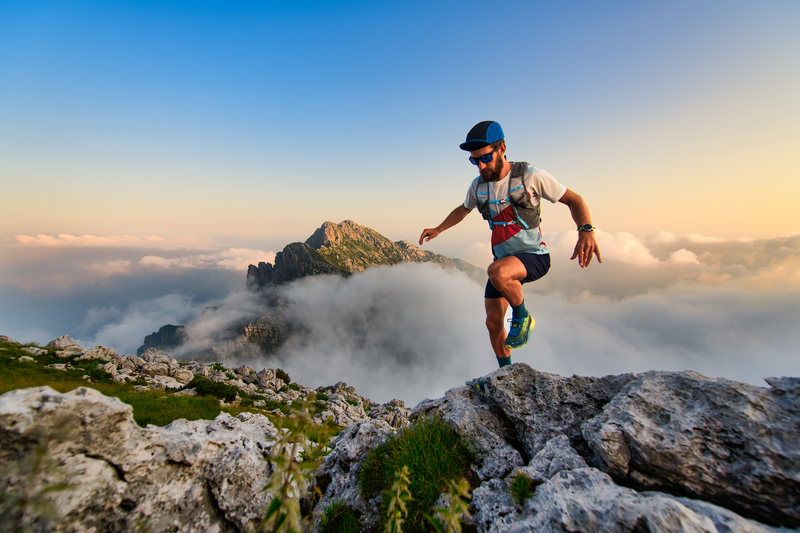
95% of researchers rate our articles as excellent or good
Learn more about the work of our research integrity team to safeguard the quality of each article we publish.
Find out more
ORIGINAL RESEARCH article
Front. Educ. , 22 November 2023
Sec. Higher Education
Volume 8 - 2023 | https://doi.org/10.3389/feduc.2023.1187251
Introduction: The first part of the worldwide lockdown starting in March 2020 forced teachers in higher education to implement emergency remote teaching (ERT) in an online learning environment. Some students appreciated the autonomy they acquired and the appeal to their self-discipline. Other students, preferring structure and guidelines, perceived these new learning circumstances as ambiguous and unclear. Pressing circumstances, such as a pandemic forcing students into a new learning environment, pose a challenge to their academic motivation. On that premise, this study suggests that the sudden change of learning environment following ERT report an impact on the fulfillment of the basic psychological needs of learners and consequently, on their motivation. More concretely, we hypothesized that this new learning environment had a demotivating effect on students' motivation. The central question in this research is therefore “To what extent did students' motivation shift with ERT.”
Methods: This embedded mixed method study, where one dataset (qualitative) plays a secondary role in a study that is primarily based on the other dataset (quantitative), consists of two measurement points: academic motivation was measured among students from the Royal Military Academy (RMA), before the WHO's declaration of the pandemic (T1, December 2019) and during the pandemic (T2, June 2020). To measure autonomous motivation, we used the Learning Self-Regulation Questionnaire (SRQ-L). To measure satisfaction and frustration for autonomy, relatedness, and competence, we used the Psychological Needs Satisfaction and Frustration Scale (BPNSFP). To identify new issues not captured in the closed questions, the survey at T2 included a qualitative second part with one open-ended question.
Results: When comparing pre-pandemic to pandemic academic motivation results by a repeated-measure analysis, we found that the first college year students' motivation was the most negatively affected, followed by that of the second college year students. In addition, by using a multiple regression, we found that ERT affected perceived competence suggesting that lower perceived competence contributes to a lower academic motivation.
Discussion: Based on these results, this study underlines the importance of assessing learners' sense of competence before immersing them into an online learning environment or changing their learning environment in any other way. This study implies that higher education teachers should provide students with the necessary knowledge to use self-regulatory strategies, encourage self-discipline to improve learning outcomes and endorse a role of helpful coach with the possibility of interaction. By doing so, higher education teachers can meet students' basic needs and can keep autonomous motivation as high as possible among learners. This study adds to the literature insights that can help to optimize educational practices and set up classroom-wide interventions during teacher training so that teachers can facilitate these skills among their students.
The COVID-19 crisis disrupted education worldwide. The WHO declared the spread of the disease as a pandemic on 11 March 2020 (Sohrabi et al., 2020; World Health Organization, 2020). For global containment and quarantine efforts, many governments around the world closed their educational institutions temporarily to curb the spread of the virus (Babbar and Gupta, 2022). Teachers and students across the globe faced an exceptional challenge (Watermeyer et al., 2021; Babbar and Gupta, 2022; McGaughey et al., 2022). Teachers had to switch their lessons to an online form in no time; students took lessons from home through online learning. Many scholars have referred to switching very quickly from traditional classroom teaching to online and distance education as emergency remote teaching (ERT) (e.g., Ferri et al., 2020; Hodges et al., 2020; Huang and Wang, 2022).
ERT is a kind of online instruction delivered in pressing circumstances, which contrasts with deliberate and well-planned online learning education (Daniel, 2020; Hodges et al., 2020; Murphy, 2020; Huang and Wang, 2022). In other words, ERT is a temporary shift from education to an alternative form of instruction due to crisis conditions. The primary goal in these circumstances is to provide temporary access to instruction and instructional support. Teachers, students, and institutions were not able to prepare fully for effective remote education in terms of infrastructure, mindset, curriculum construction, and pedagogy (Nguyen et al., 2021; Watermeyer et al., 2021; Babbar and Gupta, 2022). The rapid approach required for ERT may come at the expense of the quality of the courses delivered and may have an impact on student motivation. Saykili (2018, p. 5) presented online learning education as “a form of education which brings together the physically distant learner(s) and the facilitator(s) of the learning activity around planned and structured learning experiences via various two or multi-way mediated media channels that allow interactions between/among learners, facilitators as well as between learners and educational resources.”
Albuquerque et al. (2022) identified strengths and weaknesses in higher education during ERT. Comfort and time management were mentioned as positive aspects, whereas assessments, interaction, and self-confidence were mentioned as negative aspects during ERT.
In this study, academic motivation was measured among students from the Royal Military Academy (RMA), a Belgian university, once before the WHO's declaration of the pandemic (December 2019) and once during the pandemic (June 2020). These RMA students faced the same challenges and obstacles as other higher education students besides having to conform to military life (Kelly et al., 2014). The students suddenly had to leave their usual learning environment, where structure and control apply, for an individual autonomic learning environment, where self-discipline applies. During the pandemic, a more autonomously regulated learning environment was introduced, in the form of ERT learning: students needed to appeal more to their self-discipline to decide when and how to study (autonomy) and find new ways to relate to their peers (relatedness) and to feel that they had learned effectively (competence). McGaughey et al. (2022) linked positive and negative aspects to online teaching and reported, for example, that online teaching can have a potential for greater autonomy if more flexible working agreements are maintained but can negatively impact student learning outcomes and competence when teachers, for example, do not have digital skills. A challenge for online teaching is to cultivate relatedness.
Pressing circumstances force students into a new learning environment and create a challenge to accomplish motivation. On that premise, several researchers have looked into the motivation of students during ERT. Different researchers reported a decrease in motivation due to ERT (e.g., Aguilera-Hermida, 2020; Oliveira et al., 2021; Yates et al., 2021). However, those studies were cross-sectional and none of them compared the students' level of motivation before ERT and during ERT, raising questions about the temporal relationship between these pressing circumstances and the decrease in motivation. We also hypothesized that this new learning environment due to ERT had a demotivating effect on students' motivation. Our research question was as follows: “To what extent did students' motivation shift with ERT?” In doing so, we wanted to explore what part did basic psychological needs have in this motivational shift. To the best of our knowledge, the present study is the first longitudinal one on this subject.
Within this research, two main constructs, the learning environment and motivation, are used, each with its theoretical framework.
The learning environment is a broad concept to define (Abualrub et al., 2013). Getzels and Thelen (1960) discovered that interactions of students' personal needs and expectations with their classroom environment determine students' behavior and outcomes. Adams and Granic (2009) defined the learning environment as the created conditions to improve the learning experience.
In the past, researchers considered student motivation as a purely personal student attribute (e.g., Goodenow and Grady, 1993; Dai et al., 1998). Nowadays, researchers recognize the impact of external influences on motivation, such as the role of the family or the teachers (e.g., Zepke, 2011; Malik, 2015). Therefore, current researchers study motivation from an ecological perspective, considering the student's interaction with the learning environment (e.g., Anderson et al., 2004; Mercer, 2019). Within the learning environment for each individual student, motivation is something that evolves over time and varies depending on the situation (Arnold, 2014). Students' perception of the learning environment is also an important aspect as objective support from the environment is subjectively interpreted (Arnold, 2014).
According to Moos (1974, 2002), the learning environment is a psychosocial situation with three dimensions of experience: the relationship dimension, the growth dimension, and the change dimension. The relationship dimension defines the quality of the personal relationships in the learning environment and relates to aspects such as personal commitment to others, attachment to the group, mutual support, and cooperation between people in a social environment. In other words, the relationship dimension pertains to the interactions between students and teachers, and between students. The growth dimension includes the way in which the learning environment encourages personal development and the level of control the students have over their own learning. The change dimension relates to the clarity of expectations and rules in the learning environment, the differentiation between teaching methods, the approach to the evaluation of competencies, etc. Teachers can build a supportive classroom climate by appealing to students' sense of efficacy, student connections to others, and students' choices (Stolk et al., 2018, October). We choose the dimensional framework of Moos (1974) as it closely aligns with the SDT contextual framework (Deemer and Smith, 2018).
The self-determination theory (SDT; Deci and Ryan, 2000) has added value within the academic educational context (Reeve, 2002; Niemiec and Ryan, 2009; Guay, 2022) and highlights the processes of motivation within the learning environment (Deemer and Smith, 2018). The SDT puts motivation on a continuum, ranging from amotivation to autonomous motivation, with controlled motivation in between. The level of motivation will steer behavior, such as students' activities to learn, develop their competencies, and succeed in their academic curriculum. The SDT describes different types of regulations, that is, the process by which a motive is bound to a given behavior.
Amotivation is the absence of any form of motivation, action-orientation, and positive feelings about a given behavior (Vallerand et al., 1992). Amotivation is different from demotivation. Amotivation means that there is no contingency between certain behavior and outcomes (Deci and Ryan, 1985), while demotivation occurs when someone was once motivated to show a given behavior but has had a negative experience that reduced his/her motivation (Kiziltepe, 2008). For example, while an individual can have absolutely no interest in ever learning a second language, another individual confronted with the obligatory learning of a second language in high school may develop motivation to succeed, but later experience demotivation to further engage in the studies of language and literature in higher education. According to Howard et al. (2021), amotivation was associated with poor performance.
Students engaged in each curriculum experience both controlled motivation and autonomous motivation for the courses they take. Controlled motivation is determined by external factors (external regulation) and by internal pressure (introjected regulation). With controlled motivation, the reason why a student learns is rather instrumental, for example, to avoid a punishment or to get a reward; to avoid shame or to feel proud. This implies that other people, e.g., teachers, or what the student thinks other people would think, are at the origin of this form of motivation. Controlled motivation can lead to good performance and success, but this type of regulation disappears when the extrinsic motivators are gone (Van den Broeck et al., 2008). Introjected motivation was only weakly predictive of persistence and performance goals (Howard et al., 2021).
Autonomous motivation exists when one identifies his/her behavior with a personal value (identified regulation) and when one associates his/her behavior with interest, competence (integrated regulation), and enjoyment (intrinsic regulation) (Vansteenkiste et al., 2020). Both intrinsic and identified regulations were related to higher student performance; identified regulation was particularly important for persistence (Howard et al., 2021). With autonomous motivation, a student puts effort into his/her work because of an internal source of energy. As a result, other people such as teachers have less impact on this form of motivation. Autonomous motivation is the most successful form of motivation to predict student achievement, engagement, and self-esteem (Howard et al., 2020, 2021) through which we wish to pursue this within education.
Furthermore, SDT is a process theory based on the idea that basic psychological needs are the source of all human behaviors. The concept of basic psychological needs is, according to Deci and Ryan (2000), universal and must be satisfied to guarantee healthy functioning among students (Deci and Ryan, 2000, 2012). This theory distinguishes three basic needs: the need for autonomy, the need for competence, and the need for relatedness. The need for autonomy concerns a subjective experience of psychological freedom and choice (Deci and Ryan, 2000; Vansteenkiste et al., 2020) and stands for the desire of students to tackle freely their learning assignments without perceived compulsion. The need for competence is the need to interact effectively with the environment (Deci and Ryan, 2000; Vansteenkiste et al., 2020) and expresses the desire of students to be good at something and to be impactful in a given knowledge domain. The need for relatedness is the desire to feel connected to others (Deci and Ryan, 2000; Vansteenkiste et al., 2020) and steer a course for students to interact with students and teachers. The satisfaction of these basic psychological needs nurtures autonomous motivation for education and learning and gives students the opportunity to enhance their quality of motivation (e.g., Minnaert et al., 2008, 2011; Jang et al., 2009; Stolk et al., 2018). The basic psychological needs (Deci and Ryan, 2000; Vansteenkiste et al., 2020) align closely with the dimensions of experience (Moos, 1974, 2002): the relationship dimension with the need for relatedness, the growth dimension with the need for competence, and the change dimension with the need for autonomy (Deemer and Smith, 2018). This relates to how the learning environment influences motivation through the satisfaction of basic needs. According to Cayubit (2021), the learning environment influences the motivation of students, or put another way, the learning environment influences motivation through basic need experiences.
In summary, the SDT suggests that a supportive learning environment that meets all three basic needs (need for autonomy, competence, and relatedness) will nurture students' motivation (Niemiec and Ryan, 2009; Huang and Wang, 2022). In other words, the better basic needs are satisfied, the more one evolves toward behaviors associated with interest, personal value, and pleasure. On the other hand, a student will experience stress and a feeling of instability when the three basic needs are not satisfied (Bartholomew et al., 2018). Put differently, the more basic needs are unsatisfied, the more behavior is determined by external factors and internal pressure.
The learning environment is one of the most important factors of learning that affects the motivation to learn (Wang et al., 1990). Students are more likely to experience positive outcomes when the learning environment responds to their needs (Gutman and Eccles, 2007).
Previous studies (e.g., Fraser, 1998; Pai et al., 2014) show that the learning environment positively affects a range of student outcomes (Cayubit, 2021; Howard et al., 2021; Guay, 2022). Student outcomes can range from identifying own strengths and weaknesses to acquiring new abilities. A change in the learning environment can influence motivation in several ways.
Creating a learning environment in which teachers support autonomy is important to the needs' fulfillment and hence students' motivation (Bureau et al., 2021; Guay, 2022). A supporting autonomy—learning environment is characterized by a high degree of structure and a low level of control (Sarrazin et al., 2006). Standage et al. (2003) have made a distinction between a free learning environment and a controlled learning environment and showed that a controlled learning environment characterized by a high degree of structure and a high level of control (Sarrazin et al., 2006) is detrimental to motivation. Accordingly, a free learning environment characterized by a high level of liberty (Sarrazin et al., 2006), possibly characterizing online learning, should boost the satisfaction of the need for autonomy and should have a positive role on autonomous motivation (Standage et al., 2003). When students experience support for their need for autonomy, students behave with greater autonomy in the educational environment (Guay and Vallerand, 1996).
The need for competence is the most predictive of self-determined motivation (Levesque-Bristol et al., 2020; Bureau et al., 2021) and is associated with positive educational outcomes. The students' perceived learning outcomes in online learning depend on a teacher's competence in online teaching (Liu et al., 2022). Teachers were not able to prepare fully in terms of pedagogy during ERT (Nguyen et al., 2021; Watermeyer et al., 2021; Albuquerque et al., 2022). Students whose teachers have a high level of pedagogical knowledge instill better academic performance and a higher level of motivation in their students (Liu et al., 2022). Consequently, confronted with a teacher during ERT, who does not fully possess the pedagogical competencies for online teaching may instill weaker learning outcomes and can have a demotivating effect on the student. Online learning requires other specific skills for students, such as asking thoughtful questions to make the subject matter more comprehensible (Roper, 2007). From the learner's point of view, this involves self-regulatory behavior to instill mastery of skills. Self-regulation plays an essential role here in instilling competence in a subject matter (Nokelainen et al., 2017). Students could thus benefit when teachers are engaged in knowledge- and skill-based programs by showing how to support competence.
Although the need for relatedness associates weaker with motivation, making a connection with fellow students remains a positive factor in explaining motivation (Bureau et al., 2021). To accomplish the need for relatedness, teachers and students can find it difficult to communicate clearly on a subject matter through online platforms, and less spontaneous contacts between students in an online learning environment are possible (Tichavsky et al., 2015; Aguilera-Hermida, 2020; Holzer et al., 2021). Hence, the need for relatedness could be less well satisfied in an online learning environment, which can have a negative role on autonomous motivation.
Existing studies report different challenges of online learning compared with traditional in-class learning (e.g., Aguilera-Hermida, 2020; Holzer et al., 2021; Scherer et al., 2021). On the one hand, in an online learning environment, students generally have more autonomy for completing their day program (Lewis et al., 2014; McGaughey et al., 2022), but on the other hand, they must be able to demonstrate at certain points in time what they have processed in a certain subject matter (McGaughey et al., 2022). This appeals to their sense of autonomy. For many students, online learning requires new learning skills, such as time or data management (Broadbent, 2017), because it is a completely new way of learning for them (Livingston and Condie, 2006) and may negatively impact their sense of competence (McGaughey et al., 2022). Some may probably feel separated from their fellow students, but at the same time closer to their families (Muilenburg and Berge, 2005). This appeals to their sense of relatedness. A meta-analysis showed that competence is the most predictive of self-determined motivation, followed by autonomy, and then by relatedness (Bureau et al., 2021). The question is now whether these findings also apply within an online learning environment during ERT.
Hence, in this study, we hypothesized that changing drastically the learning environment by ERT affected students' satisfaction of their basic psychological needs and, in turn, their academic motivation (see Figure 1). In addition, we wanted to explore which characteristics and/or psychological needs affected students' motivation the most. In a sample of students from the RMA, we measured autonomous motivation before and during ERT, and the satisfaction of their basic needs during ERT. We also asked them to report their subjective experience of ERT with an open question during ERT. In other words, does ERT push a motivational shift on students' motivation before and during ERT, and if this is so, what part do basic psychological needs have in this motivational shift? Using a longitudinal study, we aim to answer these questions. Based on literature and existing research (e.g., Aguilera-Hermida, 2020; Bureau et al., 2021; Holzer et al., 2021), we predicted an overall negative impact on autonomous motivation as, despite more perceived autonomy, students would experience less perceived competence and relatedness.
Figure 1. ERT has an effect on basic psychological needs satisfaction that in turn affects motivation.
We invited all students to participate, but we only used data from students who participated in both measurements (T1 n = 200; T2 n = 249; both n = 155) of this longitudinal study. Participants were male (n = 127) or female students (n = 28), French-speaking (n = 74) or Dutch-speaking (n = 81), those who followed the social and military sciences curriculum (SMS) (n = 104) or the polytechnics one (ENG) (n = 51), and those in one of the following college years: first year (BA1; n = 84), second year (BA2; n = 48), third year (BA3; n = 23).
A particular feature of the RMA is the language aspect as a cultural difference, which can affect motivation (Kuśnierz et al., 2020). We choose to include both curricula because the content of the curriculum as the learning environment is quite different, and the initial motivation to choose a curriculum can differ (Skatova and Ferguson, 2014). We included the different college years because students' motivations differ throughout their academic paths (Rizkallah and Seitz, 2017). Social and military sciences lean toward human behavior sciences (Goldenberg, 2022); another name for polytechnics is civil engineering sciences. The higher education programs in Belgium are expressed in credits, in accordance with the European Credit Transfer System (ECTS). A bachelor program comprises 180 ECTSs, and a master's program comprises at least 60 ECTS. Here, the social and military sciences curriculum (SMS) counts 60 ECTSs and the polytechnics one (ENG) counts 120 ECTSs (OECD, n.d.). Only the bachelor students are included in this study. Belgium, and thus also the RMA, is a full member of the Bologna process (EHEA, n.d.).
In this study, we used an embedded mixed method (Behmanesh et al., 2020), where one dataset (qualitative) plays a secondary role in a study that is primarily based on the other dataset (quantitative) (Creswell et al., 2003). An advantage specific to this design is that this one can be used when a researcher does not have sufficient time or resources to commit to extensive qualitative data collection because the quantitative data type was given priority (Creswell and Plano Clark, 2011). In general, the strength of incorporating the qualitative component is that it increases the validity and reliability of the results of quantitative analyses (Leal et al., 2018). This study comprised two parts. The first part assessed motivation with only closed questions. The second part assessed motivation and the satisfaction of basic needs by a quantitative approach and explored the students' perceptions and experiences toward new issues that were not captured in the first part. Here, we used quantitative and qualitative approaches, including closed and open-ended questions.
The research was conducted according to the ethical rules presented in the General Ethical Protocol of the Faculty of Psychology and Educational Sciences of Ghent University and the Faculty of Social and Military Sciences of the Royal Military Academy. We invited the 303 college students of the RMA to participate in a survey regarding their academic motivation. The invitation and the link to the questionnaires were distributed to all RMA students by e-mail through their supervisors, once before the WHO's declaration of the pandemic (T1, December 2019) and once during the pandemic (T2, June 2020). In Belgium, the federal government decided to suspend all physical classes from 13 March at midnight (Decision Belgian Government, 2020) to 18 May 2020. Participation in the survey was voluntary, informed consent was provided and signed by the participants, and the students could withdraw their participation in the study at any time. The questionnaire at T1 included the SRQ-L and was implemented in Google Forms; the questionnaire at T2 included the SRQ-L, the BPNSFP, and the open-ended question and was implemented in the learning management system of the RMA (ILIAS®). Both surveys included an attention question to check whether the participants completed the questionnaires carefully: “Please mark number 2 if you have read this question carefully” (Postiaux, 2017). The response rate was 66.01% at T1 and 82.18% at T2. In this study, 155 students completed the questionnaires at T1 and T2. Only the data of those 155 students were used in the analyses.
To measure autonomous motivation, we used the Learning Self-Regulation Questionnaire (SRQ-L). The SRQ-L is a well-used and validated questionnaire. We downloaded this questionnaire from the Centre for Self-Determination Theory (CSDT) and operationalized motivation from the SDT framework. The SRQ-L was specifically developed for university students and addresses the motives behind three major aspects of behavior related to studying: participating actively in classes, following the teacher's instructions, and reasons to continue broadening skills. The questionnaire was slightly adapted to the context of the Royal Military Academy and was translated into Dutch and French, using the “translation-back translation method” of Douglas and Craig (2007). The SRQ-L consists of 14 items that participants rated with a Likert scale ranging from totally disagree (1) to totally agree (7). The SRQ-L contains two subscales: one measuring controlled motivation and one measuring autonomous regulation. An item measuring controlled motivation is, for example, “I will participate actively in the classes because the others would think bad of me if I did not do it.” An item that gauges autonomous motivation is “I will participate actively in the classes because I feel it is a good way to improve my knowledge and skills in order to become a good officer.” The Relative Autonomy Index (RAI) (Black and Deci, 2000) offers a direct measure of motivational autonomy and is a dependent variable in this study. This RAI score will be used to assess whether motivation has decreased from T1 to T2. We calculated the RAI by first averaging the items of the respective subscales to compute scores in autonomous and controlled motivation and then subtracting the mean of the items of controlled motivation from the mean of the items of autonomous motivation. Accordingly, the RAI score theoretically ranges from −6 to +6 with 0 as the midpoint where controlled motivation and autonomous motivation are even. In our sample, the effective range was −1.14 to 3.14 for RAI at time 1 (T1) and−0.29 to 3.00 for RAI at time 2 (T2). In previous studies, the RAI predicted autonomous or self-determined behavior for example (e.g., Ryan and Connell, 1989; Williams and Deci, 1996; Black and Deci, 2000). In the context of this study, the Cronbach alpha was 0.86 for RAI at time 1 (T1) and 0.85 for RAI at time 2 (T2), which means that the internal consistency was good in our sample.
To measure satisfaction and frustration with the satisfaction of the needs for autonomy, relatedness, and competence, we used the Psychological Needs Satisfaction and Frustration Scale (BPNSFP) (Chen et al., 2015). This questionnaire, specifically designed for university students, has been validated in previous studies (e.g., Campbell et al., 2015; Van der Kaap-Deeder et al., 2015; Chevrier and Lannegrand, 2018) and operationalizes the basic psychological needs from the basic psychological need theory (Vansteenkiste et al., 2020). The BPNSFP was already available in Dutch and French (Chen et al., 2015; Chevrier and Lannegrand, 2018). The questionnaire includes 24 items. Examples of items are “I feel a sense of choice and freedom in the things I undertake,” “I feel that the people I care about also care about me,” and “I feel confident that I can do things well” measuring the satisfaction of autonomy, relatedness, and competence, respectively. Examples of items are “Most of the things I do feel like “I have to,” “I feel excluded from the group I want to belong to,” and “I have serious doubts about whether I can do things well” measuring the frustration with the need for autonomy, relatedness, and competence, respectively. When answering this questionnaire, participants evaluated the satisfaction and frustration of their basic needs during ERT by comparing it to their experiences during traditional class education, using a Likert scale ranging from less than in the RMA (1) to more than at the RMA (5). To compute the score on these six components (autonomy satisfaction, autonomy frustration, relatedness satisfaction, relatedness frustration, competence satisfaction, and competence frustration), we averaged the items per component and then rescaled the score into a −2 to +2 scale for the sake of interpretation (see also Boeije et al., 2011; Verboord, 2014; Mariani et al., 2016). For the global basic needs satisfaction score (BPNSFP), we first calculated each basic need by the average of the satisfaction and the frustration component and used it as a dependent variable in this study. Only then, we determined the global basic needs score by averaging the three basic needs scores. In this research, we used the three components (autonomy, relatedness, and competence) to enquire if motivation at T2 is affected by the satisfaction of basic needs. The Cronbach's alpha was 0.74 for autonomy, 0.81 for relatedness, 0.87 for competence, and 0.87 for the global basic needs satisfaction scores, which means that the internal consistency is good except for autonomy for which the internal consistency is acceptable.
The survey at T2 included one open-ended question to elaborate responses to the closed questions of the SRQ-L and BPNSFP and allow respondents to identify new issues not captured in the closed questions (O'Cathain and Thomas, 2004). The open-ended question is used to confirm answers to closed questions, detect possible problems with certain questions, and help understanding and interpreting quantitative results, thereby increasing the validity and reliability of the results (O'Cathain and Thomas, 2004). The open question read: “Do you have any comments or suggestions on the topic of the questionnaire?” and 74 students (47.7%) answered it. The length of the answer was on average 36.63, and ranged from 07 to 112 words.
All data analyses were performed using the IBM SPSS program (version 26.0), with a significance level of p < 0.05. Our approach counted three phases. First, the properties of the variables were explored. Second, a repeated-measure ANOVA was used to test the hypotheses. The two hypotheses are as follows: (a) autonomous motivation is higher at T1 than at T2 and (b) autonomous motivation (T1-T2) is different depending on the college year. We have two dependent measurements (at T1 and T2). The independent variables are as follows: (a) TIME (T1 vs. T2) and (b) Year (BA1 vs. BA2 vs. BA3); the dependent variables are as follows: (a) RAI and (b) BPNSFP. We controlled for (a) faculty (SMS vs. ENG), (b) language (Dutch speaking vs. French speaking), and (c) sex (male vs. female) to establish the correlation or relationship between our variables because they may influence the results of our study. To determine which differences were the most relevant, we calculated the effect size using Cohen's d (Cohen, 1994). The general guidelines for interpreting the effect size are as follows: 0.2 is a small effect, 0.5 is a moderate effect, and 0.8 is a large effect (Sullivan and Feinn, 2012). Third, to determine the effect of one (or more) explanatory variable(s), such as the need for autonomy, competence, and relatedness on a dependent variable such as RAI at T2, we used a regression analysis. In this way, motivation at T2 is affected by the satisfaction of basic needs. A regression analysis was performed to examine the relation between year and the satisfaction of the need autonomy, the need competence, and the need relatedness.
For the analysis of the content of the responses to the open-ended question, we tailored our approach on the three steps to O'Cathain and Thomas (2004): (1) reading a subset of the comments; (2) assigning a coding frame to describe the thematic content of the comments; and (3) assigning a selected code to all comments. The main researcher first read the comments and then used a coding frame to describe the thematic content of the comments into three headings corresponding to the three basic needs (autonomy, relatedness, and competence). She assessed whether the comment was associated positively or negatively with the basic need (negative vs. positive). Finally, she sought a suitable scholarly term for a meaningful representation of the written answer. For example, the comment: “Many teachers have replaced their assessment with assignments, and I have found these very rewarding” was coded “competence,” “positive,” and used a meaningful representation of the “assessment.”
In sum, we used an embedded mixed-methods study. For the quantitative part, academic motivation was measured among students from the RMA, before the WHO's declaration of the pandemic (T1, December 2019) and during the pandemic (T2, June 2020). To measure autonomous motivation, we used the Learning Self-Regulation Questionnaire (SRQ-L). To measure satisfaction and frustration with the need for autonomy, relatedness, and competence, we used the Psychological Needs Satisfaction and Frustration Scale (BPNSFP). For the qualitative part, to identify new issues not captured in the closed questions, the survey at T2 included one open-ended question. We executed a repeated-measure analysis to compare pre-pandemic to pandemic academic motivation results. We used a multiple regression to measure the contribution of each basic need to academic motivation. To analyze the content of the open-ended question, we applied thematic content analysis (O'Cathain and Thomas, 2004).
The descriptive statistics and bivariate latent correlations between the key variables in this study can be found in Table 1. This shows a drop in the mean for RAI2 compared with the mean of RAI1 and thus a decrease in autonomous motivation, as well as a drop in competence (shown by the negative sign), whereas autonomy and relatedness are above zero meaning. As expected, the components (autonomy, relatedness, and competence) show a small to strong significant correlation. The correlation between BPNSFP and RAI2 and between RAI2 and competence is significant.
Table 1. Descriptive statistics and bivariate latent correlations and Cronbach Alpha (α) for RAI1, RAI2, BPNSFP, AUTO, RELA, and COMP.
The repeated-measure analysis shows that motivation was higher at T1 than at T2 [F(1, 149) = 14.5; p < 0.05], independent of gender [F(1, 149) = 1.9; p > 0.05], faculty [F(1, 149) = 2.9; p > 0.05], language [F(1, 149) = 2.8; p > 0.05], but dependent on year [F(1, 149) = 4.85; p < 0.05]. Cohen's d indicates a large effect of time (d = 1.20), a large effect of year for BA1 (d = 0.87), and a medium effect of year for BA2 (d = 0.65) on autonomous motivation. A large Cohen's d of time and year for BA1 on autonomous motivation indicates that the mean difference is large compared with the variability and that the mean difference is moderate compared with the variability of year for BA1 on autonomous motivation. Figure 2 shows the drop in motivation when comparing the period pre-ERT to the period during ERT.
Figure 2. Level of motivation for college year students before and during ERT. 1BA, First College Year; 2BA, Second College Year; 3BA, Third College Year.
We conducted a regression analysis with the components (autonomy, relatedness, and competence) of BPNSFP (T2) on RAI2 (T2). A regression model with dependent variable Y = autonomous motivation at T2 and p = 3 predictors (X1 = autonomy, X2 = relatedness, X3 = competence) was analyzed. The equation of this regression model is then Y (RAI2) = α + β1 Autonomy + β2 Relatedness + β3 Competence + u. The starting point of the regression line is the intercept α, the different βs are the regression coefficients, and u is the error, meaning that part of the dependent variable cannot be explained by the explanatory variable. Multiple regression with RAI2 as the dependent variable and the components as the explanatory variable was significant [F(3, 154) = 4.14 p < 0.05]. The BPNSFP component model explains 8% variance in RAI2, and this contribution is significant. Entering the three explanatory variables in one block, we found a significant effect for competence [t(154) = 2.82; p < 0.05], a marginally significant effect for autonomy [t(154) = 1.10; p = 0.051], and no significant effect for relatedness [t(154) = −0.71; p > 0.05]. The component competence of BPNSFP contributes most to explaining the variance in autonomous motivation and thus predicting motivation, followed by the component autonomy (tendency).
A second regression model was analyzed, here with dependent variable Y = component competence of BPNSFP and p = 1 predictor (X1 = College Year). The equation of this regression model is then Y (Comp) = α + β1 College Year + u. Regression analysis showed that there was a significant effect of college year on competence [t(154) = 2.14; p < 0.05]. The year component model explains 3% in the variance of competence, and this contribution is significant.
As already mentioned, students' perception of the learning environment is an important aspect as the objective support from the environment is subjectively interpreted (Arnold, 2014). This implies that students also subjectively interpret the satisfaction and frustration of their basic needs and motivation.
For autonomy, we found two coding frames, of which one is positively related and one is negatively related to this basic need. Students reported that agency, understood as free choice in learning and the possibility of more efficient use of time, was the most effective in increasing the satisfaction of their need for autonomy. Not being able to compensate with self-discipline when there is a lack of structure, understood as a rationale where meaningful choices were possible, was the most effective in decreasing the satisfaction of their need for autonomy. For relatedness, we found one coding frame positively related and two coding frames negatively related to this basic need. Students perceived the lack of teachers' support as a lack of teacher engagement, and the shortfall of social interaction with fellow students as negative to fulfill their need for relatedness. Positive to fulfill their need for relatedness is the opportunity to interact online with the teacher. For competence, we found three coding frames, of which one is positively and two negatively related to this basic need. The fact that some teachers replaced evaluations with assignments contributed to increased satisfaction of their need for competence, but a lack of digital skills among some teachers and weakly developed learning skills among students decreased the satisfaction of their need for competence.
Combining the quantitative and qualitative results in the context of this embedded mixed-methods design, we propose that the competence component contributes most to predicting autonomous motivation. This is presumably due to the fact that teachers weakly developed learning skills among students and lacked digital skills. The component autonomy contributes the second most to predicting motivation. This is probably due to the inability of the students to compensate for the lack of structure with self-discipline. Offering skills training to higher education teachers to provide a rationale for requested self-discipline, learning strategies, and structure to furnish competence and autonomy support among learners can promote autonomous motivation. Through a classroom-wide intervention study, these practical implications can be empirically tested in future research.
When comparing measures from December 2019 to June 2020 among college students from the RMA, we found a drop in motivation. This reduced motivation may be due to the abrupt introduction of online learning (ERT) as a result of the COVID-19 pandemic. This drop in motivation was more marked in the first-year college students, followed by the second-year college students. As predicted, our results suggest that this drop is mainly associated with a reduction in the satisfaction of the need for competence. Indeed, during ERT, students had to rely more on their own learning skills, learning skills that first- and second-year college students do not yet fully possess and that may explain the bigger decrease in autonomous motivation in the first 2 years.
According to Biggs (2003) Presage–Process–Product model (3P model) of student learning, motivation is a personal attribute of the student interacting with the situational characteristics of the learning environment that can have a direct influence on students' approaches to learning (Han and Ellis, 2020). Students confronted with the uncertainty of the first year in higher education, in times of online learning, probably use an external regulation in their studies, have a need for more teacher guidance, and simply do what the teachers tell them to do (Duchatelet and Donche, 2019; Velde et al., 2021). According to Cayubit (2021), students prefer a learning environment that offers opportunities for interaction with teachers, which provides them with the opportunity to manage their time and resources aligned with the teacher's guidelines. If the teacher is not present due to ERT, the satisfaction of the need for competence may fade away, and motivation drops (Pascarella et al., 1981; Patrick et al., 2011; Cayubit, 2021).
When the learning environment supports the relationship dimension by fulfilling students' need for relatedness, the growth dimension by fulfilling their need for competence, and the change dimension by fulfilling their need for autonomy, this will nurture their autonomous motivation for their own education (e.g., Minnaert et al., 2008, 2011; Jang et al., 2009). Students can perceive the learning environments in many different ways (e.g., Fraser et al., 1996; Bureau et al., 2021). Negative student perceptions can contribute to negative outcomes such as low motivation to learn (Maltby and Whittle, 2000). This is also true for the online learning environment (e.g., Tichavsky et al., 2015; Han and Ellis, 2020), and the mean scores and correlations used in our study should mask the fact that a considerable proportion of students were negatively affected by ERT. Not only can students perceive the learning environment subjectively differently, but the learning environment can also be objectively very different. Not every student has a personal study space; some have siblings playing around, while others have an isolated individual studio with a fast internet connection (Aguilera-Hermida, 2020; Dietrich et al., 2020; Turchi et al., 2020). All of these aspects can affect the fulfillment of students' basic needs in online learning.
In our analyses, the change in the satisfaction of the need for competence was the best predictor of motivation during ERT. In the first college year, we found the highest proportion of students whose satisfaction of the need for competence was negatively affected. This may suggest that a lower satisfaction of the need for competence contributes to a lower academic motivation, more than a lower satisfaction of the need for autonomy. As shown by Levesque-Bristol et al. (2020), the need for competence is the most important need for students.
Hartnett (2015) indicated that the lack of support for learners' need for competence undermines autonomous motivation in an online context. When students are competent in a particular matter, they also feel that they have more control over the subject matter (Connell and Wellborn, 1991; Deci and Ryan, 2000), which we can relate to academic self-efficacy (Robbins et al., 2004; Kemp et al., 2019). Albelbisi and Yusop (2019) explained that self-regulated students exhibit effective positive motivation and academic self-efficacy concerning their learning processes.
In the answers to the open question, students indicated that online learning allowed classical evaluation—i.e., reproductive testing—was replaced by assignments—productive testing, which in their view ensured better alignment with the course objectives. Evaluations take a central place in students' lives (determining the amount of information learned, the efforts to learn, ranking students, etc.) (Bureau et al., 2021).
Students perceived negatively the fact that some teachers did not even give online classes and that some teachers lacked digital skills, which was seen as an educational challenge (Aguilera-Hermida, 2020; Ali, 2020; Ferri et al., 2020). It should be noted, however, that students have higher expectations of the teachers' technical and affective roles (Sason et al., 2022). Teachers have the ongoing goal to educate students to be self-directed students (Aguilera-Hermida, 2020). The students could not compensate for these lacked digital skills due to their own lack of learning skills. Through the literature review and in this study, we can cite the importance of satisfying the need for competence in order to instill autonomous motivation in traditional classes and in online teaching.
We found a marginally significance for the effect of the satisfaction of the need for autonomy on autonomous motivation. This effect tends to be in line with the results of Bureau et al. (2021) and those of Vasconcellos et al. (2020) where the need for autonomy is subordinate to the need for competence in predicting academic motivation. Research suggests that when teachers provide the necessary guidelines and feedback, they are perceived by students as autonomy-supportive (Noels et al., 2003; Sierens et al., 2009) whether within a traditional class or in online learning education. Sierens et al. (2009) found that structure, but not autonomy, provides students with the necessary knowledge to use self-regulatory strategies.
In the answers to the open question, students indicated that the lack of self-discipline affected their sense of perceived autonomy, but that agency enhanced their sense of autonomy. Costa and McCrae (1992) define self-discipline “as a capacity to begin tasks and follow through to completion despite boredom or distractions.” Online learning offers more freedom for learners but also requires more self-discipline. Aguilera-Hermida (2020) reported concentration as the biggest situational and environmental challenge for students because there are many distractors during online learning education. For that reason, self-discipline is highly important to improve learning outcomes (e.g., a better understanding of the subject matter) in the online learning environment (Gorbunovs et al., 2016). As mentioned in the research of Aguilera-Hermida (2020), the RMA students interpreted agency as a positive aspect of online learning education, in particular, because of the extra time with family and for personal improvement or hobbies.
We did not find any significant effect of the satisfaction of the need for relatedness on autonomous motivation. Furthermore, several students said in their answer to the open question that the situation did not affect their sense of relatedness. For example, they never felt excluded from the group to which they wanted to belong, neither during their lessons at the RMA nor during ERT. Our results tend to confirm Bureau et al. (2021) results that students' need for relatedness is not as important for students' motivation as the other basic needs in an educational context. According to Minnaert et al. (2011), the teacher as a helpful instructor, which students perceive as a related teacher, was more important in nurturing motivation for online learning.
In the answers to the open question, we found that the lack of social interaction and exchange of ideas can negatively influence their perceived sense of relatedness. The way students perceive their learning environment and their interaction with their teachers and peers has an influence on their motivation and engagement in school (Ryan and Patrick, 2001; Nguyen et al., 2021). Aguilera-Hermida (2020) also reported major educational challenges in the online environment (tiredness of the screen and lack of internet connection), the lack of supporting resources, and difficulties in communication. Although the satisfaction of relatedness does not play a large role in the motivation of students as the other basic needs in an educational context, we must pay attention to the possibility of interaction so that no frustration arises.
Overall, our findings presented in this study are consistent with those of the meta-analysis of Bureau et al. (2021) in which competence is the most positive predictor of self-determined motivation, followed by autonomy and then relatedness. This also means that the participants of this study, the RMA students, do not differ in this aspect from other students studied within the meta-analysis of Bureau et al. (2021).
The insights of these studies can help to optimize higher education teacher training and educational practices to foster autonomous motivation among learners through the satisfaction of basic needs in an online learning environment. Primarily, professional development of higher education teachers should focus on competence support. Teachers should provide structure by designing activities and responsibilities so that mastery can be the dominant experience. When higher education teachers replace evaluations with assignments, they contribute to increased satisfaction of students' need for competence and make students more likely to reply to feedback than evaluations (Van den Broeck et al., 2021). Furthermore, efforts should be made to improve higher education teachers' digital skills, as they provide scaffolding for active learning and skills acquisition among learners (Van den Broeck et al., 2021). When higher education teachers are engaged to show how to support competence could benefit students (Guay, 2022). Second, higher education professional development training should focus on autonomy support by fostering ownership and agency. In other words, it is important that teachers instill a rationale where meaningful choices are possible and requested behavior as self-discipline can be instilled (Van den Broeck et al., 2021). Interventions designed to professionally develop higher education teachers on how to support needs for autonomy result in higher perceptions of autonomy support (Reeve et al., 2004; Guay, 2022). Third, in terms of relatedness support, higher education professional development training should support an attitude of supportive involvement characterized by the dedication of time, attention, and resources, which is important for learner needs satisfaction and thus motivation. Satisfaction with the need for relatedness through high involvement helps students develop their potential (Guay, 2022).
This study is unique as it measured motivation before and after the WHO declaration of the COVID-19 pandemic and the subsequent lockdown of higher education. It provides us with an indication of the impact of a suddenly changed learning environment on autonomous motivation. Although we did measure autonomous motivation at two points in time, we did not administer the Psychological Need Satisfaction and Frustration Scale (BPNSFP) at time 1 of this study and there is no point of comparison for this measurement. Therefore, speculations about the causal role of reduced satisfaction of basic psychological needs in the motivation drop should be taken with caution. In the same line of thought, we acknowledge that the drop in motivation can have been the result of other variables (e.g., a time-related drop of motivation in the course of an academic year) or third variables explaining simultaneously the drop in the satisfaction of basic needs and the drop in motivation (e.g., examinations with higher stakes in June compared with December). However, we can compare our results against those found in the meta-analysis of Bureau et al. (2021) where competence is the most positive predictor of self-determined motivation, followed by autonomy and then by relatedness.
The repeated-measure analysis shows that motivation was higher at T1 than at T2, independent of gender, faculty, and language, but dependent on the year. Although we acknowledge that not everything is controlled, we have arguments to cautiously suggest that the drop in motivation was related to ERT.
Although both times of measurements are marked by a good response rate, unusual in this study is that more participants took part at time 2 than at time 1. The explanation for these data may be multiple. For example, in times of online learning, participants can perceive taking part in a study as a form of social interaction. Maybe, students had the pressing need to provide feedback about ERT and the way teachers dealt with it, explaining why almost half of the participating students answered the open question. Another explanation might be that they completed the survey because they were already working on their computer anyway. This can be an important indication that students did experience ERT as a change in their learning environment.
This study of motivation gauges the academic motivation and not the military motivation. This allows us to extend our findings to civil higher education and universities to a certain extent. We acknowledge that the military life of cadets brings specific demands; cadets also adapt to military life, physical training, and leadership development (Juhary, 2015). Despite the students of the RMA mask the same challenges as other higher education students, our results can be difficult to generalize to the general student population as our sample is overly composed of male students. In terms of academic motivation, challenge, social recognition, and competition motivate male students more than female ones, which are experiences more difficult to instill in an online learning environment. Male students were, according to Aristovnik et al. (2020), also more negative toward online learning, whereas Flores et al. (2021) and Albuquerque et al. (2022) found no significant difference related to gender in terms of perception regarding online learning. Bureau et al. (2021) indicated that male students have a stronger need to satisfy the need for competence, which is thus more difficult to instill in an online learning environment and may be the main reason for the found drop in motivation.
The systematic interpretation of the studied phenomenon has always been an aspect of subjectivity and reflexivity in qualitative research. Although the use of different assessors is not common in behavioral science research, it would have been a strength as it ensures inter-rater reliability by calculating Cohen's Kappa, the degree of agreement between different assessors (Cohen, 1994). On the other hand, although we did not calculate inter-coder reliability, qualitative interpretations were discussed among the researchers and authors of this manuscript.
Even though the “emergency aspect” of ERT will no longer occur in the near future, given that the quick switch from traditional classroom teaching to online education is now made, it is important for higher education to take the findings of this study into account when integrating well developed online learning into their curricula. For example, in this study, the first year of college needs more structure (Hornstra et al., 2016); we suggest paying more attention to the structure of online learning courses.
Many organizations and higher education have to convert classes into online learning courses (Saykili, 2018). Based on our results, we underscore the importance of assessing the learners' sense of competence before immersing them too abruptly into an online learning environment. According to Kosycheva and Tikhonova (2021), higher education offering online learning education should encourage students to recognize their existing abilities and knowledge and help them gain confidence in their own abilities. We also suggest assessing students' transversal competencies (e.g., digital literacy, time management, and data management) before allowing them in an online environment so that any remediation and assistance can be instilled.
This research identified the need for learning which can improve teachers' knowledge about learner engagement with online material. Teachers must be available or need more time to communicate with students in order to meet the students' need for relatedness but must also provide feedback and clear guidelines in order to meet the students' need for autonomy. By focusing on structure, teachers meet the students' need for competence. All this requires a skilled teacher, both pedagogically and digitally, which implies that higher education also needs to invest in appropriate training for teachers (Esdar et al., 2016; Yates et al., 2021).
Our results suggest a mediation effect of the satisfaction of the basic psychological needs between the learning environment and motivation. We recommend future researchers to test more explicitly a mediation hypothesis according to which the online learning environment affects motivation through the satisfaction of basic needs. Online learning implies a new learning environment and thus a possible impact on the motivation of students. Additionally, it would also be interesting to explore the learning outcomes in the online learning environment with the premise that the learning environment has an impact on motivation through the mediation of basic needs.
We found a drop in motivation from December 2019 to June 2020 possibly due to the sudden introduction of ERT. This drop in motivation was more marked in the first-year college students, followed by the second-year college students. Students confronted with the uncertainty of the first year in higher education could not compensate for their lack of learning skills and probably use an external type of regulation in tackling their studies (Williams and Hellman, 2004). In addition, we found that ERT did affect perceived competence, more specifically in the first and second years of college. This may suggest that lower perceived competence is associated with lower academic motivation.
The first theoretical contribution of these findings is that ERT marked a drop in motivation, which we could determine by the longitudinal design of this study. A second theoretical contribution of these findings is that they tend to be in line with the findings of Bureau et al. (2021) on meta-analysis where competence is the most positive predictor of self-determined motivation, followed by autonomy and then relatedness during ERT. The lack of support for learners' need for competence undermines autonomous motivation in an online context.
Students need social interactions during online learning so that they can manage their time and resources aligned with the teacher's guidelines and feedback. According to Flores et al. (2021), teacher support can be the strength of online learning. In this manner, higher education teachers can support the basic psychological needs in an online learning environment and foster the use of self-regulatory strategies, ownership, and self-discipline. Salas Velasco (2014) indicated that during online learning, higher education should focus extra on transversal competence acquisition for students through exercises, assignments, reflection, and digital literacy for teachers (2014).
To keep the autonomous motivation as high as possible among learners, higher education teacher training should focus on competence, autonomy, and relatedness support. Competence support can be instilled by learning how to provide structure by designing activities and responsibilities so that mastery can be instilled by giving supportive feedback and by acquiring digital skills. Autonomy support can be instilled through fostering ownership and designing exercises and assignments in such a way that students have to claim their self-discipline. Relatedness support can be set in place by showing supportive involvement.
Despite the results of this study, which are in line with the meta-analysis of Bureau et al. (2021), the population of this study, RMA students, is overly composed of male students. Therefore, it may be more difficult to generalize to the general student population.
In summary, these study results can help to optimize higher education teacher training and educational practices to foster autonomous motivation among learners through the satisfaction of basic needs in an online learning environment. Future research could focus on exploring the effects of a class-wide intervention with a focus on competence, autonomy, and relatedness support during online learning on students' motivation to test theoretical insights against the practice.
The raw data supporting the conclusions of this article will be made available by the authors, without undue reservation.
AL contributed to conception and design of the study, organized the database, performed the statistical analysis, and wrote the first draft of the manuscript. RV and SL contributed to manuscript revision, read, and approved the submitted version. All authors contributed to the article and approved the submitted version.
The authors declare that the research was conducted in the absence of any commercial or financial relationships that could be construed as a potential conflict of interest.
All claims expressed in this article are solely those of the authors and do not necessarily represent those of their affiliated organizations, or those of the publisher, the editors and the reviewers. Any product that may be evaluated in this article, or claim that may be made by its manufacturer, is not guaranteed or endorsed by the publisher.
The Supplementary Material for this article can be found online at: https://www.frontiersin.org/articles/10.3389/feduc.2023.1187251/full#supplementary-material
Abualrub, I., Karseth, B., and Stensaker, B. (2013). The various understandings of learning environment in higher education and its quality implications. Q. High. Educ. 19, 90–110. doi: 10.1080/13538322.2013.772464
Adams, R., and Granic, A. (2009). “Cognitive learning approaches to the design of accessible e-learning systems,” in Cognitive and Emotional Processes in Web-Based Education: Integrating Human Factors and Personalization. (London: IGI Global), 209–228.
Aguilera-Hermida, A. P. (2020). College students' use and acceptance of emergency online learning due to COVID-19. Int. J. Educ. Res. Open 1, 100011. doi: 10.1016/j.ijedro.2020.100011
Albelbisi, N. A., and Yusop, F. D. (2019). Factors influencing learners' self–regulated learning skills in a massive open online course (MOOC) environment. Turkish Online J. Dist. Educ. 20, 1–16. doi: 10.17718/tojde.598191
Albuquerque, F., dos Santos, P. G., and Martinho, C. (2022). Strengths and weaknesses of emergency remote teaching in higher education from the students' perspective: the portuguese case. Front. Edu. 7, 871036. doi: 10.3389/feduc.2022.871036
Ali, W. (2020). Online and remote learning in higher education institutes: a necessity in light of COVID-19 pandemic. Higher Educ. 10, 3. doi: 10.5539/hes.v10n3p16
Anderson, A., Hamilton, R. J., and Hattie, J. (2004). Classroom climate and motivated behavior in secondary schools. Learn. Environ. Res. 7, 211–225. doi: 10.1007/s10984-004-3292-9
Aristovnik, A., Keržic, D., Ravšelj, D., Tomaževic, N., and Umek, L. (2020). Impacts of the COVID-19 pandemic on life of higher education students: a global perspective. Sustainability 12, 8438. doi: 10.3390/su12208438
Babbar, M., and Gupta, T. (2022). Response of educational institutions to COVID-19 pandemic: an inter-country comparison. Policy Fut. Educ. 20, 469–491. doi: 10.1177/14782103211021937
Bartholomew, K. J., Ntoumanis, N., Mouratidis, A., Katartzi, E., Thøgersen-Ntoumani, C., Vlachopoulos, S., et al. (2018). Beware of your teaching style: a school-year long investigation of controlling teaching and student motivational experiences. Learn. Instr. 53, 50–63. doi: 10.1016/j.learninstruc.2017.07.006
Behmanesh, F., Bakouei, F., Nikpour, M., and Parvaneh, M. (2020). Comparing the effects of traditional teaching and flipped classroom methods on midwifery students' practical learning: the embedded mixed method. Technol. Know. Learn. 12, 1–10. doi: 10.1007/s10758-020-09478-y
Black, A. E., and Deci, E. L. (2000). The effects of instructors' autonomy support and students' autonomous motivation on learning organic chemistry: a self-determination theory perspective. Sci. Educ. 84, 740–756. doi: 10.1002/1098-237X(200011)84:6<740::AID-SCE4>3.0.CO;2-3
Boeije, H. R., van Wesel, F., and Alisic, E. (2011). Making a difference: towards a method for weighing the evidence in a qualitative synthesis. J. Eval. Clin. Prac. 17, 657–663. doi: 10.1111/j.1365-2753.2011.01674.x
Broadbent, J. (2017). Comparing online and blended learner's self-regulated learning strategies and academic performance. Int. High. Educ. 33, 24–32. doi: 10.1016/j.iheduc.2017.01.004
Bureau, J. S., Howard, J. L., Chong, J. X., and Guay, F. (2021). Pathways to student motivation: a meta-analysis of antecedents of autonomous and controlled motivations. Rev. Educ. Res. 92, 46–72. doi: 10.3102/00346543211042426
Campbell, R., Vansteenkiste, M., Delesie, L. M., Mariman, A. N., Soenens, B., Tobback, E., et al. (2015). Examining the role of psychological need satisfaction in sleep: a self-determination theory perspective. Pers. Ind. Diff. 77, 199–204. doi: 10.1016/j.paid.2015.01.003
Cayubit, R. F. O. (2021). Why learning environment matters? An analysis on how the learning environment influences the academic motivation, learning strategies and engagement of college students. Learn. Environ. Res. 12, 1–19. doi: 10.1007/s10984-021-09382-x
Chen, B., Vansteenkiste, M., Beyers, W., Boone, L., Deci, E. L., Van der Kaap-Deeder, J., et al. (2015). Basic psychological need satisfaction, need frustration, and need strength across four cultures. Motiv. Emot. 39, 216–236. doi: 10.1007/s11031-014-9450-1
Chevrier, B., and Lannegrand, L. (2018). “Emerging adult self-perceptions in freshman year: Role of basic psychological needs satisfaction and frustration,” in SSEA Thematic Conference: Self and Identity in Emerging Adulthood.
Cohen, J. (1994). The earth is round (p < 0. 05). Am. Psychol. 49, 997. doi: 10.1037/0003-066X.49.12.997
Connell, J. P., and Wellborn, J. G. (1991). “Competence, autonomy, and relatedness: A motivational analysis of self-system processes,” in Self Processes and Development, eds M. R. Gunnar and L. A. Sroufe (New York, NY: Lawrence Erlbaum Associates, Inc), 43–77.
Costa, P. T., and McCrae, R. R. (1992). The five-factor model of personality and its relevance to personality disorders. J. Pers. Disorders 6, 343–359. doi: 10.1521/pedi.1992.6.4.343
Creswell, J. W., and Plano Clark, V. L. (2011). Choosing a mixed methods design. Desig. Cond. Mixed Methods Res. 2, 53–106.
Creswell, J. W., Plano Clark, V. L., Gutmann, M. L., and Hanson, W. E. (2003). Advanced mixed methods research designs. Handb. Mixed Methods Soc. Behav. Res. 209, 209–240.
Dai, D. Y., Moon, S. M., and Feldhusen, J. F. (1998). Achievement motivation and gifted students: a social cognitive perspective. Educ. Psychol. 33, 45–63. doi: 10.1207/s15326985ep3302&3_1
Daniel, S. J. (2020). Education and the COVID-19 pandemic. Prospects 21, 1–6. doi: 10.1007/s11125-020-09464-3
Deci, E. L., and Ryan, R. M. (1985). The general causality orientations scale: self-determination in personality. J. Res. Pers. 19, 109–134. doi: 10.1016/0092-6566(85)90023-6
Deci, E. L., and Ryan, R. M. (2000). The “what” and “why” of goal pursuits: human needs and the self-determination of behavior. Psychol. Inq. 11, 227–268. doi: 10.1006/ceps.1999.1020
Deci, E. L., and Ryan, R. M. (2012). “Motivation, personality, and development within embedded social contexts: an overview of self-determination theory,” in The Oxford Handbook of Human Motivation, Vol. 18, ed R. M. Ryan (Oxford University Press), 85–107. doi: 10.1093/oxfordhb/9780195399820.013.0006
Decision Belgian Government (2020). Available online at: https://www.belgium.be/en/news/2021/decisions_council_ministers_12_march_2021 (accessed March 12, 2020).
Deemer, E. D., and Smith, J. L. (2018). Motivational climates: assessing and testing how science classroom environments contribute to undergraduates' self-determined and achievement-based science goals. Learn. Environ. Res. 21, 245–266. doi: 10.1007/s10984-017-9252-y
Dietrich, N., Kentheswaran, K., Ahmadi, A., Teychené, J., Bessière, Y., Alfenore, S., et al. (2020). Attempts, successes, and failures of distance learning in the time of COVID-19. J. Chem. Educ. 97, 2448–2457. doi: 10.1021/acs.jchemed.0c00717
Douglas, S. P., and Craig, C. S. (2007). Collaborative and iterative translation: an alternative approach to back translation. J. Int. Market. 15, 30–43. doi: 10.1509/jimk.15.1.030
Duchatelet, D., and Donche, V. (2019). Fostering self-efficacy and self-regulation in higher education: a matter of autonomy support or academic motivation? Higher Educ. Res. Dev. 38, 733–747. doi: 10.1080/07294360.2019.1581143
EHEA (n.d.) Belgium - Flemish Community. European Higher Education Area Bologna Process. Available online at: https://ehea.info/page-belgium_flemish_community
Esdar, W., Gorges, J., and Wild, E. (2016). The role of basic need satisfaction for junior academics' goal conflicts and teaching motivation. Higher Educ. 72, 175–190. doi: 10.1007/s10734-015-9944-0
Ferri, F., Grifoni, P., and Guzzo, T. (2020). Online learning and emergency remote teaching: opportunities and challenges in emergency situations. Societies 10, 86. doi: 10.3390/soc10040086
Flores, M. A., Barros, A., Simão, A. M. V., Pereira, D., Flores, P., Fernandes, E., et al. (2021). Portuguese higher education students' adaptation to online teaching and learning in times of the COVID-19 pandemic: personal and contextual factors. High. Educ. 6, 1389–1408. doi: 10.1007./s10734-021-00748-x
Fraser, B. J. (1998). Classroom environment instruments: development, validity and applications. Learn. Environ. Res. 1, 7–34. doi: 10.1023/A:1009932514731
Fraser, B. J., McRobbie, C. J., and Fisher, D. (1996). “Development, validation and use of personal and class forms of a new classroom environment questionnaire,” in Proceedings Western Australian Institute for Educational Research Forum, Vol. 31. Como, WA.
Getzels, J. W., and Thelen, H. A. (1960). The classroom group as a unique social system. Teachers College Record 61, 53–82. doi: 10.1177/016146816006101004
Goldenberg, I. (2022). “Military behavioral sciences: an introduction,” in Handbook of Military Sciences, ed A. Sookermany (Cham: Springer International Publishing), 1–15.
Goodenow, C., and Grady, K. E. (1993). The relationship of school belonging and friends' values to academic motivation among urban adolescent students. J. Exp. Educ. 62, 60–71. doi: 10.1080/00220973.1993.9943831
Gorbunovs, A., Kapenieks, A., and Cakula, S. (2016). Self-discipline as a key indicator to improve learning outcomes in online learning environment. Proc. Soc. Behav. Sci. 231, 256–262. doi: 10.1016/j.sbspro.2016.09.100
Guay, F. (2022). Applying self-determination theory to education: regulations types, psychological needs, and autonomy supporting behaviors. Can. J. School Psychol. 37, 75–92. doi: 10.1177/08295735211055355
Guay, F., and Vallerand, R. J. (1996). Social context, student's motivation, and academic achievement: toward a process model. Soc. Psychol. Educ. 1, 211–233. doi: 10.1007/BF02339891
Gutman, L. M., and Eccles, J. S. (2007). Stage-environment fit during adolescence: trajectories of family relations and adolescent outcomes. Dev. Psychol. 43, 522–537. doi: 10.1037/0012-1649.43.2.522
Han, F., and Ellis, R. A. (2020). Initial development and validation of the perceptions of the blended learning environment questionnaire. J. Psychoeduc. Assess. 38, 168–181. doi: 10.1177/0734282919834091
Hartnett, M. K. (2015). Influences that undermine learners' perceptions of autonomy, competence and relatedness in an online context. Austr. J. Educ. Technol. 31, 1526. doi: 10.14742/ajet.1526
Hodges, C. B., Moore, S., Lockee, B. B., Trust, T., and Bond, M. A. (2020). The Difference Between Emergency Remote Teaching and Online Learning.
Holzer, J., Lüftenegger, M., Korlat, S., Pelikan, E., Salmela-Aro, K., Spiel, C., et al. (2021). Higher education in times of COVID-19: university students' basic need satisfaction, self-regulated learning, and well-being. Aera Open 7, 23328584211003164. doi: 10.1177/23328584211003164
Hornstra, L., Weijers, D., van der Veen, I., and Peetsma, T. (2016). Motiverend lesgeven: Handleiding voor docenten.
Howard, J. L., Bureau, J. S., Guay, F., Chong, J. X., and Ryan, R. M. (2021). Student motivation and associated outcomes: a meta-analysis from self-determination theory. Persp. Psychol. Sci. 16, 1300–1323. doi: 10.1177/1745691620966789
Howard, J. L., Chong, J. X., and Bureau, J. S. (2020). The tripartite model of intrinsic motivation in education: a 30-year retrospective and meta-analysis. J. Pers. 88, 1268–1285. doi: 10.1111/jopy.12570
Huang, Y., and Wang, S. (2022). How to motivate student engagement in emergency online learning? Evidence from the COVID-19 situation. Higher Educ. 12, 1–23. doi: 10.1007/s10734-022-00880-2
Jang, H., Reeve, J., Ryan, R. M., and Kim, A. (2009). Can self-determination theory explain what underlies the productive, satisfying learning experiences of collectivistically oriented Korean students? J. Educ. Psychol. 101, 644. doi: 10.1037/a0014241
Juhary, J. (2015). Understanding military pedagogy. Proc.Soc. Behav. Sci. 186, 1255–1261. doi: 10.1016/j.sbspro.2015.04.104
Kelly, D. R., Matthews, M. D., and Bartone, P. T. (2014). Grit and hardiness as predictors of performance among West Point cadets. Military Psychol. 26, 327–342. doi: 10.1037/mil0000050
Kemp, A., Palmer, E., and Strelan, P. (2019). A taxonomy of factors affecting attitudes to- wards educational technologies for use with technology acceptance models. Br. J. Educ. Technol. 50, 2394–2310. doi: 10.1111/bjet.12833
Kiziltepe, Z. (2008). Motivation and demotivation of university teachers. Teachers and Teaching 14, 515–530. doi: 10.1080/13540600802571361
Kosycheva, M. A., and Tikhonova, E. V. (2021). “Students' self-efficacy and motivation in emergency remote learning,” in Proceedings of the 2021 12th International Conference on E-Education, E-Business, E-Management, and E-Learning, 157–162.
Kuśnierz, C., Rogowska, A. M., and Pavlova, I. (2020). Examining gender differences, personality traits, academic performance, and motivation in Ukrainian and Polish students of physical education: a cross-cultural study. Int. J. Environ. Res. Pub. Health 17, 5729. doi: 10.3390/ijerph17165729
Leal, I., Engebretson, J., Cohen, L., Fernandez-Esquer, M. E., Lopez, G., Wangyal, T., et al. (2018). An exploration of the effects of Tibetan yoga on patients' psychological well-being and experience of lymphoma: an experimental embedded mixed methods study. J. Mixed Methods Res. 12, 31–54. doi: 10.1177/1558689816645005
Levesque-Bristol, C., Richards, K. A. R., Zissimopoulos, A., Wang, C., and Yu, S. (2020). An evaluation of the integrative model for learning and motivation in the college classroom. Curr. Psychol. 18, 1–13.
Lewis, S., Whiteside, A. L., and Dikkers, A. G. (2014). Autonomy and responsibility: online learning as a solution for at-risk high school students. Int. J. E-Learn. Dist. Educ. 29, 2.
Liu, Y., Zhao, L., and Su, Y. S. (2022). The impact of teacher competence in online teaching on perceived online learning outcomes during the COVID-19 outbreak: a moderated-mediation model of teacher resilience and age. Int. J. Environ. Res. Pub. Health 19, 6282. doi: 10.3390/ijerph19106282
Livingston, K., and Condie, R. (2006). The impact of an online learning program on teaching and learning strategies. Theor. Prac. 45, 150–158. doi: 10.1207/s15430421tip4502_7
Malik, A. Y. (2015). different context, similar motives: external influences on motivation. The Am. J. Bioethics 15, 26–28. doi: 10.1080/15265161.2015.1088975
Maltby, J. R., and Whittle, J. (2000). “Learning programming online: Student perceptions and performance,” in Proceedings of the ASCILITE 2000 Conference.
Mariani, M. S., Medo, M., and Zhang, Y. C. (2016). Identification of milestone papers through time-balanced network centrality. J. Inf. 10, 1207–1223. doi: 10.1016/j.joi.2016.10.005
McGaughey, F., Watermeyer, R., Shankar, K., Suri, V. R., Knight, C., Crick, T., et al. (2022). ‘This can't be the new norm': academics' perspectives on the COVID-19 crisis for the Australian university sector. Higher Educ. Res. Dev. 41, 2231–2246. doi: 10.1080/07294360.2021.1973384
Mercer, S. (2019). Language learner engagement: setting the scene. Second Handb. Eng. Lang. Teach. 11, 643–660. doi: 10.1007/978-3-030-02899-2_40
Minnaert, A., Boekaerts, M., De Brabander, D., and Opdenakker, C. (2011). Students' experiences of autonomy, competence, social relatedness and interest within a CSCL environment in vocational education: the case of commerce and business administration. Vocat. Learn. 4, 175. doi: 10.1007/s12186-011-9056-7
Minnaert, A. E. M. G., Boekaerts, M., and Opdenakker, M. C. (2008). The relationship between students' interest development and their conceptions and perceptions of group work. Unterrichtswissenschaft. Zeitschrift für Lernforschung 36, 216–236.
Moos, R. H. (2002). Invited address: the mystery of human context and coping: an unraveling of clues. Am. J. Commun. Psychol. 30, 67–88. doi: 10.1023/A:1014372101550
Muilenburg, L. Y., and Berge, Z. L. (2005). Student barriers to online learning: a factor analytic study. Dist. Educ. 26, 29–48. doi: 10.1080/01587910500081269
Murphy, M. P. (2020). COVID-19 and emergency eLearning: consequences of the securitization of higher education for post-pandemic pedagogy. Contem. Secur. Policy 41, 492–505. doi: 10.1080/13523260.2020.1761749
Nguyen, T., Netto, C. L., Wilkins, J. F., Bröker, P., Vargas, E. E., Sealfon, C. D., et al. (2021). Insights into students' experiences and perceptions of remote learning methods: from the COVID-19 pandemic to best practice for the future. Front. Educ. 91, 647986. doi: 10.3389/feduc.2021.647986
Niemiec, C. P., and Ryan, R. M. (2009). Autonomy, competence, and relatedness in the classroom: applying self-determination theory to educational practice. Theor. Res. Educ. 7, 133–144. doi: 10.1177/1477878509104318
Noels, K. A., Pelletier, L. G., Clément, R., and Vallerand, R. J. (2003). Why are you learning a second language? Motivational orientations and self-determination theory. Lang. Learn. 53, 33–64. doi: 10.1111/1467-9922.53223
Nokelainen, P., Kaisvuo, H., and Pylväs, L. (2017). “Self-regulation and competence in formal and informal contexts of vocational and professional education,” in Competence-Based Vocational and Professional Education (Cham: Springer), 775–793.
O'Cathain, A., and Thomas, K. J. (2004). “Any other comments?” Open questions on questionnaires–a bane or a bonus to research? BMC Med. Res. Methodol. 4, 1–7. doi: 10.1186/1471-2288-4-25
OECD (n.d.). Important Note on the Belgian Education System. Available online at: https://gpseducation.oecd.org/Content/MapOfEducationSystem/BFL/BFL_Note.pdf
Oliveira, G., Grenha Teixeira, J., Torres, A., and Morais, C. (2021). An exploratory study on the emergency remote education experience of higher education students and teachers during the COVID-19 pandemic. Br. J. Educ. Technol. 52, 1357–1376. doi: 10.1111/bjet.13112
Pai, P. G., Menezes, V., Srikanth, S., and Shenoy, J. P. (2014). Medical students' perception of their educational environment. J. Clin. Diag. Res. 8, 103–107. doi: 10.7860/JCDR/2014/5559.3944
Pascarella, E. T., Walberg, H. J., Junker, L. K., and Haertel, G. D. (1981). Continuing motivation in science for early and late adolescents. Am. Educ. Res. J. 18, 439–452. doi: 10.3102/00028312018004439
Patrick, H., Kaplan, A., and Ryan, A. M. (2011). Positive classroom motivational environments: convergence between mastery goal structure and classroom social climate. J. Educ. Psychol. 103, 367. doi: 10.1037/a0023311
Reeve, J. (2002). Self-determination theory applied to educational settings. Handb. Self-Determ. Res. 2, 183–204.
Reeve, J., Jang, H., Carrell, D., Jeon, S., and Barch, J. (2004). Enhancing students' engagement by increasing teachers' autonomy support. Motiv. Emot. 28, 147–169. doi: 10.1023/B:MOEM.0000032312.95499.6f
Rizkallah, E. G., and Seitz, V. A. (2017). Understanding student motivation: a key to retention in higher education. Sci. Annal. Econ. Bus. 64, 45–57. doi: 10.1515/saeb-2017-0004
Robbins, S. B., Lauver, K., Le, H., Davis, D., Langley, R., Carlstrom, A., et al. (2004). Do psychosocial and study skill factors predict college outcomes? A meta-analysis. Psychol. Bullet. 130, 261. doi: 10.1037/0033-2909.130.2.261
Ryan, A. M., and Patrick, H. (2001). The classroom social environment and changes in adolescents' motivation and engagement during middle school. Am. Educ. Res. J. 38, 437–460. doi: 10.3102/00028312038002437
Ryan, R. M., and Connell, J. P. (1989). Perceived locus of causality and internalization: examining reasons for acting in two domains. J. Pers. Soc. Psychol. 57, 749. doi: 10.1037/0022-3514.57.5.749
Salas Velasco, M. (2014). Do higher education institutions make a difference in competence development? A model of competence production at university. Higher Educ. 68, 503–523. doi: 10.1007/s10734-014-9725-1
Sarrazin, P., Tessier, D., and Trouilloud, D. (2006). The establishment of a motivational environment by the teacher and student involvement in class: a survey of the latest research. Rev. Pedagogie 157, 12–12.
Sason, H., Wasserman, E., Safrai, M. Z., and Romi, S. (2022). Students' perception of the role of online teachers: comparing routine and emergency times. Front. Educ. 6:767700. doi: 10.3389/feduc.2021.767700
Saykili, A. (2018). Distance education: definitions, generations and key concepts and future directions. Int. J. Cont. Educ. Res. 5, 2–17.
Scherer, R., Howard, S. K., Tondeur, J., and Siddiq, F. (2021). Profiling teachers' readiness for online teaching and learning in higher education: Who's ready? Comput. Hum. Behav. 118, 106675. doi: 10.1016/j.chb.2020.106675
Sierens, E., Vansteenkiste, M., Goossens, L., Soenens, B., and Dochy, F. (2009). The synergistic relationship of perceived autonomy support and structure in the prediction of self-regulated learning. Br. J. Educ. Psychol. 79, 57–68. doi: 10.1348/000709908X304398
Skatova, A., and Ferguson, E. (2014). Why do different people choose different university degrees? Motivation and the choice of degree. Front. Psychol. 5, 1244. doi: 10.3389/fpsyg.2014.01244
Sohrabi, C., Alsafi, Z., O'Neill, N., Khan, M., Kerwan, A., and Al-Jabir, A. (2020). World health organization declares global emergency: a review of the 2019 novel coronavirus (COVID-19). Int. J. Surg. 76, 71–76. doi: 10.1016/j.ijsu.2020.02.034
Standage, M., Duda, J. L., and Ntoumanis, N. (2003). A model of contextual motivation in physical education: using constructs from self-determination and achievement goal theories to predict physical activity intentions. J. Educ. Psychol. 95, 97. doi: 10.1037/0022-0663.95.1.97
Stolk, J. D., Jacobs, J., Girard, C., and Pudvan, L. (2018). “Learners' needs satisfaction, classroom climate, and situational motivations: evaluating self-determination theory in an engineering context,” in 2018 IEEE Frontiers in Education Conference (FIE). Piscataway, NJ: IEEE, 1–5.
Sullivan, G. M., and Feinn, R. (2012). Using effect size—or why the P value is not enough. J. Grad. Med. Educ. 4, 279–282. doi: 10.4300/JGME-D-12-00156.1
Tichavsky, L. P., Hunt, A. N., Driscoll, A., and Jicha, K. (2015). “It's just nice having a real teacher”: student perceptions of online versus face-to-face instruction. Int. J. Schol. Teach. Learn. 9, n2. doi: 10.20429/ijsotl.2015.090202
Turchi, L. B., Bondar, N. A., and Aguilar, L. L. (2020). What really changed? Environments, instruction, and 21st century tools in emergency online English language arts teaching in United States schools during the first pandemic response. Front. Educ. 5, 583963. doi: 10.3389/feduc.2020.583963
Vallerand, R. J., Pelletier, L. G., Blais, M. R., Briere, N. M., Senecal, C., Vallieres, E. F., et al. (1992). The academic motivation scale: a measure of intrinsic, extrinsic, and amotivation in education. Educ. Psychol. Measurement 52, 1003–1017. doi: 10.1177/0013164492052004025
Van den Broeck, A., Howard, J. L., Van Vaerenbergh, Y., Leroy, H., and Gagné, M. (2021). Beyond intrinsic and extrinsic motivation: a meta-analysis on self-determination theory's multidimensional conceptualization of work motivation. Org. Psychol. Rev. 11, 240–273. doi: 10.1177/20413866211006173
Van den Broeck, A., Vansteenkiste, M., De Witte, D., Houdmont, H. J., and Leka, S. (2008). “Self-determination theory: a theoretical and empirical overview in occupational health psychology,” in Occupational Health Psychology: European Perspectives on Research, Education & Practive Vol. 3, eds J. Houdmont and S. Leka (Nothingham: Nothingham University Press), 63–88.
Van der Kaap-Deeder, J., Vansteenkiste, M., Soenens, S., Loeys, T., Mabbe, E., Gargurevich, R., et al. (2015). Autonomy-supportive parenting and autonomy-supportive sibling interactions: the basic psychological need satisfaction and frustration scale (BPNSNF) role of mothers' and siblings' psychological need satisfaction. Pers. Soc. Psychol. Bullet. 41, 1590–1604. doi: 10.1177/0146167215602225
Vansteenkiste, M., Ryan, R. M., and Soenens, B. (2020). Basic psychological need theory: advancements, critical themes, and future directions. Motiv. Emot. 44, 1–31. doi: 10.1007/s11031-019-09818-1
Vasconcellos, D., Parker, P. D., Hilland, T., Cinelli, R., Owen, K. B., Kapsal, N., et al. (2020). Self-determination theory applied to physical education: a systematic review and meta-analysis. J. Educ. Psychol. 112, 1444. doi: 10.1037/edu0000420
Velde, R. V. D., Blignaut–van Westrhenen, N., Labrie, N. H., and Zweekhorst, M. (2021). ‘The idea is nice… but not for me': first-year students' readiness for large-scale ‘flipped lectures'—what (de) motivates them? Higher Educ. 81, 1157–1175. doi: 10.1007/s10734-020-00604-4
Verboord, M. (2014). The impact of peer-produced criticism on cultural evaluation: a multilevel analysis of discourse employment in online and offline film reviews. New Media Soc. 16, 921–940. doi: 10.1177/1461444813495164
Wang, M. C., Haertel, G. D., and Walberg, H. J. (1990). What influences learning? A content analysis of review literature. J. Educ. Res. 84, 30–43. doi: 10.1080/00220671.1990.10885988
Watermeyer, R., Crick, T., Knight, C., and Goodall, J. (2021). COVID-19 and digital disruption in UK universities: afflictions and affordances of emergency online migration. Higher Educ. 81, 623–641. doi: 10.1007/s10734-020-00561-y
Williams, G. C., and Deci, E. L. (1996). Internalization of biopsychosocial values by medical students: a test of self-determination theory. J. Pers. Soc. Psychol. 70, 767. doi: 10.1037/0022-3514.70.4.767
Williams, P. E., and Hellman, C. M. (2004). Differences in self-regulation for online learning between first- and second-generation college students. Res. High. Educ. 45, 71–82. doi: 10.1023/B:RIHE.0000010047.46814.78
World Health Organization (2020). WHO Coronavirus Disease (COVID-19) Dashboard: World Health Organization, 2020. WHO Coronavirus Disease (COVID-19) Dashboard. Geneva: WHO.
Yates, A., Starkey, L., Egerton, B., and Flueggen, F. (2021). High school students' experience of online learning during COVID-19: the influence of technology and pedagogy. Technol. Pedag. Educ. 30, 59–73. doi: 10.1080/1475939X.2020.1854337
Keywords: higher education, academic motivation, basic needs, online learning environment, emergency remote teaching, COVID-19
Citation: Lepinoy A, Vanderlinde R and Lo Bue S (2023) Emergency remote teaching, students' motivation and satisfaction of their basic psychological needs in higher education. Front. Educ. 8:1187251. doi: 10.3389/feduc.2023.1187251
Received: 15 March 2023; Accepted: 09 October 2023;
Published: 22 November 2023.
Edited by:
Tom Crick, Swansea University, United KingdomReviewed by:
Juan Manuel Trujillo Torres, University of Granada, SpainCopyright © 2023 Lepinoy, Vanderlinde and Lo Bue. This is an open-access article distributed under the terms of the Creative Commons Attribution License (CC BY). The use, distribution or reproduction in other forums is permitted, provided the original author(s) and the copyright owner(s) are credited and that the original publication in this journal is cited, in accordance with accepted academic practice. No use, distribution or reproduction is permitted which does not comply with these terms.
*Correspondence: Anouk Lepinoy, YW5vdWsubGVwaW5veUBtaWwuYmU=
Disclaimer: All claims expressed in this article are solely those of the authors and do not necessarily represent those of their affiliated organizations, or those of the publisher, the editors and the reviewers. Any product that may be evaluated in this article or claim that may be made by its manufacturer is not guaranteed or endorsed by the publisher.
Research integrity at Frontiers
Learn more about the work of our research integrity team to safeguard the quality of each article we publish.