. (III) Studies that provide qualitative and quantitative evidence on using AI chatbots in HEIs. (IV) Articles published in English. (V) Conference proceedings and book chapters. Our exclusion criteria included articles that only tangentially mention AI chatbots or HEIs, without focusing on the intersection of the two. In addition, secondary sources not published in English were also excluded.
2.3. Data extraction and analysis
Data extraction was performed once the final selection of articles was decided based on the inclusion and exclusion criteria. We extracted critical information from each document, such as authors, publication year, study design, the specific chatbot in focus, the context of use in HEIs, primary findings, and conclusions. Data analysis was guided by a narrative synthesis approach due to the various studies involved (O’Donovan et al., 2019). We analyzed the data both descriptively and thematically. The descriptive research focused on the bibliometric characteristics of the studies, including the number of studies, countries of origin, publication years, and the specific AI chatbots under investigation (Peters et al., 2020). The thematic analysis involved categorizing the findings into themes based on familiar patterns, such as specific applications of AI chatbots in HEIs, their benefits, limitations, ethical concerns, and future research directions. This systematic approach ensured that our scoping review was rigorous and adequately captured the state of research on the impact of AI chatbots on higher education institutions.
3. Results
Figure 2 shows the articles initially identified, those excluded based on title and abstract, and those excluded based on full-text review. It also shows the number of papers included in the final analysis and the reasons for exclusion at each stage. In the first segment, “Identification of studies via other methods,” 80 records were identified, including 54 from various websites and 26 from organizations. Of these, 64 papers were sought for retrieval, while the remaining 16 were not retrieved for not satisfying the inclusion criteria. All 51 retrieved records were assessed for eligibility, and 13 were excluded due to the articles’ length restrictions, leaving 10 to be included in the review.
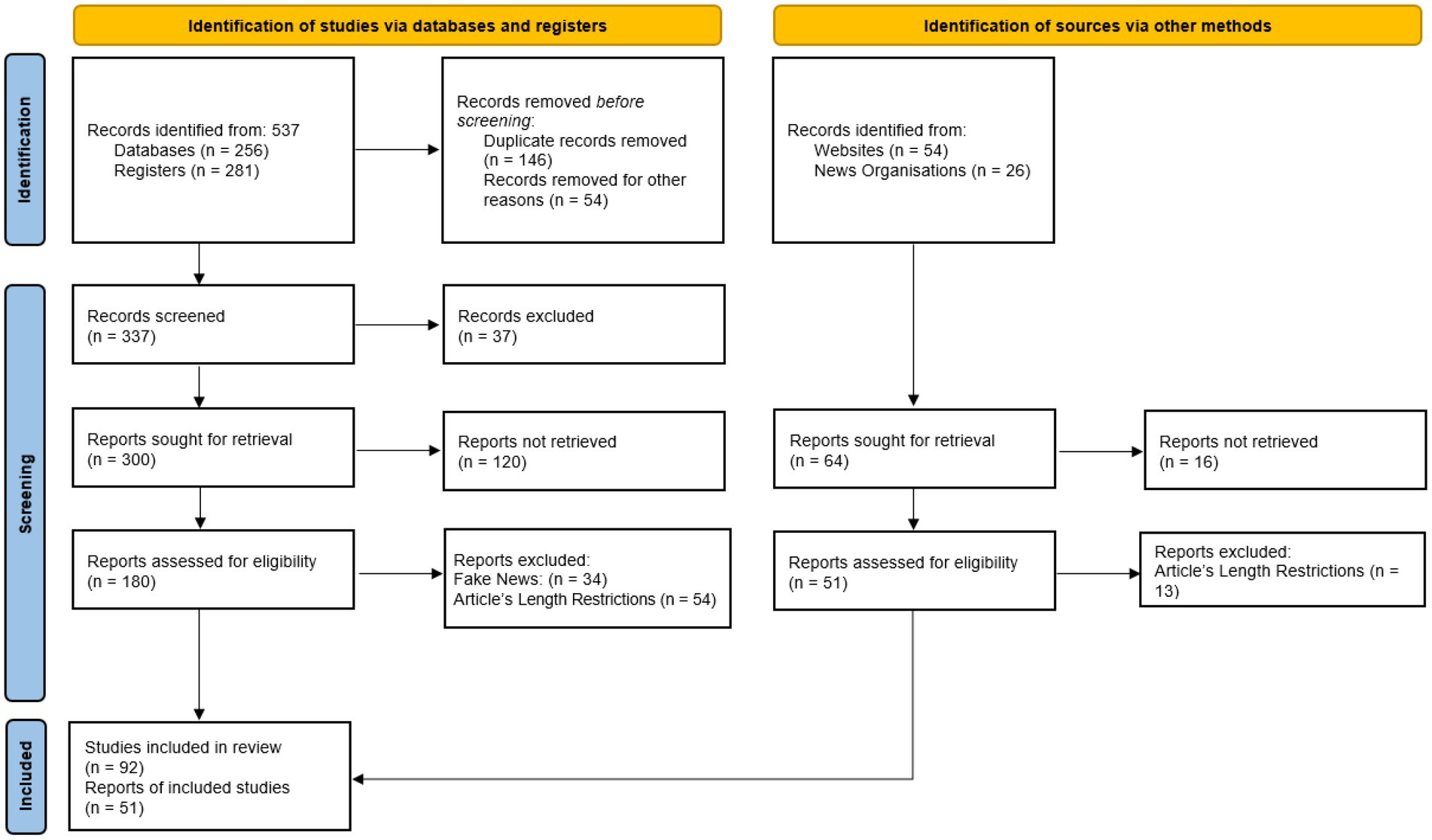
Figure 2. PRISMA flow diagram. Source: Page et al. (2021). For more information, visit: http://www.prisma-statement.org/.
In the second segment, “Identification of studies via databases and registers,” 537 records were initially identified through various databases (256 documents) and registers (281 records). Before the screening, 200 papers were removed: 146 for being duplicates and 54 for other reasons. This screening left 337 records to be screened, of which 37 were excluded for not satisfying the inclusion criteria. Following the initial screening, 300 papers were sought for retrieval, but 120 were not retrieved according to the exclusion criteria. The remaining 180 records were assessed for eligibility, out of which 88 were excluded: 34 for being identified as fake news and 54 due to the article’s length restrictions. This selection resulted in a final 92 articles being included in the review from the databases and registers, in addition to the 54 from other methods, which comprised 143 secondary sources for our analysis.
3.1. Potential approaches and inherent limitations of AI chatbots deployed to replace humans in HEIs
The concern about AI chatbots like ChatGPT replacing human beings to carry out a wide variety of tasks was expressed by The Washington Post - Editorial Board (2022), who warn that the future of AI technology significantly more potent than today’s will result in a price decline of many kinds of labors up to zero. Nevertheless, AI cannot undertake several academic tasks, including creative activities, such as inventing new courses or developing inventive teaching methods, and interpersonal interaction, such as counseling, providing personalized feedback, and resolving student issues. Additional tasks challenging to be substituted by AI comprise complex reasoning and problem-solving like selecting research projects or evaluating the effects of policy decisions, and empathy and understanding, such as coaching and providing emotional assistance (OpenAI, 2022).
Several articles support this view, including Murtarellia et al. (2021), who argue that chatbots lack valuable human traits like empathy, judgment, and discretion. Likewise, Felix (2020) warns that AI should not replace teachers since they can bring to the classroom a unique contribution that no machine can provide: their humanity. He argues that no AI application can provide valuable learning experiences regarding ethical norms and values, existential reflection, or a sense of self, history, and society. Equally, Brito et al. (2019) assert that some scholars believe AI will not supplant professors; however, they warn about the unavoidable reality of an existing AI-based technology that allows teaching-learning interactions without human intervention. This possibility represents a desirable attractive low-cost alternative, particularly for the private players in the education sector.
3.2. Harnessing AI technology to detect and deter academic fraud
The most effective technologies to tackle the challenges posed by AI chatbots like ChatGPT include AI-based plagiarism detection, text similarity detection, and deep learning-based plagiarism detection, as well as online testing platforms such as ProctorU and ExamSoft for remote exams and academic fraud detection. Other technologies include digital examination, predictive analytics, machine learning for cheating detection, blockchain for secure student data, biometric verification for authentication, and digital rights management for IP protection (GPT-2 Output Detector Demo, 2022).
The development of AI-based plagiarism detection tools is supported by factual evidence. Indeed, since the launch of ChatGPT, the list of Internet resources for AI-generated content detection tools and services has been growing weekly (OpenAI, 2023; Originality, 2023; Allen Institute for AI, n.d.; Crossplag, n.d.; Writer, n.d.).
Regarding the online testing platforms mentioned above, Hu (2020) identifies several AI-based applications for this purpose, like ProctorU, Proctorio, and ProctorTrack. He argues that these online testing platforms analyze video recordings to determine suspicious student behavior, including irruptions of people entering the test room and the test taker’s head or eye movements. Walters (2021) outlines issues associated with the widespread utilization of online proctoring software in New Zealand universities. He emphasizes the hardships students from disadvantaged backgrounds face, who may reside with extended family or in crowded housing. He may be disproportionately flagged due to unavoidable ambient noise, conversations, or people entering their exam room. In addition, those with disabilities or neurodiversity may be disadvantaged by AI-based surveillance of their movements and gaze.
The use of predictive analytics to detect academic fraud is also supported by academic research. Indeed, Trezise et al. (2019) confirm that keystroke and clickstream data can distinguish between authentically written pieces and plagiarized essays. Similarly, Norris (2019) explores strategies to thwart academic web fraud, such as predictive analytics systems. He analyzes students’ data from their interactions within their learning environments, including device details, access behavior, locations, academic advancement, etcetera, attempting to foretell students’ behavioral trends and habits to detect questionable or suspicious activities.
Several academic articles also support using ML algorithms to detect cheating by analyzing student data. Some examples include Kamalov et al. (2021), who propose an ML approach to detect instances of student cheating based on recurrent neural networks combined with anomaly detection algorithms and find remarkable accuracy in identifying cases of student cheating. Similarly, Ruipérez-Valiente et al. (2017) employed an ML approach to detect academic fraud by devising an algorithm to tag copied answers from multiple online sources. Their results indicated high detection rates (sensitivity and specificity measures of 0.966 and 0.996, respectively). Equally, Sangalli et al. (2020) achieve a 95% generalization accuracy in classifying instances of academic fraud using a Support Vector Machine algorithm.
The use of blockchain for data tampering prevention is also supported by academic research. Reis-Marques et al. (2021) analyzed 61 articles on blockchain in HEIs, including several addressing educational fraud prevention. Tsai and Wu (2022) propose a blockchain-based grading system that records results and activities, preventing post-grade fraud. Islam et al. (2018) suggest a two-phase timestamp encryption technique for question sharing on a blockchain, reducing the risk of exam paper leaks and maintaining assessment integrity.
The use of biometric verification for cheating prevention is also backed by research. Rodchua et al. (2011) review biometric systems, like fingerprint and facial recognition, to ensure assessment integrity in HEIs. Similarly, Agulla et al. (2008) address the lack of face recognition in learning management systems and propose a FaceTracking application using webcam video. Agarwal et al. (2022) recommend an ML-based keystroke biometric system for detecting academic dishonesty, reporting 98.4% accuracy and a 1.6% false-positive rate.
3.3. Potential risks of implementing AI Chatbots in HEIs
Adopting AI chatbots in HEIs presents various risks, such as privacy breaches, unlawful use, stereotyping, false information, unexpected results, cognitive bias, reduced human interaction, limited accessibility, and unethical data gathering (OpenAI, 2022). Indeed, Baidoo-Anu and Ansah (2023) emphasize certain inherent drawbacks of the chatbot, such as misinformation, augmenting preexisting prejudices through data training, and privacy concerns. Similarly, Akgun and Greenhow (2022) caution against using AI-based algorithms to predict individual actions from chatbot-human interaction information gathered, raising questions regarding fairness and self-freedom. Likewise, Murtarellia et al. (2021) draw attention to the increased information asymmetry from AI chatbots such as ChatGPT, indicating that human conversations with these bots can enable the collection of personal data to build a user profile. AI chatbots can identify patterns that create an informational advantage for the algorithm’s owner. For example, some HEIs may leverage chatbots to sway students’ attitudes toward academic advice to artificially boost enrollment in specific courses to the detriment of others.
In the same way, Miller et al. (2018) cautioned about the potential perils of using social data, including human prejudice to train AI systems, which could lead to prejudicial decision-making processes. Similarly, Akgun and Greenhow (2022) inform the risks of adopting AI-based technologies in academia, including the likely preservation of prevailing systemic bias and discrimination, the perpetuation of unfairness for students from historically deprived and marginalized groups, and magnification of racism, sexism, xenophobia, and other practices of prejudice and injustice. They also advise about the AI-based systems capable of monitoring and tracking students’ thoughts and ideas, which may result in surveillance systems capable of threatening students’ privacy.
Regarding the negative impact of replacing human interaction in the learning process in terms of engagement and learning outcomes, Fryer et al. (2017) study the chatbots’ long-term effects on task and course interest among foreign language students and find that a significant decline in students’ task interest when interacting with a chatbot but not a human partner. Regarding the risk of misinformation, Bushwick and Mukerjee (2022) suggest that AI chatbots should be subject to some form of regulation due to the risks associated with a technology capable of human-like writing and answering to a wide range of topics with advanced levels of fluency and coherence. These risks include spreading misinformation or impersonating individuals. Regarding the unanticipated outcomes of AI chatbots, they are referred to as hallucinations: unpredictable AI outputs caused by data beyond its training set. Additional information and references about this issue are provided later in this article.
The issue of stereotyping has mixed academic evidence. For example, Bastiansen et al. (2022) deployed the Stereotype Content Model to research the effects of warmth and gender of a chatbot on stereotypes, trustworthiness, aid, and capability. They find no divergent outcomes stemming from exposure to heat and assigned gender. Alternatively, Leavy (2018) argues that machine intelligence reflects gender biases in its data. Although attempts have been made to address algorithmic bias, they still need to pay more attention to the role of gender-based language. Women, who are leading this field, are best positioned to identify and solve this issue. Achieving gender parity in ML is crucial to prevent algorithms from perpetuating harmful gender biases against women.
Finally, the issue of accessibility has barely been analyzed in the academic literature. Stanley et al. (2022) identify 17 distinct sources resulting in 157 different suggestions for making a chatbot experience that is accessible, which they grouped into five groups: content, user interface, integration with other web content, developer process & training, and testing.
3.4. Academics activities that may be enhanced by the adoption of AI chatbots in HEIs
Adopting AI chatbots like ChatGPT in HEIs can positively affect various academic activities, including admissions, as they can streamline enrollment with tailored approaches to individual student needs. Student services can also benefit from AI chatbots, as they can provide personalized assistance with financing, scheduling, and guidance. Additionally, AI chatbots can enhance teaching by creating interactive learning experiences to assist students in comprehending course material, providing personal feedback, and aiding researchers in data collection and analysis. Furthermore, AI chatbots can improve student life by furnishing students with personalized support for events and activities, advice on student life, and social interaction. Lastly, AI chatbots can increase student retention by providing customized advice and assistance (OpenAI, 2022).
Regarding the benefits of AI on admissions, Page and Gehlbach (2017) assess the efficiency of a conversational AI system to assist first-year students transitioning to college through personalized text message-based outreach at Georgia State University. Their findings reveal improved success with pre-enrollment requirements and timely enrollment among study participants. Arun et al. (2019) also assess an AI-based CollegeBot’s effectiveness in providing students with university-related information, class schedules, and assessment timetables. Their study substantiates the advantages of chatbots for student services. Likewise, Slepankova (2021) finds that AI chatbot applications enjoying significant student support include delivering course material recap, study material suggestions, and assessment requirements information.
Georgescu (2018) and other academic articles suggest that chatbots can transform education by supporting content delivery and assessment on various topics, including multimedia content and AI-based speeches. Similarly, Essel et al. (2022) studied the adoption’s impact of a virtual teaching assistant in Ghanaian HEIs, finding students who interacted with the chatbot had higher academic performance than those who interacted with the course instructor. Wang et al. (2017) investigated the impact of chatbots in immersive virtual English learning environments, discovering this tech enhances students’ perception of such settings. Kerly and Bull (2006) studied chatbots’ benefits in developing university students’ negotiation skills. Tegos et al. (2015) analyzed the effects of chatbots in collaborative learning experiences among college students, finding that tech increases various knowledge acquisition measures. Lastly, Shorey et al. (2019) examined the benefits of using a chatbot as a virtual patient to develop nursing students’ communication skills, finding this technology improves students’ perceived self-efficacy and trust in their abilities.
The present article constitutes an excellent first example regarding the benefits of chatbots in research. However, additional examples would include studies analyzing the influence of AI chatbots among university students experiencing symptoms of depression and anxiety (Fitzpatrick et al., 2017; Fulmer et al., 2018; Klos et al., 2021). Similarly, Bendig et al. (2019) develop a comprehensive literature review on using chatbots in clinical psychology and psychotherapy research, including studies employing chatbots to foster mental health. Likewise, Dwivedi et al. (2023) discuss the impact of ChatGPT on academic research, noting its potential to improve the quality of writing and make research more accessible to non-experts while also posing challenges such as the authenticity and reliability of generated text and accountability and authorship issues.
Additionally, several articles report using AI chatbots for gathering qualitative information for research purposes. Some examples include Xiao et al. (2020), who create a prototype to generate two chatbots – one with active listening skills and one without – and evaluate both chatbots using 206 participants to compare their performance and conclude that their study provides practical methods for building interview chatbots effectively. Similarly, Nunamaker et al. (2011) suggest gathering human physiology and behavior information during interactions with chatbot-like technology. Pickard et al. (2017) compare the qualitative data collected from automated virtual interviewers, called embodied conversational agents, versus the information obtained by human interviewers. Tallyn et al. (2018) use a chatbot to gather ethnographic data for analysis. Xiao et al. (2020) assess the effectiveness of the limitations of chatbots in conducting surveys. Finally, Kim et al. (2019) find that chatbot-based surveys can produce higher-quality data than web-based surveys.
Concerning the use of AI chatbots to retain students, earlier articles highlight the advantages these chatbots offer, potentially improving student retention. Indeed, Lee et al. (2022) investigate a computer-generated conversational agent-aided evaluation system and realize that it advances student-achievement results, including scholarly accomplishment, assurance, learning mentality, and enthusiasm. They infer that chatbots can heighten learner participation in the educational process. Other articles analyze the benefits of chatbots to provide students with standardized academic information, like course content (Cunningham-Nelson et al., 2019), practice exercises and questions (Sinha et al., 2020), frequently asked college questions (Ranoliya et al., 2017; Clarizia et al., 2018), assessment criteria (Benotti et al., 2018; Durall and Kapros, 2020), assignment calendars (Ismail and Ade-Ibijola, 2019), etcetera.
Previous research works encompass the analysis of chatbots dedicated to informing about campus physical locations (Mabunda and Ade-Ibijola, 2019), teaching computer programming concepts (Pham et al., 2018; Zhao et al., 2020), providing academic and administrative services (Hien et al., 2018), etcetera. Equally, Sandu and Gide (2022) study the benefits of chatbots in the Indian educational sector and find that this technology can improve communication, learning, productivity, and teaching assistance effectiveness and minimize interaction ambiguity. Lastly, AlDhaen (2022) suggests that implementing AI in the academic world will improve educational and non-academic operations governance.
3.5. Impact of AI chatbots on the digital literacy of students and their AI-caused anxiety
The implications of the launch of ChatGPT refer primarily to proactive approaches to face the academic integrity challenges posed by AI chatbots like ChatGPT. Meckler and Verma (2022) suggest requiring students to write by hand during class sessions to ensure successful monitoring efforts. Alternatively, Shrivastava (2022) emphasizes the relevance of teaching digital literacy early on to allow students critically assess the source of the information they receive.
Digital literacy should also teach students the risks of relying on AI-based technologies. These risks include hallucinations: AI-generated responses not explained by training data. Several authors have studied AI-generated hallucinations. Indeed, Cao et al. (2017) find that 30% of the outputs generated by state-of-the-art neural summarization applications suffer from hallucination problems. Similarly, Falke et al. (2019) study the most recent technologically advanced summarization systems and find that they produce about 25% of hallucination errors in their summaries. Likewise, Maynez et al. (2020) see 70% + of single-sentence summaries show intrinsic/extrinsic hallucinations in AI-based systems (Recurrent, Convolutional, and Transformers).
Shuster et al. (2021) also studied neural retrieval in loop architectures. They found they enabled open-domain conversational capabilities, including generalizing scenarios not seen in training and reducing knowledge hallucination in advanced chatbots. Equally, Bang et al. (2023) find that ChatGPT has 63.41% accuracy on average in 10 different reasoning categories under logical reasoning, non-textual reasoning, and commonsense reasoning, which makes it an unreliable reasoner. The authors also report that ChatGPT suffers from hallucination problems.
Finally, digital literacy training must cover the risk of plagiarism when using AI chatbots. Ghosal (2023) notes ChatGPT’s downside of lacking plagiarism verification as it picks sentences from training data. King and chatGPT (2023) discuss AI and chatbots’ history and potential misuse, particularly in higher education, where plagiarism is a growing concern. Professors can minimize cheating via ChatGPT using various assessment methods and plagiarism detection software (GPT-2 Output Detector Demo, 2022; OpenAI, 2023; Originality, 2023; Allen Institute for AI, n.d.; Crossplag, n.d.; Writer, n.d.).
Several public and private organizations have been alarmed by the launch of ChatGPT. Lukpat (2023) reveals that New York City schools blocked access to ChatGPT on its networks and devices due to fears that students could use the AI app to answer questions, do schoolwork, or write essays. Soper (2023) details that Seattle Public Schools is also prohibiting ChatGPT. Cassidy (2023) reports that Australian universities have had to adjust their approach to testing and grading due to fears of students using AI to write essays. They have set new rules stating that using AI is considered cheating. McCallum (2023) reports that Italy initially banned OpenAI’s ChatGPT due to privacy issues by arguing that there is no legal reason to gather and store private data for training algorithms. OpenAI’s lack of transparency about its architecture, model, hardware, computing, training, and dataset construction has caused further concern (Brodkin, 2023). Ryan-Mosley (2023) informs the European Parliament’s endorsement of the preliminary guidelines of the EU AI Act, which estipulate barring the use of AI emotion-detection in specified areas, a possible banning real-time biometrics and predictive policing in public spaces, outlawing public agencies’ social scoring, prohibiting copyrighted content in LLMs’ training datasets, etcetera.
Gaceta (2023) reports Paris’ Institute of Political Science banned students from using ChatGPT to prevent academic fraud. Academic journals updated policies to prohibit ChatGPT as an author (Thorp, 2023). Dwivedi et al. (2023) recommend forbidding ChatGPT or equivalent software from producing intellectual outputs. However, in the non-academic world, Bensinger (2023) reports over 200 ChatGPT-authored e-books on Amazon. Libert (2023) reveals that Study.com questioned both teachers and learners, discovering that 72% of instructors were worried about the repercussions of ChatGPT on plagiarism, yet only 34% felt it should be banned. They uncovered that 89% of pupils employed ChatGPT for homework aid, 48% for an assessment/quiz, 53% for composition, and 22% for a paper structure. The nature of ChatGPT and the need for author disclosure make determining the number of AI-generated e-books challenging.
AI chatbots pose security concerns, with potential risks including disinformation and cyberattacks. OpenAI’s CEO, Sam Altman, is aware of the dangers but optimistic about the technology’s benefits (Ordonez et al., 2023). Check Point (2023) reports underground hacking communities using OpenAI to design malicious tools, and skilled threat actors will likely follow. Perry et al. (2022) conducted a large-scale study on using an AI code assistant for security tasks and found that participants with AI access produced less reliable code.
ChatGPT is a powerful tool for revolutionizing the academic world, and fear of overthrowing the existing order has traditionally resulted in repressive, oppressive, and other drab strategies utilized by those who dread forfeiting the positions that the current system grants them. As has been the case with numerous other scientific and technological advances that have been banned throughout human history (including Darwin’s theory of evolution, Copernicus’s heliocentric model, specific immunizations, blood transfusions, etc.), likely, ChatGPT and similar AI-powered applications may soon suffer a similar fate.
3.6. Societal and economic implications from the wide-scale adoption of AI chatbots
Individuals are apprehensive of AI owing to its capacity to disrupt many industries and result in job loss. Furthermore, many are concerned that AI could become so advanced that it would take over human control and make decisions for us. The notion of machines and robots replacing humans in the workplace can be disconcerting. Moreover, some fear that a powerful AI could become so potent that it would endanger humanity (OpenAI, 2022).
The reasons for humans to fear the development of AI chatbots like ChatGPT are many and compelling, although it is too early to support such fears with solid statistical evidence. Therefore, when writing this article, only partial and anecdotal evidence can be presented. Indeed, according to a report by researchers at Stanford University (AI Index Steering Committee, 2023), 36% of experts believe that decisions made by AI could lead to “nuclear-level catastrophes” (AI Index Steering Committee, 2023, p. 337). While the majority of researchers surveyed believe AI could lead to a “revolutionary change in society” (AI Index Steering Committee, 2023, p. 337), they also warned of the potential dangers posed by technology development.
Some of the human fears of AI are derived from its capacity to replicate academic achievements that would require years of investment in time, money, and effort, in just a few seconds. Indeed, ChatGPT3.5 and ChatGPT4 both excelled on standardized exams such as the Uniform Bar Exam, GREs, SATs, USABO Semifinal Exam 2020, Leetcode coding challenges, and AP exams. ChatGPT4 outperformed ChatGPT3.5 in all difficulty levels of the Leetcode coding challenges and subjects like Biology, Calculus BC, Chemistry, Art History, English, Macroeconomics, Microeconomics, Physics, etcetera in AP exams. Additionally, ChatGPT4 performed better than ChatGPT3.5 on Medical Knowledge Self-Assessment Program Exam, USABO Semifinal Exam 202, USNCO Local Section Exam 2022, Sommelier exams, etcetera (OpenAI, 2023). Likewise, the OpenAI (OpenAI, 2023) GPT-4 Technical Report reveals that GPT-4 demonstrates proficiency comparable to humans on multiple tests, such as a fabricated bar exam that it obtained a rank in the uppermost tenth percentile of test participants. GPT-4 outperforms existing large language models (LLMs) on a collection of NLP tasks and exceeds most reported state-of-the-art systems.
AI replacing human jobs is a mortal fear. Goldman Sachs (Hatzius et al., 2023) predicts that ChatGPT and other generative AI could eliminate 300 million jobs worldwide. Researchers estimate that AI could replace 7% of US employment, complement 63%, and leave 30% unaffected. AI’s global adoption may boost GDP by 7% (Hatzius et al., 2023). Taulli (2019) suggests that automation technology will take over “repetitive processes” in fields like programming and debugging. Positions requiring emotional intelligence, empathy, problem-solving, critical decision-making, and adaptabilities, like social workers, medical professionals, and marketing strategists, are difficult for AI to replicate.
In the same way, Felten et al. (2023) assess the impact of AI language modeling advances on occupations, industries, and geographies and find that telemarketers and post-secondary teachers of English, foreign languages, and history are most exposed to language modeling. Additionally, legal services, securities, commodities, and investments are most exposed to language modeling advances. Similarly, Tate (2021) cautions that AI’s rapid evolution could eradicate the “laptop class” of employees in the upcoming decade. At the same time, blue-collar vocations necessitating hands-on expertise and manual labor will remain safe. She further cautions of AI potentially supplanting white-collar jobs in law, finance, media, and healthcare. She advises those seeking job stability to pursue blue-collar roles instead of STEM fields and “knowledge economy” positions that will be obsolete in the imminent transformation.
Equally, Bubeck et al. (2023) argue that the emergence of GPT-4 and other large LLMs will challenge traditional notions of human expertise in various professional and scholarly fields. They suggest that the capabilities of GPT-4 may raise concerns about the potential for AI to displace or reduce the status of human workers in highly skilled professions. The rise of LLMs could also widen the “AI divide” between those with access to the most powerful AI systems and those without, potentially amplifying existing societal divides and inequalities.
Accordingly, GESTION (2023) reveals that, as established by a survey of 1,000 US business magnates by ResumeBuilder.com, virtually half of the businesses have already adopted ChatGPT, and a further 30% are looking to do so. It is supposed that 48% of ChatGPT customers have supplanted personnel and saved over $75,000. Notwithstanding this, the dominant part of business magnates is content with the standard of ChatGPT’s work, with 55% rating it ‘excellent’ and 34% rating it ‘very good.’ ChatGPT is employed for code writing (66%), content production (58%), customer service (57%), and document/meeting summaries (52%). It is also used to write job descriptions (77%), craft job interview applications (66%), and reply to job seekers (65%).
Similarly, Eloundou et al. (2023) evaluate the impact of GPTs and other LLMs on 19,262 tasks and 2,087 job processes from the O*NET 27.2 database. The study showed 80% of the US workforce may have 10% of their functions affected, and 19% may see 50% or more impacted. 15% of US worker tasks could be faster with LLMs, increasing to 47–56% with LLM-powered software. Science/critical thinking jobs are less affected, while programming/writing skills are more vulnerable. Higher-wage occupations have more LLM exposure, with no correlation to employment levels. Those with some college education but no degree have high LLM exposure. Findings suggest potential economic, social, and policy implications, requiring preparedness for disruption.
HEIs can use knowledge of AI’s impact on the job market to adjust their curriculum, prioritizing skills AI cannot replicate, such as problem-solving and critical decision-making. Additionally, institutions can teach students to use and develop AI to their advantage, preparing them for the changing job market and ensuring their success in the workplace.
4. Discussion
4.1. Implications
The hope that the AI development race will pause, as suggested by tech leaders and researchers, including Elon Musk, for 6 months or more is naive. Oxford Insights’ 2022 Government AI Readiness Index (Insights, 2022) ranks 160 countries on AI readiness for public services, with 30% having released a national AI strategy and 9% developing one. This finding highlights the importance of AI to leaders worldwide. Figure 3 shows the top 20 nations in the index.
Pausing in the AI development race will leave countries behind, and developed economies cannot afford to pay such a price. However, the emergence of ChatGPT and similar technologies may require regulatory frameworks to address privacy, security, and bias concerns, ensuring accountability and fairness in AI-based services. Rules must not impede AI-based tech development, as uncertainty can threaten investments. The US commerce department is creating accountability measures for AI tools (Bhuiyan, 2023), soliciting public feedback on assessing performance, safety, effectiveness, and bias, preventing misinformation, and ensuring privacy while fostering trustworthy AI systems.
Private HEIs will likely lead the AI revolution, driven by cost-saving, productivity, student satisfaction, and reputation. ChatGPT can revolutionize education, enterprises, and linguistics, offering 24/7 access to virtual mentors with internationally recognized wisdom, fostering inventiveness, and providing discernment into consumer conduct (Dwivedi et al., 2023). Personalized learning experiences, immediate feedback, and language support are also possible with ChatGPT. Dwivedi et al. (2023) urge embracing digital transformation in academia and using ChatGPT to stimulate discussions about fundamental principles.
Faculty should proactively embrace AI chatbots such as ChatGPT as powerful teaching, research, and service tool. By becoming informed and trained on AI, they can learn its capabilities and limitations, identify assessment strategies to reduce academic fraud and create innovative pedagogical solutions for future developments. In an AI-driven world, traditional learning will soon become obsolete. Instead, students will query AI for answers to their problems, from cooking to coding. Even better, they could use AI tools to refine existing solutions and exercise their imagination to create new solutions to future challenges with endless possibilities.
AI-based learning experiences must recognize that AI technologies are trained using existing data and are ill-equipped to tackle novel problems without training data. For instance, AI might face challenges in dealing with the unprecedented obstacles humans may encounter during space exploration. Learning experiences must teach them to analyze cases with limited historical data to train available AIs. This example is one of the many approaches for adopting AI in the current academic world, which must shift rapidly to survive.
Another significant factor when adopting AI technologies into the existing learnings experiences at HEIs is exploiting the deficiencies of current and future AI-based technologies in terms of hallucinations, bias in training data leading to biased output, AI-generated ethical dilemmas, novelty security and privacy concerns, poor generalizability of AI models, lack of accounting for human context and understanding, etcetera. All these examples constitute opportunities to include AI-assisted curricula where the notion of AI-assisted student cheating becomes unappealing.
4.2. Limitations
Our study has several limitations. Firstly, the study is based on a scoping review of existing literature, which may not provide a complete or up-to-date picture of the effects of AI-based tools in the education sector. Moreover, the research relies on anecdotal evidence and partial data, limiting the findings’ generalizability. Additionally, the study does not investigate the implementation challenges and practical implications of integrating AI chatbots into the HEIs’ systems. Furthermore, the research does not consider the social and ethical implications of AI’s increasing role in education, such as the impact on human connection and interpersonal skills development. Finally, the study does not provide concrete recommendations or guidelines for HEIs to integrate AI technologies into their teaching, research, and student services.
5. Conclusion
The primary contribution of this article is the development of qualitative research on the impact of AI chatbots like ChatGPT on HEIs by employing a scoping review of the current literature. Developing AI-based tools such as ChatGPT increases the likelihood of replacing human-based teaching experiences with low-cost chatbot-based interactions. This possibility may result in biased teaching and learning experiences with reduced human connection and support. We also provide secondary source evidence that adopting AI-based technologies like ChatGPT can provide many benefits to HEIs, including increased effectiveness on student services, admissions, retention, etcetera, and significant enhancements to teaching and research activities. We also verify that the risks involved in adopting this technology in the education sector are substantial, including sensitive issues such as privacy and accessibility concerns, unethical use, data collection, misinformation, technology overreliance, cognitive bias, replacement of human interaction, etc.
Data availability statement
The original contributions presented in the study are included in the article/Supplementary material, further inquiries can be directed to the corresponding author.
Author contributions
All authors listed have made a substantial, direct, and intellectual contribution to the work and approved it for publication.
Funding
The authors of this academic research article declare that there were no funding sources for this research, nor any competing interests.
Conflict of interest
The authors declare that the research was conducted in the absence of any commercial or financial relationships that could be construed as a potential conflict of interest.
Publisher’s note
All claims expressed in this article are solely those of the authors and do not necessarily represent those of their affiliated organizations, or those of the publisher, the editors and the reviewers. Any product that may be evaluated in this article, or claim that may be made by its manufacturer, is not guaranteed or endorsed by the publisher.
Supplementary material
The Supplementary material for this article can be found online at: https://www.frontiersin.org/articles/10.3389/feduc.2023.1206936/full#supplementary-material
References
Agarwal, N., Danielsen, N., Gravdal, P., and Bours, P. (2022). Contract cheat detection using biometric keystroke dynamics. 20th international conference on emerging eLearning technologies and applications (ICETA). IEEE Xplore, 15–21
Agulla, E., Rifón, L., Castro, J., and Mateo, C. (2008). Is my student on the other side? Applying biometric web authentication to E-learning environments. Eighth IEEE International Conference on Advanced Learning Technologies, IEEE Xplore, 551–553
AI Index Steering Committee (2023). Artificial intelligence index report 2023. Stanford University, Stanford Institute for Human-Centered Artificial Intelligence (HAI). Retrieved from https://aiindex.stanford.edu/wp-content/uploads/2023/04/HAI_AI-Index-Report_2023.pdf
Akgun, S., and Greenhow, C. (2022). Artificial intelligence in education: addressing ethical challenges in K-12 settings. AI Ethics 2, 431–440. doi: 10.1007/s43681-021-00096-7
AlDhaen, F. (2022) in The use of artificial intelligence in higher education – systematic review. ed. M. Alaali (Cham: COVID-19 Challenges to University Information Technology Governance, Springer)
Alerskans, E., Nyborg, J., Birk, M., and Kaas, E. (2022). A transformer neural network for predicting near-surface temperature. Meteorol. App. 29, 1–9. doi: 10.1002/met.2098
Allen Institute for AI. (n.d.). Grover - a state-of-the-art defense against neural fake news. Available at: https://grover.allenai.org/detect (Accessed April 8, 2023).
Alrawi, M. (2023). Davos 2023: AI chatbot to ‘change education forever within six months’. The National News, January 17, 2023. Available at: https://www.thenationalnews.com/business/2023/01/17/davos-2023-ai-chatbot-to-change-education-forever-within-six-months/ (Accessed January 22, 2023).
Altman, S. (2022). ChatGPT launched on Wednesday. Today it crossed 1 million users! [tweet]. Twitter. Available at: https://twitter.com/sama/status/1599668808285028353 (Accessed January 1, 2023).
Arun, K., Nagesh, S., and Ganga, P. (2019). A multi-model and Ai-based Collegebot management system (Aicms) for professional engineering colleges. Int. J. Innov. Technol. Explor. Eng. 8, 2910–2914. doi: 10.35940/ijitee.I8818.078919
Baidoo-Anu, D., and Ansah, L. (2023). Education in the era of generative artificial intelligence (AI): understanding the potential benefits of ChatGPT in promoting teaching and learning. SSRN Preprint (January 25, 2023). doi: 10.2139/ssrn.4337484
Bang, Y., Cahyawijaya, S., Lee, N., Dai, W., Su, D., and Fung, P. (2023). A multitask, multilingual, multimodal evaluation of ChatGPT on reasoning, hallucination, and interactivity. arXiv preprint arXiv:2302.04023. doi: 10.48550/arXiv.2302.04023
Bastiansen, M., Kroon, A., and Araujo, T. (2022). Female chatbots are helpful, male chatbots are competent? Publizistik 67, 601–623. doi: 10.1007/s11616-022-00762-8
Bellapu, A. (2021). Why transformer model for natural language processing? Analytics Insight. Available at: https://www.analyticsinsight.net/why-transformer-model-for-natural-language-processing/ (Accessed January 1, 2023).
Bendig, E., Erb, B., Schulze-Thuesing, L., and Baumeister, H. (2019). The next generation: Chatbots in clinical psychology and psychotherapy to foster mental health–a scoping review. Verhaltenstherapie 29, 266–280. doi: 10.1159/000499492
Bengio, Y., Russell, S., and Musk, E., (2020). Pause giant AI experiments: AN OPEN LETTER. Available at: https://futureoflife.org/open-letter/pause-giant-ai-experiments/ (Accessed April 1, 2023).
Benotti, L., Martnez, M., and Schapachnik, F. (2018). A tool for introducing computer science with automatic formative assessment. IEEE Trans. Learn. Technol. 11, 179–192. doi: 10.1109/TLT.2017.2682084
Bensinger, G. (2023). ChatGPT launches boom in AI-written e-books on Amazon. Reuter. Available at: https://www.reuters.com/technology/chatgpt-launches-boom-ai-written-e-books-amazon-2023-02-21/ (Accessed March 4, 2023).
Bhuiyan, J. (2023). ‘We have to move fast’: US looks to establish rules for artificial intelligence. The Guardian. Available at: https://www.theguardian.com/technology/2023/apr/11/us-artificial-intelligence-rules-accountability-measures (Accessed April 13, 2023).
Biswas, S. S. (2023a). Potential use of chat GPT in global warming. Ann. Biomed. Eng. 51, 1126–1127. doi: 10.1007/s10439-023-03171-8
Biswas, S. S. (2023b). Role of chat GPT in public health. Ann. Biomed. Eng. 51, 868–869. doi: 10.1007/s10439-023-03172-7
Brito, C., Ciampi, M., Sluss, J., and Santos, H. (2019). Trends in engineering education: a disruptive view for not so far future. 2019 18th international conference on information technology based higher education and training (ITHET), 1–5
Brodkin, J, (2023). GPT-4 poses too many risks, and releases should be halted, AI group tells FTC. Available at: https://arstechnica.com/tech-policy/2023/03/ftc-should-investigate-openai-and-halt-gpt-4-releases-ai-research-group-says/ (Accessed April 1, 2023).
Bubeck, S., Chandrasekaran, V., Eldan, R., Gehrke, J., Horvitz, E., and Zhang, Y. (2023). Sparks of artificial general intelligence: early experiments with GPT-4. arXiv preprint arXiv:2303.12712. doi: 10.48550/arXiv.2303.12712
Bushwick, S., and Mukerjee, M. (2022). ChatGPT explains why AIs like ChatGPT should be regulated. Available at: https://www.scientificamerican.com/article/chatgpt-explains-why-ais-like-chatgpt-should-be-regulated/ (Accessed January 1, 2023).
Cao, Z., Wei, F., Li, W., and Li, S. (2017). Faithful to the original: fact aware neural abstractive summarization. arXiv preprint arXiv:1711.04434v1 [cs.IR]. doi: 10.48550/arXiv.1711.04434
Cassidy, C. (2023). Australian universities to return to ‘pen and paper’ exams after students caught using AI to write essays. Available at: https://www.theguardian.com/australia-news/2023/jan/10/universities-to-return-to-pen-and-paper-exams-after-students-caught-using-ai-to-write-essays (Accessed January 18, 2023).
Castangia, M., Medina, L., Aliberti, A., Rossi, C., Macii, A., Macii, E., et al. (2023). Transformer neural networks for interpretable flood forecasting. Environ. Model. Softw. 160:105581. doi: 10.1016/j.envsoft.2022.105581
Chance, C. (2022). Has there been a second AI big Bang? Forbes. Available at: https://www.forbes.com/sites/calumchace/2022/10/18/has-there-been-a-second-ai-big-bang/?sh=7f7860fb6f74 (Accessed January 1, 2023).
Chavez, H., Chavez-Arias, B., Contreras-Rosas, S., Alvarez-Rodríguez, J. M., and Raymundo, C. (2023). Artificial neural network model to predict student performance using non-personal information. Front Educ. 8:1106679. doi: 10.3389/feduc.2023.1106679
Chen, S. (2023). ChatGPT-5 rumored to launch at the end of 2023, will it achieve AGI? [blog post]. Available at: https://www.gizmochina.com/2021/04/01/chatgpt-5-rumored-to-launch-at-the-end-of-2023-will-it-achieve-agi/ (Accessed April 2, 2023).
Clarizia, F., Colace, F., Lombardi, M., Pascale, F., and Santaniello, D. (2018). Chatbot: an education support system for students. In: A. Castiglione, F. Pop, M. Ficco, and F. Palmieri (Eds) Cyberspace safety and security, 11161. Springer: Cham
ColdFusion. (2022). It’s time to pay attention to A.I. (ChatGPT and beyond) [video]. YouTube. Available at: Retrieved from https://www.youtube.com/watch?v=0uQqMxXoNVs (Accessed January 1, 2023).
Crossplag (n.d.). AI content detector. Available at: https://crossplag.com/ai-content-detector/ (Accessed April 8, 2023).
Cunningham-Nelson, S., Boles, W., Trouton, L., and Margerison, E. (2019). A review of Chatbots in education: practical steps forward. Proceedings of the AAEE2019 conference Brisbane, Australia. Available at: https://aaee.net.au/wp-content/uploads/2020/07/AAEE2019_Annual_Conference_paper_184.pdf
Dastin, J., Hu, K., and Dave, P. (2022). Exclusive: ChatGPT owner OpenAI projects $1 billion in revenue by 2024. Reuters. Retrieved from (https://www.reuters.com/business/chatgpt-owner-openai-projects-1-billion-revenue-by-2024-sources-2022-12-15/).
Durall, E., and Kapros, E. (2020). Co-design for a competency self-assessment Chatbot and survey in science education. In: P. Zaphiris and A. Ioannou (Eds) Learning and collaboration technologies. Human and technology ecosystems, Springer: Cham, 12206
Dwivedi, Y. K., Kshetri, N., Hughes, L., Slade, E. L., Jeyaraj, A., Kar, A. K., et al. (2023). “So what if ChatGPT wrote it?” multidisciplinary perspectives on opportunities, challenges, and implications of generative conversational AI for research, practice, and policy. Int. J. Inf. Manag. 71:102642. doi: 10.1016/j.ijinfomgt.2023.102642
Elecrow. (2023). Introducing ChatGPT4: the next generation of language models. Elecrow. Available at: https://www.elecrow.com/blog/introducing-chatgpt4-the-next-generation%C2%A0of%C2%A0language-models.html (Accessed March 3, 2023).
Eloundou, T., Manning, S., Mishkin, P., and Rock, D. (2023). GPTs are GPTs: an early look at the labor market impact potential of large language models. Available at: https://arxiv.org/abs/2003.10130v4 (Accessed April 1, 2023).
Essel, H., Vlachopoulos, D., Tachie-Menson, A., Johnson, E., and Baah, P. (2022). The impact of a virtual teaching assistant (chatbot) on students’ learning in Ghanaian higher education. Int. J. Educ. Technol. High. Educ. 19, 1–19. doi: 10.1186/s41239-022-00362-6
Falke, T., Ribeiro, L., Utama, P., Dagan, I., and Gurevych, I. (2019). Ranking generated summaries by correctness: an interesting but challenging application for natural language inference. In Proceedings of the 57th annual meeting of the Association for Computational Linguistics, 2214–2220
Felix, C. (2020). “The role of the teacher and AI in education” in International perspectives on the role of Technology in Humanizing Higher Education Innovations in higher education teaching and learning. eds. E. Sengupta, P. Blessinger, and M. S. Makhanya, vol. 33 (Bingley: Emerald Publishing Limited), 33–48.
Felten, E., Raj, M., and Seamans, R. (2023). How will language modelers like ChatGPT affect occupations and industries? arXiv preprint arXiv:2303.01157v2. doi: 10.48550/arXiv.2303.01157
Fitzpatrick, K., Darcy, A., and Vierhile, M. (2017). Delivering cognitive behavior therapy to young adults with symptoms of depression and anxiety using a fully automated conversational agent (Woebot): a randomized controlled trial. JMIR Ment. Health 4:e19. doi: 10.2196/mental.7785
Fryer, L., Ainley, M., Thompson, A., Gibson, A., and Sherlock, Z. (2017). Stimulating and sustaining interest in a language course: an experimental comparison of chatbot and human task partners. Comput. Human Behav. 75, 461–468. doi: 10.1016/j.chb.2017.05.045
Fulmer, R., Joerin, A., Gentile, B., Lakerink, L., and Rauws, M. (2018). Using psychological artificial intelligence (Tess) to relieve symptoms of depression and anxiety: a randomized controlled trial. JMIR Ment. Health 5:e64. doi: 10.2196/mental.9782
Gaceta, La (2023). Prohíben usar la ChatGPT en varias universidades. Available at: https://www.lagaceta.com.ar/nota/978320/sociedad/prohiben-usar-chatgpt-varias-universidades.html (Accessed February 5, 2023).
Gao, C., Howard, F., Markov, N., Dyer, E., Ramesh, S., Luo, Y., et al. (2022). Comparing scientific abstracts generated by ChatGPT to original abstracts using an artificial intelligence output detector, plagiarism detector, and blinded human reviewers. bioRxiv preprint 2022.12.23.521610. doi: 10.1101/2022.12.23.521610
Georgescu, A. (2018). Chatbots for education - trends, benefits, and challenges. Int. Sci. Conf. eLearn. Softw. Educ. 2, 195–200. doi: 10.12753/2066-026X-18-097
GESTION (2023). Los primeros trabajos perdidos en Estados Unidos por la Inteligencia Artificial ChatGPT. Available at: https://gestion.pe/economia/management-empleo/chatgpt-los-primeros-trabajos-perdidos-en-estados-unidos-por-la-implementacion-de-la-inteligencia-artificial-nnda-nnlt-noticia/ (Accessed March 3, 2023).
Ghosal, S. (2023). ChatGPT on characteristic mode analysis. TechRxiv Preprint. 70, 1008–1019. doi: 10.36227/techrxiv.21900342.v1
Goldman, S. (2022). OpenAI CEO admits ChatGPT risks. What now? | the AI beat. VentureBeat. Available at: https://venturebeat.com/ai/openai-ceo-admits-chatgpt-risks-what-now-the-ai-beat/ (Accessed January 1, 2023).
GPT, Chat (2022). In Wikipedia. Available at: https://en.wikipedia.org/wiki/ChatGPT (Accessed January 1, 2023).
GPT-2 Output Detector Demo (2022). Hugging Face, Inc. Available at: https://huggingface.co/openai-detector/ (Accessed January 3, 2023).
Hatzius, J., Briggs, J., Kodnani, D., and Pierdomenico, G. (2023). The potentially large effects of artificial intelligence on economic growth. Available at: https://www.key4biz.it/wp-content/uploads/2023/03/Global-Economics-Analyst_-The-Potentially-Large-Effects-of-Artificial-Intelligence-on-Economic-Growth-Briggs_Kodnani.pdf (Accessed April 1, 2023).
Hien, H., Cuong, P.-N., Nam, L., Nhung, H., and Thang, L. (2018). Intelligent assistants in higher-education environments: the fit-ebot, a chatbot for administrative and learning support. Proceedings of the 9th international symposium on info and communication technology, 69–76
Hu, J. (2020). Online test proctoring claims to prevent cheating. But at what cost? SLATE. Available at: https://slate.com/technology/2020/10/online-proctoring-proctoru-proctorio-cheating-research.html (Accessed January 3, 2023).
Hu, K. (2023). ChatGPT sets record for fastest-growing user base - analyst note. Reuters. Available at: https://www.reuters.com/technology/chatgpt-sets-record-fastest-growing-user-base-analyst-note-2023-02-01/ (Accessed February 5, 2023).
Insights, Oxford. (2022). Government AI readiness index 2022. Available at: https://static1.squarespace.com/static/58b2e92c1e5b6c828058484e/t/639b495cc6b59c620c3ecde5/1671121299433/Government_AI_Readiness_2022_FV.pdf
Islam, A., Kader, F., and Shin, S. (2018). BSSSQS: a Blockchain-based smart and secured scheme for question sharing in the Smart education system. arXiv preprint arXiv:1812.03917v1 [cs.CR]. doi: 10.48550/arXiv.1812.03917
Ismail, M., and Ade-Ibijola, A. (2019). Lecturer’s apprentice: a chatbot for assisting novice programmers. IEEE Xplore. 2019 international multidisciplinary information technology and engineering conference (IMITEC), 1–8
Jeblick, K., Schachtner, B., Dexl, J., Mittermeier, A., Stüber, A., Topalis, J., et al. (2022). ChatGPT makes medicine easy to swallow: an exploratory case study on simplified radiology reports. arXiv preprint arXiv:2212.14882v1 [cs.CL]. doi: 10.48550/arXiv.2212.14882
Kamalov, F., Sulieman, H., and Calonge, D. (2021). Machine learning-based approach to exam cheating detection. PLoS One 16:e0254340. doi: 10.1371/journal.pone.0254340
Kasepalu, R., Prieto, L. P., Ley, T., and Chejara, P. (2022). Teacher artificial intelligence-supported pedagogical actions in collaborative learning coregulation: a wizard-of-Oz study. Front Educ. 7, 1–15. doi: 10.3389/feduc.2022.736194
Kerly, A., and Bull, S. (2006). The potential for chatbots in negotiated learner modeling: a wizard-of-oz study. Lect. Notes Comput. Sci 4053, 443–452. doi: 10.1007/11774303_44
Kim, S., Lee, J., and Gweon, G. (2019). “Comparing data from Chatbot and web surveys” in Proceedings of the 2019 CHI conference on human factors in computing systems - CHI, vol. 19, 1–12.
King, M. R., chatGPT (2023). A conversation on artificial intelligence, Chatbots, and plagiarism in higher education. Cell. Mol. Bioeng. 16, 1–2. doi: 10.1007/s12195-022-00754-8
Klos, M., Escoredo, M., Joerin, A., Lemos, V., Rauws, M., and Bunge, E. (2021). Artificial intelligence–based Chatbot for anxiety and depression in university students: pilot randomized controlled trial. JMIR Formative Res. 5:e20678. doi: 10.2196/20678
L’Heureux, A., Grolinger, K., and Capretz, M. A. M. (2022). Transformer-based model for electrical load forecasting. Eng. 15:4993. doi: 10.3390/en15144993
Leavy, S. (2018). Gender bias in artificial intelligence: the need for diversity and gender theory in machine learning. In Proceedings of the 1st international workshop on gender equality in software engineering (GE ‘18). New York, NY, USA, 14–16
Lee, Y. F., Hwang, G. J., and Chen, P. Y. (2022). Impacts of an AI chatbot on college students’ after-class review, academic performance, self-efficacy, learning attitude, and motivation. Educ. Technol. Res. Dev. 70, 1843–1865. doi: 10.1007/s11423-022-10142-8
Libert, K. (2023). Only 34% of educators support the decision to ban ChatGPT. EINPRESSWIRE, January 16, 2023. Available at: https://www.einpresswire.com/article/611597643/only-34-of-educators-support-the-decision-to-ban-chatgpt (Accessed January 22, 2023).
Lopez-Lira, A., and Tang, Y. (2023). Can ChatGPT forecast stock Price movements? Return Predictability and Large Language Models. SSRN Preprint. Available at: http://dx.doi.org/10.2139/ssrn.4412788
Lukpat, A. (2023). ChatGPT banned in new York City public schools over concerns about cheating, learning development. Available at: https://www.wsj.com/articles/chatgpt-banned-in-new-york-city-public-schools-over-concerns-about-cheating-learning-development-11673024059 (Accessed at January 8, 2023).
Lund, B., and Agbaji, D. (2023). Information literacy, data literacy, privacy literacy, and ChatGPT: Technology literacies align with perspectives on emerging technology adoption within communities (January 14, 2023). SSRN Preprint. Available at: http://dx.doi.org/10.2139/ssrn.4324580
Mabunda, K., and Ade-Ibijola, A. (2019). Pathbot: an intelligent chatbot for guiding visitors and locating venues. IEEE Xplore. 2019 6th international conference on soft computing & machine intelligence (ISCMI), 160–168
Maynez, J., Narayan, S., Bohnet, B., and McDonald, R. (2020). On faithfulness and factuality in abstractive summarization. In Proceedings of the 58th annual meeting of the Association for Computational Linguistics, 1906–1919
McCallum, S. (2023). ChatGPT banned in Italy over privacy concerns. BBC News. Available at: https://www.bbc.com/news/technology-60929651 (Accessed April 13, 2023).
Meckler, L., and Verma, P. (2022) Teachers are on alert for inevitable cheating after the release of ChatGPT. The Washington Post. Available at: https://www.washingtonpost.com/education/2022/12/28/chatbot-cheating-ai-chatbotgpt-teachers/ (Accessed January 2, 2023).
Miller, F., Katz, J., and Gans, R. (2018). AI x I = AI2: the OD imperative to add inclusion to the algorithms of artificial intelligence. OD Practitioner 5, 6–12.
Murtarellia, G., Gregory, A., and Romentia, S. (2021). A conversation-based perspective for shaping ethical human–machine interactions: the particular challenge of chatbots. J. Bus. Res. 129, 927–935. doi: 10.1016/j.jbusres.2020.09.018
Norris, M. (2019). University online cheating -- how to mitigate the damage. Res. High. Educ. J. 37, 1–20.
Nunamaker, J., Derrick, D., Elkins, A., Burgoon, J., and Patton, M. (2011). Embodied conversational agent-based kiosk for automated interviewing. J. Manag. Inf. Syst. 28, 17–48. doi: 10.2753/MIS0742-1222280102
O’Donovan, M. A., McCallion, P., McCarron, M., Lynch, L., Mannan, H., and Byrne, E. (2019). A narrative synthesis scoping review of life course domains within health service utilisation frameworks [version 1; peer review: 2 approved]. HRB Open Res. 2, 1–18. doi: 10.12688/hrbopenres.12900.1
Okonkwo, C. W., and Ade-Ibijola, A. (2021). Chatbots applications in education: a systematic review. Comput. Educ. 2:100033. doi: 10.1016/j.caeai.2021.100033
OpenAI (2022). Playground. Available at: www.OpenAI.com and https://beta.openai.com/playground
OpenAI (2023). AI text classifier [website]. Available at: https://beta.openai.com/ai-text-classifier (Accessed April 8, 2023).
OpenAI (2023). GPT-4. Available at: https://openai.com/research/gpt-4 (Accessed April 9, 2023).
OpenAI (2023). GPT-4 technical report. arXiv preprint arXiv:2303.08774. doi: 10.48550/arXiv.2303.08774
Ordonez, V., Dunn, T., and Noll, E. (2023). OpenAI CEO Sam Altman says AI will reshape society, acknowledges risks: ‘a little bit scared of this’. Available at: https://abcnews.go.com/Technology/openai-ceo-sam-altman-ai-reshape-society-acknowledges/story?id=76971838 (Accessed April1, 2023).
Originality. AI – plagiarism checker and AI detector (2023). Available at: https://originality.ai/#about (Accessed January 3, 2023).
Ortiz, S. (2022). What is ChatGPT, and why does it matter? Here’s what you need to know. ZDNET. Available at: https://www.zdnet.com/article/what-is-chatgpt-and-why-does-it-matter-heres-what-you-need-to-know/ (Accessed January 1, 2023).
Page, L., and Gehlbach, H. (2017). How an artificially intelligent virtual assistant helps students navigate the road to college. AERA Open 3:233285841774922. doi: 10.1177/2332858417749220
Page, M. J., McKenzie, J. E., Bossuyt, P. M., Boutron, I., Hoffmann, T. C., Mulrow, C. D., et al. (2021). The PRISMA 2020 statement: an updated guideline for reporting systematic reviews. BMJ 372:n71. doi: 10.1136/bmj.n71
Patel, S. B., and Lam, K. (2023). ChatGPT: the future of discharge summaries? Lancet Digit. Health 5, e107–e108. doi: 10.1016/S2589-7500(23)00021-3
Pavlik, J. (2023). Collaborating with ChatGPT: considering the implications of generative artificial intelligence for journalism and media education. J. Mass Commun. Educ 78, 84–93. doi: 10.1177/10776958221149577
Perry, N., Srivastava, M., Kumar, D., and Boneh, D. (2022). Do users write more insecure code with AI assistants? arXiv preprint arXiv:2211.03622v2 [cs.CR]. doi: 10.48550/arXiv.2211.03622
Peters, M. D. J., Godfrey, C. M., Khalil, H., McInerney, P., Parker, D., and Soares, C. B. (2015). Guidance for conducting systematic scoping reviews. Int. J. of Evid. Based Healthc. 13, 141–1461. doi: 10.1097/XEB.0000000000000050
Peters, M. D. J., Marnie, C., Tricco, A. C., Pollock, D., Munn, Z., Alexander, L., et al. (2020). Updated methodological guidance for the conduct of scoping reviews. JBI Evi. Synthesis 18, 2119–2126. doi: 10.11124/JBIES-20-00167
Pham, X., Pham, T., Nguyen, Q., Nguyen, T., and Cao, T. (2018). Chatbot as an intelligent personal assistant for mobile language learning. ICEEL 2018: Proceedings of the 2018 2nd international conference on education and E-learning, 16–21
Pickard, M., Schuetzler, R., Valacich, J., and Wood, D. (2017). Next-generation accounting interviewing: a comparison of human and embodied conversational agents (ECAs) as interviewers. SSRN Electron. J. April, 1–21. doi: 10.2139/ssrn.2959693
Point, Check (2023). OPWNAI: Cybercriminals starting to use CHATGPT. Available at: https://research.checkpoint.com/2023/opwnai-cybercriminals-starting-to-use-chatgpt/ (Accessed January 18, 2023).
Qin, C., Zhang, A., Zhang, Z., Chen, J., Yasunaga, M., and Yang, D. (2023). Is ChatGPT a general-purpose natural language processing task solver? arXiv preprint arXiv:2302.06476. doi: 10.48550/arXiv.2302.06476
Rahim, N. I. M., Iahad, N. A., Yusof, A. F., and Al-Sharafi, M. A. (2022). AI chatbots adoption model for higher-education institutions: a hybrid PLS-SEM-neural network modelling approach. Sustainability 14:12726. doi: 10.3390/su141912726
Ramos-Pérez, E., Alonso-González, P., and Núñez-Velázquez, J. (2021). Multi-transformer: a new neural network-based architecture for forecasting S&P Volatility. Math 9:1794. doi: 10.3390/math9151794
Ranoliya, B., Raghuwanshi, N., and Singh, S. (2017). Chatbot for university-related faqs. IEEE Xplore. 2017 international conference on advances in computing, communications, and informatics (ICACCI), 1525–1530
Reis-Marques, C., Figueiredo, R., and Neto, M. (2021). Applications of Blockchain technology to higher education arena: a bibliometric analysis. Eur. J. Investig. Health Psychol. Educ. 11, 1406–1421. doi: 10.3390/ejihpe11040101
Reza, S., Campos, M., Machado, J., and Tavares, J. (2022). A multi-head attention-based transformer model for traffic flow forecasting with a comparative analysis of recurrent neural networks. Expert Syst. Appl. 202:117275. doi: 10.1016/j.eswa.2022.117275
Rodchua, S., Yaiadom-boakye, G., and Woolsey, R. (2011). Student verification system for online assessments: bolstering quality and integrity of distance learning. J. Technol. Manag. Appl. Eng. 27, 1–8.
Roose, K. (2022). The brilliance and weirdness of ChatGPT. The New York Times. Available at: https://www.nytimes.com/2022/12/05/technology/chatgpt-ai-twitter.html (Accessed January 1, 2023).
Ruipérez-Valiente, J., Muñoz-Merino, P., Alexandron, G., and Pritchard, D. (2017). Using machine learning to detect ‘multiple-account’ cheating and analyze the influence of student and problem features. IEEE Trans. Learn. Technol. 12, 112–122. doi: 10.1109/TLT.2017.2784420
Ryan-Mosley, T. (2023). Five big takeaways from Europe’s AI act. MIT Technology Review. Available at: https://www.technologyreview.com/2023/06/19/1075063/five-big-takeaways-from-europes-ai-act/ (Accessed June 25, 2023).
Sandu, N., and Gide, E. (2022). Adoption of AI-Chatbots to enhance student learning experience in higher education in India. IEEE Xplore. 2019 18th Int. Conf. On information technology based higher education and training (ITHET)
Sangalli, V., Martinez-Muñoz, G., and Cañabate, E. (2020). “Identifying cheating users in online courses” in IEEE Xplore. 2020 IEEE global engineering education conference (EDUCON), 1168–1175.
Shankland, S. (2022). Why Everyone’s obsessed with ChatGPT, a mind-blowing AI Chatbot. CNET. Available at: https://www.cnet.com/tech/computing/why-everyones-obsessed-with-chatgpt-a-mind-blowing-ai-chatbot/ (Accessed January 1, 2023).
Shorey, S., Ang, E., Yap, J., Ng, E., Lau, S., and Chui, C. (2019). A virtual counseling application using artificial intelligence for communication skills training in nursing education: development study. J. Med. Internet Res. 21:e14658. doi: 10.2196/14658
Shrivastava, R. (2022). Teachers fear ChatGPT will make cheating easier than ever. Forbes. Available at: https://www.forbes.com/sites/rashishrivastava/2022/12/12/teachers-fear-chatgpt-will-make-cheating-easier-than-ever/?sh=5b0ab2ac1eef (Accessed on January 2, 2023).
Shuster, K., Poff, S., Chen, M., Kiela, D., and Weston, J. (2021). Retrieval augmentation reduces hallucination in conversation. arXiv preprint arXiv:2104.07567v1 [cs.CL]. doi: 10.48550/arXiv.2104.07567
Sinha, S., Basak, S., Dey, Y., and Mondal, A. (2020). An educational Chatbot for answering queries. In: J. Mandal and D. Bhattacharya (Eds) Emerging Technology in Modelling and Graphics, 937. Springer: Singapore
Slepankova, M. (2021). Possibilities of artificial intelligence in education: an assessment of the role of AI Chatbots as a communication medium in higher education (dissertation). Available at: http://urn.kb.se/resolve?urn=urn:nbn:se:lnu:diva-108427 (Accessed January 1, 2023).
Smith, C. (2023). GPT-5 might make ChatGPT indistinguishable from a human. BGR. Available at: https://bgr.com/tech/gpt-5-might-make-chatgpt-indistinguishable-from-a-human/ (Accessed April 1, 2023).
Sobania, D., Briesch, M., Hanna, C., and Petke, J. (2023). An analysis of the automatic bug fixing performance of ChatGPT. arXiv preprint arXiv:2301.08653. doi: 10.48550/arXiv.2301.08653
Soper, T. (2023). Seattle public schools bans ChatGPT; district ‘requires original thought and work from students’. GeekWire. Available at: https://www.geekwire.com/2023/seattle-public-schools-bans-chatgpt-district-requires-original-thought-and-work-from-students/ (Accessed January 1, 2023).
Stanley, J., Brink, R., Valiton, A., Bostic, T., and Scollan, R. (2022). “Chatbot accessibility guidance: a review and way forward” in Proceedings of sixth international congress on information and communication technology. eds. X. S. Yang, S. Sherratt, N. Dey, and A. Joshi, vol. 216 (Singapore: Springer)
Susnjak, T. (2022). ChatGPT: the end of online exam integrity? arXiv preprint arXiv:2212.09292v1 [cs.AI]. doi: 10.48550/arXiv.2212.09292
Tallyn, E., Fried, H., Gianni, R., Isard, A., and Speed, C. (2018). The Ethnobot: gathering ethnographies in the age of IoT. Conference on human factors in computing systems - proceedings
Tate, K. (2021). Artificial intelligence will destroy ‘laptop class’ workers. The Hill. Available at: https://thehill.com/opinion/technology/541331-artificial-intelligence-will-destroy-laptop-class-workers (Accessed April 2, 2023).
Taulli, T. (2019). Artificial intelligence basics: A non-technical introduction. 1st Edn. CA: Apress Berkeley.
Tegos, S., Demetriadis, S., and Karakostas, A. (2015). Promoting academically productive talk with conversational agent interventions in collaborative learning settings. Comput. Educ. 87, 309–325. doi: 10.1016/j.compedu.2015.07.014
The Washington Post - Editorial Board (2022). Opinion. We asked an AI bot hundreds of questions. Here’s what we learned. Available at: https://www.washingtonpost.com/opinions/interactive/2022/chatgpt-questions-predictions-ethics/ (Accessed January 2, 2023).
Trezise, K., Ryan, T., Barba, P., and Kennedy, G. (2019). Detecting contract cheating using learning analytics. J. Learn. Anal. 6, 90–104. doi: 10.18608/jla.2019.63.11
Tsai, C., and Wu, J. (2022). “A Blockchain-based fair and transparent homework grading system for online education” in Principles and practice of blockchains. eds. K. Daimi, I. Dionysiou, and N. El Madhoun (Cham: Springer)
Vallés-Pérez, I., Soria-Olivas, E., Martínez-Sober, M., Serrano-López, A., Gómez-Sanchís, J., and Mateo, F. (2022). Approaching sales forecasting using recurrent neural networks and transformers. Expert Syst. Applica. 201:116993. doi: 10.1016/j.eswa.2022.116993
Vaswani, A., Shazeer, N., Parmar, N., Uszkoreit, J., Jones, L., and Polosukhin, I. (2017). Attention is all you need. arXiv preprint arXiv:1706.03762 [cs.CL] 31st Conf. On neural Inf. Process. Systems (NIPS 2017), Long Beach, CA, USA
Walters, L. (2021). Universities expand controversial AI monitoring of students taking online exams. Stuff. Available at: https://www.stuff.co.nz/national/education/126908715/universities-expand-controversial-ai-monitoring-of-students-taking-online-exams (Accessed January 4, 2023).
Wang, Y., Petrina, S., and Feng, F. (2017). VILLAGE—virtual immersive language learning and gaming environment: immersion and presence. Br. J. Educ. Technol. 48, 431–450. doi: 10.1111/bjet.12388
Writer. (n.d.). AI content detector | GPT-3 | GPT-4 | ChatGPT. Available at: https://writer.com/ai-content-detector/ (Accessed April 8, 2023).
Wu, N., Green, B., Ben, X., and O’Banion, S. (2020). Deep transformer models for time series forecasting: the influenza prevalence case. arXiv preprint arXiv:2001.08317v1 [cs.LG]. doi: 10.48550/arXiv.2001.08317
Xiao, Z., Zhou, M. X., Chen, W., Yang, H., and Chi, C. (2020). If I hear you correctly: building and evaluating interview Chatbots with active listening skills. In CHI ‘20: Proceedings of the 2020 CHI conference on human factors in computing systems 1–14)
Xiao, Z., Zhou, M., Liao, Q., Mark, G., Chi, C., Chen, W., et al. (2020). Tell me about yourself: using an AI-powered Chatbot to conduct conversational surveys. ACM Trans. Comput.-Hum. Interact. 27, 1–37. doi: 10.1145/3381804
Zhai, X. (2023). ChatGPT for next generation science learning. SSRN Preprint. (January 20, 2023). doi: 10.2139/ssrn.4331313
Keywords: ChatGPT, AI, chatbot, artificial intelligence, higher education, natural language processing, transformers, digital revolution
Citation: Dempere J, Modugu K, Hesham A and Ramasamy LK (2023) The impact of ChatGPT on higher education. Front. Educ. 8:1206936. doi: 10.3389/feduc.2023.1206936
Edited by:
Julius Nganji, University of Toronto, CanadaReviewed by:
Ayesha Kanwal, University of Glasgow, United KingdomIjeoma John-Adubasim, University of Plymouth, United Kingdom
Tareq Al Jaber, University of Hull, United Kingdom
Copyright © 2023 Dempere, Modugu, Hesham and Ramasamy. This is an open-access article distributed under the terms of the Creative Commons Attribution License (CC BY). The use, distribution or reproduction in other forums is permitted, provided the original author(s) and the copyright owner(s) are credited and that the original publication in this journal is cited, in accordance with accepted academic practice. No use, distribution or reproduction is permitted which does not comply with these terms.
*Correspondence: Juan Dempere, amRlbXBlcmVAaGN0LmFjLmFl