- Faculty of Educational Sciences, University of Helsinki, Helsinki, Finland
Introduction: Epistemic cognition, which explores how knowledge is acquired, applied, and critically evaluated, is closely linked to learning. However, despite numerous studies from various perspectives, much remains to be learned about this essential and predominantly positive connection. Despite the positive connection between epistemic cognition and learning, epistemic measures have not been widely used in predicting students’ academic achievement. One possible reason for this is the difficulties in measuring personal epistemology. However, in the last decade, learning analytics has emerged as a field of study and practice with new means to collect data on different psychological constructs. This study focused on a learning analytics tool, a structured learning diary, that can support student learning while being used to record student thinking. This study explores the connection of student diaries with academic achievement, students’ epistemic beliefs’ connection with academic achievement, and the viability of implementing an epistemic belief questionnaire and a structured learning diary in a naturalistic degree program setting (N = 105).
Methods: Connections between these and academic achievement were investigated at four temporal measurement points. The first aim was to test which measures of the diary tool correlated with academic achievement. The second aim was to test epistemic beliefs’ correlation (Pearson correlation coefficient) with academic achievement. Models of linear regression were then designed and tested at different times. The overarching general aim of the study was to fill the gap in the use of reflective learning diaries in engineering education and epistemic beliefs in predicting academic achievement.
Results and discussion: The results show that we should collect student-originated learning process data for the best predictive power and connect that with independent psychological measures. Despite the significant effort required to use the learning diaries, the results indicate that with further design, digital journaling tools are viable learning and measurement tools to be used at scale and for long periods. Future studies should investigate the possibility implement epistemic measures with structured learning diaries more closely and study the connections between diary use and personal epistemology.
1. Introduction
Epistemic cognition examines how knowledge is gained, applied, and evaluated critically. This has been shown to be closely intertwined with learning. Despite the many studies from many perspectives, there is still much to learn about this mainly positive and essential connection. One aspect of epistemic cognition is the beliefs individuals have about knowledge. These beliefs shape and influence individuals’ epistemic cognition. In addition to seeing the nature of knowledge as important for learning, modern digital environments add extra challenge for everyone’s higher-order thinking skills. Learners and citizens, in general, need critical thinking skills to make justified decisions in this complex world from scattered pieces of information. Further, digital learning tools and environments offer new methods for collecting trace data that could be used to measure conceptual change.
Abundant empirical evidence has shown a connection between personal epistemology and learning (e.g., Hofer and Pintrich, 1997; Muis, 2008; Greene et al., 2010, 2018). While the relationship has typically been perceived as linear, more precisely, the better the academic achievement, the more sophisticated epistemic beliefs (Rodríguez and Cano, 2006), this positive connection has been challenged. In their study on physics students, Redish et al. (1998) revealed that one semester of university studies did not significantly result in more sophisticated views of their learning; students remained binary thinkers, thinking that the knowledge comes from authoritative sources. Further, Köller et al. (2000) found that those students who studied longer and more intensively had stronger beliefs that the truth can be reached in physics. Since similar results have been reported by Kienhues et al. (2008), who studied epistemological changes between two methods of teaching instruction: refutational and informational. They found unexpectedly, in both groups, that the learners labeled as sophisticated learners shifted towards more naïve. Refutational teaching has been designed to give the opposite results. Even so, in their meta-analysis of 132 nonexperimental studies, Greene et al. (2018) found epistemic cognition correlating positively with academic achievement. In sum, the link between epistemic beliefs and learning is tight and mostly positive, yet, as Mason and Bromme (2010) state, “the nature of this relationship is still unclear.”
Factors contributing to academic performance have been studied for at least a century (Hellas et al., 2018). In their systematic literature review of 565 scientific articles since 2010, Hellas et al. (2018) found that the most used predictive values were course grades (or ranges), exam scores, program or module graduation, or retention. Grade point average (GPA, or range of GPA) was also one of the most predicted measures. The features most often used for predicting academic performance ranged from pre-course, and course performance to different demographic (gender, age, family) features to personality, self-regulation, and engagement measures. Behavioral data (log data) was one of the features mentioned in the study (2018). However, epistemic cognition was not mentioned.
Structured learning diaries are systematic and organized records or journals where individuals document and reflect upon their learning experiences, progress, goals, and strategies, typically following a predefined format or template. These tools can double as interventions, e.g., through self-monitoring and measurement tools (Kivimäki et al., 2023). In fact, the use of such tools can enhance academic achievement. In their meta-analysis, Dignath et al. (2023) found self-monitoring tools effecting academic achievement moderately (d = 0.42). They (Dignath et al., 2023) found that the most effective tools were those that had been incorporated in shorter studies and promoted monitoring of learning content and learning behavior while stimulating metacognitive monitoring.
The usefulness of academic performance, or academic achievement, depends on how these measures, such as course grades or module graduation, are operationalized in the institution. In the context of this study, the participating university awards the highest grade for every student who fully (or almost) meets all the predefined learning outcomes. The university is rewarded with funding based on the number of degrees finished in normative time (Kivimäki and Meriluoto, 2018). Therefore, it can be argued that GPA and the number of courses (or ECTS credits) completed are relevant student outcomes.
As mentioned before, epistemic cognition was not one of the features found in the systematic literature review (2018). Nevertheless, epistemic cognition, i.e., “how people acquire, understand, justify, change, and use knowledge in formal and informal contexts” (Greene et al., 2016), has been studied quite extensively concerning academic achievement (Greene et al., 2018). Still, there is much to learn from this mostly positive connection between the psychological epistemic construct and performance. This study explores the connections between diary data (learning process data), epistemic beliefs (self-report questionnaires), and academic performance.
1.1. Related literature
Structured learning diaries have been typically designed to foster reflective learning. These differ from regular learning diaries in utilizing standardized questions that students answer repeatedly over a period of time. Structured learning diaries and more traditional learning diaries have been successfully used as intervention tools and instruments of measurement (Schmitz and Wiese, 2006; Schmitz and Perels, 2011; Kawalkar and Vijapurkar, 2015; Broadbent et al., 2020; Bellhäuser et al., 2021). The focus of these studies has been on self-regulated learning. Further, structured learning diaries have been argued to be the third wave in measurement (SRL), as they combine log data with students’ self-report data (Panadero et al., 2016). Much less attention has been given to the connection between these diaries and epistemic cognition.
Epistemic cognition has been seen as an essential psychological construct related to other metacognitive constructs. As Pieschl et al. (2013) summarize studies in the field: “[−-] these studies show superior SRL [self-regulated learning] processes and outcomes for learners with sophisticated epistemic beliefs, namely, main effects of epistemic beliefs.” These types of connections are essential. However, as Hofer (2016) points out, epistemic cognition has been mainly studied as one construct related to other constructs, “seldom used by others as an additional explanatory variable in broader studies.”
Epistemic beliefs can be seen as a foundation for epistemic cognition. Individuals’ epistemic beliefs shape their approach to learning and knowledge acquisition. Thus, the epistemic beliefs can be seen as a window to personal epistemology; or example, “valuing metacognition may indicate an attempt to determine whether one can justify one’s beliefs, whereas valuing collaborative knowledge construction may indicate a new, increasingly sociocultural epistemic view” (Lonka et al., 2021, 789). These beliefs influence how individuals interpret and evaluate information, what sources of knowledge they trust, and how they make sense of new ideas or conflicting viewpoints.
In their meta-analysis of 132 nonexperimental studies, Greene et al. (2018) found that epistemic cognition correlated positively with academic achievement. The correlation was relatively small (r = 0.162, p < 0.001) but an important one. They found varying effect sizes over different measures used to capture epistemic cognition. These measures are typically self-report questionnaires, which have been criticized on the reliability and validity of the measures, response bias, and the fact that students may encounter the terminology used for the first time in the questionnaire they are being measured by Hofer and Sinatra (2010), and that epistemic cognition might not be enacted at the time of the measure (Kelly, 2016). Despite the critique, self-report measures are still commonly used in the research literature, and, as Sandoval et al. (2016) point out, “people can self-report best about thoughts and attitudes that are explicit in their minds and that require little construction in the moment.” In addition, Likert scales are biased to measure certain or simple knowledge over source of knowledge and justification of knowledge, as the two latter are hard to quantify and thus are studied more commonly in qualitative studies (Lonka et al., 2021).
The epistemic belief scales used in this study have been build on the multidimensional models developed Schommer (Schommer, 1990, 1993). In Greene et al. (2018), the closest comparable scales were Schommer/Schommer-Aikins scales which were found to deliver a statistically significant effect size of 0.144 (p < 0.001) in predicting academic achievement. Further, the specific scale of certain knowledge had 0.136 effect size (p < 0.001), and general epistemic cognition, where authors had summed sub-scales into one measure, had an effect size of 0.266 (p < 0.01).
The diary template employed achievement emotions from the control-value theory, which suggests that students’ emotions can be improved by enhancing their sense of competence and control in academic tasks and results while also influencing their evaluation of the importance of these activities and outcomes (Pekrun, 2006). Emotions, especially positive emotions, have been found to be connected with academic achievement (Oriol-Granado et al., 2017; Rodríguez-Muñoz et al., 2021). On the other hand, Pekrun has raised the complexity of some of the emotions, such as anxiety, that can trigger positive correlations to enjoyment and motivation (Pekrun et al., 2002). Factors like experiencing challenges, confusion, or difficulties can make students more resilient and thus become better learners. On the other hand, experiencing too many or severe difficulties can make students more passive towards their studies. Nevertheless, an experience of difficulty has been seen as a relevant factor close to affect (emotions) (Lodge et al., 2018) and an area of interest in this study. The emotion, the competence, and the difficulty assessed repeatedly by the student, should empower them to enhance their learning while promoting (meta-) reflective learning.
This study corroborates the self-report questionnaires with learning process data collected with structured learning diaries. Despite being a (possibly) positive intervention promoting academic achievement, the effect of the structured learning diaries is not the focus of this study. In addition, the validity and reliability of the measures used regarding what they were initially intended to measure is not the primary concern of this study. As criticized earlier, the epistemic belief questionnaires capture only some aspects of personal epistemology. This study is more inclined to explore using these measures’ capability to predict academic achievement. This study aims to increase our understanding of designing or developing tools for collecting relevant data that is possible to collect and analyze at scale and to predict student achievement, which we will discuss further in the next section.
1.2. Present study
In an earlier study (Kivimäki, 2022b), the author examined the connection between learning process data (structured learning diary) and epistemic beliefs (questionnaires) to academic achievement (register data). The rich diary data was defined to include only the structured item scales (competence, difficulty, and feeling) that are most suitable for the quantitative explorative purposes of this study. Textual diary data, i.e., topic-level comments and student-drawn relations between courses and topics, require qualitative approaches, which is not the focus of this study. This paper introduces a new research question related to user activity and consequently introduces relevant new data, methods, and analysis.
Based on the literature and prior research with the structured learning diary, the following research questions (RQ) and hypotheses (H) were formulated:
RQ1: Are the structured item entries in the diaries connected with academic achievement?
H1: Literature suggests (Greene et al., 2018) a connection (possibly through self-regulation), but the connection is weak or non-existent.
RQ2: Are students’ epistemic beliefs connected with academic achievement?
H2: Literature (Greene et al., 2018) suggests a weak connection that varies between different belief measures.
RQ3: How does a theory-based expert model perform compared to a statistical regression model as a forced model over time?
H3: Predictive power (effect size) is better when diary data is combined with epistemic beliefs. The statistical model will outperform the theory-based model. Possible connection diminishes over time due to the dynamic nature of the student’s learning patterns.
RQ4: Is implementing epistemic belief questionnaires and the diary process viable in degree programs?
This overarching research question is a design-driven question and does not come with a hypothesis. The empirical results will be combined here with log data observations to seek signals on the viability of making students fill out the questionnaires and use the diaries throughout their two-year master’s degree endeavor. What can we induce from this data, and how should these findings affect future designs of such data collection and student activation processes?
2. Materials and methods
2.1. Participants
This study is based on an entire cohort (N = 105) of new master’s degree students in a large European research university. All but one agreed to participate in this study. The study was designed to accommodate a randomized controlled trial to measure structured learning diary tool use and the diaries’ effect on self-regulated learning and engagement (Pesonen et al., 2020). The experiment group (n = 70) used the tool in the first semester in autumn, and the control group (n = 34) used the tool in the spring. This study focuses only on the experiment group’s data for temporal coherence.
The students randomly selected for the experiment group were predominantly male (63 male, seven female), which is typical in engineering. Partipants’ median age was 24, and the average was 25 years at the start of the study. The youngest participants were 22, and four students were over 30 years. All students gave their informed consent at the beginning of the study. Consent was reaffirmed at every point of data collection.
The participants answered the first questionnaire (pre-test) at the start of the academic year. Students answered the same questionnaire again at the end of the autumn semester (post-test). Participants were asked to submit their current version of the learning diary weekly during the first semester. A total of 64 students returned their diary at least once. Hence, the total number of participants in this study is 64.
Participants were not given any incentives to take part in this study. The structured learning diary was integrated with the degree program curriculum for that year. The use of the diary was, therefore, compulsory, but participating in the study was voluntary and participants could opt-out at any time.
2.2. Procedure
A pre-test questionnaire was opened during the orientation week for new students. The questionnaire was closed before the first course lectures. The second questionnaire, the post-test questionnaire, was opened at the end of the first semester.
Participating students had yet to use structured learning diary before. They were only trained to use the software and submit their weekly diaries. Students were instructed to keep updating the same file but renaming it weekly to represent the latest week number and submit the current version each week. The instructions were shared on the course home page. Students were reminded three times during the semester to keep submitting their weekly diaries: the first week, the fourth week, and two weeks before the end of the experiment.
Structured learning diary data were aggregated at the group level and visualized on a public dashboard. The dashboard was designed to motivate using the learning diary. With this, students could see how others have experienced the courses and course topics (Figure 1).
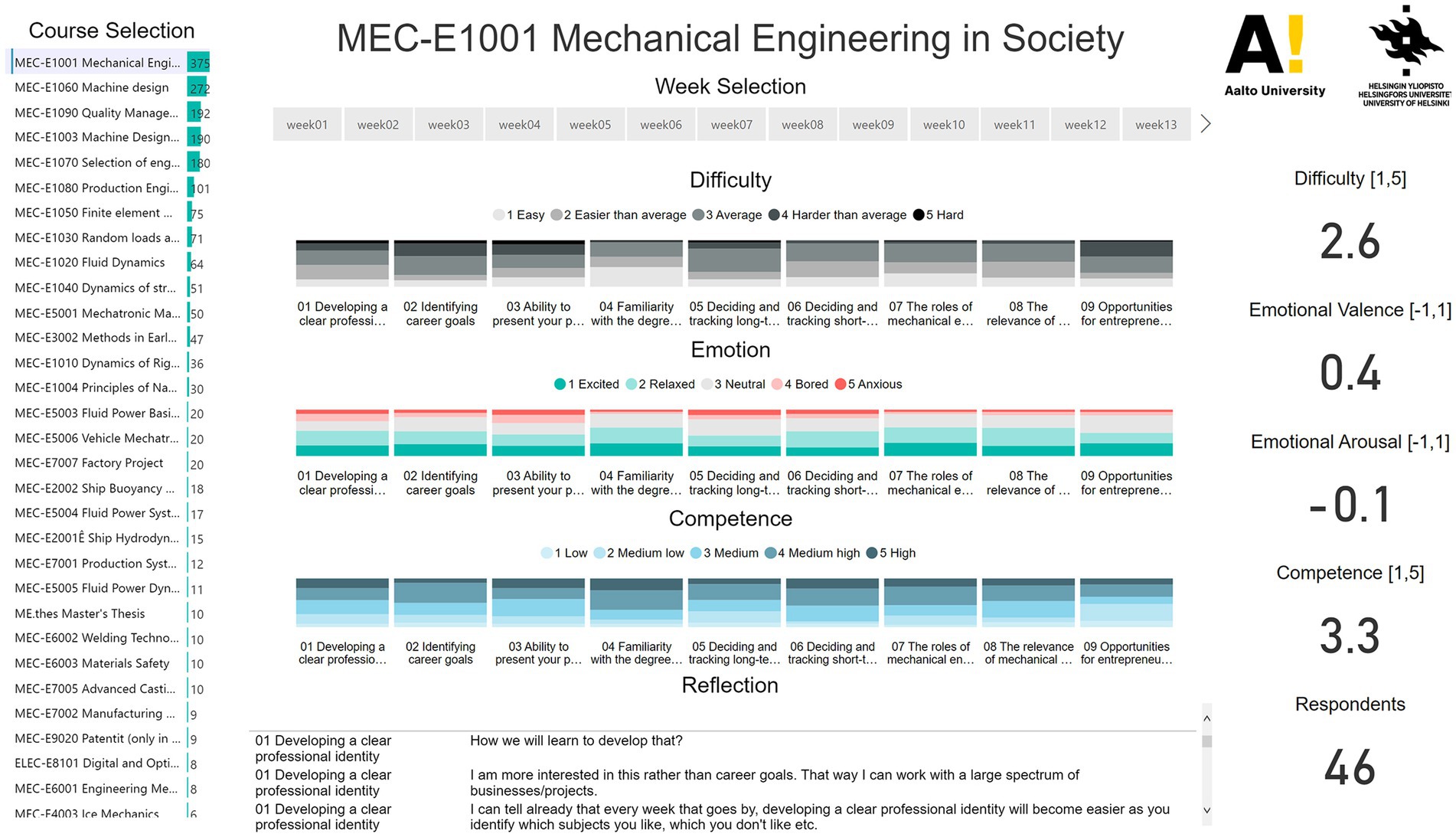
Figure 1. Example dashboard view based on aggregated diary data showing distributions of students’ difficulty, emotion, and competence reflections.
After the experiment period, the teachers in the participating degree program were interviewed on their notions related to their instructional designs during the experiment. Teachers reported that they had yet to have any significant pedagogical experiments. Teaching instruction methods were typical to engineering teaching: lectures, assignments, group work, exams, and exercises.
2.3. Data and instruments
2.3.1. Structured learning diary
Kivimäki et al. (2019) designed this study’s structured learning diary tool. This study focuses on the competence, emotion, and difficulty scales explained in Figure 1. Participant data were collected during the first semester (autumn).
The structured learning diary template was built by two researchers, extracting the degree program structure and course topics from the official study guide and course catalog. The topics were further validated with the teachers on each course (limited to the autumn semester).
Participants were given the structured learning diary template. The template included a hierarchical visual representation of students’ degree program, including all the major subject possibilities with courses of each study field and typically 5–7 course topics. The author and degree program specialist created the course topics. The topics were derived from the course descriptions revised by each course’s teacher. Students were instructed to update the diary each week or as often as they felt meaningful. Students’ diaries were collected once a week during the 14-week autumn semester.
Students’ weekly submissions were summarized at an individual level depicting, e.g., the overall count of course topics for which students had selected, e.g., the emotion excited, hard difficulty, and medium competence. Five measures of each category: emotion, difficulty, and competence, were used. In addition, a total measure of each diary item was used. Further, for each diary item, an average value was calculated. The averages were calculated using numerical scales (1 to 5 for competence and difficulty; −2 to +2 for emotions). In total, 19 measures were based on the content of the learning diaries (Figure 2).
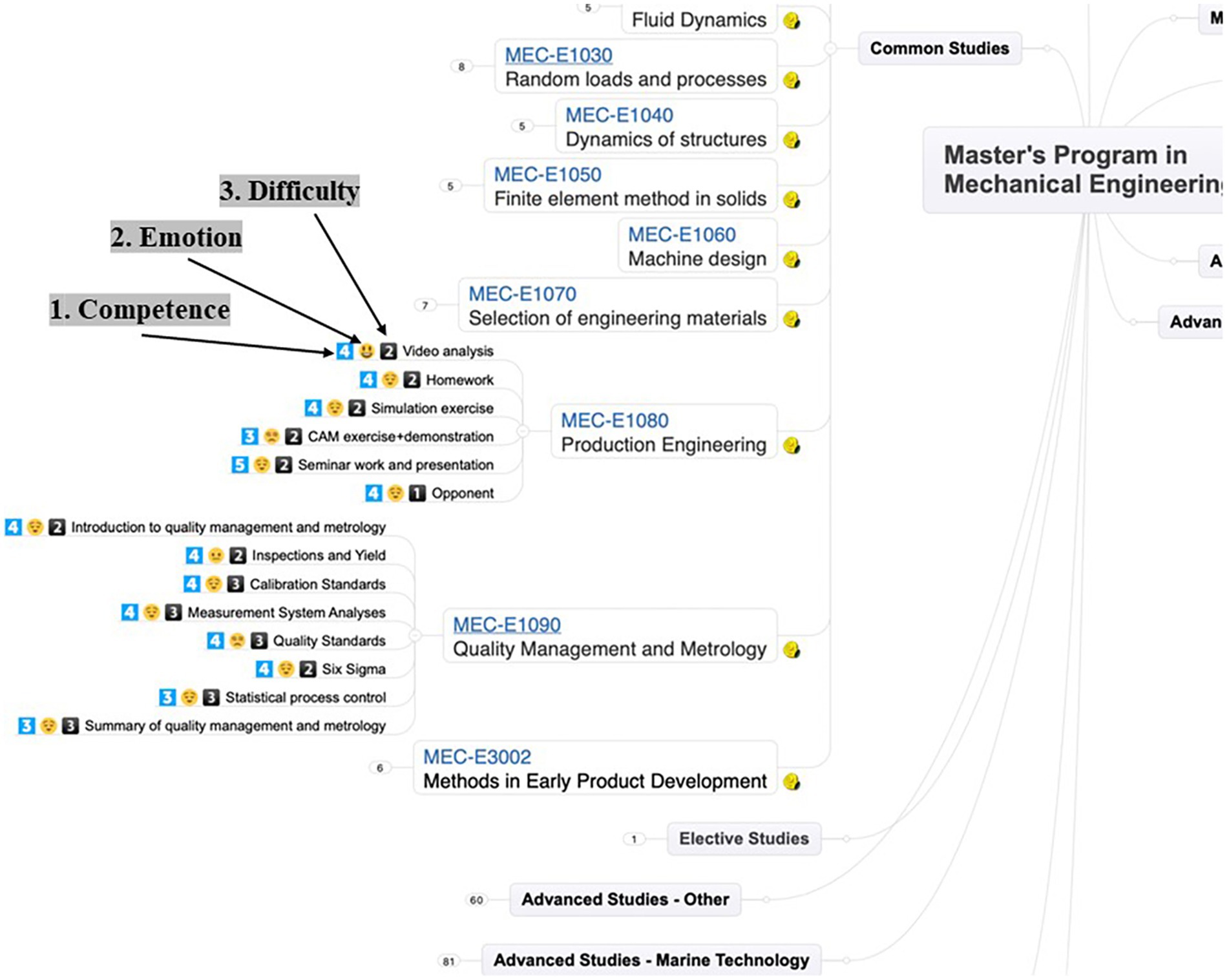
Figure 2. Excerpt from a learning diary with all main diary elements. The learning diary template consists of the degree program as a center node that breaks down into study units (e.g., majors, minors, and electives), courses, and course-level topics. On this topic level, student can click 1. competence (selectable scale:1 = low, 2 = medium low, 3 = medium, 4 = medium high, 5 = high), 2. emotion (student selects between excited, relaxed, neutral, bored, and anxious), and 3. difficulty (selectable scale: 1 = easy, 2 = easier than average, 3 = average, 4 = harder than average, 5 = hard).
For this study, a set of five achievement emotions (Pekrun, 2006) were selected, two positives, and two negatives. One of these was selected to be activating, and the other passive. Excited was selected as activating positive, relaxed positive and passive, bored as negative and passive, and anxious as negative and activating. Also, a neutral option was offered for students. These emotions were presented to all participants and offered as both text and visual emoticons in the diary template and its instructions in the same way as the competence and the difficulty scales. The emoticons were selected three researchers and further validated by several students, who were asked whether the proposed emoticons expressed written emotions. Competence and difficulty scales were the same as in the structured learning diary presented in Kivimäki et al. (2019).
Diary data used in the current study was extracted programmatically from the diaries. Example data is available at a group level (Kivimäki, 2022a). The data has been grouped by the number of students attained credits during their first academic year to maintain the anonymity of the participants. Students were able to enrich the template openly. However, only the interaction with the original template’s tree of nodes was extracted and aggregated for this study. For example, if a student started a new tree of nodes or added, e.g., floating comments, that content was not extracted from the diaries.
Weekly participation activity was recorded throughout the first semester. Students submitted their learning diaries into the Moodle learning management system as logged-in users. The time and user ID were recorded by Moodle. Students had been instructed to name their files to match the week number the diary represented. Based on the submission time, user ID, and file naming, each submitted file was assigned a pseudonymized research ID and week. This data was used to measure the submissions by each participant and the number of submissions received each week.
Diary-based variables were aggregated on the student level for the whole semester as the longitudinal within-student changes are not the focus in the present study. In addition, a total number of student-originated entries and an average for each item (emotion, competence, difficulty) was calculated.
2.3.2. Questionnaire measures
Epistemic beliefs were measured using a set of scales from the MED NORD questionnaire (Lonka et al., 2008). The survey (Table 1) consisted of several measures, including self-regulation, learning approach, and motivation. The self-report online questionnaire comprised 60 statements with 5, 6, and 7-point scales. Volunteers tested the time needed to answer the questionnaire to be 15 to 20 min. The present study employs the questionnaire’s epistemic belief scales: certain knowledge, collaborative knowledge building, practical value, reflective learning, and valuing metacognition. These were measured with a 6-point Likert scale (1 = completely disagree, 6 = completely agree).
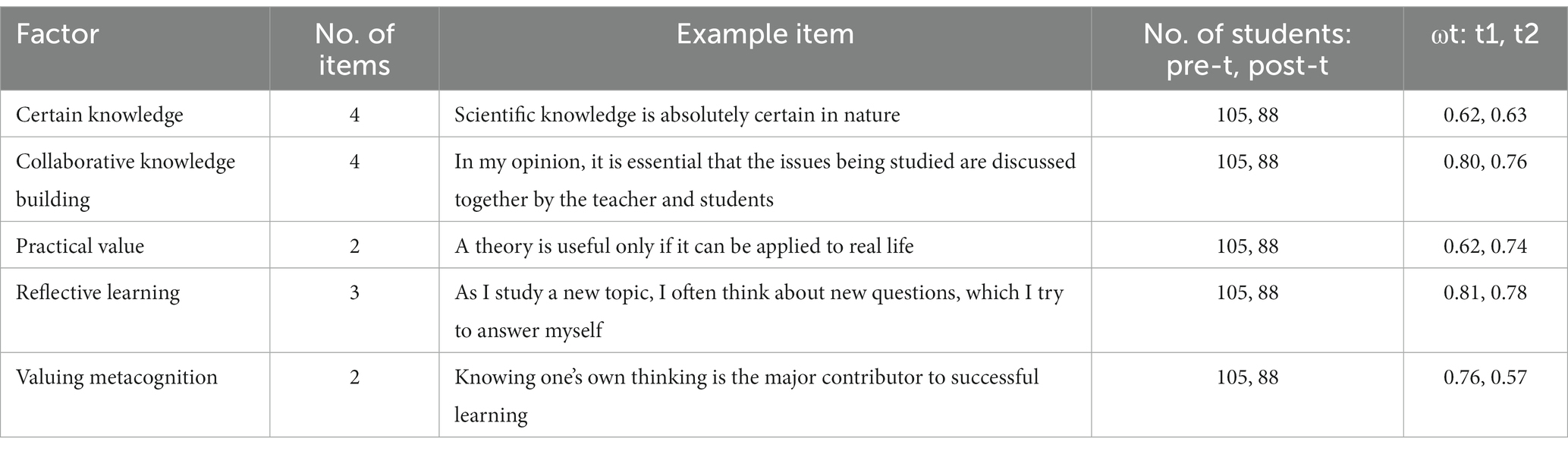
Table 1. Questionnaire scales, participants in each questionnaire time (t), and McDonald’s Omega (ωt).
All students in the master’s program were asked to participate in the pre-test (pre-t) and post-test (post-t). The scales’ reliability was measured with McDonald’s Omega (McDonald, 1999). All scales were acceptable apart from the post-test valuing metacognition (ω = 0.57). The personal identification code was used to match the survey results with students’ personal learning diaries.
2.3.3. Registry data
The student registry was used to measure academic achievement. This study used grade point average (GPA) and the number of ECTS credits (CR).
The study registry service calculates the GPA. The service calculates the grade averages weighted by the number of credits of each course. The grade point average is similar to the plain grade average value as the courses in the participating organization were designed to be primarily 5 ECTS each, with only a few exceptions.
2.4. Statistical analyzes
Pearson correlation was used to calculate the correlation between the dependent (academic achievement) and independent variables (diary data, epistemic belief scales). Multiple linear regression was used to model the relationship between academic achievement and the diary and epistemic belief variables. This study uses forward selection and stepwise selection methods, i.e., statistical regression (Tabachnick and Fidell, 2014), to find the variable that best predicts the dependent variable (GPA and CR).
A mixed approach was designed to increase the validity and reliability of this study. First, two competing models were built. One model was created based on literature, combining variables from the diary tool with epistemic beliefs. The second model was built stepwise in SPSS software to find the best predictive model between all the variants. Second, the models were tested to predict academic achievement at three times for the GPA and CR dependent variables, i.e., 12 tests were performed.
3. Results
3.1. Diary submission activity
A total of 64 students (91%) out of the 70-person experiment group submitted at least one version of them learning diary. Histogram (Figure 3) shows that 43 students (67%) of the 64 participants returned their diaries weekly during the first semester. Only 15 students (23%) missed more than 1 week’s submission.
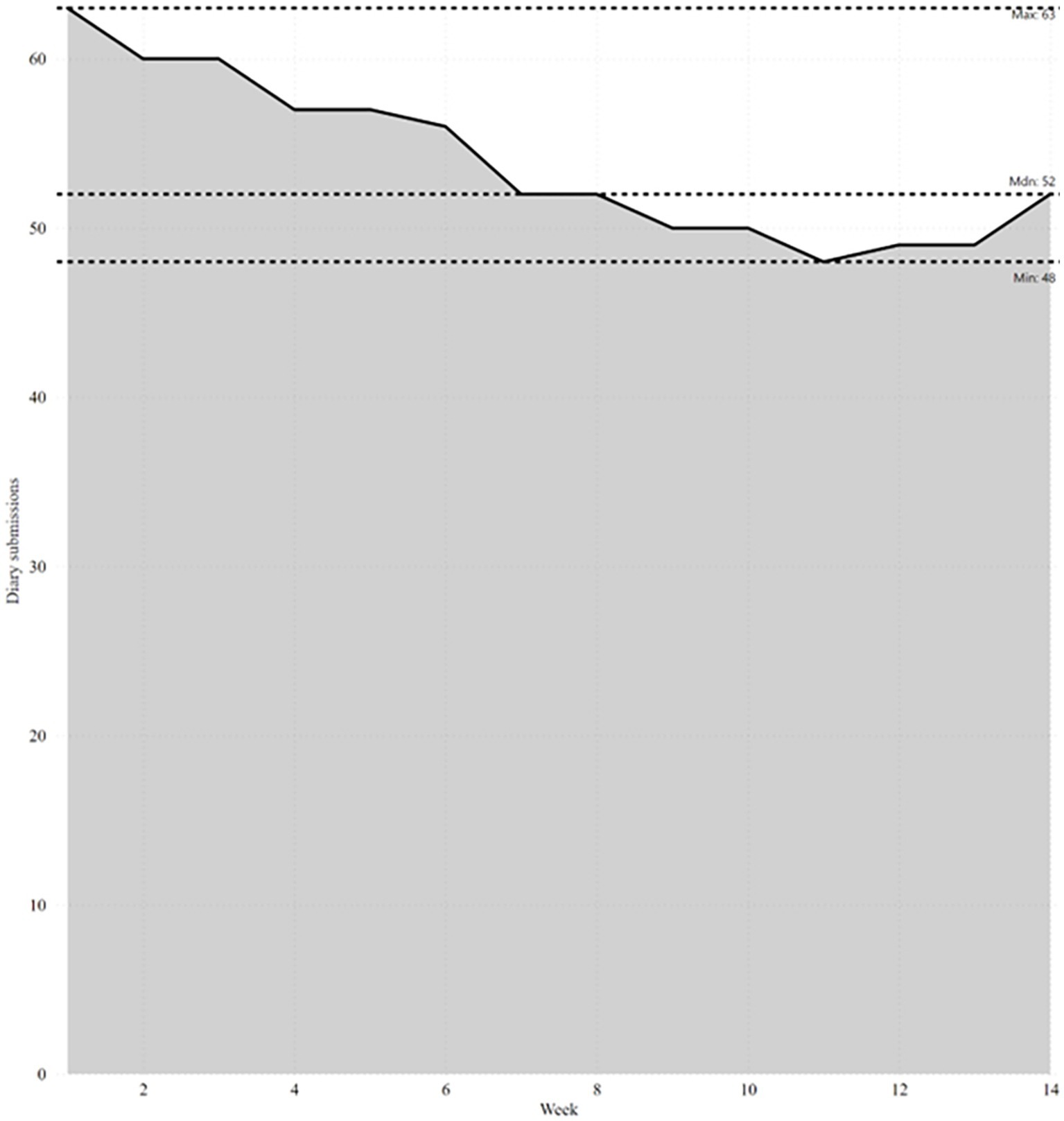
Figure 3. The number of participants’ diary submissions per week (Mdn: 52, Max: 63, Min: 48, SD: 4.67).
Further participant-level analysis showed that one student started them submissions after the first week. Ten students discontinued their submissions before the end of the experiment. Ten students missed one or more weekly submission schedules but continued with submissions later.
Weekly submission review (Figure 4) shows that students’ submission activity steadily decreased throughout the semester, showing only slight increase towards the end of the semester.
3.2. Correlation coefficients: diary scales and submission activity
Academic achievement variables, the grade point average and the number of attained credits correlated statistically significantly (Table 2), r(62) = 0.293, p = 0.019. Students’ higher value of competence correlated with higher GPA statistically significantly, r(60) = 0.288, p = 0.023. The averages of emotion and difficulty did not correlate with the academic achievement variables. However, these variables correlated positively, r(60) = −0.251, p = 0.049, i.e., negative emotions associated with harder course topics. Moreover, more positive emotions associate with higher competence, r(60) = 0.353, p = 0.005, linking all the diary items together. The submission activity did not correlate statistically significantly with other variables.
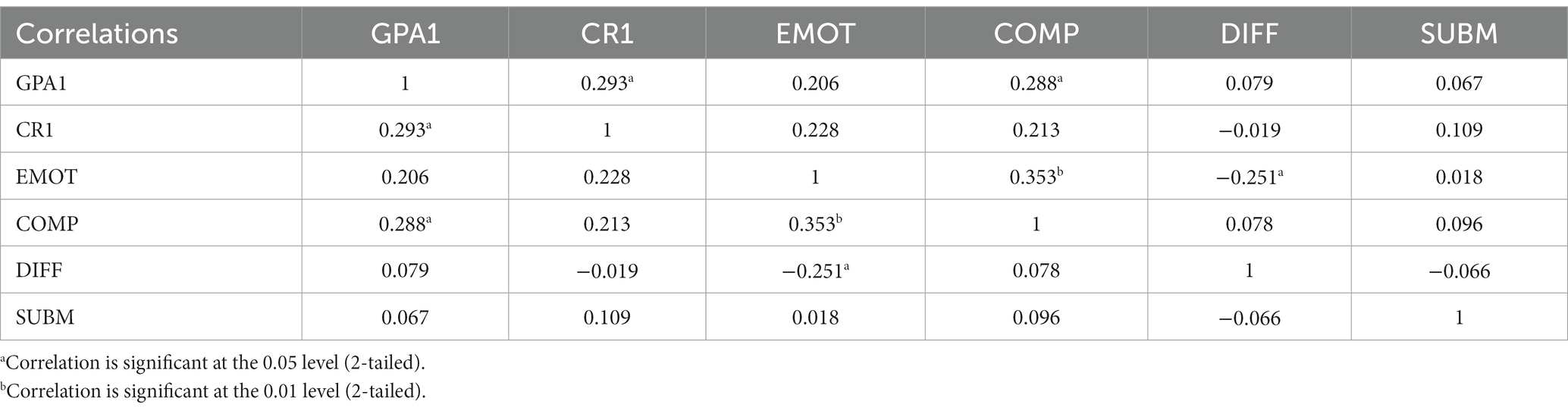
Table 2. Pearson correlation matrix: first autumn grade point average (GPA1), first autumn credits (CR1), emotion (EMOT), competence (COMP), difficulty(DIFF), and diary submission percentage (SUBM).
None of the single emotion items correlated statistically significantly with GPA or CR (Table 3). Still, the number of total emotion selections correlated positively with the first autumn GPA, r(60) = 0.284, p = 0.025. Students who assessed their competence at the medium high level (Table 3), resulted in a positive correlation with the autumn GPA, r(58) = 0.314, p = 0.015. The total number of competence selections correlated positively with GPA, r(60) = 0.283, p = 0.026. Average difficulty (Table 3), i.e., a number 3 selection on the 1–5 scale, had a statistically significant positive correlation with GPA, r(60) = 0.281, p = 0.027. Like in other scales, the total number of difficulty selections had a statistically significant positive correlation with GPA, r(60) = 0.283, p = 0.026. Hard difficulty experiences resulted in a significant positive correlation with CR, r(34) = 0.373, p = 0.025.
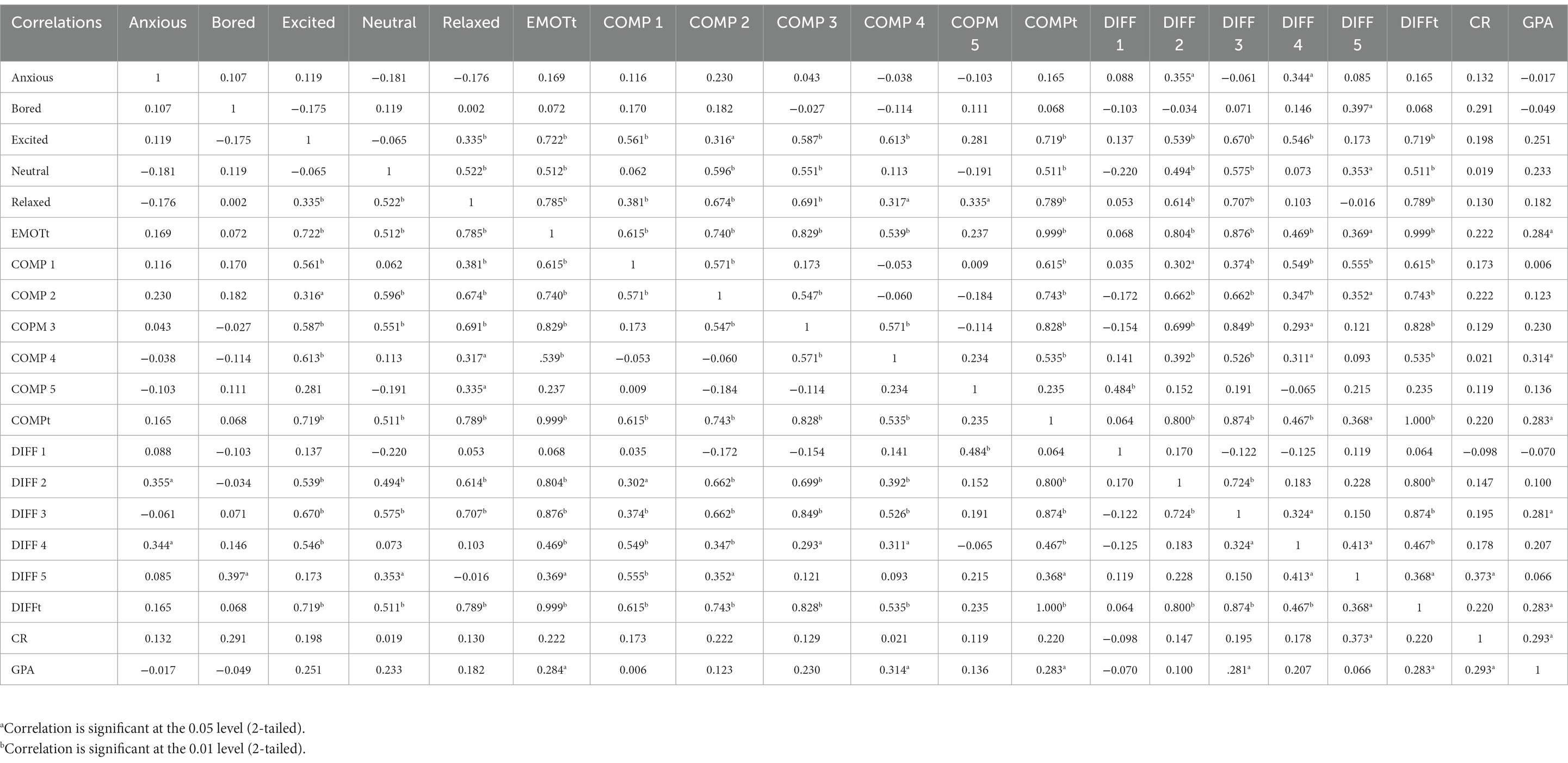
Table 3. Correlation coefficients from diary: five emotions and emotions total (EMOTt), competence variables 1–5 and the competence total (COMPt), difficulty variables 1–5 and the difficulty total (DIFFt), and academic achievement variables: study attainments (CR = credits), and grade point average (GPA).
3.3. Correlation coefficients: epistemic beliefs
The pre-test epistemic beliefs did not reach a statistically significant correlation with GPA (Table 4). However, all the post-test epistemic belief scales positively correlated with GPA: reflective learning r(64) = 0.363, p = 0.003; collaborative knowledge building r(62) = 0.335, p = 0.007, valuing metacognition r(62) = 0.350, p = 0.005; certain knowledge r(62) = 0.388, p = 0.002; and practical value r(62) = 0.290, p = 0.020. Certain knowledge and practical value scales in the pre-test positively correlated with CR: r(62) = 0.347, p = 0.005; r(62) = 0.324, p = 0.009, respectively. All post-test epistemic belief scales in the post-test questionnaire reached statistically significant correlation with CR: reflective learning r(62) = 0.324, p = 009, collaborative knowledge building r(62) = 0.270, p = 0.031; valuing metacognition r(62) = 0.281, p = 0.025; certain knowledge r(62) = 0.430, p < 0.001, practical value r(62) = 0.334, p = 0.007.
3.4. Model 1: theory-based expert model
Based on the literature and correlation coefficients, the theory-based linear regression models were designed for GPA and CR. The first model consisted of medium-high competence, average difficulty, excitement (although it did not reach statistical significance), and all post-test epistemic beliefs for GPA. Another model consisting of excited emotion, hard difficulty, certain knowledge (pre- and post-test), and practical value (post-test) was designed for CR. The positive emotions were strong candidates based on the literature. These were dropped from the model due to low correlation coefficients. Observations that were further than two standard deviations from the predicted values were handled as outliers. The GPA model did not reach statistical significance. For the CR model, a significant regression equation was found (F(4, 31) = 5.612, p = 0.002), with an R2 of 0.420. Participants’ predicted CR is equal to −5.937 – 0.317 (practical value) + 5.484 (post-test certain knowledge) + 3.819 (pre-test certain knowledge) + 0.242 (hard difficulty). The number of CR increased by 0.242 ECTS credits for each hard difficulty selected, increased by a higher certain knowledge measure, and decreased when students believed more in bringing theory into practice. None of the independent variables in the model reached statistical significance. Based on this finding, the pre-test certain knowledge (p = 0.224) and practical value (p = 0.889) were removed from the model.
After this statistical iteration, the new model consisted of hard difficulty and post-test certain knowledge, which reached a statistically significant regression equation as (F(2, 33) = 10.530, p < 0.001), with an R2 of 0.353. Participants’ predicted CR equals 4.647 + 0.262 (hard difficulty) + 6.155 (certain knowledge). Participants’ CR number increases by 0.262 for every hard difficulty selection and 6.155 for those who believe in certain knowledge.
3.5. Model 2: statistical regression model
The model to predict GPA was formulated with the forward and stepwise procedures, which produced the same result, a model consisting of three independent variables: medium-high competence, neutral emotion, and hard difficulty. A significant regression equation was found (F(3,12) = 9.239, p = 0.002), with an R2 of 0.698. Participants’ predicted GPA is equal to 2.115–0.020 (hard difficulty) + 0.016 (neutral emotion) + 0.008 (medium-high competence). GPA increased by 0.008 for each medium-high selection, 0.016 for the neutral emotion selection, and the GPA was 0.020 better for those who expressed less hard difficulty in their diary. Medium high competence, neutral emotion, and hard difficulty were significant predictors of GPA.
The second model was formulated following the same procedure to predict CR. The best model consisted of the post-test certain knowledge scale and neutral emotion. A significant regression equation was found (F(2, 13) = 13.508, p < 0.001), with an R2 of 0.675. Participants’ predicted CR equals −41.347 + 0.220 (neutral emotion) + 13,969 (certain knowledge), where neutral emotion is measured as a number of times selected in a student’s diary, and certain knowledge is measured as a summarized Likert-scale multiple-item variable from 1 to 6. CR increased by 13.969 for each number of certain knowledge measures, and neutral emotion selection increased CR by 0.220 credits. Both certain knowledge and neutral emotion were significant predictors of CR.
3.6. Model testing
The regression equation models formulated by theory and statistical selection were tested with a forced (enter) method at three times of measure (autumn, 1st year, 2nd year). Each model’s related effect size (R square) was calculated, and the independence of the independent variables for all statistically significant models was tested for collinearity (condition index less than 10 in SPSS collinearity diagnostics). A linear relationship, residual normality, and homoscedasticity were visually reviewed from scatter plots and histograms. Autocorrelation was analyzed with the Durbin-Watson test, which showed no problems in autocorrelation in the models used (d close to 2).
Four multiple linear regression models were tested (Table 5) with the autumn data as forced (enter procedure in SPSS) models. The theory-based expert model for GPA prediction consisted of medium high competence, average difficulty, excited emotion, and all post-test epistemic belief independent variables. Theory-based CR models consisted of hard difficulty and post-test certain knowledge. The statistical model predicting GPA consisted of medium high competence, neutral emotion, and hard difficulty. The statistical CR model was based on a post-test certain knowledge scale and neutral emotion.
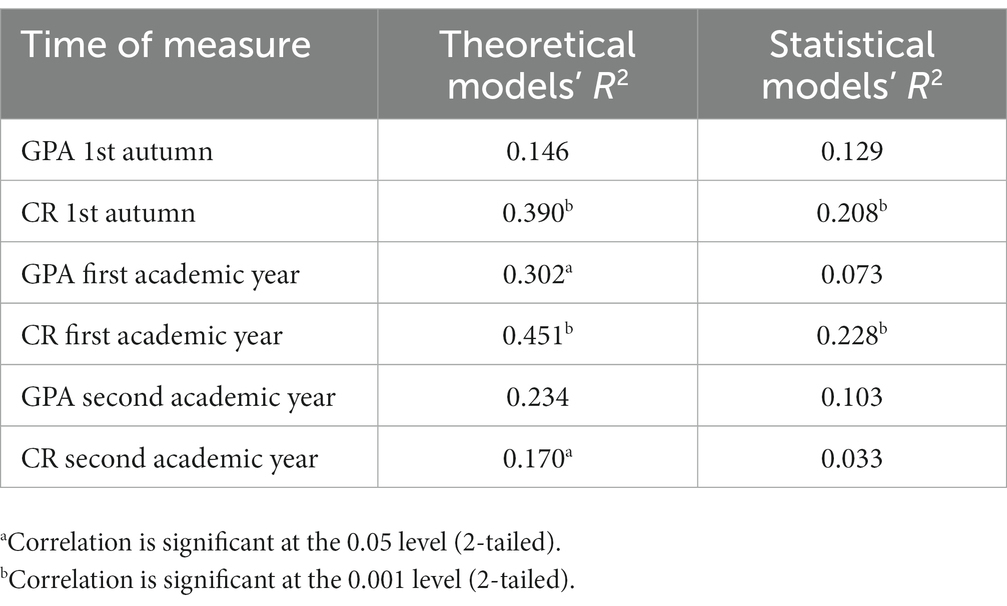
Table 5. The R squares of the linear multiple regression models used to predict the first autumn. first academic year. and second academic year grade point average (GPA) and the number of credits (CR).
4. Discussion
The first research question asked whether the structured items in the diaries were connected with academic achievement. The hypothesis was that there was a connection. All the dimensions were statistically significantly positively correlated with GPA regarding the total selections (Table 3). This suggests that the correlation was mainly higher activity-based since more active (engaged) students also achieved more in their studies. Still, medium-high competence as a single variable correlated the most (0.314) with the best GPA. This, along with the positive average difficulty correlation (0.281), suggests that in addition to the overall activity, there is a more profound content-based correlation between the scales used and student GPA. Students who had expressed more hard difficulty in their autumn studies resulted in them completing more ECTS than their colleagues, or at least there was a significant correlation.
The second research question and hypothesis concerned possible connections between epistemic beliefs and academic achievement. Interestingly, none of the pre-test beliefs correlated statistically significantly with the GPA (Table 4). In contrast, all the post-test beliefs correlated positively with GPA. Similarly, all the post-test beliefs correlated with CR. In addition, certain knowledge and practical value reached a statistically significant positive correlation with CR. Findings align with the literature (Greene et al., 2018), where the general epistemic belief scales and certain knowledge as a single scale correlated positively with academic achievement.
The hypothesis suggested that the statistical model would outperform the theory-based expert model to the third research question. Results are dichotomous. The statistical regression model suggested that over 65% of the academic achievement variance was explainable with our independent variables. However, when the model was tested over time and fitted as a forced model, compared with the theory-based model, the statistical model hardly delivered any reasonable predictive power. The step-by-step statistical procedures (likewise forward and backward procedures) have been criticized for failing to deliver reproducible results (Austin and Tu, 2004). The result of this study is in line with the criticism.
Moreover, when the model was tested with students’ later academic achievement, the R square rapidly lost statistical significance. In turn, the theory-based CR model succeeded in predicting over 45% (p < 0.001) of the first year’s CR variance and maintained statistically significant predictive power over the second-year CR as well (Table 5). However, the connection with the second-year CR is likely better linked with the first-year CR. This relation was not controlled in this study.
A statistically significant correlation was found between diary entries and the epistemic beliefs. A linear regression model based on step-by-step statistical procedures promised a high R square but failed to deliver meaningful predictive power against the theory-based model. The theory-based expert model combining epistemic belief and diary content delivered a meaningful R2. Results suggest that predictions related to CR should consider mixing different types of independent variables, e.g., learning diary data and questionnaires related to a psychological construct. The predictive power diminishes over time. This can be due to the dynamic nature of students behavior and their learning experiences. One solution is to predict using measures that students update periodically. Implementing a structured learning diary as part of the study path is another suitable method to consider.
Earlier study (Pesonen et al., 2020) suggests that active use of a structured learning diary is related to keeping up the study dedication and academic achievement. Passive use of the tool relates to lower study dedication and academic achievement. The tool use divided the students in engagement and academic achievement, a vital notion that could be used in further design of the tool. The study found that the structured learning diary was ineffective as an (SRL) intervention tool. This aligns with other studies (Fabriz et al., 2014; Dörrenbächer and Perels, 2016). Positive effects on students’ strategy use were observed when the diaries were combined with training (Broadbent et al., 2020). Despite the challenges in intervention studies, learning diaries function as support measurement tools. This study suggests that the content of a structured learning diary can be used to predict academic achievement, especially when corroborated with questionnaires.
This study explored the possibility of using deeper content of the learning diaries as measures to feed prediction models (RQ1, RQ2, RQ3). Prediction model is only a viable product if it can be implanted into a naturalistic setting. Research question 4 was about the viability of implementing the methods used in this study in a real study environment. Given the fact that this study was conveyed in an authentic learning environment and the software used to author the learning diary was a new element for the participants, it demonstrates the fluency of master’s level engineering students in adopting and integrating new tools into their learning processes. A total of 64 students (91%) used the tool and were able to submit their diary. Of them, all but two (89%), used the tool for more than one week, while 77% used it throughout the semester (13–14 submissions). Moreover, the decline in the participation activity also occured in the questionnaire activity. 64 students (91%) from the 70-student experiment group answered the post-test questionnaire. That number is expected to keep declining over a longer period, and the number of repeated questionnaires. Based on the findings, further designs of learning diaries should consider combining diaries with research-based questionnaire scales, e.g., epistemic beliefs, that thematically fit nicely with learning diaries.
Despite the rather positive adoption of the tool, it proved to be demanding. The weekly submissions declined throughout the semester from 63 (week 1) to 48 (week 11). The slight increase in the number of submissions at the end of the semester is most likely related to the e-mail prompt sent to all participating students (week 12). The effort needed to keep up doing this journaling task likely resulted in 10 students discontinuing their weekly submissions. Moreover, the other ten students missing some weeks but continuing the submissions later could result from mixed feelings. These students could be motivated to continue with the experiment but find the significant effort required by the learning diary task to be too heavy on them. This has also been noted in other studies (Klug et al., 2011; Pesonen et al., 2020).
More focus should be put on selecting the diary items carefully (Schmitz and Wiese, 2006), making the learning potential explicit (Panadero et al., 2016), combining the diary task with relevant training, e.g., on self-regulation (Fabriz et al., 2014; Otto and Kistner, 2017; Broadbent et al., 2020), and providing (automatic) feedback for students (Bellhäuser et al., 2023). One idea for future development, raised by the findings of this study, is to increase the prompts of updating the diary, but at the same time, remind students that they only need to update the diary only once a week. Moreover, this could be incorporated in the instructional design of the degree program.
4.1. Limitations and future directions
This study comes with limitations. Main limitations are related to the research environment and the selection process of the theory-based expert model.
As the naturalistic learning environment is a strength in this study, it also generates many uncontrolled variables that might have affected the results of this study. New students in the master’s program could feel outside pressure to do as they are asked even though they were volunteering to participate in this study and were always able to opt out of the study. Students might have just clicked something to do the task and keep up with the submission, rather than thinking reflectively. Teachers on several courses that students spread out to study during the experiment period could have prompted students about the learning diaries unevenly. Teachers were not instructed on this. After the experiment, teachers in the participating master’s program reported that they had not conducted any overlapping pedagogical experiments during this study.
The theory-based expert model was selected based on literature and personal, possibly biased, educational science expertise by researchers and engineering education teachers. Expert view was discussed with two other educational science experts. Still, the selections made are based on the personal views of only a few experts and the affordance of the data availability: the available items and scales in the structured learning diary and the epistemic belief scales that cannot be seen as purely epistemic and the Likert scale measure method focuses on measuring beliefs of certain or simple knowledge.
Due to the relatively small sample (N = 70), no causal inferences can be made from the results.
Future studies on structured learning diaries and epistemic beliefs should be investigated. How could epistemic cognition be measured while using learning diaries? Another type of questionnaires should be tested to investigate further how epistemic cognition interplays with structured learning diaries. When developed further, integrating epistemic cognition measurement with learning diaries could inform teachers of the progress in student’s epistemic thinking and give them opportunities to react. At a degree program level, the epistemic awareness of each program could be measured and reacted accordingly. This is worth pursuing.
4.2. Implications
Earlier empirical studies have demonstrated a close relationship between monitoring strategies and more sophisticated epistemic cognition (Bromme et al., 2010). Despite the connection between self-regulated learning strategies and epistemic cognition, the structured learning diaries have been one-sided so far. This study sheds some light on how the structured learning diaries and epistemic cognition correlate with academic achievement.
Students had been customed to answer regular questionnaires but had yet to use structured learning diaries before. Therefore, the inferences based on the student activity data can be helpful to further designs of learning diaries. The results showed a decrease in the submission activity. This should inform other designs of such tools. The research on the use of reflective diaries in engineering education is abundant (Tanner, 2012; Wallin and Adawi, 2018). This study contributes as a case study of integrating such a diary at degree program level, while other research focuses on the course level.
For practice, this study stresses the need for making theory-based prediction models rather than using out-of-the-box-ready analytics tools that are based on statistical regression models.
5. Conclusion
This two-phase study first explored the connection of two student-originated variables, structured learning diaries, and epistemic beliefs, to academic achievement (RQ1, RQ2). In the second phase, two competing regression models were created, a theory-based expert model and a purely statistical regression model (RQ3). The models were compared to student achievement at three measure points. Academic achievement was explored through grade-point average (GPA) and the number of credits (CR). An overarching research question (RQ4) of this study was to investigate the viability of implementing a learning diary and a questionnaire into the instructional design of a degree program.
The results showed correlations between structured learning diaries and academic achievement and epistemic beliefs and academic achievement. Both, the expert designed and the statistical regression model did claim to predict academic achievement in terms of the number of credits. However, only the theory-based expert model delivered statistically significant prediction to the GPA and excelled to deliver small but promising predictive power over the number of the second-year CR.
The student activity measured in a naturalistic learning environment reveals that the effort needed to update the learning diary is too demanding for some. Still, most of the students succeeded in building a routine to weekly update and submit their diary and answer the questionnaires.
The results of this study show promise in further designs of structured learning diary -tools. The implementation of such tools is plausible, and among many other affordances, these tools can be used as measurement tools to feed predictive analytics at scale. While doing that, the diary approach fostering self-monitoring can yield better academic achievement. Again, by further developing structured learning diaries, e.g., by integrating the diaries with automatic feedback, the effects could be enhanced.
Data availability statement
The original contributions presented in the study are included in the article/Supplementary material, further inquiries can be directed to the author.
Ethics statement
Ethical review and approval was not required for the study on human participants in accordance with the local legislation and institutional requirements. The patients/participants provided their written informed consent to participate in this study.
Author contributions
The author confirms being the sole contributor of this work and has approved it for publication.
Acknowledgments
The content of this manuscript has been presented in part at the 28th International Congress of European University Information Systems (EUNIS 2022) (Kivimäki, 2022b).
Conflict of interest
The author declares that the research was conducted in the absence of any commercial or financial relationships that could be construed as a potential conflict of interest.
Publisher’s note
All claims expressed in this article are solely those of the authors and do not necessarily represent those of their affiliated organizations, or those of the publisher, the editors and the reviewers. Any product that may be evaluated in this article, or claim that may be made by its manufacturer, is not guaranteed or endorsed by the publisher.
Supplementary material
The Supplementary material for this article can be found online at: https://www.frontiersin.org/articles/10.3389/feduc.2023.1153618/full#supplementary-material
References
Austin, P. C., and Tu, J. V. (2004). Automated variable selection methods for logistic regression produced unstable models for predicting acute myocardial infarction mortality. J. Clin. Epidemiol. 57, 1138–1146. doi: 10.1016/j.jclinepi.2004.04.003
Bellhäuser, H., Dignath, C., and Theobald, M. (2023). Daily automated feedback enhances self-regulated learning: a longitudinal randomized field experiment. Front. Psychol. 14:1125873. doi: 10.3389/fpsyg.2023.1125873
Bellhäuser, H., Mattes, B., and Liborius, P. (2021). Daily fluctuations in motivation. Z. Entwicklungspsychol. Padagog. Psychol. 51, 228–242. doi: 10.1026/0049-8637/a000226
Broadbent, J., Panadero, E., and Fuller-Tyszkiewicz, M. (2020). Effects of mobile-app learning diaries vs online training on specific self-regulated learning components. Educ. Technol. Res. Dev. 68, 2351–2372. doi: 10.1007/s11423-020-09781-6
Bromme, R., Kienhues, D., and Porsch, T. (2010). Who Knows What and Who Can We Believe? Epistemological Beliefs are Beliefs About Knowledge (Mostly) to be Attained from Others. Cambridge University Press. Cambridge.
Dignath, C., Van Ewijk, R., Perels, F., and Fabriz, S. (2023). Let learners monitor the learning content and their learning behavior! A Meta-analysis on the effectiveness of tools to Foster monitoring. Educ. Psychol. Rev. 35:2. doi: 10.1007/s10648-023-09718-4
Dörrenbächer, L., and Perels, F. (2016). Self-regulated learning profiles in college students: their relationship to achievement, personality, and the effectiveness of an intervention to foster self-regulated learning. Learn. Individ. Differ. 51, 229–241. doi: 10.1016/j.lindif.2016.09.015
Fabriz, S., Dignath-Van Ewijk, C., Poarch, G., and Büttner, G. (2014). Fostering self-monitoring of university students by means of a standardized learning journal—a longitudinal study with process analyses. Eur. J. Psychol. Educ. 29, 239–255. doi: 10.1007/s10212-013-0196-z
Greene, J. A., Cartiff, B. M., and Duke, R. F. (2018). A meta-analytic review of the relationship between epistemic cognition and academic achievement. J. Educ. Psychol. 110, 1084–1111. doi: 10.1037/edu0000263
Greene, J. A., Muis, K. R., and Pieschl, S. (2010). The role of epistemic beliefs in students’ self-regulated learning with computer-based learning environments: conceptual and methodological issues. Educ. Psychol. 45, 245–257. doi: 10.1080/00461520.2010.515932
Greene, J. A., Sandoval, W. A., and Bråten, I. (2016). Handbook of epistemic. Cognition. doi: 10.4324/9781315795225
Hellas, A., Ihantola, P., Petersen, A., Ajanovski, V. V., Gutica, M., Hynninen, T., et al. Predicting academic performance: a systematic literature review. Proceedings companion of the 23rd annual ACM conference on innovation and Technology in Computer Science Education, 2018-07-02. (2018).
Hofer, B. K. (2016). “Epistemic cognition as a psychological construct” in Handbook of epistemic cognition. eds. J. A. Greene, W. A. Sandoval, and I. Bråten (New York: Routledge)
Hofer, B. K., and Pintrich, P. R. (1997). The development of epistemological theories: beliefs about knowledge and knowing and their relation to learning. Rev. Educ. Res. 67, 88–140. doi: 10.3102/00346543067001088
Hofer, B. K., and Sinatra, G. M. (2010). Epistemology, metacognition, and self-regulation: musings on an emerging field. Metacogn. Learn. 5, 113–120. doi: 10.1007/s11409-009-9051-7
Kawalkar, A., and Vijapurkar, J. (2015). Aspects of teaching and learning science: what students’ diaries reveal about inquiry and traditional modes. Int. J. Sci. Educ. 37, 2113–2146. doi: 10.1080/09500693.2015.1067933
Kelly, G. J. (2016). Methodological Considerations for the Study of Epistemic Cognition in Practice. Handbook of Epistemic Cognition. Routledge. New York
Kienhues, D., Bromme, R., and Stahl, E. (2008). Changing epistemological beliefs: the unexpected impact of a short-term intervention. Br. J. Educ. Psychol. 78, 545–565. doi: 10.1348/000709907X268589
Kivimäki, V. (2022a). Learning Diary Emotions, Competence Self-Assessment and Difficulty Experience Connected with Epistemic Beliefs. Zenodo Genèva
Kivimäki, V. (2022b). “Predicting academic achievement with students’ learning diary and epistemic beliefs” in Proceedings of EUNIS 2022 – The 28th international congress of European university information systems. eds. J.-F. Desnos, R. Yahyapour, and R. Vogl, vol. 86 (Göttingen: EUNIS), 168–177.
Kivimäki, V., Ketonen, E. E., and Lindblom-Ylänne, S. (2023). Engineering students’ justifications for their selections in structured learning diaries. Front. Educ. 8:1223732. doi: 10.3389/feduc.2023.1223732
Kivimäki, V., and Meriluoto, S. (2018). “Holistic perspective to individual study plan: personal development project plan” in Transforming Our World Through Design, Diversity and Education: Proceedings of Universal Design and Higher Education in Transformation Congress 2018. STUDIES in Health Technology and Informatics. eds. G. Craddock, C. Doran, L. McNutt, and D. Rice, vol. 256 (Amsterdam: IOS PRESS), 514–526.
Kivimäki, V., Pesonen, J., Romanoff, J., Remes, H., and Ihantola, P. (2019). Curricular concept maps as structured learning diaries: collecting data on self-regulated learning and conceptual thinking for learning analytics applications. J. Learn. Anal. 6, 106–121. doi: 10.18608/jla.2019.63.13
Klug, J., Ogrin, S., Keller, S., Ihringer, A., and Schmitz, B. (2011). A plea for self-regulated learning as a process: modelling, measuring and intervening. Psychol. Sci. 53:51.
Köller, O., Baumert, J., and Neubrand, J. (2000). “Epistemologische Überzeugungen und Fachverständnis im Mathematik- und Physikunterricht” in TIMSS-III. Dritte Internationale Mathematik- und Naturwissenschaftsstudie. Mathematische und naturwissenschaftliche Bildung am Ende der Schullaufbahn. 2. Mathematische und physikalische Kompetenzen am Ende der gymnasialen Oberstufe. ed. J. Baumert (Opladen: Leske u. Budrich)
Lodge, J. M., Kennedy, G., Lockyer, L., Arguel, A., and Pachman, M. (2018). Understanding difficulties and resulting confusion in learning: an integrative review. Front. Educ. (Lausanne) 3:49. doi: 10.3389/feduc.2018.00049
Lonka, K., Ketonen, E., and Vermunt, J. D. (2021). University students’ epistemic profiles, conceptions of learning, and academic performance. High. Educ. 81, 775–793. doi: 10.1007/s10734-020-00575-6
Lonka, K., Sharafi, P., Karlgren, K., Masiello, I., Nieminen, J., Birgegård, G., et al. (2008). MED NORD-A tool for measuring medical students’ well-being and study orientations. Med. Teach. 30, 72–79. doi: 10.1080/01421590701769555
Mason, L., and Bromme, R. (2010). Situating and relating epistemological beliefs into metacognition: studies on beliefs about knowledge and knowing. Metacogn. Learn. 5, 1–6. doi: 10.1007/s11409-009-9050-8
Muis, K. R. (2008). Epistemic profiles and self-regulated learning: examining relations in the context of mathematics problem solving. Contemp. Educ. Psychol. 33, 177–208. doi: 10.1016/j.cedpsych.2006.10.012
Oriol-Granado, X., Mendoza-Lira, M., Covarrubias-Apablaza, C.-G., and Molina-López, V.-M. (2017). Positive emotions, autonomy support and academic performance of university students: the mediating role of academic engagement and self-efficacy. Revista de Psicodidáctica 22, 45–53. doi: 10.1387/RevPsicodidact.14280
Otto, B., and Kistner, S. (2017). Is there a Matthew effect in self-regulated learning and mathematical strategy application? - assessing the effects of a training program with standardized learning diaries. Learn. Individ. Differ. 55, 75–86. doi: 10.1016/j.lindif.2017.03.005
Panadero, E., Klug, J., and Järvelä, S. (2016). Third wave of measurement in the self-regulated learning field: when measurement and intervention come hand in hand. Scand. J. Educ. Res. 60, 723–735. doi: 10.1080/00313831.2015.1066436
Pekrun, R. (2006). The control-value theory of achievement emotions: assumptions, corollaries, and implications for educational research and practice. Educ. Psychol. Rev. 18, 315–341. doi: 10.1007/s10648-006-9029-9
Pekrun, R., Goetz, T., Titz, W., and Perry, R. P. (2002). Academic emotions in students’ self-regulated learning and achievement: a program of quantitative and qualitative research. Educ. Psychol. 37, 91–105. doi: 10.1007/s10648-006-9029-9
Pesonen, J. A., Ketonen, E. E., Kivimäki, V., and Ihantola, P. (2020). Does Using Structured Learning Diaries Affect Self-Regulation or Study Engagement?: An Experimental Study in Engineering Education. In 2020 IEEE Frontiers in Education Conference (FIE 2020), Uppsala, 2020. Frontiers in Education Conference: Proceedings, IEEE, [S.I], IEEE Frontiers in Education Conference, Uppsala, Sweden, 21/10/2020.
Pieschl, S., Stahl, E., and Bromme, R. (2013). Adaptation to Context as Core Component of Self-Regulated Learning: The Example of Complexity and Epistemic Beliefs. International Handbook of Metacognition and Learning Technologies. Springer New York.
Redish, E. F., Saul, J. M., and Steinberg, R. N. (1998). Student expectations in introductory physics. Am. J. Phys. 66, 212–224. doi: 10.1119/1.18847
Rodríguez, L., and Cano, F. (2006). The epistemological beliefs, learning approaches and study orchestrations of university students. Stud. High. Educ. 31, 617–636. doi: 10.1080/03075070600923442
Rodríguez-Muñoz, A., Antino, M., Ruiz-Zorrilla, P., and Ortega, E. (2021). Positive emotions, engagement, and objective academic performance: a weekly diary study. Learn. Individ. Differ. 92:102087. doi: 10.1016/j.lindif.2021.102087
Sandoval, W. A., Greene, J. A., and Bråten, I. (2016). Understanding and promoting thinking about knowledge: origins, issues, and future directions of research on epistemic cognition. Rev. Res. Educ. 40, 457–496. doi: 10.3102/0091732x16669319
Schmitz, B., and Perels, F. (2011). Self-monitoring of self-regulation during math homework behaviour using standardized diaries. Metacogn. Learn. 6, 255–273. doi: 10.1007/s11409-011-9076-6
Schmitz, B., and Wiese, B. S. (2006). New perspectives for the evaluation of training sessions in self-regulated learning: time-series analyses of diary data. Contemp. Educ. Psychol. 31, 64–96. doi: 10.1016/j.cedpsych.2005.02.002
Schommer, M. (1990). Effects of beliefs about the nature of knowledge on comprehension. J. Educ. Psychol. 82, 498–504. doi: 10.1037/0022-0663.82.3.498
Schommer, M. (1993). Comparisons of beliefs about the nature of knowledge and learning among postsecondary students. Res. High. Educ. 34, 355–370. doi: 10.1007/BF00991849
Tanner, K. D. (2012). Promoting student metacognition. CBE Life Sci. Educ. 11, 113–120. doi: 10.1187/cbe.12-03-0033
Keywords: structured learning diary, epistemic beliefs, prediction, academic achievement, engineering education, higher education
Citation: Kivimäki V (2023) Structured learning diary and epistemic beliefs predict academic achievement in higher education. Front. Educ. 8:1153618. doi: 10.3389/feduc.2023.1153618
Edited by:
Tom Rosman, Leibniz-Institute for Psychology (ZPID), GermanyReviewed by:
Tore Ståhl, Tampere University, FinlandHenrik Bellhäuser, Johannes Gutenberg University Mainz, Germany
Copyright © 2023 Kivimäki. This is an open-access article distributed under the terms of the Creative Commons Attribution License (CC BY). The use, distribution or reproduction in other forums is permitted, provided the original author(s) and the copyright owner(s) are credited and that the original publication in this journal is cited, in accordance with accepted academic practice. No use, distribution or reproduction is permitted which does not comply with these terms.
*Correspondence: Ville Kivimäki, dmlsbGUua2l2aW1ha2lAaGVsc2lua2kuZmk=