- 1Escuela de Ingeniería y Ciencias, Tecnológico de Monterrey, Mexico City, Mexico
- 2Vicerrectoría de Investigación y Transferencia de Tecnología, Tecnológico de Monterrey, Monterrey, Mexico
Higher education is a multivariable system by nature; thus, it is a complex task to maintain consistent academic success for students. This is a key factor to understand the positive and negative effects generated by the COVID-19 lockdown, particularly during the current stage of the “New Normal” period. The research presented herein considers a set of variables corresponding to students and faculty as causal factors to track, analyze, and assess the impact on the academic performance of engineering students in an urban Mexican university in both periods: online teaching during lockdown, and returning to face-to-face learning during the “New Normal.” Through a hybrid survey, looking for representative learning styles, academic personality traits, and technology competencies, academic performance in both periods has been recorded along with each student's learning preference. The suggested analysis model sought correlations in the stated causal factors to find valuable behavioral patterns. The outcomes show that good students in both models have attained a high level of adaptation and feel competitive in them. On the contrary, students with lower adaptation have shown poor academic performance in both models, but they perceived the online model as the less effective learning environment. Particularly, personality traits appoint on a notable impact on performance. In addition, learning styles are not significant. Still, it has been suggested this situation could be due to a greater diversity of teaching approaches established by the faculty to take care of student performance.
1. Introduction
Education is one of the leading human activities involving a large proportion of the world population. It suddenly halted due to an unprecedented global pandemic, exposing a vital delicate ecosystem in our contemporary society. Developing countries went through a challenging process to adopt technologies and models of online Learning, still uncommon in the contemporary education of those countries. Once adopted, many problems had to be resolved at an institutional level. It culminated in the most plausible scenario for each country and institution. The next level was the situation of each student. Students were immersed in imposed models, for which many were unprepared and remained outside their educational expectations. Several critical aspects emerged as lockdowns continued.
Underneath the generic reality of online education were many pending tasks not considered in traditional education. Even before the confinement and online education, aspects still not wholly addressed in education included accessibility to resources, appropriate learning environments, availability of services, disposition of learning time, and previous academic background. Those aspects were unavoidably intensified during the confinement despite the accompaniment and massive teaching techniques implemented. For many, if not most, students, the pandemic will mark education and generations for many years. Some students abandoned or truncated their studies due to the lack of inclusion in education during the period. Others suffered from organizational deficiencies in learning forever (UNESCO, 2020a). Higher education had several disruptions and obstacles to overcome during the COVID-19 pandemic. They did not appear uniformly in all countries, universities, or programs, nor were they the same for all students and learning areas, an unsustainable situation for education. Profoundly disrupted by the global emergency, education had lessons to learn through the worldwide decision to adopt the online approach and barely overcame the crisis.
This paper analyzes different dimensions during the COVID-19 educative scenario. In a general framework, other studies have sought to identify elements that markedly influence the learning process (Peltonen and Ruohotie, 1992). It was sought to contextualize the analysis in the confinement period and the educational transitions. For example, much more educational technology was developed during the pandemic than in any other period. Students and teachers' technological competencies were standardized as never before, notably accelerating the digital transformation in education.
The motivation for this report arose from analyzing the academic transition to the “New Normal” period, particularly comparing online and face-to-face education, based on possible causal factors of learning styles, academic personality, and previously acquired skills. In this work, an insight analysis limited to first-semester engineering students enrolled in the Physics course (Delgado, 2022) has been extended. It is not centered on a specific course but a broad random sample of engineering students in our university (25%) covering all engineering programs, semesters, and educational plans. Section 2 of this report presents the theoretical context of the population of students (several personality traits, learning styles, previous skills, and university orientation, possibly impacting their university success, particularly during the COVID-19 pandemic). The third section establishes the research objectives, methods, sample, and instrument used to collect data. The fourth section presents the results summarized in graphical representations, with direct comparisons showing some differentiation. Section 5 discusses some notable previous outcomes, introducing more specific and hidden information teachers provide concerning the facts and putting them in proper context. The last section states the conclusions related to the research objectives.
2. Background
The COVID-19 confinement moved education into the unexplored terrain of fully online education. For a contemporary period, the event involved teachers and educational practitioners in a deep discussion of the theoretical framework of education based on technology, causing mandatory updating of their competencies. Nevertheless, it also exposed a new aspect to be attended: the non-reviewed knowledge (theoretical and practical) by many teachers about online education. Such tasks should have been undertaken before the pandemic; unfortunately, the crisis caught un-updated educators with their hands in the cookie jar.
2.1. Context of educative transitions imposed by the COVID-19 pandemic
Online education implies basic considerations such as the affordability of a device to receive the classes, lessons, and activities, and complex ones like the diversity of family characteristics, spaces, and services to have the adequate minimal environment for it, which the school had provided in normal times. In addition, multiple concurrent problems impacted each family (siblings studying and parents working at home), sometimes with COVID-19 or deaths in the family.
The return to the “New Normal” was as complex and multi-factorial as the pandemic. It left a series of unattended tasks in addition to those existing before the confinement period. In Mexico, the 2 years developed a diverse spectrum of situations, both in health and social, political, and behavioral scenarios (Vázquez-Sánchez et al., 2021). Dismantling the entire national, institutional, family, and personal education assembly during the lockdown implied a less agile return to schools (Cárdenas et al., 2022). Regrettably, the lessons learned and sustained by the health emergency were not assimilated, vanishing into the same practices before the pandemic. Had this critical stage we lived in no teaching for us? As a result of a lack of sustainable vision in education, it is not yet possible to measure the long-term social impact of the pandemic's educational disruption on the global, national, and personal scenarios (De la Riva et al., 2015).
The schools' closures were announced in Mexico on March 16, 2020. Plagued by uncertain dates to set academic continuity, all educative levels began a forced migration to digital education models. The transition began from primary schools to public and private universities (OECD, 2020). The experience was worse and more delayed for primary levels and public education, commonly without experience in using such technologies. Private higher education schools began as soon as 1 week after the closure, like this institution where the current research was performed, mainly because it has offered teacher training programs in educational technologies for more than 20 years (Delgado et al., 2012). Also, collaboration among universities became valuable (ITESM, 2020) to accelerate the transition to effective online models. However, public higher education institutions were still delayed for months in preparing their online educative models (Vieyra et al., 2020).
Recently, a more expansive interpretation of the sustainability of Higher Education has been extended toward the inclusivity provided by universities (Goldbach et al., 2022), referring to the overall actions and initiatives established to attract and retain students (Fien, 2022). Such initiatives have been explored: well-being, technical support for teachers to improve their online teaching quality, training programs for teachers on hybrid education and learning technologies, flexible policies for students, etc. Identifying causal issues of academic success in Higher Education takes us into terrains related to inclusivity. Teaching strategies have superseded traditional education based on lectures. Those aspects were important for Higher Education in the pre-pandemic era, but during the online education imposed by the COVID-19 confinements, they became mandatory, contributing to developing a broader vision of university sustainability (Clugston and Calde, 2000).
The current analysis occurred at a private university system in Mexico in 2022 during the transition to the “New Normal” (the first semester of 2022). The research was implemented at one campus in the Mexico City area. Hard-engineering students (Mechanical, Electrical, Electronics, Mechatronics, and Computer Sciences) were considered to gain insights about the educative pandemic transitions affecting them, particularly their academic performance.
2.2. Background of education theories for the current research
The domain of education theories was central to pandemic challenges. The absence of fundamental distinctions in education theories regarding how learning behaviors and interests have shaped educative practices resulted in a lack of guidelines to develop online teaching models, particularly in Higher Education, where commonly there is no professional training for teachers in this modality. This indicated the lack of sustainability due to an unstable equilibrium of customs and traditions and little variability in the teaching environment.
Many aspects of online education are lacking in daily teaching in university communities, so distinctions and guidelines to establish a roadmap for effective online teaching were also missing (Tennyson and Rasch, 1998). This situation had to change quickly at the beginning of the pandemic, as online teaching became mandatory, involving most of the faculty. Theoretical approaches to the origin and motivation of Learning became useful in understanding how to maintain student engagement in education under those critical circumstances.
Several education theories have proposed motivational elements for successful Learning in their approaches. For instance, Cognitive Learning Theory (CGLT) states that learning practices should be designed to align with the learner's thinking style (Collins and Stevens, 1983). CGLT has been the traditional and most disseminated approach in modern education practices. Constructivist Learning Theory (CNLT) assumes that knowledge is constructed by steps building on previous knowledge and adding experiences (Narayan et al., 2013). In a more practical and external approach, Behavioral Learning Theory (BLT) suggests that learners act based on their interactions with their environment and community (Watson, 1966); thus, their Learning is based on interests and necessities. From a contemporary point of view, Connective Learning Theory (CCLT) states that learners are moved to learn through the overall connections in their lives, linking the past, needs, people, and duties (Siemens, 2005).
Thus, those theoretical approaches provide several considerations to be included as possible causal factors of Higher Education success. Aspects such as learning styles involve considerations of CGLT, together with some faculty actions for a broader representation and inclusion of teaching methodologies in the constructivist domain, commonly introduced as a part of institutional training for teachers. Alternatively, some academic personality traits are identified as possible success factors under a behavioral approach in BLT. Finally, elements related to educative technology regard a connectivist approach to Learning success, as CCLT states. Figure 1 shows the contextual ecosystem underlying the conceptual research for the current report, centered on the student's perception of their academic performance through the COVID-19 educative stages. The gray arrows represent primary relations and interdependence. While the university scaffolds each student through its structure and profile (teaching strategies and teaching environment), students' academic traits (Learning styles, academic personality) may create limitations. In addition, the external environment also feeds the learning process. Those relations mean potential academic success becomes different for each student in terms of adaptability and inclusivity. In such a structure, the sudden educative scenario imposed by the COVID-19 confinements impacted the students' outcomes through its different stages. In the following subsection, some causal facts as they are afforded in contemporary literature are addressed.
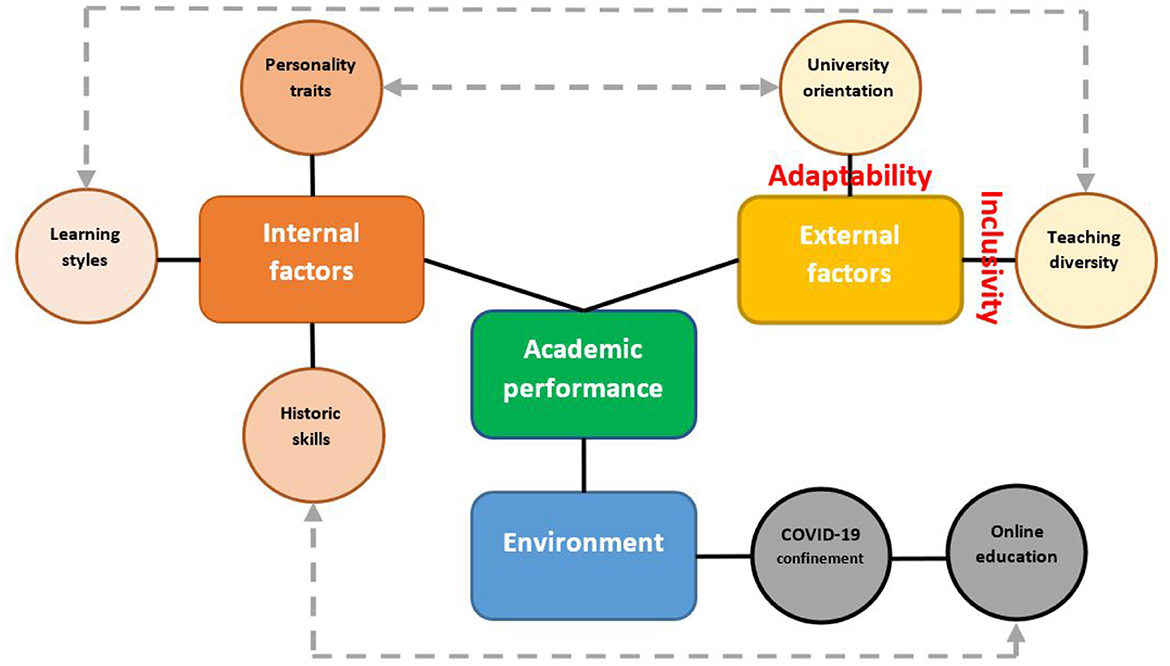
Figure 1. Contextual diagram for the analysis of academic performance. Individual traits (learning styles, academic personality, and skills) have been shaped by the historical background of each student and then scaffolded by the university profile (orientation and teaching diversity), sustaining potential academic success. The environment dampens the last interaction: online education during the COVID-19 confinement (resources, services, learning spaces, teaching, etc.). The gray arrows indicate primary relations in the ecosystem.
2.3. Academic causal factors inherent to students
This subsection addresses some internal student aspects considered in the research, such as their academic history, learning preferences, and personality traits influencing academic performance.
2.3.1. Learning styles as an academic success factor
The introduction to the learning styles concept, despite being criticized (see the further discussion on this issue), particularly the scale introduced by Kolb (1976) categorizing the student learning paths (divergers, assimilators, convergers, and accommodators), established that at least under certain circumstances and topics, students can organize their paths to receive information more efficiently (Cagiltay, 2008). Kolb's experiential learning cycle is a four-stage process depicting how we acquire and embed new knowledge. The teaching and evaluation designs should incorporate a broad spectrum of approaches to these categories, representatively collecting the outcomes for each type of student instead of only addressing some of them.
Subsequently, those categories were reformulated into four bipolar dimensions classifying the Learning that each student could be receiving: sensitive/intuitive (external/internal or also concrete/abstract), visual/auditory (visual/verbal), active/reflective (active/passive), and sequential/global (Felder and Silverman, 1988), and sometimes adding a fifth: deductive/inductive. Names differ because they could refer to teaching instead of learning. It is doubtful these approaches have been considered or represented even approximately throughout online lessons. Notably, the lack of higher-education teachers' specialization in those considerations and the lack of interaction did not promote the realistic perception of those needs in each student.
Each learning design delivered online showed characteristics of each instructor's conceptions of their teaching, sometimes more focused on some learning styles, only being able to privilege certain types. This affected the primordial construction of texts, images, demonstrations, and discussions based on facts, abstractions, concepts, or theory. The organization within a learning sequence and its relationship to the everyday world are equally important. In this study, Felder's dimensions (Felder and Silverman, 1988) were considered; although not the most modern, they are the most used in the literature for comparison; thus, we could categorize the study participants. Information was collected using some traditional methods described in the following sections (García-Cué et al., 2009).
The concept of Learning Styles, despite its popularity, has been recently questioned due to the mismatch between didactic practices and academic outcomes (Gleichgerrcht et al., 2015; Aslaksen and Lorås, 2018; Nancekivell et al., 2020). Although not universally accepted and considered partially inaccurate (Furey, 2020), this concept, when carefully interpreted, still provides valuable tools to close the gaps between interaction among learners, teachers, and educational content (Willingham et al., 2015). Rather than defining different ways to learn (Felder, 2020).
2.3.2. Academic personality as a factor of higher education success
Personality traits are assumed to predispose the limiting or favoring of learning in specific disciplines. Many universities bet on identifying these personality traits because they can develop a better competitive differentiation in their students, a notable effort of sustainability in education. When some traits combine with stressful emergencies, such as the COVID-19 pandemic, they can interfere with students' Learning (Balkhi et al., 2020). Literature has widely established these facts unveiling the behaviors expressed in the pandemic confinement, particularly at the beginning. The crisis established abrupt changes in students' daily routines and learning performance (Nixon et al., 2021).
Statistical relationships between student personality traits and outcomes could be matched by moving students in the right direction toward their academic achievement via the cycle of generating student satisfaction/improving their performance (Pawlowska et al., 2014). Then, the teacher's knowledge about academic traits becomes vital for a better understanding of how students progress in each course, particularly to meet expectations (Pike, 2006). In this terrain, the University Personality Traits Scale (UBPS) comprises six dimensions (Rauschnabel et al., 2016), while the Myers-Briggs Type Indicator (MBTI) marks between four to sixteen personality types (Jessee et al., 2006). Despite criticisms (see the further discussion on the issue), the latter states an extensive, classical, and broad classification of personality traits. Analysis was established based on this scale.
MBTI has been criticized for its lack of reliability (Stein and Swan, 2019) and usability for decision-making (Bailey et al., 2018). Despite this, MBTI is still valuable to track some behavioral traits correlated with academic performance (Sipps et al., 2016). It becomes concomitant in specific learning contexts (Harvey, 1996) and it is also concurrent in personality traits (Sipps et al., 2016). MBTI is generally considered to be reliable for academic purposes (Randall et al., 2017).
The current research aims to correlate Learning styles and Personality traits with institutional initiatives based on methodologies and different approaches for delivering academic content. Both, Learning styles and Personality traits describe different stimuli perceived and shown by students. The surveyed student population can be characterized and correlated with successful tendencies amid learning activities.
2.4. Academic success associated with technological competencies previously acquired: the hidden curriculum
Universities had to migrate to diverse instructional formats to mitigate the COVID-19 lockdown effects (Stewart et al., 2021). This large-scale implementation impacted students' grades and Learning, the familiarization with educational technology by students and teachers, and the aspects favoring completing each course. The impact on Learning and well-being from confinement and online education has not been adequately quantified. It will take years to analyze its effects: low level of attendance and Learning, decreased number of skills learned, delayed advancement in school levels due to failure or dropout, and others (Dorn et al., 2021). Additionally, after the confinement, old pending challenges in education intensified their negative effects, but it is also true that a more uniform command of technology among teachers was gained (Rapanta et al., 2021). The correlation between technological mastery and effective Learning must be quantified, as the factors affecting the mastery, and the skills acquired. It is also essential to analyze the relationships between previous technological competencies and academic success during online teaching (Al-Qasemi et al., 2022). Teachers' digital competencies and overall institutional mastery played a significant role in integrating technology during the pandemic and post-pandemic periods (Akram et al., 2021).
In Higher Education, this impact will become crucial in the future, not only professionally but also personally and socially (Rodrigues et al., 2021). We can distinguish basic competencies using the Digital Economy and Society Index (DESI). Also, the Human Capital Indicator (HCI) indicates the population level using those services. In the previous study (Rodrigues et al., 2021), based on the European Digital Competence Framework for Citizens (EDCFC) (King et al., 2004; Carretero et al., 2017), five areas of competency are defined: (1) domain competency over information and data search (ID), (2) communication and collaboration (CC), (3) creation of digital content (CD), (4) computer security (CS), and (5) problem-solving (TS).
Based on a self-diagnosis of training difficulties, workload, and negative emotions, some studies have established categorizations of digital competencies (Portillo et al., 2020), the technological integration in education, and the ability to update it (Akram et al., 2021). However, discipline and perseverance are also required in the online teaching model to learn effectively (Autio, 2011). Thus, during the COVID-19 lockdown, online education surprised teachers and students, not in the obvious aspects of internet access or functional services, but in the adequate training necessary to manage online Learning and the practical daily actions to sustain it (Akram et al., 2021).
Today, with more than 2 years of confinement behind us, we treasure learning about the relationship between education and technology. But it is worrying that students and teachers are just looking forward to returning to pre-pandemic academic daily life. We are not exempt, even in the medium term, from facing another crisis forcing us to adopt distance education models entirely or partially (Perrow, 2013). It is crucial to develop assessment instruments reflecting the impact of mastery of educational technology and their measurable impact on Learning (Akram et al., 2021). In this study, based on the anchor vignettes approach (King et al., 2004), a self-perception evaluation to classify the degree of mastery and usefulness of the applied technology was established. The anchor vignettes have been defined in each of the five competency areas by EDCFC mentioned above (Carretero et al., 2017). Information was collected on the type of software used, its purpose, and usage frequency.
2.5. Teaching strategies of the faculty to promote inclusivity
Teaching strategies are connected with each student's learning styles, but they also facilitate creative or meaningful ways to deliver the content, looking for more vivid Learning and helping deliver content in a more egalitarian way (UNESCO, 2020a). The impact of those strategies may not be observed immediately but over the years on each student's academic trajectory. During COVID-19 confinement, schools were not prepared beyond more than the immediate primary technology: videoconferencing. This limited teachers in reproducing basic instruction techniques (Zapata-Garibay et al., 2021). It initially depended on the support offered by the university to teachers at the beginning of confinement, the culture and institutional practices, the state of the art in the university operations, and the prevailing culture, methods, and daily operations. In this vacuum, teachers and students learned to self-regulate, learn, and adopt metacognitive strategies for teaching and Learning (Boström et al., 2021).
Detailed inventories of didactic approaches were constructed and tested in different fields, disciplines, and situations over decades because teaching diversity became relevant in pedagogical studies (Chamberland et al., 1995). Active learning techniques, competency-based teaching, and other straightforward didactic techniques are occasionally combined now in Higher Education with lecture-based classes. They are part of school assessments in Higher Education, retrieved from several authors for the current evaluation (Wieman and Gilbert, 2017).
2.6. Behavioral considerations from the COVID-19 lockdown
The COVID-19 lockdown induced several behavioral aspects not always documented by the educative experiences: family coexistence, crowded spaces, critical events such as sickness or deaths in the nuclear family, loneliness, and other emotional disruptions. They appeared in academic communities with null or only partial support from the schools. As soon as lockdowns began, social Learning was one of the most impacted, affecting the ability of people to share and mutually enrich their knowledge (Delgado, 2021b), but it recovered as a positive response to the crisis (Delgado, 2021a). Other factors conditioning learning performance were diminished capacity to successfully exercise digital competencies due to the stress of transitioning to online education (Toto and Limone, 2021; Vergara-Rodríguez et al., 2022), stress depending on cultural and behavioral factors (Boneh et al., 2022), and stress due to the level of digitalization, technology, and innovation in the environment (Antón-Sancho et al., 2022). In the current research, those aspects were collected as a global perception of students' academic performance during the transition to the “New Normal.” They affected each student differently based on their attitudinal background evaluated by the personality traits. Some characteristics like introversion or shyness could affect academic performance during the lockdowns and reintegration into the school during the “New Normal.”
Changes produced by the COVID-19 pandemic, and the “New Normal,” are positive and negative. Although, for a couple of years, some students missed the possibility to access education, there has been advancement in educative technologies and in their effectiveness (Cáceres et al., 2021; Delgado et al., 2021). These aspects have been differentiated by some students' characteristics, and also, as a function of the technological literacy of students, teachers, and schools, as well as the technological culture within such schools and actions to improve the quality and effectiveness of online learning (Corell-Almuzara et al., 2021; Delgado, 2021b). Still, this culture (in case it becomes widespread) becomes useful and effective for certain groups of students as a function of their personality (Rodicio-García et al., 2020; Delgado, 2021a; Jung et al., 2021). Records on the quality of learning and the improvement or deterioration in the quality of education show a diverse perspective. While some studies have appointed to deterioration with irretrievable consequences (McLure et al., 2021; Sharma and Alvi, 2021), others, less skeptical, remark that many students reported learning satisfaction during the lockdown in terms of academic discipline, which stated students' future expectations of learning (Bashir et al., 2021; Kaqinari et al., 2022). In a more specific and detailed analysis, outcomes appoint to diversified effects in education as a function of other aspects related to students, teachers, and institutions (Goudeau et al., 2021; Hendriksen et al., 2021). In the current research, a diverse effect was found, considering students' personalities, learning and teaching styles, as well as technological culture.
2.7. Research questions and objectives
Success factors in Higher Education are inherent knowledge to both students and institutions. In the outer and immediate sphere, success refers to the various actions of an institution's teaching. It is natural to ask what the impact was on academic success due to the transition to online education and returning to school more than 2 years later. A comprehensive analysis was performed on our current engineering students, addressing the following questions:
a) Which personality traits, learning styles, and previous hidden curricula during the pandemic can be characterized by the entire student engineering population?
b) How have those traits, styles, and skills impacted their academic performance through the pandemic?
c) Considering the aforementioned aspects, is it possible to define a measure of inclusivity and adaptation from our students that explains the different impacts on individual academic performance?
Thus, for the present study, the following four specific research objectives were set:
a) To establish a demographic characterization of the representative sample of students considering learning styles, personality, and underlying technological skills.
b) To analyze the agreement between previous characterization groups relative to the perceived characteristics of training provided by the institution.
c) To analyze the correlation between the previous characterization groups relative to examples of successful adaptation to online education during the COVID-19 lockdown.
d) To analyze the correlation between the previous characterization groups relative to the success and adaptation of face-to-face education under the “New Normal.”
In the next section procedures, materials, and type of research followed to reach the mentioned research objectives are detailed.
3. Materials and methods
The main interest in the current report is to analyze causal factors stating differences in the performance patterns as online and face-to-face approaches introduced them during the stages of the COVID-19 lockdown. They were sought corresponding to some learning and academic characteristics considered in Education literature. There was a particular interest in engineering programs. The interest in online education during the period was central but understood through the educational transitions, first from face-to-face to online, then from online to face-to-face again. Students' adaptation and the natural scaffolding provided by the university are other issues to be analyzed as interpretative causal factors. In this section, a description of the research plan developed in the report is performed. First, a depiction of the sample population and its demographic and academic characteristics is stated. Next, a depiction of the instrument and sampling method applied to gather information is included.
3.1. Demographic target: population and sample
As previously stated, this research was conducted at one private nationwide university system campus. Located in the Mexico City area, the students are in an urban region with well-developed services. The study centered on engineering students in the upper middle class with an ample base of scholarships. The population under study corresponded to undergraduate engineering students in Mechanics, Electronics, and Computer Sciences programs. Students randomly surveyed covered all semesters in any of those programs. The sample responded to an instrument depicted below.
Researchers were interested mainly in the proportions of non-parametric tests. Sample sizes above 15% of recommended respondents for parametric tests estimating proportions were used (Cohen, 1988; Lehmann, 2006). Discrimination scales of at least six points were used to locate absolute confidence around δ = 0.25 and standard deviations not higher than s≈1.5. Assuming a 95% (α = 0.1) desired confidence implied minimum sample sizes of (considering the extreme case of large populations). The sample group was selected randomly from the entire population of 607 students (not groups by semesters or specific programs were performed in the sampling). A sample group representing 30% of the total was chosen. Finally, approximately only 25% of the population came back with a filled questionnaire. The final sample group was composed of 31 women (20%) and 122 men (80%) in the second to ninth semesters of their programs (the first semester was not represented during the semester of application because enrollment is performed yearly in August). For gender analysis, the recommended sample size was at least 15 elements by group (Lehmann, 2006).
In 2019, our institution's educational model transitioned to a new Tec21 model. Its semesters are divided into three 5-week sub-periods, and each course presents a semester challenge for the students to solve related to the course contents. The former, more traditional educative model was called the Tec20 model. Thus, 45 of participating students fell under the previous Tec20 model, and 108 came under the new Tec21 plan. Of these, 74 students were enrolled in the university before the pandemic, and 79 did so during the confinement; therefore, they received remote classes coming from High School. Table 1 shows detailed demographic statistics and the mode of some key responses related to academic issues during COVID-19. All Tec20 students were enrolled in the university before the pandemic (the Tec21 model began in Fall 2019). When comparing the number of men and women enrolled in the university before and during the pandemic, the percentage of men increased by 2%, increasing from 79 to 81% (consequently, women decreased from 21% to 19%), which is not meaningful, but it shows that the gender composition of the population was little affected.
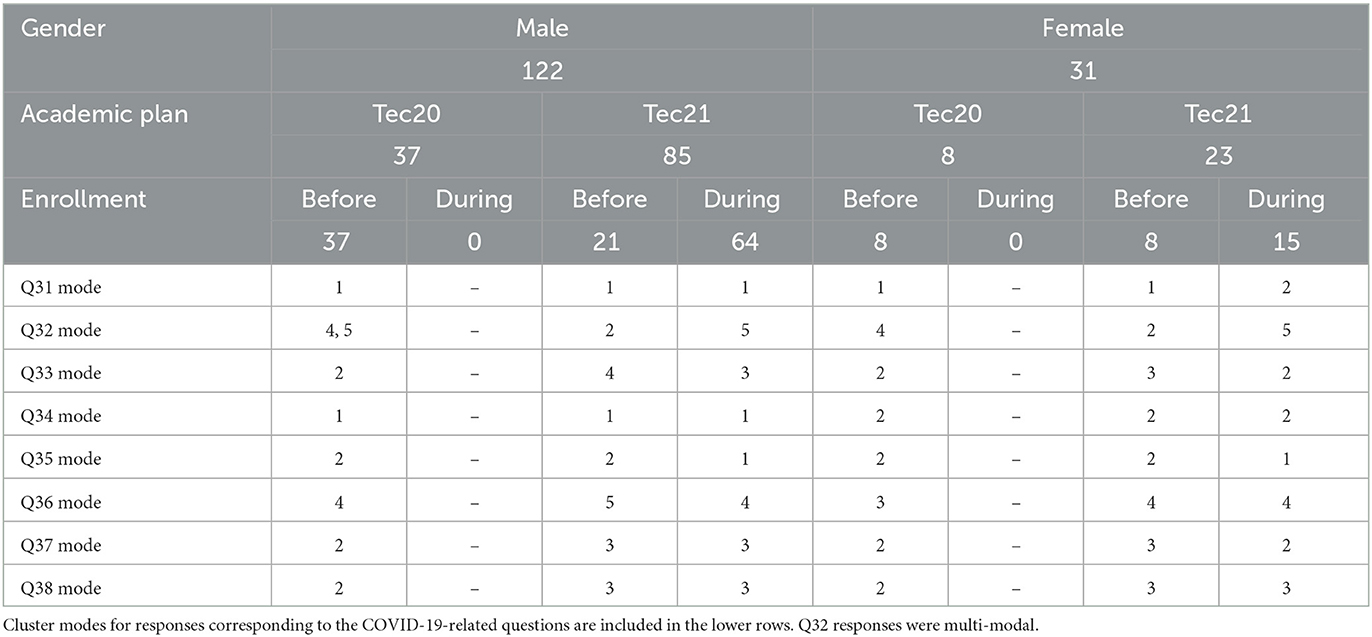
Table 1. Clustering of students in the research considering gender, academic plan, and enrollment plan.
3.2. Methods
3.2.1. Questionnaire
The general research objective was to analyze how behavioral, environmental, and academic factors conditioned educational transitions during the COVID-19 pandemic. To achieve this goal, the tools mentioned in Section 2 were combined independently to collect information about learning styles and personality traits declared by each participant. Also, each student's perception of the university teaching orientation was collected. The technological skills of each student are part of their hidden curriculum, so a skills self-evaluation was compiled, together with their perception of their teachers' mastery of technology. A specific question included the self-perception about the current educational context and its relevance to their careers during the period they were surveyed. The final sections gathered their self-perception of academic performance and adaptation during confinement and subsequently during the “New Normal” (corresponding to the semester in which this instrument was applied: summer 2022). Students were also asked about the online learning received during confinement regarding the value and their preference between online and face-to-face instruction. Information was also collected if each student experienced the transition from high school to university during the confinement. The instrument was previously used and reported as an insight into a limited group of students enrolled in the introductory Physics course (Delgado, 2022) (see the Supplementary material as reference). Each question was identified with a Code label with format Qx, x being the response being gathered. Each question gathered the answer on a number scale indicated in each response section. Questions Q31 through Q38 corresponded to academics and the COVID-19 facts. Table 1 shows the statistical mode for some relevant questions and each cluster of students synthetically. Note that question Q32 for males in the Tec20 model was multi-modal.
In agreement with the first research objective, the demographic correlations collected were analyzed. Then, per the second objective, the causal impact was observed on the performance and adaptation reported by each student. For the third objective, researchers analyzed the existing correlations of self-perception on the transition to the “New Normal” education.
3.2.2. Analysis methods and approach
The questionnaire was sent out as a Google Form inviting the sample target to fill in the form. A couple of reminders were sent during May 2022, which was the period during the semester when the lockdown finished in Mexico and students were compelled to go back to university on a 100% face-to-face basis. At that time, the semester was also finishing, so, it was possible for students to compare both scenarios. The type of research pursued was Primary Quantitative Research, oriented to reach conclusions through certain statistical and correlational analyses. Information on students was gathered in a file in the format of comma-separated values and then uploaded to a notebook in Mathematica. It allowed the creation of specialized methods to analyze information statistically, graphically, or correlational, and particularly programming own algorithms of analysis to compare and plot the outcomes, which was valuable because of the wide number of variables intended to become involved as causal issues.
Thus, for the initial multivariable analysis, the use of multivariable graphical correlations was preferred with the ability to print plots. Such plots, despite being complex in some cases, provide a glance at the comparison between variables to find possible primary correlations, which were selectively analyzed using inference tests. For this reason, the use of clustering of data in discrete categories for each dimension being analyzed was considered. Once some correlations were noticed in the primary analysis, more concrete inference tests were performed as a two-way analysis of variance (ANOVA) test to set more conclusive outcomes for those detected correlations noticed in the first graphical insight, and in addition, getting a double check for the statistical meaningfulness.
4. Results
In this section, the direct outcomes gathered from the questionnaire are presented. Because a proper interpretation should consider diverse experiences and perceptions occurring during the COVID-19 pandemic considering adaptations required to return to face-to-face education [family and social environment, internet facilities, impact on academic performance (Ghasem and Ghannam, 2021; Hermanto and Srimulyani, 2021)], the following section includes a proper discussion. Thus, just graphical correlations among them are presented without contextual interpretations. Outcomes state a characterization of Learning, students, and institution. It relates to the perception of academic experiences through the COVID-19 pandemic, particularly during its two transitions, regarding the concerns expressed in the objectives and gathered by the instrument (Delgado, 2022). The raw outcomes regarding the Code labels and Scale numbers are reported in the Supplementary material.
4.1. First research objective: learning styles and academical traits as causal factors of academic success
This section includes the outcomes for the declared learning styles compared with the student perception of the parallel university orientation in the same terms. Also, the academic personality traits are reported stating a characterization of the students in the sample. In most parts of this section, the analysis was performed through graphical correlations by crossing over the categories of the dimensions involved as variables.
4.1.1. Distribution of learning styles and student perception of teaching orientation
Figure 2 presents the sample distribution outcomes for each learning style declared by students (questions Q1–Q5) compared with the perceived university orientation in the same category (questions Q6–Q10). Each dot graphically corresponds with one student in each comparative quadrant, they are presented as that for aesthetic purposes. Absolute percentages for each crossed-over group (institution vs. student) are shown correspondingly in red at the center of each figure. Thus, Figures 2A–E report the learning styles: external (Co)/internal(Ab), visual (Vi)/auditory-verbal (Au), deductive (De)/inductive (In), active(Ac)/reflexive(Re), and sequential(Se)/global(Gl), respectively.
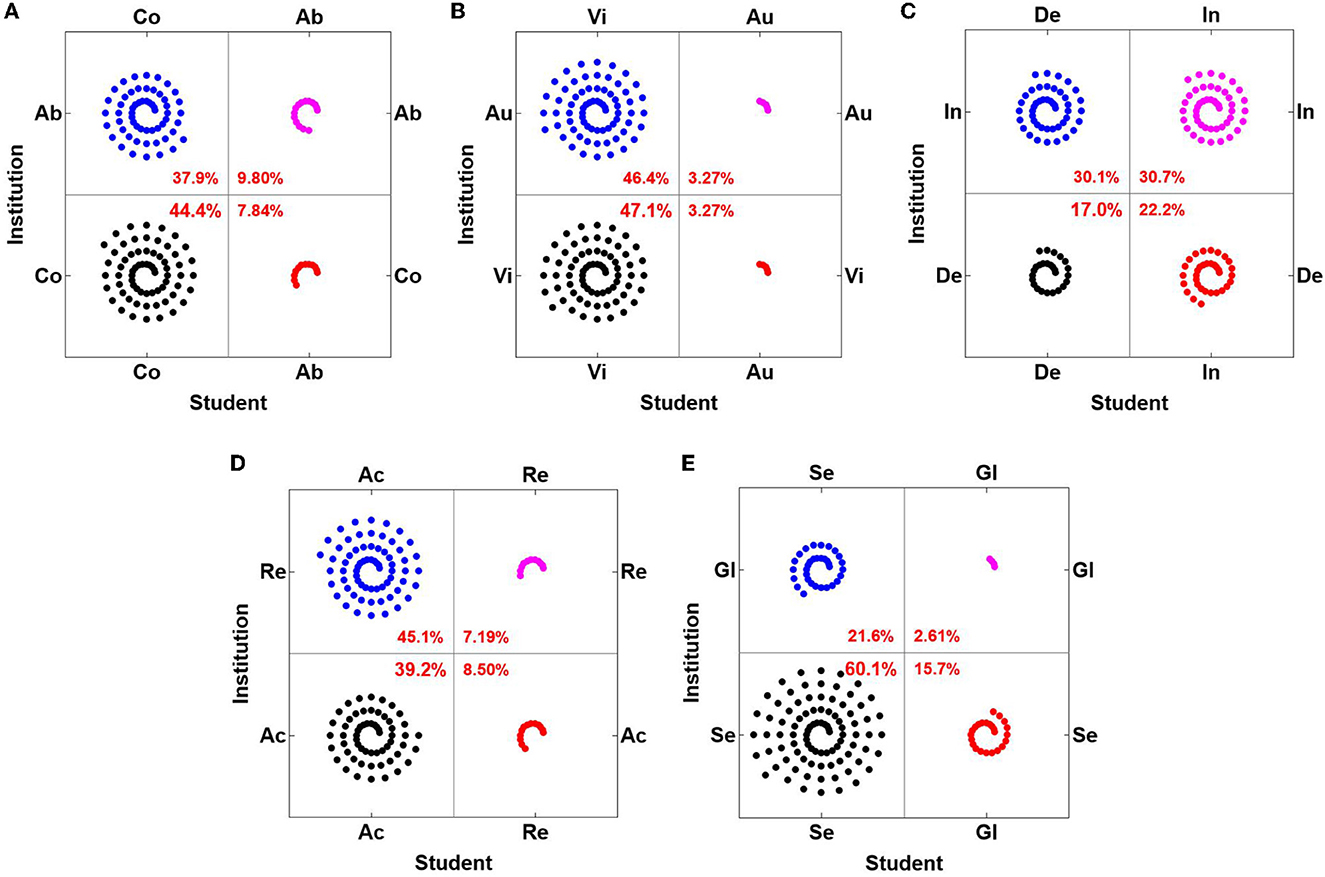
Figure 2. Learning styles of students vs. learning styles of faculty as perceived by students. (A) Concrete/abstract, (B) visual/verbal, (C) deductive/inductive, (D) active/reflexive, and (E) sequential/global.
Figure 2A shows that the surveyed population mainly uses factual information to achieve Learning instead of abstract facts. Their knowledge is based on facts rather than theories or indirect information, which characterizes a practical learning orientation (Mena et al., 2019). However, a few students combined facts with abstractions (principles, formulas, diagrams). Notably, few students perceived themselves as demanding a more profound comprehension; instead, they just followed the facts, agreeing with Kolb (2014). The teaching style was perceived as balanced between concrete and abstract knowledge, despite some students preferring factual information vs. the perceived abstract instruction.
In Figure 2B, the students are again grouped mainly around visual Learning instead of auditory. This most frequent orientation within engineering programs also involves active and reflective styles (analyzed below). Note that teaching was again perceived as balanced, combining theory, simulation, and practice (Katsioloudis and Fantz, 2012). However, some students perceived teachers as delivering excessive knowledge through writing and lecturing. Figure 2C shows a broader diversity. With a balanced orientation within the group of students (and presumably in the population), they slightly perceive themselves as less deductive (47.1%) than inductive (52.9%). Meanwhile, the university was perceived as slightly less deductive (39.2%) than inductive (60.8%). The even distribution of both styles is notable but leaves out 52.3% of students from their natural learning style.
Figure 2D confirms that engineering students perceive themselves as primarily active rather than reflective: experimentation over reflection, although the faculty orientation appears notably balanced. Despite this, as Felder and Silverman (1988) pointed out, students gradually mature and recognize that mere discussion or execution of activities is not enough; introspection or abstraction is always required for meaningful Learning. Then, it is a correct institutional balance. Figure 2E notably exhibits a recognized pattern: engineering students are generally structured and like to follow algorithms, 81.7 vs. 19.3%. Interestingly, those students who self-declared as global learners do not recognize such a faculty orientation.
4.1.2. Main learning styles and perceived teaching orientation by the surveyed sample
This subsection describes the statistical method regarding the previous results presented on radar plots of frequency. Figure 3A resumes the learning styles distribution for the student sample. Learning styles are concentrated in 22 combinations (from 32 possible), and six stand out: CoViInAcSe, CoViDeAcSe, AbViInAcSe, CoViDeReSe, CoViInAcGl, and CoViDeAcGl. They mainly share four characteristics (Concrete, Visual, Active, and Sequential), differing only in how the students catch information: Inductive or Deductive. Note particularly that in the six main combinations, Visual style was recurrent. When learning styles are broken down by gender in Figure 3B, only five stand out, and two are dominant. Women: CoViInAcSe, CoViDeAcSe, and AbViInAcSe. Men: CoViInAcSe, CoViDeAcSe, and CoViDeReSe. The first two dominant combinations are the same in both genders, being more dominant in women than men. Women are grouped in three main combinations and men in four. Among these, they differ only in Inductive or Deductive. Notably, women agree more with the abstract, sequential, and inductive styles, while men are inclined toward concrete and global.
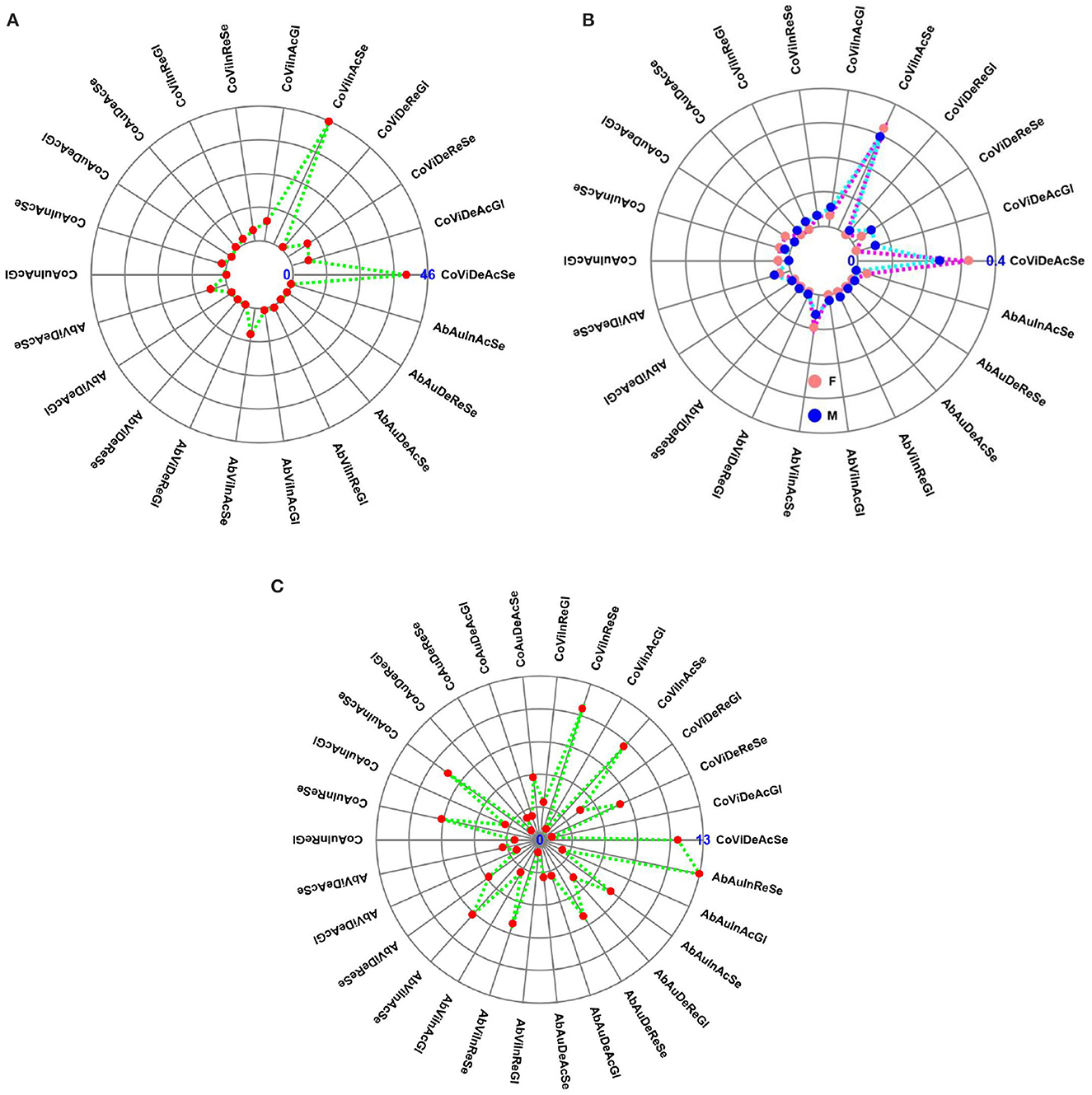
Figure 3. (A) Distribution of main learning styles being present in the group surveyed in absolute frequency, (B) same distribution split by genders, female (magenta) and male (cyan) in percentage, and (C) distribution of main declared faculty learning styles promoted as they are perceived by students (in absolute frequency).
In another trend, Figure 3C shows the 30 combinations of learning styles that the faculty focus on. Seven stand out, but only three are dominant: AbAuInReSe, CoViDeAcSe, and CoViInReSe. It highlights that the Verbal and Passive styles do not appear to be those students prefer, despite teachers most frequently using them. In the seven groups standing out, the Sequential style is recurrent. The five learning styles dimensions should be interpreted as reference styles, which, combined, set a wide range of Learning styles (Honey and Mumford, 1989). However, learning styles prevail within a classroom, and the teaching style is also decisive, becoming tied in the teaching-learning process (Honey and Mumford, 1989). Some similar results were exposed by Kolb (2007), although doing a different description than Honey and Mumford (1989).
4.1.3. Academic personality traits and their analysis by gender
The statistical distribution of outcomes for the MBTI (Jessee et al., 2006) is shown in Figure 4, coming from questions Q11–Q14. Those plots represent the frequency of occurrence for the personality traits shown by the group of students surveyed. Figure 4A reports the main personality traits. From the 15 expressed, just five stand out: ExSeThPe, InSeThPe, InItThJu, InItThPe, and ExItThPe. The dominant group: ExSeThPe, focuses on the world around them, taking outside information. They think before acting, willing to change if something impulses them. Students prefer introversion over extroversion in the second group but agree with the first group on the remaining characteristics. The third and fourth groups agree on the first three characteristics. Figure 4B shows the personality distribution by gender. Men are mainly in five groups, while women are in six. Thus, for women: ExSeThJu, ExSeThPe, InItThJu, InSeThPe, InSeThJu, and ExSeFePe; and men: ExSeThPe, InSeThPe, InItThJu, InItThPe, and ExItThPe. Due to the gender balance in the sample (and population), the men's distribution is almost dominant, noting a radical change in that of women, where greater diversity is present.
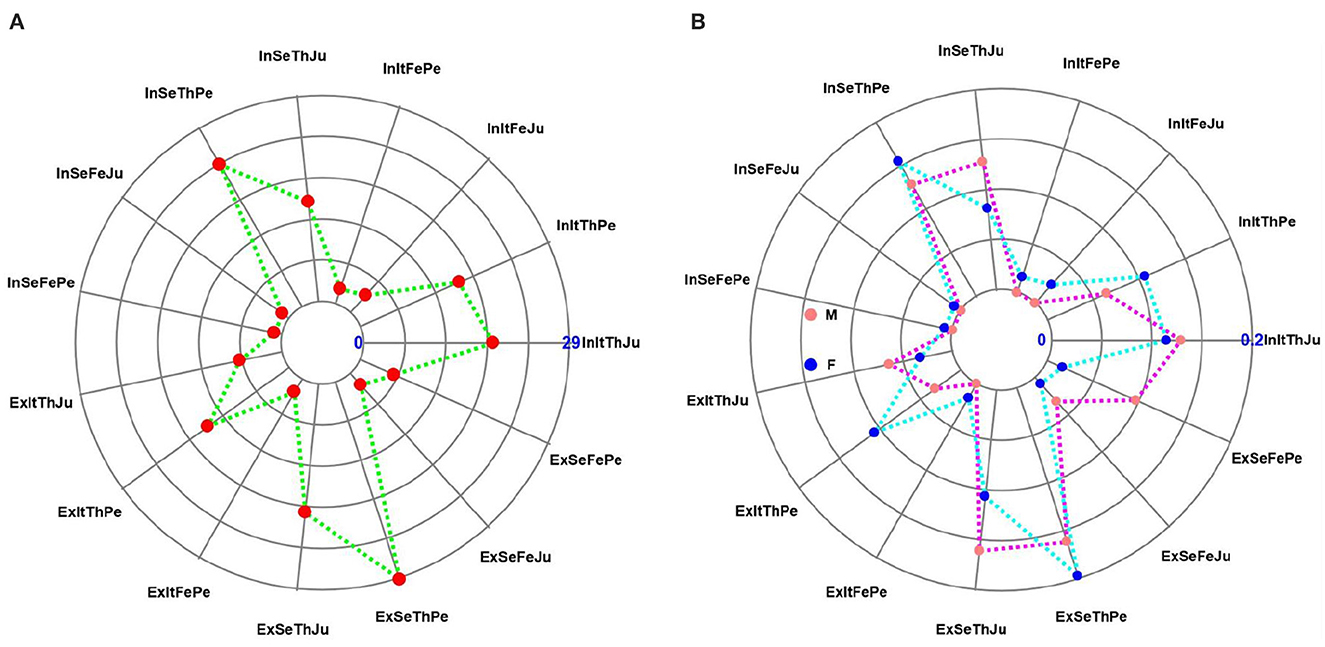
Figure 4. (A) Distribution of primary personality traits (extroversion/introversion, sensitive/intuitive, thinking/feeling, judgement/perception) self-perceived by each student in absolute frequency, and (B) the same distribution split by gender, female (pink) and male (blue) as a fractional composition.
The transition from online to face-to-face education after the confinement showed that the teaching/learning process did not favor all learning or teaching styles. Also, it did not work for all personality types. Teaching was not perceived as uniform, generating differences in student commitment, even if teachers and students aligned in the same teaching and learning styles (Mansor and Ismail, 2012). The personalities of both were a factor playing a decisive role in the evolution of education during the confinement.
4.2. First and second research objectives: technological competencies of the surveyed population and perceived faculty abilities
Digital competencies outcomes in agreement with the EDCFC have been plotted in Figure 5 as radar plots regarding the outcomes in the scale defined in the questionnaire, where each dimension of the technological dominion is reported (continuous yellow line). Dispersion is also illustrated (plus/minus one standard deviation with dashed green and red lines, respectively). Outputs were obtained from the responses to questions Q15–Q19 for students and Q20–Q24 for the student's perception of his faculty. Each type of technology was evaluated in one of the five dominion levels defined. Students perceived themselves as having fewer competencies in information search and skills related to communication and collaboration but with more excellent skills in computational security and problem-solving. Figure 5A summarizes those outcomes. By gender, men perceive themselves with less information search skills but as balanced in the remaining competencies, according to Figure 5B. There, women present an interesting variation around digital competencies: information search skills are the weakest point, but their most significant strength is creating digital content and computer security (see Figure 5C). Most students in the sample were male and had more consistent expressions; women's results displayed more dispersion in those skills.
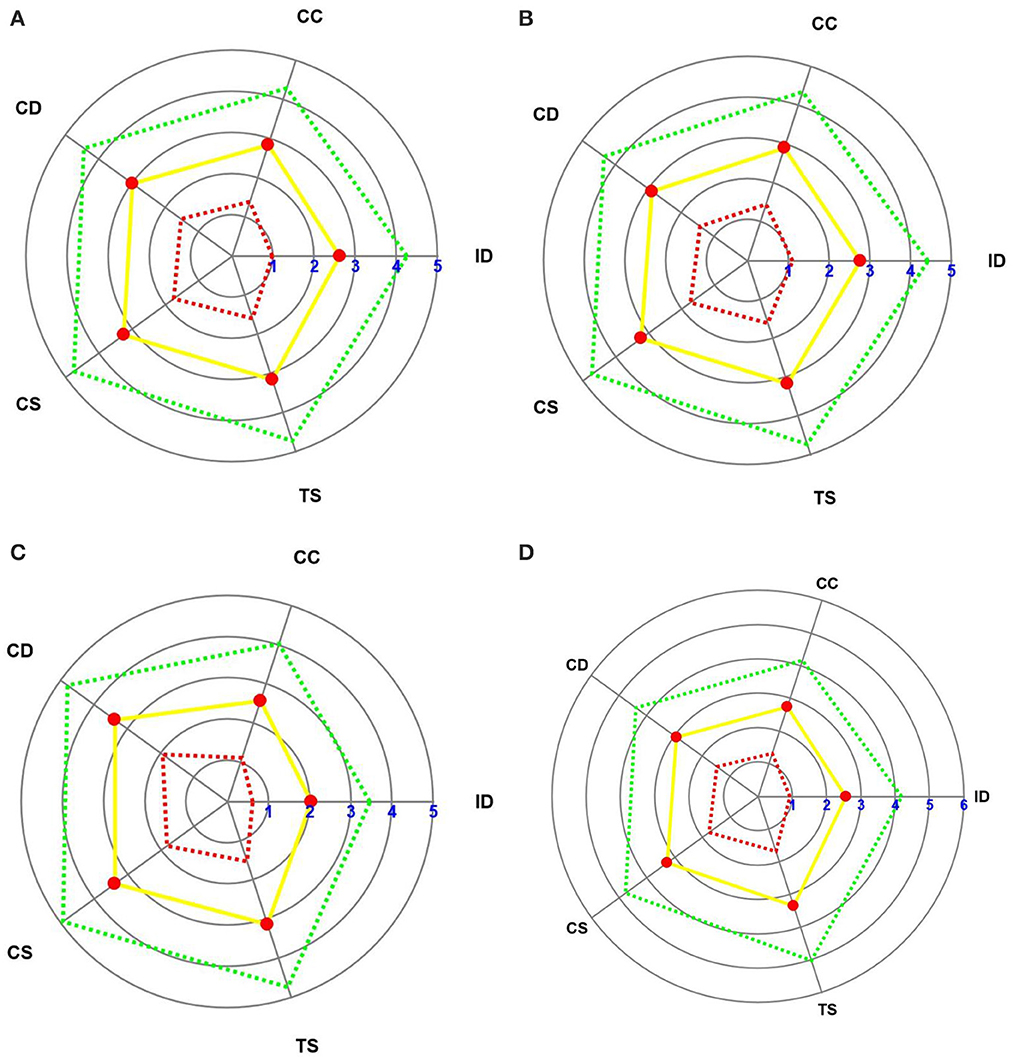
Figure 5. (A) Technological competencies (search for information and data-ID, communication and collaboration-CC, creation of digital content-CD, computer security-CS, and troubleshooting-TS) self-perceived by each student on a scale of 1–6 (less-more), indicated by the yellow line and distribution lines representing plus/minus (green/red) one standard deviation, (B) same last distribution for male students, and (C) for female students. (D) Technological competencies perceived by students of their faculty on the same scale.
Figure 5D shows students' corresponding perception of their faculty, becoming practically identical to that in Figure 5A. That is, students indicated teachers were least competent in information search and strongest in problem-solving, in agreement with Sánchez et al. (2020). No significant differences in Information and Communication Technologies (ICT) skills among teachers were associated with gender. The outcomes among male and female students suggest more research to establish why they are different. According to Petridou et al. (2009), women are more interested in acquiring knowledge and developing skills, while men consider communication skills the most important. In general, the technological competencies of students and teachers were higher than those of the general European population (Portillo et al., 2020). Indeed, our university widely promotes the use of technology to a great extent (teaching, learning, and research), strongly supporting the development of such competencies.
4.3. Third research objective: Affinity and Inclusivity, a comparison between pre-pandemic and confinement periods
Learning styles, academic personality traits, and teaching style indicators should arise a well-characterized set of parameters impacting academic performance. Thus, researchers have defined two indexes: Affinity and Inclusivity. Affinity is constructed for each student as the normalized score of matches between each learning style xQki ∈ {1, 2}, k = 1, …, 5 (questions Q1–Q5) and the perception of the same student about the institutional teaching orientation, yQk+5i ∈ {1, 2}, k = 1, …, 5 (questions Q6–Q10). Then, the Affinity for each student i (Ai) is defined as:
where δi, j is equal to 1 if i = j, otherwise 0 (Kronecker delta). The fraction of the five possible matches defines the Affinity with values 0.0, 0.2, 0.4, …, 1.0. Such an index measures how much each student is similar to the faculty in the learning/teaching styles.
For each student i, Inclusivity Ii is an index reflecting how the university effectively supports each student concerning the differences and diversity of Learning styles and academic personality traits promoted. Here, the index is constructed by adding points for each item declared and attained with the teaching strategies: for Q25–Q30 except Q27 or for Q27. This for each teaching practice j = 1, …, nk (nk = 2 for Q25–Q30 except Q27 and nk = 4 for Q27). Here, k ∈ {25, …, 30} respectively. Thus, each practice adds one of the six dimensions stated by questions Q25–Q30 to get at least one point for each. Those scores are finally averaged, attaining outcome values between zero and one:
In the subsequent analysis, these indexes are used recurrently, completely calculated from the questionnaire outcomes, to relate them with other academic aspects through the COVID-19 pandemic, such as performance, adaptation, Learning preferences, and teaching quality.
4.3.1. Perceived diversity of teaching strategies and inclusivity
Complete outcomes for the perception of teaching practices are presented in Figure 6 as normalized radar charts, including, as before, dashed lines for dispersion. Students perceived teaching strategies as diversified and balanced overall. Possibly, due to the diversity of topics studied each semester, students recognized that teachers managed the Learning neatly. Also, they perceived feedback, Social Learning, and motivation as being promoted. Practices and metacognition were still the weakest resources. Those scores, by the student, define his Inclusivity index implemented as a possible causal factor in the subsequent analysis.
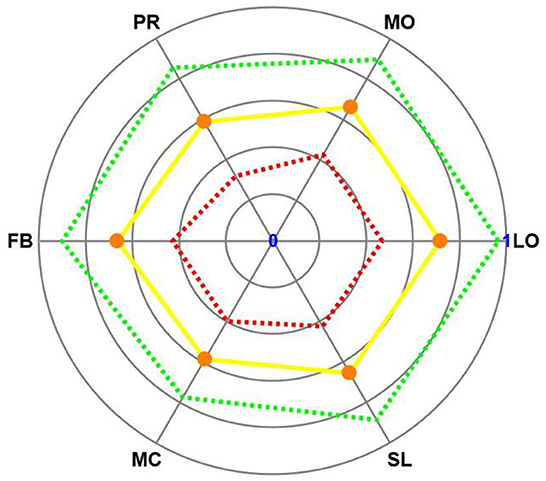
Figure 6. Main teaching strategies perceived by students on a cumulative scale (learning organization-LO, motivation-MO, practice-PR, feedback-FB, metacognition-MC, and social learning-SL) indicated by the yellow line and distribution lines representing plus/minus (green/red) one standard deviation.
4.3.2. Affinity and inclusivity indexes related with the declared academic performance
Outcomes in the next sections present several variables together to find correlations between their categorical values. Two variables are commonly used and presented on each axis, either directly from the questionnaire or calculated in the same way as some of the indexes introduced in Section 4.3. The third variable is commonly reported in color to cross over variables in agreement with the corresponding bar at the center-bottom of the plot. Individual dots presented make reference to individual students classified in clusters. Figures 7A, B exhibit the relation between Affinity and Inclusivity indexes with the quartile distribution of declared performances by students (questions Q32 and Q36). Tiny horizontal displacements of dots (each one corresponding to one student) around the Affinity values are non-meaningful, just appearing to represent all students analyzed. Performance quartile belonging is shown in color in agreement with the color bar. Both plots show that during the online teaching and face-to-face periods in the “New Normal,” increased Affinity and Inclusivity played a crucial role in the performance (red dots), despite the lowest performance in both cases not appearing completely related to them.
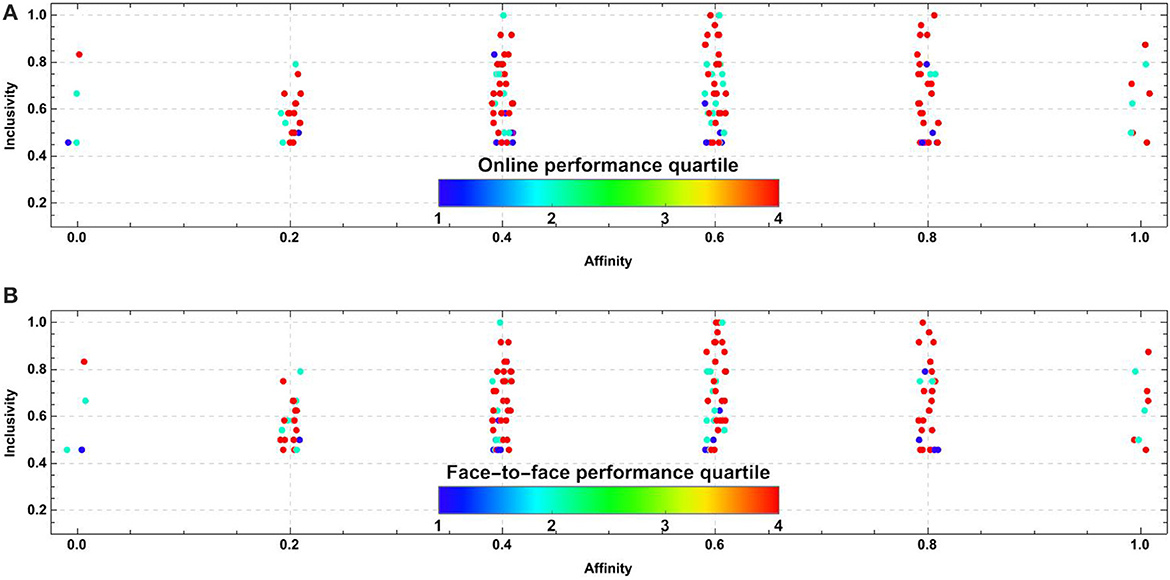
Figure 7. (A) The incremental affinity between students and faculty vs. the degree of inclusivity measured as cumulative teaching strategies perceived. Each student is additionally characterized in color by his online performance quartile, where he self-perceives. (B) The same distribution but exhibiting in color the face-to-face performance quartile where the student perceives. The dashed white line is a symbolic division from the best against the worst/best (lower/higher) learning conditions perceived.
4.4. Fourth research objective: perceived student performances during the COVID-19 stages: a summary of causal factors
The integrated Affinity and Inclusivity indices are analyzed in this subsection as causal factors in terms of performance yet compared with other factors such as teaching quality (Q34), Learning preference (Q38), Online Learning (Q32), or face-to-face Learning (Q36) by pandemic period.
4.4.1. Compared academic performances through the pandemic periods: teaching quality and adaptation
Figure 8A compares Adaptation during the lockdown under online education (Q33) and the “New Normal” under face-to-face education (Q37) with other related variables. The scale used for Adaptation is 1–4 (in agreement with the color bar below it). Figure 8A compares Adaptation vs. Teaching quality (Q34) on a scale of 0–3 (worst-better) and Online performance (Q32) on a scale of 1–6 (worst-better). Figure 8B compares Adaptation vs. face-to-face education preference (Q38) on a scale of 0–3 (lower-higher) and also the face-to-face performance (Q36) using a scale of 1–6 (worst-better). Performances are reported in color in agreement with the color bar below it. The dashed white lines split the regions with the highest/lowest Adaptation vs. Teaching quality or face-to-face preference. Note that students form clusters where the small displacements are non-meaningful; the purpose is to show each student separately.
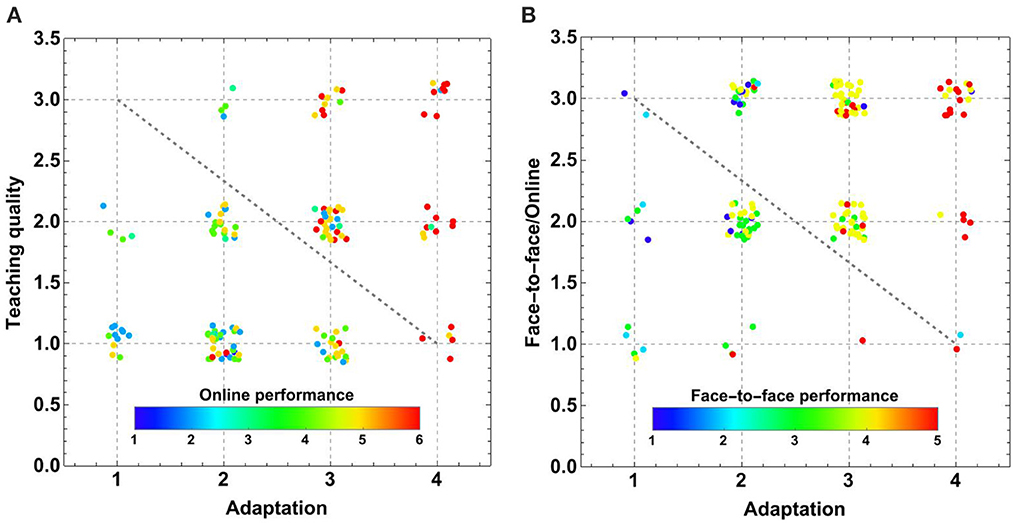
Figure 8. (A) Degree of adaptation to the online teaching model during the COVID-19 confinement vs. self-perceived online Teaching quality. Students are characterized by their self-perceived performance in the online teaching model represented in color. (B) Degree of adaptation to the online teaching model during the COVID-19 confinement vs. preference for face-to-face on online teaching, with students again characterized by their self-perceived performance in the face-to-face teaching model.
Figure 8A shows that students with better Adaptation exhibit better performance during the online learning period, while students with the lowest Adaptation had lower online performance. The latter students evaluated online Teaching quality as the lowest. For the “New Normal period,” students perceiving the highest performance clearly preferred face-to-face teaching and had the highest Adaptation for returning. Still, students with the lowest performance preferred the face-to-face learning approach. The last analysis shows most students' clear preference for the face-to-face model, independently from their performance in any period. In the online learning period, Teaching quality perception was down independently from the level of Adaptation. Comparisons between the two types of Adaptation are presented and discussed below.
4.4.2. Academic performances compared through the pandemic periods: learning preferences, gender, and academic model
Compared performances in each pandemic period (online and face-to-face teaching) are presented here. A third variable is included in Figures 9A–C, respectively: face-to-face preference over online preference, Gender, or Program plan (Tec20 or Tec21). The dashed white line splits the cases with the highest/lowest performances during each period as a reference.
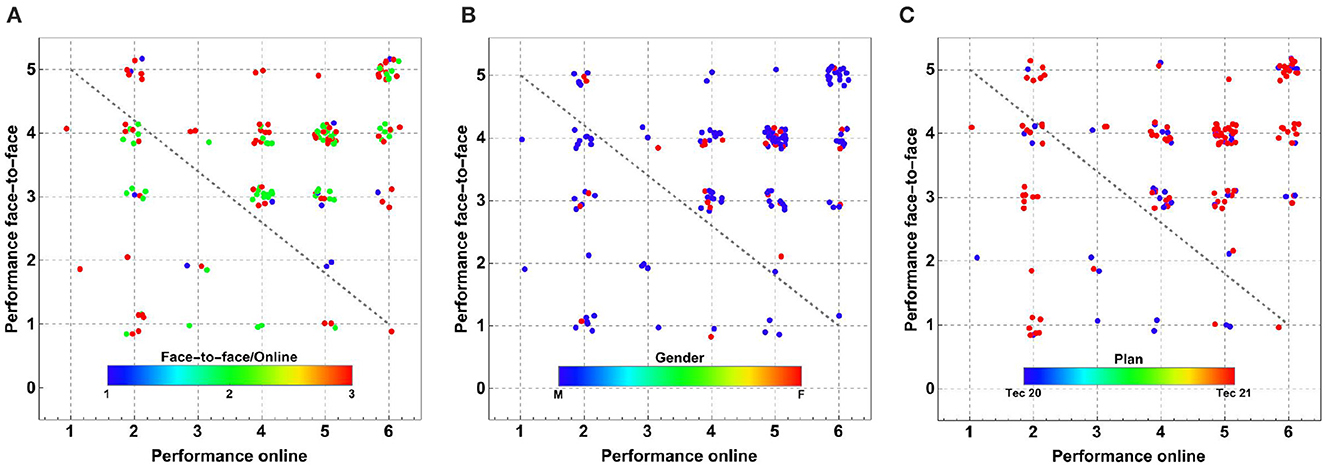
Figure 9. Comparative online vs. face-to-face perceptions by each student, exhibiting in color the following, splitting: (A) by the degree of preference for the face-to-face model (red) against the online modality (blue), (B) by gender, female (red) vs. male (blue), and (C) by educative model, Tec20 (blue) vs. Tec21 (red).
Figure 9A shows that most students with medium or higher performances mainly prefer face-to-face Learning. Just a few students surveyed exhibited low performances in both periods together. Considering most of the surveyed students were men, Figure 9B does not reveal apparent differences by gender in perceptions. Note the little groups of women and men with low online performances after having a better face-to-face performance. Figure 9C compares the two educational models, noting that most students surveyed fell under the Tec21 model, as expected (see Table 1). Only a tiny segment of Tec21 students exhibited low perceptions during online Learning but after notably higher face-to-face perceptions (red dots on the left side and in the upper region). By comparing the data in more detail, Figure 9C highlights that 20% of the students of the Tec20 plan did not have the expected performance in either of the two learning models. Meanwhile, Tec21 students (75 of 108 had not experienced the face-to-face model in the university) also perceived their performance was inadequate in both models.
4.5. Fourth research objective: impact of academic personality traits on the online and face-to-face education success
Finally, researchers compared academic personality traits with both online and face-to-face performances. Figure 10A plots several variables. Each personality trait defined in the sample is considered a category on the radar plot. The average Online performance (on a scale of 1–6) is shown radially [see the questionnaire Delgado (2022)] as an average for the corresponding personality trait. In addition, each dot is colored in agreement with its average face-to-face performance on the personality trait group being reported (shown in the color bar below). Considering that the contents and the teachers did not change considerably during the face-to-face and online models, the students' perceptions could be influenced by the faculty accompaniment and the educational quality offered (Keržič et al., 2021). Then, the percentage of students declaring the same academic quality in both models also declared that their performance did not change.
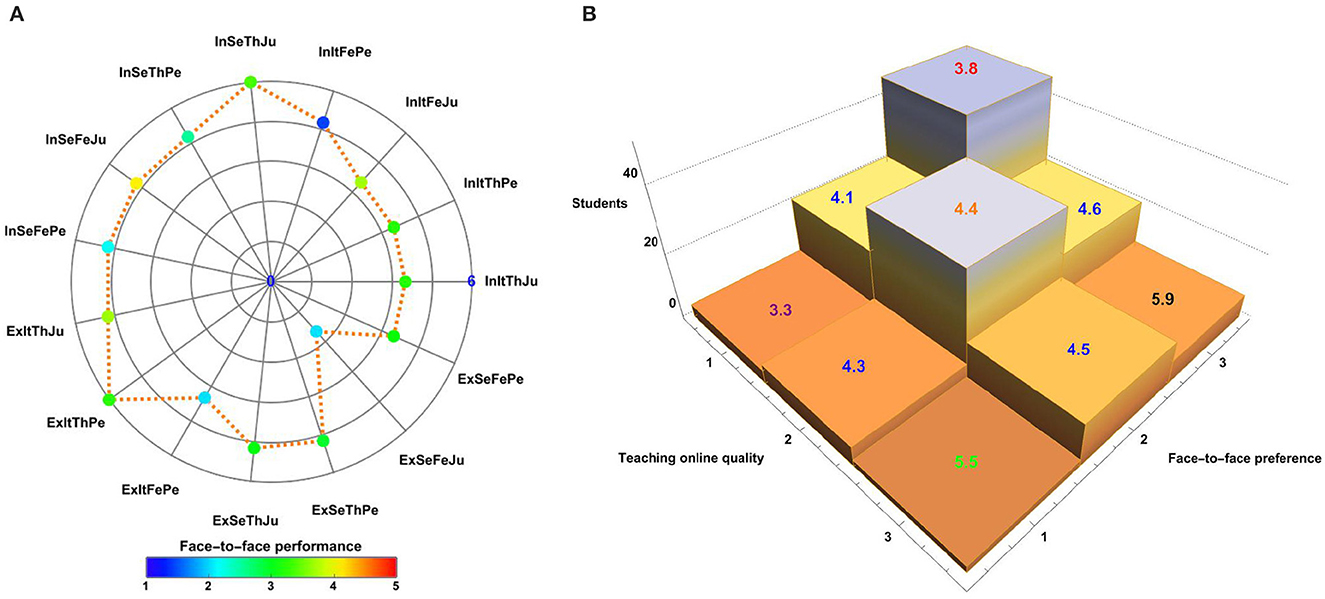
Figure 10. (A) Online performance declared (radial) and face-to-face performance (color) by personality traits. (B) Frequency comparison of online Teaching quality (Q34) and face-to-face education preference (Q38), including the Online performance average on the top.
Interestingly, we note that traits on the right had lower Online performances. This fact is important because two of the most represented traits belong to this region. Nevertheless, note that both performances are in the middle of the scale in such cases, thus suggesting no differences during both periods. Other students dominantly represented in the sample also exhibited good performances in both cases. Interestingly, students with the traits InItFePe and ExItFePe showed lower face-to-face perceptions of Intuitive, Feeling, and Perception, an orientation few align with learning in engineering programs.
Note that the students with the best-adapted personalities in both models are commonly extroverted but combined with intuitive, thinking, or perceptive. On the contrary, the least adapted are introverted, particularly combined with the feeling trait representing people who analyze and make decisions based on other people's opinions, changing their own decisions.
5. Discussion
The research objectives have been covered through the data analysis. Nevertheless, other aspects regarding the previous outcomes should be discussed by integrating contextual information:
1) Performance transition as a causal consequence of students' traits (partially suggested by Figure 10A).
2) Adaptation differences between two types of instruction: online and face-to-face (as suggested by Figure 8).
3) Real preference for the type of instruction and associated outcomes relative to online education (suggested by Figure 9A).
Those aspects are central and suitable for our analysis considering the advantages of online education: learning at any time and from anywhere, access and availability of information, flexibility in schedules, personalized learning paces, economic savings, and development of technological competencies (Delgado, 2021b). Also, the disadvantages include the absence of laboratories, workshop practices (Gowda and Ayush, 2020; Hasan and Khan, 2020; Debes, 2021), low effectiveness of teaching and evaluation, lower motivation, and lack of interaction (Delgado, 2021b), sometimes inappropriate learning environments (Hermanto and Srimulyani, 2021), and lack of study habits and ability for adaptation (Aristeidou and Cross, 2021). Many interpretative factors contribute to academic success, many external to the school ecosystem. In this section, the last itemized aspects relating to teachers' opinions are discussed, particularly assessing the return transition from online education to face-to-face education under the “New Normal.”
5.1. Revisiting research objectives: remarkable aspects derived from the contextual analysis of survey outcomes
Several indicators were analyzed statistically through the last transition: Performance, Adaptation, and Learning Model acceptance by conducting two-way ANOVA tests to compare them.
5.1.1. Performance transition as a function of learning styles and personality traits
Relations suggested by Figure 10A between Personality traits and academic performance should be analyzed quantitatively. Performances at each stage were compared in agreement with the survey outcomes classified by a Personality trait, subjecting this to a two-way ANOVA test (Cohen, 1988; Gelman, 2005) to decide the real variability through each dimension. Note that Performances in each stage have different scales (level 1 in Q32 is not present in Q36 due to the short period of face-to-face education). Performance values were normalized on the scale [0, 1], becoming barely comparable. Then, considering the Personality trait as a first factor and the pandemic stage as a second factor, the normalized Performance was compared using the test with a significance of α = 0.05. The test is shown in Table 2. The p-values do not indicate statistically meaningful differences by Personality trait or Pandemic stage (α < p) despite, under a different context, a limited impact is observed between personality and engagement in the online courses during the lockdown (Quigley et al., 2022).
Repeating the procedure, this time using the Learning style as the first factor, it was found that the test did not indicate meaningful differences either (Table 3). This outcome could be explained due to the impact of Affinity, as observed in Figure 7, possibly overshadowing the direct effect on Performance because of the adaptability exhibited. Such findings are interesting because discipline could maintain students' efforts through the transition. Such outcomes are positive; personality traits and learning styles did not play a fundamental role in the Performances cluster, possibly to the Affinity with the primary learning styles and the broad Inclusivity of the leading academic Personality traits. Outcomes were compatible with those observed in Figure 9, impacting most students.
5.1.2. Adaptation transition as a function of learning styles and personality traits
From the findings in Figure 8, denoting the impact of Adaptation on Performance, a quantitative analysis was developed. Researchers assessed a meaningful transition by considering the declared Adaptation index in each stage, first considering Personality traits (Table 4) and then Learning styles (Table 5) as the first factor, respectively. The support given to teachers adapting their courses to an online version promoted the use of technological resources. Also, the institutional knowledge acquired during the confinement, the follow-up given to students, and the continuous assessment boosted the continuous improvement of the blended teaching approach, as observed in similar contexts (Akram et al., 2021).
In this case, only the Personality trait exhibited as a statistically significant factor for Adaptation (α = 0.05 < p = 0.0224), interestingly not for Learning styles. It suggests that Adaptation could be more related to academic Personality than Learning styles. Also, there is no significant change in Adaptation going to the “New Normal.” This fact is also positive and already guessed from Figure 8, where most students exhibited satisfactory Adaptation only differentiated by Teaching quality or Teaching preference.
5.1.3. Preference for educative style under the transitions
The outcomes observed in Figures 8A, 9A suggest that teaching quality is well evaluated by students with good Online performances, despite their preference for the face-to-face model. Students were asked about the Online teaching quality (Q34) and face-to-face education preference (Q38). If both questions are well evaluated, there is no clear evidence about a real preference for the face-to-face approach. If they are not concordant (either poor online education with face-to-face preference or high online education with poor face-to-face preference), it means a double check for the preference on one of the models (face-to-face or online, respectively). To perform such a comparison, Figure 10B shows the number of students (vertical axis) fulfilling with each pair of outcomes: Teaching online quality (Q34) and face-to-face education preference (Q38); then with the average Online performance reported at the top of each bar, and calculated for each subset of students.
Regarding the previous remarks, most students preferring face-to-face education had low perceptions of online Teaching quality. Interestingly, such students expressed almost the lowest Online performance (3.8 in red). It possibly means those students were academically affected by the type of education during the confinement. Students in the middle, the second most, indicated a medium online Teaching quality and no preference between online and face-to-face education, with moderately high Online performance (4.4 in orange). Thus, such students undoubtedly correspond to all those indifferent to the teaching model, probably because they had no notable academic losses. The less frequent group, students considering the online model as the best quality, notably had the second-highest Online performance (5.5), which agreed with their preference. Two other groups are located on each side. The first was those expressing their preference for the face-to-face model but evaluating their experience with the Online model very well. They exhibited the highest Online performance (5.9 in black), undoubtedly corresponding with the best students academically. The other group, those poorly evaluating the Online model but also preferring it, exhibited the lowest Online performance (3.3 in purple). Such a group certainly experienced more academic difficulties and obstacles.
The first two groups represent most of the students, it was found that the entire population was mainly split between those easily adapting to both education scenarios and those preferring the face-to-face model because their outcomes or performance under the online model was average or lower. For the latter students, education under the “New Normal” opened opportunities to overcome the confinement experience. In similar studies, the preference for online education appeared multivariate despite the technological conditions (Muthuprasad et al., 2021), or quality, flexibility, self-paced classes, or productivity (Gaba et al., 2021).
5.1.4. Final contextual analysis of findings and a summary of the research objectives
It was found in our engineering students, on average, the sustained discipline to maintain their academic performance through the lockdown. Our university was prepared to a great extent for such contingency in terms of technology culture, online and blended courses as part of the standard academic offering, teacher training in educational technologies and didactic methodologies by discipline, and a well-settled infrastructure for internet services, resources, support services, equipment for teachers, and technological culture. All that intangible value was already present to face the COVID-19 crisis with a rapid response and a sustained, but never before experienced, online education (Kaqinari et al., 2022).
Preferences expressed by students for face-to-face education should be interpreted as situations reflecting their effective learning and performance. In the analysis, it was observed that some students genuinely adapted to both models regarding performance but still preferred the face-to-face approach. Other groups favored one or the other models, and then expressed their opinion as a function of this fact. Such diversity, again, is the expression of the narrowed scenario for the sudden and emergent online education imposed during the COVID-19 confinement, but also accompanied by a set of institutional scaffolding actions. Those, together with previously developed culture, training, and facilities for online and blended learning (Mayers et al., 2022), could be compared with other experiences (Andreou et al., 2022).
5.2. Research limitations, practical, and theoretical implications
Our analysis was conducted on Electronic, Mechanical, and Mechatronics Engineering and Computer Science students. These academic programs require the most comprehensive analytical backgrounds in terms of Math and Basic sciences. Such programs could be more based on academic rigor and orientation instead of a closer professional environment contact. Comparing other engineering programs, such as Industrial and Chemical engineering, would be interesting. Our student sample reflects the real proportion between male and female genders (77%/23%) in engineering. In addition, it also reflects the proportion for the two active educational models in our institution (Tec20 and Tec21, 29%/71%). Thus, these demographic aspects are well-represented in our analysis. In addition, the sample (and population) represents students in a university with a solid culture in information technology compared to other Mexican universities. This parameter became well-valued and uniform among the sample of students. Those are limitations in our analysis to generalize the outcomes to an entire student engineering community in other universities.
To analyze the results, there are a set of theoretical and practical implications derived. One of the most remarkable theoretical aspects already observed in another study (Bashir et al., 2021) is the impact of online learning. Also emphasized by Delgado (2022), online learning has represented the opportunity for some students to adapt to a new educative model, which requires a different type of self-discipline. Sometimes it involves certain flexibility, but also demands a self-imposed agenda for learning. Nevertheless, as it was noticed all around the world, such a model could be difficult for many students once they feel lost in it or as they advance in their course (Jung et al., 2021; Sharma and Alvi, 2021). As a result of our analysis, it has been noticed that certain personality traits, more than learning styles, have not become engaged with the model. Then, some of those students appear to have academic difficulties still in the face-to-face approach. In another trend, students who succeeded in both models still preferred the face-to-face approach. In terms of practical recommendations, a blended-learning model requires a series of measures in place not always noticed in the face-to-face approach, thus they were unnoticed by many teachers, who only tried to reproduce the standard model in an online media. In addition, whether significant or not, no differences were made in students in terms of their academic personality or learning styles (Delgado, 2021b). At least in our university, the affordability of computer resources, as well as the widespread of their literacy, were not a crucial factor, despite the extended time that online learning introduced with new aspects to be considered for a full-time implementation (Delgado et al., 2021; Hendriksen et al., 2021). The last aspect was waived because of the technological culture present at the university where the study was conducted (students/teachers/training programs). Despite this, the last aspects are not true in general under different educative contexts (Ogundari, 2023). Transitions in terms of self-assessment, particularly going on the “New Normal” period, smoothing such transition for students have become a crucial factor to correctly evaluate online education, but also the real background of skills, discipline, and knowledge in higher education students. This aspect appeared consistently in some groups of students involved in the current research (Sandvik et al., 2023).
5.3. Recommendations for the teaching practice in university
5.3.1. Development of technological skills and didactic methodologies
In the present research, technological skills assess an element of academic success during confinement (Lorente-Rodríguez and Pulido-Montes, 2022). Students recognized themselves as competent in four of the five domains. Problem-solving is a skill the teacher/institution needs to support more. Notably, this is the lowest domain in women. Figure 6 also reflects the need for practical activities, class activities, and motivation using technology and combining individual and team didactic strategies. These actions may support more students who otherwise would drop courses or fail.
5.3.2. Learning styles, affinity, and inclusion
The outcomes in Figure 2 show a good relationship between Learning styles and teaching in the community on average but also show evidence of the necessity to increase active learning, reflection, auditory attention, and ability to simplify, doing what is necessary to use several communication channels to give instructions. For engineering students, during the transition from online to face-to-face classes under the “New Normal,” the academy must adapt activities to be carried out in the classroom or the university facilities considering the combinations of learning and teaching styles.
5.3.3. Continuous self-assessment of teaching strategies
A no less important, permanent, and relevant aspect is the ability of each teacher to self-assess their didactic strategies considering a possible characterization of their students. The ability of each teacher to understand the causes and effects of their design decisions becomes relevant, not only to determine how they impact each type of student but also to understand the evolution of generations, adapting to the external impositions of the institution or society.
5.3.4. Smoothing the educative transitions
This aspect requires recurrent design activities to be carried out in the classroom or within the university facilities to boost the construction of interpersonal relationships and promote teamwork or peer-to-peer work. Interactive learning experiences should be encouraged, and the importance of the contents to be applied should be explained to reduce the inertia of overconfidence and comprehend the time to perform them.
5.3.5. Affinity and inclusion
It is recommended that the faculty accentuates and uses the pillars of affinity and inclusion to motivate student participation and make them aware of self-regulation. Communicating by writing and verbally what is expected of students in their deliverables and providing feedback will result in the desired student success and graduation profile. This will overcome the absence of recorded classes and the lack of other resources during the online model. The knowledge and application of diverse teaching and learning methodologies must be strengthened to strengthen academic inclusion (Jaegler, 2022).
6. Conclusions
This analysis sought to understand how some known academic traits impacted online learning in Higher Education, particularly during the COVID-19 transitions to “New Normal” for engineering students. Some essential facts regarding those issues were considered for the conclusions below and certain future research opportunities in this area are discussed.
It has been noticed that only certain learning styles appear to be represented in the sample, so it is assumed to be true for the entire population. The control group reflects a very well-characterized student population (concrete, visual, active, and mainly sequential). Other than that, the faculty teaching style appears more balanced throughout categories, with the exception of visual and sequential styles. Notably, combinations of different learning styles from students are clustered around a couple of learning style types, which are notably not differentiated by gender. A similar trend is noticed for personality traits, despite being slightly more spread throughout their different types.
In another aspect of this search of causal variables, technology competencies rank on the 20% higher levels for students, showing a limited dispersion. Altogether, faculty competencies are notably comparable, and almost perfectly aligned with those of students. Another trend found was that faculty stated a medium level of diversity in terms of their learning activities, but still with certainly remarkable dispersion. This shows that there are still opportunities for the faculty to diversify the use of resources, teaching approaches, and teaching methods, thus preventing the possible dispersion of learning styles in the students' group.
Nevertheless, another explanation suggests that faculty teaching methods could have evolved to become useful for larger groups combining learning styles. In fact, results also show meaningful levels of Affinity (as defined in this research) between the learning styles of students and faculty practices. This implies that faculty is offering wide teaching/learning practices in their courses. No meaningful differences are found for the last outcomes presented if they are split as referring to online or face-to-face learning approaches, which refers to a well-trained faculty in teaching methodologies, practices, and/or resources.
Students with a higher level of Adaptation (as defined in this research) showed higher preferences for the face-to-face teaching approach, also reaching the best performances, but also notable outcomes in the online model. Instead, students with poorer Adaptation also exhibited a preference for the face-to-face model but assigning, in addition, a bad quality to the online model. Most of those students obtained anyway lower performances in both learning models. No differences were observed splitting the sample by gender in those last outcomes. Poor evaluation of the online model coming from the students with lower performances suggests a certain correlation with academic personality traits.
Finally, in a two-way ANOVA test, the level of Adaptation appeared recurrently with a meaningful correlation with personality traits (for instance, extroversion and introversion stand out as discriminators), but notably not with learning styles. A possible reason for this is that faculty still deployed good inclusivity actions, shadowing the impact of learning styles on performance. It means that the diversity of learning activities developed by the faculty seemed to scaffold the differences in affinity between learning styles and the orientation of those activities, thus ensuring not to induce a discriminating effect on such dispersion.
Regarding the university where the study was conducted, in the last years, but more particularly through the COVID-19 pandemic, it extended its depth for the effectiveness of Higher Education considering external factors as drivers of education and other internal factors inherent to students' traits. Such a fact is now partially visualized and capitalized in the current analysis. In general, other distinctions in Education owned by the faculty and university organization should be analyzed for impact, for example, programs effectively oriented to scaffold academic diversity (in learning styles, personality, and future perspectives) and to develop an egalitarian hidden curriculum, all of which contribute to the sustainability of Higher Education (UNESCO, 2020b).
Data availability statement
The original contributions presented in the study are included in the article/Supplementary material, further inquiries can be directed to the corresponding author.
Ethics statement
The studies involving human participants were reviewed and approved by Instituto Tecnológico y de Estudios Superiores de Monterrey. Written informed consent for participation was not required for this study in accordance with the national legislation and the institutional requirements.
Author contributions
All authors listed have made a substantial, direct, and intellectual contribution to the work and approved it for publication.
Acknowledgments
We are grateful to all students in Tecnológico de Monterrey due to their dedication from March 2020 to June 2022 during the COVID-19 lockdown. The authors also acknowledge the technical and financial support of Writing Lab, Institute for the Future of Education, Tecnológico de Monterrey, Mexico, in the production of this work.
Conflict of interest
The authors declare that the research was conducted in the absence of any commercial or financial relationships that could be construed as a potential conflict of interest.
Publisher's note
All claims expressed in this article are solely those of the authors and do not necessarily represent those of their affiliated organizations, or those of the publisher, the editors and the reviewers. Any product that may be evaluated in this article, or claim that may be made by its manufacturer, is not guaranteed or endorsed by the publisher.
Supplementary material
The Supplementary Material for this article can be found online at: https://www.frontiersin.org/articles/10.3389/feduc.2023.1156724/full#supplementary-material
References
Akram, H., Yingxiu, Y., Al-Adwan, A. S., and Alkhalifah, A. (2021). Technology integration in higher education during COVID-19: an assessment of online teaching competencies through technological pedagogical content knowledge model. Front. Psychol. 12, 736522. doi: 10.3389/fpsyg.2021.736522
Al-Qasemi, A., El-Garbiah, B., and Dor-Haim, P. (2022). Teachers' technological competence and success in online teaching during the COVID-19 crisis: the moderating role of resistance to change. Int. J. Educ. Manag. 36, 1–13. doi: 10.1108/IJEM-03-2021-0086
Andreou, E., Roussi, C., Tsermentseli, S., Menabò, L., and Guarini, A. (2022). Teachers' self-efficacy during the COVID-19 pandemic in greece: the role of risk perception and teachers' relationship with technology. Educ. Sci. 12, 600. doi: 10.3390/educsci12090600
Antón-Sancho, A., Vergara, D., and Fernández-Arias, P. (2022). Influence of country digitization level on digital pandemic stress. Behav. Sci. 12, 203. doi: 10.3390/bs12070203
Aristeidou, M., and Cross, S. (2021). Disrupted distance learning: the impact of COVID-19 on study habits of distance learning university students. Open Learn. J. Open Distance e-Learn. 36, 263–282. doi: 10.1080/02680513.2021.1973400
Aslaksen, K., and Lorås, H. (2018). The modality-specific learning style hypothesis: a mini-review. Front. Psychol. 9, 1538. doi: 10.3389/fpsyg.2018.01538
Autio, O. (2011). The development of technological competence from adolescence to adulthood. J. Technol. Educ. 22, 71–89. doi: 10.21061/jte.v22i2.a.5
Bailey, R. P., Madigan, D. J., Cope, E., and Nicholls, A. R. (2018). The prevalence of pseudoscientific ideas and neuromyths among sports coaches. Front. Psychol. 9, 641. doi: 10.3389/fpsyg.2018.00641
Balkhi, F., Nasir, A., Zehra, A., and Riaz, R. (2020). Psychological and behavioral response to the coronavirus (COVID-19) pandemic. Cureus 12, e7923. doi: 10.7759/cureus.7923
Bashir, A., Bashir, S., Rana, K., Lambert, P., and Vernallis, A. (2021). Post-COVID-19 adaptations; the shifts towards online learning, hybrid course delivery and the implications for biosciences courses in the higher education setting. Front. Educ. 6, 711619. doi: 10.3389/feduc.2021.711619
Boneh, M., Feniger-Schaal, R., Bivas, T., and Danial-Saad, A. (2022). Teachers under stress during the COVID-19: cultural differences. Teach. Teach. 28, 164–187. doi: 10.1080/13540602.2021.2017275
Boström, L., Collén, C. U D, and Gidlund, U. (2021). A rapid transition from campus to emergent distant education; effects on students' study strategies in higher education. Educ. Sci. 11, 721. doi: 10.3390/educsci11110721
Cáceres, M. P., Marín, J. A., Navas-Parejo, M. R., and Berral, B. (2021). O impacto do estado de alarme decretado pela COVID-19 na inclusão educacional. Texto Livre 14, e34204. doi: 10.35699/1983-3652.2021.34204
Cagiltay, N. (2008). Using learning style theory in engineering education. Eur. J. Eng. Educ. 33, 415–424. doi: 10.1080/03043790802253541
Cárdenas, S., Lomelí, D., and Ruelas, I. (2022). “COVID-19 and post-pandemic educational policies in mexico. what is at stake?” in Primary and Secondary Education During COVID-19, ed F. M. Reimers (Cham: Springer), 153–75. doi: 10.1007/978-3-030-81500-4_6
Carretero, S., Vuorikari, R., and Punie, Y. (2017). DigComp 2.1. The Digital Competence Framework for Citizens with Eight Proficiency Levels and Examples of Use. Luxembourg: Publications Office of the European Union.
Chamberland, G., Lavoie, L., and Marquis, D. (1995). 20 Formules Pédagogiques. Québec, QC: Presses de l'Université du Québec.
Clugston, R., and Calde, W. (2000). Critical dimensions of sustainability in higher education. In sustainability and university life. Int. J. Sustain. High. Educ. 1, 53. doi: 10.1108/ijshe.2000.24901aae.005
Collins, A., and Stevens, A. (1983). “A cognitive theory of inquiry teaching,” in Instructional-Design Theories and Models: An Overview of the Current Status, ed C. M. Reigeluth (Hillsdale, NL: Lawrence Erlbaum Associates), 198.
Corell-Almuzara, A., López-Belmonte, J., Marín-Marín, J.-A., and Moreno-Guerrero, A.-J. (2021). COVID-19 in the field of education: state of the art. Sustainability 13, 5452. doi: 10.3390/su13105452
De la Riva, G. A., Espinoza, C. C., and Juárez, M. (2015). “Sustainability in engineering education: an approach to reach significant learning and character skills,” in Sustainability in Higher Education, ed P. Davim (Waltham, MA: Elsevier-Chandos Publishing), 97–125.
Debes, G. (2021). Distance learning in higher education during the COVID-19 pandemic: advantages and disadvantages. Int. J. Curric. Instr. 13, 1109–1118.
Delgado, F. (2021a). Teaching physics for computer science students in higher education during the COVID-19 pandemic: a fully internet-supported course. Future Internet 13, 35. doi: 10.3390/fi13020035
Delgado, F. (2021b). Transforming a blended learning course in numerical methods into a flexible digital course during the COVID-19 crisis. Int. J. Mob. Learn. Organ. 15, 332–353. doi: 10.1504/IJMLO.2021.10036916
Delgado, F. (2022). Post-COVID-19 transition in university physics courses: a case of study in a mexican university. Educ. Sci. 12, 1–41. doi: 10.3390/educsci12090627
Delgado, F., Enríquez-Flores, M., and Jaimes-Nájera, A. (2021). Lessons in the use of technology for science education during COVID-19 age under a teachers' collaboration cluster. Educ. Sci. 11, 543. doi: 10.3390/educsci11090543
Delgado, F., López, C., and Gutiérrez, F. (2012). Collegiate Deployment and Assessment of a Mobile Learning Program in Higher Education. Mexico City: FIMPES.
Dorn, E., Hancock, B., Sarakatsannis, J., and Viruleg, E. (2021). COVID-19 and Education: The Lingering effects of Unfinished Learning. Chicago, IL: McKinsey and Company, 27.
Felder, R. (2020). Opinion: uses, misuses, and validity of learning styles. Adv. Eng. Educ. 8, 1–16.
Felder, R., and Silverman, L. (1988). Learning and teaching styles in engineering education. Eng. Educ. 78, 674–681.
Fien, J. (2022). Advancing sustainability in higher education: issues and opportunities for research. High. Educ. Policy 15, 143–152. doi: 10.1016/S0952-8733(02)00005-3
Gaba, A. K., Bhushan, B., and Rao, D. K. (2021). Factors influencing the preference of distance learners to study through online during COVID-19 pandemic. Asian J. Distance Educ. 16, 194–206. doi: 10.5281/zenodo.4965925
García-Cué, Santizo Rincón, J. A., and Alonso García, C. M. (2009). Instrumentos de medición de estilos de aprendizaje. Rev. Estilos Aprendiz. 2, 1–19. doi: 10.55777/rea.v1i2.849
Gelman, A. (2005). Analysis of variance- why it is more important than ever. Ann. Stat. 33, 1–53. doi: 10.1214/009053604000001048
Ghasem, N., and Ghannam, M. (2021). Challenges, benefits and drawbacks of chemical engineering on-line teaching during COVID-19 pandemic. Educ. Chem. Eng. 36, 107–114. doi: 10.1016/j.ece.2021.04.002
Gleichgerrcht, E., Lira-Luttges, B., Salvarezza, F., and Campos, A. L. (2015). Educational neuromyths among teachers in Latin America. Mind Brain Educ. 9, 170–178. doi: 10.1111/mbe.12086
Goldbach, A., Hauser, M., Schuppener, S., Leonhardt, N., van Ledden, H., Bergelt, D., et al. (2022). Social responsibility in the context of inclusive higher education development–experiences and insights from the perspective of participatory teaching. Int. J. Sustain. High. Educ. 23, 799–814. doi: 10.1108/IJSHE-04-2021-0140
Goudeau, S., Sanrey, C., and Stanczak, A. E. A. (2021). Why lockdown and distance learning during the COVID-19 pandemic are likely to increase the social class achievement gap. Nat. Hum. Behav. 5, 1273–1281. doi: 10.1038/s41562-021-01212-7
Gowda, R., and Ayush, G. K. (2020). “A study on advantages and disadvantages of online teaching during COVID-19 with special reference to Mangalore University students,” in Proceedings of International e-Conference on Impact of COVID-19 on Various Areas of Global Economy, Science and Humanities (Gadcchiroli: Gondwana University).
Harvey, R. (1996). “Reliability and validity,” in MBTI Applications: A Decade of Research on the Myers-Briggs Type Indicator, ed A. L. Hammer (Palo Alto, CA: Consulting Psychologists Press), 227.
Hasan, N., and Khan, N. H. (2020). Online teaching-learning during COVID-19 pandemic: students' perspective. Online J. Distance Educ. e-Learn. 8, 202–213.
Hendriksen, P., Garssen, J., Bijlsma, E., Engels, F., Bruce, G., Verster, J., et al. (2021). COVID-19 lockdown-related changes in mood, health and academic functioning. Eur. J. Investig. Health Psychol. Educ. 11, 1440–1461. doi: 10.3390/ejihpe11040103
Hermanto, Y. B., and Srimulyani, V. A. (2021). The challenges of online learning during the COVID-19 pandemic. Pendidikan Dan Pengajaran 54, 46–57. doi: 10.23887/jpp.v54i1.29703
Honey, P., and Mumford, A. (1989). Learning styles Questionnaire. Organization Design and Development, Incorporated. London: Peter Honer Publications Limited.
ITESM (2020). Working together due to COVID-19. Available online at: https://tec.mx/en/news/national/institution/tec-unam-and-other-universities-working-together-due-COVID-19 (accessed October 1, 2021).
Jaegler, A. (2022). How to measure inclusion in higher education: an inclusive rating. Sustainability 14, 8278. doi: 10.3390/su14148278
Jessee, S., O'Neill, P., and Dosch, R. (2006). Matching student personality types and learning preferences to teaching methodologies. J. Dent. Educ. 70, 644–651. doi: 10.1002/j.0022-0337.2006.70.6.tb04120.x
Jung, J., Horta, H., and Postiglione, G. (2021). Living in uncertainty: the COVID-19 pandemic and higher education in hong kong. Stud. High. Educ. 46, 107–120. doi: 10.1080/03075079.2020.1859685
Kaqinari, T., Makarova, E., Audran, J., Döring, A. K., Göbel, K., and Kern, D. A. (2022). Latent class analysis of university lecturers' switch to online teaching during the first COVID-19 lockdown: the role of educational technology, self-efficacy, and institutional support. Educ. Sci. 12, 607. doi: 10.3390/educsci12090607
Katsioloudis, P., and Fantz, T. D. (2012). A comparative analysis of preferred learning and teaching styles for engineering, industrial, and technology education students and faculty. J. Technol. Educ. 23, 61–69. doi: 10.21061/jte.v23i2.a.4
Keržič, D., Alex, J. K., Balbontín-Alvarado, R., Bezerra, D. D. S., Cheraghi, M., and Dobrowolska, B. (2021). Academic student satisfaction and perceived performance in the e-learning environment during the COVID-19 pandemic: evidence across ten countries. PLoS ONE 16, e0258807. doi: 10.1371/journal.pone.0258807
King, G., Murray, C., Salomon, J., and Tandon, A. (2004). Enhancing the validity and cross-cultural comparability of measurement in survey research. Am. Polit. Sci. Rev. 98, 191–207. doi: 10.1017/S000305540400108X
Kolb, D. A. (1976). Management and the learning process. Calif. Manag. Rev. 18, 21–31. doi: 10.2307/41164649
Kolb, D. A. (2014). Experiential Learning: Experience as the Source of Learning and Development. Upper Saddle River, NJ: Pearson Education.
Lorente-Rodríguez, M., and Pulido-Montes, C. (2022). Use of digital resources in higher education during COVID-19: a literature review. Educ. Sci. 12, 612. doi: 10.3390/educsci12090612
Mansor, M. S. A., and Ismail, A. (2012). Learning styles and perception of engineering students towards online learning. Procedia Soc. Behav. Sci. 69, 669–674. doi: 10.1016/j.sbspro.2012.11.459
Mayers, T., Mathis, B. J., Ho, C. K., Morikawa, K., and Hisatake, K. (2022). Factors affecting undergraduate medical science students' motivation to study during the COVID-19 pandemic. Educ. Sci. 12, 628. doi: 10.3390/educsci12090628
McLure, F., Koul, R., and Fraser, B. (2021). University students' classroom emotional climate and attitudes during and after COVID-19 lockdown. Educ. Sci. 12, 31. doi: 10.3390/educsci12010031
Mena, J. L., Rodríguez, J., Mena, J. A., Navarro, F. J. I., and Cabrera, J. S. (2019). Estilos de aprendizaje del alumnado de ingeniería: curso, rendimiento y género. Eur. J. Educ. Psychol. 12, 175–189. doi: 10.30552/ejep.v12i2.282
Muthuprasad, T., Aiswarya, S., Aditya, K., Girish, K., and Jha, G. (2021). Students' perception and preference for online education in india during covid -19 pandemic. Soc. Sci. Humanit. Open 3, 100101. doi: 10.1016/j.ssaho.2020.100101
Nancekivell, S. E., Shah, P., and Gelman, S. A. (2020). Maybe they're born with it, or maybe it's experience: toward a deeper understanding of the learning style myth. Educ. Psychol. 112, 221–235. doi: 10.1037/edu0000366
Narayan, R., Rodriguez, C., Araujo, J., Shaqlaih, A., and Moss, G. (2013). “Constructivism-constructivist learning theory,” in The Handbook of Educational Theories, eds B. Irby, G. Brown, R. Lara-Alecio, and S. Jackson (Washington, DC: Information Age Publishing), 169–183.
Nixon, E., Trickey, A., Christensen, H., Finn, A., Thomas, A., Relton, C., et al. (2021). Contacts and behaviours of university students during the COVID-19 pandemic at the start of the 2020/2021 academic year. Sci. Rep. 11, 1–13. doi: 10.1038/s41598-021-91156-9
OECD (2020). School Education During COVID-19: Were Teachers and Students Ready? Paris:OECD Publishing. Available online at: http://www.oecd.org/education/Mexico-coronavirus-education-country-note.pdf (accessed September 9, 2022).
Ogundari, K. (2023). Student access to technology at home and learning hours during COVID-19 in the U.S. Educ. Res. Policy Pract. 18, 1–18. doi: 10.1007/s10671-023-09342-7
Pawlowska, D. K., Westerman, J. W., Bergman, S. M., and Huelsman, T. J. (2014). Student personality, classroom environment, and student outcomes: a person-environment fit analysis. Learn. Individ. Differ. 36, 180–193. doi: 10.1016/j.lindif.2014.10.005
Peltonen, M., and Ruohotie, P. (1992). Learning Motivation: Theory, Studies and Examples of Willingness to Learn. Keuruu: Otava.
Perrow, C. (2013). The Next Catastrophe: Reducing Our Vulnerabilities to Natural, Industrial, and Terrorist Disasters. Luxembourg: Princeton University Press.
Petridou, E., Sarri, A., and Kyrgidou, L. P. (2009). Entrepreneurship education in higher educational institutions: the gender dimension. Gend. Manag. 24, 286–309. doi: 10.1108/17542410910961569
Pike, G. (2006). Students' personality types, intended majors, and college expectations: further evidence concerning psychological and sociological interpretations of holland's theory. Res. High. Educ. 47, 801–821. doi: 10.1007/s11162-006-9016-5
Portillo, J., Garay, U., Tejada, E., and Bilbao, N. (2020). Self-perception of the digital competence of educators during the COVID-19 pandemic: a cross-analysis of different educational stages. Sustainability 12, 10128. doi: 10.3390/su122310128
Quigley, M., Bradley, A., Playfoot, D., and Harrad, R. (2022). Personality traits and stress perception as predictors of students' online engagement during the COVID-19 pandemic. Pers. Individ. Differ. 194, 111645. doi: 10.1016/j.paid.2022.111645
Randall, K., Isaacson, M., and Ciro, C. (2017). Validity and reliability of the Myers-Briggs personality type indicator: a systematic review and meta-analysis. J. Best Pract. Health Prof. Divers. 10, 1–27.
Rapanta, C., Botturi, L., Goodyear, P., Guàrdia, L., and Koole, M. (2021). Online university teaching during and after the COVID-19 crisis: refocusing teacher presence and learning activity. Postdigital Sci. Educ. 2, 923–945. doi: 10.1007/s42438-020-00155-y
Rauschnabel, P. A., Krey, N., Babin, B. J., and Ivens2, B. S. (2016). Brand management in higher education: the university brand personality scale. J. Bus. Res. 69, 3077–3086. doi: 10.1016/j.jbusres.2016.01.023
Rodicio-García, M. L., Ríos-de Deus, M. P., Mosquera-González, M. J., and Penado Abilleira, M. (2020). La brecha digital en estudiantes españoles ante la crisis de la COVID-19. Rev. Int. Educ. Para Justicia Soc. 9, 103–125. doi: 10.15366/riejs2020.9.3.006
Rodrigues, A., Cerdeira, L., Machado-Taylor, M., and Alves, H. (2021). Technological skills in higher education-different needs and different uses. Educ. Sci. 11, 326. doi: 10.3390/educsci11070326
Sánchez, J., Trujillo Torres, J. M., Gómez, M., and Gómez, G. (2020). Gender and digital teaching competence in dual vocational education and training. Educ. Sci. 10, 84. doi: 10.3390/educsci10030084
Sandvik, L. V., Svendsen, B., Strømme, A., Smith, K., Sommervold, O. A., Angvik, S. A., et al. (2023). Assessment during COVID-19: students and teachers in limbo when the classroom disappeared. Educ. Assess. 28, 11–26. doi: 10.1080/10627197.2022.2122953
Sharma, A., and Alvi, I. (2021). Evaluating pre and post COVID 19 learning: an empirical study of learners' perception in higher education. Educ. Inf. Technol. 26, 7015–7032. doi: 10.1007/s10639-021-10521-3
Siemens, G. (2005). Connectivism: A Learning Theory for the Digital Age. PressBooks, Available online at: https://pressbooks.pub/lidtfoundations/chapter/connectivism-a-learning-theory-for-the-digital-age/ (accessed January 24, 2023).
Sipps, G., Alexander, R., and Friedt, L. (2016). Item analysis of the Myers-Briggs type indicator. Educ. Psychol. Meas. 45, 789–796. doi: 10.1177/0013164485454009
Stein, R., and Swan, A. B. (2019). Evaluating the validity of Myers-Briggs type indicator theory: a teaching tool and window into intuitive psychology. Soc. Personal. Psychol. Compass 13, e12434. doi: 10.1111/spc3.12434
Stewart, B. L., Goodson, C. E., and Miertschin, S. L. (2021). COVID-19 technology student success challenges: influence of tools and strategies computers in education journal. Comput. Educ. 12, 1–27.
Tennyson, R., and Rasch, M. (1998). Linking cognitive learning theory to instructional prescriptions. Instr. Sci. 17, 369–385. doi: 10.1007/BF00056222
Toto, G., and Limone, P. (2021). Motivation, stress and impact of online teaching on italian teachers during COVID-19. Computers 10, 75. doi: 10.3390/computers10060075
Vázquez-Sánchez, A., Cruz-Villar, C., Delgado, F., Chávez-Alcaraz, E., and Chong-Quero, J. (2021). Statistical modelling of factor analysis to set causals of hybrid learning success during COVID-19 lockdown. J. Phys. Conf. Series 1, 1–9. doi: 10.1088/1742-6596/2090/1/012072
Vergara-Rodríguez, D., Antón-Sancho, A., and Fernández-Arias, P. (2022). Variables influencing professors' adaptation to digital learning environments during the COVID-19 pandemic. Int. J. Environ. Res. Public Health 19, 3732. doi: 10.3390/ijerph19063732
Vieyra, A., Belden, M., de la Calle, J., and Martínez, A. (2020). The Impact of the COVID-19 Pandemic on Higher Education in Mexico, Colombia and Peru. London: EY-Parthenon Education sector, Ernst and Young LLP.
Wieman, C., and Gilbert, S. (2017). The teaching practices inventory: a new tool for characterizing college and university teaching in mathematics and science. CBE-Life Sci. Educ. 13, 552–569. doi: 10.1187/cbe.14-02-0023
Willingham, D., Hughes, E., and Dobolyi, D. (2015). The scientific status of learning styles theories. Teach. Psychol. 42, 266–271. doi: 10.1177/0098628315589505
Zapata-Garibay, R., González-Fagoaga, J. E., González-Fagoaga, C. J., Cauich-García, J. R., and Plascencia-López, I. (2021). Higher education teaching practices experience in Mexico, during the emergency remote teaching implementation due to COVID-19. Front. Educ. 6, 628158. doi: 10.3389/feduc.2021.628158
Keywords: higher education, COVID-19 transitions, engineering education, adaptation, inclusivity, academic performance, educational innovation
Citation: Vázquez-Sánchez A and Delgado F (2023) Educative performance transitions in engineering students through the COVID-19 pandemic. Front. Educ. 8:1156724. doi: 10.3389/feduc.2023.1156724
Received: 01 February 2023; Accepted: 17 August 2023;
Published: 01 September 2023.
Edited by:
M. Meghan Raisch, Temple University, United StatesReviewed by:
Abha Shree, Mizoram University, IndiaJosé-Antonio Marín-Marín, University of Granada, Spain
Copyright © 2023 Vázquez-Sánchez and Delgado. This is an open-access article distributed under the terms of the Creative Commons Attribution License (CC BY). The use, distribution or reproduction in other forums is permitted, provided the original author(s) and the copyright owner(s) are credited and that the original publication in this journal is cited, in accordance with accepted academic practice. No use, distribution or reproduction is permitted which does not comply with these terms.
*Correspondence: Francisco Delgado, ZmRlbGdhZG8mI3gwMDA0MDt0ZWMubXg=
†These authors have contributed equally to this work and share first authorship