- 1Concord Consortium, Concord, MA, United States
- 2Graduate School of Education, University of Pennsylvania, Philadelphia, MA, United States
Real-world complex systems research seeks to understand how systems in the world can follow the same rules of complexity. Scientists have found similarities in processes—such as self-organization, micro-to macro-level emergence, and feedback loops—in seemingly disparate phenomena such as the spread of infectious diseases and how traffic patterns are formed. Our project, BioGraph 2.0, was developed to respond to the issue of students’ disjointed understanding of biology due to the fragmented nature of how high school biology is taught in high school classrooms. We hypothesized that by framing multiple biology concepts through the lens of complexity using dynamic simulations, or models featuring complex systems processes, students would be able to see complex systems as a unifying concept throughout biology. We built a series of units modeling phenomena on biological concepts such as gene regulation, ecology, and evolution using an agent-based modeling tool called StarLogo Nova. While previous research over the last decade of this project has highlighted students’ growth in complex systems understanding, in this study, we explored the relationship between complex systems and agent-based models. We investigated pre and post intervention data from over 300 high school students to determine how their metamodeling knowledge influenced their understanding of complex systems. Through a regression analysis, we demonstrate that growth in students’ modeling understanding significantly predicted growth in complex systems understanding. We further triangulate our findings with interview data from students who highlight the importance of the modeling tool to support their complex systems learning.
1. Introduction
The natural and social world that surrounds us is made up of systems that follow the rules of complexity (Servedio et al., 2014; Camazine et al., 2020). Complex systems can be defined as macrolevel patterns or structures that emerge from the activity of microlevel interacting agents (Yoon et al., 2018a). Researchers from different disciplines have noted that, regardless of the kinds of agents (e.g., predator and prey) and the ontological phenomenon under investigation (e.g., ecosystems), complex systems are composed of web-like structures in which individuals follow rules (e.g., wolves eat rabbits; Chi et al., 2012; West, 2014; Bar-Yam, 2016). Complex systems also have intricate interdependencies and structures that exist at different scales (e.g., trophic levels in ecosystems; Bar-Yam, 2016). Because of this web-like nested structure, information travels in nonlinear ways, which makes understanding cause and effect in complex systems behaviors challenging (Grotzer and Tutwiler, 2014). Moreover, often the dynamics that fuel complex systems behaviors (e.g., feedback loops and self-organization) are hidden and take place over large time spans (e.g., evolution) or spatial scales (e.g., climate change), which limits what we can understand about the whole system at any point in time or place (Grotzer and Tutwiler, 2014).
It is not surprising then that students in K–12 education harbor misconceptions about systems. A number of empirical studies have shown that they tend to adopt a linear approach when thinking about the relationships among system components rather than recognizing their nested non-linear nature (Sweeney and Sterman, 2007; Gotwals and Songer, 2010; Riess and Mischo, 2010). For example, Gotwals and Songer (2010) found that students struggled with reasoning about how a disruption in one part of a food chain could impact changes in another part of the food chain that was not directly connected to it. These indirect relationships, as Chi et al. (2012) argue, are hard to comprehend because the perceptual apparatus through which we observe phenomenon is limited to the information about the system we have access to at a particular point in time. Another common challenge that researchers have discussed is the tendency for students to attribute an outcome to a central agent or cause (Penner, 2000; Taber and García Franco, 2010; Levy and Wilensky, 2011). Students are unable to recognize that often control in systems is decentralized and that structures or behaviors at macro levels emerge from micro-level system activities. For example, ecosystems are able to stay in equilibrium (macro-level pattern) because of the combined activities of micro-level components (e.g., predator–prey interactions). But even more fundamentally, in a series of studies, Ben-Zvi Assaraf and colleagues have found that students often struggle to accurately identify the components that comprise a system and how those components are interrelated or exist as an integrated whole (e.g., Assaraf and Orion, 2010; Assaraf and Orpaz, 2010; Assaraf and Knipples, 2022).
To address these learning challenges, researchers have posited that computational modeling tools such as agent-based simulations could provide access to structures and behaviors of systems to support sense making and have been researching their uses and affordances (Wilensky and Jacobson, 2015; Wilensky and Rand, 2015; Yoon et al., 2018a; Mambrey et al., 2022; Yoon, 2022). A majority of this research has examined learning of biological systems. In our recent systematic review of complex systems research in K–12 science education, we found that topics within the field of biology were investigated in 83% of studies (Yoon et al., 2018a). Within these studies, agent-based simulations have been used to represent the complexity of biology systems in a more tangible and accessible format for students to explore complex systems thinking (Hmelo-Silver et al., 2017; Markauskaite et al., 2020; Housh et al., 2022; Jacobson and Wilenski, 2022; Yoon et al., 2022).
Models and modeling approaches have, in fact, received a great deal of attention in science education research due to their importance in conducting real-world scientific inquiry (NGSS Lead States, 2013). However, while learning and participation outcomes through the study of computational complex systems models have been generally understood to be positive, we found that only two studies in our systematic review (Yoon et al., 2018a) explored the relationship between instructional approaches that use complex systems models and student learning of complex systems. However, there is extensive research into how students conceive of models (e.g., Nicolaou and Constantinou, 2014; Nielsen and Nielsen, 2021). While content knowledge is important for working with models, so is metacognitive knowledge of models or metamodeling knowledge (Schwarz et al., 2009; Upmeier Zu Belzen et al., 2019; Chiu and Lin, 2022). This study explores how the instructional approach of agent-based models to represent complex systems afforded change in students’ metamodeling and complex systems knowledge and the relationship between the two.
The research reported here builds on more than a decade of work in which we have explored the use of computational complex systems models to support teaching and learning in high school biology. We built a series of units modeling phenomena of biological concepts such as gene regulation, ecology, and evolution using an agent-based modeling tool (described in more detail below). In this program of research, we have explored various educational goals such as designing curriculum and instruction to support complex systems and biology learning (Yoon et al., 2016), professional development for classroom instruction (Yoon et al., 2017), building teachers’ social capital for complex systems teaching (Yoon et al., 2018b), a learning progression for complex systems understanding (Yoon et al., 2019a), and supports for teacher community building to scale complex systems PD in online platforms (Yoon et al., 2020a,b). In this study, we address the need articulated in the review by Yoon et al. (2018a) for more studies that investigate the relationship between instructional approaches and student learning outcomes. Specifically, we investigated how students’ understanding of biological models using the modeling tool influenced their understanding of complex systems. To this end, we ask the following questions:
1. To what extent did biology students’ complex systems and modeling knowledge change over time?
2. To what extent is there a relationship between students’ modeling knowledge and their complex systems understanding for biology systems?
3. What affordances of the modeling tool and process can explain this relationship?
2. Theoretical background
Knowledge and understanding of complex systems and scientific models are inextricably linked due to the nature of complex systems and the need to create models to understand and analyze them, however there is an additional need to understand how high school students perceive and utilize this link in building their complex systems knowledge. The Next Generation Science Standards (NGSS) emphasize the connection in combining the two into a single crosscutting concept, systems and system models, which is explained as “defining the system under study—specifying its boundaries and making explicit a model of that system—provides tools for understanding and testing ideas that are applicable throughout science and engineering” (NGSS Lead States, 2013, Appendix C, p. 1). As such, there is a need to explore how both complex systems and scientific models are conceived by students and how those conceptions might influence knowledge development across both areas and their combined real-world applications.
2.1. Dimensions of complex systems understanding
Within K–12 research, several conceptual frameworks have been applied to what has been generally called systems learning (Yoon et al., 2018a). Specifically in biology, three frameworks have been popular for providing the theoretical foundation to understand how students learn: (a) systems thinking; (b) components-mechanisms-phenomena (CMP); and (c) complexity from emergence. Briefly, systems thinking focuses on the interrelationships and interdependence of system structures, which first requires identifying the components that comprise the system (e.g., the boundaries) and then considering the dynamic relationships between the components (Assaraf and Orion, 2010; Assaraf et al., 2013). Thus, the focus is on understanding particular qualities of the system under investigation that are unique from system to system. Similarly, a CMP framing emphasizes components, connections, and behaviors that phenomenologically define a particular system (Hmelo-Silver et al., 2017). Researchers have investigated aspects of systems understanding in CMP categories, noting that instruction often only focuses on macro-level structural components (e.g., trees, oxygen) at the expense of learning about mechanisms or behaviors (e.g., photosynthesis, carbon cycle) that underpin the function of a system (e.g., Jordan et al., 2014).
The third characterization of systems learning—complexity from emergence—aims to apply common processes that fuel systems. Researchers from this tradition recognize that systems from within and between disciplines often exhibit similar characteristics (e.g., feedback loops, self-organization, nonlinearity) that happen in microlevel interactions to produce macrolevel patterns (Chi et al., 2012; Wilensky and Jacobson, 2015; Yoon et al., 2017). This framing of emergent behaviors from local (simpler) behaviors to global (more complex) structures has supported research in notable organizations, like the Santa Fe Institute, to investigate some of the worlds’ most pressing problems such as disease epidemics and climate change. Our own work has taken this approach to learning about systems and has sought to understand how students reason through specific complex systems dimensions (Yoon et al., 2016, 2017) that include (a) the predictability of effects caused by small changes to the system, (b) the dynamism of the mechanisms and processes underlying the system, (c) the level of centralization of the organization of the system, and (d) the scale of the effects and capacities of the system (see Yoon et al., 2016 for more details). These four components are comprehended on a scale that ranges from, on one end, a clockwork framework of systems, in which systems are examined as individual parts, to, on the other end, a complex framework of systems understanding that acknowledges that the whole is greater than the sum of the parts. In other words, the properties of the whole complex system are properties that none of the parts have alone (Jacobson et al., 2011). In order for students to develop their understanding of complex systems, they must shift their ontological categories and move from a clockwork to a complex understanding of systems (Chi, 2005).
2.2. Scientific modeling and the importance of metamodeling knowledge
As the NGSS crosscutting concept systems and system models suggests, models and modeling are a vital part of science education but have also been identified as primary tools for achieving STEM integration (Kelley and Knowles, 2016; Hallström and Schönborn, 2019). As technological advances make computational models easier and more accessible, the ability to interpret these models is a driving factor for the integration of technology into other fields of science and engineering that, in turn, creates a need to include modeling as a component of STEM courses (Schwarz et al., 2009; Kelley and Knowles, 2016). To this end, numerous research studies have been conducted to understand and measure how students conceive of scientific models (e.g., Schwarz et al., 2009; Louca and Zacharia, 2012). The knowledge to understand and work with models, to create models within scientific practice, and to apply that knowledge to authentic context is often referred to as modeling competence (Upmeier Zu Belzen et al., 2019; Nielsen and Nielsen, 2021; Chiu and Lin, 2022). In a systematic review of empirical research on assessing modeling competence, Nicolaou and Constantinou (2014) found that modeling competence falls into two primary categories—namely, modeling practice, which is the ability to create and use models, and meta knowledge of models (also referred to as metamodeling knowledge), which is the understanding of the purpose, process, and use of models. This second category, meta knowledge of models, refers to the epistemological awareness about the nature and purposes of models and modeling, which is a form of metacognitive knowledge (e.g., Grosslight et al., 1991; Schwarz et al., 2009; Fortus et al., 2016; Upmeier Zu Belzen et al., 2019; Lazenby et al., 2020) rather than cognitive knowledge of the modeling process. In this project, students did not create their own models but instead engaged in activities that highlighted the utility of the modeling process to interpret simulated biological phenomenon. Thus, we use metamodeling knowledge as a measure of students’ understanding of scientific modeling.
In a highly cited article based on their work on the Modeling Designs for Learning Science (MoDeLS) project, Schwarz et al. (2009) sought to develop a set of learning progressions for metamodeling knowledge. They identified three components of metamodeling knowledge: nature of models, purpose of models, and the criteria for evaluating and revising models. The nature of models component includes an understanding that models are an abstract rather than literal representation of real-world phenomenon and that different models have different advantages and limitations. Purpose of models includes an understanding that models are a tool to advance knowledge about the world and specific phenomena (e.g., for explanation or for prediction). Finally, there should be an understanding that models change based on information that is generated from accumulated empirical data. Thus, the component of change as an essential criterion for evaluating and revising models is an important aspect of metamodeling knowledge (Grosslight et al., 1991; Gogolin and Krüger, 2018; Upmeier Zu Belzen et al., 2019).
In comparing student metamodeling knowledge to that of experts, three levels of thinking about models have been identified (Grosslight et al., 1991; Upmeier Zu Belzen et al., 2019). In Level 1 thinking, models are viewed as exact replicas of reality and are assessed based on whether they “correctly” illustrate reality. In Level 2 thinking, models are understood to have a purpose that dictates the nature of the model. The model can be used to communicate something about the already known reality it represents, but the main focus is on the model itself rather than the underlying ideas. A Level 3 understanding identifies models as part of the scientific process from which data can be collected and analyzed. Gogolin and Krüger (2018) found that most high school students have a Level 2 understanding of the nature of models and a Level 1 understanding of the purpose of models, though with some variation across grade level and context. They noted that only a handful of students reached Level 3 understanding about the nature and purpose of models and theorized that this was due to a lack of emphasis on models as tools for hypothesis and prediction within classroom instruction. As models are becoming more ubiquitous in science classrooms and are an integral tool for learning about complex systems, there is a need for a more explicit focus on promoting understanding of scientific models across contexts at the high school level (Nicolaou and Constantinou, 2014; Gogolin and Krüger, 2018; Upmeier Zu Belzen et al., 2019; Lazenby et al., 2020).
2.3. Complex systems modeling
Scientific computational models such as agent-based simulations can help the process of developing systems thinking and an understanding of complexity by enabling students to dynamically observe the interactions and interdependencies of individual parts and emergent system-wide patterns as they develop over time (Chi, 2005; Jacobson et al., 2011; Markauskaite et al., 2020; Yoon et al., 2022). Several studies have been conducted on complex systems modeling using agent-based simulation tools such as NetLogo and StarLogo Nova (e.g., Hmelo-Silver et al., 2017; Yoon et al., 2017; Markauskaite et al., 2020). The use of the agent-based modeling simulation StarLogo Nova allows for three different representations of the complex system being modeled: first, a visual representation of the interactions of the complex system model; second, mathematical representations of specific outputs over time; and, finally, the blocks-based code representation used to build the model (see Figure 1). It has been shown that multiple representations of the same system can support students’ understanding of the system (Jacobson et al., 2011; Ryu et al., 2015; Hmelo-Silver et al., 2017).
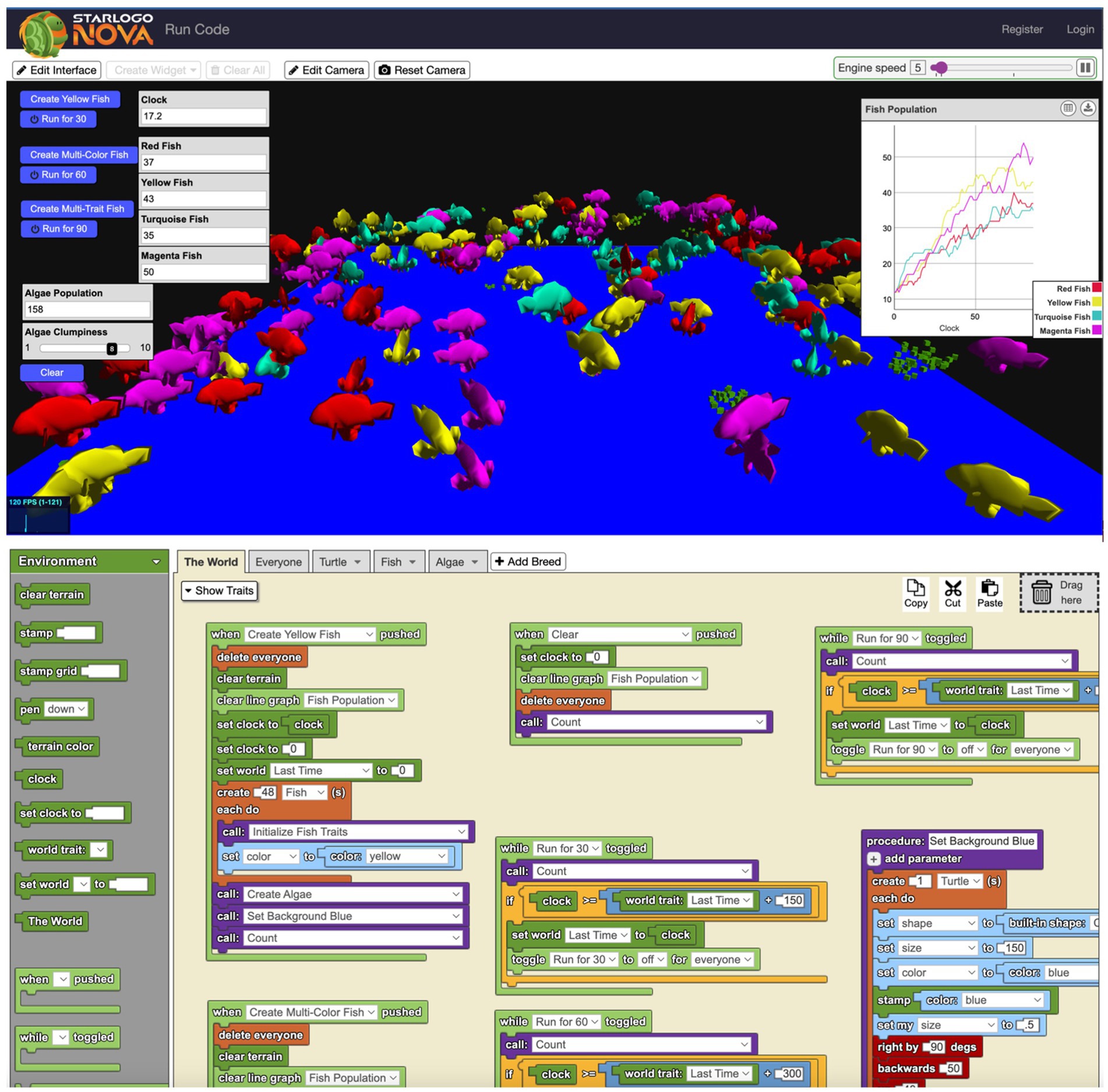
Figure 1. StarLogo Nova Interface: Model on Evolution. The top image shows the simulations of the fish interacting in the virtual environment. The mathematical representation can be seen in the top right in the form of a time-series graph, and the bottom half of the figure depicts the code used to build and run the simulation.
In our previous research, we have shown that the use of biological agent-based simulation in StarLogo Nova led to improvement in both biology and complex systems understanding (Yoon et al., 2017, 2020b). These findings are supported by the work of others, which showed that agent-based simulations of complex systems support the development of students’ understanding of complexity (e.g., Jacobson et al., 2011; Hmelo-Silver et al., 2017). Hmelo-silver et al. (2017) found that the use of an agent-based computational model of an ecosystem led students to a deeper understanding of the causal mechanisms within a complex system compared to students in a control group who did not engage with models. However, a CMP framework for complex systems understanding only focuses on macro-level structural components and does not consider understanding of complexity from emergence. Additionally, the study measured modeling practice against complex systems knowledge, rather than focusing on metamodeling knowledge. Similarly, Markauskaite et al. (2020) examined modeling practices in connection with a specific complex system of climate change but focused more on the content knowledge connections than generalizable components of complex systems knowledge. This suggests there is space for more research into the explicit nature of the relationship between students’ metamodeling knowledge and their knowledge of complex systems (Markauskaite et al., 2020) and how the affordances of the models support growth in understanding of complexity.
3. Methods
This is a mixed methods study that combines qualitative coding and analysis of open-ended responses with quantitative analysis of the coding in order to explore the relationship between students’ knowledge of modeling and knowledge of complex systems.
3.1. Intervention details and study parameters
This study is part of a long-standing program of research that has sought to increase engagement with and understanding of biology systems through the design and dissemination of a curriculum to teach common topics in high school biology through agent-based complex systems models. The curriculum is built around the computational modeling tool StarLogo Nova. The curriculum includes five units, each of which utilize their own complex system model, and each of which focuses that model on a particular topic typically taught in high school biology: genetics, evolution, ecology, the human body, and animal systems. They entail working with the scientific models to engage in core scientific practices as outlined in the NGSS, such as analyzing and interpreting data, engaging in argument from evidence, and obtaining, evaluating, and communicating knowledge claims. The student and teacher materials for the units engage learners with the nature and purpose of models by asking students to make predictions about what will occur in the system and then having them change the model parameters to test and observe what happens. Figure 2 presents a page from the student activity packet for the human body model; students are asked to observe the model, predict what the model will do using different input conditions, and then run the model with different conditions and record what the model does. Students normally worked in groups of two to complete the units, each of which take 2 to 3 days to complete. The program of research has been published on extensively; see previously published work for more details on the context of the program (e.g., Yoon et al., 2019b; Yoon, 2022).
This study encompasses data collected during the 2019–2020 and 2020-2021school years. The project shifted from an in-person format for teacher recruitment and training to an online format in 2018; 2019 was the first year that the program was fully accessible online for teachers to participate in training. It is important to note that the Coronavirus pandemic began during spring of 2020 and, as a result, the context of classroom implementation shifted across the time period of this study, as many teachers switched from in-person to hybrid or fully remote learning.
3.2. Participants
One of the goals of the larger study was to understand the efficacy and effects of the curriculum across different contexts. As such, this study involved eight teachers from five different schools in two countries (U.S. and India). These teachers were chosen from the larger group of 42 teachers who completed the online training course in 2019 based on several parameters, including their high level of engagement with the PD course, their commitment to implementing at least three of the five modules throughout the school year, their student populations and the degree of survey completion, and their interest in and enthusiasm for participating in the study. Ultimately the primary reason for selection was the teachers’ agreement to participate in the research. The study encompasses 2 years of implementation. Three of the eight teachers implemented the curriculum in both years of the study. The teachers all identified as female, and their teaching experience ranged from 3 to 28 years in the classroom. A summary of the teachers’ descriptive statistics can be found in Table 1. Each of the participating teachers implemented at least three of the units; therefore, the participating students worked with at least three different agent-based simulations of complex systems.
A total of 369 students participated in this study. Descriptive statistics for the student participants can be found in Table 2. Most of the teachers implemented the curriculum with ninth-grade students; however, a few of the classes were mixed grade and therefore included upper classmen.
3.3. Data sources
To investigate our research questions, survey tests of students’ pre-and post-implementation complex systems knowledge and metamodeling knowledge were conducted in both years, and student focus group interviews were conducted in Year 2 to further probe the relationship between modeling and complex systems knowledge.
Students completed two surveys pre-implementation and two surveys post-implementation. Though the surveys contained the same questions, they were administered 9 months apart, to mitigate the effects of item exposure. The first survey consisted of one open-ended question to measure their knowledge of complex systems (i.e., “Imagine a flock of geese arriving in a park in Philadelphia, where geese have not lived before. Describe how the addition of these geese to the park may affect the ecosystem over time. Consider both the living and nonliving parts of the ecosystem.”). The second survey included three open-ended questions about scientific models. These were: (a) How would you describe what a scientific model is to someone who did not know what a model is?; (b) Describe what models are used for and how they could be used in science; and (c) What, if anything, would cause a scientist to change a model of a scientific concept? These three prompts about models were designed to solicit understanding of the three components of metamodeling knowledge (Schwarz et al., 2009).
In Year 2, which was taught mostly remotely or through hybrid remote and in-person learning, virtual semi structured focus group interviews were conducted over Zoom with one or two groups of three to five students from each class for a total of six focus group interviews across the four teachers participating in Year 2 implementation. These interviews sought to explore how students experienced the models in relation to their understanding of complex systems and conduct deeper exploration into the affordances those models and the process of modeling provided in order to more fully answer the third research question. Some example questions from these interviews include: Based on your understanding of biological systems, what characteristics do they exhibit, and how do you know this from the models? and What do you think are characteristics of good scientific models or explanations in terms of helping you learn or understand the science behind them? These interviews ranged in length from 52 to 66 min and were recorded and transcribed for analysis.
3.4. Data analysis
Analysis for this study was conducted using a mixed methods approach that combined qualitative and quantitative strategies for measuring student learning of scientific models and complex systems.
3.4.1. Coding of students’ complex systems and metamodeling knowledge
Three separate rounds of qualitative analysis were conducted on the data for this study: coding of the open-ended responses on content knowledge for complex systems; coding of the open-ended responses on metamodeling knowledge; and mining of interview transcripts for information that supported the findings from the coding and quantitative analyses.
The coding manual used for coding the complex systems open responses has been reported on previously (see Yoon et al., 2016, 2020b). The coding manual was originally constructed from theories presented in Pavard and Dugdale (2000) and refined based on Jacobson et al. (2011) and through over a decade of use in studying complex systems understanding. The manual consists of four components each scored on the level of understanding as 1 (clockwork), 2 (emerging complexity), or 3 (complex) for a possible total score from 3 to 12. Table 3 presents descriptions of the components and example responses from students at the clockwork and complex levels of understanding. For example, the student response provided below is an example of a Level 3 (completely complex) understanding in the component of predictability because the student lists many different options for potential directions the ecosystem could take and uses the word “could” to show unpredictability:
Since the geese arrive at a place they haven’t ever been before, there are many ways they can affect the ecosystem and it is impossible to say exactly how. For example, they could drive other birds away so that they can lay eggs. They could drive other birds away because they compete for the same kind of food. They could cause the increase of other animals who feed on geese. They could cause the increase of other birds because the geese have become an alternative food source for existing predators. It’s really hard to tell.
However, despite representing completely complex thinking for predictability, this response also depicts a Level 2 (emerging complexity) understanding for the other three components. For example, while acknowledging the existence of other species with agency in the ecosystem, the response is still centered on the geese as the central driving factor in the changes that occur in the system, which is scored as a Level 2 understanding in the category of order.
Responses to the complex systems survey were coded by three members of the research team in two rounds, one for each year of the study. As there were two responses that needed coding for each student (pre-and post-test), there were 380 responses from Year 1 and 358 responses from Year 2. One of the researchers was involved in coding responses from previous iterations of the project and conducted training on the codebook for the other two researchers. After multiple rounds of test coding, an inter-rater reliability test was conducted on 80 responses (21%), and a Cronbach alpha correlation coefficient of α = 0.863 was achieved, which represents good reliability (Stemler and Tsai, 2008). After the disagreements were discussed and resolved, the remaining responses were divided evenly among the three researchers for coding. For Year 2 coding, the three researchers reconvened about 9 months later and conducted a second inter-rater reliability test on 72 of 358 responses (20%) from Year 2 and received a correlation coefficient of α = 0.858. The disagreements were again discussed and resolved, with the remaining responses divided evenly among the three researchers for coding.
The coding manual for the modeling responses was adapted from prior work conducted on measuring metamodeling knowledge (Grosslight et al., 1991; Schwarz et al., 2009; Fortus et al., 2016; Gogolin and Krüger, 2018; Lazenby et al., 2020). Responses were scored on a scale of 1 (models as copies of reality) to 3 (models as tools for understanding and predicting reality) for each of three different dimensions of metamodeling knowledge (Schwarz et al., 2009). These dimensions are listed and explained in Table 4. The responses to three separate open-ended questions were combined into a single response for coding, and codes of 0 were allowed for responses that consisted of “I do not know” or blank answers for one of the dimensions. Therefore, total possible scores ranged from 0 to 9.
To explain the coding in a little more detail, below is a sample response from a student:
I would describe [a scientific model] as something that shows or represents in detail what the science is trying to show. Models are used to visualize things and to get a better look and understanding. [A scientist might change a model if] they saw that their model didn’t accurately represent the data they’re trying to show.
In this response, the use of the words “show” and “accuracy” demonstrates an understanding of a model as a static representation of an intended outcome whose role is to depict that outcome in alignment with expected reality. This response was scored as a Level 1 for all three properties. In contrast, the following example response demonstrates a more advanced level of metamodeling knowledge:
A scientific model is a concept to make something easier to understand. It could be any type of model to visualize something that is being experimented. Models are used to represent something in the real world. It is a way that scientists can make predictions and propose new ideas. [A scientist might change a model] based on their new findings and concepts that they are developing in their experiment.
In this second response, the student recognizes the active role of models in the scientific process (scored as a 3 for purpose) and cycle of changing models as part of that process (scored as a 3 for change). While they still connect models to real-world representations, they understand that a model is not an exact replica (scored as a 2 for nature of the model).
We worked with a member of the research team who was not involved in creating the codebook to test the coding manual for understanding and clarity. Two additional researchers were trained on the codebook who achieved an inter-rater reliability Cronbach alpha coefficient of α = 0.90 on 70 responses (9% of the total of 738 over the 2 years). We realize that this is less than the standard of 20% of the data used to obtain interrater reliability, there were additional time constraints and availability of the coders decreased substantially due to the time of year that coding was requested. However, as the alpha coefficient is well over the 0.70 limit indicating good reliability (Stemler and Tsai, 2008), and as the sample is large, we deemed this was a sufficient measure of reliability and decided to proceed with coding of the remaining responses. After the differences were discussed, one researcher (first author) coded the remaining responses.
The student focus group interviews were mined by the first author for responses that could explain how the curriculum and models afforded better understanding of complex systems. Responses were then grouped into themes that supported the three categories of metamodeling knowledge.
3.4.2. Relationship between students’ complex systems and metamodeling knowledge
The resulting codes were compared pre-to post-test scores for both modeling knowledge and complex systems understanding. A paired samples t-test was conducted to determine whether there was positive significant growth in both measures. The results were then analyzed to understand whether there was a relationship between the two measures through hierarchical regression modeling. The analysis was conducted to determine whether there was a significant effect on complex system understanding beyond their prior knowledge of modeling and understanding of complex systems measured at the pre-test survey.
4. Findings
Results from the analysis of the coded open-ended survey responses revealed significant growth in both metamodeling and complex systems knowledge. The results of the regressions analysis showed that modeling knowledge had a significant positive effect on complex systems understanding when holding all other variables constant. Finally, the student focus group interviews supported these findings with quotes from students depicting how aspects of the models were viewed to enhance their learning of the complex biological systems.
4.1. Knowledge growth in both scientific modeling and complex systems
The results of the surveys showed growth from pre-test to post-test for both measures, where a paired samples t-test showed positive significant growth t (368) = 6.03, p < 0.001 with a Cohen’s d effect size of 0.39 for students’ modeling knowledge which is a small to medium effect (Lakens, 2013), and positive significant growth t (368) = 4.62, p < 0.001 with a Cohen’s d effect size of 0.27 which is a small effect for students’ complex systems understanding (see Table 5 for more details).
While these results supported previous findings that students experienced growth in their complex systems knowledge, in this study we were primarily interested in the relationship between change in modeling knowledge and complex systems knowledge. This relationship was explored through a regression analyses.
4.2. Change in metamodeling knowledge has significant positive impact on change in complex system understanding
To test if students’ metamodeling knowledge improved their understanding of complex systems beyond their prior knowledge of modeling and understanding of complex system measured at the pre-test, a hierarchical regression was conducted with two blocks of variables. The first block included students’ pre-test of knowledge of modeling and pre-test of knowledge of complex system as the predictors, and with students’ post-test measure of understanding of complex system as the dependent variable. In block two, students’ post-test measure of metamodeling knowledge was also included as the predictor variable, with students’ post-test measure of understanding of complex system as the dependent variable (see Table 6 for a summary).
Overall, the results show that the first model was significant F (2,366) = 28.85, p < 0.001, R2 = 0.14. But only students’ pre-test measure of understanding of complex system was significantly associated with the post-test measure of understanding of complex system (b = 0.37, t = 6.81, p < 0.001). The second model (F (1,365) = 32.49, p < 0.001, R2 = 0.21), which included students’ post-test measure of modeling knowledge (b = 0.33, t = 5.70, p < 0.001), showed significant improvement from the first model, ∆R2 = 0.07, p < 0.001. Overall, when students’ pre-test of knowledge of modeling and pre-test measure of understanding of complex system were included in the model, the variables explained 14% of the variance. The final model, including students’ post-test measure of understanding of modeling, accounted for 21% of the variance. Thus, with the addition of the second independent variable of students’ post-test modeling scores, results showed that it significantly predicted students’ complex systems understanding in the post-test beyond students’ prior knowledge of modeling and understanding of complex systems measured at the pre-test.
4.3. How metamodeling knowledge supports complex systems learning
To answer our third research question about the specific affordances that allowed for the connection between metamodeling knowledge and complex systems understanding, an analysis of the student focus group interviews was conducted. The three components of metamodeling knowledge: nature of models, purpose of models, and the criteria for evaluating and revising models (Schwarz et al., 2009) were identified within the interviews and three themes emerged that connect those components of metamodeling knowledge to complex systems understanding and highlight specific affordances of the StarLogo Nova models that support students’ complex systems understanding development. For the nature of models, students focused on the “realistic” quality of the models, which allowed for aspects of complex systems in biology to be viewed and explored. Students understood the purpose of the models to have a role in communicating different aspects of the system through the different representations within the model. Finally, students engaged with the changeability of models through manipulating parameters to highlight characteristics of complex systems such as randomness and interconnectedness.
4.3.1. The nature of models as “realistic” representations of complex systems
While viewing scientific models as exact copies of reality supports a low-level understanding of the nature and purpose of models, it is important to understand that models are used to represent reality in some way that is useful. The connection to reality was a component of the models that students were drawn to, and which was brought up by multiple students in response to a question about the nature of good scientific models. For example, a student from a focus group for Teacher 5 said, “They model a real-life system, so we can see how, in real life, they work. We can actually see each component of every system, and that really helped me, at least, understand how all these things work.” In response to the same prompt, a student from Teacher 8’s class identified the importance of keeping models close to reality while also modifying them to make them simple:
I generally think of things that are easy to navigate, but also keeping it realistic. So, they're not so simple that it's not enough information, but just the right amount that it still looks relatively real to what you're learning about. Keeping it simple but realistic at the same time, because if it's not realistic, it's not benefiting you for learning what that system really looks like.
These quotes support students’ metamodeling knowledge of the nature of models as useful representations of reality. Students also connected the realistic nature of the models to characteristics of complex systems. One student from Teacher 8’s class said, for example, that “Even if you would test [the model] again and again, it was super unlikely you’d come to the same answer twice just because they are trying to make it as realistic as possible.” A student from Teacher 5’s class had a similar observation, saying “Getting different outcomes with different numbers or even that the same numbers, just like a more realistic model, and I think that’s how scientific models should be.” These quotes show that students were making connections between the nature of models and the nature of complex systems.
4.3.2. The purpose of models: to communicate through different representations of the system
Most of the students interviewed spoke about the purpose of models to communicate and explain complex systems through multiple representations of the system and the data within it. While the students did not talk about the code representation, both the visual and mathematical representations were highlighted as important factors in building their understanding of the complex systems and underlying concepts. A student from a focus group for Teacher 8 mentioned that having the visual representation was an added benefit over auditory methods she was more used to encountering, saying:
I feel like it really helped just to put a visual to the things that we were learning. Not just have the words in an auditory explanation of what was going on, but to see what was actually going on and have a good visual of it.
Mathematical representations, in the form of graphs that tracked output data from the simulations, were also a source of information that students used to interpret complex systems. In the focus group with students from Teacher 5, one student responded to a comment about tracking changes in the system by highlighting the graphs, saying, “We could usually tell that by the two graphs on the side, which would kind of help to see how dramatic or undramatic the changes were.”
Students also made connections between the visual and mathematical representations within the simulations. One student from a focus group for Teacher 6 talked about how the visual representation helped simplify the complexity while the mathematical representation helped him understand the process:
These models, they help simplify a very complex scientific idea and it helps me visualize and, for example, the graph for the gene regulation, it helps you understand how the graph was developed and instead of making biology feeling like it's something that's just needs to be memorized, it helps you understand the process more.
The students recognized that having multiple representations of the model in the StarLogo Nova simulation allowed them to explore complex systems in multiple ways that helped them understand the concepts and complex systems in general. For these students, the model was a tool for building understanding.
4.3.3. Changing the model parameters to explore randomness and connectivity as components of complex systems
Though the students did not change the underlying code of the simulations, they did change the parameters that were used as initial conditions for running the model and chose different scenarios to model, which served as examples for thinking about model changeability. This ability to change the model in response to the data produced by the model in order to further explore the system being simulated supported students in developing complex systems knowledge. A student from Teacher 6’s class explained this process:
Something else I noticed with this simulation was that you could customize the different scenarios, so that it fit with what you were trying to learn. I remember we would put in different barriers in different types of sugar. I remember that was really helpful because we could, and with all of the simulations too, you could create these different scenarios to separately explore different concepts.
The changeability of the models allowed students to observe the connected nature of the complex systems and the way that the models were able to make those connections observable to them. In talking about the ecology model, one student in Teacher 5’s class said,
What we learned was how when one species is affected, it's not just affected individually. It's kind of like a domino effect that affects the organisms it feeds on and the organisms that feed on it, which was really interesting.
A student from Teacher 6’s class made an explicit connection between this interconnectedness of the components of the models and the fact of that as a defining characteristic of complex systems saying,
There are a lot of different parts to all of [the models], it's just part of what a complex system is, and they all work together, and there are different outcomes based on how they work together, so I would say that's the characteristic that they exhibit.
The students also noticed the ability of the models to simulate the emergent nature of complex systems, which can seem like randomness due to the complexity of the interactions of the components within the system. One student from Teacher 5’s class noted the relationship between the randomness displayed by the models and what might happen in real life, saying,
One thing I noticed about these [models] is that the outcomes were kind of different every time. If multiple people in the class did the same numbers or same data, it wasn't guaranteed to get the same response and the same outcome. Obviously, that’s how it is in real life.
A student in Teacher 6’s class made a connection between the randomness displayed by all the models and the unpredictability of complex systems, showing a high-level understanding of both the nature of models and of complex systems, saying, “All the models had different models of different parts of things, and they all moved randomly, and you have that element of unpredictability, which would be a characteristic of complex systems.” Finally, a student from Teacher 7’s class summed up all three of the themes from the interviews in a single quote, saying,
The graph there in the Gene Regulation, that's useful. So, it's not just that I think there's more of these over time and then they decrease, you can see the graph, you can see it's actually happening. Also, I think the randomness … In all of these, if you run the simulation multiple times, it's not just the same exact thing. The factors are working off each other with a bit of randomness. You can tell that whatever is happening is actually happening. In the real world, it's not going to be the same every single time. It's more realistic.
These three themes and the quotes that illustrate them add further support to the quantitative analysis of the student knowledge surveys and suggest that there is a significant connection between students’ metamodeling knowledge and their learning about complex systems. Students’ ability to see models as useful representations of an aspect of reality and to understand that they could be manipulated to view that reality from different angles and different starting scenarios allowed them to develop a deeper understanding of complex systems and their emergent nature.
5. Discussion
Our findings answer our research questions in the following ways. There was significant growth both in students’ metamodeling knowledge and in their complex systems understanding across both years of the study. The hierarchical regression analysis also showed a significant effect of students’ growth in metamodeling knowledge on their growth in complex systems understanding. Furthermore, student interviews identified three distinct ways that their modeling experiences supported learning about complex systems, highlighting supports for metamodeling knowledge reported in the literature review (i.e., the nature, purpose, and changeability of models; Schwarz et al., 2009). From the focus interview responses about the nature of models, the agent-based simulations in our study enabled students to observe system structures through visualizations of system component interactions (Chi, 2005; Jacobson et al., 2011; Markauskaite et al., 2020). These dynamics are normally hidden to the naked eye, which makes it challenging to understand how system patterns emerge (Yoon et al., 2018a). Emergent patterns in biology, such as climate change or natural selection, are also difficult to witness in real time because they appear over large geographic and temporal scales (Grotzer and Tutwiler, 2014). Many students in our study noted that being able to see the system all at once was important to their learning. Regarding the purpose of models, the existence of multiple representations of the scientific phenomenon under investigation provided students with strategies to interpret data generated from multiple runs and to develop explanations of the system (Gogolin and Krüger, 2018; Upmeier Zu Belzen et al., 2019). Finally, regarding the changeability of models, the ability to manipulate initial conditions and the ability to compare varying results allowed students to develop more sophisticated scientific theories (e.g., that there is built-in variation and randomness in all systems) than what only a single run of the simulation would otherwise afford. These findings support previous research showing the affordances of computational models as tools for increasing students’ complex systems understanding (e.g., Hmelo-Silver et al., 2017; Yoon et al., 2017; Markauskaite et al., 2020; Nguyen and Santagata, 2021).
While these findings support previous research and add to the research on modeling and complex systems by explicitly demonstrating a quantitative significant effect of metamodeling knowledge on complex systems understanding, there are limitations to the study. The sample of teachers in the study were self-selecting into the professional development for the StarLogo Nova simulations and resources, and into the study. As such, the teachers were highly motivated and likely represented an ideal population of students. Additionally, the Covid-19 pandemic made working with the teachers and students in India impossible for the second year of the study which limited the diversity of the students in the study and may have skewed the regression model. Another limitation is that, while this study focused on students’ metamodeling knowledge, modeling practices were not measured and certain components of modeling competence such as multiple models and testing models (Upmeier Zu Belzen et al., 2019) were not included in the study. Finally, we acknowledge that this work is embedded firmly within the context of Biology and while metamodeling knowledge is conceived as content general knowledge, it has been found that there exists a difference between contextualized and decontextualized metamodeling knowledge so our results may only speak to contextualized knowledge (Göhner et al., 2022).
While acknowledging some limitations, the findings reported here emerge from over a decade of research on this project that involved years of iterative design and implementation cycles to reach a point where the curriculum and PD experiences fully supported teachers and students in using models to support learning of complex systems (see Yoon et al., 2016, 2020b; Yoon, 2022). Our research has produced significant outcomes for student learning and supported attempts to scale up access to project resources more globally (Yoon et al., 2020b). Developing greater understanding of complex systems (Yoon et al., 2018a) and systems more generally (NGSS Lead States, 2013) has also been a focus of educators and educational researchers for many years. Despite this longstanding interest, however, complex systems curricula and tools have still not made their way widely into biology classrooms (Gilissen et al., 2020; Markauskaite et al., 2020). Perhaps this slow progress is related to the lack of studies that make explicit the connection between growth in student understanding of complex systems and specific instructional approaches such as agent-based modeling, as noted in a previous literature review (Yoon et al., 2018a). Without assurances that learning outcomes will improve, it may be difficult to convince teachers to adopt new pedagogies and tools like ours that add additional time to the standard biology curriculum. That we found improvements in both student measures of metamodeling knowledge and complex systems understanding even in Year 2 of the project—where teaching and learning happened fully online—is also worth highlighting given the documented learning losses that we have experienced due to the pandemic (Nowicki, 2022).
6. Conclusion
In this study, we investigated how students’ understanding of biological models using an agent-based modeling tool influenced their understanding of complex systems. Through many years of design iterations, we developed a curriculum that supports growth in students’ knowledge of scientific models and complex systems understanding in high school biology. Through a regression analysis of 2 years of student data, we demonstrated that growth in students’ modeling knowledge significantly predicted growth in their understanding of complex systems. We further showed that students perceived multiple aspects of the agent-based modeling tool as important to supporting their understanding of complex systems. Studies that demonstrate explicit relationships between instructional approaches and improvements in complex systems content learning are rare, which underscores the overall value and contribution of this research. We hope that future research will continue to explore the relationship between metamodeling knowledge and complex systems understanding both to replicate our work with different systems’ representations to show that the effects are significant in other contexts and content areas, and to expand upon our work to include more components of modeling competencies (Schwarz et al., 2009; Fortus et al., 2016; Upmeier Zu Belzen et al., 2019). Specifically, embedding agent-based simulations within the scientific inquiry process to support students’ deeper exploration of complex systems and their development of systems thinking.
Data availability statement
The raw data supporting the conclusions of this article will be made available by the authors, without undue reservation.
Ethics statement
The studies involving human participants were reviewed and approved by University of Pennsylvania IRB. Written informed consent to participate in this study was provided by the participants’ legal guardian/next of kin.
Author contributions
KM and SY contributed to conception and design of the study. KM performed the analysis and wrote the first draft of the manuscript. SY wrote sections of the manuscript. All authors contributed to the article and approved the submitted version.
Funding
This research is supported by a grant from the U.S. National Science Foundation under the program Discovery Research K-12 (DRL #1019228).
Conflict of interest
The authors declare that the research was conducted in the absence of any commercial or financial relationships that could be construed as a potential conflict of interest.
Publisher’s note
All claims expressed in this article are solely those of the authors and do not necessarily represent those of their affiliated organizations, or those of the publisher, the editors and the reviewers. Any product that may be evaluated in this article, or claim that may be made by its manufacturer, is not guaranteed or endorsed by the publisher.
References
Assaraf, O. B. Z., Dodick, J., and Tripto, J. (2013). High school students’ understanding of the human body system. Res. Sci. Educ. 43, 33–56. doi: 10.1007/s11165-011-9245-2
Assaraf, O. B., and Knippels, M. P. J. (2022). “Lessons learned: synthesizing approaches that foster understanding of complex biological phenomena,” in Fostering understanding of complex systems in biology education. eds. O. B. Z. Assaraf and M. C. P. Knippels (Cham: Switzerland: Springer), 249–278.
Assaraf, O. B., and Orion, N. (2010). System thinking skills at the elementary school level. J. Res. Sci. Teach. 47, 540–563. doi: 10.1002/tea.20351
Assaraf, O. B., and Orpaz, I. (2010). The life at the poles study unit: developing junior high school students’ ability to recognize the relations between earth systems. Res. Sci. Educ. 40, 525–549. doi: 10.1007/s11165-009-9132-2
Bar-Yam, Y. (2016). From big data to important information. Complexity 21, 73–98. doi: 10.1002/cplx.21785
Camazine, S., Deneubourg, J. L., Franks, N. R., Sneyd, J., Theraula, G., and Bonabeau, E. (2020). Self-organization in biological systems. Princeton: Princeton University Press.
Chi, M. T. (2005). Commonsense conceptions of emergent processes: why some misconceptions are robust. J. Learn. Sci. 14, 161–199. doi: 10.1207/s15327809jls1402_1
Chi, M. T. H., Roscoe, R., Slotta, J., Roy, M., and Chase, M. (2012). Misconceived causal explanations for emergent processes. Cogn. Sci. 36, 1–61. doi: 10.1111/j.1551-6709.2011.01207.x
Chiu, M. H., and Lin, J. W. (2022). “Research on modeling competence in science education from 1991 to 2020 with cultural and global implications,” in International handbook of research on multicultural science education. ed. M. M. Atwater (Cham: Springer International Publishing), 961–1000.
Fortus, D., Shwartz, Y., and Rosenfeld, S. (2016). High school students’ meta-modeling knowledge. Res. Sci. Educ. 46, 787–810. doi: 10.1007/s11165-015-9480-z
Gilissen, M. G., Knippels, M. C. P., and van Joolingen, W. R. (2020). Bringing systems thinking into the classroom. Int. J. Sci. Educ. 42, 1253–1280. doi: 10.1080/09500693.2020.1755741
Gogolin, S., and Krüger, D. (2018). Students' understanding of the nature and purpose of models. J. Res. Sci. Teach. 55, 1313–1338. doi: 10.1002/tea.21453
Göhner, M. F., Bielik, T., and Krell, M. (2022). Investigating the dimensions of modeling competence among preservice science teachers: Meta-modeling knowledge, modeling practice, and modeling product. J. Res. Sci. Teach. 59, 1354–1387. doi: 10.1002/tea.21759
Gotwals, A. W., and Songer, N. B. (2010). Reasoning up and down a food chain: Using an assessment framework to investigate students’ middle knowledge. Sci. Ed. 94, 259–281.
Grosslight, L., Unger, C., Jay, E., and Smith, C. L. (1991). Understanding models and their use in science: conceptions of middle and high school students and experts. J. Res. Sci. Teach. 28, 799–822. doi: 10.1002/tea.3660280907
Grotzer, T. A., and Tutwiler, M. S. (2014). Simplifying causal complexity: how interactions between modes of causal induction and information availability lead to heuristic-driven reasoning. Mind Brain Educ. 8, 97–114. doi: 10.1111/mbe.12054
Hallström, J., and Schönborn, K. J. (2019). Models and modelling for authentic STEM education: reinforcing the argument. Int. J. STEM Educ. 6, 1–10. doi: 10.1186/s40594-019-0178-z
Hmelo-Silver, C. E., Jordan, R., Eberbach, C., and Sinha, S. (2017). Systems learning with a conceptual representation: a quasi-experimental study. Instr. Sci. 45, 53–72. doi: 10.1007/s11251-016-9392-y
Housh, K., Hmelo-Silver, C. E., and Yoon, S. A. (2022). “Theoretical perspectives on complex systems in biology education” in Fostering understanding of complex systems in biology education. eds. O. B. Z. Assaraf and M. C. P. Knippels (Cham: Switzerland: Springer), 1–16.
Jacobson, M. J., Kapur, M., So, H. J., and Lee, J. (2011). The ontologies of complexity and learning about complex systems. Instr. Sci. 39, 763–783. doi: 10.1007/s11251-010-9147-0
Jacobson, M. J., and Wilenski, U. (2022). “Complex systems and the learning sciences: educational, theoretical, and methodological implications” in The Cambridge handbook of the learning sciences. ed. R. K. Sawyer (Cambridge, MA: Cambridge University Press), 504–522.
Jordan, R. C., Brooks, W. R., Hmelo-Silver, C., Eberbach, C., and Sinha, S. (2014). Balancing broad ideas with context: an evaluation of student accuracy in describing ecosystem processes after a system-level intervention. J. Biolog. Educ. 48, 57–62. doi: 10.1080/00219266.2013.821080
Kelley, T. R., and Knowles, J. G. (2016). A conceptual framework for integrated STEM education. Inter. J. STEM Educ. 3, 1–11. doi: 10.1186/s40594-016-0046-z
Lakens, D. (2013). Calculating and reporting effect sized to facilitate cumulative science: a practical primer for t-tests and ANOVAs. Front. Psychol. 4:863. doi: 10.3389/fpsyg.2013.00863
Lazenby, K., Stricker, A., Brandriet, A., Rupp, C. A., Mauger-Sonnek, K., and Becker, N. M. (2020). Mapping undergraduate chemistry students’ epistemic ideas about models and modeling. J. Res. Sci. Teach. 57, 794–824. doi: 10.1002/tea.21614
Levy, S. T., and Wilensky, U. (2011). Mining students’ inquiry actions for understanding of complex systems. Comput. Educ. 56, 556–573. doi: 10.1016/j.compedu.2010.09.015
Louca, L. T., and Zacharia, Z. C. (2012). Modeling-based learning in science education: cognitive, metacognitive, social, material, and epistemological contributions. Educ. Rev. 64, 471–492. doi: 10.1080/00131911.2011.628748
Mambrey, S., Schreiber, N., and Schmiemann, P. (2022). Young students’ reasoning about ecosystems: The role of systems thinking, knowledge, conceptions, and representation. Res. Sci. Ed. 52, 79–98.
Markauskaite, L., Kelly, N., and Jacobson, M. J. (2020). Model-based knowing: how do students ground their understanding about climate systems in agent-based computer models? Res. Sci. Educ. 50, 53–77. doi: 10.1007/s11165-017-9680-9
NGSS Lead States (2013). Next generation science standards: for states, by states. Washington, DC: National Academies Press.
Nguyen, H., and Santagata, R. (2021). Impact of computer modeling on learning and teaching systems thinking. J. Res. Sci. Teach. 58, 661–688. doi: 10.1002/tea.21674
Nicolaou, C. T., and Constantinou, C. P. (2014). Assessment of the modeling competence: a systematic review and synthesis of empirical research. Educ. Res. Rev. 13, 52–73. doi: 10.1016/j.edurev.2014.10.001
Nielsen, S. S., and Nielsen, J. A. (2021). A competence-oriented approach to models and modelling in lower secondary science education: practices and rationales among danish teachers. Res. Sci. Educ. 51, 565–593. doi: 10.1007/s11165-019-09900-1
Nowicki, J. M. (2022). Pandemic learning: as students struggled to learn, teachers reported few strategies as particularly helpful to mitigate learning loss. Report to the Congressional Committees. GAO-22-104487. Available at: https://files.eric.ed.gov/fulltext/ED620297.pdf
Pavard, B., and Dugdale, J. (2000). “The contribution of complexity theory to the study of socio-technical cooperative systems,” in Unifying themes in complex systems. eds. A. A. Minai, D. Braha, and Y. Bar-Yam (Berlin, Germany: Springer), 39–48.
Penner, D. E. (2000). Explaining systems: investigating middle school students’ understanding of emergent phenomena. J. Res. Sci. Teach. 37, 784–806. doi: 10.1002/1098-2736(200010)37:8<784::AID-TEA3>3.0.CO;2-E
Riess, W., and Mischo, C. (2010). Promoting systems thinking through biology lessons. Int. J. Sci. Educ. 32, 705–725. doi: 10.1080/09500690902769946
Ryu, S., Han, Y., and Paik, S. H. (2015). Understanding co-development of conceptual and epistemic understanding through modeling practices with mobile internet. J. Sci. Educ. Tech. 24, 330–355. doi: 10.1007/s10956-014-9545-1
Schwarz, C. V., Reiser, B. J., Davis, E. A., Kenyon, L., Achér, A., Fortus, D., et al. (2009). Developing a learning progression for scientific modeling: making scientific modeling accessible and meaningful for learners. J. Res. Sci. Teach. 46, 632–654. doi: 10.1002/tea.20311
Servedio, M. R., Brandvain, Y., Dhole, S., Fitzpatrick, C. L., Goldberg, E. E., Stern, C. A., et al. (2014). Not just a theory—the utility of mathematical models in evolutionary biology. PLoS Biol. 12:e1002017. doi: 10.1371/journal.pbio.1002017
Stemler, S. E., and Tsai, J. (2008). “Best practices in interrater reliability: three common approaches,” in Best practices in quantitative methods. ed. J. W. Osborne (Thousand Oaks, CA: Sage), 29–49.
Sweeney, L., and Sterman, J. (2007). Thinking about systems: student and teacher conceptions of natural and social systems. Syst. Dyn. Rev. 23, 285–311. doi: 10.1002/sdr.366
Taber, K. S., and García Franco, A. (2010). Learning processes in chemistry: drawing upon cognitive resources to learn about the particulate structure of matter. J. Learn. Sci. 19, 99–142. doi: 10.1080/10508400903452868
Upmeier Zu Belzen, A., Van Driel, J., and Krüger, D. (2019). “Introducing a framework for modeling competence,” in Towards a competence-based view on models and modeling in science education. eds. A. U. Zu. Belzen, J. DrielVan, and D. Krüger Cham, Switzerland: Springer 3–20.
West, G. B. (2014). A theoretical physicists journey into biology: from quarks and strings to cells and whales. Phys. Biol. 11, 1–6. doi: 10.1088/1478-3975/11/5/053013
Wilensky, U., and Jacobson, M. J. (2015). “Complex systems and the learning sciences,” in Cambridge handbook of the learning sciences. ed. K. Sawyer (New York, NY: Cambridge University Press), 319–338.
Wilensky, U., and Rand, W. (2015). An introduction to agent-based modeling. Modeling natural, social and engineered complex systems in net logo. (Cambridge MA: MIT Press).
Yoon, S. A. (2008). An evolutionary approach to harnessing complex systems thinking in the science and technology classroom. J. Learn. Sci. 30, 1–32.
Yoon, S. A. (2022). “Designing complex systems curricula for high school biology: A decade of work with the BioGraph project” in Fostering understanding of complex systems in biology education. eds. O. B. Z. Assaraf and M. C. P. Knippels (Cham: Switzerland: Springer), 227–247.
Yoon, S. A., Anderson, E., Klopfer, E., Koehler-Yom, J., Sheldon, J., Schoenfeld, I., et al. (2016). Designing computer-supported complex systems curricula for the Next Generation Science Standards in high school science classrooms. Sys. 4, 1–18.
Yoon, S. A., Anderson, E., Koehler-Yom, J., Evans, C., Park, M., Sheldon, J., et al. (2017). Teaching about complex systems is no simple matter: Building effective professional development for computer-supported complex systems instruction. Instr. Sci. 45, 99–121.
Yoon, S. A., Evans, C., Miller, K., Anderson, E., and Koehler, J. (2019b). Validating a model for assessing science teachers’ adaptive expertise with computer-supported complex systems curricula and its relationship to student learning outcomes. J. Sci. Teach. Ed. 30, 890–905.
Yoon, S. A., Goh, S., and Park, M. (2018a). Teaching and learning about complex systems in K-12 science education: A review of empirical studies 1995-2015. R. Ed. Research 88, 285–325.
Yoon, S. A., Goh, S. E., and Yang, Z. (2019a). Toward a learning progression of complex systems understanding. Complicity: J. of Complexity and Ed. 16, 1–19.
Yoon, S. A., Koehler-Yom, J., and Yang, Z. (2018b). The effects of teachers’ social and human capital on urban science reform initiatives: Considerations for professional development. Teach. Coll. Rec. 119, 1–32.
Yoon, S. A., Miller, K., and Richman, T. (2020b). Comparative study of high-quality professional development for high school biology in a face-to-face versus online delivery mode. Ed. Tch. & Soc. 23, 68–80.
Yoon, S. A., Miller, K., Richman, T., Wendel, D., Schoenfeld, I., Anderson, E., et al. (2020a). Encouraging collaboration and building community in online asynchronous professional development: Designing for social capital. J. Comp. Coll. Learn. 15, 351–371.
Keywords: complex systems, modeling, agent-based simulation, biology, metamodeling knowledge
Citation: Miller KM and Yoon SA (2023) Teaching complexity in biology through agent-based simulations: the relationship between students’ knowledge of complex systems and metamodeling knowledge. Front. Educ. 8:1198307. doi: 10.3389/feduc.2023.1198307
Edited by:
Orit Ben Zvi Assaraf, Ben-Gurion University of the Negev, IsraelReviewed by:
Watcharee Ketpichainarong, Mahidol University, ThailandKarel Vojíř, Charles University, Czechia
Annette Upmeier Zu Belzen, Humboldt University of Berlin, Germany
Copyright © 2023 Miller and Yoon. This is an open-access article distributed under the terms of the Creative Commons Attribution License (CC BY). The use, distribution or reproduction in other forums is permitted, provided the original author(s) and the copyright owner(s) are credited and that the original publication in this journal is cited, in accordance with accepted academic practice. No use, distribution or reproduction is permitted which does not comply with these terms.
*Correspondence: Katherine M. Miller, a21pbGxlckBjb25jb3JkLm9yZw==