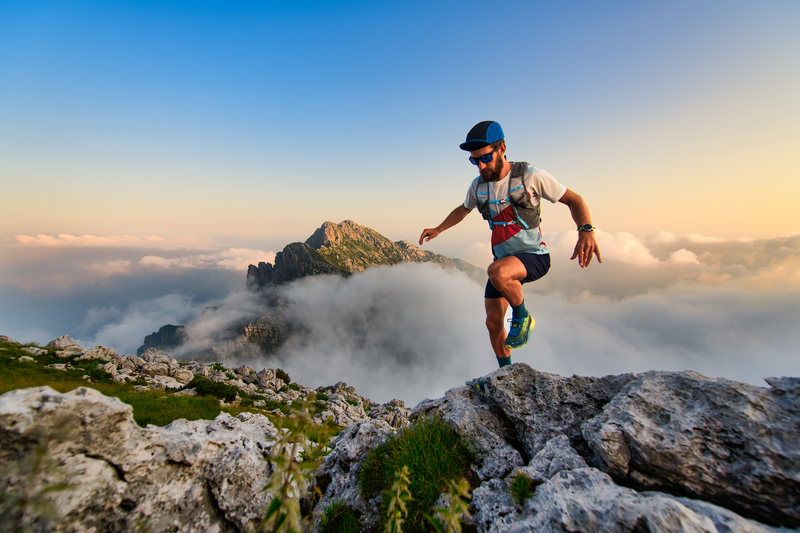
94% of researchers rate our articles as excellent or good
Learn more about the work of our research integrity team to safeguard the quality of each article we publish.
Find out more
ORIGINAL RESEARCH article
Front. Educ. , 27 July 2023
Sec. Educational Psychology
Volume 8 - 2023 | https://doi.org/10.3389/feduc.2023.1107341
This article is part of the Research Topic Digitalization in Education: Developing Tools for Effective Learning and Personalisation of Education View all 12 articles
The present research aimed to investigate whether Japanese elementary and secondary schools can accept computer-adaptive tests, which is an important issue under consideration for its future introduction to achievement assessments. We conducted two studies that asked elementary and secondary school students to take a computer-adaptive test and complete the questionnaires. We assessed individual differences in achievement goals and tested whether they predicted achievement scores on a computer-adaptive test. Moreover, we asked the students about their attitudes toward different forms of tests. The study results were twofold. First, those with high performance-avoidance goals did not perform worse than those with low performance-avoidance goals after controlling for individual differences in the approach to learnings, the mediating variable. This implies that the computer-adaptive test does not reinforce students’ anxiety about test taking. Second, students did not exhibit a more negative attitude toward the computer-adaptive test than the traditional fixed-item test but had a negative perception of human-adaptive tests (tests tailored by the teacher). Our results provide practical implications that a computer-adaptive test could be carefully introduced into the achievement assessment for Japanese elementary and secondary school children while considering their acceptance of the test.
A computer-adaptive test is an advanced testing algorithm based on psychometric theory and information technology. When students work on a computer-adaptive test, their ability level is estimated by their responses, and the most informative problems are subsequently presented to estimate their abilities with enhanced accuracy. Thus, students work on different problems, resulting in different time requirements. Computer-adaptive tests are widely used worldwide and have been introduced in the Test of English as Foreign Language (TOEIC), Program for International Student Assessment (PISA), and other academic achievement surveys in countries such as the US (Colwell, 2013) and Australia (Martin and Lazendic, 2018). The Ministry of Education, Culture, Sports, Science, and Technology in Japan is discussing a plan to introduce computer-adaptive tests into the achievement assessment for elementary and secondary school students. However, the culture of having all students attempt to solve the same problems simultaneously is deeply rooted in academic context in Japan, such as university entrance examinations (Arai and Mayekawa, 2005). Therefore, it is possible that individually tailored problems that are determined by computers may negatively impact students’ acceptance of computer-adaptive tests. We aimed to investigate whether Japanese elementary and secondary school students could accept computer-adaptive testing.
Students’ acceptance is a pertinent issue. It determines the measurement fairness of computer-adaptive testing. A computer-adaptive test is assumed to be a theoretically efficient testing technique as it personalizes problems to make a set of the most informative test problems for accurately estimating individual ability. However, if a computer-adaptive test is unacceptable and difficult for a particular student to work on, their abilities will not be fairly assessed using this testing technique.
Students will have a different experience when taking a computer-adaptive test as compared to a traditional test. The differences between these two test formats are summarized in Table 1. When taking a traditional test, all students work on the same problems, which include a wide range of difficulties. As the problems are predetermined, students can work on them in any order. In contrast, when taking a computer-adaptive test, each student works on a personalized problem set, as the problems are selected by an algorithm based on each student’s answer history. A computer-adaptive test does not provide too easy or too difficult problems for each student, which are inefficient for estimating student ability. As the problems are not predetermined, students must work on them in the order that they are presented.
Previous research on student acceptance of computer-adaptive testing has yielded mixed results. Theoretically, researchers have indicated that all students should be highly motivated to work on a computer-adaptive test as it can continue to present them with the most challenging problems (Weiss and Betz, 1973). Since the most challenging problems are neither too hard nor too easy, it is believed that students with high ability will not be bored by easy problems, and those with low ability will not be frustrated by difficult problems. Martin and Lazendic (2018) conducted a large-scale classroom experiment on elementary and secondary school students to compare their psychological responses to traditional and computer-adaptive testing. They reported that ninth-grade students who worked on a computer-adaptive tests experienced more positive motivations (e.g., self-efficacy and mastery orientation) and less negative motivations (e.g., anxiety and disengagement) than those who worked on a fixed-item test. Fritts and Marszalek (2010) conducted a classroom experiment with middle school students to compare their anxiety between the two test forms. They also reported that secondary school students who took a computer-adaptive test felt less situational anxiety than those who took a traditional paper-and-pencil test (i.e., a fixed-item test).
However, other studies have reported results suggesting that students find it difficult to accept computer-adaptive testing. Some studies report that highly anxious students are more likely to perform poorly on computer-adaptive tests (Ortner and Caspers, 2011; Lu et al., 2016). These results may be due to the familiarity with the traditional fixed-item test. When working on a fixed-item test, students can infer their abilities based on the number of problems they have solved. However, when working on a computer-adaptive test, students cannot infer their ability as they are constantly facing challenging problems, and the rate of correct answers is stable at approximately 50%. In a laboratory experiment on university students’ psychological responses to the two test forms, Tonidandel and Quinones (2002) found that students with large discrepancies between actual and perceived performance on computer-adaptive tests were less likely to accept test feedback. Students may feel that they are not solving the problems as well as they thought they were capable of when working on traditional tests, which may reinforce their anxiety about computer-adaptive tests.
Students’ acceptance of computer-adaptive testing procedures can also depend on their perceptions of their degree of freedom or controllability when solving test problems. Generally, as a set of problems is already determined and presented simultaneously in a fixed-item test, students can decide the order in which they will solve these. In contrast, as the set of problems is updated as the student progresses through the computer-adaptive test, they have almost no control over the problem order. In a survey on university students’ preferences towards various test forms, Tonidandel and Quinones (2000) discovered that students preferred a test in which they could skip problems over a test wherein they could not. Similar studies show that students feel more anxious when taking a computer-adaptive test than a fixed-item test (Ortner et al., 2014) or a computer-adaptive test that allows them the freedom to choose problems (Pitkin and Vispoel, 2001).
Although computer-adaptive testing is a technique actualized using information technology, in principle, it is possible to create a human-adaptive test in which some human experts, such as teachers, can determine problems for individuals based on their estimated abilities. Thus, whether the diagnosis is made by a human or computer is an important issue in research on the acceptance of algorithm-based diagnostic technology. With a few exceptions (Logg et al., 2019), laboratory experiments and survey research have revealed that people are less likely to accept results diagnosed and predicted by a computer than those predicted by a human expert (Promberger and Baron, 2006; Eastwood et al., 2011; Yokoi et al., 2020). This negative attitude toward computers is partially due to the concern that they cannot adequately infer human nature and ignore human uniqueness when making diagnoses or predictions (Lee, 2018; Castelo et al., 2019; Longoni et al., 2019).
Such a low acceptance of computer diagnostics is also observed in educational settings. Kaufmann and Budescu (2020) conducted a vignette-based experiment on teachers to compare their acceptance attitudes toward advice from human experts versus computer algorithms. They found that, when teachers decided which students should receive a remedial course based on students’ profiles and past performance, they were more likely to adopt advice from human experts (school counselors) than from computer algorithms. Kaufmann (2021) also reported that pre-service teachers made similar decisions due to their low evaluation of the reliability, accuracy, and trustworthiness of computer algorithms. Although they targeted teachers who were in a position to provide tests and not the students who work on tests, there is a commonality in that the target of diagnoses is human nature. Whether students can accept the test format may depend on whether the test is computer- or teacher-adaptive.
The present research aimed to investigate whether Japanese elementary and secondary school students can accept computer-adaptive testing. To the best of our knowledge, no research has examined this topic in a Japanese population. As the culture of having all students solve the same problems simultaneously is deeply rooted in academic context in Japan (Arai and Mayekawa, 2005), it is possible that Japanese students hold more negative attitudes towards computer-adaptive testing,. Our research aims to provide important insights into the generalizability of past findings about students’ acceptance of computer-adaptive testing. Moreover, our research sheds insight on how information technologies in educational practice in Japanese elementary and secondary schools could be utilized.
We began by investigating whether students who have testing anxiety performed more poorly on tests than those who do not. As a trait, we focused on individual differences in achievement goals. These goals are traditionally classified into mastery goals (to develop competence) and performance goals (to demonstrate competence) (Ames and Archer, 1987). Previously, researchers have revealed that students with high-performance goals are more likely to give up when confronted with difficult problems, while those with high-mastery goals persistently challenge them (Dweck, 1986). This is because students are anxious about avoiding situations in which failure exposes their personal weaknesses. If a computer-adaptive test reinforces students’ anxiety, those with high-performance goals are more likely to perform poorly. Achievement goals are known to correlate with students’ approach to learning (Senko et al., 2011, 2013) — conceptualized as the individual differences in students’ process and intention in learning (Entwistle et al., 1979). Thus, we investigated the direct effect of achievement goals on performance score after controlling for approaches to learning as mediators.
Second, we investigated whether students preferred computer-adaptive testing over other test formats. Specifically, we focused on comparisons between computer-adaptive testing, traditional fixed item testing, and human adaptive testing. It is essential to ascertain whether taking a “personalized” or “personalized by computer” test changes students’ preference for adaptive testing, as this finding can contribute to considering how teachers, as experts, should be involved in administering personalized tests using information technology.
In Study 1, we provided elementary and secondary school students in Japan with an opportunity to work on a computer-adaptive test and asked them to participate in our survey. We assessed their achievement goals and investigated the relationship between these goals and ability scores after controlling for the approach to learning. Furthermore, we assessed their attitudes toward three types of tests:(1) a test with the same problems (traditional fixed-item test), (2) a test tailored by the teacher (human-adaptive test), and (3) a test tailored by the computer (computer-adaptive test). We assessed these attitudes twice: before and after they worked on a computer-adaptive test, as their attitudes may have changed after completion.
A total of 870 students (474 fifth grade, 174 sixth grade, 163 seventh grade, and 58 unknown grade) from the Kansai region in Japan participated in this study. Students were recruited with the cooperation of school principals and district officials from June to September 2020, and their informed and voluntary consent was obtained prior to engaging in the study. A total of 415 students reported being male and 383 students reported being female.
The students participated in a classroom setting using a tablet PC. They were asked to log into an online system using a unique ID and password assigned to them in advance. First, an overview of the Computer Adaptive Test for Achievement Assessment of Elementary Education in Japan (CAT-ElemJP), the computer-adaptive test system originally developed by the authors, was provided by the test administrators. The CAT-ElemJP has a database of problems from the national achievement assessment (AY2019) with its psychometric properties, and is designed to ask for the most appropriate problems to estimate individual ability according to a certain algorithm. Students were told that their abilities would be estimated in real time, and that the most appropriate problems for each student would be asked to estimate their abilities, similar to a vision test. They were also informed that the number and types of problems they encountered could vary from person to person.
Next, the participants were asked to convey their attitudes toward the types of tests. The questions were: ‘How do you feel when you hear that everyone in your class will be tested on the same problems?’, “How do you feel when you hear that everyone in your class will be tested on the problems that the teacher tailored to each student?,” and “How do you feel when you hear that everyone in your class will be tested on the problems that the computer tailored to each student?” They were asked to report their attitudes on a 3-point Likert scale, where 1 = bad, 2 = neither good nor bad, and 3 = good.
In the next step, they worked on CAT-ElemJP. The first three problems were tutorials familiarizing them with the operation of this application (e.g., answer submission and text/numerical value input). The next three problems were the same for all participants. However, from the fourth problem, different problems were presented to each individual according to their answer history, as assigned by the programmed algorithm. Participants were given problems in three subjects (reading and writing in Japanese, arithmetic, and science), and their ability values for each subject were estimated according to their answer histories.
After completing the CAT-ElemJP, the participants completed two psychological scales: the scale for achievement goals and the scale for the approach to learning developed by Goto et al. (2018). They found that these scales were sufficiently reliable (α = 0.56 ~ 0.81). Moreover, they tested the validity of these scales by running a correlation analysis between achievement goals, approach to learning, self-efficacy, and affective experiences in learning. They reported that mastery-approach goals and a deep approach to learning were positively correlated with self-efficacy and enjoyment, and negatively correlated with boring. As we initially focused on science, one of the three subjects, in Study 1, we used these scales in a format that asked about learning science.
The scale for achievement goals consisted of two subscales (mastery-approach and performance-approach goals) with three items each. The students were asked to indicate the extent to which each item was true regarding the goals set when studying science. Example items are “to learn as much as possible by studying science” (mastery-approach goal) and “to get a better grade at science exams than other classmates” (performance-approach goal). Ratings were made on 4-point Likert-type scale with anchors ranging from 1 (not true of me) to 4 (extremely true of me).
The scale for the learning approach consists of two subscales (deep and surface approaches) with two items each. The students were asked to indicate how often they engaged in each activity when studying science. Example items are “I try to read science books even though they contain material I have not learned in science class” (deep approach), and “When I read a textbook, I try to remember the topics that might come up in exams” (surface approach). Ratings were made on 4-point Likert-type scales, with anchors ranging from 1 (never) to 4 (always).
Finally, participants were asked to respond with their impressions of the three types of tests with the same questions posed to them prior to taking the CAT-ElemJP.
We conducted a path analysis to examine the relationship between achievement goals, approaches to learning, and ability scores estimated by the CAT-ElemJP. In the tested model, the two subscales of achievement goals were hypothesized to have direct and indirect effects on ability scores through the two subscales of approaches to learning. The two subscales of achievement goals were hypothesized to covary, as were the two subscales of approach to learning and the three ability scores. Students’ grades and gender (female = 1, male/others = 0) were entered as control covariates.
We report the estimated effects in Figure 1, but omit the non-significant paths to avoid complicating the diagrams. All the estimated coefficients are reported in the Supplementary material (Supplementary Table S1). As the tested model was a satiated model, we did not report any fit values. The ability scores were positively and negatively predicted by deep and surface approaches to learning, respectively. The mastery goal was strongly associated with the deep approach to learning but did not predict any of the ability scores directly. Similarly, the performance-approach goal was strongly associated with the surface approach to learning but did not predict any of the ability scores directly.
Figure 1. Significant path (standardized coefficients) for the path model in Study 1. Tested but non-significant paths and control variables (gender and grade) were omitted in the diagram.
We conducted a 2 (before vs. after) × 3 (the same problems vs. tailored by the teacher vs. tailored by the computer) ANOVA to compare the attitudes toward the different test types before and after the students worked on the computer-adaptive test. The results revealed that the main effect of the test type was significant, F (2, 1,338) = 90.16, p < 0.05, η2g = 0.04. Multiple comparisons with Shaffer’s methods showed that students preferred the test tailored by the computer (M = 2.71, SD = 0.52), followed by the test tailored by the teacher (M = 2.67, SD = 0.55) and the test with the same problems (M = 2.44, SD = 0.59). Neither the main effect of time, F (1, 669) = 0.06, p = 0.80, η2g = 0.00 nor the interaction effects, F (2, 1,338) = 0.64, p = 0.52, η2g = 0.00, were revealed (see Figure 2).
Figure 2. Students’ attitudes toward each test before and after they worked on a computer-adaptive test in Study 1.
The results indicated that the students had acceptable attitude toward a computer-adaptive test in the same way as they did the traditional fixed-item test. The performance goal did not directly predict the students’ ability scores, which implies that the computer-adaptive test did not reinforce their anxiety about test taking. Furthermore, the students had more positive attitudes toward the computer-adaptive test than the traditional fixed-item and human-adaptive tests, even though they worked on a computer-adaptive test. These results imply that the computer-adaptive test is not immediately rejected by Japanese elementary and secondary school students.
Study 2 involved a more detailed investigation of what was revealed in Study 1 by refining the procedures regarding two points. First, we included the classification of performance-approach and performance-avoidance goals in our assessment of student achievement goals. Performance goals can be classified into two categories in terms of valance, approach, and avoidance, and these goals mainly cause anxiety and performance impairment (Elliot et al., 1999; Elliot and McGregor, 2001). We investigated whether the performance-avoidance goal would not be a direct negative predictor of ability scores on a computer-adaptive test.
Second, we assessed students’ attitudes toward the tests, asking them to imagine a more detailed situation in which teachers planned to utilize each test type to refine the educational process. Tests are not only used to estimate students’ abilities but also to enhance the way students learn and are taught based on these estimations. A computer-adaptive test, which is a technique for personalizing or tailoring tests for each student, may also be used to personalize their learning environment. By asking the students to imagine such prospects for teachers planning to utilize each test, we assessed their attitudes toward four test types: a test with the same problems (a traditional fixed-item test), a test with different problems (a fixed-item but somewhat non-traditional test), a test tailored by the teacher (a human-adaptive test), and a test tailored by the computer (a computer-adaptive test). Since their experiences in working on a computer-adaptive test did not change their attitudes for each test in Study 1, we only assessed their attitudes toward test types once they had completed the computer-adaptive test.
By focusing on the students’ attitudes toward each test, we can investigate individual differences in perceived test values and their relationship with their attitudes. Suzuki (2011) has classified the perceived test values into four aspects of “improvement,” “pacemaker,” “enforcement,” and “comparison.” Students who consider “improvement” and “pacemaker” to be more valuable are more likely to be motivated by intrinsic factors and engage in a deep approach to learning, whereas those who place greater value on “enforcement” and “comparison” are the opposite. If students communicate adequately with their teachers to share the standards and purposes of the test, they can accept the test value of “improvement” and “pacemaker” (Suzuki, 2012). Thus, by examining the relationship between perceived test values and accepting attitudes toward tests, it is possible to consider how communication can change attitudes toward acceptance of computer-adaptive testing.
A total of 745 students (540 fifth grade, 90 sixth grade, 100 seventh grade, and 15 unknown grades) from the Kansai region in Japan participated in the study. Students were recruited with the cooperation of school principals and district officials from March to July 2021. Students’ informed and voluntary consent to participate was obtained prior to participating in the study. A total of 334 students reported being male and 353 students reported being female.
In Study 2, participants first worked on the CAT-ElemJP. Next, they completed two psychological scales: the scale for achievement goals and the scale for approach to learning. In Study 2, we used these scales in a subject-free format and asked about learning in general. In addition, the three types of achievement goals were assessed using single-item measures for each of the following goals: mastery (“to learn as much as possible by studying”), performance-approach (“to do better than other classmates in science.”), and performance avoidance (“to avoid feeling bad about not being able to study as well as other classmates”). Thus, we intended to reduce the participants’ response efforts. We determined which items to use based on the results obtained by analyzing the datasets from Study 1 and the authors’ previous studies applying item response theory. We estimated the item parameters by applying generalized partial credit model for each subscale separately (the estimated parameters were reported in the Supplementary Tables S2−S4). We attempted to pick up item with high Discrimination and ordered thresholds. Ratings were made on a 4-point Likert-type scale with anchors ranging from 1 (not true of me) to 4 (extremely true of me). The scale for the learning approach was the same as that used in Study 1.
When asking students about their acceptance attitudes toward each test, we presented a pictualized vignette in the format of a four-panel comic to support students in imagining how the teacher planned to utilize each test to refine educational practice. The first panel described a situation wherein two students took an achievement assessment test in one of the four test formats. The second and third panels described how the problems were presented for one student who answered the first set of problems correctly and the other student who answered them incorrectly. The fourth panel described how the teacher dealt differently with these two students based on their test scores. We used free illustrations provided by https://www.irasutoya.com/ in our vignettes and created eight four-panel vignettes: one with male and one with female characters (to control for response bias due to characters’ gender), for the four tests: the test with the same problems (a traditional fixed-item test), the test with different problems (a fixed-item, but somewhat non-traditional test), the test tailored by the teacher (a human-adaptive test), and the test tailored by the computer (a computer-adaptive test). We present examples of male character vignettes for each condition in Figures 3–6. One of the eight vignettes was presented randomly to each participant. After the participants read the assigned vignettes, they rated their attitudes toward the test format on a four-point scale, where 1 = bad, 2 = rather bad, 3 = rather good, and 4 = good.
Figure 3. The vignette for the test with same problem conditions with male characters (translated into English).
Figure 4. The vignette for the test with different problem conditions with male characters (translated into English).
Figure 5. The vignette for the test tailored by the teacher condition with male characters (translated into English).
Figure 6. The vignette for the test tailored by the computer condition with male characters (translated into English).
Finally, the children responded to the scale regarding their perceptions of the test value. The children’s mindsets about test values were assessed using a single-item measure for each factor based on the scale developed by Suzuki (2012): “The purpose of the test was to check how I could understand what I learned.” (Improve), “The purpose of the test is to get students to develop study habits.” (Pacemaker), “Unless any tests are imposed, the students will not study at all.” (Enforcement), and “The purpose of the test is to distinguish between those with high intelligence and those without.” (Comparison). For each of the four items, the children were asked to indicate the extent to which they agreed using a 5-point Likert-type scale with anchors ranging from 1 (disagree) to 5 (agree).
We conducted a path analysis to examine the relationship between achievement goals, approaches to learning, and ability scores estimated by the CAT-ElemJP. In the tested model, the two subscales of achievement goals were hypothesized to have both direct and indirect effects on ability scores through the two subscales of approaches to learning. Three subscales of achievement goals were hypothesized to covary, as were the two subscales of approach to learning and the three ability scores. Students’ grades and gender (female = 1, male/others = 0) were entered as control covariates.
We report the estimated effects in Figure 7, and once again, we do not show non-significant paths to avoid complicating the diagrams. All estimated coefficients are reported in the Supplementary material (Supplementary Table S5). As the tested model was a satiated model, we did not report any fit values. Ability scores were positively predicted by the deep approach to learning, which is almost consistent with the results of Study 1. The mastery goal was strongly associated with the deep approach to learning, but did not predict any of the ability scores directly. Similarly, the performance-avoidance goal was associated with the surface approach to learning, but did not predict any of the ability scores directly.
Figure 7. Significant path (standardized coefficients) for the path model in Study 2. Tested but non-significant paths and control variables (gender and grade) were omitted from the diagram.
We conducted a 4 (the same problems vs. different problems vs. tailored by the teacher, vs. tailored by the computer) × 2 (targets are males vs. targets are females) ANOVA to compare the attitudes toward the different test types (Figure 8). The results revealed that the main effect of the test type was significant, F (3, 700) = 3.19, p < 0.05, η2g = 0.01. Multiple comparisons with Shaffer’s methods showed that only the difference between the attitude toward the test tailored by the computer and that tailored by the teacher was significant. Thus, students preferred the test tailored by the computer (M = 3.14, SD = 0.85) to that tailored by the teacher (M = 2.84, SD = 1.01). Neither the main effect of target gender, F (1, 700) = 1.67, p = 0.20, η2g = 0.00, nor the interaction effects, F (3, 700) = 1.06, p = 0.36, η2g = 0.00, were revealed.
We conducted a multiple regression analysis to investigate the predictive relationship of the four aspects of the perceived test values on the acceptance attitude toward each test after controlling for students’ gender and grade. The estimated coefficients are reported in Table 2. Results indicated that the “improvement” and “pacemaker” values significantly predicted only the acceptance attitude toward the test with the same problems. In addition, the “comparison” values significantly predicted acceptance attitude toward the test tailored by the teacher. No other relationships were revealed between perceived test values and acceptance attitudes toward each test.
Table 2. Estimated coefficients by multiple regression analysis on acceptance attitude toward each test type (Study 2).
Consistent with Study 1, the results indicated that students had acceptive attitudes toward a computer-adaptive test as they did a traditional fixed-item test. The performance-avoidance goal did not directly predict students’ ability scores, which supports the notion that the computer-adaptive test did not reinforce their anxiety.
Furthermore, even though the students considered how the teacher planned to utilize the results of each test, they did not have a more negative attitude toward the computer-adaptive test than the traditional fixed-item test. Rather, they had a negative attitude toward the human-adaptive test. These results support the notion that computer-adaptive tests are not immediately rejected by Japanese elementary and secondary school students. Adaptive aspects of the perceived test values (i.e., improvement and pacemaker) were related only to the acceptance attitude toward the traditional fixed-item test, but not to the other tests.
The present study sought to investigate whether Japanese elementary and secondary school students can accept computer-adaptive testing. First, we tested whether students who exhibited anxiety toward test-taking were more likely to perform poorly than those who did not. By focusing on achievement goals as a trait, the results revealed that those with high performance goals (Study 1) or performance-avoidance goals (Study 2) did not perform more poorly after controlling for the mediating variable, individual differences in the approach to learning. These results contrast with those of Lu et al. (2016), who reported that students with high anxiety were more likely to perform poorly on a computer-adaptive test. However, there is a difference in the analytical model as their research did not control for mediators, and our research did not examine anxiety directly. Therefore, future research should address whether this discrepancy can be integrated or is due to differences in test culture or other properties in computer-adaptive testing. Regarding the context in which Japanese elementary and secondary school students work on achievement assessment, we find that the format of a computer-adaptive test does not reinforce their test anxiety.
Second, we tested whether the students preferred computer-adaptive tests to other types of tests. The results of Study 1 revealed that students did not have a more negative attitude toward the computer-adaptive test than the traditional fixed-item test, even though they actually worked on a computer-adaptive test. While previous research has revealed that students have negative attitudes toward adaptive tests due to the lack of freedom in the order of problem solving (Tonidandel and Quinones, 2000; Pitkin and Vispoel, 2001; Ortner et al., 2014), students did not change their attitude after they worked on the computer-adaptive test in an order-fixed manner.
Consistent with Study 1, the results of Study 2 revealed that students did not hold negative attitudes towards the computer-adaptive test, even though they took into account how the teacher utilized the results of each test. Despite the dominant testing culture in Japan, where all students solve the same problems simultaneously (Arai and Mayekawa, 2005), we discovered that students do not have a negative attitude toward the test in which different problems are presented to each individual via a computer algorithm. These results suggest that the introduction of a computer-adaptive test for ability assessment in elementary and secondary school students is not immediately rejected due to the fairness in Japan.
When comparing computer-adaptive and human-adaptive tests, students consistently preferred the former in both studies, contrary to recent findings that people prefer diagnoses generated by a human expert over those formulated by a computer algorithm (Promberger and Baron, 2006; Eastwood et al., 2011; Kaufmann and Budescu, 2020; Yokoi et al., 2020). Even though the human-adaptive test is unrealistic, as long as teachers utilize computer diagnostics, a low-acceptance attitude toward teacher involvement in adaptive testing may break down in the classroom. Given the findings from previous research, which revealed that computer diagnoses were less acceptable due to low humanity (Lee, 2018; Castelo et al., 2019; Longoni et al., 2019) or trustworthiness (Kaufmann, 2021), trust may be the key to explaining this discrepancy in the results. Since Japanese students perceived the quality of student–teacher relationships to be low (Mikk et al., 2016), such perceived relationship quality may have impaired their acceptance attitudes toward the test tailored by the teacher. However, this is merely a speculation and needs to be empirically verified in future research, along with cultural and school climate differences.
The results of Study 2 revealed that perceived test values did not predict acceptance attitudes toward the computer-adaptive test. Among the four test types, only the traditional fixed-item test was positively predicted by adaptive values (i.e., “improvement” and “pacemaker”). While we did not find these values to predict an acceptance attitude toward a computer-adaptive test, this does not imply that we cannot encourage students to realize their adaptive value. It is possible that most students have only worked on traditional fixed-item tests, and even those who understand the adaptive value of the test well have still only accepted the traditional test. If teachers and students communicate sufficiently about the purpose and standard of the newly introduced test format (i.e., the computer-adaptive test), students may accept it. Conversely, the results also suggested that students may conservatively believe that traditional testing should continue. Given the results of Study 1, which revealed that merely taking the computer-adaptive test did not change their attitudes, we might have reached a tipping point to introducing the computer-adaptive test, where acceptance depends on whether the teacher can or cannot understand and share its value with students. It would be worthwhile for future research to examine these possibilities in an intervention study.
While our results can provide important implications for using computer-adaptive tests in Japanese elementary and secondary schools, there remains some lack of clarity due to some methodological limitations. First, as we asked students to work on our computer-adaptive test as a survey, it is not clear whether they would react in the same way if they worked on it as a high-stakes test, like an entrance exam or a diagnostic test. Second, we revealed that students have acceptable attitudes toward computer-adaptive testing but did not investigate whether this test would help students learn more efficiently. It is necessary to accumulate and systematically organize research findings with different test settings and investigate different outcomes.
This study provides valuable insights into whether students can accept a computer-adaptive test where the survey was conducted on a highly generalizable sample of elementary and secondary school students in an ecologically valid classroom setting. Our results also provide practical implications that a computer-adaptive test could be introduced into the achievement assessment in Japanese elementary and secondary schools as students have not rejected it due to the fairness. As there are other issues involved in applying computers to testing than usability and judging the correctness of answers, it is necessary to consider what form of testing should be introduced from multiple perspectives. In addition, the time of conducting the research (2020–2021) should be considered when interpreting the results. While computer-adaptive testing is not yet widespread in Japanese schools, it is possible that in the future, as computer-adaptive testing becomes more widespread, students’ acceptance attitudes could change in either a positive or negative direction. Future research should continue to examine students’ acceptance attitudes toward computer-adaptive testing, considering that attitudes may shift with changes in school circumstances.
The raw data supporting the conclusions of this article will be made available by the authors, without undue reservation.
The studies involving human participants were reviewed and approved by ethical committee of Shiga University. The participants’ legal guardian/next of kin provided their written informed consent to participate in this study on behalf of the participants. Participants also provided their assent for their participation in the survey form.
TG, KK, and TS developed the study concept and design, and collected the data in Study 1 and Study 2. TG analyzed the data and wrote the manuscript. KK and TS provided critically revised the manuscript. All authors contributed to the article and approved the submitted version.
This research was supported by the Japan Society for the Promotion of Science (Grant-in-Aid for Scientific Research (B) 18H01070).
We appreciate the teachers and students for participating in the study and cooperating with data collection.
The authors declare that the research was conducted in the absence of any commercial or financial relationships that could be construed as a potential conflict of interest.
All claims expressed in this article are solely those of the authors and do not necessarily represent those of their affiliated organizations, or those of the publisher, the editors and the reviewers. Any product that may be evaluated in this article, or claim that may be made by its manufacturer, is not guaranteed or endorsed by the publisher.
The Supplementary material for this article can be found online at: https://www.frontiersin.org/articles/10.3389/feduc.2023.1107341/full#supplementary-material
Ames, C., and Archer, J. (1987). Mothers’ beliefs about the role of ability and effort in school learning. J. Educ. Psychol. 79, 409–414. doi: 10.1037/0022-0663.79.4.409
Arai, S., and Mayekawa, S. (2005). The characteristics of large-scale examinations administered by public institutions in Japan: from the viewpoint of standardization. Jpn. J. Res. Test. 1, 81–92.
Castelo, N., Bos, M. W., and Lehmann, D. R. (2019). Task-dependent algorithm aversion. J. Mark. 56, 809–825. doi: 10.1177/0022243719851788
Colwell, N. M. (2013). Test anxiety, computer-adaptive testing, and the common core. J. Educ. Train. Stud. 1, 50–60. doi: 10.11114/jets.v1i2.101
Dweck, C. S. (1986). Motivational processes affecting learning. Am. Psychol. 41, 1040–1048. doi: 10.1037/0003-066X.41.10.1040
Eastwood, J., Snook, B., and Luther, K. (2011). What people want from their professionals: attitudes toward decision-making strategies. J. Behav. Decis. Mak. 25, 458–468. doi: 10.1002/bdm.741
Elliot, A. J., and McGregor, H. A. (2001). A 2 × 2 achievement goal framework. J. Pers. Soc. Psychol. 80, 501–519. doi: 10.1037/0022-3514.80.3.501
Elliot, A. J., McGregor, H. A., and Gable, S. (1999). Achievement goals, study strategies, and exam performance: a mediational analysis. J. Educ. Psychol. 91, 549–563. doi: 10.1037/0022-0663.91.3.549
Entwistle, N., Hanley, M., and Hounsell, D. (1979). Identifying distinctive approaches to studying. High. Educ. 8, 365–380. doi: 10.1007/BF01680525
Fritts, B. E., and Marszalek, J. M. (2010). Computerized adaptive testing, anxiety levels, and gender differences. Soc. Psychol. Educ. 13, 441–458. doi: 10.1007/s11218-010-9113-3
Goto, T., Nakanishi, K., and Kano, K. (2018). A large-scale longitudinal survey of participation in scientific events with a focus on students’ learning motivation for science: antecedents and consequences. Learn. Individ. Differ. 61, 181–187. doi: 10.1016/j.lindif.2017.12.005
Kaufmann, E. (2021). Algorithm appreciation or aversion? Comparing in-service and pre-service teachers’ acceptance of computerized expert models. Comput. Educ. 2:100028. doi: 10.1016/j.caeai.2021.100028
Kaufmann, E., and Budescu, D. V. (2020). Do teachers consider advice? On the acceptance of computerized expert models. J. Educ. Meas. 57, 311–342. doi: 10.1111/jedm.12251
Lee, M. K. (2018). Understanding perception of algorithmic decisions: fairness, trust, and emotion in response to algorithmic management. Big Data Soc. 5:205395171875668. doi: 10.1177/2053951718756684
Logg, J. M., Minson, J. A., and Moore, D. A. (2019). Algorithm appreciation: people prefer algorithmic to human judgement. Organ. Behav. Hum. Decis. Process. 151, 90–103. doi: 10.1016/j.obhdp.2018.12.005
Longoni, C., Bonezzi, A., and Morewedge, C. K. (2019). Resistance to medical artificial intelligence. J. Consum. Res. 46, 629–650. doi: 10.1093/jcr/ucz013
Lu, H., Hu, Y., and Gao, J. (2016). The effects of computer self-efficacy, training satisfaction and test anxiety on attitude and performance in computerized adaptive testing. Comput. Educ. 100, 45–55. doi: 10.1016/j.compedu.2016.04.012
Martin, A. J., and Lazendic, G. (2018). Computer-adaptive testing: implications for students’ achievement, motivation, engagement, and subjective test experience. J. Educ. Psychol. 110, 27–45. doi: 10.1037/edu0000205
Mikk, J., Krips, H., Säälik, Ü., and Kalk, K. (2016). Relationships between student perception of teacher-student relations and PISA results in mathematics and science. Int. J. Sci. Math. Educ. 14, 1437–1454. doi: 10.1007/s10763-015-9669-7
Ortner, T. M., and Caspers, J. (2011). Consequences of test anxiety on adaptive versus fixed item testing. Eur. J. Psychol. Assess. 27, 157–163. doi: 10.1027/1015-5759/a000062
Ortner, T. M., Weißkopf, E., and Koch, T. (2014). I will probably fail: higher ability students’ motivational experiences during adaptive achievement testing. Eur. J. Psychol. Assess. 30, 48–56. doi: 10.1027/1015-5759/a000168
Pitkin, A. K., and Vispoel, W. P. (2001). Differences between self-adapted and computerized adaptive tests: a meta-analysis. J. Educ. Meas. 38, 235–247. doi: 10.1111/j.1745-3984.2001.tb01125.x
Promberger, M., and Baron, J. (2006). Do patients trust computers? J. Behav. Decis. Mak. 19, 455–468. doi: 10.1002/bdm.542
Senko, C., Hama, H., and Belmonte, K. (2013). Achievement goals, study strategies, and achievement: a test of the “learning agenda” framework. Learn. Individ. Differ. 24, 1–10. doi: 10.1016/j.lindif.2012.11.003
Senko, C., Hulleman, C. S., and Harackiewicz, J. M. (2011). Achievement goal theory at the crossroads: old controversies, current challenges, and new directions. Educ. Psychol. 46, 26–47. doi: 10.1080/00461520.2011.538646
Suzuki, M. (2011). How learning strategies are affected by the attitude toward tests: using competence as a moderator. Jpn. J. Res. Test. 7, 51–65. doi: 10.24690/jart.7.1_51
Suzuki, M. (2012). Relation between values of a test and the method of testing: focusing on informed assessment and the contents of tests. Jpn. J. Educ. Psychol. 60, 272–284. doi: 10.5926/jjep.60.272
Tonidandel, S., and Quinones, M. A. (2000). Psychological reactions to adaptive testing. Int. J. Sel. Assess. 8, 7–15. doi: 10.1111/1468-2389.00126
Tonidandel, S., and Quinones, M. A. (2002). Computer-adaptive testing: the impact of test characteristics on perceived performance and test takers’ reactions. J. Appl. Psychol. 87, 320–332. doi: 10.1037/0021-9010.87.2.320
Weiss, D. J., and Betz, N. E. (1973). Ability measurement: conventional or adaptive? [Research Report 73–1]. [Minneapolis, MN]: University of Minnesota
Keywords: computer-adaptive test, acceptance, elementary school, secondary school, achievement goal, perceived value of test
Citation: Goto T, Kano K and Shiose T (2023) Students’ acceptance on computer-adaptive testing for achievement assessment in Japanese elementary and secondary school. Front. Educ. 8:1107341. doi: 10.3389/feduc.2023.1107341
Received: 24 November 2022; Accepted: 21 June 2023;
Published: 27 July 2023.
Edited by:
Laura Sara Agrati, University of Bergamo, ItalyReviewed by:
Abdulla Al Darayseh, Emirates College for Advanced Education, United Arab EmiratesCopyright © 2023 Goto, Kano and Shiose. This is an open-access article distributed under the terms of the Creative Commons Attribution License (CC BY). The use, distribution or reproduction in other forums is permitted, provided the original author(s) and the copyright owner(s) are credited and that the original publication in this journal is cited, in accordance with accepted academic practice. No use, distribution or reproduction is permitted which does not comply with these terms.
*Correspondence: Takayuki Goto, Z290by50Lmh1c0Bvc2FrYS11LmFjLmpw
Disclaimer: All claims expressed in this article are solely those of the authors and do not necessarily represent those of their affiliated organizations, or those of the publisher, the editors and the reviewers. Any product that may be evaluated in this article or claim that may be made by its manufacturer is not guaranteed or endorsed by the publisher.
Research integrity at Frontiers
Learn more about the work of our research integrity team to safeguard the quality of each article we publish.