- 1Institute of Education, Universität Hildesheim, Hildesheim, Germany
- 2Department of Educational Psychology, Rutgers University, New Brunswick, NJ, United States
- 3Biology Education, Faculty of Biology, Ludwig-Maximilians-Universität München, Munich, Germany
- 4Physics Education, Faculty of Physics, Ludwig-Maximilians-Universität München, Munich, Germany
Diagnostic competences of teachers are an essential prerequisite for the individual support of students and, therefore, highly important. There is a substantial amount of research on teachers’ diagnostic competences, mostly operationalized as diagnostic accuracy, and on how diagnostic competences may be influenced by teachers’ professional knowledge base. While this line of research already includes studies on the influence of teachers’ content knowledge (CK), pedagogical content knowledge (PCK), and pedagogical-psychological knowledge (PK) on the diagnosis of subject-specific knowledge or skills, research on the diagnosis of cross-domain skills (i.e., skills relevant for more than one subject), such as scientific reasoning, is lacking although students’ scientific reasoning skills are regarded as important for multiple school subjects (e.g., biology or physics). This study investigates how the accuracy of pre-service teachers’ diagnosis of scientific reasoning is influenced by teachers’ own scientific reasoning skills (one kind of CK), their topic-specific knowledge (i.e., knowledge about a topic that constitutes the thematic background for teaching scientific reasoning; which is another kind of CK), and their knowledge about the diagnosis of scientific reasoning (one kind of PCK) and whether the relationships between professional knowledge and diagnostic accuracy are similar across subjects. The design of the study was correlational. The participants completed several tests for the kinds of professional knowledge mentioned and questionnaires for several control variables. To ensure sufficient variation in pre-service teachers’ PCK, half of the participants additionally read a text about the diagnosis of scientific reasoning. Afterwards, the participants completed one of two parallel video-based simulations (depicting a biology or physics lesson) measuring diagnostic accuracy. The pre-service teachers’ own scientific reasoning skills (CK) were a statistically significant predictor of diagnostic accuracy, whereas topic-specific knowledge (CK) or knowledge about the diagnosis of scientific reasoning (PCK), as manipulated by the text, were not. Additionally, no statistically significant interactions between subject (biology or physics) and the different kinds of professional knowledge were found. These findings emphasize that not all facets of professional knowledge seem to be equally important for the diagnosis of scientific reasoning skills, but more research is needed to clarify the generality of these findings.
1. Introduction
Teachers’ abilities to assess students’ knowledge or skills, i.e., their diagnostic competences, play a crucial role in successful teaching (Helmke and Schrader, 1987; Ready and Wright, 2011) because specific and individually targeted support of a student’s learning process can only be achieved if the student’s current cognitive skill levels are continuously diagnosed (Schrader, 2009). Therefore, diagnostic competences also influence student learning, at least indirectly (Behrmann and Souvignier, 2013; Urhahne and Wijnia, 2021). For the purpose of diagnosing their students, teachers need to rely on the continuous observation of students’ performance on tasks (e.g., tasks designed for fostering a specific skill in students) within lessons to gather relevant information that they consequently use for the diagnosis of their students’ knowledge or skills. The assessment often takes place during interactions between students and teachers (see Furtak et al., 2016) and, therefore, usually happens on-the-fly. Because of their importance, diagnostic competences (e.g., Chernikova et al., 2020a), as well as specific diagnostic activities (e.g., Bauer et al., 2020), have been studied in many different contexts, but so far, most research on teachers’ diagnostic competences has focused on subject-specific knowledge or skills or students’ overall academic ability (see Südkamp et al., 2012); while important cross-domain skills such as scientific reasoning (e.g., Fischer et al., 2018) are rarely investigated. This lack of consideration seems especially puzzling because of the broader relevance of cross-domain skills for multiple school subjects and, as a consequence, the broader potential impact of research on teachers’ diagnostic competences concerning these cross-domain skills. Overall, research on teachers’ diagnostic competences is often focused on diagnostic accuracy as an indicator of diagnostic competences and the analysis of various factors that might influence diagnostic accuracy (e.g., Hoge and Coladarci, 1989; Südkamp et al., 2012).
1.1. Teachers’ diagnostic competences
1.1.1. Structure and indicators of teachers’ diagnostic competences
In a school context, diagnosing can be characterized as the “goal-oriented collection and integration of case-specific information [e.g., information about learners’ performance] to reduce uncertainty in order to make educational decisions” (Heitzmann et al., 2019, p. 4). This collection and integration of information can be difficult because, typically, learner characteristics are not directly observable and have to be inferred from learners’ activities. Therefore, teachers have to rely on (acquired) diagnostic competences during various diagnostic situations (Heitzmann et al., 2019), which may enable them to formulate adequate diagnoses. According to Heitzmann et al. (2019), diagnostic competences are considered to comprise the ability to carry out certain diagnostic activities (e.g., evaluating evidence about students’ performance). Performing these diagnostic activities may then lead to the generation of specific diagnoses, which can be evaluated in terms of diagnostic quality (indicated by the accuracy and the efficiency of diagnoses) and is supposed to be influenced by a professional knowledge base (relevant kinds of knowledge). This extensive approach allows for detailed analyses of teachers’ diagnostic activities and a closer look at what specific activities are most common and most efficient when diagnosing students’ knowledge or skills (e.g., Bauer et al., 2020).
Most research on teachers’ diagnostic competences focuses exclusively on the accuracy of teachers’ diagnoses as an indicator of diagnostic competences (see Hoge and Coladarci, 1989; Südkamp et al., 2012). In this approach, (relative) diagnostic accuracy is usually operationalized as the correlation between teachers’ judgments and students’ actual knowledge or skill levels, as measured by (standardized) tests or other objective measures (Hoge and Coladarci, 1989; Südkamp et al., 2012; Urhahne and Wijnia, 2021). Differences in this kind of operationalization emerge mainly from different ways of measuring teachers’ judgments. For one, teachers’ judgments can be direct (i.e., the estimation of students’ performance on an achievement test that is used for measuring the students’ knowledge or skills) or indirect (i.e., estimation using other instruments) evaluations of students’ knowledge or skill levels. In addition, teachers’ judgments can, for example, be expressed in terms of class or peer norms, or their assessments can be completely peer-independent (see, e.g., Hoge and Coladarci, 1989). Regardless of the specific operationalizations, using diagnostic accuracy as a (sole) indicator of diagnostic competences has often been criticized as an overly simplified approach (see Klug et al., 2013) that omits the analysis of specific diagnostic activities (see Heitzmann et al., 2019), but focusing primarily on diagnostic accuracy may still be an adequate approach, as it might be the most relevant indication of teachers’ actual diagnostic competences. Findings from previous research showed that, on average, most teachers perform rather well in diagnosing students’ knowledge or skills, but that the quality of teachers’ judgments strongly varies depending on various judgment characteristics (e.g., norm-referenced vs. peer-independent judgments or informed vs. uninformed judgments; Südkamp et al., 2012). Furthermore, teachers also seem to overestimate students’ achievements, at least on standardized tests (Urhahne and Wijnia, 2021), and therefore, there still appears to be room for improvement (Südkamp et al., 2012). However, despite the large body of research, there is still a lack of studies focusing on differentiated diagnoses of a more “qualitative” type, such as detailed diagnoses of students’ profiles of knowledge and skill levels, rather than on more global quantitative assessments, such as rating scales or school grades (e.g., Schrader, 2009).
1.1.2. The professional knowledge underlying teachers’ diagnostic competences
The professional knowledge base underlying diagnostic activities can be characterized similarly as in other cases of teachers’ professional activities (see Förtsch et al., 2018). Based on the original taxonomy of Shulman (1986, 1987), it has become common practice to differentiate at least three separate facets of teachers’ professional knowledge: content knowledge (CK), pedagogical content knowledge (PCK), and pedagogical-psychological knowledge (PK). CK is a teacher’s own mastery of the content he or she wants to teach (Baumert et al., 2010); it comprises subject-specific knowledge and skills, including knowledge about how relevant information is interconnected (Loewenberg Ball et al., 2008). PCK is knowledge about instructional approaches used for making specific content accessible for students (Depaepe et al., 2013); it comprises knowledge about content-specific tasks, student knowledge and typical misconceptions, representations, and explanations (Baumert et al., 2010). PK is knowledge about how to arrange teaching and learning situations across subjects (Voss et al., 2011). Therefore, only CK and PCK are subject- or topic-specific. In contrast, PK can be regarded as topic-independent and may therefore be more relevant for the diagnosis of general characteristics (e.g., motivational traits) and less relevant for the diagnosis of students’ knowledge and skill levels. This might also be true for the diagnosis of cross-domain skills, such as scientific reasoning, because these skills also have to be applied to concrete subject- or topic-specific content.
Previous research has shown that some facets of teachers’ professional knowledge affect not only the ability to deliver high-quality instruction in class (Stough and Palmer, 2003; Baumert et al., 2010; Kunter et al., 2013) but also diagnostic activities and diagnostic accuracy (Kramer et al., 2021). However, past research has mainly focused on the diagnosis of subject-specific knowledge or skills or students’ overall academic ability (see Südkamp et al., 2012); in contrast, research on the diagnosis of cross-domain skills is still scarce. This holds despite the fact that cross-domain skills (such as ICT literacy, learning strategies, argumentation, or scientific reasoning) are also of high educational relevance and are considered important objectives, which are mentioned in many school curricula across the world (see, e.g., Wecker et al., 2016; Chinn and Duncan, 2018). In addition, cross-domain skills, such as scientific reasoning skills, can be regarded as especially relevant because of their importance for more than one subject or more than one discipline (see, e.g., Wecker et al., 2016; van Boxtel and van Drie, 2018).
1.2. The diagnosis of cross-domain skills
Cross-domain skills are skills that can be applied to various topics from different school subjects (e.g., scientific reasoning skills that can be applied to topics in subjects such as biology and physics; see also Wecker et al., 2014). This application to various topics involves the interplay of knowledge and skills on two levels when performing specific tasks: On the one hand, performing such tasks involves the application of the respective cross-domain skill (e.g., scientific reasoning skills). From the perspective of support for cross-domain skills, these skills constitute the so-called learning domain (Renkl et al., 2009). On the other hand, such tasks also require topic-specific knowledge for successful performance. Topic-specific knowledge comprises relevant background knowledge determined by the respective cross-domain skill and the specific task. For example, in a classroom setting, students’ scientific reasoning skills can be fostered by means of inquiry tasks involving experiments conducted by the students. These experiments necessarily take place within a specific school subject and a specific topic (e.g., biology, experiments with plants). From the perspective of support for cross-domain skills, the specific topic constitutes the so-called exemplifying domain (Renkl et al., 2009). The exemplifying domain is usually not the main focus of interest, but knowledge about it is still essential for mastering the task (Renkl et al., 2009). Whenever fostering cross-domain skills is among the goals of instruction, the corresponding diagnosis of learning progress has to focus on them as the learning domain while at the same time taking learners’ knowledge of the exemplifying domain into account because learners’ task performance will, at least to some degree, reflect both.
1.2.1. The cross-domain skill of scientific reasoning
In the present study, we focus on scientific reasoning as an exemplary case of a cross-domain skill because of its notable importance for several natural science subjects, such as biology or physics. Its relevance and special status is emphasized by a wide array of research from developmental psychology focused on the (co-)development of scientific reasoning in different domains and, therefore, its special status as a cross-domain skill (see, e.g., Kuhn et al., 1992; Zimmerman, 2007).
According to the theory of Scientific Discovery as Dual Search (Klahr and Dunbar, 1988), scientific reasoning can be characterized as search within two different spaces: the hypothesis space and the experiment space. Within these spaces, scientific reasoning comprises the operations of generating hypotheses (by searching the hypothesis space consisting of all possible hypotheses), testing hypotheses by designing and conducting experiments (by searching the experiment space consisting of all possible experiments), and evaluating the results in order to draw conclusions about hypotheses or formulate further hypotheses (Klahr and Dunbar, 1988; see also van Joolingen and de Jong, 1997; de Jong and van Joolingen, 1998). Hence, the ability of scientific reasoning can be described as an array of skills, all frequently used in the practice of science (Dunbar and Fugelsang, 2007; see also Klahr and Dunbar, 1988; Kuhn et al., 1992; de Jong and van Joolingen, 1998; Schunn and Anderson, 1999; Zimmerman, 2000, 2007). Nevertheless, the number of relevant skills can vary depending on research focus or research aims. Additionally, those skills can be organized into a system of sub-skills. Based on the theoretical considerations above as well as the literature in developmental psychology (Zimmerman, 2000, 2007), the following classification of 11 sub-skills of scientific reasoning was used as the basis for the present study: The formulation of hypotheses comprises (1) the ability to formulate specific hypotheses (depending on prior knowledge, hypotheses should be as specific as possible) and (2) the ability to systematically approach the formulation of hypotheses (with the goal of formulating hypotheses about all relevant independent variables). Experimentation comprises (3) the ability to efficiently sequence the approach to conducting experiments (e.g., using experiments that have already been conducted for comparison), (4) the ability to correctly manipulate one specific variable while (5) holding all other variables constant, also referred to as the Control of Variables Strategy (CVS; e.g., Tschirgi, 1980; Chen and Klahr, 1999; Lorch et al., 2014; Schwichow et al., 2016a), and (6) the ability to completely work through all experiments that are necessary to examine the impact of each potentially relevant (independent) variable. Drawing conclusions comprises (7) the ability to draw specific conclusions from experiments in which the independent variable influences the dependent variable or (8) from experiments in which it does not, (9) the ability to abstain from drawing a conclusion when an experiment is inconclusive (e.g., when more than one variable was manipulated by mistake), (10) the ability to recognize those inconclusive experiments, and (11) the ability to reject a hypothesis when the results of an experiment points in a different direction.
The teaching of scientific reasoning in school is often based on relatively simple inquiry tasks designed to help students gather new knowledge or new ideas on their own. The emphasis is on “new” as there can be no inquiry when a solution to a task can be recalled from one’s memory (Chinn and Duncan, 2021). Especially popular are experimentation tasks that are designed to let students explore whether a small number of predetermined independent variables influence a specific dependent variable. To do so, students have to conduct experiments in which they should manipulate only one independent variable while keeping all other independent variables constant. For implementation, such experiment tasks have to be embedded within a specific topic that constitutes the thematic background. A specific example from the subject of biology would be experiments concerning the growth of plants. Here, students would have to formulate hypotheses (e.g., “More water leads to better plant growth.”) for all predetermined independent variables, conduct a sufficient number of experiments, and draw conclusions. These conclusions should include rather specific reflections of the results of a correctly conducted experiment (e.g., “The amount of water positively influences the growth of a plant.”). This example also clarifies the special status of a cross-domain skill, such as scientific reasoning, by indicating the aforementioned interaction between the learning domain (scientific reasoning skills) and the exemplifying domain (topic-specific knowledge, e.g., knowledge about the growth of plants). In this example, the exemplifying domain only acts as a surface feature and may therefore be less relevant for mastering the inquiry tasks. Generally speaking, this assumption may hold true in all cases in which inquiry tasks are designed to be a sequence of simple experiments that do not require much background information or in which these tasks themselves artificially limit the availability and relevance of background knowledge (e.g., as predetermined variables are limited, it may be impossible to investigate the role of sunlight, even though highly relevant, in an experiment concerning the growth of plants). However, the role of the exemplifying domain might be different in more complex scientific experiments. Although it is a common approach, reliance on simplified experimental tasks (“toy tasks”) is sometimes criticized for its lack of resemblance to the role scientific reasoning plays in professional research activities (Chinn and Malhotra, 2002). Nevertheless, those kinds of inquiry tasks are both highly researched and widely used and, therefore, of some relevance. It has also been argued that simple CVS-related experimentation tasks (see scientific reasoning sub-skills 4 and 5) are relevant because the ability to master them might be an appropriate indicator of (students’) cognitive development (see, e.g., Ford, 2005; Schwichow et al., 2016b) and that it can therefore be regarded as a cornerstone of the development of a more sophisticated understanding of scientific reasoning. It is also well-established that many students (and also adults) struggle with simple inquiry tasks, including those requiring the CVS (Kuhn et al., 1992; Chen and Klahr, 1999) or those requiring the formulation of specific hypotheses (Lazonder et al., 2010), and sometimes even the recurring application of scientific reasoning sub-skills that have been successfully applied before can be a source of problems (Borkowski et al., 1987; van Joolingen and de Jong, 1997).
1.2.2. The role of professional knowledge for the diagnosis of scientific reasoning skills
As argued above, performing tasks that involve the application of cross-domain skills, such as scientific reasoning skills, requires an interplay between the cross-domain skills in question, on the one hand, and topic-specific knowledge, on the other hand. This also has implications for the professional knowledge base on part of the teacher as a diagnostician: As CK is a teacher’s own mastery of the knowledge or skill that students need to perform their tasks successfully, two kinds of CK relevant to the diagnosis of cross-domain skills have to be distinguished: On the one hand, CK comprises teachers’ own scientific reasoning skills (learning domain), on the other hand, it comprises teachers’ topic-specific knowledge, i.e., knowledge about a specific topic that constitutes the thematic background for teaching and diagnosing scientific reasoning (exemplifying domain). Even if this topic is not the focus of the diagnosis, such topic-specific knowledge may also be of relevance for the diagnosis of scientific reasoning skills.
In terms of PCK, a similar distinction might be made. However, as the focus of the present study is on the diagnosis of the cross-domain skill of scientific reasoning, the mainly relevant kind of PCK is teachers’ knowledge about the diagnosis of scientific reasoning. This is knowledge about how to diagnose students’ scientific reasoning skills and comprises information about specific difficulties, such as common errors, typical challenges in the development of these skills (see Zimmerman, 2000, 2007), and broader strategies to diagnose scientific reasoning skills (e.g., knowledge on how to recognize whether a student masters a specific scientific reasoning sub-skill).
When analyzing the relation between professional knowledge and diagnostic competences in diagnosing cross-domain skills (such as scientific reasoning), it is especially the facets of teachers’ professional knowledge specific to the knowledge or skill to be diagnosed (CK and PCK) that are of main interest. In contrast, teachers’ PK is topic-independent and may therefore be of lesser interest when investigating the interaction between teachers’ professional knowledge and their diagnostic competences in diagnosing students’ skill levels in general (see Kramer et al., 2021), and between teachers’ professional knowledge and their diagnostic competences in diagnosing cross-domain skills, such as scientific reasoning, in particular, because, as previously mentioned, scientific reasoning skills have to be applied to a subject- or topic-specific content (the exemplifying domain).
1.3. Using simulations for measuring diagnostic accuracy
In the context of medical education as well as teacher education, possibilities for measuring and fostering (future) professionals’ diagnostic competences in real-life settings, and, therefore, with real patients or students, may be limited due to ethical (Ziv et al., 2003) or practical considerations. Over many years, simulations of diagnostic assessment situations have proven to be a suitable substitute not only for the training of diagnostic competences (see, e.g., Chernikova et al., 2020b) but also for their measurement and analysis (see, e.g., Südkamp et al., 2008). Above all, this seems to be true in the field of medical education (e.g., Peeraer et al., 2007), but these days simulations are also gaining more and more attention in (pre-service) teacher education (e.g., Südkamp et al., 2008; Chernikova et al., 2020b). Simulations can represent specific segments of reality while offering opportunities to actively manipulate aspects of the segments of reality in question (Heitzmann et al., 2019). From a design perspective, simulations also allow for the possibility of simplifying segments of reality in order to reduce their complexity. As a consequence, the amount of information that needs to be processed can be decreased compared to corresponding real-life situations (see also Grossman et al., 2009). Simulation-based tools can therefore depict real-life situations in a more accessible way, which makes them suitable for a broader and more diversified field of applications, especially in instances in which real-life situations might be too complex to provide efficient research opportunities. Another benefit of simulations is the possibility to standardize assessment situations in ways that could not be implemented in a real-life setting, which again adds to the usefulness of simulations (see Schrader, 2009). In the context of the present study, for example, a (video-based) simulation is employed for having pre-service teachers diagnose identical students in identical assessment situations, even across different subjects (biology and physics). As a consequence of these advantages and despite some limitations, simulations are often viewed as an authentic way to depict specific classroom assessment situations (Codreanu et al., 2020; Kramer et al., 2020; Wildgans-Lang et al., 2020). Simulations can therefore also be regarded as a suitable tool for conducting research on (pre-service) teachers’ diagnostic competences.
Because working with a simulation can nevertheless be exhausting (especially when it comes close to the effort required in a real-life situation), it presupposes that participants go along with the idea of a simulation replacing real-life situations and are motivated to work with it (see Chen and Wu, 2012). Hence, motivational variables such as interest and current motivation constitute important factors that need to be controlled for when using simulations for research purposes (see also Kron et al., 2022). Taking these considerations into account, simulations can provide appropriate opportunities for investigating teachers’ diagnostic competences and potential relations between teachers’ professional knowledge and diagnostic accuracy in diagnosing scientific reasoning skills.
1.4. Aims and hypotheses
Teachers’ diagnostic competences are a cornerstone of successful teaching. Therefore, it is surprising that, despite extensive research on diagnostic competences, there is still a lack of studies addressing the diagnosis of cross-domain skills such as scientific reasoning. Cross-domain skills have their peculiarities because they are relevant for different school subjects (in contrast to subject-specific skills) and can be applied to various subject-specific content. Consequently, they are of high relevance for multiple (natural science) subjects and closely interact with topic-specific knowledge when performing specific tasks. As theoretical approaches and past research suggest that diagnostic accuracy in diagnosing subject-specific knowledge or skills may depend on teachers’ professional knowledge, the same can be expected for cross-domain skills, where it is important to assess whether teachers’ own mastery of a cross-domain skill (CK), their topic-specific knowledge (CK), as well as their knowledge about the diagnosis of a cross-domain skill (PCK) are related to their diagnostic accuracy. Based on these assumptions, the aim of this study was to extend our knowledge of the relations between (pre-service) teachers’ professional knowledge and their diagnostic accuracy by testing the following hypotheses:
H1: Pre-service teachers’ own scientific reasoning skills (CK) are positively related to their diagnostic accuracy in diagnosing scientific reasoning skills.
H2: Pre-service teachers’ topic-specific knowledge (CK) is positively related to their diagnostic accuracy in diagnosing scientific reasoning skills.
H3: Pre-service teachers’ knowledge about the diagnosis of scientific reasoning (PCK) is positively related to their diagnostic accuracy in diagnosing scientific reasoning skills.
As the cross-domain skill of scientific reasoning may be applied by learners in different subjects and may therefore also be diagnosed in the context of different subjects, the question arises whether the aforementioned relations are similar across subjects or whether they vary by subject. Hence, we also investigated the generality of these relations by testing the following interaction hypothesis:
H4: The relations between pre-service teachers’ scientific reasoning skills (CK), pre-service teachers’ topic-specific knowledge (CK), as well as pre-service teachers’ knowledge about the diagnosis of scientific reasoning (PCK), on the one hand, and their diagnostic accuracy, on the other hand, differ between school subjects such as biology and physics.
2. Materials and methods
2.1. Participants
Participants were recruited at three German universities, where data collection took place as required activities in the context of several mandatory university courses within university programs for pre-service teachers (e.g., courses intended for pre-service teachers focusing on biology/physics and more generally relevant courses, such as courses on teaching and lesson design). University students who attended one of the selected courses but were not enrolled in a teacher preparation program (i.e., studied other subjects, such as educational science) also participated but were not considered eligible for this study. Participants were free to decide whether their data could be used for the purpose of this study or not; their decision had no impact on grading or the successful completion of the course. In case the lecturer of the particular course was open and receptive to the idea, we gave away vouchers as compensation for participation. In accordance with the local legislation and institutional requirements, ethical approval was not required for this study. Participants who participated in one of two data collection sessions that resulted in unusable data due to major technical problems were entirely excluded from the data sample. Additionally, individual participants with specific, significant technical problems (19 participants) and participants who skipped essential tests/questionnaires or did not finish the simulation (7 participants) were also excluded. The sample size used for analysis included 161 pre-service teachers overall (54% female, 44% male; age: M = 22.76, SD = 2.48). Participants in the sample differed in terms of the school type and the subject (combination) that their university program was focused on; 55% of the pre-service teachers specialized in biology, 7% in physics, < 1% in biology and physics, and 37% neither in biology nor in physics. They also differed in terms of their individual progress within the university programs, but a majority had already been studying for several semesters at the time of the data collection (semester: M = 4.94, SD = 1.78).
2.2. Design
The design was correlational, focusing on the predictors scientific reasoning skills (CK), knowledge about the diagnosis of scientific reasoning (PCK), and topic-specific knowledge (CK), as well as the dependent variable of diagnostic accuracy. Because we expected low values and limited variation for the predictor knowledge about the diagnosis of scientific reasoning (PCK), we decided to experimentally manipulate this kind of knowledge as a between-subjects factor using an explanatory text, describing the different sub-skills of scientific reasoning and possible strategies for diagnosing them, in a control-group design. The participants were randomly assigned to one of the two groups. We used the dichotomous variable manipulation of knowledge about the diagnosis of scientific reasoning (PCK) instead of the measure of knowledge about the diagnosis of scientific reasoning (PCK) for further analysis because we expected the variable to deviate markedly from a normal distribution. Furthermore, control variables were included as additional predictors (interest and current motivation) because we expected those variables to be of particular importance for the diagnostic accuracy.
2.3. Procedure
All participants completed tests of scientific reasoning skills (CK), topic-specific knowledge (CK), and knowledge about the diagnosis of scientific reasoning (PCK), as well as questionnaires on interest and current motivation. Participants who were assigned to the experimental group additionally read a text about the diagnosis of scientific reasoning right before working on the corresponding test (see Table 1 for more information). Afterwards, the participants were introduced to one of two video-based simulations that depicted either a biology or a physics lesson (see Table 1). We used these video-based simulations for the purpose of assessing the participants’ diagnostic competences in diagnosing students’ scientific reasoning skills by capturing their diagnostic accuracy. Whether participants were assigned to work on the simulation depicting a biology lesson or on the simulation depicting a physics lesson depended on the school subject they specialized in as part of their teacher education. Participants who specialized in neither biology nor physics were assigned at random. Due to the COVID-19 pandemic, data collection after March 2020 (accounting for 58.4% of the data) was conducted online. In these cases, we made sure that participants received the same detailed instructions as participants who participated during “in-person courses” by replacing live instructions with video instructions and giving participants a chance to ask questions via email throughout their participation. Overall, participants asked few questions. In terms of content, there was a shift to more technology-related questions during online participation (e.g., regarding the technical requirements for participation).
2.4. Instruments and operationalizations
2.4.1. Scientific reasoning skills (CK)
Participants’ own scientific reasoning skills (CK) were measured using a shortened and translated 7-item version of the revised edition of the Classroom Test of Scientific Reasoning (Lawson, 1978; Lawson et al., 2000). The seven items (involving “strings,” “glass tubes 1″, “glass tubes 2″, “mice” “burning candle,” “red blood cells 1″, “red blood cells 2″) were selected based on relevance and thematic proximity because they tested participants’ knowledge about the appropriate approach for conducting experiments and drawing conclusions (related to the basic principles of CVS). Five of the seven items consisted of two parts. As in the original instrument, in those cases, only the items with both parts answered correctly were scored as correct. This means that participants could score between 0 and 7 points. For further analysis, we calculated the individual score of each participant by dividing the participants’ achieved score by the maximum score of 7 points, which yields an individual score between 0 and 1.
2.4.2. Topic-specific knowledge (CK)
To measure participants’ topic-specific knowledge (CK), we used two different tests. Depending on the subject of the simulation participants were assigned to, they completed either a test in biology or physics. The test in biology was a shortened and translated 4-item version of a test on the growth of plants (Lin, 2004). The four items (involving “importance of water for germination,” “importance of soil for germination,” “importance of sunlight for growth,” “energy source for growth”) were selected based on thematic relevance, as they tested participants’ knowledge about content also featured in the biology case of the simulations. The test in physics was a custom-made 4-item test on optical lenses (e.g., “Does the refraction of light through an optical lens depend on the type of lens?”). The custom-made test was designed to be structurally identical to the test in biology, including the same type and number of questions. In both cases, all four items consisted of two parts, with the second part always asking about the reason for the answer given in the first part. Again, only those items with both parts answered correctly were scored as correct. Participants could score between 0 and 4 points per test. We calculated the individual score of each participant by dividing the participants’ achieved score by the maximum score of 4 points, which yields an individual score between 0 and 1. Because each participant’s topic-specific knowledge (CK) was only measured with one of the two tests, depending on the simulation, z-scores instead of raw scores were used in the statistical analyses.
2.4.3. Knowledge about the diagnosis of scientific reasoning (PCK)
2.4.3.1. Text about the diagnosis of scientific reasoning
A text was used to manipulate the participants’ knowledge about the diagnosis of scientific reasoning (PCK). It was a comprehensive summary (consisting of 1,221 words) about the structure of scientific reasoning and included instructions on how to diagnose students’ scientific reasoning (sub-) skills. It was written to cover the content of the simulations and was specifically tailored for this purpose. The text contained a short introduction to scientific reasoning and featured information about the 11 scientific-reasoning sub-skills that were structurally divided into three groups: formulation of hypotheses (e.g., “precision of hypotheses”), experimentation (e.g., “manipulation of one specific variable”), and drawing conclusions (e.g., “conclusions about variables that have an influence on the dependent variable”; for a listing of all scientific reasoning sub-skills see Section 1.2.1). For all sub-skills, the text contained an explanation of the respective sub-skill and a description of how to diagnose it. For this purpose, it was described how to identify a student’s skill level on a specific sub-skill. For example, the skill level on the “precision of hypotheses” sub-skill is indicated by the degree of detail in hypotheses with the levels (1) hypotheses about the strength of an influence of the independent on the dependent variable, (2) hypotheses about the direction of an influence, (3) hypotheses about the existence of an influence, and (4) the absence of specific hypotheses (see also, e.g., Lazonder et al., 2008). An additional section on diagnostic strategies explained the relation between observable behavior and non-observable latent skills. It described how the absence of a certain behavior is insufficient for concluding that the person lacks the respective skill and that the person’s skill has to be probed by specific questions in this case. Participants in the experimental group who received the text spent an average of 6.93 min reading the text (SD = 2.26).
2.4.3.2. Test of knowledge about the diagnosis of scientific reasoning
To establish the effectiveness of the manipulation of knowledge about the diagnosis of scientific reasoning (PCK) by means of the text, we conducted a manipulation-check by administering two interrelated open-ended questions about the content of the text (e.g., “Now describe which problems you expect during experimentation on part of the students. Consider which student errors are common in the field of scientific reasoning. Subdivide your answer according to the areas formulating hypotheses, planning and conducting experiments, and drawing conclusions.”). Two persons independently coded both answers (as a whole) based on a pre-specified coding system. Participants received points for mentioning (one point) and correctly explaining (one point) each individual sub-skill (resulting in up to two points per sub-skill). A correct explanation contained descriptions on how to differentiate between students’ skill levels when diagnosing their individual scientific reasoning (sub-)skills. Participants received an additional point for explaining the significance of distinguishing between manifest behavior and latent skills. They could therefore score between 0 and 23 points. Once again, we calculated the individual score of each participant by dividing the participants’ archived score by the maximum score of 23 points, which yields an individual score between 0 and 1. A two-way mixed intra-class correlation showed high agreement between the raters; ICCabsolute (160,160) = 0.95. Averaged scores of both raters were used for further analyses.
2.4.4. Interest
Interest in three objects of interest was measured by questionnaire scales: interest in diagnosing (e.g., “I want to know more about diagnosing.”), interest in scientific reasoning (e.g., “I enjoy working on the topic of scientific reasoning.”), and interest in teaching biology/physics (e.g., “I think teaching biology and physics is interesting.”). Each scale consisted of three items. The items were translated and adapted from a questionnaire by Rotgans and Schmidt (2014). Participants rated each statement on a 5-point scale. The scales’ internal consistencies amounted to Cronbach’s α = 0.80 (diagnosing), 0.82 (scientific reasoning), and 0.96 (teaching biology/physics).
2.4.5. Current motivation
The expectation component of current motivation was measured by a shortened 4-item version of the Questionnaire on Current Motivation (e.g., “It is likely that I will not be able to solve this task.”; Rheinberg et al., 2001). The participants rated each statement on a 7-point scale. The scale’s internal consistency amounted to Cronbach’s α = 0.77.
The value component of current motivation was measured by four items, which were developed based on the expectancy-value theory of achievement motivation (e.g., “I think it is important to be able to solve this task”; Wigfield, 1994). Participants rated each statement on a 5-point scale. The scale’s internal consistency amounted to Cronbach’s α = 0.84.
2.4.6. Diagnostic accuracy
2.4.6.1. Inquiry tasks used in the simulations
Diagnostic accuracy was measured using video-based simulations mimicking a certain segment of reality: a classroom situation involving small groups of students who independently conduct inquiry tasks. During such tasks, students have to formulate hypotheses, plan and run experiments, and draw conclusions about their initial hypotheses based on the results of the experiments. To keep track of their experiments, they usually would take notes about every trial they conduct. In this scenario, the teacher would be a rather passive observer who keeps a watch on the experimenting students and occasionally takes a look at their notes. He or she would only interact with the students by asking questions about their current activities in order to gather additional information, especially in situations in which the students’ intentions are unclear or when their observable activities are insufficient as a basis for diagnostic inferences. Because this kind of inquiry task could be implemented within different school subjects, two different simulations, one depicting a biology lesson and one depicting a physics lesson, were developed (see Figure 1). Both simulations feature all of the defining characteristics of the segment of reality described above. The biology lesson comprises experiments concerning the growth of plants, and the physics lesson comprises experiments concerning optical lenses. In both scenarios, the students’ goal is to find out which of a set of independent variables influence a specific outcome variable. In each scenario, the students have to investigate the influence of four different independent variables. As an example, the biology lesson is focused on the independent variables (1) amount of water (possible values: all values between 5 and 40 milliliters), (2) a fertilizer stick (possible values: yes or no), (3) salt (possible values: all values between 0 and 5 grams), and (4) an undefined white powder (possible values: yes or no). The students’ goal is to find out whether or not each of those variables influences the growth of a mustard plant; in this case, the growth is influenced by amount of water and salt but not by a fertilizer stick and an undefined white powder. There are no interaction effects of the independent variables in this scenario. Both simulations are structurally identical, only differing in terms of the topic used as the exemplifying domain for introducing the inquiry task.
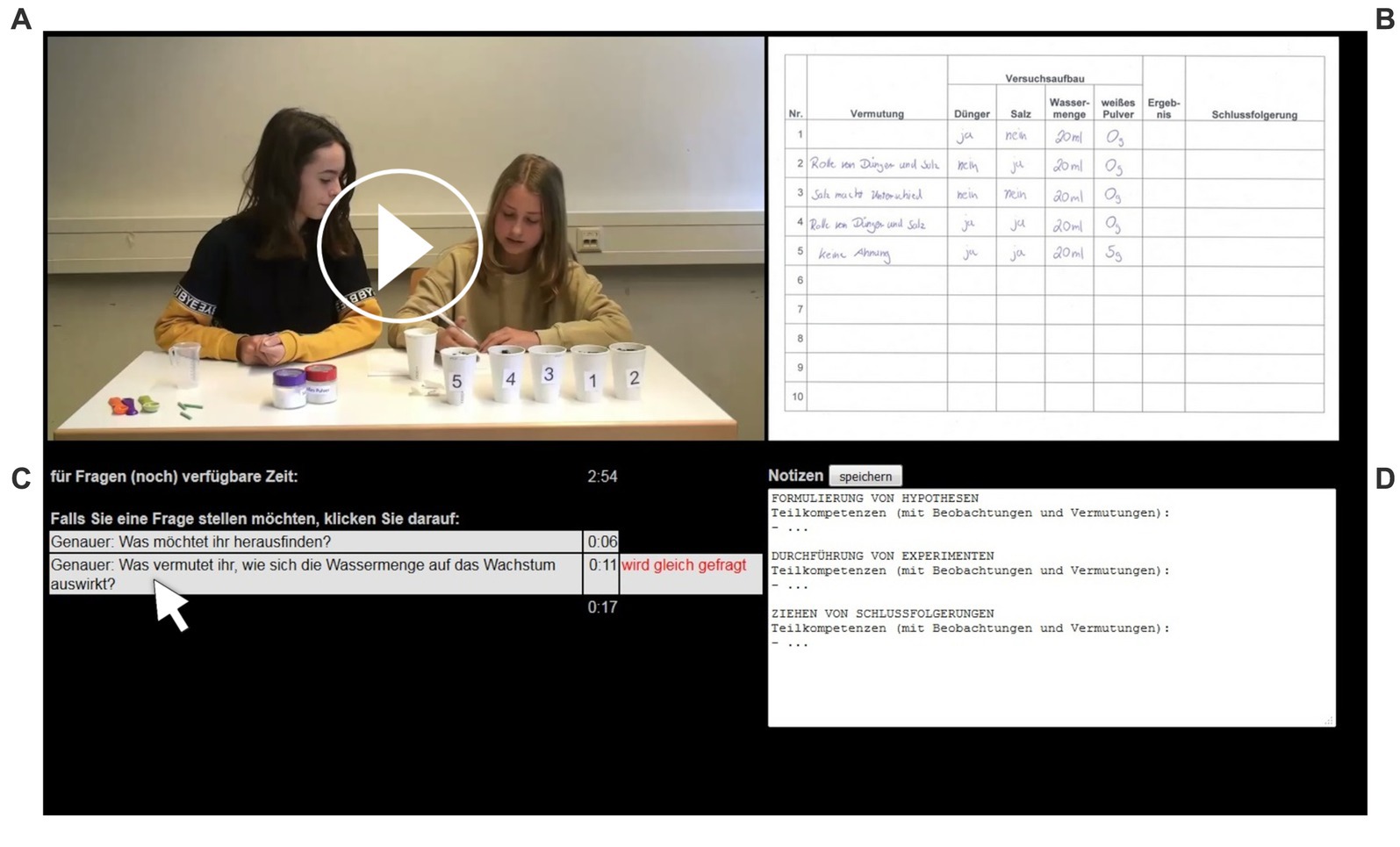
Figure 1. Biology case of the video-based simulations: (A) video area (B) inquiry table (C) navigation area (D) notepad.
2.4.6.2. Simulation interface
When working with the simulations, the participants are instructed to view the setting as a kind of role-play scenario in which they take on the role of a teacher who tries to collect information about a certain student’s scientific reasoning skills while observing him or her during a school lesson. In the simulation interface, the computer screen is divided into four areas (see Figure 1). In the video area (see Figure 1A), the participants can watch videos of two experimenting students. The inquiry table (see Figure 1B) contains hand-written notes by the students featured in the videos. It was designed with the purpose of helping the participants keep track of all the experiments already conducted by the students and is updated automatically when the students in the videos write down additional notes. In the notepad (see Figure 1D), participants can write down notes on their own. To help them focus on essential information, the notepad contains structuring headings identical to those used in the text about the diagnosis of scientific reasoning (formulation of hypotheses, experimentation, and drawing conclusions). Participants are free to decide how to use the notepad; it is also possible to delete the headings. In the navigation area (see Figure 1C), up to three pre-formulated questions appear and disappear simultaneously at certain points of the videos (e.g., “Do you already have a specific idea about how exactly salt influences the growth of the plant?”). Participants can ask one or more questions by clicking on the individual question links. Asking a question stops the video automatically (at the next appropriate moment), and a video segment containing the associated answer is then played in the video area. After the answer has been played back, the primary video continues. Participants have a limited amount of time to ask questions. Taking this limitation into account, not all of the questions were designed to be potentially useful; instead, some are also supposed to be redundant or even completely irrelevant. Based on these preliminary considerations, participants have just enough time on their “time account” to ask all of the useful questions. After the students featured in the videos declare that they are finished with the task and that there is no other meaningful experiment left to do, participants can spend their remaining “time account” on asking additional questions that appear at the end of the simulations (e.g., “Is there one or even more than one experiment that was not completely necessary and, therefore, could have been left out?”; see also Pickal et al., 2022).
2.4.6.3. Video material
The videos (including the answer-videos) were recorded based on scripts. These scripts were developed based on a fictitious student profile for one of the two students in the video. This profile contained specifications of levels for each of the scientific reasoning sub-skills. Both profiles and scripts (for the biology and the physics videos) were designed to be structurally identical. The dialogues in the biology case and the physics case are composed of essentially the same moves and differ in only two respects: (1) The subject-specific content refers to either the biology or the physics inquiry task (see also Pickal et al., 2022). (2) In the biology case, the video is divided into two main parts: One covering a session in which the students experiment with already sprouted mustard plants and one covering a session that takes place roughly 2 weeks later in which they measure the growth of the plants. The distinction between these two parts is emphasized by the fact that the two students wear different clothes. In the first part, they formulate a research question and/or hypothesis for each trial and choose the values of the four independent variables, whereas in the second part, they read the value of the dependent variable and draw a conclusion. In the physics case, on the other hand, each trial conducted by the students comprises the formulation of a research question and/or hypothesis, the setting of the four independent variables, as well as the reading of the dependent variable, and the drawing of a conclusion in one continuous session. Hence, although the dialog moves are distributed across two sessions (in biology) or only one session (in physics), they are structurally equivalent and uttered in the same sequence within each session.
2.4.6.4. Diagnoses
The participants were instructed to arrive at a diagnosis for one pre-specified student from the pair of students in the video. After completing the simulation, they were asked to write down a diagnosis of this student’s individual scientific reasoning skills. In doing so, they could use their notes from the notepad (see Figure 1D) from the video-based simulation. The participants’ written diagnoses (but not their notes) were analyzed to determine diagnostic accuracy.
For each sub-skill of scientific reasoning (identical to the sub-skills mentioned in the text about the diagnosis of scientific reasoning; for more information, see Sections 1.2.1 and 2.4.3.1), it was coded whether the written diagnosis contained a statement about that sub-skill and if so, whether the respective sub-skill was diagnosed correctly or not (based on the student profile underlying the video case). Participants received one point per mentioned sub-skill and one additional point per correctly diagnosed sub-skill (for an example, see Table 2). If the diagnosis also contained a statement about how the participant used information gained by observing a certain behavior or by observing the absence of a certain behavior to make assumptions about an underlying latent skill (e.g., based on asking a specific question during working on the simulation), he or she received an additional point. This led to an overall 23-point maximum. The individual score for each participant’s diagnostic accuracy was calculated by dividing the archived score by the maximum score of 23 points, which yields an individual score between 0 and 1. Two independent raters coded the diagnoses of all participants. There was a high agreement between the coders, as indicated by a two-way mixed intra-class correlation; ICCabsolute (160,160) = 0.83. Averaged scores of both raters were used for all further analyses.
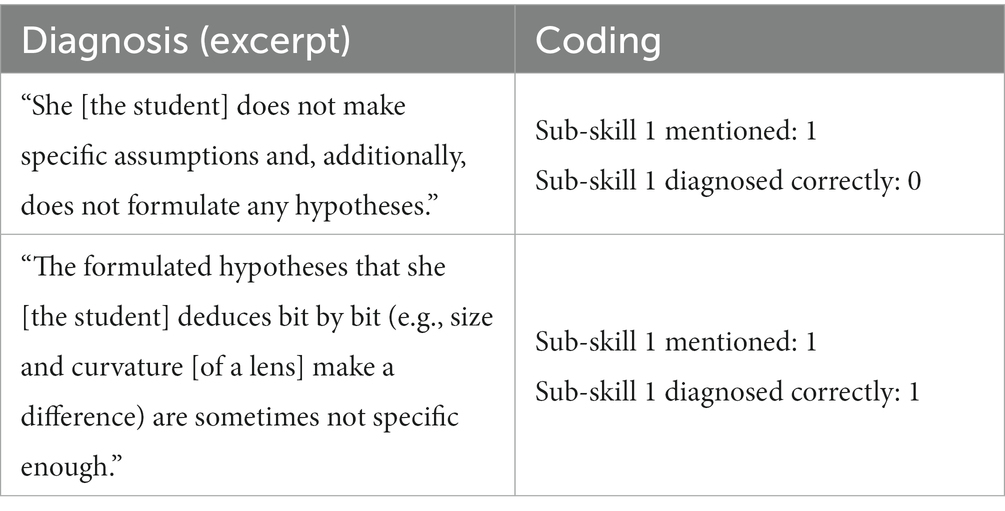
Table 2. Diagnostic accuracy – coding examples (scientific reasoning sub-skill 1: the ability to formulate specific hypotheses).
2.5. Statistical analysis
Two regression analyses with diagnostic accuracy as the criterion variable were conducted. In the first regression analysis, only the control variables were included: interest in diagnosing, interest in scientific reasoning, interest in teaching biology/physics, expectation component of current motivation, and value component of current motivation. The second regression analysis additionally included the following predictors: participants’ own scientific reasoning skills (CK), topic-specific knowledge (CK), and the manipulation of knowledge about the diagnosis of scientific reasoning: text vs. no text (PCK). The subject of the simulation (biology/physics) and the interaction terms between the subject of the simulation and scientific reasoning skills (CK), topic-specific knowledge (CK), and the manipulation of knowledge about the diagnosis of scientific reasoning were also added as predictors in the second regression analysis. For all analyses, the level of significance was set to p < 0.05.
3. Results
3.1. Preparatory analyses
A treatment check indicated that knowledge about the diagnosis of scientific reasoning (PCK) was higher in participants who had read the text about the diagnosis of scientific reasoning (M = 0.16, SD = 0.14) than in participants who had not read the text (M = 0.02, SD = 0.04). This difference was statistically significant and amounted to a large effect size, t (93.30) = 8.56, p < 0.001, d = 1.11. As expected, the distribution of knowledge about the diagnosis of scientific reasoning (PCK) was significantly different from a normal distribution, as shown by a significant Kolmogorov–Smirnov test; D(161) = 0.27, p < 0.001.
Although the biology case and the physics case of the simulations were designed to be structurally equivalent, the average diagnostic accuracy was higher for the biology case (M = 0.28, SD = 0.12) than for the physics case (M = 0.22, SD = 0.14). The test of significance for the predictor subject of the simulation in the second linear regression analysis indicated that this difference is statistically significant.
We decided to report the findings from analyses without online vs. in-person participation as an additional variable in the linear regression because analyses in which this variable was included did not yield substantially different results.
3.2. Predictors of diagnostic accuracy
Descriptive statistics for all tests and questionnaires used for measuring the potential predictors of diagnostic accuracy can be found in Table 3. The table indicates relatively high variances and ranges of the distributions for all tests and questionnaires, indicating no floor or ceiling effects for any instrument.
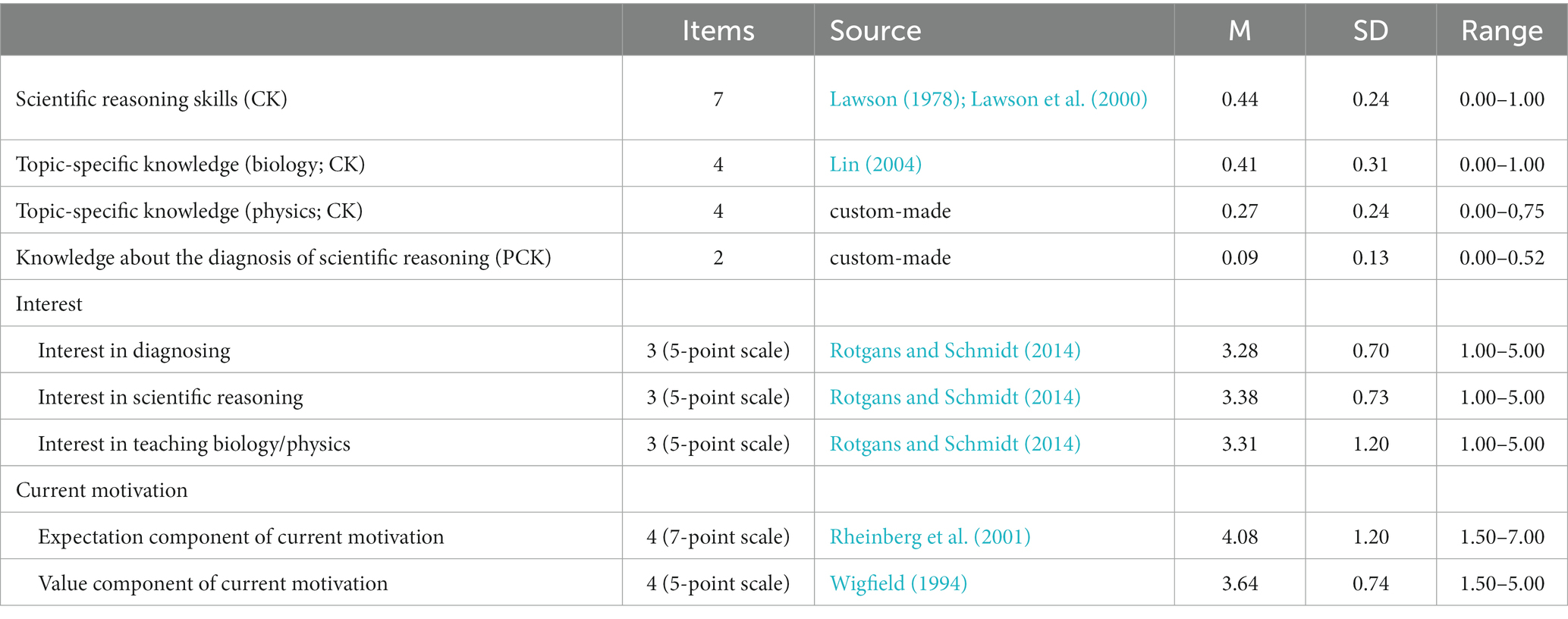
Table 3. Instrument descriptions and descriptive statistics including means (M), standard deviations (SD), and observed range of the tests and questionnaires.
In the regression analysis including only control variables (Model 1, Table 4), 16.5% of variance in pre-service teachers’ diagnostic accuracy was explained by the predictors, F (5, 155) = 6.14, p < 0.001. Two control variables were statistically significant predictors of diagnostic accuracy: interest in diagnosing as well as the value component of current motivation. Thus, higher interest in diagnosing, as well as a higher value component of current motivation, was associated with higher accuracy in diagnosing. Interest in scientific reasoning, interest in teaching biology/physics, and expectation component of current motivation were not found to be statistically significant predictors of diagnostic accuracy.
In the regression analysis including all predictors (Model 2, Table 4), 23.2% of variance in pre-service teachers’ diagnostic accuracy was explained by the predictors, F (12, 148) = 3.73, p < 0.001. However, the R2 change of 0.067 between both analyses did not reach statistical significance, F (7, 148) = 1.84, p = 0.084. In testing the first hypothesis, the results of this regression analysis indicated that scientific reasoning skills (CK) are a statistically significant predictor of pre-service teachers’ diagnostic accuracy. Thus, the higher the scientific reasoning skills (CK), the more accurate the diagnosis, a result that directly supports H1. In testing the second hypothesis, topic-specific knowledge (CK) was not found to be a statistically significant predictor of pre-service teachers’ diagnostic accuracy. A similar result was found in testing the third hypothesis: Manipulation of knowledge about the diagnosis of scientific reasoning (PCK) was also not a statistically significant predictor of pre-service teachers’ diagnostic accuracy. Thus, no empirical support was found for H2 and H3.
Concerning the fourth hypothesis, none of the interactions of the three professional knowledge variables with the subject of the simulation was statistically significant. This includes the interaction term for subject and scientific reasoning skills (CK), the interaction term for subject and topic-specific knowledge (CK), and the interaction term for subject and manipulation of knowledge about the diagnosis of scientific reasoning (PCK). Thus, no support for H4 was found.
4. Discussion
The aim of this study was to broaden our understanding of the relations between teachers’ professional knowledge and their diagnostic accuracy by extending research beyond the predominant focus on subject-specific knowledge or skills or overall academic ability (see Südkamp et al., 2012). To this purpose, we focused on the specific relation between professional knowledge and the diagnosis of a cross-domain skill, scientific reasoning. The results indicated that, controlling for interest and current motivation, pre-service teachers’ diagnostic accuracy is related to their own scientific reasoning skills (CK). No such relations could be established for their topic-specific knowledge (CK) or knowledge about the diagnosis of scientific reasoning (PCK), as manipulated by the text in this study; although the results regarding the relation between topic-specific knowledge (CK) and diagnostic accuracy, and, even more so, regarding the relation between knowledge about the diagnosis of scientific reasoning (PCK) and diagnostic accuracy, descriptively point in a positive direction. The study yielded no evidence that these results vary across subjects. In addition, it has to be mentioned that two of the control variables (interest in diagnosing and the value component of current motivation) had the strongest effects on pre-service teachers’ diagnostic accuracy. Consequently, although the second regression analysis (including all variables) explained about 7% more variance in pre-service teachers’ diagnostic accuracy compared to the first regression analysis (including only the control variables), the increase in explained variance did not reach statistical significance.
As discussed above, when diagnosing students’ scientific reasoning skills, teachers’ own scientific reasoning skills play a role that corresponds to teachers’ CK. Hence, on a general level, the result concerning the first hypothesis is in line with theories emphasizing the importance of professional knowledge for the accurate diagnosis of students’ knowledge or skills (e.g., Förtsch et al., 2018). In particular, one kind of CK seems to be a prerequisite for diagnostic accuracy, i.e., teachers’ own mastery of the knowledge or skill to be diagnosed seems to play an important role in this respect. Still, research on further cross-domain skills is necessary in order to assess the generality of these findings.
Contrary to the second hypothesis, knowledge about the growth of plants and knowledge about optical lenses, respectively, as instances of topic-specific knowledge (CK), were not significantly related to diagnostic accuracy. First, it should be noted that there was sufficient variance in the participants’ topic-specific knowledge (CK; see Table 3), indicating that the absence of a significant relation cannot be explained by limited variation. Hence, a substantive explanation is required for this result. According to our argument in Section 1.2.2, topic-specific knowledge constitutes an aspect of teachers’ CK beyond teachers’ own scientific reasoning skills. However, it does not correspond directly to the skills to be diagnosed but only to the exemplifying domains (Renkl et al., 2009) employed in the diagnostic situations, i.e., the specific topics that are needed in order to provide a thematic context for the application of scientific reasoning skills. In line with the view that the exemplifying domains are more of a surface feature (see Renkl et al., 2009), these topics have structurally little relevance for mastering the inquiry tasks. Hence, the present findings suggest that the mainly relevant kinds of CK, in terms of diagnostic accuracy, may be those that constitute the diagnosticians’ own mastery of the knowledge or skill to be diagnosed rather than aspects that refer to knowledge of exemplifying domains used in diagnostic situations. Alternatively, it may be assumed that topic-specific knowledge (CK) is only less relevant for the diagnosis of scientific reasoning skills when relying on simple CVS-related experiment tasks, such as the ones used in this study. It may therefore be rather unlikely that identical results would be obtained when using more complex experiment tasks for measuring teachers’ diagnostic competences; as professional knowledge might play a different role for the diagnosis of scientific reasoning skills in such instances. Further research is needed to follow up on these possibilities.
Contrary to the third hypothesis, no support was found for a positive relation between teachers’ knowledge about the diagnosis of scientific reasoning (PCK), as manipulated by the text, and their diagnostic accuracy. Analogous to the second hypothesis, the low variance in knowledge about the diagnosis of scientific reasoning (PCK) was not a statistical problem because, in this case, the manipulation of knowledge about the diagnosis of scientific reasoning (PCK; text vs. no text) and not the knowledge about the diagnosis of scientific reasoning (PCK), as measured by the subsequent test, was used as a predictor in the linear regression analysis. The result is a rather counter-intuitive finding, as it seems obvious that it should be helpful to have knowledge about how to diagnose scientific reasoning skills when diagnosing them in students. This would also have been in line with theoretical assumptions (see Förtsch et al., 2018). Even if the manipulation of knowledge about the diagnosis of scientific reasoning (PCK) proved to be quite effective for the participants’ actual knowledge about the diagnosis of scientific reasoning (PCK), it might be argued that the average knowledge level of the manipulation group was still insufficient. As the participants’ average diagnostic accuracy was also relatively low, it may also have been the case that it was too difficult for the participating pre-service teachers to benefit substantially from their (newly gained) knowledge during the specific assessment situation within the video-based simulations (see Heitzmann et al., 2019).
As we could not find any evidence supporting H4, the results do not indicate that the above-described pattern does not hold true across different school subjects (biology and physics), at least on a type of task that is highly isomorphic across two topics. Further research is needed to specify the extent to which these results can be replicated across other less isomorphic tasks, other school subjects, or other cross-domain skills (such as ICT literacy, learning strategies, or argumentation skills). Regardless, the findings reveal an interesting pattern concerning the role of the different kinds of pre-service teachers’ professional knowledge for their diagnostic accuracy when diagnosing scientific reasoning, as especially one kind of teachers’ professional knowledge, their own scientific reasoning skills (CK), seems to be more relevant than others, i.e., teachers’ topic-specific knowledge (CK) and their knowledge about the diagnosis of scientific reasoning (PCK).
The limitations of the present study, first of all, include the relatively heterogeneous sample. Even though the majority of the participating pre-service teachers specialized in one of the two subjects biology and physics, the sample also included pre-service teachers who specialized in neither of these two subjects. This means that for them, the simulations depicted an assessment situation with a thematic background that might have been completely new and of little obvious relevance for their own subjects. Because the simulations were focused on the diagnosis of scientific reasoning skills, which can also be regarded as important for subjects other than biology or physics, and because of the simple design of the inquiry tasks featured in the simulations, we would argue that the assessment situations are nevertheless accessible and relevant for pre-service teachers of other subjects. An inclusive sample seemed therefore justifiable. The sample also included pre-service teachers specializing in different school types (e.g., primary and secondary schools). Even though teaching scientific reasoning can be regarded as relevant for all school types, it might appear to be less relevant for (pre-service) primary school teachers. Another limitation of the present study is that, due to time restrictions, the instruments used to measure teachers’ own scientific reasoning skills (CK) and their topic-specific knowledge (CK) employed only a limited number of items with low thematic overlap between items. For example, the instrument measuring topic-specific knowledge (CK) in biology included an item on the “importance of water for germination” and an item on the “importance of sunlight for growth” (see also Lin, 2004). As a consequence of this limited coverage of the respective topics, it cannot be fully ruled out that the respective regression coefficients in the present study may have been underestimated and that, therefore, the corresponding tests of significance may have been overly conservative. However, the chance that a relevant effect of topic-specific knowledge (CK) was overlooked is limited in light of the rather low regression coefficient for this predictor.
Future research on teachers’ diagnostic competences might benefit from extending the approach of the present study by using a broader array of measures of diagnostic competence, which could cover additional aspects beyond diagnostic accuracy. This approach should also consider measures of teachers’ diagnostic activities, which would also take the increased complexity of recent competence models of diagnostic competences into account (see Heitzmann et al., 2019). Furthermore, future research should also reassess the role of (pre-service) teachers’ PCK for the diagnosis of students’ scientific reasoning skills, as other studies that focused on subject-specific diagnosis found pre-service teachers’ PCK to be significantly related to their diagnostic accuracy (Kramer et al., 2021). With respect to the low average knowledge about the diagnosis of scientific reasoning (PCK), even in the text-group, and the relatively low average level of diagnostic accuracy of the participants in the present study, reducing the complexity of the situation and the diagnostic task should be considered for further studies using simulation-based assessment situations, including studies using our own video-based simulation environment. This could, for example, be achieved by offering the participants more time to process the information provided or by offering them more opportunities to apply the newly gained knowledge to diagnostic situations. In addition, pre-service teachers could be supported within the simulations by computer-based scaffolding. Scaffolding might comprise functions for guiding the observation of students in the video-based simulations in terms of the structure of scientific reasoning (sub-) skills as well as for guiding participants’ documentation of their observations with respect to this structure. This also opens up new lines of research concerning support for the development of (pre-service) teachers’ diagnostic competences.
Data availability statement
The raw data supporting the conclusions of this article will be made available by the authors, without undue reservation.
Ethics statement
Ethical approval was not required for the study on human participants in accordance with the local legislation and institutional requirements. The participants provided their written informed consent to participate in this study. Written informed consent was obtained from the parents/guardians of the child actors involved in the simulation videos, for the use of the videos in the study and the publication of screenshots.
Author contributions
CW and AP developed the study design and conducted the data collection. AP analyzed the data and wrote the draft of the manuscript. CW, KE, CC, BN, and RG contributed to writing the manuscript. All authors contributed to the article and approved the submitted version.
Funding
The research presented in this paper was funded by a grant of the Deutsche Forschungsgemeinschaft (DFG) to CW, BN, and RG (WE 5426/2–1) as part of the Research Unit “Facilitation of Diagnostic Competences in Simulation-Based Learning Environments in Higher Education” (COSIMA, FOR 2385). The publication fee was borne by Stiftung Universität Hildesheim.
Acknowledgments
We want to thank Philipp Schmiemann and his team for supporting us during data collection. We also want to thank the students who acted in the videos, all participants, and the research assistants who helped to code the data: Jennifer Weber and Moritz Klippert.
Conflict of interest
The authors declare that the research was conducted in the absence of any commercial or financial relationships that could be construed as a potential conflict of interest.
Publisher’s note
All claims expressed in this article are solely those of the authors and do not necessarily represent those of their affiliated organizations, or those of the publisher, the editors and the reviewers. Any product that may be evaluated in this article, or claim that may be made by its manufacturer, is not guaranteed or endorsed by the publisher.
References
Bauer, E., Fischer, F., Kiesewetter, J., Shaffer, D. W., Fischer, M. R., Zottmann, J. M., et al. (2020). Diagnostic activities and diagnostic practices in medical education and teacher education: an interdisciplinary comparison. Front. Pyschol. 11:562665. doi: 10.3389/fpsyg.2020.562665
Baumert, J., Kunter, M., Blum, W., Brunner, M., Voss, T., Jordan, A., et al. (2010). Teachers’ mathematical knowledge, cognitive activation in the classroom, and student progress. Am. Educ. Res. J. 47, 133–180. doi: 10.3102/0002831209345157
Behrmann, L., and Souvignier, E. (2013). The relation between teachers’ diagnostic sensitivity, their instructional activities, and their students’ achievement gains in reading. Z. Päd. Psych. 27, 283–293. doi: 10.1024/1010-0652/a000112
Borkowski, J. H., Carr, M., and Pressley, M. (1987). “Spontaneous” strategy use: perspectives from metacognitive theory. Intelligence 11, 61–75. doi: 10.1016/0160-2896(87)90027-4
Chen, Z., and Klahr, D. (1999). All other things being equal: acquisition and transfer of the control of variables strategy. Child Dev. 70, 1098–1120. doi: 10.1111/1467-8624.00081
Chen, C.-H., and Wu, I.-C. (2012). The interplay between cognitive and motivational variables in a supportive online learning system for secondary physical education. Comput. Educ. 58, 542–550. doi: 10.1016/j.compedu.2011.09.012
Chernikova, O., Heitzmann, N., Fink, M. C., Timothy, V., Seidel, T., and Fischer, F. (2020a). Facilitating diagnostic competences in higher education: a meta-analysis in medical and teacher education. Educ. Psychol. Rev. 32, 157–196. doi: 10.1007/s10648-019-09492-2
Chernikova, O., Heitzmann, N., Stadler, M., Holzberger, D., Seidel, T., and Fischer, F. (2020b). Simulation-based learning in higher education: a meta-analysis. Rev. Educ. Res. 90, 499–541. doi: 10.3102/0034654320933544
Chinn, C. A., and Duncan, R. G. (2018). “What is the value of general knowledge of scientific reasoning?” in Scientific reasoning and argumentation: The roles of domain-specific and domain-general knowledge. eds. F. Fischer, C. A. Chinn, K. F. Engelmann, and J. Osborne (New York, London: Routledge), 77–101.
Chinn, C. A., and Duncan, R. G. (2021). “Inquiry and learning” in International handbook of inquiry and learning. eds. R. G. Duncan and C. A. Chinn (New York: Routledge), 1–14. doi: 10.4324/9781315685779-1
Chinn, C. A., and Malhotra, B. A. (2002). Epistemologically authentic inquiry in schools: a theoretical framework for evaluating inquiry tasks. Sci. Ed. 86, 175–218. doi: 10.1002/sce.10001
Codreanu, E., Sommerhoff, D., Huber, S., Ufer, S., and Seidel, T. (2020). Between authenticity and cognitive demand: finding a balance in designing a video-based simulation in the context of mathematics teacher education. Teach. Teach. Educ. 95:103146. doi: 10.1016/j.tate.2020.103146
de Jong, T., and van Joolingen, W. R. (1998). Scientific discovery learning with computer simulations of conceptual domains. Rev. Educ. Res. 68, 179–201. doi: 10.2307/1170753
Depaepe, F., Verschaffel, L., and Kelchtermans, G. (2013). Pedagogical content knowledge: a systematic review of the way in which the concept has pervaded mathematics educational research. Teach. Teach. Educ. 34, 12–25. doi: 10.1016/j.tate.2013.03.001
Dunbar, K., and Fugelsang, J. (2007). “Scientific thinking and reasoning” in The Cambridge handbook of thinking and reasoning. ed. K. J. Holyoak (Cambridge: Cambridge Univ. Press), 705–725.
Fischer, F., Chinn, C. A., Engelmann, K. F., and Osborne, J., (Eds.). (2018). Scientific reasoning and argumentation: The roles of domain-specific and domain-general knowledge. New York, London: Routledge.
Ford, M. J. (2005). The game, the pieces, and the players: generative resources from two instructional portrayals of experimentation. J. Learn. Sci. 14, 449–487. doi: 10.1207/s15327809jls1404_1
Förtsch, C., Sommerhoff, D., Fischer, F., Fischer, M., Girwidz, R., Obersteiner, A., et al. (2018). Systematizing professional knowledge of medical doctors and teachers: development of an interdisciplinary framework in the context of diagnostic competences. Educ. Sci. 8:207. doi: 10.3390/educsci8040207
Furtak, E. M., Kiemer, K., Circi, R. K., Swanson, R., de León, V., Morrison, D., et al. (2016). Teachers’ formative assessment abilities and their relationship to student learning: findings from a four-year intervention study. Instr. Sci. 44, 267–291. doi: 10.1007/s11251-016-9371-3
Grossman, P., Compton, C., Igra, D., Ronfeldt, M., Shahan, E., and Williamson, P. W. (2009). Teaching practice: a cross-professional perspective. Teach. Coll. Rec. 111, 2055–2100. doi: 10.1177/016146810911100905
Heitzmann, N., Seidel, T., Hetmanek, A., Wecker, C., Fischer, M. R., Ufer, S., et al. (2019). Facilitating diagnostic competences in simulations: a conceptual framework and a research agenda for medical and teacher education. Frontline Learn. Res. 7, 1–24. doi: 10.14786/flr.v7i4.384
Helmke, A., and Schrader, F.-W. (1987). Interactional effects of instructional quality and teacher judgement accuracy on achievement. Teach. Teach. Educ. 3, 91–98. doi: 10.1016/0742-051X(87)90010-2
Hoge, R. D., and Coladarci, T. (1989). Teacher-based judgments of academic achievement: a review of literature. Rev. Educ. Res. 59, 297–313. doi: 10.3102/00346543059003297
Klahr, D., and Dunbar, K. (1988). Dual space search during scientific reasoning. Cogn. Sci. 12, 1–48. doi: 10.1207/s15516709cog1201_1
Klug, J., Bruder, S., Kelava, A., Spiel, C., and Schmitz, B. (2013). Diagnostic competence of teachers: a process model that accounts for diagnosing learning behavior tested by means of a case scenario. Teach. Teach. Educ. 30, 38–46. doi: 10.1016/j.tate.2012.10.004
Kramer, M., Förtsch, C., Boone, W. J., Seidel, T., and Neuhaus, B. J. (2021). Investigating pre-service biology teachers’ diagnostic competences: relationships between professional knowledge, diagnostic activities, and diagnostic accuracy. Educ. Sci. 11:89. doi: 10.3390/educsci11030089
Kramer, M., Förtsch, C., Stürmer, J., Förtsch, S., Seidel, T., and Neuhaus, B. J. (2020). Measuring biology teachers’ professional vision: development and validation of a video-based assessment tool. Cogent Educ. 7:11823155. doi: 10.1080/2331186X.2020.1823155
Kron, S., Sommerhoff, D., Achtner, M., Stürmer, K., Wecker, C., Siebeck, M., et al. (2022). Cognitive and motivational person characteristics as predictors of diagnostic performance: combined effects on pre-service teachers’ diagnostic task selection and accuracy. J. Math. Didakt. 43, 135–172. doi: 10.1007/s13138-022-00200-2
Kuhn, D., Schauble, L., and Garcia-Mila, M. (1992). Cross-domain development of scientific reasoning. Cogn. Instr. 9, 285–327. doi: 10.1207/s1532690xci0904_1
Kunter, M., Klusmann, U., Baumert, J., Richter, D., Voss, T., and Hachfeld, A. (2013). Professional competence of teachers: effects on instructional quality and student development. J. Educ. Psychol. 105, 805–820. doi: 10.1037/a0032583
Lawson, A. E. (1978). The development and validation of a classroom test of formal reasoning. J. Res. Sci. Teach. 15, 11–24. doi: 10.1002/tea.3660150103
Lawson, A. E., Clark, B., Cramer-Meldrum, E., Falconer, K. A., Sequist, J. M., and Kwon, Y.-J. (2000). Development of scientific reasoning in college biology: do two levels of general hypothesis-testing skills exist? J. Res. Sci. Teach. 37, 81–101. doi: 10.1002/(SICI)1098-2736(200001)37:1<81::AID-TEA6>3.0.CO;2-I
Lazonder, A. W., Hagemans, M. G., and de Jong, T. (2010). Offering and discovering domain information in simulation-based inquiry learning. Learn. Instr. 20, 511–520. doi: 10.1016/j.learninstruc.2009.08.001
Lazonder, A. W., Wilhelm, P., and Hagemans, M. G. (2008). The influence of domain knowledge on strategy use during simulation-based inquiry learning. Learn. Instr. 18, 580–592. doi: 10.1016/j.learninstruc.2007.12.001
Lin, S.-W. (2004). Development and application of a two-tier diagnostic test for high school students’ understanding of flowering plant growth and development. Int. J. Sci. Math. Educ. 2, 175–199. doi: 10.1007/s10763-004-6484-y
Loewenberg Ball, D., Thames, M. H., and Phelps, G. (2008). Content knowledge for teaching. J. Teach. Educ. 59, 389–407. doi: 10.1177/0022487108324554
Lorch, R. F., Lorch, E. P., Freer, B. D., Dunlap, E. E., Hodell, E. C., and Calderhead, W. J. (2014). Using valid and invalid experimental designs to teach the control of variables strategy in higher and lower achieving classrooms. J. Educ. Psychol. 106, 18–35. doi: 10.1037/a0034375
Peeraer, G., Scherpbier, A. J., Remmen, R., de Winter, B. Y., Hendrickx, K., et al. (2007). Clinical skills training in a skills lab compared with skills training in internships: comparison of skills development curricula. Educ. Health 20:125. doi: 10.067/684480151162165141
Pickal, A. J., Wecker, C., Neuhaus, B. J., and Girwidz, R. (2022). “Learning to diagnose secondary school students’ scientific reasoning skills in physics and biology: video-based simulations for pre-service teachers” in Learning to diagnose with simulations: Examples from teacher education and medical education. eds. F. Fischer and A. Opitz (Cham: Springer), 83–95. doi: 10.1007/978-3-030-89147-3_7
Ready, D. D., and Wright, D. L. (2011). Accuracy and inaccuracy in teachers’ perceptions of young children’s cognitive abilities. Am. Educ. Res. J. 48, 335–360. doi: 10.3102/0002831210374874
Renkl, A., Hilbert, T., and Schworm, S. (2009). Example-based learning in heuristic domains: a cognitive load theory account. Educ. Psychol. Rev. 21, 67–78. doi: 10.1007/s10648-008-9093-4
Rheinberg, F., Vollmeyer, R., and Burns, B. D. (2001). FAM: ein Fragebogen zur Erfassung aktueller Motivation in Lern-und Leistungssituationen (Langversion, 2001) [QCM: a questionnaire to assess current motivation in learning situations]. Diagnostica 47, 57–66. doi: 10.1026//0012-1924.47.2.57
Rotgans, J. I., and Schmidt, H. G. (2014). Situational interest and learning: thirst for knowledge. Learn. Instr. 32, 37–50. doi: 10.1016/j.learninstruc.2014.01.002
Schrader, F.-W. (2009). Anmerkungen zum Themenschwerpunkt diagnostische Kompetenz von Lehrkräften [The diagnostic competency of teachers]. Z. Päd. Psych. 23, 237–245. doi: 10.1024/1010-0652.23.34.237
Schunn, C. D., and Anderson, J. R. (1999). The generality/specificity of expertise in scientific reasoning. Cogn. Sci. 23, 337–370. doi: 10.1207/s15516709cog2303_3
Schwichow, M., Christoph, S., Boone, W. J., and Härtig, H. (2016a). The impact of sub-skills and item content on students’ skills with regard to the control-of-variables strategy. Int. J. Sci. Educ. 38, 216–237. doi: 10.1080/09500693.2015.1137651
Schwichow, M., Croker, S., Zimmerman, C., Höffler, T., and Härtig, H. (2016b). Teaching the control-of-variables strategy: a meta-analysis. Dev. Rev. 39, 37–63. doi: 10.1016/j.dr.2015.12.001
Shulman, L. S. (1986). Those who understand: knowledge growth in teaching. Educ. Res. 15, 4–14. doi: 10.3102/0013189X015002004
Shulman, L. S. (1987). Knowledge and teaching: foundations of the new reform. Harv. Educ. Rev. 57, 1–23. doi: 10.17763/haer.57.1.j463w79r56455411
Stough, L. M., and Palmer, D. J. (2003). Special thinking in special settings: a qualitative study of expert special educators. J. Spec. Educ. 36, 206–222. doi: 10.1177/002246690303600402
Südkamp, A., Kaiser, J., and Möller, J. (2012). Accuracy of teachers’ judgments of students’ academic achievement: a meta-analysis. J. Educ. Psychol. 104, 743–762. doi: 10.1037/a0027627
Südkamp, A., Möller, J., and Pohlmann, B. (2008). Der simulierte Klassenraum: eine experimentelle Untersuchung zur diagnostischen Kompetenz [The simulated classroom: an experimental study on diagnostic competence]. Z. Päd. Psych. 22, 261–276. doi: 10.1024/1010-0652.22.34.261
Tschirgi, J. E. (1980). Sensible reasoning: a hypothesis about hypotheses. Child Dev. 51, 1–10. doi: 10.2307/1129583
Urhahne, D., and Wijnia, L. (2021). A review on the accuracy of teacher judgments. Educ. Res. Rev. 32:100374. doi: 10.1016/j.edurev.2020.100374
van Boxtel, C., and van Drie, J. (2018). “Historical reasoning: the interplay of domain-specific and domain-general aspects” in Scientific reasoning and argumentation: The roles of domain-specific and domain-general knowledge. eds. F. Fischer, C. A. Chinn, K. F. Engelmann, and J. Osborne (New York, London: Routledge), 142–161.
van Joolingen, W. R., and de Jong, T. (1997). An extended dual search space model of scientific discovery learning. Instr. Sci. 25, 307–346. doi: 10.1023/A:1002993406499
Voss, T., Kunter, M., and Baumert, J. (2011). Assessing teacher candidates’ general pedagogical/psychological knowledge: test construction and validation. J. Educ. Psychol. 103, 952–969. doi: 10.1037/a0025125
Wecker, C., Hetmanek, A., and Fischer, F. (2014). “Do cross-domain skills of scientific reasoning and argumentation exist?” in Proceedings of the international conference of the learning sciences (ICLS) 2014. eds. J. L. Polman, E. A. Kyza, D. K. O’Neill, I. Tabak, W. R. Penuel, and A. S. Jurow, et al. (Boulder: International Society of the Learning Sciences), 1192–1194.
Wecker, C., Hetmanek, A., and Fischer, F. (2016). Zwei Fliegen mit einer Klappe? Fachwissen und fächerübergreifende Kompetenzen gemeinsam fördern. [Two birds with one stone? Fostering domain-specific knowledge and cross-domain competences simultaneously]. Unterrichtswissenschaft 44, 226–238.
Wigfield, A. (1994). Expectancy-value theory of achievement motivation: a developmental perspective. Educ. Psychol. Rev. 6, 49–78. doi: 10.1007/BF02209024
Wildgans-Lang, A., Scheuerer, S., Obersteiner, A., Fischer, F., and Reiss, K. (2020). Analyzing prospective mathematics teachers’ diagnostic processes in a simulated environment. ZDM - Int. J. Math. Educ. 52, 241–254. doi: 10.1007/s11858-020-01139-9
Zimmerman, C. (2000). The development of scientific reasoning skills. Dev. Rev. 20, 99–149. doi: 10.1006/drev.1999.0497
Zimmerman, C. (2007). The development of scientific thinking skills in elementary and middle school. Dev. Rev. 27, 172–223. doi: 10.1016/j.dr.2006.12.001
Keywords: pre-service teacher education, diagnostic competences, professional knowledge, CK, PCK, cross-domain skills, scientific reasoning, video-based simulation
Citation: Pickal AJ, Engelmann K, Chinn CA, Neuhaus BJ, Girwidz R and Wecker C (2023) The diagnosis of scientific reasoning skills: how teachers’ professional knowledge predicts their diagnostic accuracy. Front. Educ. 8:1139176. doi: 10.3389/feduc.2023.1139176
Edited by:
Manuela Heinz, University of Galway, IrelandReviewed by:
Karin Binder, Ludwig-Maximilians-Universität München, GermanyNiclas Schaper, University of Paderborn, Germany
Copyright © 2023 Pickal, Engelmann, Chinn, Neuhaus, Girwidz and Wecker. This is an open-access article distributed under the terms of the Creative Commons Attribution License (CC BY). The use, distribution or reproduction in other forums is permitted, provided the original author(s) and the copyright owner(s) are credited and that the original publication in this journal is cited, in accordance with accepted academic practice. No use, distribution or reproduction is permitted which does not comply with these terms.
*Correspondence: Amadeus J. Pickal, cGlja2FsQHVuaS1oaWxkZXNoZWltLmRl