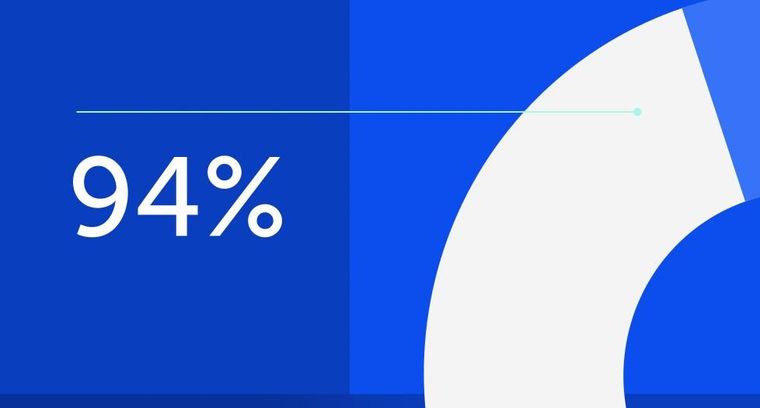
94% of researchers rate our articles as excellent or good
Learn more about the work of our research integrity team to safeguard the quality of each article we publish.
Find out more
ORIGINAL RESEARCH article
Front. Educ., 19 July 2023
Sec. Higher Education
Volume 8 - 2023 | https://doi.org/10.3389/feduc.2023.1187319
Introduction: University students are confronted with various demands that can lead to mental health problems, including depression, anxiety, or stress. One significant resource that has been shown to prevent or buffer mental health problems is social support. However, interventions enhancing students’ perception and use of social support are rare in higher education research. This study evaluates the effects of resource-oriented training following the Zurich Resource Model on students’ perception and use of social resources.
Method: Participants included 247 students from a German university who enrolled in the training program and were divided into intervention and waiting control groups.
Results: Compared to the control group, the intervention group reported higher perceived social support, increased quality of received social support, and increased seeking social support as a coping strategy four weeks after the intervention. The study results further reveal changes in support network composition in both groups over time and offer insights into the characterization of energy givers and energy eaters within one’s support network.
Discussion: The discussion concludes with implications, limitations, and directions for future research.
University students face various potentially burdensome demands from different areas, including contextual factors (e.g., financial problems, deadlines, workload; Maymon and Hall, 2021), lack of resources (Tonsing and Tonsing, 2021), or lack of university/life-balance (Pitt et al., 2018). Inadequately managed demands can lead to undesirable consequences, such as high stress levels, lower well-being, burnout, and mental health problems, including depression and anxiety (e.g., Robotham, 2008; Brown, 2018; Shankland et al., 2019). Low academic performance and even university dropout can also result (Saunders-Scott et al., 2018). Using certain resources, especially social support, can help students cope with demands and their consequences. For example, social support has been shown to be helpful in adjusting to the university context, reducing depression symptoms (Maymon and Hall, 2021), increasing motivation and performance (Eggens et al., 2008), increasing optimism and well-being (Sanagavarapu et al., 2019), and reducing stress symptoms (Pitt et al., 2018; Schmiedl et al., 2022a, 2022b). Accordingly, interventions for university students should seek to strengthen resources, especially social resources, and their use. However, despite many interventions designed to support students’ ability to deal with demands and mental health issues (e.g., Huang et al., 2018; Breedvelt et al., 2019), few scholars have addressed the strengthening and use of resources directly or have examined whether these interventions might also affect students’ social support or the use of social support as a coping strategy.
Therefore, this study aimed to illuminate possibilities for university students to enhance their resources by evaluating how resource-oriented training might affect perceived social support, individual social support networks, and students’ use of social support as a coping strategy. Our experimental design included an intervention group and a waiting control group and formed our intervention around the Zurich Resource Model (ZRM; Storch, 2004) due to the strong resource orientation of this approach.
Student demands and their emotional, psychological, and physical consequences are well-documented in higher education research. Many researchers have reported severe levels of stress (e.g., Tonsing and Tonsing, 2021), depression (e.g., Saleh et al., 2017), burnout (Robotham, 2008), anxiety (e.g., Larcombe et al., 2016; Robinson et al., 2016), and decreased well-being (Bernaras Iturrioz et al., 2018) in university students. More recently, the COVID-19 pandemic has imposed new psychological challenges, leading to acute stress disorder for many students (Ye et al., 2020).
The demands that reinforce the risk for students’ perceptions of these problems are manifold. Examples of demands include academics (e.g., exams, studying, workload; Shankland et al., 2019; Maymon and Hall, 2021), social (e.g., family-related issues, feeling pressure from family, leaving family or friends; Robotham, 2008; Tonsing and Tonsing, 2021), personal (e.g., procrastination, university/life balance, personal health; Pitt et al., 2018; Maymon and Hall, 2021), cultural (e.g., transition into the study context; Pitt et al., 2018; Sanagavarapu et al., 2019), or financial (e.g., financial concerns; Tonsing and Tonsing, 2021) issues.
The perception of high demands is not the only cause of mental health problems in university students. The lack of resources to manage those demands is another critical factor that can be a catalyst for negative emotional, psychological, and physical outcomes (e.g., Enns et al., 2018). Furthermore, following the study demands-resources (SD-R) model (Lesener et al., 2020; Salmela-Aro et al., 2022), resources can buffer the effect of demands on negative outcomes and can also have direct effects on those outcomes.
One significant and well-studied resource for students in the context of stress, mental health, and study demands is social support (for a review, see Maymon and Hall, 2021). Social support has been defined as “various forms of aid and assistance supplied by family members, friends, neighbors, and others” (Barrera, 1981, p. 69) or plainly as “resources provided by others” (Cohen and Syme, 1985, p. 4). Furthermore, social support can be differentiated in terms of the form of support (i.e., perceived versus received support), the type of support (e.g., instrumental support, emotional support) and the source of support (e.g., friends, family, colleagues) (Barrera, 1986).
Many studies report direct effects of social support on different outcomes. For example, Maymon et al. (2019) asserted that high-quality received support from staff or faculty correlated with lower stress, lower burnout, lower quitting intentions, and greater feelings of belonging and life satisfaction in first-year students. Meta-analytic results also show that students’ social support is negatively related to burnout (Kim et al., 2018). Furthermore, research has shown support from family and friends to predict lower stress (Miczo et al., 2006), better quality of life, and fewer depression symptoms (Alsubaie et al., 2019). Receiving and perceiving social support are also positively associated with better adjustment to the university context (Toews and Yazedijan, 2007) and higher subjective well-being (Zhu et al., 2013). Additionally, support can reduce negative appraisals of student demands (Kember, 2004; Schmiedl et al., 2022b). Social support has also related to stress-and demands-buffering effects. Specifically, scholars found social support to buffer against the negative effects of stress on depressive symptoms (Raffaelli et al., 2013), as well as the negative effects of stress associated with students transitioning to the university environment (Wilcox et al., 2005). On the demand level, social support can buffer the negative effects of demands on perceived stress and cortisol levels in students (Schmiedl et al., 2022a). Given these various positive effects of social support and resources for students, interventions in the study context should incorporate resource-oriented elements while emphasizing social support.
Many interventions have been designed for students or examined within a student sample targeting common mental health problems (e.g., depression, anxiety, or stress). In a meta-analysis by Breedvelt et al. (2019), effect sizes for yoga, meditation, and mindfulness-based stress reduction interventions were moderately related to depression, anxiety, and stress in students. Other meta-analytic results imply that cognitive-behavioral interventions were effective; however, other interventions (e.g., exercise, art, and peer support) had the highest effect sizes regarding depression and anxiety (Huang et al., 2018). Resource-oriented interventions (e.g., social-emotional training or coaching and self-monitoring interventions) have been shown to decrease burnout (Bresó et al., 2011), improve well-being (Schoeps et al., 2020), and reduce anxiety (Gatto et al., 2022) in students. Some studies have also included social support as a resource in their interventions. For example, Pancer et al. (2004) concluded that weekly meetings with peers and a university-provided moderator led to a better experience of adjustment to the university context. In a similar study (Cornelius et al., 2016), students who participated in an academic peer-mentoring program also described positive university-transition experiences. Furthermore, according to an evaluation study of a program designed to support STEM students by providing academic, social (through tutoring and mentoring), and financial support, students in the intervention group reported a stress-buffering effect, fewer stressors, and higher academic self-efficacy than students in the control group (McGonagle et al., 2014). Despite these valuable results from previous intervention studies, the low to moderate effect sizes reported in the meta-analyses indicate that the methods and strategies used and taught in those interventions may not fit everyone’s needs. Furthermore, existing interventions are more likely to provide resources like social support than incorporate reflection concerning one’s own resources, one’s social support network, and the use of those resources. Previous studies on stress and mental health in students have emphasized individual-level interventions as preferable to an institutional or more general level (Robotham, 2008), as well as interventions that target student coping and help-seeking (Sanagavarapu et al., 2019) and help students to reflect on their personal (social) resources (Wittner et al., 2020; Schmiedl et al., 2022a, 2022b).
One approach that can potentially link all these recommendations is the Zurich Resource Model. Accordingly, the intervention evaluated in this study was built upon this model.
The ZRM method, a self-management approach, aims to help participants activate and use their personal resources to work toward their goals and persevere, even in challenging situations (Storch and Krause, 2017). The main element of ZRM training entails developing a motivating, action-oriented personal goal and preparing to achieve it. Standard manualized ZRM training, using the Rubicon Process (Storch and Krause, 2017) as its theoretical foundation, comprises five phases. During the first phase, participants identify a currently relevant key theme significant in their lives, portrayed in a resource-activating picture which they want to work on. In our study, the participants’ theme had to be health-related. Working in groups, participants use questions and hypotheses to explore why the selected picture evokes positive responses and how it relates to the key theme (Storch, 2004). In phase two, participants develop an action-oriented personal goal based on their key theme, the picture, and motivational properties with the assistance of group members and somatic markers. Next, in phase three, the participants build up resources to enhance goal implementation and achievement (Storch and Krause, 2017). The ZRM framework defines everything activating the neuronal network connected to the goal as a resource (Storch, 2004). This phase also entails discussing reminders, goal embodiment, and the use of social resources to achieve goals. In our study, participants also reflected on specific social resources from different areas of their life (e.g., family, friends), their closeness to these resources, and the latter’s usefulness for providing support in achieving personal goals. The fourth phase involves preparing and practicing goal-oriented use of the earlier-identified resources. Working in groups, the participants discuss how to pursue goals in situations with varying difficulty while focusing on barriers, problematic behavior, or unwanted emotions. The fifth phase entails reflecting on the training contents and results and applying them in daily life (Storch and Krause, 2017).
Previous studies have reported positive effects of ZRM training. Outcomes include attenuated stress appraisals and cortisol responses in a social stress task (Storch et al., 2007), improved affect-regulation skills in patients with eating disorders (Storch et al., 2011), and increased self-efficacy and self-management (Steurer-Stey et al., 2015). However, related research focused on students remains relatively unexplored, especially regarding effects of social resources and their utilization.
This study evaluated the effects of a resource-oriented ZRM intervention addressing students’ perception and use of resources, especially social support, by encouraging the participants to identify and learn to use their own social resources with the ZRM approach. Various methods were employed, including discussing the possible use of social resources in different situations, delineating everyone’s social support network, and using the training group as a resource (e.g., in generating ideas). Accordingly, we postulated that reflecting on social resources connects to individuals’ higher perception of their social resources and exploring ways to use social resources (e.g., to cope with stress) relates to seeking social support as a coping strategy more frequently.
H1. Compared to the control group, intervention group participants’ perceived social support will increase after the training.
H2. Compared to the control group, intervention group participants will be more likely to seek social support actively after the training than before.
Some components of social support can impose detrimental effects instead of benefits. Specifically, Schmiedl et al. (2022a) noted that a large support network increased cortisol levels of students in reaction to demands. Additionally, it can be difficult to choose the right supporter within a network having a large selection of people, risking a demands-support mismatch (Ellwardt et al., 2019). Furthermore, although the persons within an individual’s support network should ideally provide support or energy (making them energy givers), some actually demand more than they give (Liang et al., 2001) or may cost energy for another reason, such as by providing unhelpful support (making them energy eaters). The ZRM intervention had students reflect on the quality of the support they received within their social support network while learning how to optimize their network composition (e.g., by strengthening energy givers and questioning energy eaters’ usefulness), aiming to decrease the proportion of energy eaters within the network, though the network might potentially become smaller in general (e.g., when low-quality providers are removed). Therefore, we hypothesized:
H3. Compared to the control group, in the intervention group members’ support networks, (a) the proportion of energy givers will increase, and (b) the proportion of energy eaters will decrease after the training.
H4. Compared to the control group, intervention group participants will report a smaller support network after the training than before.
Lastly, critical reflection on personal social support resources and subsequent changes within the network should also go along with a better received support quality from the network after the intervention.
H5. Compared to the control group, the intervention group participants’ received support quality will increase after training.
In addition, to fill a gap in the literature, we wanted to examine (1) the potential characteristics of energy givers and energy eaters within the students’ support network. Furthermore, we were also interested in (2) how the composition of the participants’ support network might change due to the intervention from pre-to post-measure.
For this study, which was part of a larger research project on student health at a German university, we offered 16 two-day training sessions over four semesters (four events per semester) for students. In all, 247 students enrolled in the training program. Four students who had participated in an earlier resource-oriented intervention were excluded from data analysis but attended the training program. We also excluded another eleven participants from the analysis because either the complete pre-questionnaire or the complete post-questionnaire was missing. The final sample comprised N = 232 students, divided into the intervention group (118 participants) and the waiting control group (114 participants). Figure 1 presents a participant flow chart and the study design.
Of the 232 participants, 50.6% were female, and 47.0% were male (2.4% missing data). Mean age was 25.31 years (SD = 2.61, ranging from 19 to 35), and most students were currently studying for their master’s degree (78.5%). Most participants were German (89.9%). Academic fields varied, including engineering sciences (62.1%), natural sciences (9.5%), technology sciences (6.7%), and two areas—transport and mobility, along with social sciences—with 6.1% each (9.5% missing data).
The intervention comprised a two-day, resource-oriented, group-based training program, with 14 to 18 participants in each group. Experienced trainers conducted the training sessions based on the ZRM training manual while focusing on social resources, as described in the Zurich Resource Model section. More specifically, on the first day, the participants went through ZRM phases one and two, meaning they identified their key theme and developed their key theme-related action-oriented goal. On the second day, they went through ZRM phases three to five, meaning they built up their resources pool to assist in goal achievement, reflected on their social resources, prepared and practiced goal-enhancing use of their resources and reflected on how to apply the training contents in daily life.
To measure perceived social support, we used the German version of the Interpersonal Support Evaluation List (ISEL; Cohen and Hoberman, 1983; Laireiter, 1996), which features 40 items (e.g., “When I need suggestions for how to deal with a personal problem, I know there is someone I can turn to.”). All items were rated on a 4-point Likert scale (1 = not true at all; 4 = exactly true). Cronbach’s α was 0.93 (pre-measure) and 0.95 (post-measure).
Seeking social support as a coping strategy was measured using the relevant subscale of the Ways of Coping Questionnaire (Folkman and Lazarus, 1988). Participants were asked to recall a specific stressful situation from the previous week and state whether they had used the described behavior in that situation (e.g., “I talked to someone about how I was feeling.”). All six items were rated on a 4-point Likert scale (0 = not used; 3 = used a great deal). Cronbach’s α was 0.76 (pre-measure) and 0.82 (post-measure).
We applied a common social network name-generating procedure (Perkins et al., 2015) by asking participants to think about their personal social support network and specify up to 20 people who somehow supported them (e.g., instrumental, emotional). We provided them with a short definition of personal social support network (persons in their environment who provided support to the participant, e.g., in stressful situations or during stressful times) to facilitate name generation. After listing the names, the participants specified different characteristics, including age, sex, type of relationship (e.g., family, friend, colleague), closeness of the relationship (1 = loose to 3 = close), contact frequency (1 = at least once a year to 5 = daily), and type of support received (e.g., emotional, professional, financial) concerning each person. For type of received support, multiple answers per listed person were possible. The support network size was indicated by the number of listed people.
We sought to determine whether each listed person was an energy giver or an energy eater by asking participants directly, “Would you describe this person as more of an energy giver or an energy eater for you?” (dummy-coded) for every person. We then calculated the proportion of energy givers and energy eaters (as a percentage) by dividing the total number of energy givers (or energy eaters) by the total number of persons listed.
Additionally, we measured received support quality by having participants estimate with a 6-point Likert scale (1 = I do not agree at all; 6 = I agree entirely) on a single item per person whether they felt optimally supported by the listed persons (for a similar approach, see Fonseca et al., 2014).
The students indicated their preferred date option for participation plus one alternative date, which we did our best to satisfy. In some cases, participants were chosen by lot if too many students chose the same participation date. Training dates were then randomly assigned to the intervention condition or the waiting control condition. All participants received comprehensive information about the study purpose, procedure, and data sampling process; each student also provided informed consent before participating. After completion of the training program, the students in both the intervention and waiting control groups received credit points. The responsible ethics committee granted study approval.
The intervention group participants completed a preliminary questionnaire (pre-measure) covering demographic data, perceived social support, and seeking social support, along with a separate support network questionnaire for social network analysis. Following the pre-measure, the intervention group attended the training intervention. Four weeks later, the participants completed a second questionnaire (post-measure) identical to the pre-measure, except for demographic data. The four-week timespan for the post-measure was chosen due to the measured outcomes; since changes in seeking social support, received social support and the social support network composition can only be visible after the participants had the chance to implement changes in their daily life and to seek for and receive social support after the training at all, the post-measure does not take place directly after the training but four weeks later.
The waiting control group completed the pre-measure questionnaires, received no intervention, then answered the post-measure questionnaires four weeks later, after which they also attended the two-day training program. Both the intervention and the control groups also filled in a daily diary on ten defined days during the four-week period after the training program to reflect on applying the training contents in their daily life. Both groups also participated in a half-day workshop four weeks after attending the training to discuss the implementation of the training learnings. The content of the daily diaries and the reflection day were not part of the current study.
All statistical analyses were conducted using SPSS version 25.0. We analyzed missing data by performing a pattern analysis of missing data (relying on our final data sample of N = 232), then examining whether data were missing at random (following Baraldi and Enders, 2010). In the first step, we identified six missing data patterns, of which is one with no variables missing (221 cases), meaning that in 11 cases, at least one variable was missing. In the next step, we performed Little’s MCAR test to determine whether variables were missing completely at random. The results were not significant (χ2 = 56.371, df = 46, p = 0.141), indicating that the variables were missing at random.
The post-training daily diaries (response rate 71.1%) were maintained between the pre-and post-measure for the intervention group. However, as they were only part of the larger research project and not part of the intervention, and because we were interested in examining only the effects of the training rather than potential diary reactivity effects (e.g., Beymer and Robinson, 2022), we controlled for completed diaries in our analyses. Therefore, to test our hypotheses, we conducted repeated measures analyses of covariance (ANCOVAs). To gain initial insights into the potential characterization of energy givers and energy eaters within the support network, we performed a correlation analysis between the characterization variables of the listed persons (age, sex, type of relationship, closeness of relationship, contact frequency, types of received support) and the rating as an energy giver or energy eater. We also compared the composition of the participants’ support network before the training program and four weeks afterward via repeated measures ANCOVAs to determine whether changes within the participants’ networks were potentially influenced by the intervention.
Our analyses revealed significant time × group interaction effects, indicating that, compared to the control group, the intervention group experienced significantly higher levels of perceived social support (F(1, 224) = 4.79, p = 0.015, η2 = 0.021), use of seeking social support as a coping strategy (F(1, 224) = 4.99, p = 0.013, η2 = 0.022), and received support quality (F(1,223) = 2.97, p = 0.044, η2 = 0.018) after participating in the training, supporting our H1, H2, and H5. A significant time × group interaction effect also emerged for support network size (F(1,223) = 8.79, p = 0.002, η2 = 0.040); specifically, the intervention group reported a greater decrease in network members than the control group, supporting our H4. Notably, the intervention group incorporated significantly more network members in the pre-measure than the control group. Regarding the proportion of energy givers and energy eaters within the network, no significant interaction effects emerged; therefore, H3 was rejected. Instead, analyses revealed main effects of time for the proportions of energy givers (F(1,221) = 4.44, p = 0.018, η2 = 0.021) and energy eaters (F(1,221) = 3.96, p = 0.024, η2 = 0.019). Specifically, the proportion of energy givers rose in both groups, while the proportion of energy eaters decreased in both groups over time. Table 1 provides an overview of the ANCOVA results.
Table 1. Means, standard deviations, t-test of independent samples for pre-measure and repeated measures analyses of covariance statistics for study variables.
Correlation analysis yielded significant positive correlations between an energy-giver rating and being a family member (r = 0.14, p = 0.033), closeness of the relationship (r = 0.32, p < 0.001), providing support in the university context (r = 0.16, p = 0.017) or in the private environment (r = 0.39, p < 0.001), and providing financial support (r = 0.22, p = 0.001), emotional support (r = 0.27, p < 0.001), or professional support (r = 0.19, p = 0.006). Furthermore, providing no support revealed a significantly negative correlation with a rating as an energy giver (r = −0.33, p < 0.001). Being rated as an energy eater had a significantly positive correlation with providing no support (r = 0.31, p < 0.001) and a significantly negative correlation with the closeness of the relationship (r = −0.32, p < 0.001), providing support in the university context (r = −0.14, p = 0.037) or in the private environment (r = −0.29, p < 0.001), and providing financial support (r = −0.21, p = 0.002), emotional support (r = −0.27, p < 0.001), or professional support (r = −0.19, p = 0.004). Table 2 displays an overview of the correlation results.
Despite the random assignment of the intervention and waiting control conditions to the training dates, according to T tests of independent samples, in the pre-measure, the intervention and control groups differed significantly in the size of the support network (t(231) = 3.52, p < 0.001), mean age of the reported persons in their network (t(221) = −2.44, p = 0.015), proportion of family members in the network (t(221) = −2.57, p = 0.011), and proportion of financial support received from the network (t(221) = −2.84, p = 0.005). Specifically, compared to the control group, the intervention group reported larger networks, a younger network demographic, and less received financial support. All other characteristics of the social support network did not differ significantly between the intervention and the control groups in the pre-measure. Table 1 displays the network composition for both groups in the pre-and post-measures.
Regarding the change in network composition due to the intervention, ANCOVA results demonstrate a significant time × group interaction effect in some descriptive network variables (see Table 1). Specifically, the mean age of network members rose in the intervention group (F(1,222) = 3.51, p = 0.031, η2 = 0.017), while the proportion of friends (F(1,222) = 6.02, p = 0.008, η2 = 0.028) and “other” contacts (F(1,222) = 2.92, p = 0.045, η2 = 0.014) within the network decreased compared to the control group. Furthermore, the proportion of contacts who provided no support decreased in the intervention group but remained relatively stable in the control group (F(1,222) = 3.44, p = 0.033, η2 = 0.016). Additionally, ANCOVA results show significant main effects of time in some network variables. Specifically, the proportion of family members increased in both groups over time (F(1,222) = 5.82, p = 0.017, η2 = 0.027), whereas the proportion of professors/teachers decreased in both groups (F(1,222) = 3.09, p = 0.040, η2 = 0.015). Furthermore, the contact frequency (F(1,222) = 34.61, p < 0.001, η2 = 0.142) and proportions of received professional support (F(1,222) = 5.58, p = 0.019, η2 = 0.026) and financial support (F(1,222) = 14.45, p < 0.001, η2 = 0.065) increased in both groups.
This study aimed to extend the research on enhancing students’ perceptions and use of resources, particularly social support, by applying an experimental approach to examine the impact of resource-oriented ZRM training on these aspects, along with their social support network composition. As expected, resource-oriented training appeared to increase the perceived support of the participants effectively, along with students’ active seeking of social support in stressful situations and support quality received from the support network. This outcome could be due to the training-based reflection regarding the students’ social support networks and how to draw from their social resources to obtain goals. Furthermore, since the ZRM method teaches specific techniques for gaining support from other people (above and beyond the reflection of one’s network and possibilities), for example, by generating ideas or maintaining a goal-supportive attitude during difficult situations (Storch and Krause, 2017), participants might have applied these methods to their everyday lives and support-seeking mechanisms. The increase in received support quality in the intervention group may further indicate that reflecting on their personal support networks and determining who could be helpful in particular situations or for certain topics might have led participants to identify better need-support matches. This possibility aligns with the social support effectiveness model (Rini and Dunkel-Schetter, 2010), which postulates that the degree of the need-support fit determines whether the received support is helpful and high in quality or potentially harmful. Furthermore, as hypothesized, a greater reduction in network size was evident in the intervention group compared to the control group, potentially due to evaluating helpful contacts during stressful times as opposed to those who were not. Research findings indicating that a large network can be stress-inducing (e.g., Schmiedl et al., 2022a) also fit our results that the received support quality, along with the perceived social support, increased in the intervention group. However, in practical terms, the two groups already differed significantly in network size in the pre-measure; thus, the network size of the intervention group tended to approach that of the control group after training. Contrary to our assumptions, over time, the proportion of energy givers increased while the proportion of energy eaters decreased in both groups, not only in the intervention group. A potential explanation for this result could be that the study survey completed by all participants included a social network questionnaire, which might itself have served as an intervention for reflection, leading participants to remove energy eaters from the network while favoring energy givers.
The second step’s exploratory analysis concerned potential characterizations of energy givers and energy eaters within the students’ support networks. Being a family member was the only type of relationship that was positively correlated with an energy-giver rating; all other types did not correlate significantly with being rated as an energy giver or energy eater (despite “others” being correlated with an energy-eater rating). This observation does not mean that friends or colleagues, for example, cannot be helpful (see also Miczo et al., 2006; Maymon et al., 2019), but other aspects of the relationship appear more relevant, especially the closeness of the relationship and that the person actually provides support. Almost all types of support (except vocational support, which was uncorrelated) correlated positively with an energy-giver rating, and providing no support correlated positively with an energy-eater rating; thus, unsurprisingly, any relevant support by a person within a support network in a student’s current situation appears to be important. Lastly, the exploratory results revealed the following changes in the intervention group’s support networks after the training: the mean age of network members rose, while the proportion of friends and “other” contacts decreased. The significant increase in mean age could be the result of fewer friends and more family members within the network, with the friends more likely to be the participant’s age, while family members (like parents or grandparents) were potentially older. An exploratory correlation analysis of age (of the listed persons in the network) and being a family member supported this assumption (r = 0.60, p < 0.001). The increase in family members in the networks of both groups may also correspond with the reported increase in financial support, as family members remain the students’ main source of financial support (e.g., Bartoszuk et al., 2021). Furthermore, the proportion of contacts who did not provide any kind of support decreased in the intervention group compared to the control group. The observed increase in family members and decrease in friends, “others,” and persons who provided no support also fits with the above-described correlations with energy givers and eaters, potentially resulting from the network-related reflection during the training. In both groups, over time, the proportion of professors/teachers decreased; nevertheless, the proportion of received professional support increased. One possible explanation could be due to the scheduling of the training during the semester, as most took place at the end of the lecture period or at the beginning of the lecture-free period, times characterized by fewer contacts with professors/teachers due to fewer events at the university. Simultaneously, professional support might have become more important for the students and was perhaps also actively sought from fellow students because of the proximity of exams. Scheduling the intervention at that time during the semester could also explain the reason for more family members in the network at the time of the post-measure, as many students might have left campus and returned home to their family during the lecture-free period.
This study acknowledges that the literature and relevant models of stress (e.g., the SD-R model) consider such resources as social support to be essential to students’ mental health. We extend the topic by describing how interventions for students can also enhance their perceptions and use of social support as a resource, which prior studies have neglected. Specifically, we add to the existing literature on the effects of interventions helping students manage demands while targeting mental health; meanwhile, we also follow calls by researchers to develop and evaluate interventions focused on student coping and reflecting on personal resources (e.g., Sanagavarapu et al., 2019; Schmiedl et al., 2022a) by showing how resource-oriented ZRM training can influence the perception and use of social support as a resource. Thus, we acknowledge the previous research on different effects and benefits of perceived and received social support (e.g., Schmiedl et al., 2022a, 2022b) by differentiating between passive (e.g., perceived support) and active (e.g., received support, seeking social support) components of social support in our evaluation. Nevertheless, both active and passive components of social support might have been influenced by our ZRM-based intervention. Furthermore, we contribute to the literature on the development of students’ social support networks (e.g., Thiele et al., 2018) by examining the dynamics of support networks and extending it by focusing on short-term changes, showing that even after four weeks, we can see significant changes in support network composition. We also account for prior researchers’ calls to include both positive and negative ties (e.g., Harrigan and Yap, 2017) in social network research, as well as affective components of network ties like closeness (Ellwardt et al., 2019), and extend the literature by examining the characteristics of positive and negative ties (i.e., energy giver and energy eater) within the context of student support. Lastly, we extend the ZRM-related research by exploring the effects on students’ perception and use of social support as a resource after participating in ZRM-based training, demonstrating the observed impact on some of the evaluated components, and thus supporting the resource-strengthening purpose of the ZRM method.
A practical implication for students is the value of reflecting on their own social support network and possible benefits (e.g., in stressful times or regarding different topics), considering the enhanced perceived social support and received support quality that emerged in our findings. Additionally, in terms of energy givers versus eaters, reflecting on the network and excluding energy eaters may not require targeted training. Implications for practice, therefore, include the potential usefulness of providing reflections in different formats, such as digital formats, without guidance in a specific intervention. As the participants’ support networks evidence changes within a short time frame, another implication for students could be the suggestion to reflect on their network and its helpfulness in different specific situations, adapting it accordingly to maximize help and support (i.e., to seek support from contacts who can provide the most helpful support in a particular situation or topic rather than always seeking support from the same persons). Additionally, many factors that are influenced by social support can be related to student dropout (e.g., Eggens et al., 2008; Saunders-Scott et al., 2018); therefore, one implication of this study for universities or other higher education institutions may be the need to offer, along with social support to students, resource-oriented interventions that encourage students to reflect on and strengthen their resources like social support to foster student mental health and possibly reduce student dropout.
This study also has several limitations to consider, which lead to further research questions. One limitation lies in the generalizability of results. Due to the unique needs of undergraduate post-secondary students in their mid-twenties, which form our sample, our results can most likely not be generalized for other samples. Furthermore, this study is missing a second follow-up due to the structure of the overall project, which restricted our results to the four-week time span and left unclear if the observed effects would persist over a longer time span. Therefore, future research should replicate our study, also with other samples, and include a second follow-up, at least. Future studies should also schedule the survey for the control group further away from training to minimize the possibility that effects in the control group are related to (mental) preparation for the training. Additionally, the data used in this study do not allow us to determine the extent to which participants applied the training insights to their daily lives, i.e., what they specifically did in the four weeks between training and post-measure. It can only be assumed, based on the differences between the intervention and waiting-control groups, that the results were due to the intervention received. Hence, future research should include detailed assessment of training content implementation and actions in daily life for the intervention group and waiting control-group in order to be able to specify the study conclusions. Lastly, our results uncovered significant but small effects. Compared to other mental health interventions in the study context (e.g., Huang et al., 2018) and another study on a ZRM intervention targeting stress responses (Storch et al., 2007), our study yielded smaller effect sizes. However, none of the studies examined effects concerning the use and perception of resources but centered around stress, anxiety, or depression. The small effect sizes in our study may also be explained by the fact that the ZRM intervention is not exclusively designed for social support; in fact, social resources are only a small component of the training.
Our study’s evaluation approach offers interesting investigational opportunities for future research. For example, in addition to established mental health interventions for students concerning the perception of outcomes like stress, depression, or anxiety, future studies might focus on the perception or use of mediators or moderators for these outcomes, like social support or other resources, as research has demonstrated the benefits of resources for many outcomes. Thus, valuable spillover effects on outcomes other than that originally examined could be detected and applied.
This study provides important findings for higher education mental health and support research. Our results indicate that a resource-oriented ZRM intervention can help students enhance their own perceived and received support and use social support as a coping strategy. We further show that support network composition can change relatively quickly and that the closeness of relationships and provided support from the network appears to determine whether students will gain or lose energy. Altogether, our results highlight the relevance of social support in the student context and offer insights into possibilities for enhancing students’ social support.
The raw data supporting the conclusions of this article will be made available by the authors, without undue reservation.
The studies involving human participants were reviewed and approved by Ethics committee of Faculty 2, TU Braunschweig (FV-2016-14). The participants provided their written informed consent to participate in this study.
AS conceived the original idea für the study, gathered the data, performed the analyses, interpreted the data, and wrote the first draft of the manuscript. SK enabled data gathering, discussed the results, and reviewed the manuscript. All authors contributed to the article and approved the submitted version.
Publication funding was provided from the TU Braunschweig publication fund.
The authors declare that the research was conducted in the absence of any commercial or financial relationships that could be construed as a potential conflict of interest.
All claims expressed in this article are solely those of the authors and do not necessarily represent those of their affiliated organizations, or those of the publisher, the editors and the reviewers. Any product that may be evaluated in this article, or claim that may be made by its manufacturer, is not guaranteed or endorsed by the publisher.
Alsubaie, M. M., Stain, H. J., Webster, L. A. D., and Wadman, R. (2019). The role of sources of social support on depression and quality of life for university students. Int. J. Adolesc. Youth 24, 484–496. doi: 10.1080/02673843.2019.1568887
Baraldi, A. N., and Enders, C. K. (2010). An introduction to modern missing data analyses. J. Sch. Psychol. 48, 5–37. doi: 10.1016/j.jsp.2009.10.001
Barrera, M. (1981). Social support in the adjustment of pregnant adolescents: assessment issues. B. H. Gottlieb (Ed.), Social networks and social support (69–96). Thousand Oaks, CA: Sage.
Barrera, M. (1986). Distinctions between social support concepts, measures, and models. Am. J. Community Psychol. 14, 413–445. doi: 10.1007/BF00922627
Bartoszuk, K., Deal, J. E., and Yerhot, M. (2021). Parents’ and college students’ perceptions of support and family environment. Emerg. Adulthood 9, 76–87. doi: 10.1177/2167696818823947
Bernaras Iturrioz, E., Insúa Cerretani, P., and Bully Garay, P. (2018). Prevalence and severity of psychological problems in university students. British J. Guidance Counsel. 46, 418–428. doi: 10.1080/03069885.2017.1286633
Beymer, P. N., and Robinson, K. A. (2022). Motivating by measuring motivation? Examining reactivity in a diary study on student motivation. Contemp. Educ. Psychol. 70:102072. doi: 10.1016/j.cedpsych.2022.102072
Breedvelt, J. J. F., Amanvermez, Y., Harrer, M., Karyotaki, E., Gilbody, S., Bockting, C. L. H., et al. (2019). The effects of meditation, yoga, and mindfulness on depression, anxiety, and stress in tertiary education students: a meta-analysis. Front. Psych. 10:193. doi: 10.3389/fpsyt.2019.00193
Bresó, E., Schaufeli, W. B., and Salanova, M. (2011). Can a self-efficacy-based intervention decrease burnout, increase engagement, and enhance performance? A quasi-experimental study. Higher Education. Diabetologia 61, 339–355. doi: 10.1007/s10734-010-9334-6
Brown, J. S. L. (2018). Student mental health: some answers and more questions. J. Ment. Health 27, 193–196. doi: 10.1080/09638237.2018.1470319
Cohen, S., and Hoberman, H. M. (1983). Positive events and social supports as a buffer of life change stress. J. Appl. Soc. Psychol. 13, 99–125. doi: 10.1111/j.1559-1816.1983.tb02325.x
Cohen, S., and Syme, S. L. (1985). Issues in the study and application of social support. S. Cohen and S. L. Syme (Eds.), Social support and health (3–22). Cambridge: Academic Press.
Cornelius, V., Wood, L., and Lai, J. (2016). Implementation and evaluation of a formal academic-peer-mentoring programme in higher education. Act. Learn. High. Educ. 17, 193–205. doi: 10.1177/1469787416654796
Eggens, L., van der Werf, M. P. C., and Bosker, R. J. (2008). The influence of personal networks and social support on study attainment of students in university education. High. Educ. 55, 553–573. doi: 10.1007/s10734-007-9074-4
Ellwardt, L., Wittek, R., Hawkley, L., and Cacioppo, J. (2019). Social network characteristics and their associations with stress in older adults: closure and balance in a population-based sample. J. Gerontol. B 75, 1573–1584. doi: 10.1093/geronb/gbz035
Enns, A., Eldridge, G. D., Montgomery, C., and Gonzalez, V. M. (2018). Perceived stress, coping strategies, and emotional intelligence: a cross-sectional study of university students in helping disciplines. Nurse Educ. Today 68, 226–231. doi: 10.1016/j.nedt.2018.06.012
Folkman, S., and Lazarus, R.S. (1988). Ways of coping questionnaire : Research Edition. Palo Alto, CA: Consulting Psychologists Press.
Fonseca, A., Nazaré, B., and Canavarro, M. C. (2014). The role of satisfaction with social support in perceived burden and stress of parents of six-month-old infants with a congenital anomaly: actor and partner effects. J. Child Health Care 18, 178–191. doi: 10.1177/1367493513485478
Gatto, A. J., Miyazaki, Y., and Cooper, L. D. (2022). Help me help myself: examining an electronic mental health self-monitoring system in college students. High. Educ. 83, 163–182. doi: 10.1007/s10734-020-00646-8
Harrigan, N., and Yap, J. (2017). Avoidance in negative ties: inhibiting closure, reciprocity, and homophily. Soc. Networks 48, 126–141. doi: 10.1016/j.socnet.2016.07.003
Huang, J., Nigatu, Y. T., Smail-Crevier, R., Zhang, X., and Wang, J. (2018). Interventions for common mental health problems among university and college students: a systematic review and meta-analysis of randomized controlled trials. J. Psychiatr. Res. 107, 1–10. doi: 10.1016/j.jpsychires.2018.09.018
Kember, D. (2004). Interpreting student workload and the factors which shape students’ perceptions of their workload. Stud. High. Educ. 29, 165–184. doi: 10.1080/0307507042000190778
Kim, B., Jee, S., Lee, J., An, S., and Lee, S. M. (2018). Relationships between social support and student burnout: a meta-analytic approach. Stress. Health 34, 127–134. doi: 10.1002/smi.2771
Larcombe, W., Finch, S., Sore, R., Murray, C. M., Kentish, S., Mulder, R. A., et al. (2016). Prevalence and socio-demographic correlates of psychological distress among students at an Australian university. Stud. High. Educ. 41, 1074–1091. doi: 10.1080/03075079.2014.966072
Lesener, T., Pleiss, L. S., Gusy, B., and Wolter, C. (2020). The study demands-resources framework: an empirical introduction. Int. J. Environ. Res. Public Health 17:5183. doi: 10.3390/ijerph17145183
Liang, J., Krause, N. M., and Bennett, J. M. (2001). Social exchange and well-being: is giving better than receiving? Psychol. Aging 16, 511–523. doi: 10.1037/0882-7974.16.3.511
Maymon, R., and Hall, N. C. (2021). A review of first-year student stress and social support. Soc. Sci. 10:472. doi: 10.3390/socsci10120472
Maymon, R., Hall, N. C., and Harley, J. M. (2019). Supporting first-year students during the transition to higher education: the importance of quality and source of received support for student well-being. Stud. Success 10, 64–75. doi: 10.5204/ssj.v10i3.1407
McGonagle, A. K., Freake, H. C., Zinn, S., Bauerle, T., Winston, J., Lewicki, G., et al. (2014). Evaluation of STRONG-CT: a program supporting minority and first-generation U.S. science students. Journal of STEM. Education 15, 52–61.
Miczo, N., Miczo, L. A., and Johnson, M. (2006). Parental support, perceived stress, and illness-related variables among first-year college students. J. Fam. Commun. 6, 97–117. doi: 10.1207/s15327698jfc0602_1
Pancer, S. M., Pratt, M. W., Hunsberger, B., and Alisat, S. (2004). Bridging troubled waters: helping students make the transition from high school to university. Guid. Counsel. 19, 184–190.
Perkins, J. M., Subramanian, S. V., and Christakis, N. A. (2015). Social networks and health: a systematic review of sociocentric network studies in low-and middle-income countries. Soc. Sci. Med. 125, 60–78. doi: 10.1016/j.socscimed.2014.08.019
Pitt, A., Oprescu, F., Tapia, G., and Gray, M. (2018). An exploratory study of students’ weekly stress levels and sources of stress during the semester. Act. Learn. High. Educ. 19, 61–75. doi: 10.1177/1469787417731194
Raffaelli, M., Andrade, F. C. D., Wiley, A. R., Sanchez-Armass, O., Edwards, L. L., and Aradillas-Garcia, C. (2013). Stress, social support, and depression: a test of the stress-buffering hypothesis in a Mexican sample. J. Res. Adolesc. 23, 283–289. doi: 10.1111/jora.12006
Rini, C., and Dunkel-Schetter, C. (2010). The effectiveness of social support attempts in intimate relationships. K. Sullivan and J. Davila (Eds.), Support processes in intimate relationships (26–67). Oxford: Oxford University Press
Robinson, A. M., Jubenville, T. M., Renny, K., and Cairns, S. L. (2016). Academic and mental health needs of students on a Canadian campus. Can. J. Couns. Psychother. 50:61100.
Robotham, D. (2008). Stress among higher education students: towards a research agenda. High. Educ. 56, 735–746. doi: 10.1007/s10734-008-9137-1
Saleh, D., Camart, N., and Romo, L. (2017). Predictors of stress in college students. Front. Psychol. 8:19. doi: 10.3389/fpsyg.2017.00019
Salmela-Aro, K., Tang, X., and Upadyaya, K. (2022). Study demands-resources model of student engagement and burnout. A.L. Reschly and S.L. Christenson (Eds.), Handbook of research on student engagement (77–93). Basel: Springer International Publishing
Sanagavarapu, P., Abraham, J., and Taylor, E. (2019). Development and validation of a scale to measure first year students’ transitional challenges, wellbeing, help-seeking, and adjustments in an Australian university. High. Educ. 77, 695–715. doi: 10.1007/s10734-018-0298-2
Saunders-Scott, D., Braley, M. B., and Stennes-Spidahl, N. (2018). Traditional and psychological factors associated with academic success: investigating best predictors of college retention. Motiv. Emot. 42, 459–465. doi: 10.1007/s11031-017-9660-4
Schmiedl, A., Schulte, E.-M., and Kauffeld, S. (2022a). The demands-buffering role of perceived and received social support for perceived stress and cortisol levels. Eur. J. Health Psychol. 29, 175–186. doi: 10.1027/2512-8442/a000110
Schmiedl, A., Schulte, E.-M., and Kauffeld, S. (2022b). Integrating appraisal processes in the study demands-resources framework - a diary study. Anxiety Stress Coping 36, 444–459. doi: 10.1080/10615806.2022.2117306
Schoeps, K., de La Barrera, U., and Montoya-Castilla, I. (2020). Impact of emotional development intervention program on subjective well-being of university students. High. Educ. 79, 711–729. doi: 10.1007/s10734-019-00433-0
Shankland, R., Kotsou, I., Vallet, F., Bouteyre, E., Dantzer, C., and Leys, C. (2019). Burnout in university students: the mediating role of sense of coherence on the relationship between daily hassles and burnout. High. Educ. 78, 91–113. doi: 10.1007/s10734-018-0332-4
Steurer-Stey, C., Storch, M., Benz, S., Hobi, B., Steffen-Bürgi, B., Steurer, J., et al. (2015). Motivational training improves self-efficacy but not short-term adherence with asthma self-management: a randomized controlled trial. Prim. Health Care Res. Dev. 16, 32–41. doi: 10.1017/S1463423613000480
Storch, M. (2004). Resource-activating self-management with the Zurich resource model (ZRM). Eur. Psychother. 5, 27–64.
Storch, M., Gaab, J., Küttel, Y., Stüssi, A.-C., and Fend, H. (2007). Psychoneuroendocrine effects of resource-activating stress management training. Health Psychol. 26, 456–463. doi: 10.1037/0278-6133.26.4.456
Storch, M., Keller, F., Weber, J., Spindler, A., and Milos, G. (2011). Psychoeducation in affect regulation for patients with eating disorders: a randomized controlled feasibility study. Am. J. Psychother. 65, 81–93. doi: 10.1176/appi.psychotherapy.2011.65.1.81
Storch, M., and Krause, F. (2017). Selbstmanagement – ressourcenorientiert: Grundlagen und Trainingsmanual für die Arbeit mit dem Zürcher Ressourcen Modell (ZRM®) (6th). Göttigen: Hogrefe.
Thiele, L., Atzmueller, M., Stumme, G., and Kauffeld, S. (2018). Frequent and/or durable? The predictive impact of initial face-to-face contacts on the formation and evolution of students’ developmental peer network relationships. Psychology 9, 633–654. doi: 10.4236/psych.2018.94040
Toews, M. L., and Yazedijan, A. (2007). College adjustment among freshmen: predictors for white and Hispanic males and females. Coll. Stud. J. 41, 891–901.
Tonsing, K. N., and Tonsing, J. C. (2021). A mixed-method study of stress and coping strategies among university social work students in the United States. Soc. Work. Educ. 41, 1222–1238. doi: 10.1080/02615479.2021.1942445
Wilcox, P., Winn, S., and Fyvie-Gauld, M. (2005). ‘It was nothing to do with the university, it was just the people’: the role of social support in the first-year experience of higher education. Stud. High. Educ. 30, 707–722. doi: 10.1080/03075070500340036
Wittner, B., Barthauer, L., and Kauffeld, S. (2020). Accessibility and mobilisation of social Capital in First-Generation Students’ social networks—a mixed-methods approach. J. Coll. Stud. Retent. Res. Theory Pract. 5:152102512097964. doi: 10.1177/1521025120979643
Ye, Z., Yang, X., Zeng, C., Wang, Y., Shen, Z., Li, X., et al. (2020). Resilience, social support, and coping as mediators between COVID-19 related stressful experiences and acute stress disorder among college students in China. Appl. Psychol. Health Well Being 12, 1074–1094. doi: 10.1111/aphw.12211
Keywords: university students, social support, ZRM, intervention, resources, students’ well-being
Citation: Schmiedl A and Kauffeld S (2023) The positive effects of resource-oriented training on students’ perception and use of social support. Front. Educ. 8:1187319. doi: 10.3389/feduc.2023.1187319
Received: 15 March 2023; Accepted: 05 July 2023;
Published: 19 July 2023.
Edited by:
Wendy Peia Oakes, Arizona State University, United StatesReviewed by:
Lindsie Spengler, Arizona State University, United StatesCopyright © 2023 Schmiedl and Kauffeld. This is an open-access article distributed under the terms of the Creative Commons Attribution License (CC BY). The use, distribution or reproduction in other forums is permitted, provided the original author(s) and the copyright owner(s) are credited and that the original publication in this journal is cited, in accordance with accepted academic practice. No use, distribution or reproduction is permitted which does not comply with these terms.
*Correspondence: Annika Schmiedl, YS5zY2htaWVkbEB0dS1icmF1bnNjaHdlaWcuZGU=
Disclaimer: All claims expressed in this article are solely those of the authors and do not necessarily represent those of their affiliated organizations, or those of the publisher, the editors and the reviewers. Any product that may be evaluated in this article or claim that may be made by its manufacturer is not guaranteed or endorsed by the publisher.
Research integrity at Frontiers
Learn more about the work of our research integrity team to safeguard the quality of each article we publish.