- Mary Lou Fulton Teachers College, Arizona State University, Tempe, AZ, United States
This paper presents a unifying account for why many biological, ecological and other science processes that are taught in school curricula and those occurring in our everyday environment (such as the spread of Covid19) are particularly difficult for students to understand. These often-misconceived processes are Emergent processes. The hypothesis is that students bring the perspective of Individualistic (a form of linear) thinking suitable for understanding Sequential processes, to explain Emergent processes, instead of the more appropriate perspective of Collective (a form of systems) thinking, thereby resulting in misunderstanding. This paper describes a framework called PAIR-C that defines the causal knowledge structures underlying both Individualistic and Collective thinking needed for understanding many science processes. PAIR-C explains why students generate misconceptions; shows how the Collective causal structure can help understand many Emergent processes; and suggests a new instructional approach.
1. Introduction
Misconceptions are ubiquitous incorrect causal explanations, manifested across a variety of science concepts in numerous disciplines, across age groups, and at all academic levels. Misconceptions differ from many other kinds of incorrect knowledge in that misconceptions are often difficult to remediate with instruction (See Chi, 2013 for other kinds of incorrect knowledge about science processes that can be more easily corrected). Thus, such misconceptions have been a persistent hindrance in preventing students from learning many science concepts with deep understanding. This paper takes the perspective that a misconception is expressed when students construct an explanation to a question or problem posed to them, by reasoning from an available causal knowledge structure. An available causal knowledge structure refers to a schema-like organized and coherent unit of core knowledge about causality relevant for understanding many science processes. To reason from an available causal knowledge structure means that students apply one or more features from this causal structure to construct a response.
This paper presents a final, updated, more coherent and complete, yet more parsimonious version of a framework that has evolved over the last three decades, starting with our initial naïve view of considering misconceptions merely as a misunderstanding between processes versus entities (Chi, 1992; Chi et al., 1994; Chi, 2005; Chi et al., 2012; Chi, 2013). The framework is now called PAIR-C (acronym for Pattern, Agents, Interactions, Relations between the interactions, and Causal mechanisms between the Agents and the Pattern). It addresses this long-standing dire learning problem by explaining (a) why students exhibit misconceptions for numerous science concepts taught in their school curricula, across biological, chemical, ecological, and other disciplines; (b) why their misconceived ideas are robust and difficult to overcome with instruction; and (c) how one might design instruction to avoid and overcome misconceptions. A sample of six misconceived responses are shown in Table 1.
Since the literature has amply documented, described, discussed, and debated misconceptions and their remediation for decades e.g., (see the two volumes on conceptual changed edited by Vosniadou, 2008, 2013), this paper therefore is not meant to be a synthesis or review of the literature. Recent syntheses and studies can be found, for example, in Grotzer et al. (2021) of students’ difficulty in understanding causality, and in Elsawah et al. (2021) for how to teach systems thinking from a post-secondary perspective. Instead, the goal of this paper is to present a completed version of PAIR-C and show how it can address the three questions stated above.
Although the extensive literature on misconceptions has not provided a satisfactory explanation for the three questions raised above, the content of misconceptions have often been characterized by what Perkins and Grotzer (2005) have referred to as “token statements.” Here are several examples. One common characterization is to say that misconceptions are teleological explanations (Coley and Gelman, 1989) regarding the purpose something serves rather than the cause by which it arises. For example, in order to explain why giraffes’ necks get longer over generations, a typical misconceived explanation is that “because giraffes need to have longer necks in order to reach the leaves on the tall trees.” A second way to characterize misconceptions is to say that they show anthropocentric thinking, such as students attributing human intentional behavior of escaping to hot molecules for heat flow, such as from a coffee cup (see Table 1, Ex. 5 below, Slotta et al., 1995). A third way to characterize misconceptions is to suggest that they are reflections of a student’s individual experiential pieces of knowledge, acquired from everyday experiences (Disessa, 1988). For example, students might believe that an object can stay in motion only if something is applying a force to it, and this motion-implies-force misconception is derived from their frequent experiences in pushing an object in a world with friction. A fourth way to characterize the content of misconceptions is to propose that students think of these process concepts as entity concepts, or the movement of entities (e.g., concrete objects or substances, Chi et al., 1994; Reiner et al., 2000). For example, heat flow is misconceived as the movement of concrete objects such as hot molecules. Similarly, for the process of diffusion of ink molecules in water, flow is usually misconceived as moving ink molecules. Likewise, for a concept such as force, students often conceived of it as substances that flow or provides an “oomph” (the substance is akin to gasoline that propels cars) that can either dissipate or be consumed (McCloskey, 1983).
However, although we and other researchers have spent considerable efforts in characterizing the content of misconceptions, such undertakings did not lead to a coherent and comprehensive framework explaining why students misconceive of many science process-concepts that way, nor how such processes should be taught. Thus, instead of a set of token statements, this paper proposes a theoretical framework, PAIR-C, that offers a unified explanation of the kind of misconceptions mentioned in the preceding paragraph.
2. Characterizing the massive amount of evidence collected about misconceptions
In order to come up with a framework that explains misconceptions and how they may be remediated, instead of characterizing the content of misconceptions with token statements, it may be more productive to consider what the vast amount of evidence on misconceptions tell us about (a) what kind of process concepts are misconceived, (b) how misconceptions differ from other forms of incorrect knowledge, (c) when misconceptions are revealed, (d) how students generate them, and (e) the kinds of instructional interventions that have not been successful, by-and-large. Below we summarize what the evidence suggests with respect to these questions and what our assumptions or interpretations of the evidence are. Again, an exhaustive review will not be provided; instead, we will briefly describe the conclusions drawn from sampling this massive amount of evidence collected over the years both from our own lab as well as in the literature (see the misconceptions compiled in the bibliographies published over five decades by Pfundt and Duit (1981, 1985, 1988, 1991, 1997, 2001), composed of well over 8,400 entries).
2.1. Misconceptions are expressed for a variety of science concepts of emergent processes taught in standard school curricula across multiple domains
As shown in Table 1, a diverse set of concepts and phenomena can be misconceived. Moreover, these misconceived phenomena and concepts discussed in the literature tend to be processes spanning multiple STEM domains commonly taught in schools’ standard science curricula (Reiner et al., 2000; see also a review by Confrey, 1990). Processes that have been frequently examined are concepts such as heat (Erickson, 1979), light (Guesne, 1985), motion (Halloun and Hestenes, 1985), electric current (Cosgrove and Osborne, 1985), pressure (Mayer, 1987), and natural selection (Gregory, 2009), even though these processes are labeled as nouns. In contrast, fewer articles were written about misconceptions of entities, such as the atom (Pfundt, 1981) or the earth (Vosniadou, 1994), perhaps because misunderstanding of entity concepts are easier to remediate (Chi, 2013). Most importantly, because many of the misconceived processes are commonly taught in school curricula, there is a critical need for students to understand them correctly.
Processes outside of STEM domains are also misconceived (Brandts et al., 2021), such as the gyrations of the stock market (Kuhlmann, 2014), how revolutions and jihads arise (Bousquet, 2011), and how religious and cultural rituals spread (e.g., Moya and Henrich, 2016). Misconceived processes also include many familiar ones in our everyday environment, such as forest fire spreading and geese flying in a V-formation. Numerous studies continue to extend the list of processes that are misconceived, documenting the existence and prevalence of misconceptions for various science processes that have not been documented before (Dickes et al., 2016) and how they might be taught (e.g., the concept of spreading, Grotzer et al., 2017).
School curricula teach numerous processes in science domains, such as: respiration, photosynthesis, mitosis, diffusion, cell division, heat transfer, phases of moon, floating and sinking, flu spreading, and so forth, but not all science processes taught in school curricula are misconceived. Chi (2005) had labeled the highly misconceived processes as a kind of Emergent processes, in contrast to Sequential processes that are less likely to be misconceived and claimed that these two kinds of processes are ontologically distinct. (How these two kinds of processes are ontologically distinct will be fully distinguished in this paper.) But often the two kinds of processes are discussed in the same paragraph, without any indication that they are different kinds of processes. For example, a biology text will often describe the circulatory system or how blood travels from the heart to the lungs (a Sequential process), and in the same paragraph, describe the diffusion of oxygenated and deoxygenated blood across membranes (an Emergent process). It seems that providing heuristics to discriminate the two kinds of process might be necessary before we try to improve understanding of the Emergent kind of processes. PAIR-C includes such heuristics for discrimination.
One conclusion or assumption to draw from this set of evidence is that there might be a common underlying similarity among all the Emergent processes that are misconceived. This similarity needs to be articulated.
2.2. Misconceptions are revealed when students explain incorrectly the causality of emergent processes
Emergent processes have the characteristics of having many elements or agents whose collective interactions at each point in time produce an outcome; and the outcomes, when observed over time, provide an often-visible pattern that are meaningful. The point to make here is that misconceptions are typically revealed when questions are asking about the causality of how the observed pattern can be caused by the agents. In Ex. 5 in Table 1, for instance, the question asking about how the pattern of heat flows from one location to another location, is really asking about how the hot molecules can cause heat or hotness to propagate from the coffee cup into the air. For the question of how ants come to walk in a single queue to find food (Ex. 2, Table 1), is really a question about what causes the ants to know to move in a single file toward the source of food. Answers to these questions reveal misconceptions when students have to reason causally to explain why agents within a process move the way they do or behave in a way to display the pattern of the process that is seen.
One conclusion from this set of observations is that we might see a finite set of misconceived reasons expressed given for each causal question. This finite set of reasons, once coded, can reflect the set of features underlying the causal knowledge structure that generated them. Details of such an underlying causal structure is described in Section 3, below.
2.3. How are misconceptions of emergent processes different from other forms of incorrect knowledge
There are many other ways that knowledge can be incorrect. That is, there are many concepts that are difficult for students to understand, thereby causing incorrect understanding, but they are not misconceptions, such as students’ initial beliefs can be false, or their initial mental models can be flawed in some ways, or they have gaps of missing knowledge, and so forth, as laid out in Chi (2013). One criterion Chi (2013) had proposed for deciding whether incorrect knowledge is misconceived is whether it can be remediated successfully, even if the instructional remediation is difficult and time-consuming.
This paper cannot expand thoroughly upon the various other forms of incorrect understanding that are not misconceptions, but a few examples can be described. As alluded to above in Section 2.2, Emergent processes tend to be misconceived. However, there are many other concepts and processes that are not Emergent, but can be difficult to understand, though not in a misconceived way. Three examples will illustrate the reasons other concepts can be difficult to understand. First, some processes can be complicated in the sense that it has several components. For example, in contrast to complex systems, decomposable systems (a process with many components in which most of the communication or connection takes place within each subsystem with little or no connections among the subsystems, Simon, 2002), such as how an automobile engine works, are difficult to understand because it has many parts with many interconnections within each part. Second, other concepts such as the process of recursion loop in programming is also inherently difficult to understand because it is not a sequence of similar actions, but a nesting of similar actions. Third, sometimes the representations used to explain a concept may be confusing. For example, cladograms have been used extensively in high school biology texts and they have been shown to be confusing for understanding macroevolution (Catley and Novick, 2008). In short, there are many reasons for why various concepts are difficult to understand but the difficulty is unrelated to the nature of misconceptions. This will be clearer after PAIR-C is presented below.
There are other forms of incorrect knowledge in everyday life, such as myths. Myths are idiosyncratic superstitious beliefs that some people believe in with no or refuted basis (Horne et al., 2015), such as a link between vaccination and autism. Misconceptions differ from myths in two major ways. First, although both myths and misconceptions are difficult to dispel, one difference between them is that students with misconceptions often agree, when pressed by instructors or experts, that their own explanations are incorrect and that experts and scientists know the correct ideas, even though it is nevertheless difficult for them to understand why their explanations are incorrect; whereas people who believe in myths do not acknowledge that their knowledge is incorrect. Second, myths are not commonly held by the majority of people within the population, whereas misconceptions are rampant and widespread, occurring for many science concepts, and revealed in students’ thought-processes at all grade levels, including college level.
One conclusion or assumption to draw from this set of evidence is that misconceptions are robust perhaps because they are generated from a familiar and reliable causal knowledge structure. The nature of what a misconception is will be clear after PAIR-C is presented below in section 3.
2.4. Two observations about how students generate misconceptions: confident and yet fickle
This section describes two observations about the ways students generate misconceptions. First, in contrast to many occasions when students are hesitant to give an explanation when they lack knowledge or are uncertain, students seem willing, confident and ready to spontaneously offer a causal explanation to the questions asked about Emergent processes, as opposed to saying “I do not know,” or pausing, or hesitating to indicate uncertainty. This suggests that they are unaware that their responses are misconceived. Thus, students’ certainty, confidence and spontaneity in giving a misconceived explanation suggest that their explanations might be generated from a familiar and robust causal knowledge structure; therefore, it is feasible to capture what this existing causal knowledge structure might be that they rely on to generate their misconceived responses.
Second, apparent in many interview studies with open-ended responses, students also seem to be fickle in the sense that they are quite willing to change their misconceived explanations when confronted by the interviewer or refuted by teachers, by providing another explanation that continues to be misconceived. Thus, by “fickle,” we mean that a variety of misconceived explanations to the same question seem to be available to students, and they seem not to hesitate to draw upon these other reasons if a given one is challenged. See interview studies by diSessa (1988), for examples. More recent scholars have referred to such variation in responses as “erratic” (Chao et al., 2018). Our interpretation of this fickleness is that when their initial explanations are challenged, they eagerly offer another response by drawing upon another feature from the same familiar and available causal knowledge structure. Thereby this alternative response is equally misconceived.
The conclusion we draw from the observations of certainty, unawareness, fickleness and willingness to change their responses, together, suggest that misconceptions may be confidently generated by reasoning from a familiar causal knowledge structure. Such a causal structure may have several dimensions with features, and students seem fickle when they are challenged, because they simply draw upon a different feature within the same causal structure to provide another misconceived explanation.
2.5. Students resist remediation: instructional challenge
The most problematic issue of misconceptions is that they are robust and resist remediation. Although there are pockets of apparent successes in remediating misunderstanding, by-and-large, remediation approaches have been unsuccessful. Moreover, instructional intervention attempts have typically addressed one concept at a time, taking a “concept-specific” approach, utilizing several different pedagogical tactics. One pedagogical tactic is to induce cognitive conflict through the presentation of anomalous data, or contradictory information, or unpredicted outcome (see Posner et al., 1982; Solomon, 1983; Champagne et al., 1985; Cosgrove and Osborne, 1985; Limon, 2001). A slight variant of this pedagogical tactic is confrontation, such as asking students to make a prediction followed by a laboratory demonstration showing students’ predictions are incorrect. A second pedagogical tactic is to use refutation. Refutation typically occurs in text, in which a correct statement would directly contradict or reject a student’s misconceived statement of a specific aspect of a concept (Sinatra and Broughton, 2011; Thacker et al., 2020). A third pedagogical tactic is to build on some aspects of students’ misconceived ideas by providing analogous cases or anchoring examples for students to compare with their own ideas (Clement et al., 1989). This bridging approach reduces a concept into its component parts and the analogy targets at bridging with individual components of students’ correct ideas. For example, a normal force is explained by first seeing that the surface is not a uniform substance but is composed of tiny springy ball-like atoms so that the surface pushes back. Research continues to explore new ways of remediating misconceptions of specific school-taught concepts through instruction. For example, for the concept of natural selection, studies continue to explore ways of teaching it to both young and older children (e.g., Shtulman et al., 2016) and college students (e.g., Legare et al., 2018).
One interpretation for this set of findings is that misconceptions about Emergent processes are robust because students can only interpret Emergent processes from a familiar causal knowledge structure perspective (this familiar causal structure will be referred to later as the Individualistic causal structure), because they lack any knowledge of the alternative causal structure (to be called the Collective causal structure) from which Emergent processes should be interpreted. This suggests that a remediation approach may require teaching students about the Collective causal structure per se, as lacking knowledge of it may be the cause of relying on the inappropriate Individualistic causal structure for understanding, thereby leading to misconceptions.
2.6. Summary of observations about misconceptions and our concluding assumptions
In sections 2.1 to 2.5, instead of characterizing the content of misconceptions, we describe our observations and interpretations about the evidence of misconceptions, in terms of (a) the kind of processes that reveal misconceptions, (b) what kind of questions elicit misconceived explanations, (c) how misconceptions differ from other forms of incorrect knowledge, (d) the two ways that students generate misconceptions (e.g., readiness to generate yet unaware that it is incorrect, and fickleness or confidence in generating an alternative but still misconceived explanation), and (e) the robustness of misconceptions. These observations led to the assumptions that (a) a core Individualistic causal knowledge structure with several features could have generated the misconceptions, that (b) this existing core structure must be very familiar to students, as explanations are generated willingly and consistently from this core knowledge structure (as opposed to saying “I do not know” or being hesitant), and (c) students’ revised explanations can be changed by reasoning from the same Individualistic knowledge structure, such as drawing upon another feature from the Individualistic causal structure, thereby the re-generated explanation continues to be misconceived, and moreover, that (d) students seem to lack any knowledge of an alternative Collective causal structure.
3. The PAIR-C framework: the causal structures underlying everyday and school-based science processes
Although preliminary ideas of PAIR-C have been presented earlier (Chi et al., 1994; Chi, 2005; Chi et al., 2012), they were incomplete and incoherent in many ways. Four prominent and significant improvements can be pointed out here, in contrast to the last version spelled out in Chi et al. (2012). First, most importantly, the current version consolidated the features more sensibly into five common dimensions that can be used to describe the causal structures of both Sequential and Emergent kind of processes. That is, these five dimensions, in addition to the dimension of time, can be conceived of as a way to represent processes. Moreover, these five dimensions also form a coherent set, by which we mean that when a process is explained with one feature of a causal structure, it can also be explained by another feature within the same causal structure. Second, most critically, explication of how the Pattern transforms dynamically and the corresponding computation of the Agent-Pattern causal mechanisms (to be referred to as “cumulative” and “collective summing”1) are clear addition to the literature and not addressed in the earlier renditions of the PAIR-C framework. For example, Penner (2000) acknowledged that the Agent-Pattern causal relation of complexity concepts is difficult for students, but he did not specify what that mechanism is that explains the relationships. In short, PAIR-C describes explicitly the mechanism of emergence, in both qualitative and quantitative terms. Third, many features expressed in misconceptions are now viewed as implications of the main contrasting features that students can infer, thus further consolidating the features captured in expressed misconceptions, making the framework itself more parsimonious, and at the same time, allowing for variations in what students infer from each feature. Fourth, in the current version as well as prior versions, the features and mechanisms are specified in basic, concrete, and more grounded terminologies (such as interacting with a “restricted other agent” vs. a “random other” agent, see Table 2, Dimension III), without resorting to the use of more cryptic and undefined terminologies such as “nonlinearity,” “self-organization,” “stochastic processes,” “direct and indirect feedback loops,” “adaptive,” to name a few, as well as avoiding the use of mathematical tools (such as causal loops diagrams, differential equations, and computer simulation technique, etc.) that can present a barrier to understanding for naïve students.
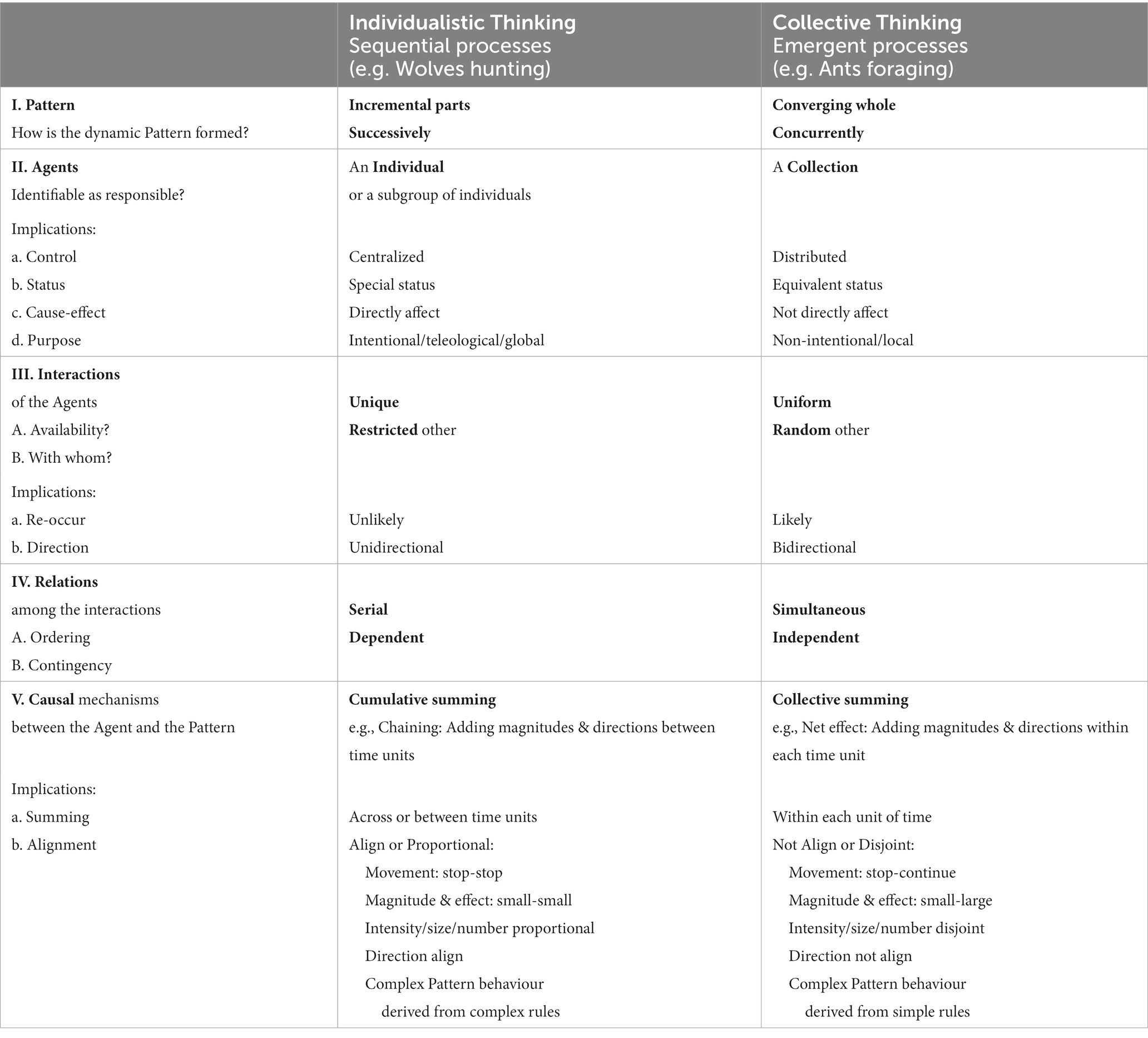
Table 2. The five dimensions, their contrasting features and implications of the features, of two kinds of causal knowledge structures (Individualistic and Collective) for understanding Sequential and Emergent processes. (Key contrasting features are bolded below and appear in quotes in the text).
For each dimension of the PAIR-C framework (Table 2), we first describe what the dimension entails, then the contrasting features for the Individualistic and Collective causal structures, followed by the implications of the features. Throughout, we illustrate mainly with familiar everyday examples, such as wolves hunting a prey as a Sequential process and ants foraging for food as an Emergent process, so that no additional explanations are needed. These two examples both exhibit a similar pattern of a queue-like line, and both have the end goal of getting food. Other pairs from our everyday environment might be geese forming a V-shape (as an Emergent process) and the corresponding pilots flying in a V-shape (as a Sequential process). We also illustrate with the simplest of science process, diffusion of ink in water or the familiar process of the peppered moths getting darker due to industrialization. Also, referrals to the dimensions of PAIR-C are capitalized.
3.1. The Pattern (P)
The Pattern dimension refers to the often-visible behaviors displayed by dynamic processes. Our notion of Pattern is similar to what complexity scholars talk about as “macro level,” “order,” “large-scale pattern” (Yoon et al., 2017, p. 2), “global pattern,” or “large patterns” of a process (Dennett, 1995, p. 102) for complex dynamic systems. In contrast to the complexity scholars’ perspective on emerging pattern, PAIR-C assumes that Patterns exist for both Sequential and Emergent processes. More critically, the importance of the Pattern differs for PAIR-C and the complexity perspective. For PAIR-C, the importance of Pattern concerns the fact that the Pattern of processes are often meaningful to the observers, thus needing to be explained (elaborated below); whereas the main focus of complexity researchers is on the unpredictability of the large-scale patterns or aggregate behaviors (Nowak and May, 1992). Most importantly, the PAIR-C framework claims that recognition of a familiar or meaningful pattern is an important source of misconceptions, to be elaborated below.
The Patterns of both Sequential and Emergent processes can have four important properties. First, the visible Pattern, at some point in time (but not necessarily throughout the process) can be “meaningful,” that is, interpretable by both young and adult students. For example, in the everyday process of wolves hunting a prey, the predominant perceptual Pattern looks like the wolves are following each other chasing the prey more-or-less in a single queue (See Figure 1, frame Time 3). Similarly, we often see ants following one another also in a single queue toward the food source in their process of foraging (See Figure 2, frame 46 min). A single queue is a meaningful Pattern because it is similar to other familiar lines in our environment, such as standing in line to wait for our turn.2 In short, throughout a process, Patterns can emerge for which students can interpret as familiar and meaningful. Other examples of familiar and meaningful dynamic Patterns are: familiar shapes (circle, triangle, etc.), increasing or decreasing size (or volume, intensity, amounts), alternations, wave, and so forth.
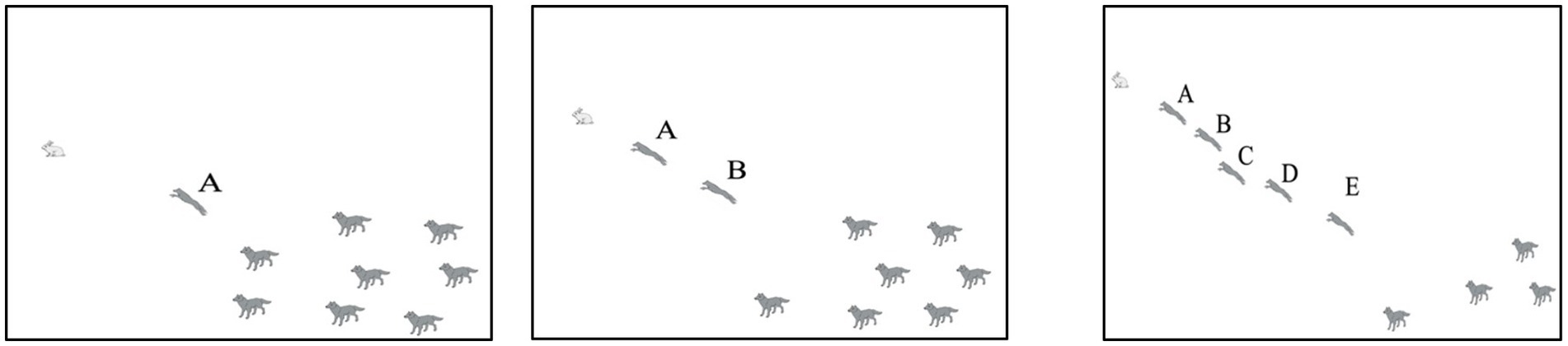
Figure 1. An additive Pattern: Wolves hunting a prey (Images reconfigured from clip art image at https://downloadclipart.org/).
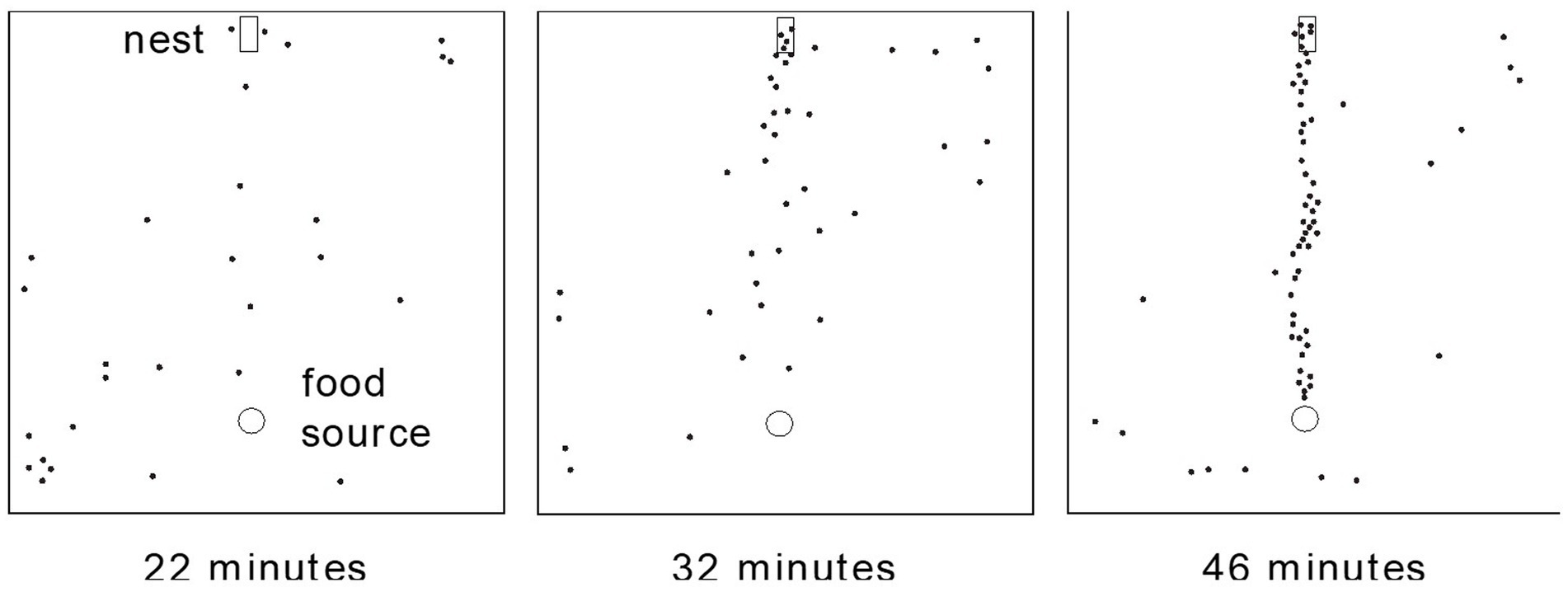
Figure 2. A converging pattern: Ants foraging for food (Adapted from Self-organization in biological systems Camazine et al., (2001), Princeton University Press).
Second, a Pattern can be detected even if it occurs only intermittently throughout a process. For example, ants do not march in a single line early in their foraging processes, but eventually a prominent dynamic and interpretable Pattern of a single line appears as they transport food to their nest (See Figure 2, frames 22 and 32 min.). Third, dynamic Patterns may be audible sound patterns rather than visual patterns (as well as Patterns detectable by other sensory systems); or be invisible such as when they span a long time period. In such cases, students can imagine the patterns with their minds’ eye (Chase and Simon, 1973), such as imagining the Pattern of moths getting darker over centuries even though they cannot directly see the darkening changes, or imagining that the giraffes’ necks getting longer over generations.
A fourth important property of dynamic Patterns of processes is that there are many external conditions or parameters in the environment that can affect the behaviors of the Patterns in terms of its speed, direction, intensity and so forth. For example, in the wolves hunting process, the terrain can affect the speed with which they chasse the prey. Similarly, for ants, rain may dilute the emitted pheromones, taking the foraging process longer to form the Pattern of a single file line. The relationship between the external conditions and the behavior of the Pattern will be termed here the “condition-Pattern relationships.” Note that an external incident (or condition) can also initiate a process, but it is independent of the process itself. For examples, pouring ink into a vessel with water is the initiating event causing the ink diffusing process, or clicking on a light switch initiates the process of electrical current flowing. Both external conditions and incidents can affect and initiate a process, but it does not explain how the Pattern of ink flow appears, which requires an Agent-Pattern explanation rather than a condition-Pattern explanation.
3.1.1. Contrasting features of the pattern dynamics: incremental parts versus converging whole
Although both Emergent and Sequential processes exhibit Patterns, the Patterns are formed in uniquely different ways. One way to describe how the Pattern changes in qualitative terms is that the dynamic Pattern of Sequential processes changes in an “incremental” way, while the Pattern of Emergent processes changes in a “converging” way. Incremental changes mean that the Pattern is created by adding the outcome of one set of interactions to the partial pattern created by an initial set of interactions of the other agents, so that at each instance of time, the Pattern is extended by the part produced from the additional interactions of a subset of agents. A succinct way to describe it is that the Pattern is an additive sum of successive interactions. Moreover, because the Pattern is formed in this successive way, the initial Pattern is often a part of the final Pattern. For example, in chaining a string of beads, the initial pattern of three beads is a part of the intermediate/final pattern of 10 beads; and every subsequent incremental change in the Pattern is an addition of another part of the Pattern. Thus, this change in the Pattern of Sequential processes can be described by the feature “incremental parts.”
This change from incremental parts (of interactions) and the role of the initial Pattern can be seen in the example of wolves chasing a prey and eventually displaying a more-or-less straight line. As shown in Figure 1, the Pattern initially, at Time 1, is of one wolf (let us say Wolf A) chasing the prey. At Time 2, Wolf B sees Wolf A chasing the prey and Wolf B follows slightly behind, displaying a line with two points. But the initial pattern of Wolf A chasing the prey remains a part of the Pattern. In the third instance, Time 3, a subgroup of three other wolves, Wolf C, D, and E, all see Wolf A and B chasing the prey, so they also join the chase, eventually approximating and continuing a more-or-less single-file line chasing after the prey. Thus, the dominant interpretable Pattern seen by students (perhaps on the Discovery Channel) is the Pattern of a more-or-less single-file line of wolves, formed incrementally, wherein the initial partial Pattern of a short line involving a subset of wolves chasing the prey is subsequently extended by some additional Interactions over successive instances of time.
For Emergent processes, on the other hand, the Pattern changes in a wholistic way, involving all the Agents’ Interactions at all instances of time, resulting in a “convergence” or a change in the proportion for a given area. When a Pattern changes in a wholistic converging way, the initial Pattern is neither a part of nor resemble the final Pattern. Instead, the initial Pattern is of the whole/entire interactions of all the Agents. Figure 2 shows an example of ants foraging for food (taken from Camazine et al., 2001). At 22 min of ants searching for food (the first panel), this initial phase shows that all the ants are randomly dispersed and distributed across space and moving around detecting and emitting pheromones. So, the initial Pattern is a distributed set of ants and it does not resemble the final Pattern of a line. Over time, for those ants that detected pheromones, they will follow the ants who emitted them and then emit pheromones themselves. This means that these ants will gravitate toward the location of the final pheromone trail (compare the 22-min panel and the 32-min panel in Figure 2). Other ants who did not detect pheromones will continue to move around randomly, perhaps away from the location of the eventual trail, as shown in the 22-min and 32-min panels of Figure 2. Over time, more of the remaining dispersed ants gravitate toward the trail, with some others still randomly searching, as shown in the 46-min panel. Thus, the Pattern can be qualitatively described as one that gradually appears or emerges from an initially randomly distributed set of all the ants’ interacting that eventually “converges” to a single queue of ants. The dynamic Pattern reflects the locations of all the ants at or within each instance of time.
Note that a “converging” Pattern for Emergent processes reflects the involvement or interactions of all the Agents within an instance of time, whereas an “incremental” Pattern of Sequential processes involves the addition of one or a few interactions between instances of time. Thus, a “converging” Pattern merely seems to appear as a reflection of the collection of all the interactions within each unit of time (see below, Dim II, Agents). In short, one could say that a Pattern for Emergent process is an epiphenomenon.
To summarize: In Dimension I, the dynamic Pattern of Sequential processes was qualitatively described as being formed by “incremental parts” from the additive participation of new agents’ interactions “successively,” and the Pattern of Emergent processes as being formed by “converging whole” from the collective participation of all the agents’ interactions “concurrently,” at each instance of time (See Table 2, Dim I).
3.1.2. Implication
One implication of the way dynamic Pattern for Sequential processes is formed is that the initial Pattern is often a part of the final Pattern. For example, in chaining beads, the initially chained parts will remain a part of the final pattern. However, in the ants converging Pattern of an Emergent process, the initial Pattern of scattered ants are no longer visible in the final Pattern.
3.2. The Agents (A)
The second dimension of processes is the Agents or elements that participate in the Pattern of a process. In the wolves hunting example, the Agents are both the wolves and the prey. In the ants foraging example, the Agents are all the worker ants (but not the Queen ant, since only the worker ants forage for food). We refer to the Agents that are constituents or participants of the Pattern as the internal Agents, whereas external Agents are any other elements in the environment with whom the internal Agents interact but may not appear as visible participants in the visual Pattern. For the example of moth darkening when the trees get sooty from industries in England, the external Agents (e.g., the birds who eat the moths) are not a part of the Pattern. A less obvious example are the water molecules in the case of diffusion of ink molecules. In this case, the water molecules are less visible and obvious compared to the prominence of the ink molecules, so the water molecules can be considered the “external” agents and the ink molecules the “internal” agents.
Identifying both the Pattern and the Agents is important because together, they specify which process one is talking about. For example, if we want to explain the Pattern of how moths get darker over generations, the Agent level to focus on is the moths and the birds, and not the genes. But if we want to explain the Pattern of how the darker color of moths arose, the Agent level to focus on is the genetic level because the allele likely arose due to genetic mutation (Kettlewell, 1955). Thus, it is important for instructional materials to articulate the Pattern and the Agents in order for students to understand which processes are being described and explained. Standard texts often ignore the Pattern and sometimes refer only to the end states, such as the initial light moths and the eventual dark moths.
3.2.1. Contrasting features: an identifiable single individual or a subgroup of individuals versus a collection
Although both Sequential and Emergent processes have Agents participating in the Pattern, there is one important difference between them. The difference is whether “one Individual or a subgroup of Individuals” or Agents can be identified as affecting or are responsible for the behavior of the Pattern. In the Sequential process of airplanes flying in a V-formation, one can easily identify the lead pilot (or along with a co-Pilot) as the captain who tells the other pilots when to get into position. On the other hand, for Emergent process of geese flying in a V-pattern, one could not identify any specific goose as responsible for the V-pattern. All geese merely seek to fly in an area with an updraft created by another goose (an area of least effort), and the Pattern emerges; thus, the responsibility is distributed across a “collection” of all the individuals. However, students do misconceive this process and typically claim that the lead goose (the one at the head of the V-formation) tells the other geese where to fly.
In PAIR-C, we assume that “a single Individual or a subgroup of Agents” can be identified as having centralized control or can directly affect the process. In the pilots flying example, as a Sequential process, it would be correct to attribute control to both the pilot and the co-pilot (if a co-pilot exists), as a subgroup that is responsible for the Pattern of flying in a V-formation. A subgroup is typically a class of Agents (as opposed to a collection, see Markman, 1979), defined by some shared external visible properties. In the case of pilot and co-pilot, they usually wear the same kind of uniform, are seated in the cockpit, and so forth. However, attributing an Emergent process to a subgroup of individuals as having responsibility for the Pattern is incorrect. For example, students often refer only to the ink molecules when explaining how/why a Pattern of ink flow is observed. Thus, in contrast to Levy and Wilensky (2008), who view reasoning about clusters, families, or other forms of subgroups as a mid-level reasoning that progresses toward collective causal thinking, our assumption is that reasoning about subgroups is equally misconceived and is not much improved over reasoning about one individual. (See Table 2, Dim II).
3.2.2. Implications
The implications of the Agent dimension are the most common features expressed in misconceptions. If one or more Agent(s) can be identified as being responsible for the Pattern, then these single or a subgroup of Agents can be inferred to have control of the Pattern. This expressed property of a process, can in fact be true or can just be inferred by the students, as expressed in their explanations. In the complexity literature, this property is referred to as centralized control (Jacobson et al., 2011).
Students often point to the existence of a plausible centralized control for Emergent processes, such as explaining that there is a leader goose telling the other geese where to fly. Attributing a causal agent is consistent with causal explanations commonly given by people that refer to an antecedent agent or event as causal (Lombrozo and Vasilyeva, 2017). However, doing so is only correct for Sequential processes, such as attributing the alpha wolf as being responsible for the single file chasing line because he decides when to initiate the chase and when to attack the prey. But it is incorrect and misconceived when a centralized controlling Agent is attributed to Emergent processes. For example, in ants foraging for food, no one ant’s Interactions can be identified as the source of the eventual queue Pattern, since all the ants merely carry out the same set of local Interactions (sniffing and emitting pheromones), and a queue automatically emerges. Thus, to express an explanation based on an identifiable ant or a subgroup of ants as controlling would be misconceived. Instead, the responsibility of the Pattern is distributed across the entire collection of Agents’ Interactions. Distributed is an implied implication of a “collection” (Table 2, Dim II).
There are many other related implications to the main feature of an identifiable Individual agent with centralized control that students can also infer, such as that the controlling agents have special status, they can directly affect the process, and may be undertaking the interactions intentionally in order to affect the process (these implications are listed in Table 2, Dimension II). For example, a commonly expressed misconceived explanation with centralized control is that the controlling Agent(s) may interact with another agent intentionally with the purpose of achieving the meaningful Pattern (Table 2, Dim IId, left column). Such teleological reasoning is appropriate for Sequential processes. For example, in wolves hunting, the chaser controlling wolves could change the direction of the chase intentionally for the purpose of cornering the prey more quickly. Explanations of intentionality and purposefulness is consistent with cognitive constraints on how human reason naturally but intuitively (Coley and Gelman, 1989). However, not having an identifiable causal Agent in Emergent processes implies that it has decentralized or distributed control (Table 2, Dim IIa, right column) with all Agents’ Interactions having equivalent status (Table 2, Dim IIb, right column), with only local goal of carrying out a set of Interactions with no intention or purpose to affect the Pattern directly or the global goal (Table 2, Dim IIc and IId, right column). Thus, when students’ explanations of Emergent kind of processes appeal to implications that they infer, such as centralized control with intentional purpose or special status, they would be revealing misconceived explanations because these implied features are also not appropriate for describing Emergent processes.
3.3. The agents’ Interactions (I)
The third dimension of processes is the agents’ Interactions, referring to how one Agent interacts with another Agent within the process. For very simple processes such as chaining a bead and diffusion, the Agents can basically interact in only one way. For example, the beads interact by having one added to the chain to occupy the location on the string adjacent to the prior strung bead. For diffusion, the molecules basically interact in one way by colliding with each other or not. However, for both Emergent and Sequential processes, the Agents can interact in more than one way. For example, in the wolves hunting process, some of the Interactions include wolves chasing the prey, an alpha wolf killing the prey, or wolves following another wolf. Similarly, in the ants foraging process, ants interact in multiple ways, such as following one another toward a source of food mediated by detecting pheromones emitted by another ant, and then following the detected pheromones, and emitting their own pheromones for other ants to detect. Interactions also occur between internal and external Agents, such as between the birds who eat moths in the process of moths getting darker. In short, within every process, there may be one or multiple (a set of) Interactions.
References to interactions are often omitted in everyday language. For example, selling a car is an interaction between a buyer and a seller, but we often say, “I bought a car” or “I sold a car,” without mentioning with whom the car is sold. This everyday habit of speech can be unfortunate when discussing natural processes as it overlooks the importance of Interactions when they are inadvertently converted into actions by omitting the agents with whom they interact.
3.3.1. Contrasting features on sub-dimensions: availability and with whom
Although processes differ in many ways in terms of what the Interactions of their Agents are, there are two subdimensions contrasting features for the nature of the Interactions of the Agents in Sequential versus Emergent processes. The first subdimension is availability, that is, whether some Agents have interactions that are “uniquely” available only to them. For Sequential processes, not all the Interactions are available to all the agents; instead, some interactions are “uniquely” available only to some of the Agents. In the wolves hunting process, the Interactions such as wolves chasing-and-cornering-the-prey, are available only to some of the wolves such as the strong male wolves, while the killing-the-prey interaction is reserved for the alpha wolf only. This means that different agents have sets of interactions that are more-or-less “uniquely” available to them.
In contrast, for Emergent processes such as ants foraging for food, every ant can undertake the same or “uniform” set of interactions, namely, detecting, emitting and following pheromone (A set of interactions can contain any number of available interactions.). The same “uniform” set of Interactions refers to the nature of the Interactions, not their identicalness. That is, the uniform set of Interaction may be carried out in different ways. For example, some ants may emit a lot of pheromones while detecting pheromones going north, while other ants may emit a little bit of pheromones while detecting pheromones going west, so that the interactions themselves are not identical even though they are a “uniform set” of Interactions meaning that they (detecting, emitting and following pheromones) are available to all the Agents of a process.
The second subdimension of the Interactions dimension refers to with whom an Agent can interact, that is, whether the Interactions can occur between any two Agents (to be referred to as “random”), or the Interactions of the Agents are “restricted” in terms of with whom an Agent may interact. For example, the alpha wolf’s interaction of killing-the-prey is “restricted” to the prey, thus the alpha wolf is not likely to kill another wolf. For Emergent processes, on the other hand, any Agent can interact with any other Agent, so that their interactions are not restricted, but “random.” In diffusion of ink example, it is not the randomness of a single molecule’s Brownian action (in terms of the random direction it can bounce to) that is critical for explaining the Pattern, but rather, it is the randomness of the Interactions with any other Agents (such as colliding with any other molecule) that is important for explaining how the Pattern of ink flow is formed. (See Table 2, Dim IIIA, IIIB).
3.3.2. Implications
The “restricted other” versus “random other” feature has two common sense implications for what else may be true. One implication is understanding the likelihood that an Interaction between two specific Agents can re-occur. When the interactions in Emergent processes are random as in ants foraging, an Interaction between the same two Agents can re-occur, even repeatedly. For example, one ant may detect and follow a second ant, leave, then follow a third ant, then return to follow the second ant again, and so on. On the other hand, in Sequential processes, when some interactions happen with “restricted other,” then they are unlikely to re-occur. For example, the alpha wolf will kill the prey just once. Another commonsense implication of the “restricted” versus “random” feature is whether or not the Interactions can be bidirectional. That is, the Interactions in Sequential processes tend to be unidirectional, such as the wolves chase the prey, but the prey does not typically chase the wolves, whereas Interactions for Emergent processes tend to be bidirectional. For example, bidirectionality is easily seen in virus spreading, as Person A can infect Person B, and vice versa. (Table 2, Dim III Implications).
3.3.3. Cautions: overlooking and underspecifying the Interactions between Agents
PAIR-C framework emphasizes the role of Interactions between Agents as critical to understanding how the Pattern arises. Here, we point out that caution is needed when Interactions are either overlooked or underspecified. For example, complexity scholars often inadvertently talk about Agents’ actions (see, for example, Table 1 from Jacobson et al., 2011), when in fact it is the Interactions that should be mentioned. Also, standard textbooks also often refer to an Agent’s actions as being random or not, such as that a molecule can bounce into any random location, rather than the Interactions being random in the sense of “with whom” an Agent can interact. Such word slippage in overlooking explicit mentioning of the Interactions can contribute to the persistence of students’ misconceptions even after instruction.
The under-specification of Interactions can also be seen in the distinction between a Collection as a static concept, such as a forest of trees, which is defined by physically shared features such as proximity, versus the dynamic concept of a Collective outcome, such as the spreading of a forest fire, which refers to the sum of all the trees’ Interactions. The blurring of this important distinction is sometimes created by using the terms “centralized” vs. “distributed.” (These terms are shown as implications in Table 2, Dim IIa, because they are frequently used in the literature.) Referring to one or a few Agents as “centralized” seems to refer to the idea that a single or a subgroup of Agents (as in PAIR-C) are responsible, implying that it is the Agents’ actions that are responsible. This implicit reference to individual Agents’ actions as responsible is more-or-less acceptable when it refers to a Sequential process, for which Agents’ actions are often mentioned without referring to the recipients of the actions (i.e., without mentioning it as Agents’ Interactions, see the same point in Section 3.3 above). However, carrying this implicit reference to actions with the term “distributed” is more problematic, because “distributed” tends to imply that the entire collection of Agents is responsible, a more static idea. Instead, “distributed” should mean the summative interactions of the entire collection, at each instance of time, is responsible. Thus, it is important to mention the Interactions to avoid persistent confusion.
3.4. The Relations among the interactions (R)
The fourth dimension of processes in PAIR-C, Relations among the interactions, refers to how one interaction (between two agents) is related to another interaction (between two other agents). Comparing one interaction (or relation) with another interaction is a second-order relation; whereas first order relations are the direct interactions available to two Agents, such as that molecules interact by colliding with each other, or that ants interact by detecting, emitting, and following pheromones.
3.4.1. Contrasting features on sub-dimensions: ordering and contingency
There are two second-order Relations of the interactions observable over time (i.e., the relations between pairs of interactions): ordering and contingency. (Table 2, Dim IVA and IVB). These two second-order relations have distinctly contrasting features for Sequential and Emergent processes. For Sequential processes, various interactions often occur in a “serial order” and with “dependency.” For example, when the alpha wolve can strike to kill the prey depends on the prey having been cornered first; although some interactions (a subset) in the hunting process can occur simultaneously, such as many wolves (a subset) can be chasing the prey at the same time. For Emergent processes, on the other hand, all interactions occur “simultaneously” and “independently,” such as in ants foraging, all the ants can detect, emit and follow at the same time and all ants can detect and emit pheromones independently of other ants’ detecting and emitting.
3.4.2. Heuristics for discrimination
These two sub-dimensions of the Relations of the interactions dimension, ordering and contingency, can be used as heuristics to serve the purpose of discriminating between Sequential and Emergent processes, because these two second-order Relations are often visible. The fact that this dimension can function to discriminate between two kinds of processes has never been articulated in the literature before.
3.5. The Causal agent-pattern mechanisms (C)
This fifth dimension is the most critical to misunderstanding as it refers to the mechanisms of how the Agents cause or produce the Pattern (the C part of the PAIR-C acronym) for Sequential and Emergent processes. As mentioned in Section 2.2, this causal question is particularly puzzling for Emergent processes because the Agents seem to behave or interact in certain ways and the Patterns seem to behave in some other ways, so how do the Agents’ behaviors produce the Pattern? This question seems less puzzling for Sequential processes because the behaviors of the Agents tend to align with the behaviors of the Pattern, whereas there is no alignment for Emergent processes. For example, in the Sequential process of wolves hunting, the Interactions of the Agents consist of individual wolves chasing a prey, but each wolf’s direction and speed is likely to align with the direction and speed of the hunt of the pack of wolves. For Emergent processes, on the other hand, the behavior of the individual Agents (e.g., the individual ants sniffing and emitting pheromones) does not align with the behavior of the Pattern. That is, individual ants can be sniffing and emitting pheromones while heading north, whereas a queue that is being formed is heading east toward the food source, so how is the Pattern produced? The question of how the perceptual Pattern is produced or caused by the Interactions of the Agents is addressed mostly for Emergent processes because the causal mechanisms are not obvious and the Pattern is not predictable, but in fact the same causal question can be asked of Sequential processes as well.
The term “level” is often used in the science texts because Agents for some processes are often invisible (such as molecules of liquid) and often smaller in scale as if they are at a lower level, so that the Agents are sometimes referred to as the “micro or lower level” and the Pattern as the “macro or higher level.” Scholars in the complexity literature concerning the learnability issue also use the term “levels” (Wilensky and Resnick, 1999). However, Agent-Pattern is a broader and perhaps less confusing term than levels for many reasons. First, for many processes, the Agents are often quite visible and large, therefore not appropriate to think of as them as the “micro” level. Second, micro–macro implies there are only two levels when in fact, there are multiple levels, depending on at which level a process is being described. For example, if the to-be-explained process is how giraffes’ necks get longer over generations, the Agent level are the giraffes. But if the to-be-explained process is how a specific giraffe got the trait of a very long neck, then the Agent level is the genes (Another example was given in Section 3.2, about explaining the Pattern of moths getting darker versus explaining the Pattern of how a darker moth is born). Third, pointing out multiple levels is important (but not to label a specific level as micro or macro) in order to avoid reasoning that skip a level, such as reasoning about the level of genes to explain the level of moths getting darker over generations. This is why it is important to be able to identify the Agents properly.
3.5.1. Contrasting mechanisms: cumulative summing versus collective summing
In Dimension I, the dynamic Pattern of Sequential processes was qualitatively described as being formed by “incremental parts” from the additive participation of the subsequent Agents’ Interactions “successively,” and the Pattern of Emergent processes as being formed by “converging whole” from the collective participation of all the agents’ interactions at each instance of time, “concurrently.” Below, we explicate the mechanisms (and examples of computation) of these two Agent-Pattern Causal relations, to be referred to here as: “cumulative summing3” and “collective summing,” for Sequential and Emergent processes, respectively. These two kinds of mechanisms account for the Pattern of either incremental parts or converging whole.
3.5.1.1. Cumulative summing
Sequential processes can display various “incremental” Patterns, such as changing size over time (e.g., a pile of leaves getting bigger and bigger as a greater number of leaves fall on the pile), changing length (e.g., the bead necklace getting longer and longer), or changing quantities, shape, size, and so forth. These incremental Patterns can be computed in various ways, such as by adding (or subtracting) a new amount to a prior existing amount; or chaining a bead necklace as described earlier, such that at each instance of time, a new bead is added to the existing partial necklace; or clicking a tally counter to add a new amount to the existing total amount. Other more complicated computations such as multiplying, adding exponentially, adding by expanding in size in all directions, etc., can also be responsible for incremental changes.
The cumulative summing mechanism accounting the incremental change in the Pattern of Sequential processes is computed by adding the Interactions from one “individual” or a subgroup of Individual Agents at one point in time to the totals of the Pattern already produced from the Interactions occurring at prior instances of time, similar to counting items by clicking a counter to add to the current total amount. This “cumulative summing” mechanism can be characterized as occurring across or between instances of time in that the Pattern accumulates or extends (such as the Pattern of a line getting longer and longer) as some new Interactions from a subsequent time period are added to the existing pattern from prior Interactions of prior time periods. In short, the two key features of cumulative summing is that the Pattern reflects the Interactions of Individual Agent(s) summing across successive interactions over time.
Thus, the perceptual Pattern of Sequential processes results from the accumulation of each successive Interactions that occur over time; and accumulates in the sense that it increases or decreases incrementally from Time N to Time N + 1, by the specific Interactions that occur between units of time. Ways of computing cumulative summing is described in the first paragraph of this Section 3.5.1.1 (also see Table 2, Dim V.)
3.5.1.2. Collective summing
The “converging” Pattern of Emergent processes can be produced by a “collective summing” mechanism, in which all the Interactions within each instance of time are summed, and the displayed Pattern merely reflects the amount summed at each instance of time. Thus, in contrast to a successive addition of the Interactions of an “Individual” Agent or a subgroup of individual Agents in the case of “cumulative summing” across time; for “collective summing,” the two key features are that the Pattern reflects the Interactions of all or the entire collection of Agents, summed within each unit of time. The dynamic converging Pattern is merely the display of the net sum of the entire collection of Interactions at each instance in time, so that there is no relationship between the net sum at one time and the net sum at a subsequent time. The observable Pattern is merely an interpretation imposed by the perceiver, thus, an epiphenomenon. A common example is the Pattern one might notice of the stock market going up over 5 days. This pattern of increasing market performance results from the total market index (or sums of all the buys and sells) from each day, with little relationship between the index of one day versus another day. The increasing trend is merely a familiar and meaningful pattern imposed by the observer. Thus, the key features of “collective summing” is that the summing of all the Interactions occurring within each unit of time, and the dynamic Pattern merely reflects what the collective net sum shows at each point in time.
A variety of computational methods that can be used to sum collectively can be considered. The simplest method to compute “collective summing” might be adding positive and negative numbers at each instance of time. Another computing mechanism is taking the average of the magnitudes and directions at each instance of time (i.e., net effect of vectors). Using the ants’ Pattern of convergence as shown in Figure 2, suppose initially the ants are randomly spread out over a small area, as shown at Time 22 min. Suppose we draw an imaginary predicted line of where the final queue of ants will be. We can compute the average distance and direction of all the ants from this imaginary predicted line (i.e., consider each ant’s path as a vector). Over time (from Time 22 min to time 32 min), the average distance of all the ants should get shorter and converge in direction toward the main trail of pheromones, and finally marching on it only, creating the final image of ants marching to and from the source of food in a single file. This means that the Pattern of appearing or emerging is produced by averaging all the ants’ distances (magnitude) and directions (toward and away from the final Pattern) at each unit of time. Thus, dynamic Patterns of Emergent processes reflect the changes in the resulting averaged quantities (or proportion, or the vector sums of magnitudes and directions, or the net effects), for each unit of time.
3.5.2. Implication: align or not align
The difference in the mechanisms of cumulative summing and collective summing implies one important implication with distinct features for Sequential and Emergent processes: the implication of aligning or not aligning between the Pattern and the Agents. For Sequential processes, the Agents’ behaviors more-or-less align with the behavior of the Pattern, whereas for Emergent processes, there is no alignment.
Alignment or non-alignment does not mean exact match; instead, it means roughly proportional. There are many ways that alignment between the behavior of the Agents and the behavior of the Pattern can be manifested (see the implication of Dim V, Table 2). One of the ways behaviors at the two levels can align is in terms of movement. For Sequential processes, movement or interactions of the Agents tend to align with the behavior of the Pattern. For example, in wolves hunting, when the hunt is over (when the Pattern of a line of running wolves chasing after a prey is no longer apparent), that means the individual wolves are also no longer chasing the prey. Thus, when the Pattern of the hunt stops, then the Agents also stop chasing. However, there is no alignment for Emergent processes. Even when the ants are no longer forming a single queue toward a food source, the individual ants continue to move around searching for food. This lack of alignment is often expressed in misconceptions when students assume for example, that in diffusion of ink molecules, when the visible ink flow Pattern stops (when it has reached equilibrium), the individual ink and water molecules also stop colliding with each other.
There are many other ways that Agents’ Interactions and the Pattern can align or not, such as whether the Agents interactions tend to go in the same direction as the direction of the Pattern, or whether the magnitudes of the individual Agents’ interactions is proportional or not to the Pattern with respect to the speed, or the intensity, the complexity/simplicity of the rules, and so forth. In wolves hunting for example, alignment can be seen in the speed, such as the faster the wolves chase, the sooner they catch the prey. But for Emergent processes, small changes in magnitude can often cause large effect at the Pattern level, referred to in the complexity literature as the “butterfly effect.” Overall, for Sequential processes, the Interactions at the Agents’ level align with the behavior of the Pattern, but not for Emergent processes.
In summary, the contrasting features of the five dimensions of Emergent and Sequential causal structures of PAIR-C are described above and shown in Table 2. Moreover, each feature can also implicate other related features.
4. How PAIR-C was constructed
PAIR-C and its features were not constructed by analyzing the properties of actual science processes. Instead, the construction of PAIR-C was based on the assumptions derived from interpreting the vast amount of misconception data, as described in Section 2.0. The causal structure for Sequential processes was derived by capturing the features students expressed when they explain the causality of Emergent processes. That is, because their explanations for Emergent processes are misconceived, we assumed that the features expressed in the explanations must be features of the Individualistic causal structure for Sequential processes. For example, in Table 1, the first response “Because they need the stripes for camouflage purpose” could suggest the implied feature of intention or purpose. (see Dim IId, Table 2.) The second response “Because the queen ants tell them where to go,” could suggest the feature of an identifiable “Individual or a group of Individuals” with centralized control. (see Dim IIa). The third example in Table 1, the misconceived explanation states that “chemical reaction stop at equilibrium.” This suggested the feature of alignment or proportionality between the behavior of the reaction at the molecular level and the termination of visible movement at equilibrium (see Dim V, the implication of alignment). The response in example 4 in Table 1 illustrates the misconception of thinking of the flow as moving ink particles. That is, it attributes the flow to a subset of “Individuals,” the ink particles. (see Dim II.) The same reasoning is shown in Example 5: only the subgroup of hot molecules are moving or escaping. (see Dim II again.) Finally, Example 6 in Table 1 shows a failure to consider collective summing of all the vectors acting on the bomb, and not just the force of gravity. (See Dim V collective summing mechanism.) In short, this is how the misconceived explanations were coded.
Because these captured features were used inappropriately to explain Emergent processes, therefore, they must be features relevant to Sequential processes since they make no sense for explanations of Emergent processes. Then, based on our analyses of various Emergent processes, it appears that the features needed to generate explanations for Emergent processes are diametrically opposite of the ones actually expressed. That is, we analyzed several dozen pairs of processes, one Sequential and one Emergent, paired on the basis of various superficial similarities, such as both processes of the pairs exhibiting relatively the same Pattern, or same function, and so forth. For example, one pair might be having the same goal of seeking food and exhibiting a more-or-less queue-like line, such as the process involved in wolves hunting and ants gathering for food, and both processes exhibit a queue-like Pattern; or pairs that were building a structure (such as termites building a mount versus people building a skyscraper); or pairs of different ways of passing news (such as distributing news through a telephone tree versus spreading of rumors). See Appendix A for a small sample of the process pairs that we analyzed.
Thus, our analyses consisted of comparing/contrasting students’ expressed (when available and accessible in the literature) explanations for a Sequential process (such as wolves hunting), with the actual misconceived explanations for an Emergent process of the same pair (such as ants foraging), to arrive at a plausible feature for the Collective causal structure. For example, if an explanation for the ants (an Emergent process) mentions the role of the Queen ant in telling the other ants where to go, this would suggest the feature of an identifiable Agent with centralized control for a Sequential process. We then conjecture that the opposite feature may be true for the ants foraging process, that there is no identifiable controlling agent. We then confirm this conjectured feature by comparing this feature with the actual scientific explanation for how ants form a line to the food. This is how the features of the causal structures were derived for both the Individalistic and the Collective causal structures.
Following the identification of all the relevant features and their opposites, they were then organized into a more coherent and meaningful set relevant to five dimensions, besides the dimension of time, with some features organized as implications of other main features. For example, having an identifiable Individual Agent as responsible for a process’ Pattern seems to imply that the identified Agent has centralized control, as well as more important status, has intentions, and can directly affect the Pattern. That is, one feature can generate a number of implications. Organizing some expressed features as implications allow for the variability seen in the expressed misconceived explanations (Note that a few implications that have not been mentioned by students were also generated).
In short, the features for the Individualistic causal knowledge structure were ones that were actually articulated by students, but in a mistaken way, as they were expressed for explanations of Emergent processes. Whereas the features for the Collective causal knowledge structure were derived from the Individualistic causal structure, by considering what the opposite feature might be, by contrasting matched pairs of processes (see Appendix A) as well as by understanding the actual explanation of how the Emergent processes of each pair should be explained. Then features were organized into dimensions common to both kinds of causal structures. The causal structure underlying Sequential processes is referred to as Individualistic because one or a subgroup of individuals whose interactions can be identified as being responsible for the process, whereas the causal structure underlying Emergent processes is referred to as Collective because the Interactions of the entire collection of all the Agents’ interactions are responsible. Thus, this is how the framework was constructed.
5. Discussion
In this discussion, a brief explanation of the three questions posed in the introduction is provide. Then, a few remaining issues relevant to situating PAIR-C in the context of related literature are addressed. The section concludes by briefly mentioning how PAIR-C contributes to the broader literature.
5.1. Answers to the three initial questions
Having described PAIR-C, the three questions raised in the introduction can now be explained. First, misconceptions are expressed for numerous Emergent science processes because they are all generated incorrectly from the same familiar Individualistic causal structure, instead of the appropriate Collective causal structure. Second, students seem to generate explanations readily and easily for Emergent processes based on features of the Individualistic causal structure, unaware that their explanations are incorrect, suggesting that they may lack any knowledge of the Collective causal structure. Lacking knowledge of the Collective causal structure is consistent with the observation that students also seem to be fickle in having no reservations in offering a revised explanation when challenged, but the revised explanation continues to be misconceived because it is based on another feature from the same inappropriate Individualistic causal structure. Third, students resist concept-specific kind of remediation approaches (see Section 2.5) because new instructional ideas continue to be assimilated to and interpreted by the same Individualistic causal structure. They cannot assimilate them to the Collective causal structure because they have no knowledge of it. That student can assimilate concept-specific, piecemeal instruction to their Individualistic causal structures suggests that the Collective causal structure itself should be taught as a coherent whole, to be explained in the next Section 5.2.
5.2. A concept-specific instructional approach versus a concept-general approach
As briefly described in Section 2.5 above, several ways of remediating misconception through instruction have been tried, using pedagogical tactics such as inducing cognitive conflict, refuting or confronting students’ ideas and predictions, and bridging approaches. We characterized these tactics as a “concept-specific” approach because the remediation is targeted at directly contradicting, denying, or confronting the specific incorrect statement of a specific concept. Given that PAIR-C explains the reasons for the appearance and persistence of misconceptions as arising from lacking knowledge of the Collective causal structure, this suggests an alternative “concept-general” remediation approach of teaching students about the Collective causal structure itself (its dimensions, features and implications), followed by instantiating a specific concept within this newly taught structure. The idea is that students need the availability of the appropriate general structure first, with which they can then assimilate and interpret new instructional information. Such a concept-general instruction approach has shown promise at improving middle school students’ understanding of the process of diffusion (Chi et al., 2012), as well as the process of natural selection for college students (Su et al., 2023). Appendix B also gives an example of how explanation of natural selection from a popular early high school module can be improved, based on PAIR-C.
5.3. Differences between PAIR-C’s instruction and the direct-teaching-of-complexity literature
There is an emerging literature on directly teaching students to understand complex systems per se, such as understanding how climate change occurs (Jacobson et al., 2017). Complex dynamic systems are often defined as a system composed of many elements that interact with emergent large-scale properties, such as a tsunami (Bar-Yam, 1997; Mitchell, 2009). The term “‘emergence’ is used to describe how large-scale patterns arise from the multiple interactions of individuals; and the resultant patterns are called emergent phenomena.” (Wilensky and Jacobson, 2014, p. 319). Note that in such a definition, the term “emergence” does not actually spell out the mechanism by which the large-scale pattern arises. This instructional interest stems from the question of whether students can understand complex systems per se, “given the well documented difficulties students have with learning conventional science subjects” (Jacobson et al., 2011, p. 764). For example, Yoon et al. (2017) recently reviewed 75 studies on learning complex systems. In short, this emerging set of scholarly work does not directly address the issues of misconceptions, as does PAIR-C, but rather, its central research question concerns the “learnability” of new scientific ideas about complex systems by K-16 students. Thus, it takes a concept-specific approach. Below, we compare and contrast four differences between the two strands of research: the “misconceptions” strand and the “learnability” strand.
5.3.1. Difference in the goals
The main goal of PAIR-C is to design instruction that can remove misconceptions or at least not allowing misconceptions to hinder correct understanding of a variety of conventional science concepts taught in school curricula, whereas complexity educators are interested in teaching complex concepts and phenomena per se, such as the desert ecosystem (Basu et al., 2015) or the process of spreading (Grotzer et al., 2017). In purely instructional terms, the goal of PAIR-C is to achieve transfer, in the hope that understanding Collective causal structure may enhance students’ understanding of many Emergent processes. In contrast, the goal of the “learnability” strand is helping students learn the specifically taught complex systems concept.
5.3.2. Difference in the choice of emergent processes to analyze
As explained in Section 4.0, PAIR-C was derived by inferring from students’ misconceived explanations of Emergent process taught in standard school curricula. The “learnability” community focuses on a much wider set of emergent processes commonly referred to as complex dynamic systems. One possible difference is that school-taught kind of Emergent processes are more deterministic, by which we mean that the Pattern can be predicted with known variables. For example, the speed of diffusion flow (the Pattern) is governed by Fick’s Law, which says that the rate of diffusion through the material (i.e., the Pattern of flow) depends on the difference in concentration between the two ends of the material, and so forth. In contrast, the complex dynamic processes analyzed in the wider literature often have Patterns that behave unpredictably with many unknown variables that may affect the Patterns. Nevertheless, given that Emergent process features are similar to complex dynamic processes (see Section 5.3.4 below), it may be safe to say that Emergent processes may be a subset (or a “narrower form of emergence” c.f., Grotzer et al., 2017, p. 58) of complex dynamic processes.
5.3.3. Difference in the actual causal structure underlying the science processes versus the cognitive causal knowledge structures underlying the source of students’ understanding
Most work on teaching complex systems has examined the actual scientific accounts of the phenomena in order to adduce physical features or structures to be taught. The goal of our work is to specify what are the possible cognitive knowledge structures that students rely on for generating explanations of science processes. These cognitive structures should correspond in some ways to the actual physical features governing the behaviors of science processes, such as whether or not there exists an identifiable Agent who controls the process. These physical features may form a coherent structure if a process with one feature also has the behavior of another feature. However, the two mappings are unlikely to be perfect. That is, the cognitive structures might be more simplistic, perhaps containing just a few core features, than the actual physical features identified by scientists. The next Section 5.3.4 gives one comparison between PAIR-C’s features and a proposed set of features for complex systems.
5.3.4. Mapping of the identified features between PAIR-C and complex systems
As described in Section 4.0 above, the features of PAIR-C were derived by inferring and coding the opposites of students’ expressed misconceptions for Emergent processes. In short, the features were derived by induction. In contrast, research in the “learnability” strand identified the features of complex processes by analyzing the actual physical features of complex processes themselves. Thus, these two methods are quite distinct. The following illustrates a mapping between the features of PAIR-C and the features of complex systems taken from Jacobson et al. (2011), who have also characterized complex system with a set of five “properties” (listed in Table 1, Jacobson et al., 2011). Their five properties do not exactly map onto the features of the five dimensions of PAIR-C’s Collective structure, but they do map to the implications, as described below.
The first important property mentioned in Jacobson et al. (2011) is “decentralized control or order,” meaning that the behavior at the system level of a process is not organized or caused by a central controlling entity. This property is focused upon by many scholars, such as the work of Resnick (1997) and Wilensky and Resnick (1999); this property corresponds to the implication of PAIR-C’s main feature of the Agents (Dimension IIa, Table 2, which is whether an identifiable Individual Agent is responsible for the process).
The second important property mentioned in Jacobson et al. (2011) is that actions are non-linear (or Action effects), also referred to as the “butterfly effect.” What this means is that the actions of the agents are not proportional to the large-scale behavior, such that a small action can result in big effects. This property again, is represented in PAIR-C as an implication of the Agent-Pattern Causal Dimension V, in terms of whether the behavior of the Agent and the Pattern align in terms of “magnitude.”
The third property mentioned in Jacobson et al. (2011), multiple agents, meaning that the large-scale behavior is caused by multiple (vs. a single) agents, corresponds to the “collection” feature of PAIR-C’s Agents Dimension II. The fourth property is that agents’ interactions are random (vs. predictable). This means that the agents’ interactions are not predictable and occur by chance, and this corresponds to the “with whom” subdimension of the Interaction Dimension III. Finally, the process itself is more like an equilibration process of continuous adjustment (Chi et al., 1994), and not as an event with a beginning-and-an-end (like a closed system). This property can be mapped to PAIR-C’s description of the Pattern as a “converging whole” rather than “incremental parts” (See Dim I, Table 2).
In short, although the five properties identified for complex systems map to many of PAIR-C’s implications and some of the features, the correspondence is not perfect. Most notably, the main features of each dimension are not systematically mentioned, nor are the causal mechanisms. Nevertheless, one could say that the features and implications captured by PAIR-C for the Collective causal structure are similar to the ones captured by complexity theorists for complex phenomena such as climate change. In this sense, the two sets of features/properties do converge. The convergence of the two sets of features/properties is interesting given that the features of Collective causal structure were derived in a quite different way than the way the properties were identified for complex processes, as described above and in Section 4.0. The convergence of the two sets of features/properties gives credence to the indirect PAIR-C method of capturing the features by relying on students’ expressed incorrect misconceptions as an indication of the absence of mentioning the correct features for Emergent processes.
5.4. Resolving a puzzling critique: ontological commitment
Pair-C is essentially a representation of two ontological causal structures, distinct in the sense that a Sequential process can be explained by any feature of the Individualistic causal structure but cannot be explained by any feature from the Collective causality, and vice versa. This means that once a process is identified or categorized with one causal ontology, then a cascade of other features within that same causal ontology applies, while no feature from the alternative causal ontology applies. “Ontological commitment” means that once categorized with a given ontology, students are not likely to shift to another ontology. Such ontological commitment applies to the robustness of students’ misconceptions, in that, once an Emergent process is misconceived as a kind of Individualistic causal ontology, then it is not likely to change to the alternative Collective causal ontology. This “locked-in” view has been challenged by Gupta et al. (2010), who claim that experts and novices can cross ontological categories flexibly, suggesting that people may not be committed to a specific ontological causal structure. However, this critique was an incorrect interpretation of the earlier versions of PAIR-C, as explained by Slotta (2011) and elaborated below.
The “locked-in or committed” view attributed to PAIR-C has three assertions that: (a) students cannot shift between ontological categories or structures flexibly, (b) that once students do shift, the original ontology is no longer maintained, and (c) that ontological knowledge structures are static. Each of these assertions is clarified below, using Individual and Collective causal structures as examples.
With respect to the first assertion, in PAIR-C’s view, having parallel stable core structures does not imply that students cannot cross or shift between them flexibly. Instead, PAIR-C’s assertion is merely that students resist “unlocking” their ontological commitment and continue to retain their misconceptions because students are either (a) not aware that they are generating explanations from an inappropriate causal knowledge structure and/or that (b) they may lack any knowledge of the appropriate alternative Collective causal knowledge. In both cases, their resistance to instructional shift seems “locked-in,” since the concept-specific approach of the prior instructional remediation attempts in the literature have never made students aware that an alternative causal structure is needed for interpreting the taught processes (see Section 2.5). If students have learned and understood the Collective causal structure, then presumably they can then change their misconceived explanations to conform to their Collective causal structure, as obviously must be the case for experts or advanced students. They may initially require some help to alert them (i.e., make them aware) to the need to change, such as pointing out the relevant conditions (such as the discriminating Relations of the Interactions, Section 3.4.2).
A concrete example may illustrate our point. For example, young children often mistake a whale as a Fish (that is, they categorize whales into the Fish category). However, once told, they can shift easily from categorizing whale as a Fish to categorizing it as a Mammal, then allowing whales to inherit the cascade of features of Mammals (Chi, 1997). In other words, their initial misconception of whales is flexible. This is because they have some knowledge of the category Mammals. Thus, our assumption is that for misconceptions, the commitment to the Individualistic causal structure is due to students’ lack of knowledge of the appropriate alternative causal structure; moreover, students may not even be aware that they lack knowledge of the Collective causal structure. The same point can be made for experts and novice physicists. As shown in Chi et al. (1981), experts have schemas for deep understanding of common problems whereas novice students tend to have schemas for shallower understanding based on superficial surface features. However, experts still have knowledge of the superficial features and can fluidly reason about both the superficial features and deep principles as needed to solve a problem. However, novices often lack knowledge of the deep structure of a problem (or their schema of problems are not organized by deep principles); therefore, it appears as if novices cannot move across schemas readily.
The second assertion attributed to PAIR-C was that once students shifted their commitment to the alternative Collective causal structure, then their original Individualistic causal structure is no longer maintained. This is obviously not true as the Individualistic causal structure is needed to understand many Sequential processes. Moreover, because the Collective causal structure is ontologically distinct, instruction must build a separate knowledge structure, perhaps through the method of contrasts (Grotzer et al., 2017; Chase et al., 2019).
The third assertion attributed to PAIR-C is that ontological structures are static. It is “static” in the sense that one structure should not be transformed into an alternative structure. For example, the Individualistic causal structure should not be transformed into the Collective structure as both are needed. However, it is not “static” in the sense that the content of every cognitive structure can be changed, such as making it more elaborated with new information, adding more examples, and so forth.
5.5. The contributions of PAIR-C to the literature at large
Taking a broader perspective, this work makes several contributions to the literature at large. First, it explores how students understand process-concepts (which is under-studied) instead of entity-concepts.
Second, most importantly, PAIR-C provides a way to represent processes in terms of the five dimensions: the Pattern, the Agents, the agent’s Interactions, Relations among the interactions, and the Causal mechanisms that relate the agents’ Interactions to the Pattern, along with implications of these dimensions as inferred by students and expressed in their explanations. In the literature, both complex systems and decomposable systems have been represented by the structure, function, and behavior framework (Hmelo and Pfeffer, 2004; Goel et al., 2009; Danish, 2014). Thus, PAIR-C provides a novel representational framework for processes. In effect, PAIR-C can be conceived of as a plausible definition for the ontology of processes, besides the dimension of time.
Third, PAIR-C raises a question of why knowledge of Individualistic causality is ubiquitous and knowledge of Collective causality is rare. One hypothesis is that people have an innate pre-disposition toward the Individualistic causality. This innate predisposition idea is related to the concept of essentialism (Gelman, 2003). Essentialism posits that even young students have an intuitive correct understanding of the ontological boundary between animate and inanimate entities, such as children assuming that a teapot cannot be changed into a skunk even if one puts a tail-like blob on the teapot. Essentialism would hypothesize that people have an intuitive understanding only for Sequential processes and its underlying Cumulative causality, but not for Emergent processes because they entail Collective causality. Working against such an innate predisposition is another explanation for why Emergent processes are challenging for students to understand.
Finally, even though Emergent processes may be a “narrower” subset of complex dynamic processes, one could generalize from PAIR-C and suggest that reasoning from the Individualistic causal structure is a form of linear thinking, whereas reasoning from the Collective causal structure is a form of systems thinking.
Data availability statement
The original contributions presented in the study are included in the article/supplementary material, further inquiries can be directed to the corresponding author.
Author contributions
The author confirms being the sole contributor of this work and has approved it for publication.
Funding
This work is supported by a grant from the U.S. Department of Education, Institute of Educational Sciences R305A150336.
Acknowledgments
The author is grateful for contributions from Polly Lai, Na Li, John Cho, Man Su, and Brandon VanBibber. Comments from Kurt VanLehn and Ny Vasil (on an earlier draft) were particularly insightful and appreciated.
Conflict of interest
The author declares that the research was conducted in the absence of any commercial or financial relationship that could be construed as a potential conflict of interest.
Publisher’s note
All claims expressed in this article are solely those of the authors and do not necessarily represent those of their affiliated organizations, or those of the publisher, the editors and the reviewers. Any product that may be evaluated in this article, or claim that may be made by its manufacturer, is not guaranteed or endorsed by the publisher.
Supplementary material
The Supplementary material for this article can be found online at: https://www.frontiersin.org/articles/10.3389/feduc.2023.1198362/full#supplementary-material
Footnotes
1. ^We use the term “cumulative summing” for the causal mechanism that produces a Pattern for Sequential process because the verb “accumulates” suggests gradual gathering; whereas the Pattern of Emergent processes is formed by a collective mechanism, referring to a mechanism that sums the Interactions of an entire collection at any given time. Unfortunately, Blikstein et al. (2006), p. 1, referred to Emergent Patterns as “cumulative ‘aggregate’ pattern” or behaviors.
2. ^Although there are a variety of foraging Patterns, this paper will oversimplify and talk only about the aspect of the Pattern that looks like a single line.
3. ^Our former term “additive” summing is changed to “cumulative” summing because both the “cumulative” and “collective” summing are additive.
References
Basu, S., Sengupta, P., and Biswas, G. (2015). A Scaffolding Framework to Support Learning of Emergent Phenomena Using Multi-Agent-Based Simulation Environments in Res. Sci. Educ. 45, 293–324. doi: 10.1007/s11165-014-9424-z
Blikstein, P., Abrahamson, D., and Wilensky, U. (2006). “Minsky, mind, and models: juxtaposing agent-based computer simulations and clinical-interview data as a methodology for investigating cognitive-developmental theory” in Paper presented at the annual meeting of the Jean Piaget society, June 2006 (Baltimore, MD)
Bousquet, A. (2011). Complexity theory and the war on terror: understanding the self-organising dynamics of leaderless Jihad. J. Int. Relat. Dev., 15, 345–369.
Brandts, J., Busom, I., Lopez-Mayan, C., and Panades, JU. (2021). Dispelling misconceptions about economics. Barcelona GSE Working Paper Series, n 1096.
Camazine, S., Deneubourg, J. L., Franks, N. R., Sneyd, J., Bonabeau, E., and Theraulaz, G. (2001). Self-organization in biological systems. Princeton, New Jersey: Princeton University Press.
Catley, K. M., and Novick, L. R. (2008). Seeing the wood for the trees: an analysis of evolutionary diagrams in biology textbooks. BioScience 58, 976–987. doi: 10.1641/B581011
Champagne, A., Gunstone, R. F., and Klopfer, L. E. (1985). “Effective changes in cognitive structures among physics students” in Cognitive structures and conceptual change. eds. L. H. T. West and A. L. Pines (New York: Academic Press), 163–187.
Chao, J., Feldon, D. F., and Cohoon, J. P. (2018). Dynamic mental model construction: a knowledge in pieces-based explanaton for computing students’ erratic performance on recursion. J. Learn. Sci. 27, 431–473. doi: 10.1080/10508406.2017.1392309
Chase, C. C., Malkiewich, L., and Kumar, A. S. (2019). Learning to notice science concepts in engineering activities and transfer situations. Sci. Educ. 103, 440–471. doi: 10.1002/sce.21496
Chase, W. G., and Simon, H. A. (1973). Perception in chess. Cogn. Psychol. 4, 461–494. doi: 10.1016/B978-1-4832-1446-7.50041-8
Chi, M. T. H. (1992). “Conceptual change within and across ontological categories: examples from learning and discovery in science” in Cognitive models of science: Minnesota studies in the philosophy of science. ed. R. Giere (Minneapolis, MN: University of Minnesota Press), 129–186.
Chi, M. T. H. (1997). “Creativity: shifting across ontological categories flexibly” in Conceptual structures and processes: emergence, discovery and change. eds. T. B. Ward, S. M. Smith, and J. Vaid (Washington, DC: American Psychological Association), 209–234.
Chi, M. T. H. (2005). Common sense conceptions of emergent processes: why some misconceptions are robust. J. Learn. Sci. 14, 161–199. doi: 10.1207/s15327809jls1402_1
Chi, M. T. H. (2013). “Two kinds and four sub-types of misconceived knowledge, ways to change it and the learning outcomes” in International handbook of research on conceptual change. 2nd ed (New York, NY: Routledge Press), 49–70.
Chi, M. T. H., Feltovich, P., and Glaser, R. (1981). Categorization and representation of physics problems by experts and novices. Cogn. Sci. 5, 121–152. doi: 10.1207/s15516709cog0502_2
Chi, M. T. H., Roscoe, R., Slotta, J., Roy, M., and Chase, M. (2012). Misconceived causal explanations for emergent processes. Cogn. Sci. 36, 1–61. doi: 10.1111/j.1551-6709.2011.01207.x
Chi, M. T. H., Slotta, J. D., and de Leeuw, N. (1994). From things to processes: a theory of conceptual change for learning science concepts. Learn. Instr. 4, 27–43. doi: 10.1016/0364-0213(94)90016-7
Clement, J., Brown, O., and Zietsman, A. (1989). Not all preconceptions are misconceptions: finding anchoring conceptions for grounding instruction on students’ intuitions. Int. J. Sci. Educ. 11, 554–565. doi: 10.1080/0950069890110507
Coley, J. D., and Gelman, S. A. (1989). The effects of object orientation and object type on children’s interpretation of the word big. Child Dev. 60, 372–380. Available at: https://www.jstor.org/stable/1130983
Confrey, J. (1990). “A review of the research on student conceptions in mathematics, science and programming” in Review of research in education. ed. C. B. Cazdan, vol. 16 (Washington, DC: AERA), 3–56.
Cosgrove, M., and Osborne, R. (1985). “Lesson frameworks for changing children’s ideas” in Learning in science: the implications of children’s science. eds. R. Osborne and P. S. Freyberg (Portsmouth, NH: Heinemann Educational Books), 101–111.
Danish, J. A. (2014). Applying an activity theory lens to designing instruction for learning about the structure, behavior, and function of a honeybee system. J. Learn. Sci. 23, 100–148. doi: 10.1080/10508406.2013.856793
Dauer, J., and Dauer, J. (2016). A framework for understanding the characteristics of complexity in biology. Int. J. STEM Educ. 3, 1–8. doi: 10.1186/s40594-016-0047-y
Dennett, D. C. (1995). Darwin’s dangerous idea: evolution and the meanings of life. New York, NY: Simon & Schuster.
Dickes, A. C., Sengupta, P., Farris, A. V., and Basu, S. (2016). Development of mechanistic reasoning and multilevel explanation of ecology in third grade using agent-based models. Sci. Educ. 100, 734–776. doi: 10.1002/sce.21217
Disessa, A. (1988). “Knowledge in pieces” in Constructivism in the computer age. eds. G. Forman and P. Pufal (Hillsdale, NJ: Lawrence Erlbaum Associates, Inc.), 49–70.
Elsawah, S., Ho, A. T. L., and Ryan, M. J. (2021). Teaching systems thinking in higher education. INFORMS Trans. Educ. 22, 66–102. doi: 10.1287/ited.2021.0248
Erickson, G. L. (1979). Children’s conception of heat and temperature. Sci. Educ. 63, 221–230. doi: 10.1002/sce.3730630210
Gelman, S. A. (2003). The essential child: origins of essentialism in everyday thought. Oxford Series in Cognitive Development. Oxford, England: Oxford University Press.
Goel, A. K., Rugaber, S., and Vattam, S. (2009). Structure, behavior, and function of complex systems: the structure, behavior, and function modeling language. Ai Edam 23, 23–35. doi: 10.1017/S0890060409000080
Gregory, T. R. (2009). Understanding natural selection: essential concepts and common misconceptions. Evolution 2, 156–175.
Grotzer, T. A., Gonzalez, E., and Schibuk, E. (2021). “Cause and effect: mechanism and prediction” in Crosscutting concepts: strengtheninig science and engineering learning. eds. J. Nordine and O. Lee (NSTA: Arlington, Va)
Grotzer, T. A., Solis, S. L., and Derbiszewska, K. (2017). Leveraging fourth and sixth graders’ experiences to reveal understanding of the forms and features of distributed causality. Cogn. Instr. 35, 55–87. doi: 10.1080/07370008.2016.1251808
Guesne, E. (1985). Light. In R. Driver, E Guesne, and A Tiberghien (Eds.), (pp.10–32) Children’s ideas in science. (Philadelphia: Open University Press).
Gupta, A., Hammer, D., and Redish, E. F. (2010). The case for dynamic models of learners’ ontologicies in physics. J. Learn. Sci. 19, 285–321. doi: 10.1080/10508406.2010.491751
Halloun, I. A., and Hestenes, D. (1985). The initial knowledge state of college physics students. Am. J. Phys. 53, 1043–1055. doi: 10.1119/1.14030
Hmelo, C. E., and Pfeffer, M. G. (2004). Comparing expert and novice understanding of a complex system from the perspective of structure, behaviors, and function. Cogn. Sci. 28, 127–138. doi: 10.1207/s155167cog2801_7
Horne, Z., Powell, D., Hummel, J. E., and Holyoak, K. J. (2015). Countering antivaccination attitudes. Proc. Natl. Acad. Sci. 112, 10321–10324. doi: 10.1073/pnas.1504019112
Jacobson, M. J., Kapur, M., So, H., and Lee, J. (2011). The ontologies of complexity and learning about complex systems. Instr. Sci. 39, 763–783. doi: 10.1007/s11251-010-9147-0
Jacobson, M. J., Markauskaite, L., Portolese, A., Kapur, M., Lai, P., and Roberts, G. (2017). Designs for learning about climate change as a complex system. Learn. Instr. 52, 1–14. doi: 10.1016/j.learninstruc.2017.03.007
Kettlewell, H. B. D. (1955). Selection experiments on industrial melanism n the Lepidoptera. Heredity 9, 323–342. doi: 10.1038/hdy.1955.36
Kuhlmann, M. (2014). Explaining financial markets in terms of complex systems. Philos. Sci. 81, 1117–1130. doi: 10.1086/677699
Legare, C. H., Opfer, J. E., Busch, J. T., and Shtulman, A. (2018). A field guide for teaching evolution in the social sciences. Evol. Hum. Behav. 39, 257–268. doi: 10.1016/j.evolhumbehav.2018.01.002
Levy, S. T., and Wilensky, U. (2008). Inventing a “mid-level” to make ends meet: reasoning between the levels of complexity. Cogn. Instr. 26, 1–47. doi: 10.1080/07370000701798479
Limon, M. (2001). On the cognitive conflict as an instructional strategy for conceptual change a critical appraisal. Learn. Instr. 11, 357–380. doi: 10.1016/S0959-4752(00)00037-2
Lombrozo, T., and Vasilyeva, N. (2017). Causal explanation. eds. M. R. Waldmann (ed). The Oxford Handbook of Causal Reasoning, Oxford, England: Oxford University Press, 415–432.
Markman, E. (1979). Classes and collections: conceptual organization and numerical abilities. Cogn. Psychol. 11, 395–411. doi: 10.1016/0010-0285(79)90018-5
Mayer, M. (1987). “Common sense knowledge versus scientific knowledge: the case of pressure, weight and gravity” in Proceedings of the second international seminar: misconceptions and educational strategies in science and mathematics, 1 (Ithaca, NY: Cornell University), 299–301.
McCloskey, M. (1983). “Naive theories of motion” in Mental Models. eds. D. Gentner and A. Stevens (Hillsdale, NJ: Lawrence Erlbaum Associates)
Moya, C., and Henrich, J. (2016). Culture–gene co-evolutionary psychology: cultural learning, language, and ethnic psychology. Curr. Opin. Psychol. 8, 112–118. doi: 10.1016/j.copsyc.2015.10.001
Nowak, M. A., and May, R. M. (1992). Evolutionary games and spatial Chaos. Nature 359, 826–829. doi: 10.1038/359826a0
Penner, D. E. (2000). Explaining processes: investigating middle school students’ understanding of emergent phenomena. J. Res. Sci. Teach. 37, 784–806. doi: 10.1002/1098-2736(200010)37:8<784::AID-TEA3>3.0.CO;2-E
Perkins, D., and Grotzer, T. A. (2005). Dimensions of causal understanding: the role of complex causal models in students’ understanding of science. Stud. Sci. Educ. 41, 117–165. doi: 10.1080/03057260508560216
Pfundt, H. (1981). The atom—the final link in the division process or the first building block? Pre-instructional conceptions about the structure of substances. Chimica didactica, 75–94.
Pfundt, H., and Duit, R. (1985). Bibliographie Alltagsvorstellungen und naturwissenschaftlicher Unterricht.
Pfundt, H., and Duit, R. (1988). Bibliography: student’s alternative frameworks and science education Institute for Science Education. Kiel Germany: University of Kiel.
Pfundt, H., and Duit, R. (1991). Bibliography: student’s alternative frameworks and science education (3rd edn). Kiel, Germany: IPN, University of Kiel.
Pfundt, H., and Duit, R. (1997). Bibliography: student’s alternative frameworks and science education (4th edn). Kiel, Germany: University of Kiel.
Pfundt, H., and Duit, R. (2001). Bibliography: student’s alternative frameworks and science education. Kiel, Germany: University of Kiel.
Posner, G. J., Strike, K. A., Hewson, P. W., and Gertzog, W. A. (1982). Accommodation of a scientific conception: toward a theory change. Sci. Educ. 66, 211–227. doi: 10.1002/sce.3730660207
Reiner, M., Slotta, J. D., Chi, M. T. H., and Resnick, L. B. (2000). Naive physics reasoning: a commitment to substance-based conceptions. Cogn. Instr. 18, 1–34. doi: 10.1207/S1532690XCI1801_01
Resnick, M. (1997). Turtles, termites, and traffic jams: explorations in massively parallel microworlds. Cambridge, MA: MIT Press.
Shtulman, A., Neal, C., and Linquist, G. (2016). Children’s ability to learn evolutionary explanations for biological adaptation. Early Educ. Dev. 27, 1222–1236. doi: 10.1080/10409289.2016.1154418
Simon, H. A. (2002). Nearly decomposability and the speed of evolution. Ind. Corp. Chang. 11, 587–599. doi: 10.1093/icc/11.3.587
Sinatra, G. M., and Broughton, S. H. (2011). Bridging reading comprehension and conceptual change in science: the promise of refutation text. Read. Res. Q. 46, 374–393. doi: 10.1002/RRQ.005
Slotta, J. D. (2011). In defense of Chi’s ontological incompatibility hypothesis. J. Learn. Sci. 20, 151–162. doi: 10.1080/10508406.2011.535691
Slotta, J. D., Chi, M. T. H., and Joram, E. (1995). Assessing students’ misclassifications of physics concepts: an ontological basis for conceptual change. Cogn. Instr. 13, 373–400. doi: 10.1207/s1532690xci1303_2
Solomon, J. (1983). Learning about energy: how pupils think in two domains. Eur. J. Sci. Educ. 5, 49–59. doi: 10.1080/0140528830050105
Su, M., Chi, M., Ha, J., and Xin, Y. (2023). “Investigating the efficacy of an ontological framework for teaching natural selection using agent-based simulations” in Proceedings of the annual meeting of the International Society of the Learning Sciences. eds. J. Slotta and E. Charles
Thacker, I., Sinatra, G. M., Muis, K. R., Danielson, R. W., Pekrun, R., Winne, P. H., et al. (2020). Using persuasive refutation texts to prompt attitudinal and conceptual change. J. Educ. Psychol. 112, 1085–1099. doi: 10.1037/edu0000434
Vosniadou, S. (1994). Capturing and modeling the process of conceptual change. Learn. Instr. 4, 45–69. doi: 10.1016/0959-4752(94)90018-3
Vosniadou, S. (2008). International handbook of research on conceptual change. Vol. 259. New York, NY: Routledge Press.
Vosniadou, S. (2013). International handbook of research on conceptual change (2nd Edn.). New York, NY: Routledge Press.
Wilensky, U., and Jacobson, M. J. (2014). “Complex systems and the learning sciences” in The Cambridge handbook of the learning sciences. ed. R. K. Sawyer. 2nd ed, (Cambridge University Press) 319–338.
Wilensky, U., and Resnick, M. (1999). Thinking in levels: a dynamic systems perspective to making sense of the world. J. Sci. Educ. Technol. 8, 3–19. doi: 10.1023/A:1009421303064
Keywords: Emergent processes, individualistic causal structure, collective causal structure, misconceptions, linear thinking, systems thinking, complexity
Citation: Chi MTH (2023) Individualistic and collective causal knowledge structures for understanding sequential and emergent processes. Front. Educ. 8:1198362. doi: 10.3389/feduc.2023.1198362
Edited by:
Tammy M. Long, Michigan State University, United StatesReviewed by:
Vicente Talanquer, University of Arizona, United StatesTina Grotzer, Harvard University, United States
Copyright © 2023 Chi. This is an open-access article distributed under the terms of the Creative Commons Attribution License (CC BY). The use, distribution or reproduction in other forums is permitted, provided the original author(s) and the copyright owner(s) are credited and that the original publication in this journal is cited, in accordance with accepted academic practice. No use, distribution or reproduction is permitted which does not comply with these terms.
*Correspondence: Michelene T. H. Chi, TWljaGVsZW5lLkNoaUBhc3UuZWR1