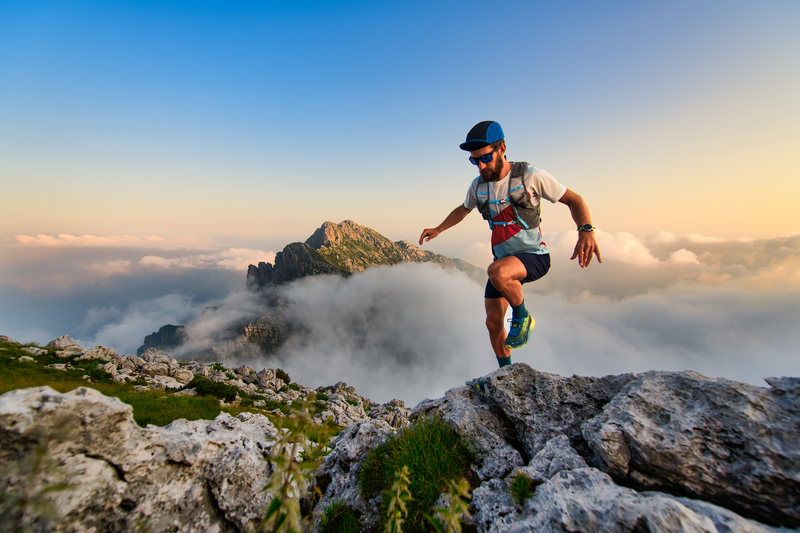
94% of researchers rate our articles as excellent or good
Learn more about the work of our research integrity team to safeguard the quality of each article we publish.
Find out more
EDITORIAL article
Front. Educ. , 28 March 2023
Sec. STEM Education
Volume 8 - 2023 | https://doi.org/10.3389/feduc.2023.1183030
This article is part of the Research Topic AI for Tackling STEM Education Challenges View all 6 articles
Editorial on the Research Topic
AI for tackling STEM education challenges
Artificial intelligence (AI), an emerging technology, finds increasing use in STEM education and STEM education research (e.g., Zhai et al., 2020b; Ouyang et al., 2022; Linn et al., 2023). AI, defined as a technology to mimic human cognitive behaviors, holds great potential to address some of the most challenging problems in STEM education (Neumann and Waight, 2020; Zhai, 2021). Amongst these is the challenge of supporting all students to meet the vision for science learning in the 21st century laid out, for example in the U.S. Framework for K-12 Science Education (National Research Council, 2012), Germany (Kulgemeyer and Schecker, 2014), Finland (Finnish National Board of Education, 2016), and the PISA framework (OECD, 2017). These policy documents call for students to develop proficiency in using ideas so that learners can use their knowledge to solve challenging problems and make sense of complex phenomena. For instance, the Framework calls for students to develop the ability to integrate their knowledge of the disciplinary core ideas (DCIs) and crosscutting concepts across different science disciplines (CCCs) with the skills to engage in major scientific and engineering practices (SEPs) to explain everyday scientific phenomena and solve real-life problems. The Framework also describes pathways, called learning progressions, of how students are expected to progress in developing the competence envisioned. However, to best support students in developing such competence, assessments that allow students to use knowledge to solve challenging problems and make sense of phenomena are needed. These assessments need to be designed and tested to validly locate students on the learning progression and hence provide feedback to students and teachers about meaningful next steps in their learning. Yet, such tasks are time-consuming to score and challenging to provide students with appropriate feedback to develop their knowledge to the next level.
AI technologies, more specifically machine learning, have successfully demonstrated to assist in automatically assessing complex constructs such as students' explanations (Nehm et al., 2012) argumentation competence (Zhai et al., 2022a), drawing models (Zhai et al., 2022b) produced by students in response to tasks that resemble the complex tasks used in instruction (for an overview see Zhai et al., 2020a). Machine learning-based assessment practices cover a range of scholarly works aiming to exploit the potential of AI technologies to assess learning in the context of STEM education to support learners in the development of the envisioned competence.
Kaldaras et al., in their paper, focus on the development of rubrics for scoring constructed-response tasks in relation to a learning progression as a basis for AI-based scoring. One of the main challenges in using machine learning is the development of rubrics that can accurately capture the complexity of the construct and yield sufficient scoring accuracy between humans and machines. While holistic scoring is traditionally used in learning progression-based assessment, analytic scoring has been identified as a means to increase human-machine agreement. Kaldaras et al. describe a systematic procedure on how to derive analytical scores from holistic rubrics, exemplify its use and reflect on the challenges as well as how to overcome them.
In a second paper, Kaldaras and Haudek detail an elaborate procedure of how to validate scores obtained through machine learning algorithms. Much of the research on machine-based scoring focuses solely on the human-machine scoring agreement. However, understanding where and why human and machine scorers disagree is highly important (Zhai et al., 2021). Drawing on a learning progression of scientific argumentation as an example, Kaldaras and Haudek demonstrate how their procedure not only helps construct a valid argument for machine-based scores but more importantly, which items and components of the respective scoring rubric pose threats to the validity of the scores.
Bertolini et al. examined the application of Bayesian methodologies to identify factors that indicate student retention and attrition in an undergraduate STEM course. The researchers found that the interaction with the course learning management system (LMS) and performance on diagnostic concept inventory (CI) assessments were the most significant predictors of final course performance. The study highlights that Bayesian methodologies provide a more pragmatic and interpretable way of assessing student performance in STEM courses. The authors suggest that the use of Bayesian techniques can help educators make more informed decisions based on data-driven insights, which can ultimately lead to more effective teaching and learning strategies. The authors emphasize the importance of carefully considering the data and prior assumptions when using Bayesian techniques for educational research and assessment.
Taking the idea of using data produced by students as they learn one step further, Kubsch et al. describe a framework that uses evidence-centered design to guide the development of learning environments providing meaningful learning activities to promote student learning. The framework also describes how data from the activities are automated and how machine learning-based analysis of this data can focus on improving students' learning. The idea is to analyze the process and product data generated as students learn using digital technologies to determine the extent to which students have mastered the learning goals of individual activities and to predict the extent to which students progress toward the overall learning goals of the unit.
Wulff et al. explored the potential of machine learning in combination with natural language processing (NLP) to enhance formative assessment of written reflections in science teacher education. The authors use ML and NLP to filter higher-level reasoning sentences in physics and non-physics teachers' written reflections on a standardized teaching vignette and then cluster the filtered sentences to identify themes and represent knowledge in the teachers' written reflections. The study found that ML and NLP can be used to filter higher-level reasoning elements and identify quality differences in physics and non-physics preservice teachers' texts. Overall, the authors argue that ML and NLP can enhance writing analytics in science education by providing researchers with an efficient means to answer derived research questions.
Consistent with prior findings (Zhai et al., 2020a), the papers included in this Research Topic suggest that machine learning and AI have the potential to address challenging problems in STEM education, including assessing complex constructs, identifying factors that indicate student performance and retention, and enhancing formative assessment. These papers demonstrate the importance of carefully considering the data and prior assumptions when using machine learning and AI techniques for educational research and assessment. These papers also show the value that AI techniques can have in improving STEM Education. AI and machine learning hold promise in supporting reforms by helping to improve how teachers provide students with timely feedback when learners engage in complex tasks that require complex scientific reasoning and use-of-knowledge. With immediate results teachers and instructors can tailor feedback to differentiate instruction to promote learning and support learners in developing the knowledge to advance to the next level of understanding. As such, these findings have important implications for educational researchers, practitioners, and policymakers seeking to improve STEM education.
As these articles illustrate, advances in AI and ML allow researchers to analyze students' complex performances captured in open-ended text responses and representations and provide immediate feedback to learners and teachers. This work has the potential to transform the teaching and learning of K−16 science education. Such research can help to improve assessment, instruction, and curriculum materials to promote student learning. AI when used appropriately can ensure that all students have the opportunities to learn the skills and knowledge needed to succeed in the 21st century.
All authors listed have made a substantial, direct, and intellectual contribution to the work and approved it for publication.
XZ and JK were funded by National Science Foundation Grants Award ID: 2101104, 2100964, and 2138854.
The authors declare that the research was conducted in the absence of any commercial or financial relationships that could be construed as a potential conflict of interest.
All claims expressed in this article are solely those of the authors and do not necessarily represent those of their affiliated organizations, or those of the publisher, the editors and the reviewers. Any product that may be evaluated in this article, or claim that may be made by its manufacturer, is not guaranteed or endorsed by the publisher.
The findings, conclusions, or opinions herein represent the views of the authors and do not necessarily represent the view of personnel affiliated with the National Science Foundation.
Finnish National Board of Education (2016). National Core Curriculum for General Upper Secondary Schools 2015. Porvoo: Porvoon Kirjakeskus.
Kulgemeyer, C., and Schecker, H. (2014). Research on educational standards in german science education–towards a model of student competences. Eurasia J. Math. Sci. Technol. Educ. 10, 257–269. doi: 10.12973/eurasia.2014.1081a
Linn, M. C., Donnelly-Hermosillo, D., and Gerard, L. (2023). “Synergies between learning technologies and learning sciences: promoting equitable secondary school teaching,” in Handbook of Research on Science Education, eds N. G. Lederman, D. L. Zeidler, and J. S. Lederman (New York, NY: Routledge), 447–498.
National Research Council (2012). A Framework for K-12 Science Education: Practices, Crosscutting Concepts, and Core Ideas. Washington, DC: National Academies Press.
Nehm, R. H., Ha, M., and Mayfield, E. (2012). Transforming biology assessment with machine learning: automated scoring of written evolutionary explanations. J. Sci. Educ. Technol. 21, 183–196. doi: 10.1007/s10956-011-9300-9
Neumann, K., and Waight, N. (2020). The digitalization of science education: déjà vu all over again? J. Res. Sci. Teach. 57, 1519–1528. doi: 10.1002/tea.21668
OECD (2017). PISA 2015 Assessment and Analytical Framework: Science, Reading, Mathematic, Financial Literacy and Collaborative Problem Solving. Paris: OECD Publishing. doi: 10.1787/9789264281820-en
Ouyang, F., Zheng, L., and Jiao, P. (2022). Artificial intelligence in online higher education: a systematic review of empirical research from 2011 to 2020. Educ. Inf. Technol. 27, 7893–7925. doi: 10.1007/s10639-022-10925-9
Zhai, X. (2021). Practices and theories: how can machine learning assist in innovative assessment practices in science education. J. Sci. Educ. Technol. 30, 1–11. doi: 10.1007/s10956-021-09901-8
Zhai, X., Haudek, K., and Ma, W. (2022a). Assessing argumentation using machine learning and cognitive diagnostic modeling. Res. Sci. Educ. 53, 405–424. doi: 10.1007/s11165-022-10062-w
Zhai, X., Haudek, K. C., Shi, L., Nehm, R., and Urban-Lurain, M. (2020a). From substitution to redefinition: a framework of machine learning-based science assessment. J. Res. Sci. Teach. 57, 1430–1459. doi: 10.1002/tea.21658
Zhai, X., He, P., and Krajcik, J. (2022b). Applying machine learning to automatically assess scientific models. J. Res. Sci. Teach. 59, 1765–1794. doi: 10.1002/tea.21773
Zhai, X., Shi, L., and Nehm, R. (2021). A meta-analysis of machine learning-based science assessments: Factors impacting machine-human score agreements. J. Sci. Educ. Technol. 30, 361–379. doi: 10.1007/s10956-020-09875-z
Keywords: artificial intelligence (AI), science technology engineering mathematics (STEM), machine learning (ML), natural language processing, computer vision, scientific practices
Citation: Zhai X, Neumann K and Krajcik J (2023) Editorial: AI for tackling STEM education challenges. Front. Educ. 8:1183030. doi: 10.3389/feduc.2023.1183030
Received: 09 March 2023; Accepted: 16 March 2023;
Published: 28 March 2023.
Edited by:
Lianghuo Fan, East China Normal University, ChinaReviewed by:
Shuhui Li, East China Normal University, ChinaCopyright © 2023 Zhai, Neumann and Krajcik. This is an open-access article distributed under the terms of the Creative Commons Attribution License (CC BY). The use, distribution or reproduction in other forums is permitted, provided the original author(s) and the copyright owner(s) are credited and that the original publication in this journal is cited, in accordance with accepted academic practice. No use, distribution or reproduction is permitted which does not comply with these terms.
*Correspondence: Xiaoming Zhai, eGlhb21pbmcuemhhaUB1Z2EuZWR1
Disclaimer: All claims expressed in this article are solely those of the authors and do not necessarily represent those of their affiliated organizations, or those of the publisher, the editors and the reviewers. Any product that may be evaluated in this article or claim that may be made by its manufacturer is not guaranteed or endorsed by the publisher.
Research integrity at Frontiers
Learn more about the work of our research integrity team to safeguard the quality of each article we publish.