- 1Department of Education and Special Education, University of Gothenburg, Gothenburg, Sweden
- 2Dart Centre for Augmentative and Alternative Communication and Assistive Technology, Sahlgrenska University Hospital, Gothenburg, Sweden
- 3Speech and Language Pathology Unit, Department of Health and Rehabilitation, Institute of Neuroscience and Physiology, Sahlgrenska Academy, University of Gothenburg, Gothenburg, Sweden
- 4Department of Behavioural Sciences and Learning, Linköping University, Linköping, Sweden
Educational researchers have challenged Bandura’s prediction that self-efficacy beliefs tend to be established early in learning and that once set, self-efficacy beliefs persist unless a critical event causes them to be reevaluated. However, the results have been mixed in previous research, including being positive, negative, and unchanged. In response, we evaluated how 75 teachers (i.e., special educators) rate their teaching self-efficacy beliefs in motivating student reading and adapting reading instruction at two time points. All teachers taught students with an intellectual disability, communication difficulties, and poor reading skills. The teachers participated in a workshop to learn teaching reading strategies with apps under various conditions (comprehension strategies, phonemic strategies, or both comprehension and phonemic strategies). We analyzed teacher self-efficacy beliefs at two time points with a 12-week span (pre-and postintervention). First, we developed measures of teacher self-efficacy through confirmatory factor analyses. Next, we analyzed the data with multiple imputation and mixed linear regression with difference-in-differences (DiD). The results indicated no statistically significant treatment effect on teachers’ rating of their teaching self-efficacy beliefs. We conclude that our results agree with Bandura’s original prediction and thus, his social cognitive theory.
Introduction
Disability constitutes one of the greatest barriers for learning. Research has shown that students with intellectual disability (ID) have lower proficiency in reading compared to typical children (Dessemontet et al., 2017; van Wingerden et al., 2017; de Chambrier et al., 2021). To overcome these barriers, students with ID need high-quality instruction. Research has shown that reading programs in phonics as well as comprehension-based instruction support students with ID literacy development (e.g., Lundberg and Reichenberg, 2013; Bruni and Hixson, 2017; Reichow et al., 2019; de Chambrier et al., 2021). The digital revolution has made reading programs both accessible for all students with disabilities and cost-effective because they can be downloaded as an app on one’s laptop, phone, or tablet. Digital reading apps hold the promise of helping children with disabilities to overcome barriers by supporting students’ capabilities to access information on their own as a basic right (UNICEF, 2022).
However, one of the major challenges of implementing digital reading apps in schools centers on its effect on teachers’ teaching self-efficacy (TSE). TSE refers to the beliefs that teachers hold about their capabilities to undertake certain teaching tasks even in challenging situations (Bandura, 1986; Tschannen-Moran and Hoy, 2007). TSE affects instructional outcomes, individual and collective efforts among teachers, and persistence within the profession (Skaalvik and Skaalvik, 2010; Skaalvik and Skaalvik, 2011). Thus, researchers have argued that TSE may be an important mediating or intervening variable to explain the relationship between reading programs and student reading ability (Tschannen-Moran et al., 1998).
Social cognitive theory posits that reading interventions depends on teachers’ TSE, and that changing teachers’ TSE will prove difficult (Bandura, 1997). Indeed, Bandura claimed that self-efficacy beliefs tend to be established early in learning and that once set, self-efficacy beliefs persist unless a critical event causes them to be reevaluated (Bandura, 2006). Nevertheless, educational researchers such as Timperley and Phillips (2003) and Holzberger et al. (2013) have challenged Bandura’s assumption in favor of an alternative interpretation. We develop this further in the section titled “Framework: Self-Efficacy and Social Cognitive Theory.”
This study makes two empirical contributions. First, although studies have investigated special educators’ TSE beliefs in mainstream schools (e.g., Leyser et al., 2011; Chao et al., 2017; Alnahdi and Schwab, 2021), no study has evaluated special educators’ TSE beliefs in schools for students with ID.1 Second, studies on TSE in the special education context have mostly examined TSE and teachers’ concerns about inclusive education and have overlooked other dimensions of teaching (Sharma et al., 2012). These studies seldom consider changes in TSE.
The current study targeted teachers’ rating of their self-efficacy beliefs in relation to two outcomes: (a) adapting reading instruction and (b) motivating student reading. The teachers taught students with ID. Finally, this study was part of a larger research project involving a reading intervention using apps.
We have structured the article as follows: First, we present a theoretical framework, discussing social cognitive theory and self-efficacy, and then derive predictions based on the discussion. Second, we describe our methods, including data collection (i.e., the reading intervention), variables and measurements, and analysis strategies (mixed linear regression and confirmatory factor analysis; CFA). Third, we report the study’s results. Finally, we highlight the study’s scope, limitations, pedagogical implications, and conclusions.
Framework: self-efficacy and social cognitive theory
Self-efficacy is grounded in a larger theoretical framework known as social cognitive theory that promotes the belief that people can shape their actions. According to the theory (Bandura, 1986, 1997), people with high self-efficacy are most likely to use their knowledge. Self-efficacy has been defined as an individual’s belief that they can cope with specific tasks or life events. Thus, self-efficacy is not a reflection of an individual’s skills but rather their beliefs about what they can accomplish with the skills they possess.
If people believe they will be successful at a given task, they are more likely to invest effort, persist in their efforts, and manage upcoming events (Bandura, 1997). Bandura also proposed that self-efficacy beliefs were self-reflective and powerful behavior motivators.
Bandura proposed four sources that influence the extent of an individual’s self-efficacy. The first source consists of mastery experiences. Success strengthens self-efficacy, whereas repeated failures undermine it; in other words, the belief that teaching has been successful raises self-efficacy expectations that teaching also will be successful in the future. Early successes strengthen self-efficacy. The second source consists of vicarious experiences (someone else models the target activity). The third source consists of verbal persuasion (feedback from others in the form of verbal encouragement, pep talks, etc.). The fourth source consists of emotional and physiological states; that is, an individual’s happiness level can increase their self-efficacy, whereas stress may have a negative effect on an individual’s self-assessed capability (Bandura, 1997). The most powerful influence is mastery experiences, which for teachers, come from actual teaching accomplishments with students (Bandura, 1997).
Teacher self-efficacy
Teacher Self-Efficacy (TSE) relates to the beliefs that teachers hold about their capabilities to undertake certain teaching tasks, even in difficult situations. TSE affects instructional practices, individual and collective efforts among teachers, and persistence within the profession (Skaalvik and Skaalvik, 2010; Skaalvik and Skaalvik, 2011).
Following Bandura’s (1997) conceptualization of self-efficacy, Skaalvik and Skaalvik (2007) divided TSE into six aspects that incorporate all tasks teachers are expected to do: (a) explain and instruct, (b) adapt instruction to individual students’ needs, (c) motivate students, (d) maintain discipline and order, (e) cooperate with parents and other teachers, and (f) cope with changes. Of Skaalvik and Skaalvik’s (2011) six aspects of TSE operationalization, we found (b) and (c) especially relevant to our understanding of the beliefs that teachers hold regarding their capabilities of adapting reading instruction and motivating struggling and reluctant readers to read.
Development and stability of self-efficacy: two competing predictions
As mentioned above, Bandura (1997) proposed that self-efficacy beliefs would be most shapeable early in the life course and that self-efficacy could change in early life or during the initial stages due to the sources of self-efficacy within a given situation. Bandura (1997, 2006) further suggested that it would take some kind of shock to provoke a reassessment later in life.
Studies on how interventions change TSE have been mixed, and thus the studies have been inconclusive. One problem has been the lack of longitudinal data and the reliance on age or experience as proxy variables for development (Tschannen-Moran et al., 1998). However, cross-sectional data do not allow researchers to differentiate between changes due to experience and differences in experiences. For example, experienced teachers may have had higher self-efficacy when they entered teacher education (i.e., selection bias) or less experienced teachers might be less sensitive to on-the-job experience (i.e., resistance to treatment).
The few longitudinal studies on TSE have yielded results showing increases, decreases, or stability in self-efficacy over time (for a review, see Tschannen-Moran et al., 1998. See also Tschannen-Moran and McMaster, 2009).2 In some studies, TSE decreased. For example, in a longitudinal study, Clark (2020) examined self-efficacy beliefs at two points. The teachers rated their capability to teach reading (reading TSE) and multicultural issues. Regarding reading TSE, the teachers’ scores significantly decreased over time. Clark (2020) underscored that insufficient classroom experience (i.e., lack of mastery experiences) and the absence of role models (i.e., lack of vicarious experiences) decreases reading TSE. Such an observation agrees with Guskey’s (2002) finding that classroom experience is of pivotal importance. Reyhing and Perren (2021) found mixed outcomes in their study of educators in early childhood education and care.
In other studies, TSE followed an inverse U shape (Hoy and Spero, 2005). For example, Hoy and Spero (2005) found significant increases in TSE during student teaching, but significant declines in TSE during the teachers’ first year of teaching (agreeing with Clark, 2020). These results support Bandura’s (1997) theory of self-efficacy in suggesting that self-efficacy is most shapeable early in learning; thus, the first year of teaching could be critical to the long-term development of TSE. Hoy and Spero (2005) also noted that changes in self-efficacy during the first year of teaching related to the level of support received. However, not all studies support Bandura’s claim.
In contrast, based on longitudinal data, Holzberger et al. (2013) urged a reexamination of Bandura’s (1997) claim. Holzberger et al. (2013) hypothesized a mutual causal relationship exists between instructional quality and self-efficacy (i.e., it is reciprocal). In other words, teachers with greater self-efficacy should deliver greater instructional quality. At the same time, greater instructional quality (e.g., mastery) cultivates increased self-efficacy. Thus, Holzberger et al. (2013) underscored that instructional quality should capture the four sources of self-efficacy. However, using a cross-lagged model, Holzberger et al. (2013) found that TSE (a) exhibited strong stability and TSE did not promote instructional quality, but instructional quality promoted increases in TSE. As a theoretical implication, Holzberger et al. (2013) proposed that their result should generalize educational processes. Thus, Holzberger et al. (2013) both agreed and disagreed with social cognitive theory.
Purpose and hypotheses
We aimed to evaluate how teachers rate their self-efficacy beliefs in adapting reading instruction and motivating student reading before and after a reading intervention using research-based apps. The two apps that were used were Animega–interactive sentences (Animega–is; Heimann and Lundälv, 2020), which focuses on comprehension strategies, and Accessible Literacy Learning (ALL; Light and McNaughton, 2020), which focuses on phonemic strategies. Both apps are further described in the material section below.
The reading intervention included three treatment conditions: (a) teachers teaching and training together with their students using the Animega-is app, (b) teachers teaching and training together with their students using the ALL app, and (c) teachers teaching and training together with their students using both the Animega-is app and the ALL app. There was also a control condition: teaching as usual. We considered two research questions.
1. How did teachers’ rating of their self-efficacy beliefs in motivating students’ reading change over time between teachers assigned to the treatment conditions and teachers assigned to the control condition?
2. How did teachers’ rating of their self-efficacy beliefs in adapting reading instruction change over time between teachers assigned to the treatment conditions and teachers assigned to the control condition?
We had two hypotheses:
Hypothesis 1: Teachers’ rating of their self-efficacy beliefs in motivating student reading will increase in the treatment condition compared with the teachers in the control condition.
Hypothesis 2: Teachers’ rating of their self-efficacy beliefs in adapting reading instruction will increase in the treatment condition compared with the teachers in the control condition.
The null hypotheses of Hypothesis 1 and Hypothesis 2 can be meaningfully interpreted as support for Bandura’s (1997) prediction that no association exists between assignment to treatment conditions and self-efficacy. The null hypotheses can also be considered as the degree of stability of self-efficacy.
Methods
Participants and sampling
Data presented in this paper were collected in the larger project Digital Media Supporting Literacy Learning in Children with Communicative and Cognitive Disabilities (DiLL), and parts of the data have been used for this paper. For a full description of the collected data, see Palmqvist et al. (2020).
The teachers in the DiLL project were recruited through voluntary response sampling. We informed teachers about our study at conferences for special needs education, via email lists, and via social media, which gave the teachers the opportunity to report interest in participating in the study. Initially, more than 100 teachers representing schools from several Swedish regions responded positively. Because the project could only include a limited number of regions, not all schools were eligible. Thus, our sample consisted of teachers (n = 75) from 39 compulsory schools for students with ID in two Swedish regions. The majority of the teachers (91%) were females and 9% males. They had an average of 11 years of teaching experience and a mean age of 50 years. More than half of the teachers had a special education degree (51%).
Schools and teachers were allocated to four intervention conditions and four time windows. The intervention lasted for 12 weeks. The schools could not choose which condition of the project in which they preferred to participate. Swedish teachers are well prepared. The curricular requirements for special educator preparation in Sweden comprise 90 credits (18 months of graduate-level studies; i.e., a magister degree), and admission to a degree program is granted only to applicants who are qualified teachers. Some teacher students choose to continue with a master’s degree (1 year).
The 128 students had a mean age of 13.6 years. Forty-one students had mild ID, 68 had moderate ID, and 16 students had severe ID. Thirty-two students were reported to have no additional diagnosis besides ID. Twenty-nine students had Down syndrome and 10 students had ID and cerebral palsy. Furthermore, in addition to ID, 45 students had autism spectrum disorder, eight students had attention-deficit/hyperactivity disorder and/or attention-deficit disorder, and 13 students had a combination of ID, autism spectrum disorder, and attention-deficit/hyperactivity disorder and/or attention-deficit disorder. Ninety-eight students reported using speech as a mode of communication. One-hundred-and-eighteen students reported using one or more forms of augmentative, alternative communication (AAC) in their communication.
Materials
Two digital research-based apps were used: ALL and Animega-is. ALL builds on a phonics-based strategy and is specifically developed for children with complex communication needs and AAC (Light and McNaughton, 2020).
ALL has produced positive reading results in two small-scale studies of children with complex communication needs (Ainsworth et al., 2016). ALL consists of modules for basic reading skills and combines direct phonics-based instructions with meaningful comprehension-based reading activities.
In the present study, the teachers were instructed to work with the modules using phonics-based instruction: sound blending, phoneme segmentation, letter–sound correspondences, and word recognition. ALL consists of three levels of complexity, and the student works through them in stages. All sessions include pictures to enable participation without speech. ALL is developed to engage the teacher–student interaction, and the app has three instructional modes: full instruction, where ALL is giving all the instructions; targets only, where only the targeted sounds are provided from the app; and teacher assisted, where all the instructions are provided by the teachers. In the present study, the teacher-assisted mode was recommended to enhance the communication between student and teacher.
Animega-is is software built on a comprehension-based reading strategy and includes six levels of training: Level 1 explores individual words, whereas Level 6 contains long sentences that challenge a learner’s working memory. The learner is expected to explore the content with support from—and in interaction with—a teacher. The language material and the appended animations motivate the learner, but also give room for conversations where the learner can express his or her imagination and thoughts or ask and answer questions. The goal is to achieve meaning from text through multimedia and supportive interaction (Heimann and Lundälv, 2020). Positive findings of the theory behind the app can be found in several studies based on its predecessor, Omega-is (e.g., Gustafson et al., 2011). Both apps use animations, videoclips, and speech output (digitized or synthesized) to enhance communication in the classroom. Thus, the apps help teachers to overcome communication and language problems. Moreover, both apps can be adapted to the needs of the individual student.
Intervention design
We implemented the project over three semesters, from January 2020 to June 2021. First, the control group (teaching as usual; 12 schools and 18 teachers) began during spring of 2020. The second group used the ALL app (11 schools, 21 teachers), and began their training early during the second semester of the project, autumn 2020. The third group used the Animega-is (six schools, 15 teachers) and began later that same semester. The fourth and final group (10 schools, 21 teachers) used both apps in combination and trained during the final semester, spring 2021.
We measured TSE beliefs before and after the intervention via web-based questionnaires. In total, we analyzed the 75 teachers’ ratings of their self-efficacy beliefs in motivating student reading and in adapting their reading instruction at two time points (pre-and postintervention). However, our data contain missing values due to teachers’ illnesses that required the teachers to stay at home. In Figure 1, we describe the different stages of the intervention.
Teacher training
All teachers, except those in the control condition, participated in one or two online workshops (teachers in the combined condition participated in two), before the intervention began. The workshop instructed the teachers how to use the app during the intervention. Each workshop lasted 4 h. The workshops began with the teachers receiving information about the study procedures and background and then an introduction of the apps (ALL or/and Animega-is). Each workshop was recorded and then distributed to every teacher so they could watch the recorded workshop again when needed.
The teachers also received detailed information regarding the apps and written information describing the intervention instructions. Thus, the teachers were instructed to use the apps 90 min a week (a total of 18 h), and they were free to distribute the time according to the schedule and the students’ abilities. We also provided manuals and instructions about adjustments in the apps that were available to suit individual students (e.g., response delay, visual contrast, and text-to-speech speed). Finally, members of the research team hosted the workshops and collected the data.
Fidelity of the intervention
The study began in spring 2020, at the beginning of the COVID-19 pandemic. Although Swedish compulsory schools for students with ID remained open during the pandemic, only the staff was permitted to visit the classrooms. If the circumstances had been different (no COVID-19 restrictions), we would have been able to visit the schools during the intervention to monitor compliance. However, we monitored that the teachers complied with the assigned condition by requiring the teachers to use digital logbooks. The logbook had a structured format meaning that the teachers: (a) rated the student reading engagement, (b) reported the absence or presence of teaching assistants, (c) stated the duration of the session, and (d) described the content of the session. Thus, the logbook was not a diary but a protocol that was sent to the researchers each week. The research team resolved uncertainties via email or phone correspondence with the teachers. We monitored the total time duration devoted to using the apps. Monitoring the duration ensured that the teachers used the apps during the intervention.
Variables
Outcomes
Concerning TSE, we used two modified measures from Skaalvik and Skaalvik (2011): motivates student reading and adapts student reading instruction. The teachers were asked to respond to eight statements (Table 1). All statements began with the question stem: “How confident are you that you can….” The teachers rated the strength of their confidence on a 7-point Likert scale ranging from 1 (not at all true for me) to 7 (completely true for me; Table 1). Four of the statements focused on adapting reading instruction: “I can adapt my instruction to every student’s needs,” “I can make use of variation in the students’ abilities, so that all students are sufficiently challenged,” “I can adapt my teaching so that not only poor readers but also good readers are challenged,” and “I can organize my instruction so that poor readers and good readers have graded tasks.” Four statements focused on reading motivation: “I can encourage all students not to give up on reading and writing tasks,” “I can encourage poor readers to enjoy reading,” “I can make all students do their best even when dealing with difficult texts,” and I can motivate students who actively avoid reading to read. Teachers’ agreement with these statements indicated confidence in their capabilities to overcome factors that could create obstacles to students’ learning. The statements have previously been used (Reichenberg and Andreassen, 2019).
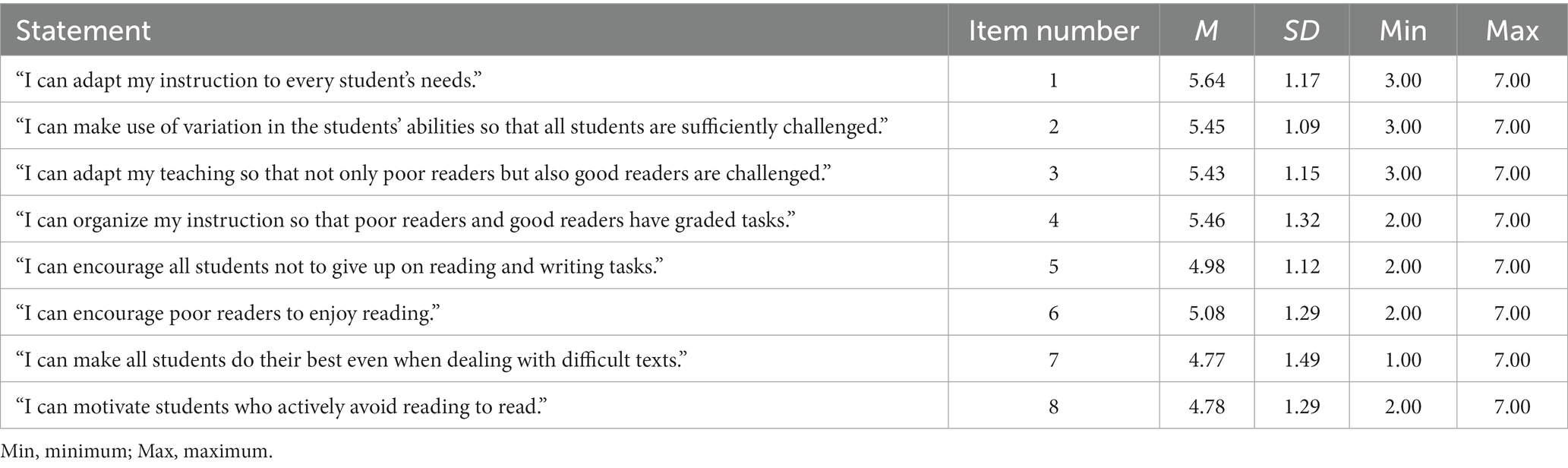
Table 1. Teachers’ self-efficacy beliefs: reading adaptation and reading motivation; means, standard deviations, minimums, and maximums.
Focal predictors
The focal predictors consisted of the three treatment conditions (phonemic, comprehension, and a combination of phonemic and comprehension) and the control condition. We simply created a dummy variable for each condition (=1) with the control as a reference (=0). Similarly, to measure time, we simply used a dummy for before (=0) and after (=1) the reading intervention.
Pretreatment predictors
In our survey, we also asked questions about teachers’ backgrounds: sex (91% females, 9% males), years of teaching experience (M = 11, SD = 6), age (M = 50, SD = 9), and special education degree (51% yes, 49% no).
Compared to population data (Swedish National Agency for Education, 2022), our sample slightly overrepresented female teachers (70% in Grundsär3 and 84% in Gymnasiesär4). Similarly, our sample also substantively overrepresented teachers with special educational degrees in the population (26% in Grundsär and 27% in Gymnasiesär). Regarding experience, the teachers in our study had less teaching experience than the average population of teachers (12 years in Grundsär and 14 years in Gymnasiesär). Regarding age, our sampled teachers fell into the typical age bracket within the teacher population in both Grundsär and Gymnasiesär.
Returning to the data preparation, for the degree variable, we coded any type of special education course mentioned. Both age and experience were standardized to z scores for interpretability (i.e., M = 0, SD = 1). To save degrees of freedom, we did not compute dummy variables. Finally, we adjusted for gender (female = 0, male = 1).
Regarding the selection of pretreatment predictors, first, we note that age and experience have typically been used as proxies for changes in TSE beliefs (Bandura, 1997; Tschannen-Moran et al., 1998). Second, age, experience, gender, and education have served as the “usual suspects” in teacher research, that is, teacher characteristics. Including pretreatment predictors (e.g., teacher characteristics) can theoretically shrink the variance of the estimated parameters and adjust for confounding variables due to potential selection bias in the assignment.
Measurement and reliability
We continued with parallel analysis, which is a sophisticated type of exploratory factor analysis (Goretzko et al., 2021). Specifically, parallel analysis offers a stopping rule to factor retention by adjusting the eigenvalues. The adjusted eigenvalues aim to avoid overextraction of the last factors that occasionally come closer to 1; that is, the Kaiser rule (Horn, 1965; Dinno, 2014). Here we used the paran package (Dinno and Dinno, 2018), assuming a common factor analysis. The parallel analysis suggested that we should retain two common factors.
The Cronbach’s α was above 0.9 for both factors (α = 0.93 and 0.95, respectively), which is considered very good. However, α should be completed with McDonald’s ω (Table 2; McNeish, 2018; Raykov and Marcoulides, 2019; Hayes and Coutts, 2020). The McDonald’s ω result was 0.93 and 0.94, respectively, for the two teacher self-efficacy measures, and 0.93 for all variables combined to one scale.5
Confirmatory factor analysis
We used the factor score from the Confirmatory Factor Analysis (CFA) to score the latent factor. Guided by self-efficacy theory and previous research we opted for the two factors: motivating student reading and adapting reading instruction. All factors have loadings above 0.5. The comparative fit index (CFI) is above the suggested cut-off, which is good for model fit. The root mean square error of approximation (RMSEA) and standardized root mean square residual (SRMR) fall below the cut-off, which is good for the model fit. In other words, the model fit statistics indicate that the model fits with the data. However, we needed to allow some errors to correlate (see Table 2 for details).
CFA offers three advantages (Hoyle, 2015). First, CFA allowed us to weigh the self-efficacy statements by importance. Second, CFA allowed us to identify the scales based on theory. Third, CFA allowed us to account for missing data on the scales (i.e., full maximum likelihood estimation).
We acknowledge three problems with our approach. First, our sample size was somewhat small for CFA (Wolf et al., 2013). Second, we disregarded that our data are ordinal because of convergence problems. Convergence problems frequently occur in CFA with ordinal data and small samples (see Bovaird and Koziol, 2015; Rosseel, 2020). However, sum scores do not necessarily offer a solution (McNeish and Wolf, 2020). Third, we accounted for the CFA and regression models in two steps rather than one step, and the second step was pragmatic; that is, suboptimal to a structural equation model (e.g., latent growth curves, see Hoyle, 2015). We made this decision based on the sample size (Hoyle, 2015).
In sum, we derived a factor score for each of the self-efficacy scales using CFA. To account for repeated measures, we used clustered standard errors for the educators (Table 2).
Missing values
In small data sets, all observations matter. Improperly handling missing data can influence the study’s results.
Gender, age, experience, and special education training were imputed with values from the first time point; that is, via carry last value forward. After the carry last value forward step, age and experience had between 1 and 2% missing values, whereas special education degree had 20% missing values. Self-efficacy item variables had between 11 and 14% missing values. After the full maximum likelihood estimation, we had about 11% of the values missing.
Thus, we still had problems with missing data primarily for other variables. Consequently, we conducted multiple imputation. Simplified, multiple imputation predicts missing values with regressions plus random error. We (a) created 20 imputed data sets, (b) ran 20 mixed regressions, and (c) averaged the results (i.e., pooling).
Analysis strategy: mixed model with difference-in-differences
To analyze the data, we estimated DiD (Angrist and Pischke, 2009). DiD refers to a quasi-experimental method for evaluating program or interventions. Briefly, DiD compares the treatment to the control condition in the following manner:
• Difference-in-treatment: The difference between post-TSE and pre-TSE for the treatment (i.e., change in the treatment)
• Difference-in-control: The difference between post-TSE and pre-TSE for the control (i.e., change in the control)
• Difference-in-differences: the difference between (a) and (b); that is, the difference in changes between treatment versus control.
To make inferences about the treatment, we require one important assumption: a common trend for all conditions. In other words, we must assume the same slope between treatment and control prior to the intervention. However, DiD does not require the same intercepts prior to the intervention for treatment and control. Thus, DiD has been advantageous whenever the common trend assumption holds for intervention or program evaluation. Therefore, the estimation proves simple; although, the idea and motivation of DiD proves complex.
A DiD can be estimated with a short format or long format. The short format implies a linear regression (e.g., least squares first-differenced estimator; subtract time 1 from time 2). A long format implies a hierarchical data structure in case we only need to estimate an interaction term between treatment and time variables in the regression model, where the interaction term corresponds to (c); that is, DiD. The long format can be estimated with least squares and robust standard errors, generalized least squares, or restricted maximum likelihood (i.e., mixed regression; Donald and Lang, 2007; Lee, 2016). Each have their advantages and disadvantages.
We estimated mixed regressions (a.k.a. hierarchical models or multilevel models; Raudenbush and Bryk, 2002; Snijders and Bosker, 2012 Bates et al., 2015). A mixed regression accounts for repeated measurements (i.e., clustering).
All analyses were conducted in R (Team TRDC, 2008) with primarily the following packages: lavaan (Rosseel, 2012), lme4 (Bates et al., 2015), mice (Van Buuren and Groothuis-Oudshoorn, 2011), Mitml (Grund et al., 2023), dplyr (Wickham and Grolemund, 2016), and ggplot2 (Wickham, 2011).
Results
This section presents the results guided by our two research questions: First, we report descriptive statistics. Second, we report the mixed regression analysis.
Descriptive statistics
Before turning to the regression model, we consider the descriptive statistics in Table 3. Descriptively, all treatment conditions improved during the intervention except for the phonics conditions.
The comprehension condition improved slightly to moderately on TSE for motivation and TSE for adaptation. The phonics and comprehension condition improved slightly to moderately on TSE for adaptation. However, the phonics condition improved slightly to moderately on TSE for adaptation but decreased in TSE for motivation. Finally, the control condition made negligible gain in TSE for adaptation, but declined on TSE for motivation.
Although the descriptive statistics indicate an improvement, we must temper our expectations due to sampling uncertainty. First, we must estimate the uncertainty around the changes. For example, changes may be due to fluctuations around the mean (i.e., regression to the mean), and thus the distributions of TSE may still overlap despite differences in means. Second, we must adjust for pretreatment predictors to improve the balance of the four conditions. For example, the difference between the phonics and control conditions on the pretreatment TSE for adaptation and TSE for motivation seem considerable.
Predicting teachers’ self-efficacy in motivating reading and adapting reading instruction: mixed regression analysis
We report the analysis of the mixed linear regression in Table 4. The table includes coefficients, standard errors, and p values. First, we report the teachers’ rated self-efficacy beliefs for (a) adapting reading instruction and (b) motivating student reading with the individual treatment conditions versus the control condition. Second, we report teachers’ rated self-efficacy beliefs in (c) adapting reading instruction and (d) motivating student reading with all treatment conditions combined versus the control condition.
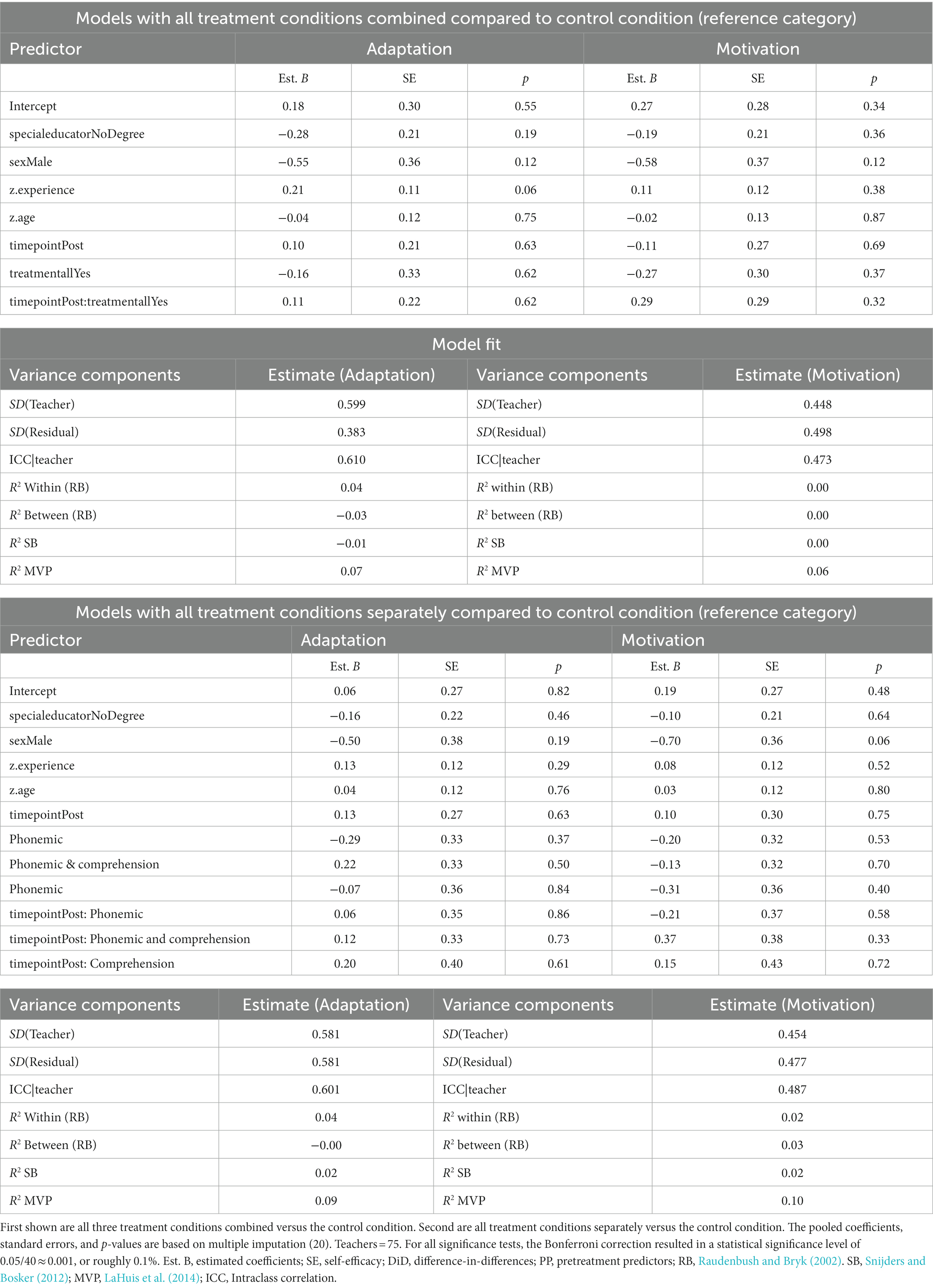
Table 4. Mixed regression table for self-efficacy (se) for reading adaptation and reading motivation.
Here, we primarily care about the DiD. The DiD consists of the average difference in self-efficacy (a) between the conditions and (b) between the two time points. In Table 4, the DiD consists of the product of the treatment and time point. However, regardless of the set up (separate or combined treatments), we found no statistically significant differences for the product terms or interaction terms (i.e., “timepoint Post:treatmentallYes”). However, we note that the sign is now positive. As with age and experience, the signs of the coefficients agreed with our expectations.
Going beyond the statistical significance, we want to understand the educational importance (disregarding uncertainty of estimation). Consider the explained variance (i.e., R2). LaHuis et al. (2014) would suggest that our model explains around 9 to 10% of the variance in TSE.
Next, consider two predictive comparisons for an unqualified female teacher for the treatment versus control condition in TSE. For simplicity, we omit, “holding all predictors at the same level.” However, we do assume the latter below. The common trend assumption in DiD (same slopes) makes no assumptions about initial differences in means.
First, we consider the strength of DiD in TSE for adaptation. Differing results in the DiD (DiT-DiC = 0.21–0.10); that is, 0.11 SD in TSE, meaning the same as the reported interaction term in Table 4 (i.e., timepoint Post treatmentallYes). Overlooking uncertainty of estimation, we find a small but positive treatment difference for TSE for adaptation (following Cohen, 1988).
Second, we consider the strength of DiD in TSE for motivation. The difference in change corresponds to the DiD for TSE for motivation of 0.29 SD (DiT-DiC = 0.18 to −0.11); that is, the same as the reported interaction term in Table 4 (i.e., timepointPost treatmentallYes).
Concerning TSE for motivation, we observe that nothing happened, or at best, conditions did not get worse (disregarding uncertainty of estimation). If anything, the treated teachers “caught up” with initial differences to teachers in the control condition (i.e., teaching as usual) because the control condition dropped in TSE for motivation (i.e., in the predictive comparison).
Thus, we did not find support for Hypothesis 1. Likewise, we did not find support for Hypothesis 2. Instead, the results suggest that Bandura’s prediction holds (i.e., self-efficacy does not necessarily change with intervention; Bandura, 1997, 2006).
Discussion
TSE beliefs are known to be a crucial variable when teaching students to read and comprehend texts (Reichenberg and Andreassen, 2019). Results of previous intervention studies on TSE have been inconclusive. With this background, we report on an intervention study involving special educators teaching students with ID. We report a null result. Unlike previous studies, we avoided proxies for evaluating change, such as age or experience (Tschannen-Moran et al., 1998). Instead, we focused on a DiD design, which can be viewed as a panel study with only two time points from an intervention perspective. Thus, our DiD design attempted to evaluate change (under the assumption of an equal trend) as one would in a natural intervention (i.e., nonrandomized; Wolf et al., 2013).
Our study advances two empirical and one theoretical contribution. First, although studies have investigated special educators’ TSE beliefs in mainstream schools (e.g., Leyser et al., 2011; Chao et al., 2017; Alnahdi and Schwab, 2021), no study has evaluated special educators’ TSE beliefs in schools for students with ID. Second, studies on TSE (in the special education context) have mostly examined TSE and teachers’ concerns about inclusive education and overlooked other dimensions of teaching (Sharma et al., 2012). These studies seldom consider changes in TSE.
Researchers disagree about whether teachers’ self-efficacy can change later in their teaching careers. Some claim that self-efficacy changes for the better (Timperley and Phillips, 2003; Holzberger et al., 2013), others for the worse Clark (2020), and some refute any changes (Reyhing and Perren, 2021). Our study contributes to clarity concerning the scientific controversy over whether teacher self-efficacy changes over time. Specifically, we contribute by evaluating whether participation in a reading intervention acts as a critical event to change the participating teachers’ self-efficacy. Finally, a strength is that our study uses multiple outcomes and multiple questions with measurement models for TSE.
Our study highlights five unresolved issues in research on social cognitive theory. The first unresolved question in this study is the timing. Social cognitive theory posits that teachers respond to interventions over time (Bandura, 1997). When teachers attempt to implement new practices, their efficacy beliefs may initially be lowered but then rebound to a higher level when the new strategies are found to be effective (Tschannen-Moran et al., 1998).
A second unresolved issue concerns the importance of the pandemic as a critical event in teachers’ lives. Bandura (2006) underscored the importance of critical events. We can never know whether the intervention (workshops, writing the logbooks, and using the apps and the manuals) acted as a critical event. A critical event maybe positive or negative and thus can either encourage or discourage the teachers’ use of the apps. Such a hypothetical explanation would also agree with Bandura’s (1997) theory because it points to the primacy of critical events.
Undoubtedly, we could not eliminate the interference of the pandemic in the study. Indeed, we do not know how much the pandemic may have undermined teachers’ self-efficacy (i.e., constituting a negative critical event; Bandura, 2006). Due to the pandemic, we were not able to visit the classrooms and give the teachers encouragement and support on the spot. Encouragement and support are particularly important as change is implemented and temporary dips in efficacy occur. In addition, teachers can be warned that initial attempts to implement new strategies may temporarily lower their feelings of efficacy. Teachers need support and training on the job to see them through the initial slump. They also need to see evidence of increased student learning before new, higher efficacy beliefs will take root (Tschannen-Moran et al., 1998).
A third unresolved issue concerns the consequences of interventions for teachers’ behaviors. If interventions fail to alter teachers’ self-efficacy, then we need to adjust our expectations that interventions ought to change teachers’ behaviors because TSE typically acts as the mechanism that explains the efficacy in the teacher behavior (e.g., treatment heterogeneity; Bandura, 1986).
A fourth unresolved issue concerns the mixed consequences of interventions on self-efficacy. Studies have reported cases of interventions where TSE decreased rather than increased Clark (2020). At the same time, some studies have reported positive outcomes (Timperley and Phillips, 2003; Holzberger et al., 2013). We hypothesize that differences in types of interventions, student population, types of self-efficacy (i.e., choice of outcome metric or variable measurement), sample size issues (e.g., small samples), choice of estimator (e.g., analysis of variance, structural equations, or mixed models), classroom interaction, and selection into treatment contribute to such mixed results. Specifically, attaining control over classroom interactions and self-selection into treatment (e.g., no random sample or randomization) is a serious concern but difficult to address (although not impossible). In addition, more effort can be made to address measurement issues and manipulate the task in focus (e.g., reading, mathematics, civics, and inclusiveness). Thus, we need to know which conditions, tasks, or teacher–student interactions lead to a negative change as opposed to a positive or no change (Tschannen-Moran et al., 1998). Our study is no exception to these concerns, yet we consider the urgency of the question of providing high-quality reading instruction for all students, including those with ID, to motivate our study.
A fifth unresolved issue concerns treatment interactions. Treatment interactions such as teacher experience may contribute to a variation in treatment outcome (Lander, 2013). A treatment may vary conditionally on another predictor such as how differences in experience contribute to responsiveness to the treatment. Less experienced teachers may be more responsive to treatment and more experienced teachers less responsive to treatment.
Limitations
To clarify the scope of our conclusions, we now turn to our study’s limitations. Despite our efforts to collect data from more teachers, our sample size was relatively small, which reduces the chances of detecting differences (Wolf et al., 2013). A larger sample size would have been preferable given the method we used. In addition, the teachers were not randomly sampled from the teacher population and thus we cannot generalize the conclusions. Despite our efforts, we were unable to randomize the participants in the treatment and the control conditions.
We had no access to observations in the classrooms due to the pandemic restrictions. Therefore, future studies should offer the teachers’ feedback seminars with video-recorded behavior, including stimulated recall (Lander, 2013).
Conclusion and pedagogical implications
We aimed to evaluate how teachers rate their self-efficacy beliefs regarding (a) adapting reading instruction, and (b) motivating student reading before and after a reading intervention. In the intervention, the teachers used specially developed digital apps for reading instruction under one control condition (teaching as usual) and three treatment conditions: (1) comprehension, (2) phonemics, and (3) comprehension plus phonemics. We state our conclusions as follows to correspond with our two research questions. We found no statistically significant differences between the three treatment conditions and the control condition in teachers’ rating of their self-efficacy in adapting reading instruction. Thus, we conclude that there is no support for Hypothesis 1, in agreement with Bandura (1997, 2006). In addition, we found no statistically significant differences between the three treatment conditions and the control condition for teachers’ rating of their self-efficacy in motivating student reading. Again, we conclude that there is no support for Hypothesis 2, in agreement with Bandura (1997, 2006).
Regarding the pedagogical implications, we believe that teacher education requires early intervention in teachers’ lives. Teacher programs, including special education programs, need to give preservice teachers more opportunities to gain actual experience with instructing and managing students in a variety of contexts with increasing levels of complexity and challenges that provide mastery experiences and specific feedback. In particular, teachers should be provided with performance feedback (i.e., verbal persuasion) early in learning to highlight their positive achievements and to encourage emphasis on effort and persistence, which will potentially have a positive effect on the development of efficacy beliefs (Bandura, 1997; Tschannen-Moran et al., 1998; Tschannen-Moran and Johnson, 2011). Thus, reallocating time, effort, and money into teacher education may be more efficient in regards to TSE (Hoy and Spero, 2005).
Finally, these results enable us to make a small contribution to the UN/UNICEF (2022) education agenda because as our study evaluates the importance of apps in aiding educators teaching students with ID.
Data availability statement
The raw data supporting the conclusions of this article will be made available by the authors, without undue reservation.
Ethics statement
The studies involving human participants were reviewed and approved by This study was reviewed and approved by the Swedish Ethical Review Authority, Uppsala, Sweden (no. 2019-03845). All participants provided written informed consent to participate in this study. The patients/participants provided their written informed consent to participate in this study.
Author contributions
MR conceived the study and took the main responsibility for writing the manuscript. GT and MH contributed to writing the method section and the final version of the manuscript. LP and JS collected the data while GT and MH supervised the data collection. EH, LP, JS, KM, and ML provided extensive feedback and assistance on the drafts. All authors read and approved the submitted version.
Funding
This research was supported by grants from the Marcus and Amalia Wallenberg Foundation (Grant 2018.0084) awarded to MH and from the Swedish Research Council (Grant 2018–04702) awarded to GT.
Acknowledgments
We wish to thank all of the schools and teachers who participated in this research. We are also grateful to Rune Andreassen for providing insightful comments on an earlier draft, and to Rolf Lander for help with the resubmission.
Conflict of interest
MH and ML are co-creators and copyright holders of Animega - interactive sentences, which is commercially available.
The remaining authors declare that the research was conducted in the absence of any commercial or financial relationships that could be construed as a potential conflict of interest.
Publisher’s note
All claims expressed in this article are solely those of the authors and do not necessarily represent those of their affiliated organizations, or those of the publisher, the editors and the reviewers. Any product that may be evaluated in this article, or claim that may be made by its manufacturer, is not guaranteed or endorsed by the publisher.
Footnotes
1. ^Swedish students with ID often attend a compulsory school for students with ID (called Grundsärskolan) as an alternative to a mainstream school.
2. ^Only one study has evaluated the importance of apps for TSE; however, the researchers found no significant association between the use of apps and TSE (Michos et al., 2022).
3. ^Compulsory schools for student with intellectual disability.
4. ^Upper secondary schools for students with intellectual disability.
5. ^Scholars disagree about whether CFA assumes correlation between the variables’ errors (i.e., “tau-equivalence”; McNeish, 2018; Raykov and Marcoulides, 2019; Hayes and Coutts, 2020). Our measures fail the assumption of uncorrelated errors. In any case, McDonald’s ω does not assume uncorrelated errors. For this analysis, we used the coefficients alpha package (Zhang and Yuan, 2016).
References
Ainsworth, M. K., Evmenova, A. S., Behrmann, M., and Jerome, M. (2016). Teaching phonics to groups of middle school students with autism, intellectual disabilities and complex communication needs. Res. Dev. Disabil. 56, 165–176. doi: 10.1016/j.ridd.2016.06.001
Alnahdi, G. H., and Schwab, S. (2021). Special education major or attitudes to predict teachers’ self-efficacy for teaching in inclusive education. Front. Psychol. 12, 1–8. doi: 10.3389/fpsyg.2021.680909
Angrist, J. D., and Pischke, J. S. (2009). Mostly harmless econometrics: an empiricist’s companion. Princeton: Princeton University Press.
Bandura, A. (1986). Social foundations of thought and action: a social cognitive theory. Prentice Hall, 23–28.
Bandura, A. (2006). Toward a psychology of human agency. Perspect. Psychol. Sci. 1, 164–180. doi: 10.1111/j.1745-6916.2006.00011.x
Bates, D., Mächler, M., Bolker, B., and Walker, S. (2015). Fitting linear mixed-effects models using lme 4. J. Stat. Softw. 67, 1–48. doi: 10.18637/jss.v067.i01
Bovaird, J. A., and Koziol, N. A. (2015). “Measurement models for ordered-categorical indicators” in Handbook of structural equation modeling. ed. R. H. Hoyle (New York Guilford Press), 495–511.
Bruni, T. P., and Hixson, M. D. (2017). Beyond sight words: Reading programs for people with intellectual disabilities. Behav. Develop. Bull. 22, 249–257. doi: 10.1037/bdb0000062
Chao, C. N. G., Chow, W. S. E., Forlin, C., and Ho, F. C. (2017). Improving teachers’ self-efficacy in applying teaching and learning strategies and classroom management to students with special education needs in Hong Kong. Teach. Teach. Educ. 66, 360–369. doi: 10.1016/j.tate.2017.05.004
Clark, S. K. (2020). Examining the development of teacher self-efficacy beliefs to teach reading and to attend to issues of diversity in elementary schools. Teach. Dev. 24, 127–142. doi: 10.1080/13664530.2020.1725102
Cohen, J. (1988). Statistical power analysis for the behavioural sciences. 2nd Edn. Hillsdale: L. Erlbaum Associates.
de Chambrier, A. F., Dessemontet, R. S., Martinet, C., and Fayol, M. (2021). Rapid automatized naming skills of children with intellectual disability. Heliyon 7:e06944. doi: 10.1016/J.HELIYON.2021.E06944
Dessemontet, R. S., de Chambrier, A. F., Martinet, C., Moser, U., and Bayer, N. (2017). Exploring phonological awareness skills in children with intellectual disability. Am. J. Intellect. Dev. Disabil. 122, 476–491. doi: 10.1352/1944-7558-122.6.476
Dinno, A. (2014). Gently clarifying the application of Horn’s parallel analysis to principal component analysis versus factor analysis. Portland State University. Available at: https://alexisdinno.com/Software/files/PA_for_PCA_vs_FA.pdf
Dinno, A. and, Dinno, M.A. (2018). Package ‘paran.’ Available at: http://bioconductor.statistik.tu-dortmund.de/cran/web/packages/paran/paran.pdf
Donald, S. G., and Lang, K. (2007). Inference with difference-in-differences and other panel data. Rev. Econ. Stat. 89, 221–233. doi: 10.1162/rest.89.2.221
Goretzko, D., Pham, T. T. H., and Bühner, M. (2021). Exploratory factor analysis: current use, methodological developments and recommendations for good practice. Curr. Psychol. 40, 3510–3521. doi: 10.1007/s12144-019-00300-2
Grund, S., Robitzsch, A., and Luedtke, O. (2023). Package ‘mitml’: tools for multiple imputation in multilevel modeling. R Package. Available at: https://cran.r-project.org/web/packages/mitml/mitml.pdf
Guskey, T. R. (2002). Professional development and teacher change. Teach. Teach. 8, 381–391. doi: 10.1080/135406002100000512
Gustafson, S., Fälth, L., Svensson, I., Tjus, T., and Heimann, M. (2011). Effects of three interventions on the reading skills of children with reading disabilities in grade 2. J. Learn. Disabil. 44, 123–135. doi: 10.1177/0022219410391187
Hayes, A. F., and Coutts, J. J. (2020). Use omega rather than Cronbach’s alpha for estimating reliability. But…. Commun. Methods Meas. 14, 1–24. doi: 10.1080/19312458.2020.1718629
Heimann, M., and Lundälv, M. (2020). Animega–interactive sentences (Animega-is) [computer software]. Available at: https://animega-is.se/
Holzberger, D., Philipp, A., and Kunter, M. (2013). How teachers’ self-efficacy is related to instructional quality: a longitudinal analysis. J. Educ. Psychol. 105, 774–786. doi: 10.1037/a0032198
Horn, J. L. (1965). A rationale and test for the number of factors in factor analysis. Psychometrika 30, 179–185. doi: 10.1007/BF02289447
Hoy, A. W., and Spero, R. B. (2005). Changes in teacher efficacy during the early years of teaching: a comparison of four measures. Teach. Teach. Educ. 21, 343–356. doi: 10.1016/j.tate.2005.01.007
LaHuis, D. M., Hartman, M. J., Hakoyama, S., and Clark, P. C. (2014). Explained variance measures for multilevel models. Organ. Res. Methods 17, 433–451. doi: 10.1177/1094428114541701
Lander, R. (2013). Nyblivna lärares upplevda kapacitet i arbetet med eleverna–varifrån kommer den? [newly qualified teachers’ self-reported capacity to work with students. Where does it come from?]. Pedagogisk Forskning Sverige 18, 216–237.
Lee, M. J. (2016). Matching, regression discontinuity, difference in differences, and beyond. Oxford: Oxford University Press.
Leyser, Y., Zeiger, T., and Romi, S. (2011). Changes in self-efficacy of prospective special and general education teachers: implication for inclusive education. Int. J. Disabil. Dev. Educ. 58, 241–255. doi: 10.1080/1034912X.2011.598397
Light, J., and McNaughton, D. (2020). “Literacy intervention for individuals with complex communication needs” in Augmentative and alternative communication. eds. D. R. Beukelman and J. C. Light. 5th ed (Baltimore: Paul H. Brookes Publishing Co.), 427–482.
Lundberg, I., and Reichenberg, M. (2013). Developing Reading comprehension among students with mild intellectual disabilities: an intervention study. Scand. J. Educ. Res. 57, 89–100. doi: 10.1080/00313831.2011.623179
McNeish, D. (2018). Thanks coefficient alpha, we’ll take it from here. Psychol. Methods 23, 412–433. doi: 10.1037/met0000144
McNeish, D., and Wolf, M. G. (2020). Thinking twice about sum scores. Behav. Res. Methods 52, 2287–2305. doi: 10.3758/s13428-020-01398-0
Michos, K., Cantieni, A., Schmid, R., Müller, L., and Petko, D. (2022). Examining the relationship between internship experiences, teaching enthusiasm, and teacher self-efficacy when using a mobile portfolio app. Teach. Teach. Educ. 109:103570. doi: 10.1016/j.tate.2021.103570
Palmqvist, L., Samuelsson, J., Holmer, E., Rudner, M., Lundälv, M., Reichenberg, M., et al. (2020). Kom Loss. doi: 10.17605/OSF.IO/X76WV
Raudenbush, S. W., and Bryk, A. S. (2002). Hierarchical linear models: applications and data analysis methods. 2nd Edn. Thousand Oaks: Sage.
Raykov, T., and Marcoulides, G. A. (2019). Thanks coefficient alpha, we still need you! Educ. Psychol. Meas. 79, 200–210. doi: 10.1177/0013164417725127
Reichenberg, M., and Andreassen, R. (2019). Self-efficacy as a predictor of reading instruction: a comparison between Norwegian and Swedish teachers. L1-educational. Stud. Lang. Lit. 19, 1–29. doi: 10.17239/L1ESLL-2019.19.01.14
Reichow, B., Lemons, C. J., Maggin, D. M., and Hill, D. R. (2019). Beginning reading interventions for children and adolescents with intellectual disability. Cochrane Database Syst. Rev. 12:CD011359. doi: 10.1002/14651858.CD011359.pub2
Reyhing, Y., and Perren, S. (2021). Self-efficacy in early childhood education and care: what predicts patterns of stability and change in educator self-efficacy? Front. Educ. 6, 1–8.doi: 10.3389/feduc.2021.634275
Rosseel, Y. (2012). Lavaan: an R package for structural equation modeling (version 0.5–12, Beta) [computer software]. J. Stat. Softw. 48, 1–36. doi: 10.18637/jss.v048.i02
Rosseel, Y. (2020). Small sample solutions for structural equation modeling. In R. V. Schootde and M. Miocević (Ed.) Small sample size solutions: A guide for applied researchers and practitioners 226–238) London and New York: Routledge.
Sharma, U., Loreman, T., and Forlin, C. (2012). Measuring teacher efficacy to implement inclusive practices. J. Res. Spec. Educ. Needs 12, 12–21. doi: 10.1111/j.1471-3802.2011.01200.x
Skaalvik, E. M., and Skaalvik, S. (2007). Dimensions of teacher self-efficacy and relations with strain factors, perceived collective teacher efficacy, and teacher burnout. J. Educ. Psychol. 99, 611–625. doi: 10.1037/0022-0663.99.3.611
Skaalvik, E. M., and Skaalvik, S. (2010). Teacher self-efficacy and teacher burnout: a study of relations. Teach. Teach. Educ. 26, 1059–1069. doi: 10.1016/j.tate.2009.11.001
Skaalvik, E. M., and Skaalvik, S. (2011). Teacher job satisfaction and motivation to leave the teaching profession: relations with school context, feeling of belonging, and emotional exhaustion. Teach. Teach. Educ. 27, 1029–1038. doi: 10.1016/j.tate.2011.04.001
Snijders, T. A. B., and Bosker, R. J. (2012). Multilevel analysis: An introduction to basic and advanced multilevel modeling. Thousand Oaks: Sage.
Swedish National Agency for Education. (2022). Statistics. Available at: https://www.skolverket.se/skolutveckling/statistik
Team TRDC. (2008). The R project for statistical computing. Available at: http://www.r-project.org
Timperley, H. S., and Phillips, G. (2003). Changing and sustaining teachers’ expectations through professional development in literacy. Teach. Teach. Educ. 19, 627–641. doi: 10.1016/S0742-051X(03)00058-1
Tschannen-Moran, M., and Hoy, A. W. (2007). The differential antecedents of self-efficacy beliefs of novice and experienced teachers. Teach. Teach. Educ. 23, 944–956. doi: 10.1016/j.tate.2006.05.003
Tschannen-Moran, M., Hoy, A. W., and Hoy, W. K. (1998). Teacher efficacy: its meaning and measure. Rev. Educ. Res. 68, 202–248. doi: 10.3102/00346543068002202
Tschannen-Moran, M., and Johnson, D. (2011). Exploring literacy teachers’ self-efficacy beliefs: potential sources at play. Teach. Teach. Educ. 27, 751–761. doi: 10.1016/j.tate.2010.12.005
Tschannen-Moran, M., and McMaster, P. (2009). Sources of self-efficacy: four professional development formats and their relationship to self-efficacy and implementation of a new teaching strategy. Elem. Sch. J. 110, 228–245. doi: 10.1086/605771
UNICEF. (2022). Digital technology. Available at: https://www.unicef.org/globalinsight/digital-technology
Van Buuren, S., and Groothuis-Oudshoorn, K. (2011). Mice: multivariate imputation by chained equations in R. J. Stat. Softw. 45, 1–67. doi: 10.18637/jss.v045.i03
Van Wingerden, E., Segers, E., van Balkom, H., and Verhoeven, L. (2017). Foundations of reading comprehension in children with intellectual disabilities. Res. Dev. Disabil. 60, 211–222. doi: 10.1016/j.ridd.2016.10.015
West, S. G., Taylor, A. B., and Wu, W. (2015). “Model fit and model selection in structural equation modeling” in Handbook of structural equation modeling. ed. R. H. Hoyle (New York: Guilford Press), 209–231.
Wickham, H., and Grolemund, G. (2016). R for data science: import, tidy, transform, visualize, and model data. Sebastopol, CA: O’Reilly Media.
Wolf, E. J., Harrington, K. M., Clark, S. L., and Miller, M. W. (2013). Sample size requirements for structural equation models: an evaluation of power, bias, and solution propriety. Educ. Psychol. Meas. 73, 913–934. doi: 10.1177/0013164413495237
Keywords: teacher self-efficacy beliefs, reading research, special education, intellectual disability, computer-assisted instruction, social cognitive theory
Citation: Reichenberg M, Thunberg G, Holmer E, Palmqvist L, Samuelsson J, Lundälv M, Mühlenbock K and Heimann M (2023) Will an app-based reading intervention change how teachers rate their teaching self-efficacy beliefs? A test of social cognitive theory in Swedish special educational settings. Front. Educ. 8:1184719. doi: 10.3389/feduc.2023.1184719
Edited by:
Luana Sorrenti, University of Messina, ItalyReviewed by:
Yan Liu, Carleton University, CanadaMaria Cristina Gugliandolo, University of Messina, Italy
Copyright © 2023 Reichenberg, Thunberg, Holmer, Palmqvist, Samuelsson, Lundälv, Mühlenbock and Heimann. This is an open-access article distributed under the terms of the Creative Commons Attribution License (CC BY). The use, distribution or reproduction in other forums is permitted, provided the original author(s) and the copyright owner(s) are credited and that the original publication in this journal is cited, in accordance with accepted academic practice. No use, distribution or reproduction is permitted which does not comply with these terms.
*Correspondence: Monica Reichenberg, monica.reichenberg@ped.gu.se
†ORCID: Monica Reichenberg, https://orcid.org/0000-0002-7403-1702
Gunilla Thunberg, https://orcid.org/0000-0002-9582-7814
Emil Holmer, https://orcid.org/0000-0002-1896-8250
Lisa Palmqvist, https://orcid.org/0000-0002-3350-0701
Jenny Samuelsson, https://orcid.org/0000-0003-2115-3746
Mikael Heimann, https://orcid.org/0000-0001-5025-9975