- 1Department of Physics Education, Goethe University, Frankfurt, Germany
- 2Department of Science, University of Education, Schwäbisch Gmünd, Germany
- 3Department of Educational Psychology, Goethe University, Frankfurt, Germany
Nowadays, teachers are facing a more and more digitized world, as digital tools are being used by their students on a daily basis. This requires digital competencies in order to react in a professional manner to individual and societal challenges and to teach the students a purposeful use of those tools. Regarding the subject (e.g., STEM), this purpose includes specific content aspects, like data processing, or modeling and simulations of complex scientific phenomena. Yet, both pre-service and experienced teachers often consider their digital teaching competencies insufficient and wish for guidance in this field. Especially regarding immersive tools like augmented reality (AR), they do not have a lot of experience, although their willingness to use those modern tools in their lessons is high. The digital tool AR can target another problem in science lessons: students and teachers often have difficulties with understanding and creating scientific models. However, these are a main part of the scientific way of acquiring knowledge and are therefore embedded in curricula. With AR, virtual visualizations of model aspects can be superimposed on real experimental backgrounds in real time. It can help link models and experiments, which usually are not part of the same lesson and are perceived differently by students. Within the project diMEx (digital competencies in modeling and experimenting), a continuing professional development (CPD) for physics teachers was planned and conducted. Secondary school physics educators were guided in using AR in their lessons and their digital and modeling competencies for a purposeful use of AR experiments were promoted. To measure those competencies, various instruments with mixed methods were developed and evaluated. Among others, the teachers’ digital competencies have been assessed by four experts with an evaluation matrix based on the TPACK model. Technological, technical and design aspects as well as the didactical use of an AR experiment were assessed. The teachers generally demonstrate a high level of competency, especially in the first-mentioned aspects, and have successfully implemented their learnings from the CPD in the (re)design of their AR experiments.
1. Introduction
To succeed in an increasingly digitized world, competencies regarding digital tools are more and more necessary. Regarding students’ everyday life and media use, those competencies need to be taught in school, which requires teachers to improve their own information and communication technology (ICT) skills (Tondeur et al., 2017). This way, teachers can build a bridge between students’ digital literacy and the subject-specific content aspects. Following Shulman’s (1986) model of pedagogical content knowledge (PCK), Mishra and Koehler (2006) developed the TPACK framework. It additionally includes educational technologies that teachers need to handle in their lessons, while emphasizing their interactions. Since the development of TPACK, digital competencies not only require using technology but further address individual and societal challenges, so they go beyond “traditional” technical skills as included in the framework. Taking this into account, the DPaCK model (Huwer et al., 2019) features aspects of digitality in an extended form.
One educational technology that has lately been gaining relevance in many areas is augmented reality (AR). It enables users to add virtual components to a real-world environment by superimposing visual information directly on a real-time camera view (Carmigniani and Furht, 2011), via a smartphone or tablet. As defined by Milgram et al. (1995), AR is set in the reality-virtuality continuum, and—instead of immersing the user in a completely virtual environment like virtual reality (VR)—augments certain aspects of the real world. The use of AR is also growing in the educational context (Altinpulluk, 2019). With this tool, abstract concepts like scientific models can be linked to a real-life context, making it easier for students to understand them (Bloxham, 2014). Many studies of different STEM subjects have assessed the use and effects of AR in science teaching and learning. In chemistry lessons, AR can be used to show invisible or submicroscopic structures, or to provide the learner with visual support (Huwer et al., 2018). Furthermore, the impact of AR on understanding submicroscopic particles through spatial continuity is investigated (Peeters et al., 2023). In biology, among others, the effect of AR on learning attitudes was analyzed (Weng et al., 2020). In mathematics education, AR can be used in the field of geometry (Koparan et al., 2023). Lastly, in physics lessons, studies measured the impact of AR on cognitive load (Altmeyer et al., 2020) and flow student experience (Ibáñez et al., 2014). One promising example of AR in the classroom can be created using GeoGebra,1 a dynamic mathematics software. It can be used to examine relationships and explore scientific laws in an interactive 3D model. The advantage here is that changes to one element of the model directly affect all objects related to it. To experiment with AR, the virtual dynamic 3D model, initially constructed with GeoGebra, is superimposed on a real experiment using the mobile app GeoGebra 3D Calculator. It allows to place the 3D model on any surface and to adjust it to real experiments (see Figure 1). This way, usually unobservable objects with an explanatory power enrich the traditional classroom experiments by linking and comparing models and experiments in real time (Teichrew and Erb, 2020).
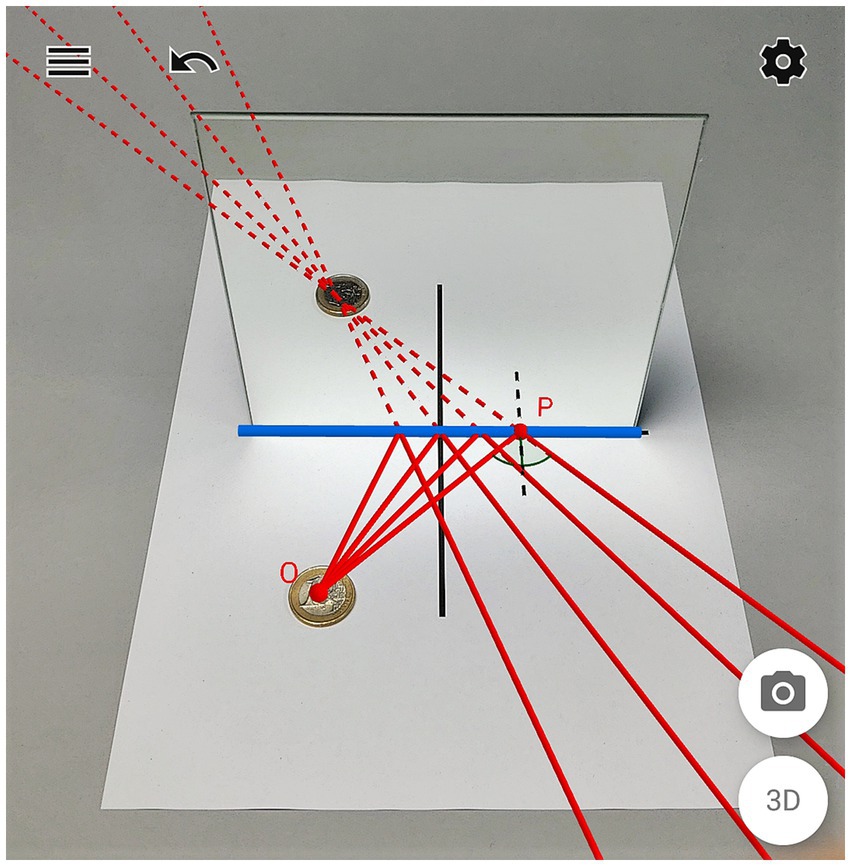
Figure 1. Example of an augmented reality (AR) experiment: image formation behind a real-life plane mirror with modeled rays of light.
Implementing and creating 3D models in the context of AR experiments can be placed within existing competency frameworks for STEM teacher education, such as DiKoLAN (Thoms et al., 2022). It provides a structured orientation about digital competencies for pre-service science teachers. Among other aspects, it includes the use of simulations and models in science lessons. It can be used as a base for university curricula to include digital competencies in teacher training, more precisely the purposeful use of AR experiments. To achieve this, a science teacher enriches the real experiment by overlaying it with a (self-created) virtual, visually appealing 3D model in AR so that the underlying phenomenon can be better understood by the students. The 3D model should be operated intuitively, and an adequate learning objective that emphasizes the AR experiment’s complexity and added value should be provided. Furthermore, the appropriate implementation of AR in the classroom has potential positive effects on students’ learning. Several studies have shown that AR experiments can successfully reduce learning-irrelevant cognitive load and promote concept learning (Altmeyer et al., 2020; Thees et al., 2020). These benefits, in addition to the rise of AR as a digital teaching tool, establish AR-related skills of teachers as relevant future-related competencies.
In STEM education, subject-specific aspects of PCK include the use of models to understand scientific phenomena. Creating and working with models is a main part of acquiring scientific knowledge and is therefore embedded in curricula (e.g., NRC, 2012). This requires model competencies, hence the teachers’ ability to work with models and to explain them to students (Upmeier Zu Belzen et al., 2019), or to discuss the (re-)construction of models and the underlying idealizations (Winkelmann, 2023). Nevertheless, international studies have shown a need for improvement in teachers’ and students’ model competencies (e.g., Louca and Zacharia, 2012; Gilbert and Justi, 2016). In STEM education, the distinction between the constructed model world and the experimental reality, which exist side by side, must be taken into account. However, students have difficulties deliberately separating them (Thiele et al., 2005). Furthermore, many students find models abstract and difficult, in contrast to experimenting (Winkelmann et al., 2022). Despite the overlap of model and experiment as presented above, this new concept of AR experiments does not mix the model and experiential worlds. Instead, scientific modeling takes place while the real experiment can be manipulated at the same time. The superimposed 3D objects, which are clearly recognizable as virtual, make their model character clear (e.g., the light rays in Figure 1). Therefore, in addition to the digital competencies, training in modeling for teachers is needed, so that models can be more widely introduced in class.
The digital competencies (based on TPACK) necessary for the meaningful use of AR experiments include the operation of the GeoGebra software, the handling of hardware (smartphones or tablets), and the didactic implementation of those digital tools in the classroom. On the other hand, STEM lessons require subject-related model competencies, which include modeling scientific phenomena, as well as understanding models as a tool of scientific knowledge acquisition. Both areas overlap in AR experiments since digital competencies are essential to acquire model competencies. The following research questions are therefore addressed in the presented project:
1. Which digital competencies are necessary to use AR purposefully in the classroom, and how can they be measured?
2. Does a CPD about using AR experiments in physics lessons improve the teachers’ modelling competence?
With digital skills as future-related competencies being the focus of this Research Topic, research question 2 is not addressed in detail in this paper. However, as imparting digital competencies without the inclusion of substantive subject matter knowledge appears to be useless, model competency was introduced here theoretically and is examined in more detail in the presented project.
2. Materials and methods
The aim of the study presented in this paper was to measure teachers’ digital and model competencies necessary for a purposeful use of AR experiments in physics lessons. For this purpose, a mixed methods approach was adopted, and various measurement instruments were designed, tested and evaluated (see Figure 2). They were applied within a continued professional development (CPD) for secondary physics teachers (N = 20) in Hesse, Germany. Over the course of 6 months in five successive sessions (see Figure 3), the participants learned to create virtual 3D models with GeoGebra by reflecting their construction process and underlying idealizations, and to implement them as AR experiments in their own lessons. Their progress was accompanied by a survey and their AR experiments were documented to measure their competencies regarding dynamic 3D models. The CPD began with the basic principles of models as a part of acquiring scientific knowledge (input phase). In three consecutive development sessions, the participating teachers learned to use GeoGebra to create (or modify existing) 3D models, accompanied by an online self-study module2 (workshop phase). They implemented the finished AR experiment in their own lessons, using the experiences and their students’ feedback to revise the models if necessary and to optimize their accompanying materials (practice phase). Lastly, the teachers reflected on the implementation with the group in a final session and peer-evaluated each other’s AR experiments.
Regarding the understanding of models, a questionnaire contained qualitative open questions about the teachers’ understanding of models and closed-ended vignettes on the didactical handling of models in science lessons (Billion-Kramer et al., 2020). The teachers’ open answers were rated by four experts according to a coding manual (see Supplementary Table S1, based on Windschitl and Thompson, 2006). Furthermore, the teachers could participate voluntarily in semi-structured interviews during the practice phase of the CPD to give individual feedback on their modeling process and their experience with using the AR experiments in their lessons. Six to nine months after completion of the CPD, the teachers were interviewed again as part of a follow-up study. At this time, developments regarding the explicit use of models and AR experiments in the classroom were investigated in order to verify the sustainability of the CPD.
In order to assess the group of participating teachers in terms of their prior experience with AR and GeoGebra, as well as their attitudes towards digital tools like AR in the classroom, those were examined in the pretest. For their GeoGebra experiences, open and closed questions, and Likert scales were used (see Table 1). For their attitudes and willingness to use digital tools in class, 14 items with four-stage Likert scales were used (adapted from Vogelsang et al., 2018), and the teachers’ statements were obtained with semi-structured interviews. Those constructs were measured because they can possibly be linked to the teachers’ digital modeling competencies and their success in creating AR experiments for their lessons.
During the workshop phase, the teachers were instructed to prepare AR experiments for their own lessons. Regarding topics, they were free to choose whichever they could fit in their lesson planning and which classes they like to implement the AR experiment in. For this purpose, they received detailed step-for-step instructions embedded in three interactive learning units which intended to convey them the skills and techniques to create 3D models from scratch in GeoGebra. However, it was not assumed that all participants implement a fully self-made AR experiment in their lessons. They were given a broad selection of prepared 3D models to provide them with ideas and the possibility to use (and, if necessary, modify) the existing AR experiments. The teachers could decide based on their individually available time and skills whether to use those or to create one from scratch. In order to document the context and the experiences with the AR experiments in a structured way directly after their use in the lessons, the teachers were given an implementation sheet. This was also intended to serve as an aid to memory in the reflection session.
To measure the teachers’ digital competencies regarding modeling and using dynamic 3D models for AR experiments, a rating system was created and applied by four AR experts after the reflection session (see Table 2). The matrix was based on the TPACK model and contains five different aspects linked to the modeling process, each with five levels of digital competencies needed for modeling dynamic 3D models. Levels 1, 3, and 5 of each aspect were fully verbalized for this purpose, while 2 and 4 each represented interim levels in case the raters considered a competency level not fully met but still higher than the previous one. The rating instrument was developed based on the questions regarding the teachers’ GeoGebra experiences assessed in the pretest of the study. The matrix is a transfer of TPACK’s general categories of technology-related knowledge of teachers to competencies related to AR experiments. TK refers to the technology knowledge which enables teachers to operate software and hardware. In terms of AR experiments as presented above, this refers specifically to the teacher’s ability to construct a 3D model in GeoGebra, or to select and/or adapt an existing model. The technological content knowledge (TCK) builds a bridge between the subject-specific content and the technologies that can represent it. Here, this means that the scientific content visualized in the 3D model is accurate. The technological pedagogical knowledge (TPK) refers to a teacher’s ability to choose the right (educational) technology for their lesson. In this category, two focal points of the CPD were identified to be included, so that it is represented twice with a user-friendly design and the formulation of an appropriate learning objective. Lastly, the technological pedagogical content knowledge (TPACK) links all prior types of knowledge with the idea of a subject-specific technology that reaches an educational goal. In the context of AR experiments, this competency refers to enhancing a real-life experiment by virtual components for a better understanding of both the model and the experiential phenomenon.
Although the advanced DPaCK model aims at new challenges in society while targeting 21st century skills (Griffin et al., 2011), the rating system developed in this study was based on TPACK. The CPD in the project trains teachers in a very technological way, which is complemented with the didactical question of a meaningful use of AR experiments in STEM lessons. Digitality, which is the key issue in the DPaCK model and defines modern society, has not yet arrived in the reality of many teachers and schools. As a previous assessment of needs revealed (Freese et al., 2021), there is still need for improvement in digital equipment at a majority of German schools. Based on this reality, the study participants were still more likely to be in a state of digitalization as considered in TPACK. Digitality, for which competencies are required according to DPaCK, is in some cases still a future state that has not yet been achieved. During the CPD, the teachers’ existing knowledge and skills were taken into account by utilizing the widely referenced TPACK framework. Nevertheless, the competency requirements in the presented rating system go further at certain points, such as the concrete didactical implementation of AR experiments in the classroom.
In a reduced form, the rating system was also given to the teachers (n = 8) during the reflection session to give peer feedback to their colleagues. For an effective CPD, providing helpful feedback is essential as it helps teachers track their improvement in competence (Lipowsky and Rzejak, 2015). The mutual evaluation provided information on their own learning progress and their understanding of AR experiments, as well. As the teachers used the same evaluation matrix for the feedback to their colleagues, the insights could be applied for a validity check. The peer feedback matrix did not contain the TK aspect, as it was purely objective based on the construction process. The focus of the matrix was on the more subjective aspects of the competencies, such as the teachers’ ability to plan and implement effective AR experiments. The teachers were provided with a description of the highest level of each competency aspect to guide their evaluation and ensure consistency in the feedback process.
3. Results
3.1. Measurement of digital competencies: the evaluation matrix
To rate the teachers’ digital competency surrounding AR experiments, a matrix was developed to assess the creation and use of AR experiments. It was used by four experts who also authored this paper (Table 3) and by the teachers in the reflection session to provide peer feedback (Table 4). The mean ratings of the five competency aspects with standard deviations for each of the demonstrated AR experiments are presented. The first two AR experiments could not be tested in class until the reflection session and therefore the TPK of those two teachers was not evaluated (n. e.). The objective TK aspect was not individually rated by all four experts, which is why no standard deviation is given there. As it was not rated by the peer teachers, either, the aspect is omitted in Table 4 to avoid redundancy.
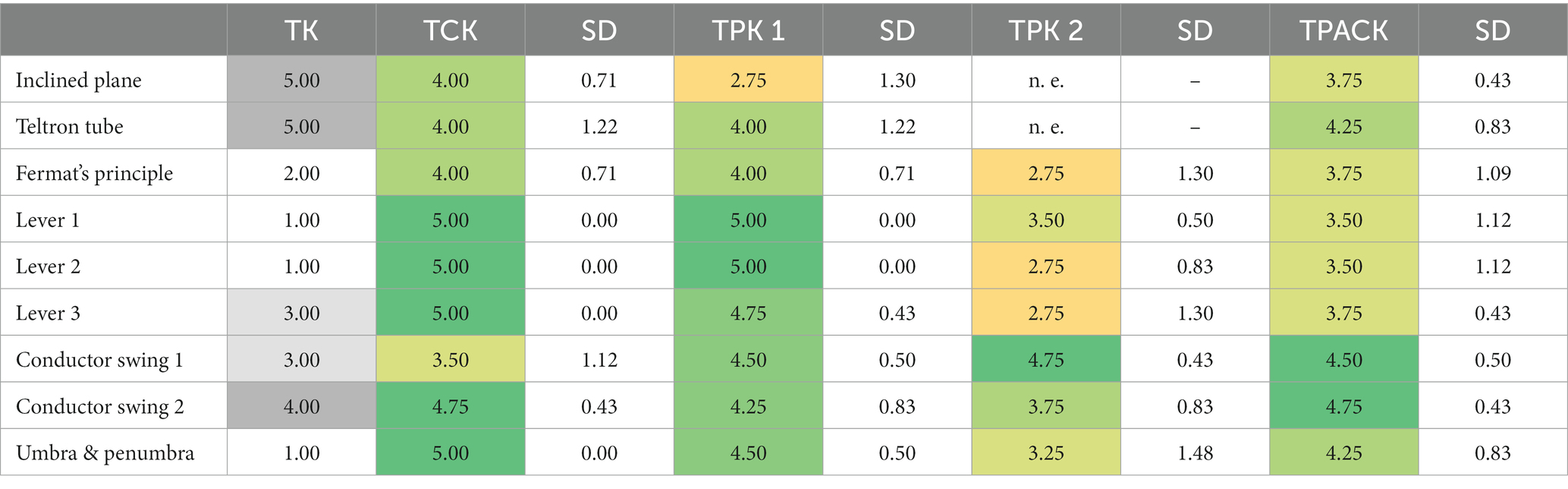
Table 3. Expert-rated competency aspects of the teachers’ AR experiments (mean and standard deviation).
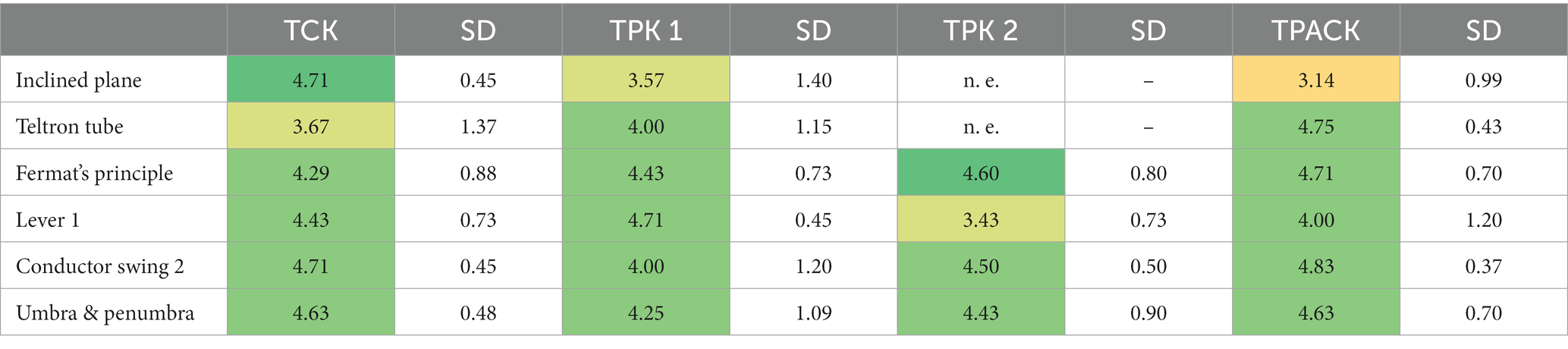
Table 4. Peer-rated competency aspects of the teachers’ AR experiments (mean and standard deviation).
Even at first glance, a high proportion of green cells can be seen, which stand for the highest competency rating (level 4 or 5). Especially regarding TCK and TPK 1, i.e., the content-related and didactical design of the models, the results are predominantly very good. Even though only three teachers developed their models from scratch, the other teachers were credited for successfully finding a suitable existing 3D model for their lessons. Nevertheless, it should be noted that the TPK 2 aspect (didactical use) reaches the lowest score in the expert rating (3.36 points on average). In contrast, the standard deviations here and for the TPACK aspect are particularly high. This is also reflected in the inter-rater correlation. Spearman’s ρ shows no to small correlation in these two aspects (Cohen, 1977), whereas for TCK and TPK 1, the correlation is moderate and partially significant (see Table 5). These results are discussed in the following section. The assessment of the AR experiments by the didactic experts shows agreement with the peer feedback that the teachers gave each other (see Table 4). The overall average score in the peer rating (4.29 points) is higher than in the expert rating (4.03 points). The rankings of the AR experiments are similar: In both ratings, the Teltron tube and the conductor swing 2 (both created from scratch) are rated the highest overall, each obtaining more than 86% of the maximum achievable score. The lowest peer rating scores are found in the TPK 1 and TPK 2 aspects, which in several cases was not even rated at all by the teachers. Possible reasons for this circumstance are also discussed below. Not all eight teachers always fully assessed each AR experiment in all competency aspects, which is reflected in the mean scores.
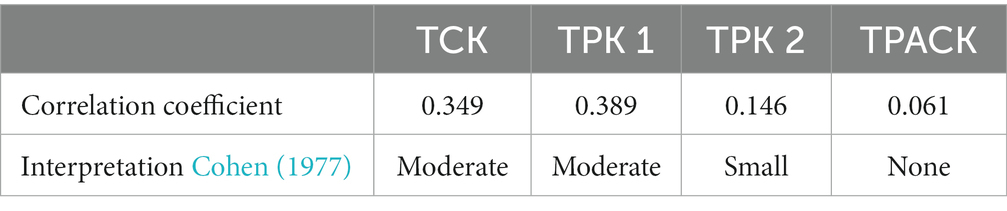
Table 5. Inter-rater correlation (Spearman’s ρ) in the expert rating, means for each individually rated aspect.
3.2. Measurement of digital competencies: examples of teachers’ AR experiments
During the three workshop sessions, the teachers were trained in creating 3D models in GeoGebra. These were used in their own lessons during the three-month practical phase, and the implementation was reflected on together in the final session. As follows, two AR experiments created or modified by participants are presented in order to demonstrate the rating system for the teachers’ digital competencies. These were chosen due to their high ratings by both the experts and the peer teachers. They originate from different physical subject areas and differ in their degree of independence during the modeling process.
Figure 4 shows an AR experiment made from scratch (TK level 5 on a scale from 1 to 5) for the determination of the specific charge of an electron in the Teltron tube (A: full view; B: detailed view), which, however, had not yet been implemented by the participating teacher until the reflection session for practical teaching reasons. The implementation was planned as a demonstration experiment in the upper secondary school. At first glance, the content-related design of the model (TCK) appears to be good to very good (predominantly level 4 or 5 in the expert rating), as the relevant subject content is correctly processed. However, the algebraic commands used for construction show that the model was not modeled physically, but only graphically. This makes it difficult to adapt it to the real experiment, the conditions of which, moreover, often vary. The didactical design is also largely convincing (TPK 1 mostly level 4 or 5). One criticism is that some labels are not clearly visible, but the comprehensibility can be ensured for use as a demonstration. Due to the fact that the AR experiment was not used, the technological-pedagogical knowledge of the teacher (TPK 2) was not assessed in this case. The assessment of the teacher’s digital modeling competency is also predominantly positive (TPACK level 4 or 5). In addition to the modeled objects, which make the invisible visible, this is also supported by the teacher’s own idea of extending the real experiment onto the Teltron tube. As a classical demonstration experiment, this usually comes up against the limit in class that in a bright environment the electron beam is no longer easily visible. Here, the modeled electron path with AR can provide a virtual supplement.
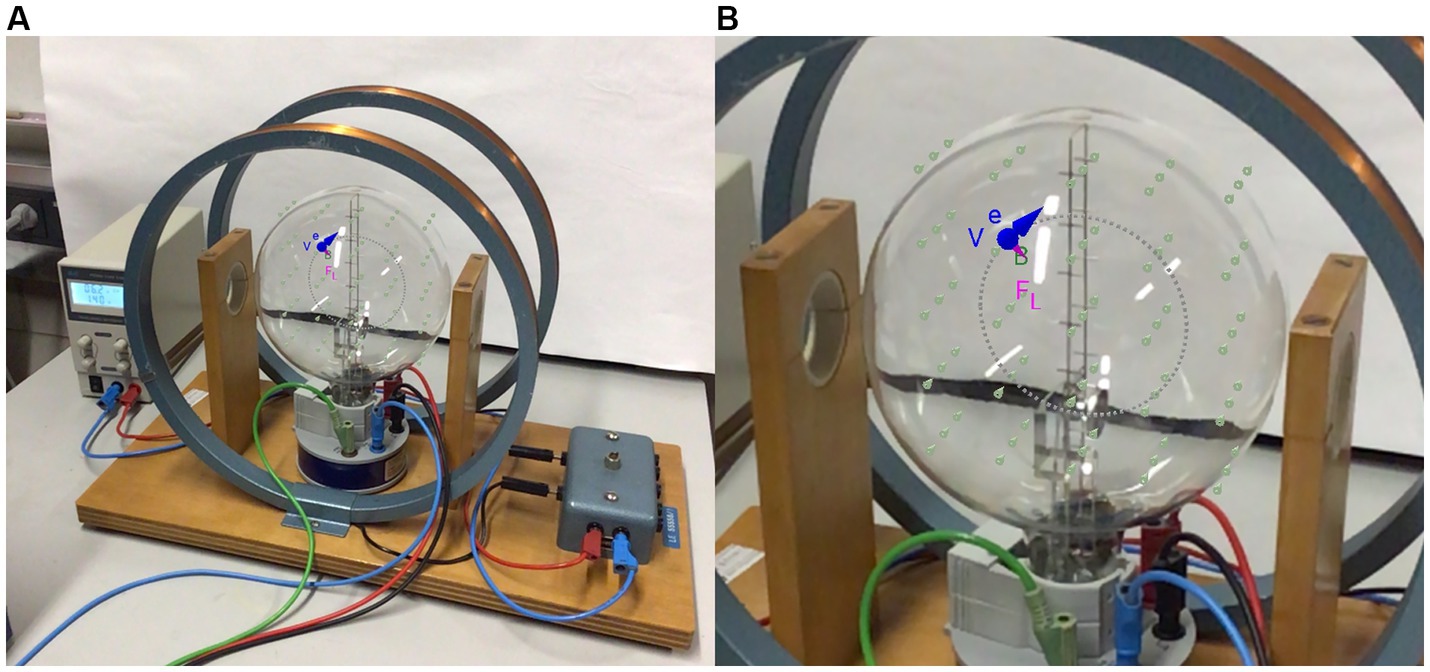
Figure 4. (A) Self-created AR experiment of a participating teacher on the Teltron tube, seen through the camera of a tablet. (B) Detailed view of the AR experiment on the Teltron tube. The electron beam is only visible in a darkened room.
Another teacher decided to adapt an existing model of a lever (see Figure 5) to the conditions in their school (TK level 3) and to use it as group work in 9th grade. The teacher’s TCK is rated as very high (level 5), the content of the model is correct and comprehensible. The didactical design (TPK 1) is also convincing. The didactical use (TPK 2), on the other hand, is predominantly rated in the medium range (level 2 or 3), since the added value of the AR experiment in the classroom seems to have been conveyed only implicitly: The reported learning objectives of “promoting understanding” and “clarity” do not emphasize the special character of an AR experiment. Overall, the teacher’s digital modeling competency (TPACK) is nevertheless predominantly assessed as good to very good (level 4), as the teaching application can basically work in class.
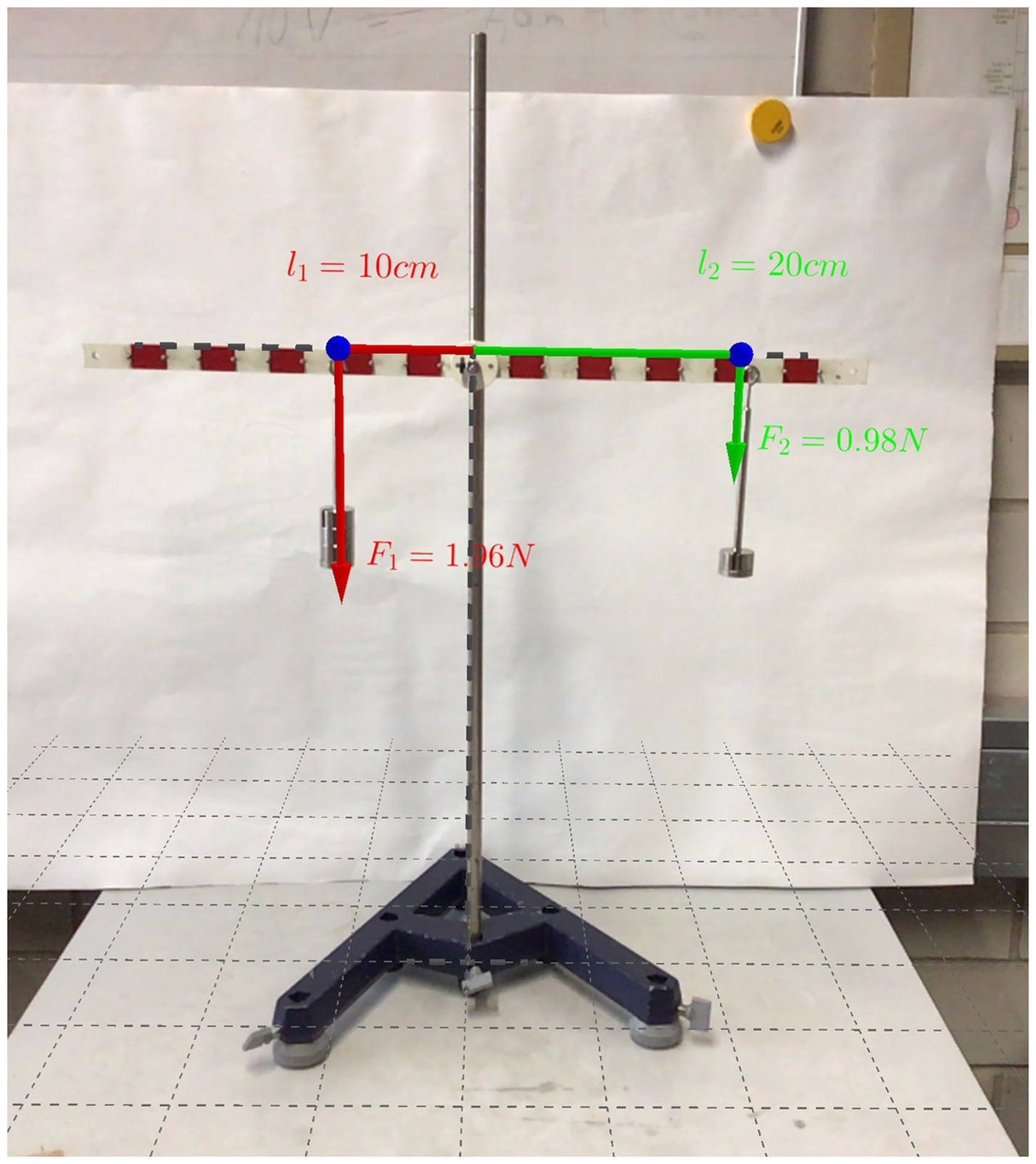
Figure 5. Adapted AR experiment of a participating teacher on the law of levers, seen through the camera of a tablet.
3.3. Measurement of model competencies
Although the focus of this paper lies on STEM teachers’ digital competencies, some findings from the measurement of the model competency of the study participants will be briefly summarized at this point. In order to be able to work successfully with AR experiments in the classroom according to the understanding described above, a certain model competency is also required, since the AR experiments and especially the virtually superimposed 3D models are based on scientific models. Even though a major advantage of AR experiments is the fact that models are displayed in real time on top of the experiment, making it easier for the learners to understand, the teacher must of course have appropriate subject-specific content knowledge about models when creating or selecting them.
The teachers’ model competencies were rated by four experts according to a coding manual. The teachers’ individual answers to the open-ended questions were classified into five different levels. For a good understanding of models, it is primarily relevant whether the teachers perceive models and modeling—in addition to experimentation—as a tool for acquiring scientific knowledge at all. Teachers with a high model competency, for example, should understand models beyond an empiricist and pictorial perspective (Windschitl and Thompson, 2006), but also as processes or systems and see their function in predicting phenomena and as a basis for scientific thinking. Nevertheless, it must be said restrictively at this point that the study does not allow for a meaningful quantitative evaluation due to the small sample of those who answered the vignette test (n = 6) and the open-ended questions about models (n = 7) before and after the CPD. As a rough overview, however, it can at least be stated that the majority of teachers slightly increased their model competency over the course of the CPD, both in the open-ended questions and in the vignette test. On average, their understanding of models slightly increased, and the didactic handling of models in the classroom also approached the expert solution in the vignette test for most teachers. Again, these results are limited due to the small number of participants, so this can only be interpreted as a rough estimate of the CPD’s success. On a larger scale, the quantitative vignette test has the potential to serve as a valid method for measuring STEM teachers’ model competency.
3.4. Teachers’ qualitative statements
In the reflection session, the teachers stated that creating their own AR experiment for the lessons involves a lot of work and that they were therefore relieved about the existing 3D models. They expressed the wish that the materials created (by the participants and the project team) be bundled in a sorted collection3 and made available as open educational resources (OER). According to the teachers’ reports, the feedback from the students was mostly positive, especially in the higher grades. Technical difficulties were mainly complained about due to the equipment at the schools or the students’ outdated mobile devices. Furthermore, it became apparent that the different subject areas of physics are differently suited for 3D modeling in the AR experiments. While the area of optics offers great potential, the added value of 3D-modeled force vectors (e.g., mechanics) in AR, compared to classical drawings or simulations, is not directly apparent to teachers and students. This should therefore be emphasized more clearly to promote model competency in the classroom, and also be discussed with the students. In order not to lose sight of the model aspect of AR experiments by dealing with the real experimental materials, a stronger focus was put on theoretical models by input lectures in the workshop phase.
4. Discussion
The evaluation of the teachers’ digital competencies based on their AR experiments is a pure expert rating, which was only used by the physics didactics members of the project diMEx, who designed and conducted the CPD. Therefore, it should be noted at this point that the matrix requires appropriate training for use by external raters in order to know exactly the criteria for good AR experiments. Although the expert raters were actively involved in creating the evaluation matrix (see above), the first round of ratings resulted in a very low inter-rater correlation. Therefore, a rater training was carried out. It consisted of an in-depth reflection between the experts on the particularly disputable ratings from the first round. Without knowing the specific ratings of the other experts or which expert rating was standing out, the definitions of each competence level in the matrix were discussed again for these aspects and ambiguities were cleared up. The second round of ratings slightly improved the inter-rater reliability, but the agreement is still not very high (especially in the TPK 2 aspect). The assessments of the competency aspects between the experts from physics didactics, who carried out the rating, strongly deviate from each other in isolated cases. As can be seen in Table 5, the inter-rater concordances for TCK and TPK 1 turn out to be moderate. For the aspects TPK 2 and TPACK, on the other hand, which refer to the actual use of AR experiments in the classroom, no or only a weak correlation can be discerned. On the one hand, this can be explained by interdependencies of the aspects since the digital modeling competency (TPACK) and didactical use (TPK 2) often depend on each other. However, even without the actual use of the AR experiment, a digital model competency of the teacher can be determined, since the design and content aspects of the model are also a part of it. Lastly, it should be noted that a majority of the existing models which some teachers selected for their lessons and partially adapted, were created by one of the experts. Therefore, this expert conducted a special examination of the content and design aspects (TCK and TPK 1), which stands out from the other experts in the rating. The rater training on how a good AR experiment can be created with GeoGebra, however, has still been reasonably effective regarding the inter-rater correlation here.
The good mean expert rating for the two competency aspects TCK and TPK 1 is due to the content focus of the CPD. Thanks to the detailed self-study module, the teachers were trained at a fundamental level for the creation of 3D models in GeoGebra, in particular with regard to technically correct interrelationships in the programming and design aspects for a student-friendly, intuitive usage. The overall lower ratings of the teachers’ TPK 2 aspects who used an AR experiment in the classroom requires a critical examination of the content of the CPD. This was strongly focused on the creation of 3D models and the basics of GeoGebra, so that the actual use in class was not clearly addressed. Also, in their feedback, the teachers expressed the wish to accompany them on the way to the implementation more closely. As they stated in the reflection session and in the interviews, their students liked the idea of using AR in class, but sometimes did not really see the purpose or added value of an AR experiment, as opposed to a simulation. This issue was also discussed by the teachers over the course of the CPD. The potential of AR applications to spark students’ interest and motivate them to learn was also mentioned by the participants. They noted that their students enjoyed using digital media to visualize scientific connections. As mobile devices and apps were already part of their everyday lives, it should be easy to make connections in the classroom. A CPD that focuses more strongly on classroom practice with AR should thus address this benefit: The participating teachers should explicitly learn to design a lesson or series of lessons around the AR experiment and accompany it with worksheets on which the learning objective is the common thread. It should also address the specific questions of the teachers concerning AR usage in the classroom, including how to operate the devices and software and how to explain it to students. As noted in many of the research papers on AR in science education presented above, they are often studies in a university setting. Student work with AR in a school context has been less frequently investigated, which includes the actual use of AR applications in the classroom (TPK 2 in the presented evaluation matrix). Therefore, the didactic preparation for this should be considered much stronger in future formats (see below).
The agreement of the results in the peer feedback with the expert rating indicates the reliability of the developed rating matrix. Even though the expert rating is more critical overall, a clear tendency emerges through the rankings of the individual AR experiments. For the peer feedback, the teachers were only able to evaluate the learning objective (TPK 2) on the basis of the short reports of their colleagues during the reflection session. The implementation sheets filled out directly after the use of the AR experiments in class, as well as the statements in the individual interviews, were not available to them. This could explain why in several cases the TPK 2 aspect was not evaluated by the peers at all. In addition, they were not able to examine the models in detail. Even though the AR experiments were demonstrated to them live, the 3D model was not analyzed in detail. Therefore, the TCK aspect in particular was more difficult for the teachers to evaluate, which also includes the interrelationships of the quantities modeled in GeoGebra. For an effective peer feedback on the TCK, the 3D model should be provided to all teachers for a deep examination of the tools used in GeoGebra.
Overall, the effectiveness of the CPD in terms of model and digital modeling competencies of the participating teachers is evident in the qualitative statements and the created AR experiments. Especially in the open answers, they increasingly considered models and the underlying idealizations as tools of scientific knowledge acquisition after the CPD, which presumably can also be attributed to the input lecture on this topic. The individual feedback by the teachers in the interviews and in the reflection session was also mostly positive, but a suggestion was made to adapt the CPD structure to the teachers’ school commitments in the future. A block event lasting several days could be an alternative to the workshops spread over a half-year period.
5. Conclusion and outlook
This paper presented a measurement tool in the form of an evaluation matrix that can be used to measure teachers’ digital competencies needed for a purposeful use of AR experiments in STEM lessons. This measurement tool was used in the context of a CPD for teachers and tested for its reliability. During the CPD, teachers were trained to develop and use their own AR experiments. It was shown that the measurement instrument is suitable for assessing digital competencies in modeling with GeoGebra. It can also be used in different disciplines to visualize microscopic objects or mental models, and it can be easily adapted to other AR applications with corresponding functions. The next research step in the project will be linking the teachers’ prior experiences with GeoGebra and their attitudes and willingness to use digital tools in their lessons to the results in the expert rating.
To link all phases of teacher training, the concept of AR experiments has been extended to the curriculum at Goethe University Frankfurt with the related project WARP-P (Wirkungsvolle AR im Praktikum Physik; translation: Effective AR in physics laboratory course). In this project, classical practical laboratory experiments were transformed into AR experiments in an electricity laboratory course for pre-service secondary school teachers. The implementation was accompanied by summative feedback and the students’ motivation and attitudes were evaluated. In the long term, the findings from the CPD and the laboratory course are used to establish AR experiments as an integral part of the teacher training curriculum. In order to ensure the reliability of the developed measurement instruments, especially the evaluation matrix for digital competencies, the CPD concept has been adapted to a seminar for pre-service teachers. In the seminar, they are likewise trained how to use GeoGebra to develop their own 3D models for AR experiments and how to apply them. The university’s student lab serves as the framework in this context, providing a venue for target-group-friendly intervention studies. In this second setting, the evaluation matrix is applied again and improved by the findings of the expert rating in the CPD. The project team can directly observe how the students’ AR experiments are working in a practical context and additionally survey the students themselves, in order to assess the TPK 2 competency aspect. This is a major advantage compared to the only verbally reported implementation of the teachers’ AR experiments.
Another research desideratum is the actual use of AR experiments in STEM education on a broad scale. The implementation in other subjects besides physics is also an area that should be further evaluated in the future. With the help of the OER for physics developed in the project, as well as the self-study modules for working with GeoGebra, the concept of AR experiments can be distributed to other disciplines. Finally, the question arises if and how AR experiments are used in everyday teaching. Longitudinally, it should also be investigated to what extent they can ultimately improve science teaching.
Data availability statement
The raw data supporting the conclusions of this article will be made available by the authors, without undue reservation.
Ethics statement
The studies involving human participants were reviewed and approved by Ethics committee of the science didactic institutes and departments (FB 13,14,15) of the Goethe University Frankfurt am Main. The patients/participants provided their written informed consent to participate in this study. Written informed consent was obtained from the individual(s) for the publication of any potentially identifiable images or data included in this article.
Author contributions
MF, JW, RE, and AT designed the project structure and CPD. AT developed the theoretical framework and most of the GeoGebra 3D models. MF and AT carried out the study. During the CPD, RE, JW, and AT provided input about their research areas. MF designed the self-study module. MF and MT analyzed the data. MT conducted and evaluated the interviews. JW and MU designed the initial project and applied for the funds. RE supervised the project. All authors contributed to the article and approved the submitted version.
Funding
This project is part of the “Qualitätsoffensive Lehrerbildung,” a joint initiative of the Federal Government and the Länder which aims to improve the quality of teacher training. The program is funded by the Federal Ministry of Education and Research. The authors are responsible for the content of this publication. The pilot run of the CPD was financially supported by the Joachim Herz Foundation. Further financial support for publishing was provided by the Open Access Publication Fund of Goethe University Frankfurt, Germany.
Conflict of interest
The authors declare that the research was conducted in the absence of any commercial or financial relationships that could be construed as a potential conflict of interest.
Publisher’s note
All claims expressed in this article are solely those of the authors and do not necessarily represent those of their affiliated organizations, or those of the publisher, the editors and the reviewers. Any product that may be evaluated in this article, or claim that may be made by its manufacturer, is not guaranteed or endorsed by the publisher.
Supplementary material
The Supplementary material for this article can be found online at: https://www.frontiersin.org/articles/10.3389/feduc.2023.1180266/full#supplementary-material
Footnotes
2. ^The hyperlink to the self-study module can be provided by the authors upon request (in German).
3. ^https://physikexperimentieren.uni-frankfurt.de/ar-experimente/ (in German).
References
Altinpulluk, H. (2019). Determining the trends of using augmented reality in education between 2006–2016. Educ. Inf. Technol. 24, 1089–1114. doi: 10.1007/s10639-018-9806-3
Altmeyer, K., Kapp, S., Thees, M., Malone, S., Kuhn, J., and Brünken, R. (2020). The use of augmented reality to foster conceptual knowledge acquisition in STEM laboratory courses—theoretical background and empirical results. Br. J. Educ. Technol. 51, 611–628. doi: 10.1111/bjet.12900
Billion-Kramer, T., Lohse-Bossenz, H., and Rehm, M. (2020). “Vignetten zum Modellverständnis – eine Chance für die Lehrerbildung in den naturwissenschaftlichen Fächern” in Vignettenbasiertes Lernen in der Lehrerbildung—Fachdidaktische und pädagogische Perspektiven. eds. M. E. Friesen, J. Benz, T. Billion-Kramer, C. Heuer, H. Lohse-Bossenz, and M. Resch (Weinheim: Beltz Juventa), 138–152.
Carmigniani, J., and Furht, B. (2011). “Augmented reality: an overview” in Handbook of augmented reality. ed. B. Furht (New York: Springer), 3–46.
Cohen, J. (1977). Statistical power analysis for the behavioral sciences Elsevier Science & Technology.
Freese, M., Winkelmann, J., Teichrew, A., and Ullrich, M. (2021). “Nutzung von und Einstellungen zu Augmented Reality im Physikunterricht” in Naturwissenschaftlicher Unterricht und Lehrerbildung im Umbruch? ed. S. Habig (Duisburg, Essen: University of Duisburg-Essen), 390–393.
Gilbert, J. K., and Justi, R. (2016). Modelling-based teaching in science education. Cham: Springer International Publishing.
Griffin, P., McGaw, B., and Care, E. (Eds.). (2011). Assessment and teaching of 21st century skills. Springer Netherlands
Huwer, J., Irion, T., Kuntze, S., Schaal, S., and Thyssen, C. (2019). “From TPaCK to DPaCK–digitalization in education requires more than technical knowledge” in Education research highlights in mathematics, science and technology. eds. M. Shelly and A. Kiray (Ames: IRES Publishing), 298–309.
Huwer, J., Lauer, L., Seibert, J., Thyssen, C., Dörrenbächer-Ulrich, L., and Perels, F. (2018). Re-experiencing chemistry with augmented reality: new possibilities for individual support. World J. Chem. Educ. 6, 212–217. doi: 10.12691/wjce-6-5-2
Ibáñez, M. B., Di Serio, A., Villarán, D., and Delgado Kloos, C. (2014). Experimenting with electromagnetism using augmented reality: impact on flow student experience and educational effectiveness. Comput. Educ. 71, 1–13. doi: 10.1016/j.compedu.2013.09.004
Koparan, T., Dinar, H., Koparan, E. T., and Haldan, Z. S. (2023). Integrating augmented reality into mathematics teaching and learning and examining its effectiveness. Think. Skills Creat. 47:101245. doi: 10.1016/j.tsc.2023.101245
Lipowsky, F., and Rzejak, D. (2015). Key features of effective professional development programmes for teachers. Ricercazione 7, 27–51.
Louca, L. T., and Zacharia, Z. C. (2012). Modeling-based learning in science education: cognitive, metacognitive, social, material and epistemological contributions. Educ. Rev. 64, 471–492. doi: 10.1080/00131911.2011.628748
Milgram, P., Takemura, H., Utsumi, A., and Kishino, F. (1995). Augmented reality: a class of displays on the reality-virtuality continuum. Proc. Telemanipul. and Telepres. Technol. 2351, 282–292. doi: 10.1117/12.197321
Mishra, P., and Koehler, M. J. (2006). Technological pedagogical content knowledge: a framework for integrating technology in teacher knowledge. Teach. Coll. Rec. 108, 1017–1054. doi: 10.1111/j.1467-9620.2006.00684.x
NRC, National Research Council (2012). A framework for K-12 science education. Practices, crosscutting concepts, and Core ideas. Washington, DC: The National Academies Press.
Peeters, H., Habig, S., and Fechner, S. (2023). Does augmented reality help to understand chemical phenomena during hands-on experiments?—implications for cognitive load and learning. Multimodal Technol. Interact. 7:9. doi: 10.3390/mti7020009
Shulman, L. S. (1986). Those who understand: knowledge growth in teaching. Educ. Res. 15, 4–14. doi: 10.2307/1175860
Teichrew, A., and Erb, R. (2020). How augmented reality enhances typical classroom experiments: examples from mechanics, electricity and optics. Phys. Educ. 55:065029. doi: 10.1088/1361-6552/abb5b9
Thees, M., Kapp, S., Strzys, M. P., Beil, F., Lukowicz, P., and Kuhn, J. (2020). Effects of augmented reality on learning and cognitive load in university physics laboratory courses. Comput. Hum. Behav. 108:106316. doi: 10.1016/j.chb.2020.106316
Thiele, M., Mikelskis-Seifert, S., and Wünscher, T. (2005). Modellieren-Schlüsselfähigkeit für physikalische Forschungs-und Lernprozesse. PhyDid A, 1, 30–46. Available at: http://www.phydid.de/index.php/phydid/article/view/29. (Accessed March 1, 2023).
Thoms, L.-J., Kremser, E., von Kotzebue, L., Becker, S., Thyssen, C., Huwer, J., et al. (2022). A framework for the digital competencies for teaching in science education—DiKoLAN. J. Phys. Conf. Ser. 2297:012002. doi: 10.1088/1742-6596/2297/1/012002
Tondeur, J., Aesaert, K., Pynoo, B., van Braak, J., Fraeyman, N., and Erstad, O. (2017). Developing a validated instrument to measure preservice teachers’ ICT competencies: meeting the demands of the 21st century. Br. J. Educ. Technol. 48, 462–472. doi: 10.1111/bjet.12380
Upmeier Zu Belzen, A., Van Driel, J., and Krüger, D. (2019). “Introducing a framework for modeling competence,” in Models and Modeling in Science Education. Towards a Competence-Based View on Models and Modeling in Science Education. eds. A. Upmeier Zu Belzen, D. Krüger, and J. DrielVan (Cham: Springer), 12, 3–19.
Vogelsang, C., Laumann, D., Thyssen, C., and Finger, A. (2018). “Der Einsatz digitaler Medien im Unterricht als Teil der Lehrerbildung-Analysen aus der Evaluation der Lehrinitiative Kolleg Didaktik:digital” in Qualitätsvoller Chemie-und Physikunterricht. ed. C. Maurer (Regensburg: University of Regensburg), 230–233.
Weng, C., Otanga, S., Christianto, S. M., and Chu, R. J.-C. (2020). Enhancing students’ biology learning by using augmented reality as a learning supplement. J. Educ. Comput. Res. 58, 747–770. doi: 10.1177/0735633119884213
Windschitl, M., and Thompson, J. (2006). Transcending simple forms of school science investigation: the impact of preservice instruction on teachers’ understandings of model-based inquiry. Am. Educ. Res. J. 43, 783–835. doi: 10.3102/00028312043004783
Winkelmann, J. (2023). On idealizations and models in science education. Sci. Educ. 32, 277–295. doi: 10.1007/s11191-021-00291-2
Keywords: augmented reality, digital competencies, physics teaching and learning, modeling, technological pedagogical content knowledge (TPACK), continuing professional development (CPD), STEM teaching and learning
Citation: Freese M, Teichrew A, Winkelmann J, Erb R, Ullrich M and Tremmel M (2023) Measuring teachers’ competencies for a purposeful use of augmented reality experiments in physics lessons. Front. Educ. 8:1180266. doi: 10.3389/feduc.2023.1180266
Edited by:
Sebastian Becker, University of Cologne, GermanyReviewed by:
André Bresges, University of Cologne, GermanyHolger Weitzel, University of Education Weingarten, Germany
Copyright © 2023 Freese, Teichrew, Winkelmann, Erb, Ullrich and Tremmel. This is an open-access article distributed under the terms of the Creative Commons Attribution License (CC BY). The use, distribution or reproduction in other forums is permitted, provided the original author(s) and the copyright owner(s) are credited and that the original publication in this journal is cited, in accordance with accepted academic practice. No use, distribution or reproduction is permitted which does not comply with these terms.
*Correspondence: Mareike Freese, freese@physik.uni-frankfurt.de