- 1School of Education, Indiana University – Bloomington, Bloomington, IN, United States
- 2School of Education, Rutgers University, New Brunswick, NJ, United States
Introduction: This study reports on a classroom intervention where upper-elementary students and their teacher explored the biological phenomena of eutrophication using the Modeling and Evidence Mapping (MEME) software environment and associated learning activities. The MEME software and activities were designed to help students create and refine visual models of an ecosystem based on evidence about the eutrophication phenomena. The current study examines how students utilizing this tool were supported in developing their mechanistic reasoning when modeling complex systems. We ask the following research question: How do designed activities within a model-based software tool support the integrations of complex systems thinking and the practice of scientific modeling for elementary students?
Methods: This was a design-based research (DBR) observational study of one classroom. A new mechanistic reasoning coding scheme is used to show how students represented their ideas about mechanisms within their collaboratively developed models. Interaction analysis was then used to examine how students developed their models of mechanism in interaction.
Results: Our results revealed that students’ mechanistic reasoning clearly developed across the modeling unit they participated in. Qualitative coding of students’ models across time showed that students’ mechanisms developed from initially simplistic descriptions of cause and effect aspects of a system to intricate connections of how multiple entities within a system chain together in specific processes to effect the entire system. Our interaction analysis revealed that when creating mechanisms within scientific models students’ mechanistic reasoning was mediated by their interpretation/grasp of evidence, their collaborative negotiations on how to link evidence to justify their models, and students’ playful and creative modeling practices that emerged in interaction.
Discussion: In this study, we closely examined students’ mechanistic reasoning that emerge in their scientific modeling practices, we offer insights into how these two theoretical frameworks can be effectively integrated in the design of learning activities and software tools to better support young students’ scientific inquiry. Our analysis demonstrates a range of ways that students represent their ideas about mechanism when creating a scientific model, as well as how these unfold in interaction. The rich interactional context in this study revealed students’ mechanistic reasoning around modeling and complex systems that may have otherwise gone unnoticed, suggesting a need to further attend to interaction as a unit of analysis when researching the integration of multiple conceptual frameworks in science education.
Introduction
Scientific modeling remains a critical practice in the process of understanding phenomena through scientific inquiry (National Research Council, 2012; Pierson et al., 2017). A persistent challenge for science educators is to teach young students modeling practices in the context of complex systems, where disparate connections and relations in a system make up a network of emergent causal processes that produce observable scientific phenomena, such as eutrophication in an aquatic ecosystems (Hmelo-Silver and Azevedo, 2006; Assaraf and Orion, 2010). Past science education research demonstrates that elementary students have the capacity to effectively engage with complex systems concepts when supported by strong scaffolds in instruction which support students’ engagement in scientific modeling practices (Yoon et al., 2018). However, mechanisms remain a challenging aspect of scientific explanations for young learners to articulate because students often do not recognize the underlying hidden relationships between elements of a system (Russ et al., 2008).
In order to scaffold the alignment of students’ modeling practices with systems thinking, we developed the modeling and evidence mapping environment (MEME) software tool (Danish et al., 2020, or see http://modelingandevidence.org/), which explicitly scaffolds the Phenomena, Mechanism, and Components (PMC) framework (Hmelo-Silver et al., 2017a), a systems thinking conceptual framework designed to support students in thinking about these three levels of biological systems, within a modeling tool (see Figure 1). MEME was created to allow students to create and refine models of a biological ecosystem through a software interface. The aim of the current study is to examine how students utilizing this tool reason about mechanisms when iteratively modeling complex systems. Towards these ends, we investigate the following research question: How do designed activities within a model-based software tool, scaffolded with the PMC framework, support the integration of complex systems thinking and the practice of scientific modeling for elementary students?
Theoretical framework
Our work is grounded in sociocultural theories of learning (Vygotsky, 1978), which assert that the cultural contexts and communities that people interact in are inseparable from the process of learning, and therefore must be rigorously analyzed. We had a particular focus on how the designed elements of a learning environment mediate (i.e., transform) the ways in which students reason about ideas in science. Mediation here refers to something in an environment that comes between a subject and their goal, and consists of mediators, or the tools, rules, community, and divisions of labor which support and transform students’ participation in an activity as they pursue particular goals (Engeström, 2001; Wertsch, 2017). A key feature of mediation is that it constitutes a reciprocal relationship between subjects and objects. So, while a mediator certainly shifts how we pursue certain goals in activity, we in turn transform the mediators through taking them up and appropriating them. This appropriation internalizing them into our own practices, and of course the goals of our activity further shape how we recognize the potential value or role of the mediator (Wertsch, 2017). For instance, while particular features in MEME, such as being able to link evidence in a model, might mediate students’ mechanistic reasoning about a complex system, that mechanistic reasoning will in turn affect how they utilize and take up that particular feature in their collaborative model creation and revision.
In the larger project this work is situated in, titled Scaffolding Explanations and Epistemic Development for Systems (SEEDS), our primary goal was to design a software tool and set of collaborative inquiry learning activities which integrated support for multiple theoretical frameworks to foster robust science learning. Specifically, we aimed to bridge complex systems thinking (Hmelo-Silver and Azevedo, 2006), scientific modeling (National Research Council, 2013), epistemic criteria (Kuhn, 1977; Murphy et al., 2021), and grasp of evidence (Duncan et al., 2018) in order to integrate the use of evidence in creating and revising models of a complex system (an aquatic ecosystem) in late elementary classrooms. We therefore adopted a design-based research approach (Cobb et al., 2003; Quintana et al., 2004) in order to pursue these goals in order to systematically and iteratively test how these frameworks integrated within a modeling unit directly in the context of a 5th/6th grade classoom. We then iteratively implemented and revised our design throughout the study, streamlining the software and classroom prompts to help explore the potential of this approach.
Complex systems thinking
Complex systems have become increasingly relevant in science education and are highlighted by the Next Generation Science Standards (NGSS) as an important crosscutting concept because of their value in understanding a wide range of emergent phenomena (NRC, 2013). Learning about complex systems often proves difficult for students because they struggle to view the system from multiple perspectives, and assume it is centrally controlled as opposed to emerging from many simple local behaviors (Jacobson and Wilensky, 2006). To learn how observable phenomena emerge in a complex system, learners must attend to, study and represent the underlying mechanisms at play in a system, rather than just the surface-level observable components or details (Wilensky and Resnick, 1999; Assaraf and Orion, 2010). This focus is necessary for students to understand how systems function instead of focusing solely on their components or individual functions (Hmelo-Silver and Azevedo, 2006). Other important aspects of complex systems that are valuable for learners to understand include multiple levels of organization, numerous connections between entities, invisible elements that connect the system, and dynamic causal chains that make up the interactions within a system. These aspects can make it difficult for young learners to begin to understand how a system functions (Jacobson and Wilensky, 2006; Hmelo-Silver et al., 2007; Chi et al., 2012). Additionally, complex systems have emergent properties that are only observable when attending to multiple parts of the system interacting and can go unseen when only considering individual elements (Wilensky and Resnick, 1999). As a result, reasoning about complex systems can often overwhelm students and create too high of a cognitive load for students to effectively reason about.
One example of this – and the focus of this study – is aquatic ecosystems. In the present study, we introduce students to a pond-based aquatic ecosystem where they can observe the eutrophication phenomena in action. This system consists of fish interacting with other entities including, but not limited to, algae, plants, predators, and levels of dissolved oxygen present in the water. When something new is introduced to the system to disrupt these interactions, it can be catastrophic for the system for reasons that may not be immediately salient to learners who may just study one aspect of the system, such as students thinking fish get sick and die because of pollution in the water rather than the system falling out of equilibrium. In our imaginary yet realistic context, nutrient runoff from local farms has washed into a local body of water during heavy rainfall, diminishing fish populations during the summer months when the algae blooms. Students are tasked with developing scientific models to represent and explain this phenomena informed by various pieces of data and evidence that we provide to them via the MEME interface. It is a challenge for many students to discern the cause of the fish population decline from disparate pieces of data and evidence, though this is a more realistic experience of scientific analysis than being presented with all of the key information in one tidy package. Our design goal was to create both a software tool (MEME) and a set of activities to scaffold students’ reasoning about this complex system.
Prior research on systems thinking has demonstrated that scholars and educators should focus on identifying instructional tools and activities that can explicitly mediate students’ reasoning about complex systems, helping orient learners to the need to understand the system on multiple levels (Danish, 2014). In this context, we grounded our learning designs in the PMC conceptual framework, which has been shown to support students in engaging with key dimensions of systems (Hmelo-Silver et al., 2017a; Ryan et al., 2021). The PMC framework is a way to support students in explicitly thinking about three key levels of biological systems (phenomena, mechanisms, and components) that can help make many of the underlying relationships within a system salient. In the PMC framework, students frame their ideas around a given phenomena (e.g., an aquatic ecosystem), uncover underlying causal mechanisms that undergird a phenomena (e.g., excess nutrients in a pond causing an algal bloom), and investigate the components (e.g., fish, algae, and dissolved oxygen) that interact to create the mechanisms. Activities, scaffolds, and tools that align with the PMC framework explicitly represent complex systems through the combinations of various components within a system, and represent the relationships between them through descriptive mechanisms, resulting in students’ developing a metacognitive awareness of the system and its various, disparate features (Saleh et al., 2019). To help orient students towards the importance of these levels (P, M, and C), we designed MEME to make them required and salient as students represented the system they were exploring.
Student modeling of complex systems
Scientific modeling has been long established as a core scientific practice relevant to young students’ science learning (Lehrer and Schauble, 2005; National Research Council, 2012). Modeling in this context refers to a representation created in order to abstract the causal mechanisms of complex phenomena, and highlight particular causal chains and features to scaffold scientific reasoning and prediction (Schwarz et al., 2009). Therefore, many educators focus on modeling as a practice that involves creating and revising a representation rather than a single representational product. Nonetheless, models can take many forms including a diagram of the water cycle illustrating how water shifts and changes form in response to environmental stimuli, or a food web highlighting interactions between organisms in an ecosystem.
When one constructs a scientific model, choices must be made in how simple or complex a model should be, and what features of a phenomenon should be highlighted. For students new to the practice of modeling, these choices can be overwhelming. When teaching modeling to students, it is necessary to not only teach students how to create a good model, but to help them to understand the epistemics of what makes a scientific model good according to the scientific community (Barzilai and Zohar, 2016). We draw on the idea of epistemic criteria, or the standards established in the scientific community of what constitutes a valid and accurate product of science (Pluta et al., 2011). For instance, in our projects we worked with the students to establish a set of epistemic criteria about what constitutes a good scientific model, including model coherence, clarity, and how well the model fits with evidence. This allowed for streamlined goals for students to work towards when constructing their models, such as fitting their models to evidence, which in turn supported the validity of the components and mechanisms they represented in their models. We then represented these criteria within MEME in the interface used for students to give each other feedback.
Pluta et al. (2011) emphasize that students’ understanding of epistemic criteria is interconnected with their understanding of modeling. They emphasize that if students “hold that models are literal copies of nature, they will likely fail to understand why models need to be revised in light of evidence” (p. 490). Models are not static entities and require revision as scientists’ understanding of phenomena changes. With this epistemic criteria in mind, we aligned our activity designs with the grasp of evidence framework which focuses on developing students’ understanding of how scientists construct, evaluate and use evidence to continually develop their understanding of phenomena, such as creating and revising models (Ford, 2008; Duncan et al., 2018).
We focused specifically on how students, who are not yet experts in scientific inquiry, interpreted evidence and determined what parts of data are significant to represent or revise in their models (Lehrer and Schauble, 2006). In fact, a primary feature in MEME was a repository of data and reports which we created for the unit, which was directly embedded into the software interface for students to explore as they created and refined their models. Students were able to directly read over empirical reports and data, and decide what reports were useful evidence that either supported or disproved claims they made about the aquatic ecosystem (Walton et al., 2008).
As the unit went on, students were tasked with revising and iterating on their model, based on their interpretation of new sets of evidence introduced to them. Students added new elements or modified existing elements in their models, and could directly link a piece of evidence to a specific feature of their model to support their reasoning. Not only were students learning to interpret and reason around empirical evidence, but the evidence they reviewed was grounded within the PMC framework as well. As students began to interpret multiple, disparate pieces of data about the aquatic ecosystem, they began to make claims about the system, and represented this in their models through various components and mechanisms.
In the current study, the practice of modeling included creating a box and arrow representation of the aquatic ecosystem (see Figure 1), collaboratively evaluating it alongside peers, and iterating on models based on peer and expert feedback (Danish et al., 2021). Models in MEME build on the idea of simple visual representations, such as stock-and-flow diagrams (Stroup and Wilensky, 2014) and concept maps (Safayeni et al., 2005), both of which can help students to link disparate ideas, and grow more complex as they iteratively refine them while also supporting the development of more coherent systems understanding. The difference here that distinguishes MEME from other model-based tools, is that the software interface was intentionally designed to directly bridge students’ developing epistemic criteria around the practice of modeling through a comment feature where peer feedback was given based off of a list of epistemic criteria.
Students’ interpretation of evidence in relation to claims around complex systems could be directly linked into their model through a “link evidence” button, and their learning of complex systems through representing aspects of the PMC framework in their models were directly scaffolded as pieces for them to create their models (see Figure 1 for a look at all these features). Taken alone, any of these concepts are difficult for students to take on, but we argue here that designing both tools and activities with the integration of these critical scientific practices, help to scaffold students in their complex scientific reasoning. In this particular study, we focus on how this integration led to incredibly rich and detailed interactions around mechanistic reasoning for the students we worked alongside.
Development of mechanisms represented in models of complex systems
In this study, we were interested in focusing on the PMC feature of a causal mechanism in order to closely examine how students’ mechanistic reasoning was mediated through the use of the MEME tool and designed learning activities. Here, we define mechanism as the “entities and activities organized such that they are productive of regular changes from start or setup to finish” of a scientific phenomena (Machamer et al., 2000, p. 3). Within complex systems, mechanisms are the underlying relationships that often go unobserved by novices, and are only made clear when focusing on how various components are interrelated to each other. As a result, mechanistic processes are a common challenge for students when first learning about phenomena (Hmelo-Silver and Azevedo, 2006).
Schwarz and White (2005) outline plausible mechanisms as a key epistemic criterion needed to understand the nature of scientific models. There is a need for students to understand that models consist of causal mechanisms in order to understand their explanatory purposes (Pluta et al., 2011). Our design goals were to engage students in scientific modeling activities which explicitly scaffolded mechanistic explanations to support students in developing their systems thinking and understanding of scientific modeling. In MEME for example, one of the core modeling features present within the tool is for students to represent processes (i.e., mechanisms) through the form of labeled arrows (see Figure 1) connecting two entities (i.e., components) in a system.1
Attending to how students represent mechanisms as they engage in constructing and iterating on a scientific model can help us to better understand how their mechanistic reasoning develops within interaction. For example, Russ et al. (2008) noted that mechanistic reasoning shifts between levels of reasoning tend to occur when students shift from describing the phenomena in a “show-and-tell manner to identifying the entities, activities, and properties of complex systems” within interaction (p. 520). They also noted that lower levels of mechanistic reasoning may act as “building blocks” to lead into higher forms of reasoning (p. 521). Further, prior work indicates that when modeling, students “generate mechanisms using a wide variety of pre-existing ideas” (Ruppert et al., 2019). Looking closely at how students’ mechanistic reasoning developed across a modeling unit through the use of various mediators can help us to understand how to better support these practices and provide insight into designing for these kinds of mediating interactions in future iterations of the project.
While the literature emphasizes that domain-specific knowledge can foster the development of mechanistic reasoning in models (Duncan, 2007; Bolger et al., 2012; Eberbach et al., 2021), a key finding in our prior work was “that neither the type nor the number of domain-specific propositions included was important to how students developed mechanisms,” (Ruppert et al., 2019, p. 942). These contradictions in the literature indicate a need for further investigation on how students’ mechanistic reasoning develops in interaction when engaging in modeling. Researchers are undertaking these kinds of efforts, such as work by Mathayas et al. (2019) utilizing epistemic tools, such as embodied representations of phenomena through gesture, which can support the development of mechanistic explanatory models. We set out with similar goals in this study to investigate how MEME and our designed learning activities can help to support these same shifts in students’ representations of mechanism in their modeling.
Methods
Design
The larger project that this study is a part of, SEEDS, aimed to understand how fifth and sixth grade students engage with evidence as they explore complex aquatic ecosystems through modeling. In commitment to our design-based research approach (Cobb et al., 2003; Quintana et al., 2004), we created conjecture maps (Sandoval, 2004; 2014) to outline how we believed our theory was represented in our design in order to achieve our curricular and design goals as we moved through designing the modeling unit (see Figure 2). Conjecture maps are visual representations of design conjectures, which “combines the how and the why, and thus allows the research to connect a value (why in terms of purpose) with actions (how in terms of design or procedures) underpinned by arguments (why in terms of scientific knowledge and practical experience)” (Bakker, 2018, p. 49). This framing allows designers to make explicit connections between theoretical commitments and material features of a design. Within the conjecture map, aspects of our design that emerged as the focus of the current study are bolded to indicate the guiding design principles that grounded this analysis.
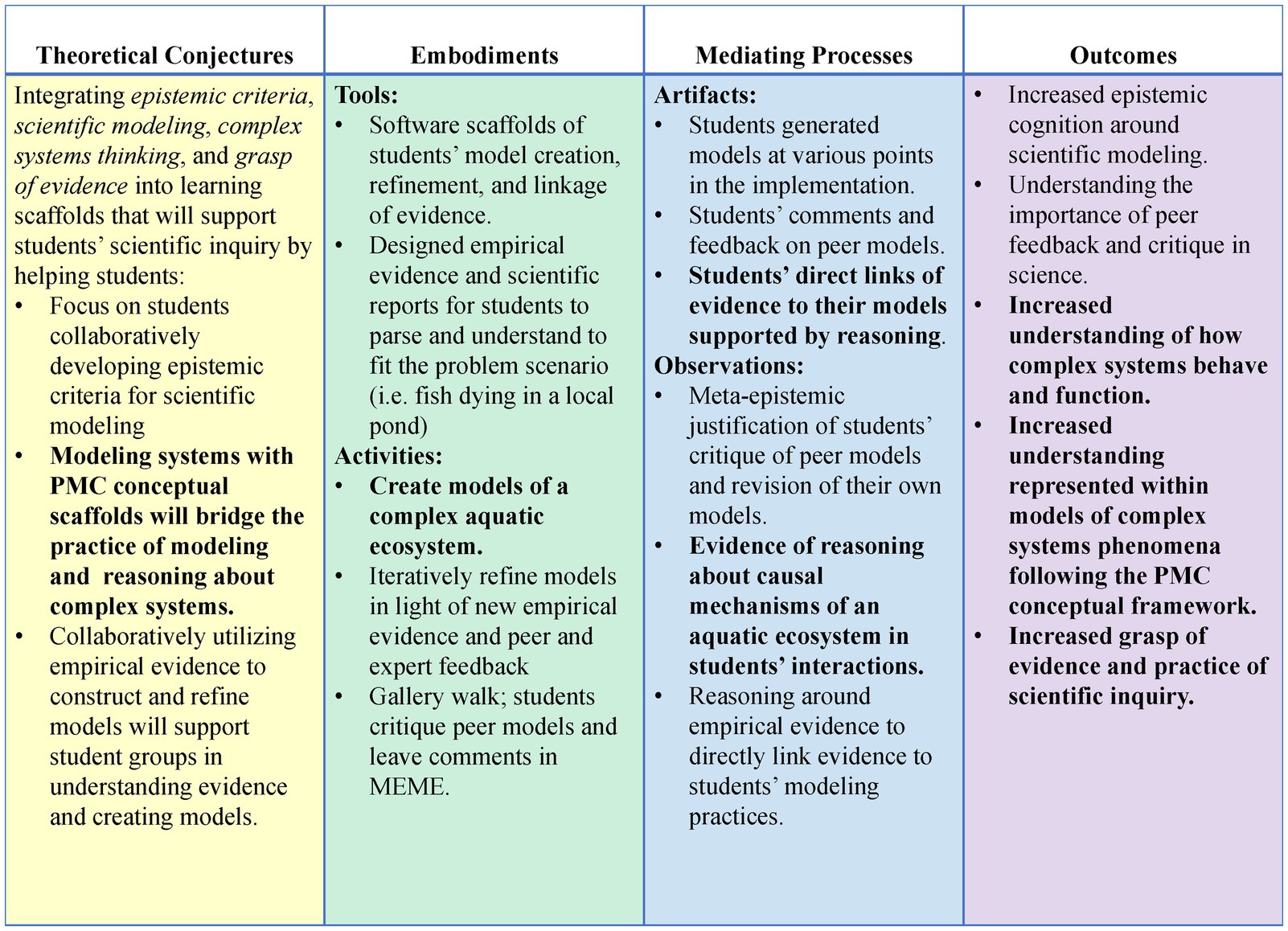
Figure 2. Conjecture map of our theoretical and embodied conjectures of the larger project. Bolded items are the focus of the present study.
In the spirit of DBR, we have iterated on the design of the MEME tool across the project’s lifespan. For example, based on prior pilot work (Moreland et al., 2020) that revealed that students had a difficult time parsing the PMC framework, we adapted the language of the framework to better accommodate our younger 5th/6th grade participants. In MEME, the tool allows students to create entities (components), processes (mechanisms), and outcomes (results of the phenomena) of the aquatic ecosystem (see Figure 1). Through these kinds of design choices, such as using simple visual elements in how students could construct their model, we were able to directly embed scaffolds for the PMC framework into the tools students utilized when learning how to create and refine their model of a complex system.
We carried out a 7 days modeling unit aimed at teaching the phenomena of eutrophication, or when a body of water receives a high amount of nutrients and creates an algal bloom and takes up all of the dissolved oxygen in the water, creating a dead zone. We took part in roleplaying with students, where a team of scientists we called the Fresh Org tasked the students with trying to figure out and create a model of what was going on in Blue Pond. Students took on the task of solving the problem of why fish were suddenly dying during the summer months in a local aquatic ecosystem. 15/17 consented students were assigned to small groups (2–3 students), and used MEME to develop a comprehensive model to explain what caused the fish to suddenly die in the summertime. On day 1, we introduced the concept of scientific modeling in a short lesson we created based on prior implementations of SEEDS (Danish et al., 2020). We also introduced the activity that students would be tasked with researching the problem and building a model to represent what was going on in the system. From days 1 through 4, students received new evidence sets from Fresh Org related to sunlight, algae, nutrients, dissolved oxygen, the fish in the pond, and water quality related to the system (see Figure 3 for an example of these reports). Each evidence set consisted of 2–3 pieces of empirical data or reports, via the evidence library in MEME (see Figure 1).
The evidence sets each had a theme (e.g., fish death, algae, fertilizer and nutrients) and disparate pieces of evidence were deliberately paired together for students to connect in their models (e.g., a piece of evidence that had fish deaths highest in the summer, and a farmer’s inventory list marking that they distributed pesticides and fertilizer in the month of June). As students interpreted evidence and created their models, facilitators including the research team and the classroom teacher went around the room helping with technical difficulties and asked scaffolded probing and discussion questions (e.g., “do you think this evidence supports anything in your model?”). At the end of each day, students would provide feedback of questions they still had about the complex system, which Fresh Org would then respond to with a summary at the start of each day.
Students collaboratively worked through this evidence in their groups, and then constructed and refined their models in MEME. During days 2 and 4, students participated in a structured “gallery walk” activity where they (1) gave peer feedback on peer models, (2) addressed comments made by peers on their models, and (3) made revisions to their models based on peer feedback. Students finalized their group models on day 5 of the unit, and on day 6 the whole class collaboratively created a consensus model. Finally on day 7 the entire class participated in a discussion of the implementation, where students discussed the epistemic nature of evidence and modeling, along with what caused the initial problem in the pond, the wider effects algal blooms and fertilizer can cause, and the possible solutions on how farmers and community members might prevent these kinds of problems from happening in the first place.
Context and participants
Across the larger DBR project, we have worked closely with multiple teachers in both public and private schools. The context of the present study was a local private school in the Midwestern United States in the fall of 2021, where we had previously worked with the 5th/6th grade teacher of the school on pilot studies of this project. We met with the teacher multiple times in the months leading up to the implementation, where he had direct input into the decisions and designs, such as our empirical reports and evidence, before we began. During the implementation, while the teacher preferred that we run the activities and technology, he was an integral facilitator and supported the activities in the classroom. He often asked discussion questions to students as we wrapped up the day. During modeling activities, he would walk around the room and assist students when creating and revising their models, and during gallery walk activities where students critiqued each other’s models he instilled a classroom norm of offering two compliments for every piece of critique offered in someone’s model. He also helped to facilitate any whole class discussions that occurred in the class, such as on day 7 when the class had a debrief discussion on the unit.
The research team went in every other day for 4 weeks for a total of 7 days, with a pre-post interview taking place at the beginning and end of the implementation According to our demographics survey we administered, of the 15 consented students who participated in the study, there were 8 girls, 6 boys, and 1 other/unspecified. Researchers taught a designed model-based inquiry unit about eutrophication in an aquatic ecosystem over 7 days, with each day being 90 min long. The unit was created by the research team, which consisted of science education and learning sciences scholars, to align with the NGSS Lead States (2013) standards and core goals, such as cross-cutting concepts. We chose to create this unit from the ground up to align it with the design of our research goals and the MEME software tool. Additionally, we collaborated with the teacher while we designed the unit. He informed us of what his students had learned in his science units already, including how to test water quality and the importance of keeping water within the community’s watershed clean. This collaboration allowed us to better integrate the modeling unit to connect with the teacher’s existing science curriculum, including the creation of pieces of evidence related to water quality that students used to inform their model construction. Each day of the activity unit took place during the students’ science block time in their schedule during regular class time. Students then worked in small groups (6 dyads and 1 triad) in MEME to iteratively build and edit scientific models using a library of designed empirical evidence.
Data collection
The primary data source for this analysis was the set of models that students created throughout the project. While students revised their models daily, we focused on their progress through the curriculum unit by examining the models on days 1, 3, and 5 out of the 7 days. These days were chosen because day 1 was the first-time students used MEME to begin constructing their models, day 3 was approximately the mid-point of the implementation, and day 5 was the final day that student groups created their models. On day 6 the class made a consensus model, and day 7 was a debrief with the whole class. A second data source consisted of video and audio recordings of classroom interactions and screen recordings of students building their models in MEME to look into what scaffolds and interactions supported students’ systems thinking. Specifically, we were interested in what within student interaction mediated their construction and reasoning around mechanisms of the complex aquatic ecosystem, as well as how their mechanistic reasoning shaped their model construction.
Data analysis
Analysis of this data consisted of qualitatively coding students’ models for mechanistic reasoning. This was followed by a close examination of content-logged video data capturing the creation of mechanisms in models, and the interactions between peers which led to their creation. Students’ models were qualitatively coded by three researchers focusing on the complexity of students’ mechanistic reasoning. We adapted Russ et al. (2008) coding of mechanistic reasoning, which specifies a hierarchy of mechanistic reasoning developments in student interactions (see Table 1). One code from the original codebook, “identifying entities,” was removed due to “entities” being one of the embedded features of the MEME modeling tool and thus we wanted to avoid inflating students’ code frequencies. While Russ’s coding scheme was originally meant for looking directly at student interactions, it has also been used to code student generated models as well (Ruppert et al., 2019). Qualitative coding of models consisted of looking at MEME models at certain points in time in the unit and coding the individual processes and entities within a model as a represented mechanism. For consistency within our data set, a mechanism in a MEME model consisted of two entities connected by a process (see Figure 1 for an example). Each of these were coded within a groups’ model, with each group having an average of 3–6 mechanisms per model, depending on the group and day of the unit.
To begin the coding process, we carried out Russ’s coding scheme on a subset of models to establish interrater reliability. Following conventions of interrater reliability (McDonald et al., 2019), Author 1 coded the models from the end of day 5 of the implementation (33% of the total data set). The final models from day 5 were initially selected because we anticipated that as the final model, they were likely to be the most complex and complete. Following coding of this subset, two additional members of the research team coded the same set of models. In two separate collaborative coding sessions, the team reviewed the coding and discussed each discrepancy that arose between the researchers. By the end of the two sessions, the coding between the three researchers reached a high degree of interrater agreement (95%). Once agreement was reached on this subset of data, we proceeded to code the remaining models from day 1 and day 3. Once models had been coded for days 1, 3, and 5, Author 1 brought the data set back to the research team to look over the results of the coding, where agreement was once again reached (95%).
Following analysis of students’ models, we conducted interaction analysis (IA; Jordan and Henderson, 1995) to closely investigate how students’ mechanistic reasoning emerged and developed across the modeling unit. We looked at previously content-logged video data consisting of students’ discourse as well as their screen recorded actions carried out on the computer within MEME. The content-logged video data identified specific moments where groups created or revised a mechanism in their MEME model, and marked the interactions occurring during these moments. Different student groups were chosen at random to analyze their interactions each day. The models were analyzed (1, 3, and 5) to report on more general group trends as opposed to the unique developments had by any one group. This way, the interactions analyzed highlighted how the students’ engagement with the different mechanisms in the system mediated and in turn were mediated by various features of MEME (e.g., the evidence linking feature) and participating in modeling activities (e.g., taking time to revise their models based on interpretation of new evidence). In these episodes, we looked for elements in MEME and the overall activity which directly influenced students’ reasoning about the complex aquatic ecosystem.
Specifically, we unpacked what occurred during group interaction through examining students’ talk and corresponding moves made within MEME just before or during the creation of mechanisms in models. We were interested closely examining interactions to better understand the ways in which students discussed and represented mechanisms in ways that might not be clear in simply reviewing the static representation of models. We focused on the reciprocal relationship of the identified mediators: students’ interpretation of evidence, features of MEME linking evidence to their models, their negotiations surrounding mechanisms, and their modeling practices. By reciprocal relationship, we mean that each mediator shaped students’ participation in the activities and how they took up other mediators present to support students’ learning throughout the unit. For instance, while students’ interpretation of evidence mediated how students’ represented mechanisms in their models, their mechanistic reasoning in turn mediated how they read through and interpreted the sets of evidence. The IA we conducted revealed how features of MEME and interaction around the creation and revision of their model transformed their mechanistic reasoning, but also how students’ focus on mechanisms within the complex system shaped the way they used MEME and developed their epistemic criteria of what makes a good model. We unpack the results of both the coding and the interaction analysis in the results below.
Results
Russ et al. (2008) hierarchy (see Table 1) of students’ mechanistic reasoning was based on what they determined to be more or less scientifically sophisticated. We took up that same hierarchy based on our prior work of adapting this coding scheme from interaction to student generated models (Ruppert et al., 2019). Our analysis of student models showed a general trend that the mechanisms represented across all group models became more complex as the unit continued (see Figure 4). Interaction analysis carried out on students’ interactions surrounding the creation of these mechanisms revealed several distinct mediators which promoted the creation or refinement of mechanisms in their group models. These include interpreting disparate forms of data in order to make claims identifying mechanisms, utilizing and linking evidence to help develop and refine their mechanistic reasoning, and how playful peer interactions helped to shape their reasoning around mechanisms.
Development of mechanistic reasoning across time
Students’ coded models clearly exhibited development in complex mechanistic reasoning as students iterated on their models of the aquatic ecosystem (see Table 2). Collectively, the development of mechanisms across student models across time improved from the end of day 1 to the end of day 5 (when student models were finalized), with high level mechanisms (levels 4–6 in our coding scheme) being present in all student groups’ models starting at the end of day 3. Not only do our results indicate that students identified and represented more mechanisms within the system as the unit went on, but the majority of mechanisms coded across all final models at the end of day 5 were coded on the upper half of the Russ’s coding scheme for mechanistic reasoning (54% of all mechanisms across all group models). This distribution was evenly spread across groups, with 7 out of 7 of the groups representing at least one high level mechanism within their final models.
These results are a clear indication that students were using MEME to represent sophisticated mechanisms of the complex system, as seen in the increase in higher coded mechanisms across all models as the unit progressed (see Figure 4). Even lower coded parts of the model, such as describing target phenomena, and identifying setup conditions indicate that students were reasoning about causal mechanisms within the system, which are critical for students to understand if they are to effectively learn about both modeling practices (Pluta et al., 2011) and complex systems thinking (Goldstone and Wilensky, 2008). This meant regardless of complexity, students were making causal connections to each and every component of the system that they chose to represent in their modeling. This highlighted that students represented what they interpreted as key aspects of complex systems as they carried out their scientific inquiry.
Furthermore, the majority of all mechanisms that emerged across models were in the middle of the hierarchy and higher, going all the way up to the highest code of chaining together causal chains of various mechanisms to explain how the aquatic ecosystem functions. The three most common coded mechanisms that emerged in students’ models were: identifying activities (level 3; 27% of mechanisms), identifying properties of entities (level 4; 25% of mechanisms), and chaining (level 6; 22% of mechanisms), accounting for 74% of all mechanisms present across student models. Two out of three of the most common codes were in the top levels of Russ’s coding scheme, with only one high level code not commonly occurring across student models in high volume, identifying organization of entities (level 5, 7% of mechanisms). However, in total 7 out of the 7 final models had >50% of their mechanisms coded as the top half of mechanistic reasoning codes. These percentages represent the distribution of mechanisms across all student models on days 1, 3, and 5, which were consistent across individual models as well.
Student models ranged from having between 3–10 mechanisms present in their model depending on the day and group, but distributions of codes were evenly spread across groups. Table 2 highlighted that the three most complex forms of mechanistic reasoning were the majority of coded mechanisms across all models (54% of all coded mechanisms). As we move across each selected day of modeling, we can see a clear development of mechanistic reasoning happening for student groups. The total number of mechanisms identified increase as we move from day 1 to day 3 to day 5 (see Figure 4), which indicated that student groups added more elements to their model in total. We also see a distinct shift in how many complex types of mechanistic reasoning begin to emerge in students’ models. Specifically, identifying activities, identifying properties of entities, and chaining appear at much higher volumes in models as we move across time in the implementation. Figure 4 provides a bar chart visualizing the distribution of coded mechanisms at the conclusion of days 1, 3, and 5 of the unit.
Students’ development of mechanistic reasoning as time went on can be seen most clearly in the development of students’ use of causal chaining in their models, or when students reason about one stage in a mechanism based on what is known about other stages of that particular mechanism (see Figure 4). For instance, at the end of day 1 few groups had used any sort of chaining to represent how components of the aquatic ecosystem were related (8% of coded mechanisms). However, at the end of day 5, when students finalized their models of the aquatic ecosystem, chaining causal mechanisms was the second most occurring code across student models (26% of coded mechanism). Breaking this down by group, 5 out of 7 groups had more than one mechanism coded as chaining in their models, and 7 out of 7 groups each had between 2–4 mechanisms coded in the top half of Russ’s coding scheme. Students’ development in their mechanistic reasoning can clearly be seen across time as they iterate and refine their models.
Mediating the creation of mechanisms within models
Analysis of student interactions around the creation of mechanisms highlighted the key role of the features of MEME, including the evidence resources that students were investigating. These directly mediated students’ talk within their groups surrounding the creation of mechanisms in their models. Below, we analyze episodes of interaction at moments where students created mechanisms within MEME during days 1, 3, and 5 of creating and refining their models of aquatic ecosystems.
Interpreting disparate evidence in the construction of mechanisms
At the start of day 1, students only had access to two pieces of evidence to support their model creation. This was intentional, as this was students’ first-time using MEME, and they were working to understand the primary features of MEME including creating new components and mechanisms to represent the system through their modeling. Students were just starting out and trying to represent and explain the initial problem they had been given – that fish were dying in the pond during the summer months. The first piece of evidence introduced the problem, and provided a graph showing what months the fish deaths rose (July–September). The second piece of evidence was a list of materials used by farmers in nearby local farms, which included pesticides and fertilizer which were distributed in June. Students were tasked with reading these two separate pieces of evidence and creating an initial model.
Students were new to MEME and scientific modeling in general, and were given the task of creating a few entities and processes of the phenomena they were just introduced to. While the mechanisms across groups began as fairly straightforward at the end of day 1 (see Table 2), student interaction revealed the nuanced interactions surrounding the creation of students’ first mechanisms within their models. For example, a group with two students created their first four processes to represent the possible causal mechanisms of farms spreading pesticides into the pond, which then kill the fish. Students had just reviewed the farmer’s inventory list in the evidence library, and like many other groups gravitated towards the use of pesticides in the farm. While pesticides were merely a part of the inventory list, they were not framed in any particular way in the data. For instance, in an exchange with a facilitator, Eddy and Lily explained why they connected the two pieces of evidence to construct their first causal mechanism (see Figure 5).
When the facilitator initially called their attention to the inventory list, Eddy noted that fertilizers and pesticides could be harmful because they were distributed to the local farms in the month of June (lines 2–3). The facilitator inquired how they knew this, and Lily navigated to the first piece of evidence in MEME, pointed the computer mouse to the graph and pointed out that fish deaths were the most in the summer months (line 7). She then made a claim that the pesticides likely took a while to get to the water, and “so you can see it goes up and up and up and up more and then a straight stop” (lines 9–10). Eddy and Lily interpreted separate pieces of evidence, and integrated them into their initial claim represented in their model of the complex systems.
They chose to only represent pesticides (possibly because of prior knowledge surrounding mainstream debates around pesticide use), but reasoned that the farm distributes pesticides, which then spread to the pond, and so the pesticides kill the fish, which then restarted the process. Figure 6 shows how their representation of this mechanism was represented across multiple entities and processes within their model.
What stopped these mechanisms from being coded at higher levels, such as chaining, was that they were isolated in how they were represented, as opposed to being informed by other mechanisms of the system. Given that this instance was at the start of the unit, this makes sense, and the interaction above marked a promising start given their sophisticated interpretation of evidence. The overall trends of mechanism codes at the end of day 1 indicate that Eddy and Lily’s model was typical of what other student groups created as well (see Table 2). This meant that students had a similar interpretation of the two disparate pieces of evidence to reason about the causal mechanisms of the system.
Within these interactions, students analyzed and interpreted novel and distinct forms of data, interpreted connections between them in order to make an initial claim, and then represented their claims through a series of causal mechanisms within their PMC model. Eddy and Lily’s grasp of evidence here, specifically their interpretation and integration of evidence, mediated the ways in which they chose to represent their initial constructions of their model. Their integration of disparate evidence directly supported the claims that they represented through their mechanisms within their model.
Negotiating and linking evidence in the model
During days 2 and 3, students had their first opportunity to offer peer feedback through a “gallery walk” activity where students went into each other’s models in MEME and commented how well they thought it represented the problem they were trying to solve (see Figure 1 for how this feature looked in MEME). They then were able to revise and refine their models based on that feedback. They also examined new sets of evidence that might help them to solve the problem of why fish were dying in the pond. This led to students iteratively improving their representation of mechanisms in their model in response to peer critiques, which we detail in an example below.
The distributions of coded mechanisms within models (see Figure 4 or Table 2) revealed that at the end of day 3 students were using MEME to represent more complex forms of causal mechanisms in their model. This occurred primarily by more explicitly naming the process that caused the mechanism between two components of the system. Our IA during this point of the implementation revealed that all student groups were collaboratively reasoning around causal mechanisms of the system to negotiate revisions and changes made to their model. For example, two students in a group, Jenny and Claire, made several revisions of both components and mechanisms within their MEME models (see Figure 7).
In one instance, upon receiving peer feedback in the form of a comment which read “it’s a great model but I think you should be more specific,” the group revised a specific entity and process to better represent the causal mechanism of the system. They modified an existing entity which originally read “More Oxygen” and revised it so that the entity was “Less Algae” and added “More Dissolved Oxygen” to the existing process “less death.” This may not seem significant, but it marked a shift in their mechanistic reasoning represented in their models (along with 5 out of 7 of the total groups). Based the feedback and new data that Jenny and Claire had just read, they modified the claim that less algae present in the pond was the main reason that there was more dissolved oxygen in the water and less fish death at the start of a new season. This was Jenny and Claire’s first mechanism that was coded as Chaining, the highest code for mechanistic reasoning present within the models. In changing one of their mechanisms from “new season ➔ less death ➔ fish” to “less algae ➔ more dissolved oxygen and less death ➔ fish lay eggs,” they began to chain together their reasoning across mechanisms (see Figure 7). What’s more, however, is that their further interactions when deciding to link a piece of evidence reveal further insight to how they worked towards representing their claims surrounding this particular mechanism of the system, that less algae in the water provided more oxygen and therefore less fish death (see Figure 8).
In this exchange, Claire and Jenny negotiated how to provide reasoning behind choosing to link a piece of evidence, a report on how much algae grew in the pond over the course of 6 months, in support of one of their claims represented in their model. Claire narrated her thoughts to Jenny, who typed for her. Jenny misunderstood Claire’s explanation during this exchange and typed an incorrect claim (that there were less algae at the same time the fish die). Claire noticed this and called this out by correcting Jenny and says “The algae is lower at the same time the fish die? [but] death is low! (lines 16–17). Jenny recognized this and corrected it quickly, but let Claire know that explanation was not what she had in her own mind (lines 18–19). Claire remarked that it still worked however, and the pair were left satisfied by their linked evidence.
Their conclusion in linking their evidence was that “Because the algae is lower the same time that the fish death is lower.” They linked this to the process in their model to support their mechanism which claimed that less algae meant more dissolved and less fish death. Two distinct mediators emerged which supported students’ mechanistic reasoning here for Claire and Jenny. The first is the act of revising their models upon receiving peer feedback. Peer feedback within their model led them to revisit evidence and negotiate how to better represent their mechanism. This supported them in making revisions to their model. These changes to existing features of their model led to higher coded mechanistic reasoning represented in their model, as evidenced by the emergence of 2 distinct instances of mechanisms coded as chaining to this group’s model at the end of day 3.
Second, MEME’s link evidence feature, which allowed for students to directly link their evidence interpretations into their models, supported further interactions and reasoning on how to explain their claims. The interaction above highlighted how the feature in turn supported negotiation on how Claire and Jenny represented their claims and led to a deeper collaborative understanding of their collective reasoning. The evidence link feature has a prompt which asks students to draw a conclusion from the connection they made to their model (see Figure 1 for an example of this). Jenny and Clair spent time negotiating on how to frame this conclusion, eventually coming to an agreement about how they should frame their reasoning (lines 16–20). This negotiation around how to frame their conclusion led to a collaborative understanding of how Jenny and Claire represented their claims within their models.
Mechanistic reasoning through creative modeling practices
On day 5 out of 7 of the unit, students received their final set of evidence, which informed them that nutrients within fertilizer helped to promote plant growth, and that the algal bloom coincided with a heavy rainfall. Here, two modeling trends began to stick out to us as we moved through the data, all of which stemmed from students using the MEME tool to represent their thinking around the complex system in novel ways. First, students began to create parts of their model to note aspects of the complex system that they did not currently have a full explanation for. For instance, in one group Ben and Henry created two processes related to algae and dissolved oxygen to mark that they thought there was a relationship between the two, but were unsure of what the specific relationship was, a practice which we encouraged to help drive conversations about how to support and clarify such claims (see Figure 9).
Ben and Henry elected to create two processes, “Low Dissolved Oxygen → idk (i.e., I do not know) algae dies or something → High Dissolved Oxygen,” and “High Dissolved Oxygen → idk algae grows or something → Algae.” They noted the relationship between algae and dissolved oxygen, but could not yet support their claims with evidence. These ended up being coded on the lower end of the coding scheme. Their other mechanisms, which were more detailed and coded higher in their models, were all directly linked to pieces of evidence (see Figure 9). Here, Ben and Henry not only represented their model as something that could be revised as they learned more, but also that the mechanisms they were confident in were directly supported by their grasp of the evidence available to them through directly linking evidence to parts of the model they were sure of (see Figure 9). This lined up with how their model at the end of day 5 was coded, with their two highest coded mechanisms of chaining being connected to the parts of their model directly supported by evidence. This is noteworthy because it indicated that even their lower coded mechanistic reasoning present in their models did not necessarily represent a lack of understanding on their part, but rather coincided with modeling practices which noted parts of their model as a work in progress which needed refinement.
Second, many groups began to engage in playful inside jokes and goofing around and tapped into what Gutierrez et al. (1995) call the underlife of the classroom, where students “work around the institution to assert their difference from an assigned role” (p. 451). As Gutierrez and colleagues point out though, this is not inherently unproductive or off-task behavior, and can be mediated through interaction to be powerful moments of learning. For instance, upon looking over evidence that showed that one fish died from a turtle attack while the others suffocated from low oxygen levels, Ben and Henry decided to add the turtle to their model as an entity.
When this turtle emerged in their model alongside a process labeled “lol” (see Figure 10), Ben and Henry decided that they needed to show this off to a facilitator. They showed the turtle to a facilitator, who proceeded to ask if this was now their primary theory of how the fish died. Ben remarked that “No! That’s why we added it to the side cause one of them killed them” (line 7). Ben then proceeded to inform the facilitator that the evidence they had learned about the turtle also confirmed their original claim that the fish had suffocated in the water due to low oxygen (line 10). Ben went on to further explain that “the turtle killed him, but he was already going to suffocate anyways because it also had low blood oxygen levels so the turtle just killed it early” (lines 12–13). Despite their fixation on the turtle, their interaction around it revealed a deep understanding of what was happening within the system on an unseen level, that fish were suffocating because of a lack of oxygen due to the algal bloom.
This particular interaction was important for us to unpack because without the rich interactional context, the static mechanism of “Turtle → lol → Fish Die” may appear to be off-task behavior or even an incorrect interpretation of the evidence and their model. Ben and Henry noted their clear interpretation of the evidence within their discourse with each other and the facilitator, which further supported already created mechanisms in their model, which they later linked with evidence to further support their claim. Ben and Henry’s interaction also highlights how levity and playfulness can lead to deeply nuanced reasoning around the causal mechanisms of a complex system. The underlife of the classroom, such as the inside jokes, silly remarks, or adding funny additions to a sophisticated scientific model further mediated and deepened students’ understandings of complex systems and their epistemic ideas of what a scientific model should consist of.
Discussion
This study contributes to larger discussions of how to better integrate ideas of teaching both scientific modeling and complex systems thinking in elementary students’ scientific inquiry. We closely investigated how students’ mechanistic reasoning progressed and developed while participating in a scientific modeling curriculum unit which was scaffolded with the PMC conceptual framework for systems thinking (Hmelo-Silver et al., 2017a). Our goals of this study were to closely analyze how our various design frameworks, including sociocultural theories of learning (Vygotsky, 1978), mediation (Wertsch, 2017), epistemic criteria (Murphy et al., 2021), grasp of evidence (Duncan et al., 2018), and mechanistic reasoning (Russ et al., 2008) were taken up by our research team and collaborating teacher to better integrate concepts of complex systems thinking (Wilensky and Resnick, 1999; Hmelo-Silver and Azevedo, 2006) and scientific modeling (Pierson et al., 2017) in teaching upper elementary students the nature of science. We sought to deeply understand how our designed activities within a model-based software tool, scaffolded with the PMC framework, support the integration of complex systems thinking and the practice of scientific modeling for elementary students.
The MEME software tool that students used to construct their models directly embodied the core elements of the PMC framework (i.e., outcomes, processes, and entities) as the building blocks in which students constructed their models, as well as making the use of evidence to revise a model salient to learners. Additionally, MEME and the designed modeling unit emphasized constant revision and iteration on student models in light of new evidence given to students surrounding the phenomena they investigated. Initially, students had middling to low levels of mechanistic reasoning emerge in their models, which is to be expected. As students progressed, their reasoning began to improve, and more sophisticated mechanisms began to emerge both in their models and in their peer interactions. By the end, students had a higher number of total mechanisms present in their models, and the majority of coded mechanisms in student models were in the top half of Russ’s mechanistic reasoning learning progression (52% of coded mechanisms across models).
Overall, the findings of this study demonstrated that not only were students able to improve their representations of causal mechanisms in these models over the course of the implementation, but that this type of sophisticated reasoning was mediated in students’ interactions in a number of ways across the implementation. These mediators included (1) the designed materials such as the empirical reports and data structuring students’ inquiry, (2) features of MEME such as the PMC representation, evidence library and evidence linking features, and (3) students’ diverse and playful interactions with their peers which provided constant feedback and opportunities to negotiate meaning of parts of their models.
Limitations
There were several limitations of this study. First, while the overall project collected data at a number of diverse sites and contexts, the data for this study was collected at a private school with much more flexibility in curriculum and structure of students’ day than a typical public school. The school had a free form curriculum that was in complete control of the teachers, which made it easier for us to collaborate with and integrate our unit alongside our partner teacher. While we have run implementations of the SEEDS project in public school contexts, we had also previously worked with this teacher before, so these specific findings may not be generalizable to school settings with more rigid schedules and curriculum without further investigation. Second, students were creating a very specific kind of model within MEME, and it is difficult to say whether or not a similar result of the development of mechanistic reasoning may emerge when students engage in different kinds of modeling, such as agent-based simulations (Wilensky and Resnick, 1999) or embodied models (Danish, 2014). Finally, the population of students we worked with, along with the identities of researchers, were fairly homogenous and is likely reflected in the ways in which we interacted with students, the materials we designed, and the models that were created during this implementation. Further work is needed to investigate these findings in more heterogeneous spaces, to see what possibilities students have to contribute as they develop their own mechanistic reasoning in new contexts.
Future directions
These findings contribute to ongoing research by demonstrating the effectiveness of bridging together aspects of scientific modeling and systems thinking concepts to teaching scientific inquiry to elementary students. It highlights the effectiveness of embedding aspects of systems thinking directly into modeling tools and curriculum to support students reasoning around complex systems, particularly in relation to students’ understanding of underlying and emergent relationships within systems. The results of this study support prior research that demonstrated students’ mechanistic reasoning developing on a similar trajectory (Ryan et al., 2021), and extend prior work in analyzing students’ development of mechanistic reasoning (Ruppert et al., 2019).
Overall, across the modeling unit students participated in, it was evident that within their interactions with both peers and facilitators, students developed competencies in their reasoning around the causal mechanisms of complex systems, the epistemic criteria that made up a scientific model, and interpreting data to develop their understanding and represent their claims within their model. What’s more, students’ interactions revealed how intimately connected these aspects of their scientific inquiry were. Their reasoning around mechanisms of the complex system was directly influenced by their interpretations of evidence, which in turn influenced their modeling practices to focus more explicitly on refinement and iteration rather than a single, static representation of the complex system.
Further investigation into how researchers and practitioners can scaffold systems thinking frameworks, such as the PMC framework (Hmelo-Silver et al., 2017b), into modeling tools, curricula, and activities which focus on developing students’ epistemic criteria of models (Pluta et al., 2011), and their grasp of evidence (Duncan et al., 2018), can help to improve the bridge between these two core pieces of scientific inquiry. We continue to work to more developed more nuanced understandings of how our designs mediate students’ developing understanding of both modeling and complex systems, and hope that this study can offer researchers pursuing similar kinds of work design focal points which may further help to bridge these essential processes of scientific inquiry.
Data availability statement
The original contributions presented in the study are included in the article/supplementary material, further inquiries can be directed to the corresponding author.
Ethics statement
The studies involving human participants were reviewed and approved by Indiana University Bloomington Internal Review Board. Written informed consent to participate in this study was provided by the participants’ legal guardian/next of kin.
Author contributions
ZR led the majority of research, analysis, and writing on this project. JD helped substantially with research and revisions to the analysis process. JZ and CS helped substantially with interrater reliability and analysis. DM helped with initial designs of the larger project. JD, RD, CC, and CH-S are Co-PIs on the larger projects. ZR, JD, JZ, CS, DM, RD, CC, and CH-S helped substantially with revisions and feedback to drafts of this manuscript. All authors contributed to the article and approved the submitted version.
Funding
This work was produced through the research project Scaffolding Explanations and Epistemic Development for Systems, which is supported by the National Science Foundation under grant Nos. 1761019 & 1760909.
Conflict of interest
The authors declare that the research was conducted in the absence of any commercial or financial relationships that could be construed as a potential conflict of interest.
Publisher’s note
All claims expressed in this article are solely those of the authors and do not necessarily represent those of their affiliated organizations, or those of the publisher, the editors and the reviewers. Any product that may be evaluated in this article, or claim that may be made by its manufacturer, is not guaranteed or endorsed by the publisher.
Footnotes
1. ^The names here were substituted based on feedback from students in prior iterations of SEEDS. For instance, In earlier implementations, students remarked even after the unit concluded that they were unclear what a mechanism was, but understood it as a process.
References
Assaraf, O. B.-Z., and Orion, N. (2010). System thinking skills at the elementary school level. J. Res. Sci. Teach. The Official Journal of the National Association for Research in Science Teaching, doi: 10.1002/tea.20351
Bakker, A. (2018). Design research in education: a practical guide for early career researchers. London, UK: Routledge.
Barzilai, S., and Zohar, A. (2016). “Epistemic (meta) cognition: ways of thinking about knowledge and knowing” in Handbook of epistemic cognition. eds. J. A. Greene, W. A. Sandoval, and I. Bråten (New York, NY, USA: Routledge), 409–424.
Bolger, M. S., Kobiela, M., Weinberg, P. J., and Lehrer, R. (2012). Children’s mechanistic reasoning. Cogn. Instr. 47, 540–563. doi: 10.1080/07370008.2012.661815
Chi, M. T., Roscoe, R. D., Slotta, J. D., Roy, M., and Chase, C. C. (2012). Misconceived causal explanations for emergent processes. Cogn. Sci. 36, 1–61. doi: 10.1111/j.1551-6709.2011.01207.x
Cobb, P., Confrey, J., Di Sessa, A. A., Lehrer, R., and Schauble, L. (2003). Design experiments in educational research. Educ. Res. 32, 9–13. doi: 10.3102/0013189X032001009
Danish, J. A. (2014). Applying an activity theory lens to designing instruction for learning about the structure, behavior, and function of a honeybee system. J. Learn. Sci. 23, 100–148. doi: 10.1080/10508406.2013.856793
Danish, J. A., Enyedy, N., Saleh, A., and Humburg, M. (2020). Learning in embodied activity framework: A sociocultural framework: A sociocultural framework for embodied cognition. Int. J. Computer-Support Collab Learn. 15, 49–87. doi: 10.1007/s11412-020-09317-3
Danish, J., Vickery, M., Duncan, R., Ryan, Z., Stiso, C., Zhou, J., et al. (2021). Scientific model evaluation during a gallery walk. In E. VriesDe, Y. Hod, and J. Ahn (Eds.), Proceedings of the 15th International conference of the learning sciences-ICLS 2021. (pp. 1077–1078). Bochum, Germany: International Society of the Learning Sciences.
Duncan, R. G. (2007). The role of domain-specific knowledge in generative reasoning about complicated multileveled phenomena. Cogn. Instr. 25, 271–336. doi: 10.1080/07370000701632355
Duncan, R. G., Chinn, C. A., and Barzilai, S. (2018). Grasp of evidence: problematizing and expanding the next generation science standards’ conceptualization of evidence. J. Res. Sci. Teach. 55, 907–937. doi: 10.1002/tea.21468
Eberbach, C., Hmelo-Silver, C. E., Jordan, R., Taylor, J., and Hunter, R. (2021). Multidimensional trajectories for understanding ecosystems. Sci. Educ. 105, 521–540. doi: 10.1002/sce.21613
Engeström, Y. (2001). Expansive learning at work: toward an activity theoretical reconceptualization. J. Educ. Work. 14, 133–156. doi: 10.1080/13639080020028747
Ford, M. (2008). Grasp of practice as a reasoning resource for inquiry and nature of science understanding. Sci. & Educ., 17. 147–177, doi: 10.1007/s11191-006-9045-7 1573-1901.
Goldstone, R. L., and Wilensky, U. (2008). Promoting transfer by grounding complex systems principles. J. Learn. Sci. 17, 465–516. doi: 10.1080/10508400802394898
Gutierrez, K., Rymes, B., and Larson, J. (1995). Script, counterscript, and underlife in the classroom: James Brown versus Brown v. Board of Education. Harv. Educ. Rev. 65, 445–472.
Hmelo-Silver, C. E., and Azevedo, R. (2006). Understanding complex systems: some core challenges. J. Learn. Sci. 15, 53–62. doi: 10.1207/s15327809jls1501_7
Hmelo-Silver, C. E., Jordan, R., Sinha, S., Yu, Y., and Eberbach, C. (2017a). “PMC-2E: conceptual representations to promote transfer” in E. Manalo, Y. Uesaka, and C. Chinn (Eds). Promoting spontaneous use of learning and reasoning strategies (London, UK: Routledge), 276–291.
Hmelo-Silver, C. E., Jordan, R., Eberbach, C., and Sinha, S. (2017b). Systems learning with a conceptual representation: a quasi-experimental study. Instr. Sci. 45, 53–72. doi: 10.1007/s11251-016-9392-y
Hmelo-Silver, C. E., Marathe, S., and Liu, L. (2007). Fish swim, rocks sit, and lungs breathe: expert-novice understanding of complex systems. J. Learn. Sci. 16, 307–331. doi: 10.1080/10508400701413401
Jacobson, M. J., and Wilensky, U. (2006). Complex systems in education: scientific and educational importance and implications for the learning sciences. J. Learn. Sci. 15, 11–34. doi: 10.1207/s15327809jls1501_4
Jordan, B., and Henderson, A. (1995). Interaction analysis: foundations and practice. J. Learn. Sci. 4, 39–103. doi: 10.1207/s15327809jls0401_2
Kuhn, T. S. (1977). The essential tension: selected studies in scientific tradition and change. Chicago, Illinois, USA: University of Chicago Press.
Lehrer, R., and Schauble, L. (2005). “Developing modeling and argument in the elementary grades” in Understanding Mathemat: Cs and science matters. eds. T. A. Romberg, T. P. Carpenter, and F. Dremock (New Jersey: Lawrence Erlbaum), 29–53.
Lehrer, R., and Schauble, L. (2006). “Cultivating model-based reasoning in science education” in The Cambridge handbook of the learning sciences. ed. R. K. Sawyer (New York, NY, USA: Cambridge University Press), 371–387.
Machamer, P., Darden, L., and Craver, C. F. (2000). Thinking about mechanisms. Philos. Sci. 67, 1–25. doi: 10.1086/392759
Mathayas, N., Brown, D. E., Wallon, R. C., and Lindgren, R. (2019). Representational gesturing as an epistemic tool for the development of mechanistic explanatory models. Sci. Educ. 103, 1047–1079. doi: 10.1002/sce.21516
McDonald, N., Schoenebeck, S., and Forte, A. (2019). “Reliability and inter-rater reliability in qualitative research: norms and guidelines for CSCW and HCI practice” in Proceedings of the ACM on human-computer interaction, vol. 3 CSCW), 1–23.
Moreland, M., Vickery, M., Ryan, Z., Murphy, D., Av-Shalom, N., Hmelo-Silver, C. E., et al. (2020). “Representing modeling relationships in systems: student use of arrows” in The Interdisciplinarity of the learning sciences, 14th International conference of the learning sciences (ICLS) 2020. eds. M. Gresalfi and I. S. Horn, vol. 3 (Nashville, Tennessee: International Society of the Learning Sciences), 1773–1774.
Murphy, D., Duncan, R. G., Chinn, C., Danish, J., Hmelo-Silver, C., Ryan, Z., et al. (2021). Students’ justifications for epistemic criteria for good scientific models. In E. VriesDe, J. Ahn, and Y. Hod (Eds.), 15th International conference of the learning sciences – ICLS 2021 (pp. 203–210). Bochum, Germany: International Society of the Learning Sciences
National Research Council. (2012). A framework for K-12 science education: practices, crosscutting concepts, and core ideas. Washington, DC: The National Academies Press.
NGSS Lead States. (2013). Next generation science standards: for states, by states. Washington, DC: National Academies Press.
Pierson, A. E., Clark, D. B., and Sherard, M. K. (2017). Learning progressions in context: tensions and insights from a semester-long middle school modeling curriculum. Sci. Educ. 101, 1061–1088. doi: 10.1002/sce.21314
Pluta, W. J., Chinn, C. A., and Duncan, R. G. (2011). Learners' epistemic criteria for good scientific models. J. Res. Sci. Teach. 48, 486–511. doi: 10.1002/tea.20415
Quintana, C., Reiser, B. J., Davis, E. A., Krajcik, J., Fretz, E., Duncan, R. G., et al. (2004). A scaffolding design framework for software to support science inquiry. J. Learn. Sci. 13, 337–386. doi: 10.1207/s15327809jls1303_4
Ruppert, J., Duncan, R. G., and Chinn, C. A. (2019). Disentangling the role of domain-specific knowledge in student modeling. Res. Sci. Educ. 49, 921–948. doi: 10.1007/s11165-017-9656-9
Russ, R. S., Scherr, R. E., Hammer, D., and Mikeska, J. (2008). Recognizing mechanistic reasoning in student scientific inquiry: a framework for discourse analysis developed from philosophy of science. Sci. Educ. 92, 499–525. doi: 10.1002/sce.20264
Ryan, Z., Danish, J., and Hmelo-Silver, C. E. (2021). Understanding students’ representations of mechanism through modeling complex aquatic ecosystems. In E. VriesDe, Y. Hod, and J. Ahn (Eds.), Proceedings of the 15th International conference of the learning sciences – ICLS 2021. (pp. 601–604). Bochum, Germany: International Society of the Learning Sciences.
Safayeni, F., Derbentseva, N., and Cañas, A. J. (2005). A theoretical note on concepts and the need for cyclic concept maps. J. Res. Sci. Teach. 42, 741–766. doi: 10.1002/tea.20074
Saleh, A., Hmelo-Silver, C. E., Glazewski, K. D., Mott, B., Chen, Y., Rowe, J. P., et al. (2019). Collaborative inquiry play: a design case to frame integration of collaborative problem solving with story-centric games. Inf. Learn. Sci. 120, 547–566. doi: 10.1108/ILS-03-2019-0024
Sandoval, W. A. (2004). Developing learning theory by refining conjectures embodied in educational designs. Educ. Psychol. 39, 213–223. doi: 10.1207/s15326985ep3904_3
Sandoval, W. A. (2014). Conjecture mapping: an approach to systematic educational design research. J. Learn. Sci. 23, 18–36. doi: 10.1080/10508406.2013.778204
Schwarz, C. V., Reiser, B. J., Davis, E. A., Kenyon, L., Achér, A., Fortus, D., et al. (2009). Developing a learning progression for scientific modeling: making scientific modeling accessible and meaningful for learners. J. Res. Sci. Teach. 46, 632–654. doi: 10.1002/tea.20311
Schwarz, C. V., and White, B. Y. (2005). Metamodeling knowledge: developing students’ understanding of scientific modeling. Cogn. Instr. 23, 165–205. doi: 10.1207/s1532690xci2302_1
Stroup, W. M., and Wilensky, U. (2014). On the embedded complementarity of agent-based and aggregate reasoning in students’ developing understanding of dynamic systems. Technol. Knowl. Learn. 19, 19–52. doi: 10.1007/s10758-014-9218-4
Vygotsky, L. S. (1978). Mind in society: development of higher psychological processes. Cambridge, Massachusetts, USA: Harvard university press.
Wertsch, J. V. (2017). “Mediated action” in A companion to cognitive science. eds. W. Bechtel and G. Graham (Hoboken, New Jersey, USA: Blackwell Publishing Ltd), 518–525. doi: 10.1002/9781405164535.ch40
Wilensky, U., and Resnick, M. (1999). Thinking in levels: a dynamic systems approach to making sense of the world. J. Sci. Educ. Technol. 8, 3–19. doi: 10.1023/A:1009421303064
Keywords: science education, modeling, complex systems, elementary education, design-based research (DBR)
Citation: Ryan Z, Danish J, Zhou J, Stiso C, Murphy D, Duncan R, Chinn C and Hmelo-Silver CE (2023) Investigating students’ development of mechanistic reasoning in modeling complex aquatic ecosystems. Front. Educ. 8:1159558. doi: 10.3389/feduc.2023.1159558
Edited by:
Laura Zangori, University of Missouri, United StatesReviewed by:
Sarah Digan, Australian Catholic University, AustraliaChristina Krist, University of Illinois at Urbana-Champaign, United States
Jaime Sabel, University of Memphis, United States
Copyright © 2023 Ryan, Danish, Zhou, Stiso, Murphy, Duncan, Chinn and Hmelo-Silver. This is an open-access article distributed under the terms of the Creative Commons Attribution License (CC BY). The use, distribution or reproduction in other forums is permitted, provided the original author(s) and the copyright owner(s) are credited and that the original publication in this journal is cited, in accordance with accepted academic practice. No use, distribution or reproduction is permitted which does not comply with these terms.
*Correspondence: Zach Ryan, enJ5YW5AaXUuZWR1