- Institute of Physics and Technical Education, Karlsruhe University of Education, Karlsruhe, Germany
The use of digital technologies and media in physics classrooms has pedagogical potential. In addition to everyday common technologies (e. g., presenters or computers), highly subject-specific media and technologies (e. g., simulation and digital data acquisition systems) are now available for these purposes. As the diversity of these technologies/media increases, so do the required competencies on the part of the (pre-service) physics teachers who must be able to exploit the given potential. Corresponding competency frameworks and related evaluation instruments exist to describe and assess the corresponding competencies. These frameworks and scales are characterized by their generality and do not reflect the use of highly subject-specific technologies. Thus, it is not clear how relevant they are for describing competencies in highly subject-specific technological situations, such as working with digital data acquisition systems in educational lab work settings. Against this background, two studies are presented. Study 1 identifies empirically 15 subject-specific competencies for handling digital data acquisition systems in lab work settings based on a literary review of lab manuals and thinking aloud. In Study 2, based on the 15 identified competencies, an abbreviated content- and construct-validated self-efficacy scale for handling digital data acquisition systems is provided. We show that general technological-specific self-efficacy is only moderately related to the highly subject-specific self-efficacy of handling digital data acquisition systems. The results suggest that specific competency frameworks and measurement scales are needed to design and evaluate specific teaching and learning situations.
1. Introduction
New opportunities for learning physics have emerged alongside digitization advancements. For example, everyday digital technologies and media (e. g., presenters, laptops, and internet connecting devices) are used to visualize content, conduct research, and enable learners to work in digital learning environments. On the other hand, highly subject-specific media and technologies are also available, including digital data acquisition systems and computer simulations for educational lab settings. Although simulations can be used to visualize complex phenomena that would otherwise be invisible or too expensive to obtain (Schwarz et al., 2007; Wieman et al., 2010; Darrah et al., 2014; Hoyer and Girwidz, 2020; Banda and Nzabahimana, 2021); digital data acquisition systems enable this exploration of otherwise hidden phenomena by collecting measurement data at higher sampling rates, longer measurement duration, and multiple measurands simultaneously (e. g., Benz et al., 2022).
To leverage this educational potential in physic classrooms and educational lab work settings, (pre-service) physics teachers must have the appropriate technological-related competencies (TRCs) to handle these capabilities, technologies and media (e. g., Koehler et al., 2011). With the increased diversity of modern digital technologies, the corresponding TRCs required for adequate handling become more complex. For example, when using everyday technologies (i. e., internet searching), a (physics) teacher must be able to evaluate the digital sources for instructional quality or select appropriate presentation methods for a large group (Becker et al., 2020). Evaluating internet sources and presentation methods are basic examples of general digital competencies that teachers in all disciplines must possess (Janssen et al., 2013). In addition, digital competencies include adequate knowledge of subject-specific tools for highly subject-specific situations, such as those requiring the description of measurement characteristics (e. g., sampling rate and resolution) or speed measurements (Becker et al., 2020). In this paper, we focus on the use of these highly subject-specific digital technologies and media in highly subject-specific situations in the context of using digital data acquisition systems in educational lab settings, which are a core working situation in science research and classrooms worldwide (NGSS Lead States, 2013).
Competency frameworks are needed to identify, address, and promote specific practices using these technologies and media through specifically designed interventions. One of the most prominent framework is the technological-pedagogical-content knowledge framework (TPACK) of Mishra and Koehler (2006), who extended the pedagogical-content knowledge framework of Shulman (1986) by including technological knowledge (TK) component (Hew et al., 2019). Shulman (1986) explained how professional knowledge consists of content knowledge (CK) component and pedagogical knowledge (PK) and their intersection: pedagogical-content knowledge (PCK). CK reflects one's subject-specific knowledge, PK reflects knowledge of teaching and learning processes (e. g., different methods for knowledge transfer) and PCK reflects how subject-specific content can be taught in the best possible way.
By adding the TK component (i. e., knowledge about the adequate handling of a technology that is unrelated to the content to be taught or teaching itself, such as connecting a sensor to the digital data acquisition system acquisition software), further intersections arise, including the technological CK (TCK), which reflects knowledge of how technologies can be used to open up subject-specific content (e. g., that high-precision measurements are possible through digital data acquisition systems, e. g. for the detection of gravitational waves), technological PK (TPK), which reflects knowledge of how the use of technologies can support teaching/learning (e. g., the influences of a digital data acquisition system during lab work on the motivation of learners), and technological PCK (TPCK), which reflects knowledge of how a subject-specific content can be taught using technologies (e. g., that digital measurement, by measuring with higher sampling rates, can generate larger amounts of data that make previously hidden phenomena accessible; Mishra and Koehler, 2006; Benz et al., 2022). There are critics of the TPACK framework, such as the fuzziness of its individual knowledge components (Cox and Graham, 2009; Archambault and Barnett, 2010; Jang and Tsai, 2012; Willermark, 2017; Scherer et al., 2018), scant evidence on the relationships among individual knowledge components (e. g., Jang and Chen, 2010; Schmid et al., 2020; von Kotzebue, 2022), and that the TPACK framework is very general. The latter means that while it covers as many disciplines as possible, it cannot be used to specifically address subject-, situation-, and technology-specific competencies and requires differentiation first (Becker et al., 2020). These criticisms also apply to other frameworks [overview of frameworks in Falloon (2020) and Jam and Puteh (2020)]; therefore, in remains difficult promote TRCs in different subject areas and highly subject-specific situations, such as lab work. Even if the generalization from TK to digitization-related knowledge (DK), that considers socio-social, cultural, and communication-related aspects, when dealing with everyday digital technologies/media is useful (Huwer et al., 2019), it still seems unhelpful to describe the handling of highly specialized technologies/media like digital data acquisition systems. Nevertheless, future work should consider digital measurement in the context of digitization-related PCK (DPaCK; Huwer et al., 2019) because digital measurement now has high societal importance due to its ubiquity.
One approach to differentiating TPACK for (pre-service) science teachers is the Digital Competencies for Teaching Science Education (DiKoLAN) framework (Becker et al., 2020). DiKoLAN is a basic competence model that has no claim to completeness. It was derived from digital elements of science works, and its relevance to science teaching (Thyssen et al., 2020). Becker et al. (2020) identified seven central areas of knowledge framed by technical core competencies and legal frameworks. These areas are further subdivided into general competence areas (e. g., documentation, presentation, communication/collaboration, information searching, and evaluation) and subject-specific competency areas (e. g., data acquisition, data processing, simulation, and modeling). These general competency areas refer to the use of everyday technologies and media, whereas the subject-specific competence areas refer to the handling of highly specialized digital technologies/media. The assigned competencies are further differentiated into three levels (i. e., name, description and application). Because the focus of this paper is on handling digital data acquisition systems, the data acquisition aspect is explained next (see Figure 1).
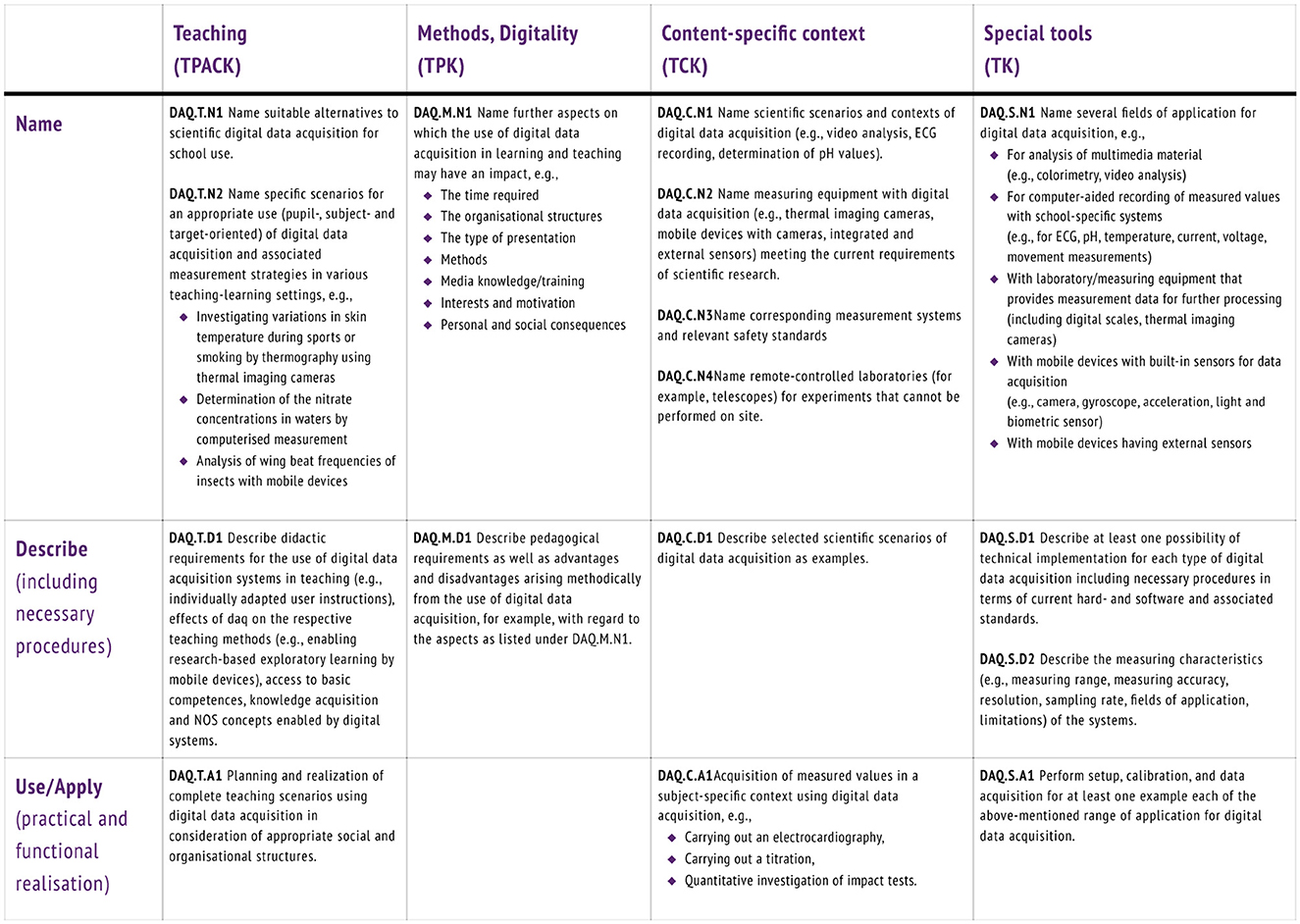
Figure 1. Competencies in the area of data acquisition of the DiKoLAN framework (von Kotzebue et al., 2021, p. 10, is licensed under CC BY 4.0).
The data acquisition area of DiKoLAN (Becker et al., 2020) describes competencies for the direct or indirect measurements of data using digital tools. Data acquisition systems handling is covered by the competency DAQ.S.A1, “Perform setup, calibration, and data acquisition for at least one example each of the above-mentioned range of application for digital data acquisition.” The data acquisition competency area extends beyond the digital acquisition of measurement data (referred as computer-aided data acquisition in Becker et al., 2020) and addresses also the use of other digital technologies and media, such as thermal imaging cameras, mobile phones, and video analysis tools. We argue that describing the handling of a technology/medium based on one competency is insufficiently differentiated to identify, address, and promote the appropriate TRCs from (pre-service) science teachers for handling highly subject-specific technologies/media. The fact that DiKoLAN describes the use of technologies/media for data acquisation with only one competency is unsurprising in light of the fact that DiKoLAN is an basic competence model and does not claim to completeness. von Kotzebue et al. (2021) suggested that “competency areas can be adapted and supplemented” (p. 5). In the context of this work, we contribute to this knowledge gap by more precisely differentiate the handling of digital data acquisition systems in educational lab settings.
The aim of this paper is not only describing the TRCs of handling digital data acquisition systems but also assessing them empirically. Therefore, Lachner et al. (2019) described different ways of determining TPACK dimensions: self-report assessments, performance assessments, and measurements of availability versus quality. In this paper, we focus on self-efficacy as a self-reporting assessment tool, which is a well-documented analog to competency (e.g., Baer et al., 2007; Gehrmann, 2007; Rauin and Meier, 2007). Furthermore, it is a predictor of actual teacher behavior (Tschannen-Moran and Hoy, 2001). Self-efficacy reflects one's perceived ability to cope successfully with challenges in non-routine situations and is a prerequisite for experiencing one's own competence (Bandura, 1997; Schwarzer and Jerusalem, 2002; Pumptow and Brahm, 2021). Among other things, self-efficacy depends on various experiences (Bandura, 1997). For example, experimenting with digital data acquisition systems in lab courses can influence one's self-efficacy in handling digital data acquisition systems as the experimenter can gain experience and confidence. The use of self-efficacy to measure self-assessed competencies is advantageous in that it is an economical approach (i. e., easily accessible and inexpensive; Scherer et al., 2018; Lachner et al., 2019). Moreover, it is reliable and valid, especially regarding technology handling (Scherer et al., 2017, 2018; Lachner et al., 2019). Notably, the use of self-efficacy as an analog for competency is not free of criticism, as it depends on social desirability and the subjective perception of one's true competency (Archambault and Barnett, 2010; Brinkley-Etzkorn, 2018), which is generally weakly related to actual performance (e. g., Kopcha et al., 2014; Akyuz, 2018; von Kotzebue, 2022). Nonetheless, capturing self-efficacy as an analog for competency level seems appropriate given that it is a predictor of technology acceptance in classrooms (Ifenthaler and Schweinbenz, 2013; Scherer et al., 2019).
In the context of teacher education, a large body of research has focused on capturing and identifying technological-related self-efficacy (e. g. Schmidt et al., 2009; Archambault and Barnett, 2010; Scherer et al., 2017; Scherer et al., 2018; Schmid et al., 2020; von Kotzebue, 2022; overview in Chai et al., 2016; Willermark, 2017). Willermark (2017) showed that 71.8 % of existing TPACK instruments capture self-efficacy, and of these, only 4 % relate to specific teaching scenarios. The specific contextualization of context/situation and technology/medium of self-efficacy is preferable because self-efficacy should always be measured in terms of its context (Bandura, 1997, 2006). Furthermore, the meanings of “media” and “technology” are often unclear (Willermark, 2017). Regarding media, subject specificity is discussed in most corresponding studies, but the descriptions are often quite superficial (von Kotzebue, 2022). For example, Schmidt et al. (2009) referred to context of “science,” which is to nebulous to characterize adequately for measurement. Regarding technologies, the application must be discretely account to avoid fuzziness being applied to the TK construct; otherwise, the focus areas cannot be clearly distinguished (Archambault and Barnett, 2010; Willermark, 2017; Scherer et al., 2018). Ackerman et al. (2002) showed that general self-efficacy correlates to technological applications more than specific self-efficacy. Owing to the prevalence of general TPACK self-efficacy scales, their relevancy has to be questioned with regards to capturing highly subject-specific technological-related processes, such as handling digital data acquisition systems during lab work.
Recently, a number of very subject-specific instruments exist by Deng et al. (2017), von Kotzebue et al. (2021), and Mahler and Arnold (2022), that capture subject-specific TPACK in terms of self-concept, self-efficacy, and performance assessment in biology and chemistry contexts. The scales show due to their inherently higher contextualization higher discriminatory validity (von Kotzebue, 2022). However, these instruments remain superficial in their technology references as they also use the formulation of “technology”, which lacks clarity as described above. For this reason, this study assesses the highly subject-specific technological-related self-efficacy of dealing with digital data acquisition systems during experimentation.
Against this backdrop, this is the first study (that we know of) to elucidate how general TRCs relate to highly subject-specific situations. This must be clarified because a significant portion of media/technological-related self-efficacy research relates to general assessments, and it remains unclear whether these scales can be used to describe and assess the highly subject-specific handling of digital data acquisition systems. We argue that separate offerings and evaluation tools are needed to promote and evaluate TRCs when handling highly subject-specific technologies so that (pre-service) physics teachers can take advantage of the didactic potential given by digital technologies and media. von Kotzebue (2022) discussed that basic TRCs must first be promoted before the subject and its PK can be accounted for. In this article, we address this by clarifying whether general scales and competency frameworks, which are used up-to-now to assess the handling of everyday technologies and media, can adequately capture and explain the use of highly subject-specific technologies and media such as digital data acquisition systems by (pre-service) physics teachers. In other words, are more differentiated frameworks, scales, and explicitly targeted courses needed to train teachers in a targeted way?
Our studies addressed the following research questions (RQs):
(RQ 1) What specific TRCs do (pre-service) physics teachers need to handle digital data acquisition systems in educational lab work settings?
(RQ 2) How can (pre-service) physics teachers' self-efficacy regarding the handling of digital data acquisition systems in educational lab work settings be measured?
(RQ 3) How is self-efficacy regarding the use of digital data acquisition systems in educational lab work settings related to more general constructs, such as TK- and TPK-related self-efficacy?
Two studies are conducted to answer these RQs. The first applies a qualitative approach to answer RQ 1 by identifying and empirically validating physics-specific digital TRCs when using digital data acquisition systems in educational laboratory settings. The second answers RQ 2 and RQ 3 by developing a technological-related self-efficacy instrument for handling with digital data acquisition systems in educational lab work settings and empirically comparing the highly subject-specific technological-related self-efficacy needed in educational lab work settings to handle with digital data acquisition systems in educational lab work settings to the general technological-related self-efficacy of pre-service physics teachers. Figure 2 shows the individual steps taken to identify the competencies and the self-efficacy scale.
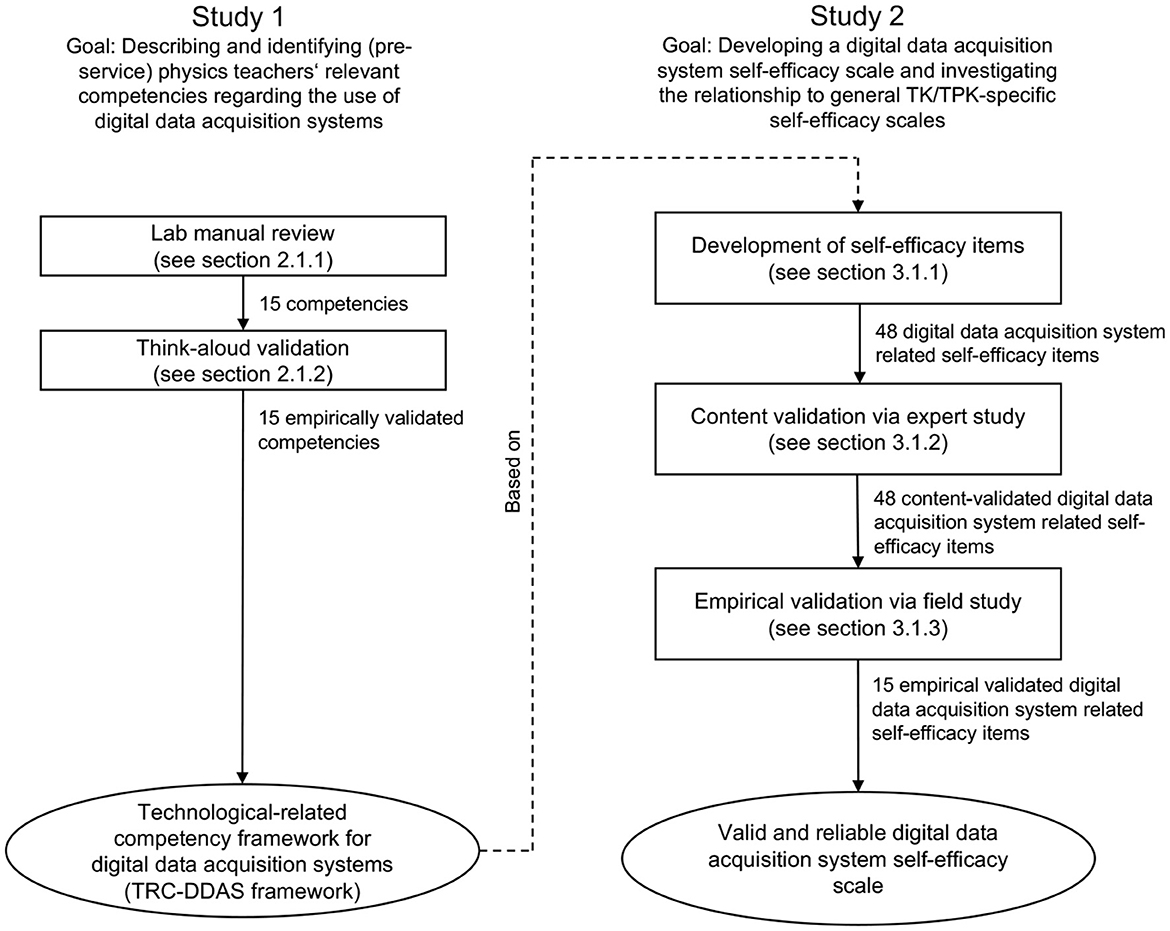
Figure 2. Performed steps to develop the technological-related competency framework for digital data acquisition systems (TRC-DDAS framework) and the abbreviated digital data acquisition system self-efficacy scale.
2. Study 1: developing and evaluating a fine-grained technological-related competency framework for digital data acquisition systems
2.1. Method
2.1.1. Identifying the technological-related competency framework for digital data acquisition systems
First, 15 lab manuals from typical digital data acquisition systems educational material manufacturers (e. g., Phywe, Leybold, Pasco, and Vernier) were selected and analyzed to identify the necessary practical steps for achieving the manuals' postulated goal(s). During the selection process, care was taken to ensure that the manuals were as diverse as possible while covering a variety of teaching material, physics education topics, analysis methods, and physics measurands. An appropriate lab manual step would, for example, require the student to perform a practical activity (e. g., press an icon on a computer to launch the digital data acquisition system acquisition software) and apply underlying technical knowledge (e. g., describe the concept of “sample rate”). The identified practical steps were cleansed to describe only the TRCs according to Mishra and Koehler (2006) and contain operators (e. g. “(Pre-service) physics teacher installs the sensor correctly”). Finally, the competencies were compared to those of the DiKoLAN framework (Becker et al., 2020).
2.1.2. Think-aloud validation of technological-related competency framework for digital data acquisition systems
To obtain empirical evidence regarding the identified TRCs in using digital data acquisition systems in an educational lab work setting (summarized as TRC-DDAS framework), a think-aloud study was conducted. The primary goal of this study was not to uncover additional competencies but to empirically prove the competencies found in the lab manual review, i.e., which competencies play an actual role. For this purpose, the study participants first received think-aloud training. The method requires participants to continuously express all thoughts, actions, and impressions about the process in which they parake (Ericsson and Simon, 1980; Eccles, 2012). In this case, each participant worked in two of three settings to distribute and lessen the workload. The contents of three exemplary lab manuals were chosen for diversity, and, apart from those directly related digital data acquisition systems (e. g., integration, data acquisition, data storage, and presentation), each included the goal of the lab work, identified the necessary physics knowledge, and provided a full description of the experimental setup (e. g., resistors properly placed on a plug-in board). Setting 1 is covered mechanics (measurement of velocity and acceleration of a constantly accelerated body), Setting 2 covered acoustics (measurement of the pressure variation of a struck tuning fork) and Setting 3 covered electricity (recording of the characteristic curve of a light bulb). More detailed descriptions are provided in the Supplementary material.
Participants were provided with a selection of digital data acquisition systems with corresponding sensors from the various educational manufacturers, from which they were open to choose. These are the latest available versions of digital data acquisition systems, including digital sensors from manufacturers such as Phywe, Leybold, Pasco, and Vernier. The selection of a particular system, including sensors, was left to the participants as part of the study. The reasons for this were to keep the experimentation process as open as possible and because we suspected a competency in selecting an appropriate digital data acquisition system (based on the lab manual review).
2.1.2.1. Sample
In this study, n = 4 pre-service high school physics teacher students (two male gender, two female, none nonbinary) from our institution's master's program participated. In an informal pre-interview, the participants reported that they had little or no prior knowledge of digital data acquisition systems. They reported that their basic experiences were the results of coursework.
2.1.2.2. Data analysis
The think-aloud narratives were transcribed and sorted by two coders who used a structured content analysis to organize the practice steps into the TRC-DDAS framework with a reliably (Cohen's κ) of 0.68 (Döring and Bortz, 2016; Kuckartz, 2018). This allowed the TRC-DDAS framework to be inductively extended, revised, and empirically tested (see Section 2.2.1).
2.2. Results
2.2.1. Technological-related competencies using digital data acquisition systems
Table 1 show the TRC-DDAS framework, which comprises 15 fine-grained digital data acquisition system competencies for educational lab work settings. The results were derived from a review of the lab manuals, DiKoLAN (Becker et al., 2020) comparisons, and the think-aloud study.
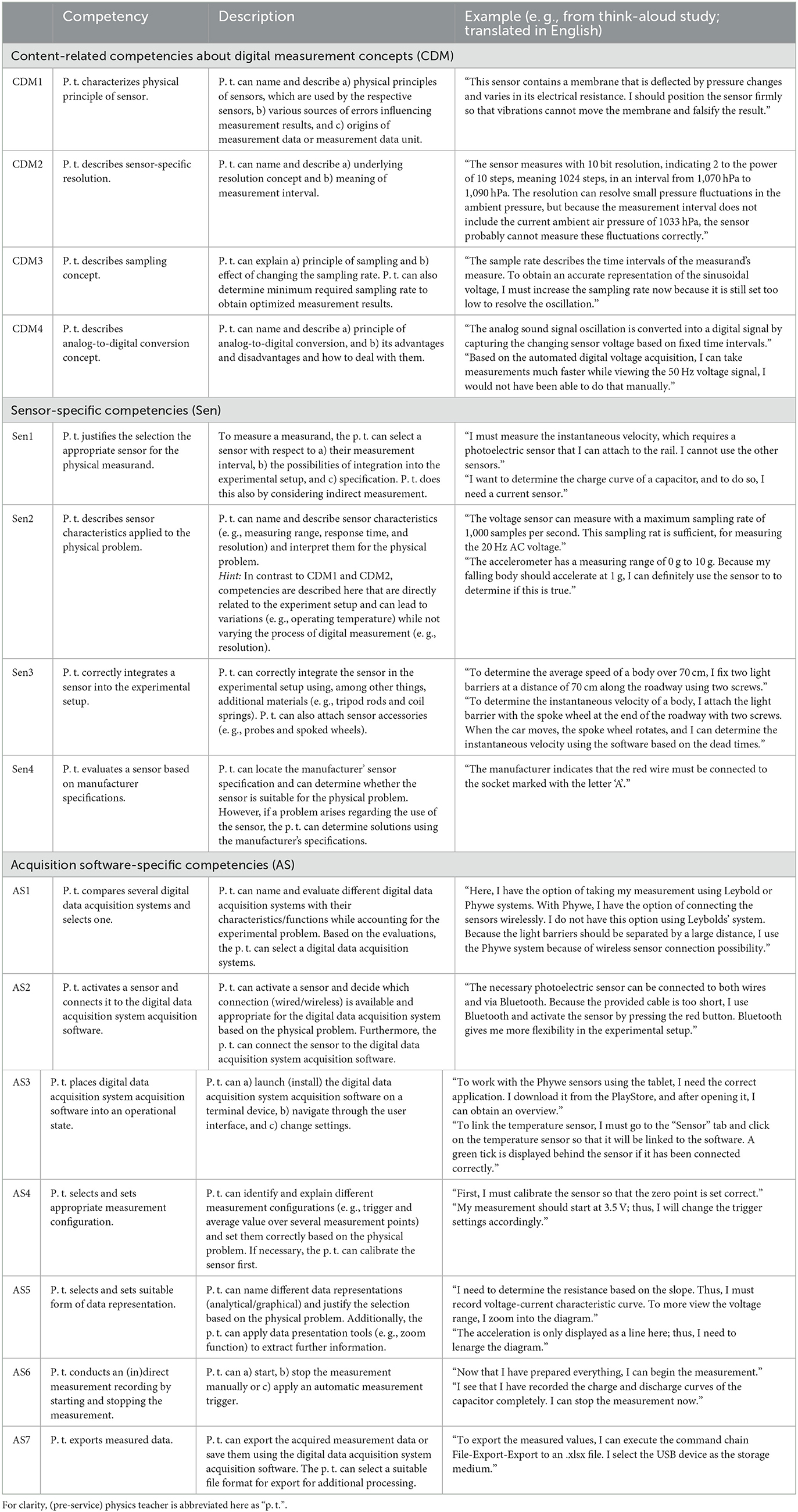
Table 1. TRC-DDAS framework consisting of 15 competencies that can be assigned to the three dimensions (i. e., concepts of digital measurement-, sensor-, and acquisition software-specific), including competency descriptions and examples from the think-aloud study.
We created a three-dimensional content-related structure comprising content-related competences about concepts of digital measurement, sensor-specific competencies, and digital data acquisition system acquisition software-specific competencies. The concepts of digital measurement dimension covers physical-layer digitization and rendering methods, such as the principle of analog-to-digital conversion and the underlying physical principles of a sensors (e. g., pressure-based piezoresistors). Contrary to sensor-specific and acquisition software-specific, concepts of digital measurement competencies can be observed apart from the experimentation process. Sensor-specific competencies are directly related to the application of digital sensors and can be observed during hardware selection, installation, calibration, and during experimentation. The acquisition software-specific dimension includes competencies directly related to the digital data acquisition system acquisition software, as well as the digital data acquisition system as a total solution for data acquisition. Examples include data acquisition, data formatting, sensor software settings, and connectivity between sensors and digital data acquisition system acquisition software.
Based on the DiKoLAN comparison, no additional dimensions were identified.
2.2.2. Validation of the identified competencies related to digital data acquisition systems
From the think-aloud study, all 15 derived competencies were empirically demonstrated. Furthermore, every aspect was supplemented or further elaborated that did not emerge from the lab manual review or the DiKoLAN framework. Table 1 lists descriptions and examples of each competency.
The participants used different digital measurement systems with corresponding sensors in the same lab work settings, generally demonstrating the same competencies. If a participant did not need a certain competence, it was because that participant used a different approach in the experimentation process than the other participants. Nevertheless, saturation of the identified competencies was not achieved, but as we clarify in Section 4.4.1, the goal was not to identify all possible emerging competencies, only those that play an actual role while experimenting in physics classrooms.
3. Study 2: developing a self-efficacy questionnaire for dealing with digital data acquisition systems and investigating the relationship to general technological-/technological-pedagogical-knowledge-specific self-efficacy scales
It is vital to accurately identify and describe the needed highly subject-specific TRCs (e. g., handling of digital data acquisition systems in educational lab work settings) so that specific interventions can be developed. It is also important to empirically validate the interventions (Krauss et al., 2008; Voss et al., 2011). For this purpose, a digital data acquisition system self-efficacy item battery assessment was developed. For testing economy, the scale was shortened, and evidence for content and construct validity was provided (according to RQ 2). To answer RQ 3, this study determined whether more general TK/TPK-specific self-efficacy scales could be used to assess digital data acquisition system self-efficacy by examining the relationships between the scales.
3.1. Method
3.1.1. Development of digital data acquisition system self-efficacy items
A questionnaire with 48 digital data acquisition system self-efficacy items based on the 15 competencies and their TRC-DDAS framework distribution (see Section 2.2.1) was developed. The items were formulated context- and situation-specifically due to existing recommendations of Bandura (2006), as well as technological-specific for handling of digital data acquisition systems.
3.1.2. Investigating content validity
The 48 digital data acquisition system self-efficacy items were provided to the experts who were tasked to assign them to the three TRC-DDAS competency dimensions. We want to stress out, that the items do not capture competencies; instead, the items capture the digital data acquisition system self-efficacy and the task was to assign them to their underlying competency dimensions. This approach was sufficient because the items were developed using the TRC-DDAS competencies, and self-efficacy is a well-documented analog to competency, as outlined in Section 1.
3.1.2.1. Sample
For test economy, a sample of n = 3 science didactics experts were recruited, each with at least a master-degree and experience modeling TRCs in science teacher education.
3.1.2.2. Data analysis
Assignment reliability was assured using the Fleiss κ, as the data were unordered and nominal. The expert assignment reliability had a Fleiss κ = 0.89 and can be interpreted as reliable. Any items that could not be assigned by at least one expert to a competency dimension or that were assigned to more than one were excluded to ensure unidimensionality. As a result, 31 items remained.
3.1.3. Empirical investigation of of the digital data acquisition system self-efficacy items
Further validation criteria (Messick, 1995) were examined empirically of the set of 31 items. Following Messick (1995), we focused on construct validity. The study design included first assessing the control variables, followed by digital data acquisition system self-efficacy and general TK/TPK-specific self-efficacy (answering RQ 3).
The study comprised an online questionnaire due to COVID-19 lockdown in the winter of 2020 and early 2021 and for test economy reasons.
3.1.3.1. Sample
In this study, n = 69 pre-service physics teachers from 16 universities across Germany participated. The goal was to sample participants from all physics education students to ensure functionality across all years. Such as, 39 participants were in a bachelor's program, and 30 were in a master's program. Personal data, such as gender or age, were not requested.
3.1.3.2. Operationalization
Digital data acquisition system and TK/TPK-specific self-efficacy in using everyday digital media was acquired via five-step Likert scale which 0 means “does not apply” and 4 means “fully applies”. TK/TPK-specific self-efficacy items from Schmidt et al. (2009) were used in a modified manner (e. g., the term “digital technologies” was replaced with “digital media”; for TK-specific self-efficacy: “I have the technical skills I need to use digital media.”, for TPK-specific self-efficacy: “I can choose digital media that enhance students' learning for a lesson.”) and translated into German. Full item text can be found in the Supplementary material.
The number of completed university semesters and attended courses related to digital data acquisition systems based on self-assessed semester hours were recorded to control for digital data acquisition system self-efficacy. As described in Section 1, one's experience may influence self-efficacy by providing contact time and gaining their own experience with digital data acquisition systems (Bandura, 1997).
3.1.3.3. Data analysis procedures
Analyses were performed in three steps. First, the scale was reduced to a total of 15 items (five per TRC-DDAS dimension) to improve future test economy and reduce the number of estimated parameters in statistical methods in the field of latent variable modeling. Therefore, item reduction was performed within the three scales concepts of digital measurement-specific self-efficacy, sensor-specific self-efficacy, and acquisition software-specific self-efficacy (resulting from the TRC-DDAS dimensions), and care was taken to ensure that the items addressed as many facets of digital data acquisition system self-efficacy as possible by ensuring that the selected items could remain assigned to different competencies of the TRC-DDAS dimensions. The reduction was carried out using the psych package (Revelle, 2016) in R (R Core Team, 2020). An additional selection coefficient1 was calculated for item reduction because selection purely on the basis of discriminatory power does not differentiate extreme trait expressions, as differences in item difficulty reduce the inter-item correlation (Lienert and Raatz, 1998; Moosbrugger and Kelava, 2020). Items with the highest discriminatory power and selection parameters were selected.
Second, to investigate the three-factorial structure of the scale, a confirmatory factor analysis (CFA) with the reduced digital data acquisition system self-efficacy scales was computed using lavaan package (Rosseel, 2012) in R (R Core Team, 2020). The three-factorial assumption stems from the provision of three TRC-DDAS competency dimensions. Maximum likelihood procedures with robust standard errors were performed using the full-information maximum likelihood method estimation to prevent bias caused by missing values (< 0.5% in our study; Enders and Bandalos, 2001). This also accommodated the small sample size (Lei and Wu, 2012; Rhemtulla et al., 2012). Parameter estimation was assessed using both the χ2-test statistic and several relative model fit indices [e. g., the root-mean-square-error of approximation (RMSEA), standardized-root-mean-residuum (SRMR), and comparative-fit index (CFI)]. Limits described by Bentler (1990) and Brown (2006) were used to evaluate model fitness (RMSEA ≤ 0.08, SRMR ≤ 0.08, CFI ≥0.90). A χ2-difference test of the robust parameters was used to compare the one-factorial model with the three-factorial model, as this allowed us to check to see if the more constrained model fit the data better.
Third, to answer RQ 3, Pearson product-moment correlations were calculated using the manifest means of the scales to identify relationships between the three digital data acquisition system self-efficacy scales and the TK/TPK-specific self-efficacy scales and control variables. These analyses were also performed in R (R Core Team, 2020).
3.2. Results
3.2.1. Content and construct validation of the digital data acquisition system self-efficacy questionnaire
As a part of the expert study, the experts assigned 31 digital data acquisition system self-efficacy items unambiguously to the three competency dimensions of the TRC-DDAS framework to ensure a one-dimensional structure. Seventeen items could not be assigned unambiguously or were no assigned at all; hence, they were excluded from further analysis. The excluded items can be found in the Supplementary material. The 31 remaining items (both in the German language used in the studies presented and in the English translation for this publication) are presented in the Supplementary material. Seven items were assigned to concepts of digital measurement dimension, seven to sensor-specific dimension, and 17 to the acquisition software-specific dimension. Consequently, content validity was ensured for all 31 items.
The discriminatory power, r, of the concepts of digital measurement-specific self-efficacy items fell in the interval 0.52 < r < 0.74, that of sensor-specific self-efficacy items in 0.30 < r < 0.74, and of the acquisition software-specific self-efficacy items in 0.28 < r < 0.74. The psychometric parameters for all 31 items are listed in the Supplementary material. For additional analyses and for future test economy, the 15 items with the highest discriminatory power and selection coefficients were used for the three shortened scales. Table 2 lists the shortened scales and their psychometric parameters. The results presented in the following refer to the shortened scales.
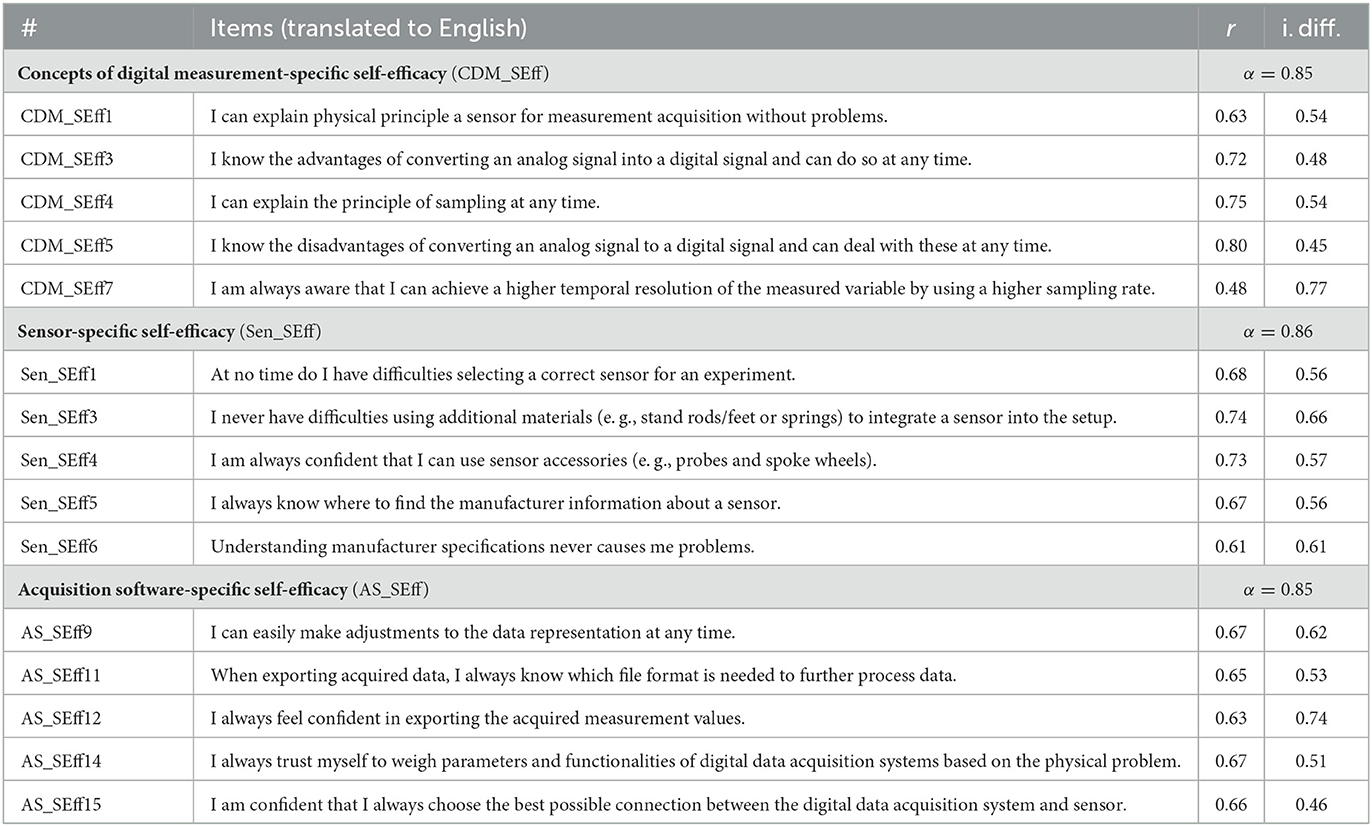
Table 2. Overview of the shortened digital data acquisition system self-efficacy items according to associated TRC-DDAS competency dimensions, with corresponding item difficulties (i. diff.), discriminatory powers r, and Cronbach's α.
The factorial structure was examined by testing a one-factorial model against a three-factorial model. It is shown that the three-factorial model (χ2(87) = 140.10, p < 0.001, CFI = 0.91, RMSEA = 0.09, SRMR = 0.06) had a better model fit than the one-factorial model (χ2(90) = 172.40, p < 0.001, CFI = 0.86, RMSEA = 0.12, SRMR = 0.07). Furthermore, the χ2-difference test favored the three-factorial model because the χ2-value is smaller; hence, there was a significant difference between the models (Δχ2(3) = 22.97, p < 0.001).
Reliability was determined based on the discriminatory power and in terms of internal consistency (Cronbach's α) of the shortened scales. Their internal consistency were for concepts of digital measurement-specific self-efficacy α = 0.85, sensor-specific self-efficacy α = 0.86, and acquisition software-specific self-efficacy α = 0.85. These can be rated as high (Weise, 1975; Bühner, 2011). The discriminatory power of the items was rated high to very high (concepts of digital measurement: 0.48 < r < .80, sensor-specific: 0.61 < r < 0.73, and acquisition software-specific: 0.63 < r < 0.67; Moosbrugger and Kelava, 2020). Table 2 shows the discriminatory power of the shortened scale items.
3.2.2. Relationships between digital data acquisition system self-efficacy scales and technological-/technological-pedagogical-knowledge-specific self-efficacy and control variables
The relationships among the scales and their control variables are shown in Table 3. Weak and moderate correlations (0.29 < r < 0.44) were found between the digital data acquisition system and TK/TPK-specific self-efficacy scales (Cohen, 1988). Further, a weak correlation was identified between the number of university semesters completed and the concepts of digital measurement-specific self-efficacy (r = 0.28; Cohen, 1988). Weak and moderate correlations were identified between the number of digital data acquisition system-related courses attended and sensor-specific self-efficacy (r = 0.35) and acquisition software-specific self-efficacy (r = 0.28; Cohen, 1988).
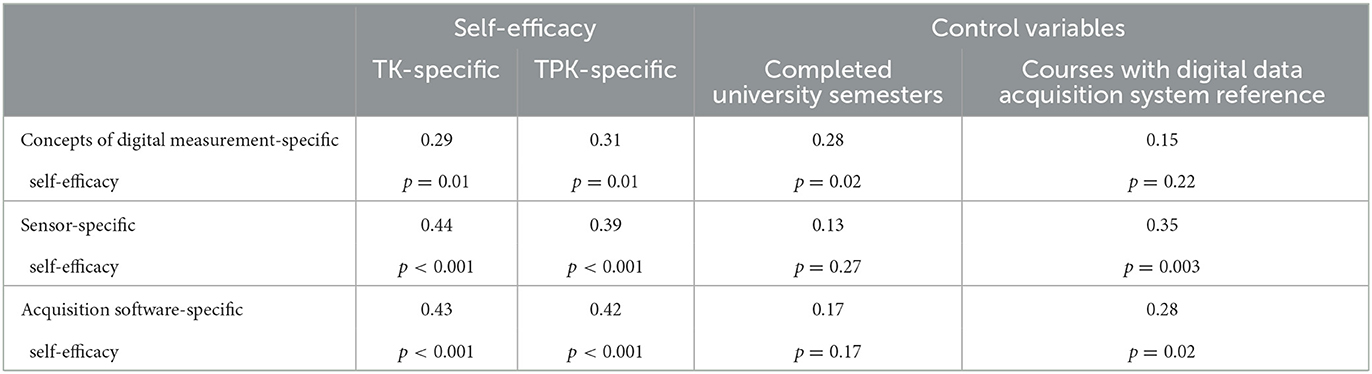
Table 3. Pearson product-moment correlation coefficients testing the associations between digital data acquisition system self-efficacy scales and the general TK/TPK-specific self-efficacy scales, as well as the control variables number of university semesters and courses with digital data acquisition system reference.
4. Discussion
In this paper, we asserted that there were no evaluated competency frameworks and evaluation tools for describing and assessing TRCs in a fine-grained way that deal with highly subject-specific digital media/technologies, and allow development of targeted interventions to promote certain competencies. Therefore, we identified the need to create appropriate competency frameworks and assessment instruments to more fully develop interventions in this regard (according to RQ 1 and RQ 2).
4.1. Technological-related competency framework for digital data acquisition systems: an instrument for describing fine-grained digital technological-related competencies for digital data acquisition systems use
To address the postulated desideratum, we developed the TRC-DDAS framework as a first step. This competency framework comprises 15 TRCs for handling digital data acquisition systems in educational lab work settings. The TRC-DDAS framework breaks down the digital measurement process for data collection in way that is more fine-grained than other frameworks, including DiKoLAN (Becker et al., 2020). For example, TRC-DDAS framework separates the expectation of competence DAQ.S.A1 “Perform setup, calibration, and data acquisition for at least one example each of the above-mentioned range of application for digital data acquisition” (Becker et al., 2020) from DiKoLAN framework into Sen3, AS2, AS3, and AS6. This increases the potential of the TRC-DDAS framework in terms of offering more starting points for specific competency development and promises to facilitate the resolution of potential problem areas in physics teacher education. Furthermore, the TRC-DDAS framework is content-valid due to being based on the identification of lab manuals that use digital data acquisition systems for data collection. Other competency frameworks were accounted for, such as DiKoLAN, and we observed competencies from the think-aloud study that were reliably mapped to the TRC-DDAS framework. As described, in contrast to DiKoLAN, we chose an approach based on a thorough review of lab manuals and a think-aloud study, which allowed us to identify a larger set of relevant competencies related to digital data acquisition systems. Hence, the new framework is better suited to promote the handling of digital data acquisition systems in physics teacher programs. Moreover, by reaching the same results using two different approaches, content validity can be strengthened for both frameworks.
We also identified new aspects that DiKoLAN (Becker et al., 2020) missed, such as a recourse to the various digital data acquisition system manufacturers' data sheets (e. g., Sen4) or the content-related competencies on digital measurement concepts (CDM1, CDM2, CDM3, and CDM4). The use of digital data acquisition systems data sheets to solve problems during experimentation with digital data acquisition systems and basic fundamental understanding of digital measurements, are essential from metrological, physical, and physics educational perspectives. For example, if one cannot understand the concept of a sampling rate, it would not be possible to justify the concepts of digital measurement during the lab work due to the sample rate being inextricably linked to the phenomenon studied. For example, when digitally measuring the displacement-time law of a Hooke's oscillator with an eigenfrequency of f = 4Hz, the sample rate must be set to at least fs = 8Hz. We are not insinuating that the DiKoLAN framework cannot be used to address these aspects; however they are only implicitly covered. This is not surprising at this point since, as we have stressed, the objective of DiKoLAN is to provide a basic competency model that addresses digitization-related aspects in science teacher education; it never claimed to offer a complete digital data acquisition system rubric.
As a result of this study, the new TRC-DDAS framework is now available, and it describes 15 fine-grained competencies that (pre-service) physics teachers must master in educational digital data acquisition system-supported lab work settings. Furthermore, the framework can be used to extend DiKoLAN (Becker et al., 2020) in all digital data acquisition system-related aspects as it allows one to develop appropriate interventions, as well as qualitative and quantitative evaluation tools. We assert that the TRC-DDAS framework is the first that can be used to plan tailored interventions and university courses for pre-service physics teachers on modern physics curriculum with focus on dealing digital data acquisition systems, especially since we were able to observe the identified competencies in dealing with different digital data acquisition systems and under different procedures in the experimentation process. Further, the competency formulations of the TRC-DDAS framework were designed in such a way that the competencies described are independent form the characteristics of specific materials and different manufacturers and thus allow the handling of digital data acquisition systems developed in the future, those from new manufacturers, and the usage of new functions/methods in the acquisition software to be described.
4.2. Digital technological-related self-efficacy questionnaire on the use of digital data acquisition systems: an assessment tool for evaluating educational lab settings
To further address the postulated desideratum, a three-factorial digital data acquisition system self-efficacy scale was established. Compared with similar instruments (e.g., Deng et al., 2017; von Kotzebue, 2022; Mahler and Arnold, 2022), our scales are not only subject-specific, but they also address the use of a highly subject-specific digital technology: digital data acquisition systems. The digital data acquisition system self-efficacy scales are characterized by high reliability and discriminatory power. Considering that its Cronbach's α and discriminatory power are sensitive to the number of items (e. g., Bühner, 2011; Moosbrugger and Kelava, 2020), and that five-items scales can be classified as low, the results show high measurement accuracy and testing validity. The scales of other subject-specific instruments have comparable internal consistencies. Furthermore, one-dimensionality was ensured by the expert study. Thus, the digital data acquisition system self-efficacy assessment instrument can be used to investigate the growth of competencies through self-reported digital data acquisition system self-efficacy measures in lab settings by (pre-service) physics teachers.
In terms of construct validity, we found that digital data acquisition system self-efficacy measures can be differentially considered based on concepts of digital measurement-, sensor-specific and acquisition software-specific self-efficacy. In contrast, the scales of Deng et al. (2017), Mahler and Arnold (2022), and von Kotzebue (2022), which are subject-specific but not technology/medium-specific, assume a single-factor subject-specific TK assessment. Our three-factorial structure allows future interventions to developed competencies tied strongly to concepts of digital measurement-, sensor- and acquisition software-specific aspects. Pertaining to TK assessment tool development, the use of digital technologies and media must be further differentiated to better identify multi-factorial structures. Frameworks such as DiKoLAN (Becker et al., 2020) suggest multi-factorial structures within the TPACK dimensions; however, they have not yet been empirically investigated and lack granularity.
The fact that a three-factorial model fits better than a one-factorial model increases the construct validity of the TRC-DDAS framework. Notably, we organized the competencies into three intuitive utilitarian domains and supported them with valid empirical evidence. Assuming that self-efficacy assessment correlates well to competency expression (see Section 1), the three-factorial structure found can be applied to the TRC-DDAS framework and strengthens its construct validity.
4.3. Relationship between subject-specific self-efficacy when dealing with digital data acquisition systems and general technological-/technological-pedagogical-knowledge-specific self-efficacy
As presented in Section 1, plenty of general TPACK assessments have been provided, but they have little relevance to the application of highly subject-specific digital technologies and media (i. e., digital data acquisition systems), especially in highly subject-specific situations, such as digitally-supported experimentation settings. We successfully identified low- and medium-strength correlations between general TK/TPK-specific self-efficacy and our digital data acquisition system self-efficacy scales, which support the claims that general TK/TPK-specific self-efficacy scales are insufficient by themselves in describing dealing with highly subject-specific technologies in highly subject-specific situations, especially when the relevant technologies and media extend beyond general TK! Reasons for the rather low and medium strengths of the correlations relate to there being different underlying situations when handling highly subject-specific digital technologies and media rather than everyday digital technologies and media. This disparity directs the use of different TRCs for adequate handling. On the other hand, it may be the case that our digital data acquisition system self-efficacy scales captured TCK-specific self-efficacy due to their proximity to the experimentation process and the fuzzy distinctions among the TPACK (Mishra and Koehler, 2006) knowledge components (Cox and Graham, 2009; Archambault and Barnett, 2010; Jang and Tsai, 2012; Willermark, 2017; Scherer et al., 2018). These aspects suggest that highly subjective thinking is needed if TRCs are to be used to handle digital technologies and media. Consequently, specific competency frameworks and evaluation instruments are needed, as we have demonstrated.
Furthermore, we controlled for the influence of completed university semesters and number of attended digital data acquisition system-related courses on digital data acquisition system self-efficacy, and we noted low- and medium-strength correlations between sensor/acquisition software-specific self-efficacy and the number of digital data acquisition system-related courses attended, as well as the number of university semesters completed and concepts of digital measurement-specific self-efficacy. However, correlations between sensor/acquisition software-specific self-efficacy and university semesters completed and concepts of digital measurement-specific self-efficacy and digital data acquisition system-related courses attended cannot be hypothesized. Assuming that pre-service physics teachers gain higher content-related competencies with increasing numbers of university semesters, the results suggest that studying longer enables pre-service physics teachers to deal better with content-related concepts of digital measurement aspects; however, it does not enable them to deal with digital data acquisition systems and their corresponding sensors. This means that studying (in physics education) alone is insufficient; digital data acquisition system-related competencies explicitly require digital data acquisition system-related courses in teacher education programs! Therefore, their presence in curricula is essential. Ultimately, these findings reinforce the notion that we must go beyond dealing with general technologies, especially in physics (and other laboratory-based science fields) for teacher education.
While this has been exemplified in the present work using digital data acquisition systems that is primarily in the field of physics, we are convinced that this claim can be equally extended to other science sub-disciplines (such as biology and chemistry), not only because digital data acquisition systems plays a role in these subjects (such as measuring the PH value during a titration with digital devices), but also because there are a number of chemistry- and bio-specific digitally-supported methods in the laboratory field (e. g., photometry, spectroscopy).
4.4. Limitations
4.4.1. Limitations in the development and evaluation of the technological-related competency framework for digital data acquisition systems
The validity of the TRC-DDAS framework competencies may be perceived as limited to the lab manuals that we happened to include in our study. Given the wide range of available sensors, experimental setups, system handling procedures, and experimentation constraints there may be room for TRC-DDAS framework expansion in terms of experimentation scenarios. Hence, the addition of lab manuals could be used to identify new aspects of the many shared competencies. Nevertheless, facing limited time and resources, we ensure that our selection was strongly and comprehensively related to the TRCs of the most generalizable digital data acquisition system applications.
Additionally, because we want to put science teacher education practices at university level forward, the think-aloud study was limited to pre-service physics teacher students who each participated in two of three exemplary lab work settings. As a future study, it may be beneficial to consider physics teachers with several years of professional experience and strong competencies in handling digital data acquisition systems, as doing so may reveal new facets of these competencies. The fact that our think-aloud study was limited to three exemplary contexts may also seem to limit the generalizability of the TRC-DDAS framework to the full scale of digital data acquisition system applications. Hence, additional settings could be added to future evaluations. Nevertheless, the three settings that were selected represent the fullest extent of TRC diversity. Hence, the triangulation of competencies was quite strong, and the content validity remains empirically valid, especially noting that DiKoLAN includes the same competencies. Further, the aim of the think-aloud study was also to confirm the competencies from the lab manual review, as we believe they play an actual role in educational lab work settings, and not to identify all competencies, which could vary by setting and lab worker competence, among other factors. That is why saturation of the identified competencies was not ensured.
4.4.2. Limitations in the development and evaluation of the self-report questionnaire on self-efficacy in dealing with digital data acquisition systems
As described, the results of the empirical study are based on a relatively small sample of n = 69, which might suggest that the results ought to be interpreted cautiously. However, we argue that the sample was quite sufficient to the study objectives because it was relatively representative of pre-service physics teachers in Germany as the selection of participants is enrolled at a total of 16 German universities. Moreover, the estimation procedures, among other things, were robust to small samples (Lei and Wu, 2012; Rhemtulla et al., 2012).
We also excluded several indicators to form shortened scales to contrast the results. However, it can be argued that doing so may have increased the risk of overfitting (Henson and Roberts, 2006).
4.4.3. Limitations in the evaluation of correlations between the highly specific digital data acquisition system and general technological-/technological-pedagogical-knowledge-specific self-efficacy scales
Notwithstanding the assurances provided, the identified correlations should be interpreted with some caution as it is well known that the validation processes of measurement instruments and the subsequent derivation of theses are controversial in practice. Actually, a separate sample would have been taken, or the given sample could have been randomly subdivided for testing and validation (of the self-efficacy relationships). The first option was desirable but strongly hampered by COVID-19 and other test economy restrictions. However, the degrees-of-freedom complications of the latter would have been impossible to overcome. Future research should validate these correlations with sufficient time and resources.
5. Conclusion
In conclusion, the use of digital technologies and media in physics education has become a standard practice for which highly subject-specific digital media and technologies (e. g., simulations and digital data acquisition systems) are available alongside everyday media and technologies (e. g., presenters and laptops). We claimed that for ensure the adequate handling of these highly subject-specific digital media and technologies, highly subject-specific TRCs are needed that are distinct from the competencies needed for everyday technologies and media. Using the one example, dealing with digital data acquisition systems in educational lab settings, we showed that general TK/TPK-specific self-efficacy scales that are neither subject-specific nor address the use of a highly subject-specific technologies are sufficient for this purpose.
In the context of the two studies designed to answer RQ 1, RQ 2, and RQ 3, we first developed the TRC-DDAS competency framework (see Table 1), which, unlike to other competency frameworks, describes the fine-grained competencies needed for dealing with digital data acquisition systems. We then provided an associated abbreviated self-efficacy scale (see Table 2) for dealing with digital data acquisition systems. The TRC-DDAS framework can be used to identify and promote appropriate competencies in pre-service physics teachers courseware and dedicated learning environments that address different competencies. The shorted self-efficacy scale can be used for further evaluate of these learning environments.
A comparison of self-efficacy in dealing with everyday media and technologies and digital data acquisition system self-efficacy showed that both are only slightly-to-moderately related, and the self-efficacy in handling digital data acquisition systems can be increased by specifically targeted courses and not studying alone. Based on these results, we strongly suggest that future digital education directed in subject-specific terms and not in general! To train pre-service teachers in the best ways possible to handle highly subject-specific digital media and technologies, more specifically oriented coursework are needed. Furthermore, to guarantee up-to-date teaching with digital media and technologies, the training programs must be strongly tailored to provide this specific knowledge for teachers. Future research should begin with further developments and associated evaluations of these programs. The first starting points for the use of digital data acquisition systems are the TRC-DDAS framework alongside the abbreviated self-efficacy scale for evaluation.
Data availability statement
The raw data supporting the conclusions of this article will be made available by the authors, without undue reservation.
Ethics statement
Ethical review and approval was not required for the study on human participants in accordance with the local legislation and institutional requirements. The patients/participants provided their written informed consent to participate in this study.
Author contributions
GB: theoretical grounding, data curation, data analysis, writing, and revising of the draft (lead). TL: idea, supervision, and feedback to the manuscript. All authors contributed to the article and approved the submitted version.
Funding
This project is part of the Qualitätsoffensive Lehrerbildung, a joint initiative of the Federal Government and the Länder which aims to improve the quality of teacher training. The program is funded by the Federal Ministry of Education and Research (Grant No. 01JA2027). The authors are responsible for the content of this publication.
Acknowledgments
We thank Katrin Arbogast and Fabian Kneller for their support of the studies, as well as all participants and experts for their participation.
Conflict of interest
The authors declare that the research was conducted in the absence of any commercial or financial relationships that could be construed as a potential conflict of interest.
Publisher's note
All claims expressed in this article are solely those of the authors and do not necessarily represent those of their affiliated organizations, or those of the publisher, the editors and the reviewers. Any product that may be evaluated in this article, or claim that may be made by its manufacturer, is not guaranteed or endorsed by the publisher.
Supplementary material
The Supplementary Material for this article can be found online at: https://www.frontiersin.org/articles/10.3389/feduc.2023.1180973/full#supplementary-material
Footnotes
1. ^S = r/(2·σ) with discriminatory power r and standard deviation σ (Lienert and Raatz, 1998, p. 118).
References
Ackerman, P. L., Beier, M. E., and Bowen, K. R. (2002). What we really know about our abilities and our knowledge. Person. Indiv. Differ. 33, 587–605. doi: 10.1016/S0191-8869(01)00174-X
Akyuz, D. (2018). Measuring technological pedagogical content knowledge (TPACK) through performance assessment. Comput. Educ. 125, 212–225. doi: 10.1016/j.compedu.2018.06.012
Archambault, L. M., and Barnett, J. H. (2010). Revisiting technological pedagogical content knowledge: Exploring the TPACK framework. Comput. Educ. 55, 1656–1662. doi: 10.1016/j.compedu.2010.07.009
Baer, M., Dörr, G., Fraefel, U., Kocher, M., Küter, O., Larcher, S., et al. (2007). Werden angehende Lehrpersonen durch das Studium kompetenter? Kompetenzaufbau und Standarderreichung in der berufswissenschaftlichen Ausbildung an drei Pädagogischen Hochschulen in der Schweiz und in Deutschland. Unterrichtswissenschaft 35, 15–47. doi: 10.25656/01:5485
Banda, H. J., and Nzabahimana, J. (2021). Effect of integrating physics education technology simulations on students' conceptual understanding in physics: A review of literature. Phys. Rev. Phys. Educ. Res. 17, 023108. doi: 10.1103/PhysRevPhysEducRes.17.023108
Bandura, A. (2006). Guide for constructing self-efficacy scales. Self-Effic. Beliefs Adolesc. 5, 307–337.
Becker, S., Bruckermann, T., Finger, A., Huwer, J., Kremser, E., Meier, M., et al. (2020). “Orientierungsrahmen Digitale Kompetenzen Lehramtsstudierender der Naturwissenschaften DiKoLAN,”? in Digitale Basiskompetenzen: Orientierungshilfe und Praxisbeispiele für die universitäre Lehramtsausbildung in den Naturwissenschaften, eds. S., Becker, J., Meßinger-Koppelt, and C., Thyssen (Hamburg: Joachim Herz Stiftung) 14–43.
Bentler, P. M. (1990). Comparative fit indices in structural models. Psychol. Bull. 107, 238–246. doi: 10.1037/0033-2909.107.2.238
Benz, G., Buhlinger, C., and Ludwig, T. (2022). 'Big data' in physics education: discovering the stick-slip effect through a high sample rate. Phys. Educ. 57, 045004. doi: 10.1088/1361-6552/ac59cb
Brinkley-Etzkorn, K. E. (2018). Learning to teach online: Measuring the influence of faculty development training on teaching effectiveness through a TPACK lens. Internet High. Educ. 38, 28–35. doi: 10.1016/j.iheduc.2018.04.004
Chai, C. S., Koh, J. H. L., and Tsai, C.-C. (2016). “A review of the quantitative measures of technological pedagogical content knowledge (TPACK),”? in Handbook of Technological Pedagogical Content Knowledge (TPACK) for Educators, eds. M. C., Herring, M. J., Koehler, and P., Mishra (New York: Routledge) 87–106.
Cohen, J. (1988). Statistical Power Analysis for the Behavioral Sciences. 2 edition New York: Lawrence Erlbaum Associates.
Cox, S., and Graham, C. R. (2009). Diagramming TPACK in practice: using an elaborated model of the TPACK framework to analyze and depict teacher knowledge. TechTrends 53, 60–69. doi: 10.1007/s11528-009-0327-1
Darrah, M., Humbert, R., Finstein, J., Simon, M., and Hopkins, J. (2014). Are virtual labs as effective as hands-on labs for undergraduate physics? A comparative study at two major universities. J. Sci. Educ. Technol. 23, 803–314. doi: 10.1007/s10956-014-9513-9
Deng, F., Chai, C. S., So, H.-J., Qian, Y., and Chen, L. (2017). Examining the validity of the technological pedagogical content knowledge (TPACK) framework for preservice chemistry teachers. Australasian J. Educ. Technol. 33, 1–14. doi: 10.14742/ajet.3508
Döring, N., and Bortz, J. (2016). Forschungsmethoden und Evaluation in den Sozial- und Humanwissenschaften. 5 edition Berlin, Heidelberg: Springer. doi: 10.1007/978-3-642-41089-5
Eccles, D. W. (2012). “Verbal reports of cognitive processes,”? in Measurement in Sport and Exercise Psychology, eds. G., Tenenbaum, R. C., Eklund, and A., Kamata (Champagne, IL: Human Kinetics) 103–118. doi: 10.5040/9781492596332.ch-011
Enders, C. K., and Bandalos, D. L. (2001). The relative performance of full information maximum likelihood estimation for missing data in structural equation models. Struct. Equat. Model. 8, 430–457. doi: 10.1207/S15328007SEM0803_5
Ericsson, K. A., and Simon, H. A. (1980). Verbal reports as data. Psychol. Rev. 87, 215–251. doi: 10.1037/0033-295X.87.3.215
Falloon, G. (2020). From digital literacy to digital competence: the teacher digital competency (TDC) framework. Educ. Technol. Res. Dev. 68, 2449–2472. doi: 10.1007/s11423-020-09767-4
Gehrmann, A. (2007). “Kompetenzentwicklung im Lehramtsstudium,”? in Forschung zur Lehrerbildung. Kompetenzentwicklung und Programmevaluation, eds. M., Lüders, and J., Wissinger (Münster, New York, München, Berlin: Waxmann) 85–102.
Henson, R. K., and Roberts, J. K. (2006). Use of exploratory factor analysis in published research common errors and some comment on improved practice. Educ. Psychol. Measur. 66, 393–416. doi: 10.1177/0013164405282485
Hew, K. F., Lan, M., Tang, Y., Jia, C., and Lo, C. K. (2019). Where is the “theory" within the field of educational technology research? Br. J. Educ. Technol. 50, 956–971. doi: 10.1111/bjet.12770
Hoyer, C., and Girwidz, R. (2020). Animation and interactivity in computer-based physics experiments to support the documentation of measured vector quantities in diagrams: An eye tracking study. Phys. Rev. Phys. Educ. Res. 16, 020124. doi: 10.1103/PhysRevPhysEducRes.16.020124
Huwer, J., Irion, T., Kuntze, S., Steffen, S., and Thyssen, C. (2019). Von TPaCK zu DPaCK- Digitalisierung im Unterricht erfordert mehr als technisches Wissen. MNU J. 72, 356–364.
Ifenthaler, D., and Schweinbenz, V. (2013). The acceptance of Tablet-PCs in classroom instructions: The teachers' perspectives. Comput. Hum. Behav. 29, 525–534. doi: 10.1016/j.chb.2012.11.004
Jam, N. A. M., and Puteh, S. (2020). “Developing a conceptual framework of teaching towards education 4.0 in TVET institutions,” in International Conference on Business Studies and Education (ICBE) 74–86.
Jang, S.-J., and Chen, K.-C. (2010). From PCK to TPACK: developing a transformative model for pre-service science teachers. J. Sci. Educ. Technol. 19, 553–564. doi: 10.1007/s10956-010-9222-y
Jang, S.-J., and Tsai, M.-F. (2012). Exploring the TPACK of Taiwanese elementary mathematics and science teachers with respect to use of interactive whiteboards. Comput. Educ. 59, 327–338. doi: 10.1016/j.compedu.2012.02.003
Janssen, J., Stoyanov, S., Ferrari, A., Punie, Y., Pannekeet, K., and Sloep, P. (2013). Experts' views on digital competence: Commonalities and differences. Comput. Educ. 68, 473–481. doi: 10.1016/j.compedu.2013.06.008
Koehler, M. J., Mishra, P., Bouck, E. C., DeSchryver, M., Kereluik, K., Shin, T. S., et al. (2011). Deep-play: Developing TPACK for 21st century teachers. Int. J. Learn. Technol. 6, 146–163. doi: 10.1504/IJLT.2011.042646
Kopcha, T. J., Ottenbreit-Leftwich, A., Jung, J., and Baser, D. (2014). Examining the TPACK framework through the convergent and discriminant validity of two measures. Comput. Educ. 78, 87–96. doi: 10.1016/j.compedu.2014.05.003
Krauss, S., Brunner, M., Kunter, M., Baumert, J., Blum, W., Neubrand, M., et al. (2008). Pedagogical content knowledge and content knowledge of secondary mathematics teachers. J. Educ. Psychol. 100, 716–725. doi: 10.1037/0022-0663.100.3.716
Kuckartz, U. (2018). Qualitative Inhaltsanalyse. Methoden, Praxis, Computerunterstützung. 4 edition Weinheim, Basel: Beltz Juventa.
Lachner, A., Backfisch, I., and Stürmer, K. (2019). A test-based approach of modeling and measuring technological pedagogical knowledge. Comput. Educ. 142, 103645. doi: 10.1016/j.compedu.2019.103645
Lei, P.-W., and Wu, Q. (2012). “Estimation in structural equation modeling,”? in Handbook of structural equation modeling, eds. R. H., Hoyle (New York: The Guilford Press) 164–180.
Lienert, G. A., and Raatz (1998). Testaufbau und Testanalyse. Weinheim: Belt, Psychologie Verl.-Union.
Mahler, D., and Arnold, J. (2022). MaSter-Bio - Messinstrument für das akademische Selbstkonzept zum technologiebezogenen Professionswissen von angehenden Biologielehrpersonen. Zeitschrift für Didaktik der Naturwissenschaften 28, 3. doi: 10.1007/s40573-022-00137-6
Messick, S. (1995). Validity of psychological assessment. Validation of inferences from persons' responses and performances as scientific inquiry into score meaning. Am. Psychol. 50, 741–749. doi: 10.1037/0003-066X.50.9.741
Mishra, P., and Koehler, M. J. (2006). Technological pedagogical content knowledge: A framework for teacher knowledge. Teach. College Rec. 108, 1017–1054. doi: 10.1111/j.1467-9620.2006.00684.x
Moosbrugger, H., and Kelava, A. (2020). Testtheorie und Fragebogenkonstruktion. 3 edition Berlin, Heidelberg: Springer. doi: 10.1007/978-3-662-61532-4
Pumptow, M., and Brahm, T. (2021). Students' digital media self-efficacy and its importance for higher education institutions: development and validation of a survey instrument. Technol. Knowl. Learn. 26, 555–575. doi: 10.1007/s10758-020-09463-5
Rauin, U., and Meier, U. (2007). “Subjektive Einschätzungen des Kompetenzerwerbs in der Lehramtsausbildung,”? in Forschung zur Lehrerbildung. Kompetenzentwicklung und Programmevaluation, eds. M., Lüders, and J., Wissinger (New York, München, Berlin: Waxmann, Münster) 103–131.
Revelle, W. (2016). psych: Procedures for Psychological, Psychometric, and Personality Research. Software.
Rhemtulla, M., Brosseau-Liard, P. E., and Savalei, V. (2012). When can categorical variables be treated as continuous? A comparison of robust continuous and categorical SEM estimation methods under suboptimal conditions. Psychol. Methods 17, 354–373. doi: 10.1037/a0029315
Rosseel, Y. (2012). lavaan: an R package for structural equation modeling. J. Stat. Softw. 48, 1–36. doi: 10.18637/jss.v048.i02
Scherer, R., Siddiq, F., and Tondeur, J. (2019). The technology acceptance model (TAM): A meta-analytic structural equation modeling approach to explaining teachers' adoption of digital technology in education. Comput. Educ. 128, 13–35. doi: 10.1016/j.compedu.2018.09.009
Scherer, R., Tondeur, J., and Siddiq, F. (2017). On the quest for validity: Testing the factor structure and measurement invariance of the technology-dimensions in the Technological, Pedagogical, and Content Knowledge (TPACK) model. Comput. Educ. 112, 1–17. doi: 10.1016/j.compedu.2017.04.012
Scherer, R., Tondeur, J., Siddiq, F., and Baran, E. (2018). The importance of attitudes toward technology for pre-service teachers' technological, pedagogical, and content knowledge: Comparing structural equation modeling approaches. Comput. Hum. Behav. 80, 67–80. doi: 10.1016/j.chb.2017.11.003
Schmid, M., Brianza, E., and Petko, D. (2020). Developing a short assessment instrument for Technological Pedagogical Content Knowledge (TPACK.xs) and comparing the factor structure of an integrative and a transformative model. Comput. Educ. 157, 103967. doi: 10.1016/j.compedu.2020.103967
Schmidt, D. A., Baran, E., Thompson, A. D., Mishra, P., Koehler, M. J., and Shin, T. S. (2009). Technological pedagogical content knowledge (TPACK). The development and validation of an assessment instrument for preservice teachers. J. Res. Technol. Educ. 42, 123–149. doi: 10.1080/15391523.2009.10782544
Schwarz, C. V., Meyer, J., and Sharma, A. (2007). Technology, pedagogy, and epistemology: opportunities and challenges of using computer modeling and simulation tools in elementary science methods. J. Sci. Teach. Educ. 18, 243–269. doi: 10.1007/s10972-007-9039-6
Schwarzer, R., and Jerusalem, M. (2002). “Das Konzept der Selbstwirksamkeit,”? in Selbstwirksamkeit und Motivationsprozesse in Bildungsinstitutionen (Beltz, Weinheim) 28–53.
Shulman, L. S. (1986). Those who understand: Knowledge growth in teaching. Educ. Resear. 15, 4–14. doi: 10.3102/0013189X015002004
Thyssen, C., Thoms, L.-J., Kremser, E., Finger, A., Huwer, J., and Becker, S. (2020). “Digitale Basiskompetenzen in der Lehrerbildung unter besonderer Berücksichtigung der Naturwissenschaften,”? in Digitale Innovationen und Kompetenzen in der Lehramtsausbildung, eds. M., Beiäwenger, B., Bulizek, I., Gryl, and F., Schat (Duisburg: Universitätsverlag Rhein-Ruhr) 77–98.
Tschannen-Moran, M., and Hoy, A. W. (2001). Teacher efficacy: capturing an elusive construct. Teach. Teach. Educ. 17, 783–805. doi: 10.1016/S0742-051X(01)00036-1
von Kotzebue, L. (2022). Two is better than one - Examining biology-specific TPACK and its T-dimensions from two angles. J. Res. Technol. Educ. 2022, 1–18. doi: 10.1080/15391523.2022.2030268
von Kotzebue, L., Meier, M., Finger, A., Kremser, E., Huwer, J., Thoms, L.-J., et al. (2021). The Framework DiKoLAN (Digital competencies for teaching in science education) as Basis for the Self-Assessment Tool DiKoLAN-Grid. Educ. Sci. 11, 775. doi: 10.3390/educsci11120775
Voss, T., Kunter, M., and Baumert, J. (2011). Assessing teacher condidates' general pedagogical/psychological knowledge: test construction and validation. J. Educ. Psychol. 103, 952–969. doi: 10.1037/a0025125
Wieman, C. E., Adams, W. K., Loeblein, P., and Perkins, K. K. (2010). Teaching physics using PhET simulations. Phys. Teach. 38, 225–227. doi: 10.1119/1.3361987
Keywords: technological knowledge, TPACK, DiKoLAN, self-efficacy, digital data acquisition system, experimentation, physics education
Citation: Benz G and Ludwig T (2023) Going beyond general competencies in teachers' technological knowledge: describing and assessing pre-service physics teachers' competencies regarding the use of digital data acquisition systems and their relation to general technological knowledge. Front. Educ. 8:1180973. doi: 10.3389/feduc.2023.1180973
Received: 06 March 2023; Accepted: 13 June 2023;
Published: 28 June 2023.
Edited by:
Sebastian Becker, University of Cologne, GermanyReviewed by:
André Bresges, University of Cologne, GermanyPascal Klein, University of Göttingen, Germany
Copyright © 2023 Benz and Ludwig. This is an open-access article distributed under the terms of the Creative Commons Attribution License (CC BY). The use, distribution or reproduction in other forums is permitted, provided the original author(s) and the copyright owner(s) are credited and that the original publication in this journal is cited, in accordance with accepted academic practice. No use, distribution or reproduction is permitted which does not comply with these terms.
*Correspondence: Gregor Benz, Z3JlZ29yLmJlbnpAcGgta2FybHNydWhlLmRl