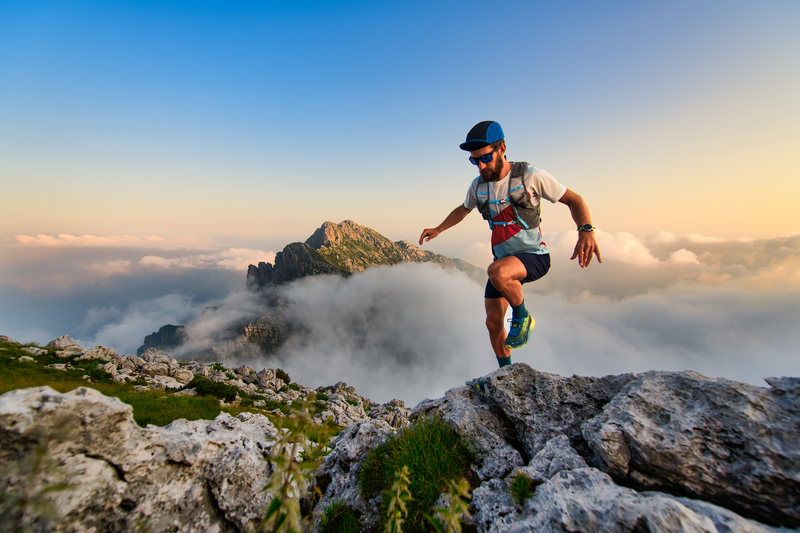
94% of researchers rate our articles as excellent or good
Learn more about the work of our research integrity team to safeguard the quality of each article we publish.
Find out more
ORIGINAL RESEARCH article
Front. Educ. , 06 February 2023
Sec. Assessment, Testing and Applied Measurement
Volume 8 - 2023 | https://doi.org/10.3389/feduc.2023.1092714
Psychological factors play an important role in higher education. With respect to students' understanding of scientific research methods, research self-efficacy (RSE) has been studied as a core construct. However, findings on antecedents and outcomes of RSE are oftentimes heterogeneous regarding both its theoretical and empirical structures. The present study helps disentangle these findings by (a) establishing and validating an integrated, multi-dimensional assessment of RSE and (b) introducing a developmental perspective on RSE by testing the impact of the disciplinary context and academic seniority on both mean level and latent structure of RSE. The construct validity of the new measure was supported based on RSE assessments of 554 German psychology and educational science students. Relations to convergent and discriminant measures were as expected. Measurement invariance and LSEM analyses revealed significant differences in latent model parameters between most sub-groups of training level and disciplinary context. We discuss our findings of measurement non-invariance as a feature rather than a bug by stressing a process-oriented perspective on RSE. In this regard, we conclude potential future directions of research and RSE theory development, alongside implications for methods education practice in higher education.
Teaching the understanding and application of scientific research methods is a central aim of almost every empirical higher education program. Apart from mere research knowledge and skills, psychological factors play an important role in student development. At this, research self-efficacy (RSE) was defined as students' “confidence in successfully performing tasks associated with conducting research” (Forester et al., 2004, p. 4). Interestingly, RSE is not only an outcome of academic education itself but also a predictor of other desirable outcomes of university education. According to social cognitive career theory (Lent et al., 1994), these are interest in research, research productivity, or career choice (Livinţi et al., 2021).
The available international literature on RSE in higher education is relatively broad. However, the proposed theoretical and empirical structures of RSE are oftentimes inconsistent. First, theoretical conceptions of RSE vary depending on the number and nature of sub-factors (Forester et al., 2004). Second, some measures even show discrepancies between theoretically posed and empirically found structures within themselves (Forester et al., 2004; Bieschke, 2006), thus calling into question their validity. The present study helps disentangle these findings by (a) establishing and validating an integrated, multi-dimensional measure of RSE and (b) introducing a developmental perspective on RSE by testing the impact of the disciplinary context and academic seniority on both structure and level of RSE. Such a developmental perspective on RSE might contribute to explaining the current heterogeneous landscape and, thus, enable a systematic and coherent investigation of RSE antecedents, development, and outcomes. Such findings can help improve methods education by understanding differentiated student needs linked to their individual background or facilitating diagnostics for mentoring. On a larger scale, a developmental perspective on RSE can help develop and evaluate evidence-based learning settings, which take differentiating effects on RSE into account. Pointedly fostering RSE facilitates sustainable educational outcomes such as interest and productivity, beyond mere knowledge and skill generation.
Perceived self-efficacy is a loose hierarchical construct and assumes that a general self-efficacy factor is generalized from domain-specific self-efficacy beliefs (Bandura, 2006). All specific self-efficacy beliefs are related to general self-efficacy. However, since specific self-efficacy beliefs develop from domain-specific factors, they also differ from the general factor, as well as from each other (Bandura, 1997). Specific influences are, for example, epistemological beliefs about the domain at hand (Mason et al., 2013), the value a person ascribes to this specific domain (Finney and Schraw, 2003; Bandura, 2006), and, most importantly, domain-specific mastery experience (Bandura, 2006). Thus, self-efficacy varies intra-individually based on its different domains and the formation of a specific realm. Each specific self-efficacy, thus, needs to be given individual in-depth theoretical and empirical attention. Here, we focus on research self-efficacy, a sub-domain of academic self-efficacy, in the context of empirical social sciences.
Despite its importance, the RSE theory is not well-developed yet, and the theoretical structures of RSE that have been proposed so far are quite varied (Forester et al., 2004). Most scholars assume a second-order factor structure (Phillips and Russell, 1994; Bieschke et al., 1996; O'Brien et al., 1998; Bieschke, 2006). Commonly, the sub-factors represent the different stages in the research process, such as (1) literature review and development of a research question, (2) research design and data collection, (3) data analysis and Interpretation, and (4) communication of results to the scientific community. Following Bandura's (2006) suggestion on self-efficacy assessment, the respective items that are assumed to assess each sub-factor list concrete research tasks (e.g., “develop researchable questions,” “obtain appropriate subjects,” “chose an appropriate method of data analysis,” and “write a thesis”). However, apart from this general assumption, the number and nature of these sub-factors vary (Forester et al., 2004) between scholars.
Prominent RSE measures are the Research Self-Efficacy Scale (RSES, Bieschke et al., 1996), the Self-Efficacy in Research Measure (SERM, Phillips and Russell, 1994), the Research Attitudes Measure (RAM, O'Brien et al., 1998), and the Research Self-Efficacy Scale (Holden et al., 1999). These have been employed in various studies, as just recently mentioned in a meta-analysis by Livinţi et al. (2021). While these measures are all valuable to measure RSE within their perspective, the conceptual differences between them invite skepticism on whether results drawn from studies that employ each instrument can be compared and pooled meaningfully.
Bieschke et al. (1996) propose four factors based on principal components analysis of items that were generated to represent the whole research process. The factors represent self-efficacy regarding research conceptualization, early tasks, research implementation, and presenting the results.
Similarly, Phillips and Russell (1994) also propose a four-factor structure of RSE, namely self-efficacy regarding research design, practical, writing, and quantitative skill. However, this structure is based on previous results of a principal components analysis of research skills employed by Royalty and Reising (1986). The respective items are drawn in part from this skill list, as well as from additional theoretical reflections to represent the four factors. Therefore, the content domain that items are sampled from differs from the one targeted by Bieschke et al. (1996), as do the proposed factor qualities.
Adding to the confusion, O'Brien et al. (1998) propose a six-factor structure of RSE that is based on a PCA of items written to represent the whole research process. These six factors are self-efficacy regarding discipline and intrinsic motivation, analytical skills, preliminary conceptualization skills, writing skills, application of ethics and procedures, and contribution and utilization of resources. Thus, the theoretically targeted content domain that items were drawn from (the whole research process) was the same as the domain targeted by Bieschke et al. (1996), but empirical analyses yielded a different number of sub-factors (six vs. four).
Concluding, in line with differences in theoretical conceptualization, these measures show discrepancies in the content and factor structure, not only between measures but even within themselves when comparing results from different studies (Forester et al., 2004; Bieschke, 2006). Such inconsistencies call into question both the conceptual and measurement validity and call for the advancement and integration of RSE measurement. Subsequently, enhanced RSE measurement enables coherent research that produces valid and comparable results.
A first step to resolve the heterogenous measurement landscape of RSE was initiated in 2004 by Forester and colleagues. The authors conducted a common factor analysis of 107 items from the three prominent U.S. American RSE measures, the SERM (Phillips and Russell, 1994), the RSES (Bieschke et al., 1996), and the RAM (O'Brien et al., 1998). Their analyses provided “information about the dimension of RSE that is not detectable in an analysis of respondents to just one instrument” (Forester et al., 2004, p. 6), thus laying the ground for advances in RSE measurement.
Based on EFA results from Forester et al. (2004), the Assessment of Self-Efficacy in Research Questionnaire (ASER, Klieme, 2021) was recently developed as a progression of RSE measurement and theory. Thus, previous progress achieved by various scholars was taken into account instead of starting from scratch. The ASER empirically finds a comprehensive understanding of RSE operationalization by integrating items from the existing heterogeneous approaches. The ASER is explicitly designed for Bachelor and Master students and is available in both German and English versions. It, thus, lays the groundwork for cross-national research due to its international developmental context. A detailed description of item selection based on EFA factor loadings and psychometric properties is provided by Klieme (2021).
Due to the promising but heterogeneous research landscape of RSE, further investigation seems beneficial: Is this heterogeneity (a) based on invalid measurement, or can it (b) be explained by a theory on RSE development? The measurement issue was tackled by the ASER development and validation (i.e., eliminating a bug). The present article focuses on a theory-based, developmental account for RSE heterogeneity (i.e., identifying a feature) by employing analyses of measurement (in)variance, taking a non-traditional approach.
Measurement invariance (MI) analyses are employed to test whether a measure's manifest scores represent the same latent construct in different groups, for example, RSE in psychology and educational science students. Commonly, nested models are fitted through multi-group confirmatory factor analyses (MGCFA, Jöreskog, 1971), increasingly constraining model parameters to be equal—i.e., invariant—across groups. Coined by Meredith (1993), these models represent the configural (equal factor structure), metric or weak (equal factor loadings), scalar or strong factorial (equal item intercepts), or strict (equal item residual variances) invariance of model parameters. MI should ideally be scalar at least for a valid comparison of manifest test scores (Fischer and Karl, 2019). Usually, a lack of MI is regarded as a weakness in measurement and eliminating or reducing it is an important endeavor during test development.
Another approach to dealing with a lack of MI might be to systematically probe potential reasons. One reason for the fractured picture of research self-efficacy might be that results stem from unidentified heterogeneous populations. If measurement variance was to occur between sub-groups of students that have not yet been recognized explicitly, the heterogeneous results might be systematic. Indeed, Fischer and Karl (2019) urge researchers to value non-invariance findings the same as invariance findings. Such findings might help us understand heterogeneous empirical results in the latent structure estimation of a malleable construct: if the “true” latent structure simply is heterogeneous, so should our empirical estimations. There are at least two dimensions where structural differentiation effects should be expected in RSE: students' training level in research methods and the different roles and/or amounts of specific methodologies in an academic discipline.
The first possible differentiating effect for RSE is the training level. Self-efficacy beliefs stem from mastery experience (Bandura, 2006). Hence, it can be reasoned that the amount of methods training affects RSE, as expanded methods training allows for extended mastery as well as failure experience—a differentiating effect in student self-efficacy. Such experiences of mastery or failure are particularly prevalent in hands-on training settings. Oftentimes, methods training in Bachelor programs is rather theoretical, with hands-on experience increasing in graduate training. Still, any investigation of RSE development and potential differentiation effects should cover all levels of higher education.
First, apart from mastery experience, self-efficacy is affected by epistemological beliefs and the value a person ascribes to the respective domain (Bandura, 2006), namely research. These two factors are probably stressed in undergraduate training already. Once in specific methods classes, but also in subject-matter classes by communicating the relevance of research for theory development. Second, undergraduate research experiences have been employed increasingly over the past years. The heft of this development is mirrored by the recent publication of the Cambridge Handbook of Undergraduate Research (Mieg et al., 2022) which provides an overview of theoretical approaches as well as practice examples in diverse disciplines and from countries across all continents.
Consequently, from their first semester on, students can be exposed to forces that shape their RSE. These forces may increase and specialize as training advances from undergraduate to graduate training. The amount of training might not only affect mean levels of RSE (Livinţi et al., 2021) but also engender differentiation and specification of self-efficacy beliefs regarding the various research tasks and the way they constitute students' self-efficacy in this domain. As a consequence, variation in construct structure might be interpreted as a conceptual change of research in the process of methods education. For example, Rochnia and Radisch (2021) argue that in educational contexts, learning implies change over time (hopefully), both regarding the mean level and construct structure. Thus, measurement non-invariance between training levels does not necessarily indicate low measurement validity (i.e., a bug), but rather a differentiation of a concept due to learning (i.e., a feature; Putnick and Bornstein, 2016; Rochnia and Radisch, 2021). Delineating the amount of measurement (in-) variance might help to understand the intra-individual differentiation of research as a specific domain of self-efficacy across the training level.
A second possible differentiating effect is the research culture in an academic discipline. Although most university training is comprised of research classes, their focus on specific methodology may vary between disciplines, partly due to differences in their genesis and research targets. For example, psychology and educational science are both empirical social sciences and share some overlap (e.g., educational psychology). However, even disciplines that appear akin at first sight may differ regarding their methodological gist. In German academics, a traditional focus on qualitative methods in educational science still holds strong today and qualitative methods are employed and refined in current research, whereas psychological research employs a wider range of rather advanced quantitative methods. Consequently, most German undergraduate methods training in psychology focus almost exclusively on quantitative methods, while methods training in educational science stress qualitative methods and vary much more across universities regarding the extent of undergraduate methods training. These and other potential disciplinary differences may foster different value perceptions and epistemological beliefs regarding research in students, which affect their self-efficacy beliefs (Bandura, 2006; Mason et al., 2013).
One may then ask how broadly RSE can be assessed invariantly across disciplines such as psychology and educational science? Otherwise put, do differences between disciplines evoke differentiated development of RSE in their students?
Disciplines might not only differ in the actual tasks or research methods but also in the emphasis given to a discipline's methods. As a consequence, if methods education is emphasized stronger in one discipline than in another, differences between disciplines might increase over time as students are socialized into the research culture of their respective programs. This might lead to an interaction effect of discipline and time in the program, as socialization and differences in research-specific mastery experiences increase over time.
The main focus of the study is to account for RSE heterogeneity. Nevertheless, the validity of measurement is an indispensable prerequisite for any research that aims to disentangle the heterogeneous findings that float around the RSE research realm. Because the ASER is a relatively new measure, we will delineate validity evidence before addressing the differentiation hypotheses central to this article.
This study was conducted in Germany with a German-speaking sample.1 Thus, same-construct measures used for convergent validity estimation should ideally be worded and validated in German so that they provide a legitimate anchor for the ASER items. Unfortunately, validated measures of RSE are missing at the Bachelors' and Masters' levels in the German-speaking realm. Lachmann et al. (2018) developed an instrument that assesses RSE in Ph.D. graduates and targets respective attainments that are not adequate for Bachelors' and Masters' levels (e.g., “I can build cooperations with central researchers in my field”). In younger students, RSE has mostly been included rather as a side note with unvalidated post-hoc items, or with a divergent structural focus (e.g., Gess et al., 2018; Pfeiffer et al., 2018). Still, items from these two studies seem to be the best option in selecting convergent validity items: They do assess RSE specifically and they were developed to assess German students at a pre-doctoral level.
Estimating the discriminant validity of the ASER requires constructs that are theoretically and empirically related to, but still distinct from RSE (Clark and Watson, 2019) to prevent a jangle fallacy.2 Those constructs are general self-efficacy (Bandura, 2006) and academic self-concept. While self-concept is rather general and past-informed, self-efficacy is more task-specific and future-oriented. However, both target self-beliefs (Choi, 2005). A further theoretically and empirically positive relation to RSE is given by attitudes toward research (Kahn and Scott, 1997; Livinţi et al., 2021).
Relations between RSE and neuroticism have not yet been tested empirically, but can be reasoned to be negative, as low self-esteem and a heightened sensitivity toward failure (Zhao et al., 2010) impede mastery experience, which is a core requisite for acquiring (research) self-efficacy (Bandura, 2006). Indeed, negative relations with a neuroticism that have been found for academic self-efficacy (Stajkovic et al., 2018) support this hypothesis regarding research self-efficacy.
Research self-efficacy construct validity is also supported by a lack of relation with constructs that are theoretically unrelated to RSE. Correspondingly, several authors report non-significant near-null correlations between agreeableness and academic self-concept (Marsh et al., 2006) or self-efficacy regarding college credits (De Feyter et al., 2012). Theoretically reasoned, the tendency to help others or not to be harsh would not affect a person's confidence in performing research tasks. Thus, based on theoretical reflections and considerations of empirical findings on the relation between agreeableness and other self-efficacies, a lack of relation between RSE and agreeableness would support RSE construct validity.
Test bias regarding gender will be explored in order to delineate whether the complete sample can be used for our analyses. Even today, the ratio of female and male students presumably differs between programs. It is, therefore, important to detect potential test bias before all students are analyzed in a common model. Meta-analytic results on gender mean differences in academic self-concept show an overall effect size of 0.08 favoring male students, specifically regarding mathematics and social-sciences self-efficacy (Huang, 2013). In contrast, in a meta-analysis on the relation specifically between research self-efficacy and gender, Livinţi et al. (2021) report non-significant differences between male and female students. However, those (non-) differences refer to manifest mean scores and can only be validly interpreted in unbiased measurement.
In addition, if MI can be established between female and male students, this indicates that the ASER is generally fit for valid invariant measurement. Following, a lack of MI between certain groups, as tested under the differentiation hypothesis, can be attributed to real group differences in measurement and probably does not stem from the weakness of the instrument.
Taken together, the aim of this study is pursued in two steps. First, the ASER will be evaluated as an integrative assessment progression, testing construct validity in the nomological net and gender bias. This way, the empirical usefulness of the ASER as a measure of RSE and the validity of the following analyses can be supported. Second, potential differentiating effects will be tested through measurement invariance (MI) analyses. These analyses will investigate potential reasons for the heterogeneous results reported in the literature so far. In particular, the following hypotheses will be tested in order to evaluate the ASER validity (hypotheses 1–4) and to identify a structural differentiation of the RSE construct (hypotheses 5–7).
H1—Convergent validity: Satisfactory fit of a comprehensive measurement model of RSE including items from different RSE measures is expected.
H2a–e—Discriminant validity: RSE will display moderate to high correlations with general self-efficacy, academic self-concept, research attitudes, and neuroticism (negative relation). The relation between agreeableness and RSE will be non-significant.
H3—Construct validity: Convergent validity coefficients will be higher than discriminant validity coefficients.
H4—Test bias: MI is expected between female and male students. Results will indicate whether further analyses should be conducted for both genders together or separately.
H5: Limited measurement invariance is expected between different training levels.
H6: Limited measurement invariance is expected between psychology and educational science students.
H7: An interaction effect of training level and discipline on the level of MI is expected.
Between June 2019 and January 2020, 648 students of psychology and educational science from various German universities filled out the questionnaires either during a lecture as a paper–pencil assessment, or online. Informed consent was confirmed by all participants before starting the questionnaire.
The initial data were prepared for analysis. Missing values were only examined for items on the psychological scales. Fifty-two cases with >10% (N = 2) missing values on ASER items were excluded from further analyses. The remaining data set (N = 596) contained 0.20% missing values on ASER items. No single ASER item showed ≥1% missing values. Missing value analysis suggested that data were missing completely at random (MCAR) according to a non-significant Little's MCAR test [ = 360.12, p < 0.10]. Thus, EM imputation of missing values was performed (Tabachnick and Fidell, 2013).
Univariate and multivariate outliers were calculated for each item individually on the ASER and for scale scores for the other constructs. Twenty-five cases with univariate outliers were found through z-scores (z > |3.11|) so that five cases with more than two extreme scores were deleted, but cases with one or two extreme scores were kept in the sample (Tabachnick and Fidell, 2013). Based on Cook's distance of < 0.03 for all variables as a more robust indicator of multivariate outliers, none were found in the sample.
Last, 37 students in the sample turned out to be neither psychology nor educational science majors in Bachelor or Master programs and were excluded from further analyses. Thus, the final sample comprised 554 students with a mean age of 23.72 years (SD = 4.53), of which 62% identified as female students, 33% as male students, 4% as non-binary, and 1% did not indicate their gender. Participants were sampled from different programs in psychology (56.5%) and educational science (43.5%) at different universities in Germany (48% from Frankfurt a. M., 44% from Bremen, and 8% from others like Jena, Kiel, or Freiburg PH). In this study, 68% were Bachelor students and 32% were Master students with a mean of 5.87 (SD = 4.22) total cumulated semesters at the university, including potential Bachelor and Master programs taken.
Research self-efficacy was assessed by the ASER, comprising 19 items. Students rated their “confidence in successfully performing the following tasks” on a 0 (“not at all confident”) to 10 (“completely confident”) Likert scale. Internal consistencies were good with Cronbach's α = 0.94 and McDonald's ωh = 0.77 for a general factor model (all ASER items). Parallel analysis suggested a three-factor model for which McDonald's ωt was 0.95 (sub-factors and items see Table 1). McDonald's ω is interpreted similarly to Cronbach's α but allows for different item loadings on the scale factor instead of assuming all loadings to be equal (as does Cronbach's α). Following McDonald's ω, ASER sub-scales should be used whenever possible, but a general RSE one-factor model is also reliable. In order to estimate ASER's convergent validity, an additional assessment of RSE was realized with 12 same-construct items: Nine items originally developed by Gess et al. (2018, e.g., “Analyze data qualitatively, even if I have never used this specific method before”) and three items developed by Pfeiffer et al. (2018, e.g., “Plan a research project”).
General self-efficacy was assessed by three items rated on an 11-point Likert scale (e.g., “In difficult situations, I can rely on my abilities;” Beierlein et al., 2012). The authors report reliability indicators of rtt = 0.50 as well as satisfactory construct and factorial validity. In this sample, the parallel analysis suggested a one-factor model with McDonalds ωt = 0.74.
General academic self-concept was measured by a five-item scale, developed by Dickhäuser et al. (2002) with McDonald's ωt = 0.83 in this study. Students rate their perceived abilities on a seven-point Likert scale from “very low” to “very high” (e.g., “I think my abilities in this program are...”).
Agreeableness and neuroticism were assessed on an 11-point Likert scale by four items each, taken from the short version of the Big Five Inventory (Rammstedt and John, 2005) with Cronbach‘s α ranging from 0.74 to 0.77 for neuroticism (McDonald's ωh = 0.79 in this sample) and from 0.59 to 0.64 for agreeableness (McDonald's ωh = 0.68). Considering the scale shortage and indicators of the strong factorial and construct validity, psychometric properties are acceptable.
Research attitudes were measured by the Revised Attitudes Toward Research Scale (Papanastasiou, 2014), a 13-item measure. In this sample, the parallel analysis suggested a model with four factors or two components and EFA revealed an interpretable two-factor solution with all positive and all negative attitudes items loading onto the two factors, respectively. Thus, this solution was used in this study with McDonalds ωt = 0.89 for the two-factor model. Sample items are “Research is connected to my field of study” or “Research makes me nervous.”
Furthermore, prior training was estimated by total semesters in university. Demographics included age, gender, current program and institution, and information on degrees.
Measurement and construct validity covariance structure models were fitted using the lavaan (Rosseel, 2012) and lavaan.survey (Oberski, 2014) packages in R (R Core Team, 2022).
Measurement invariance (MI) between categorical groups (gender, discipline, and program level) was analyzed by multi-group confirmatory factor analysis (MGCFA, Jöreskog, 1971). MI judgment was based on changes in fit statistics between the configural, metric, scalar, and strict models as suggested by Chen (2007) with cut-offs of ΔCFI < 0.01, ΔRMSEA < 0.015, and ΔSRMR < 0.03 (metric MI) or ΔSRMR < 0.015 (scalar and strict MI). RMSEA was considered cautiously because it tends to over-reject correct models in small samples (Chen et al., 2008).
In the case of non-categorical groups, MGCFA has the disadvantage of losing information due to categorizing continuous “grouping” variables into circumscribed groups which are (a) potentially variant within themselves and (b) in many cases arbitrarily divided (Hildebrandt et al., 2016). Local structural equation modeling (LSEM, Hildebrandt et al., 2009) overcomes this issue by testing continuous moderator effects on model parameters, e.g., across the number of semesters. Furthermore, LSEM can identify the onset of potential differences without requiring researchers to specify a moderating function a priori (Hildebrandt et al., 2016). Thus, in addition, (in)variance of model parameters across different levels of scientific training (operationalized by the amount semesters) was investigated with LSEM. LSEM analyses were computed in R using the sirt package (Robitzsch, 2015), and the wrapper function lsem.estimate as well as the R function lsem.permutation (Hildebrandt et al., 2016). For a comprehensible introduction to this relatively recent method, we refer to Hildebrandt et al. (2009, 2016).
The two-factor structure reported by Klieme (2021) could not be confirmed in this study. Here, the ASER items were better represented by a non-hierarchical three-factor model [ = 711.746, p = 0.000, CFI = 0.911, RMSEA = 0.083, SRMR = 0.048] which can be inspected in Table 1. This overall only moderate fit might be explained by non-invariance between different sub-groups, which renders a very good model fit impossible when the whole sample is analyzed together. Thus, differentiating effects between certain groups are still worthwhile to be analyzed, even if the overall model fit with data comprising all these potentially heterogenous groups indicates some issues. Consequences hereof will be addressed in the following MI analyses and the discussion. The three RSE sub-factors refer to Theoretical Aspects of research (nine items, e.g., “Generate researchable questions” or “Write a discussion section for my thesis”), Technical aspects (seven items, e.g., “Collect data” or “Know which data analysis method to use”), and Interpretation (three items, e.g., “Interpret and understand the results of my research”). The 12 convergent validation items were best represented by individual factors corresponding to their origin of publication (Gess et al., 2018; Pfeiffer et al., 2018, respectively).
Construct validity was investigated by a comprehensive model, comprising a measurement and a structure model (see Figure 1). RSE was modeled as a second-order factor with five sub-factors: three ASER sub-factors and one sub-factor for each set of validation items (Gess et al., 2018; Pfeiffer et al., 2018). In the structure model, discriminant constructs were modeled as one-factorial. One exception is attitudes toward research, which shows a hierarchical structure with a positive and negative attitudes sub-factor. Model fit was again moderate to poor [ = 4,539.186, p < 0.001, CFI = 0.838, RMSEACI95% = (0.056; 0.058), SRMR = 0.059]. Still, the loadings of the five sub-factors on the RSE super factor were all similar in size (see Figure 1). Thus, the ASER items represent RSE similarly to the validation items (H1a). Latent factor correlations between the ASER and each validation factor 1 and 2 were 0.92 (p < 0.001) and 0.86 (p < 0.001), respectively (for factor naming see Figure 1).
The relations in the nomological net hypothesized in H2 were confirmed, supporting the positioning of RSE in the nomological net. Validity coefficients are shown in Figure 1. Corresponding to H3, correlations supporting convergent validity were stronger than those supporting discriminant validity.
Measurement bias was tested through MI analyses using MGCFA. MI Interpretation was based on differences in fit statistics between the increasingly constrained models, with cut-offs of ΔCFI < 0.01, ΔRMSEA < 0.015, and ΔSRMR < 0.015 as suggested by Chen (2007). In addition, chi-square difference tests were considered in case of unclear results. However, since they tend to be overly conservative (Putnick and Bornstein, 2016), they were included with caution.
Subjects who identified as non-binary were excluded, because this group was too small (N = 23), as were seven subjects who did not indicate any gender. For the remaining 524 participants (183 male students and 341 female students), the ASER three-factor model displayed satisfactory fit in the MGCFA configural model [ = 699.938, p < 0.001, CFI = 0.908, RMSEA = 0.084, SRMR = 0.049]. Strict measurement invariance between female and male students was found, meaning that both groups displayed non-invariant factor loadings, item intercepts, and residual variances (for model statistics see Supplementary material B). Thus, the latent factor scores can be compared between genders (see Table 2). Differences in mean factor scores between female and male students were significant for the Technical Aspects factor only (p = 0.016) with manifest mean values of M = 5.25 (female students) vs. M = 5.60 (male students). The mean factor score differences were non-significant for the Theoretical Aspects factor and the Interpretation factor.
As a central purpose of this study, structural differentiation was tested through MI analyses of training level and academic discipline.3 In the first step, MGCFAs were conducted for categorical group comparisons. The MGFCA configural model was the same for MGCFA analyses regarding both grouping variables and included all subjects (N = 554). It displayed satisfactory fit [ = 711.746, p < 0.001, CFI = 0.911, RMSEA = 0.083, SRMS = 0.049]. Again, judgments were based mainly on changes in fit statistics. Figure 2 displays the findings of MI analyses of main and interaction grouping. For clarity, results are condensed to show the main trends. Detailed fit statistics can be inspected in Supplementary material B. In a second step, LSEM on the training level was employed exploratorily to determine specific points of measurement (in)variance.
Figure 2. Levels of measurement invariance between discipline and program level for total and sub-groups.
Overall strict MI was found between psychology and educational science students, that is non-invariant factor loadings, items intercepts, and residual variances. For the sub-factors, MI was scalar (equal factor means) for the Theoretical Aspects and strict for the Interpretation factor. Thus, means can be compared between both disciplines on these two sub-factors (see Table 2). For the Technical Aspects factors, configural MI indicated that the latent meaning of this factor is different for psychology and educational science students due to differences in factor loadings. Manifest scores should, thus, not be used for group comparison. The mean factor scores were significantly different for Theoretical Aspects (p = 0.017, manifest M = 6.13 and M = 5.79, respectively) but not for Interpretation (manifest M = 6.30 for both groups). Concluding, MI results between both disciplines varied for the three sub-factors.
Overall MI between program levels was found on a scalar level, that is invariant factor loadings and latent means. For the sub-factors, MI was strict for the Technical Aspects and Interpretation factors and metric for the Theoretical Aspects factor. Thus, test scores can be validly compared between training levels on the Technical Aspects and the Interpretation factor, while the Theoretical Aspects factor differ in meaning in Bachelor and Master students. Differences in mean factor scores were significant for both the Technical Aspects and the Interpretation factor, with p < 0.001 for both (see Table 2). Manifest scale means for Bachelor and Master students were M = 5.19 and M = 5.72 on the Technical Aspects scale, and M = 6.11 and M = 6.71 on the Interpretation scale, respectively. Again, MI results between program levels were mixed.
For a more detailed analysis, LSEM was conducted with training level as a moderator of model parameters. This way, changes in factor Interpretations across the training level can be analyzed continuously, and potential areas or onsets of changes can be delineated. Here, the training level was operationalized as the number of semesters.
For LSEM analyses, only cases without missing values on the analyzed variables (ASER items and the number of semesters) were included, resulting in a sample size of N = 519. Focal points for the local model estimations were chosen for values of 1–10 on the moderator, with an interval of one semester. This ensured a large enough effective sample size that entered each local analysis, ranging between Neff = 63.36 (10 semesters) and Neff = 151.50 (three semesters), with a mean of 123.55 (SD = 27.92). The number of permutations for testing the global and pointwise hypotheses was set to 1,000.
Fit indices for the locally estimated models were moderate to poor (see Supplementary material C for results of LSEM analyses). Overall model fit changed across training level, indicated by significant variation in SRMR (M = 0.065, SD = 0.052, p < 0.05). On α = 0.05 level, variations of loadings were overall significant for three items, and variations of residual variances were overall significant only for ASER6theory (“Write the introduction, theory, and discussion part of my thesis”). The three-item loadings that varied across semesters were ASER12theory (“write the discussion section for my thesis”), ASER1theory (“develop researchable questions”), and ASER2emp (“sample participants”). This means that the latent meaning of these items differs significantly across training levels and might explain why the model fit varies significantly.
Interaction effects occurred between discipline and training levels. When separating Bachelor and Master students, MI between disciplines changed with the training level: it decreased for the Theoretical Aspects factor but augmented for the Technical Aspects factor (see Supplementary material B5, B6). Viewed from the other angle, MI between Bachelor and Master was lower in the psychology than in the educational science sub-sample. This means that the differentiating effect of discipline on RSE latent structure is moderated by training level, or else, the differentiating effect of training level is stronger in psychology students.
This study aimed to propose and empirically test a differentiation hypothesis that might explain some of the heterogeneity in results on the RSE structure and measurement. We hypothesized differentiating effects that become salient in the course of higher education, affecting the latent structure and thus the construct meaning of RSE. The effects we investigated were program level and academic discipline, as well as their interaction. If these differentiating student characteristics go unregarded in the RSE literature, scholars miss out on an important factor to be considered in RSE research regarding the integration of results from common research efforts.
Since valid measurement of RSE is a prerequisite for our endeavor, we delineated the validity of the employed and recently developed ASER regarding construct validity and gender bias. RSE has been claimed by other scholars to be multi-faceted, and the existing American instruments all propose a multi-factor structure (Forester et al., 2004). Regarding the factor structure, the ASER is still under development. In this sample, a hierarchical three-factor structure emerged as the best-fitting model. The three sub-factors refer to research self-efficacy regarding Theoretical Aspects, Technical Aspects, and Interpretation.
Assessment of Self-Efficacy in Research Questionnaire construct validity was supported for all our hypotheses: relations to convergent items and discriminant constructs met expected directions and sizes. Thus, the ASER is fit to measure RSE as hypothesized within the nomological net, and ASER scores can be used to explore important relations in academic higher education.
Strict MI indicated that latent scores can be validly compared between female and male students. Doing so, the ASER reveals significant differences for the Technical Aspects factor but not for the other two factors. These mixed findings support the mixed findings reported previously: it may be that gender differences occur on the sub-scale level, but not on the total score level. The non-significant gender differences reported in a meta-analysis by Livinţi et al. (2021) are in accordance with overall MI and MI for the Theoretical Aspects and Interpretation factors. However, Huang (2013) reports gender differences specifically regarding mathematics and social-sciences self-efficacy, sub-scales that may relate to the Technical Aspects factor of RSE that showed non-invariance in this study.
Previous empirical approaches to RSE measurement yield heterogeneous results regarding factor structure. We hypothesized that these differences can partly be explained by sample differences that engender structural differentiation, and that have gone unregarded as of yet. We coined this hypothesis the differentiation hypothesis, and it attempted to reconcile heterogeneous findings on the RSE structure. Hence, a systematization in (in-)variance of the ASER three-factor structure was explored. Findings of strict MI between female and male students indicate that the ASER is generally fit for valid invariant measurement. Following, a lack of MI between certain groups can be attributed to real group differences and does probably not stem from weakness in measurement.
Measurement non-invariance was expected and tested between different academic disciplines and between different training levels through MGCFA. The overall moderate model fit can be explained by non-invariance between different sub-groups, which renders a very good model fit including the whole sample impossible. The results from the MI analyses are, thus, still valuable.
The results of MI analyses between psychology and educational science students were mixed. At least scalar MI was found for the Theoretical Aspects and the Interpretation factor, but not for the Technical Aspects factor. Thus, RSE appears to constitute slight differences in meaning across disciplines, which was especially apparent in research tasks like processes of data collection or knowledge of appropriate analysis methods.
Concluding, while RSE should be of importance in almost every academic program, the specific research and/or training cultures apparently differ between academic disciplines. The difference in mean scores on the Theoretical Aspects factor also underscores the importance of considering disciplines when researching RSE. It seems that differences do, indeed, occur both on structural and mean score levels, as well as regarding a variety of research tasks.
It would be interesting to investigate the reasons for these disciplinary differences in the perception of RSE. Do the main research practices differ across disciplines, and are they communicated differently to the students? Is the nature or the role of research perceived differently by students? Are research methods regarded and taught differently? In addition to psychology and educational science that were investigated in this study, taking into account further disciplines even beyond the social sciences might be insightful with respect to the investigation of disciplinary differentiation effects on RSE development.
Measurement invariance analyses between training levels, again, yielded mixed results. MI was scalar for the Technical Aspects and Interpretation factors, and metric for the Theoretical Aspects factor. Local structural equation modeling provided a more detailed insight due to the continuous moderation of model parameters: Significant variations in fit statistics, item loadings, and item residuals across semesters were revealed. These findings indicate a change in the meaning of the latent construct of “research self-efficacy” (Molenaar et al., 2010). Most importantly, differences in model fit may indicate that not even configural MI (relating to the basic construct structure) can be validly assumed across all training levels: not only the meaning of each latent factor but also the amount and segmentation of RSE factors might differentiate with time at university.
Concluding, RSE changes with the training level on two levels: First, change in mean values can be reasoned to stem from increasing mastery/failure experience (Bandura, 2006). Second, factor differentiation can be attributed to conceptual change in how research is regarded (Rochnia and Radisch, 2021).
For an understanding of RSE development covering the whole qualification period of junior researchers, future studies might extend the investigation of the differentiation hypothesis to the doctoral level. However, different from other countries, doctoral qualification in Germany is not standardized: while there are a few distinguished doctoral training programs, most PhD candidates complete their PhD research within the context of common employment as assistant researchers at universities without ongoing formal methods education. Thus, Bachelor's and Master's programs are more similar to each other regarding method training, since this is where coursework and institutionalized learning takes place. The ASER was developed to assess RSE in Bachelor and Master students as RSE measurement for this level was missing. RSE at the doctoral level can already be validly assessed by a questionnaire by Lachmann et al. (2018). This measure comprises research tasks that are relevant on the PhD level, but not yet for Bachelor and Master students (e.g., “I can build cooperations with central researchers in my field”). Delineating whether and how these two measures can be employed to capture RSE development over the whole qualification span would be an interesting endeavor for future RSE research.
Four independent MI analyses were conducted to investigate dichotomous interaction effects of training level and discipline on RSE structure. Results indicate that there is an interaction effect: Invariance seems to change with program level, especially so for psychology students. The data support the notion that psychology students at the master level perceive research differently than the other sub-groups. One reason might be differences in emphases on methods education between the disciplines. Presumably, methods education is more emphasized in psychology than in educational science. Even for programs such as school or clinical psychology, where most students plan a career in applied settings, American graduate education aims to also strengthen students' scientific competencies by explicitly implementing the so-called “scientist practitioner approach” (Jones and Mehr, 2007). Klieme et al. (2020) called for a similar focus in educational science programs through research-based learning.
Especially based on the fact that measurement variance increases with the program level, it can be reasoned that these differences are less due to primal interests that influence the choice of program, but due to socialization processes once a program is studied. Future research might analyze both differences in research practices (methodology) as well as the research training environment.
Our results indicate that differentiating effects on RSE are worth considering both in theory development and in higher education practice. However, implications drawn from them are limited since the current study is based on cross-sectional data. Deducing a process theory of intra-individual RSE development is, thus, beyond the scope of this study. Furthermore, evidence-based recommendations for higher education practice are to be considered with caution. Longitudinal RSE development might differ from the effects found in the cross-sectional data. In addition, predictors of RSE level and RSE differentiation are important factors to be considered in educational practice. These predictors potentially comprise various environmental factors as well as person factors. In this regard, longitudinal data as called for some time ago (Kahn, 2000) and again recently (Livinţi et al., 2021) will be necessary to specify which effects are actually salient in educational practice. Thus, this cross-sectional study is only the first step toward a systematic investigation of RSE development as well as toward evidence-based practices in methods education.
In addition, the results stem from a German sample. The cultural generalizability of our results needs to be delineated. Potential differences in RSE development and structure might be attributed to 2-fold cultural effects: differences between national culture in general, and differences in educational culture. Investigating relevant factors in these regards will be an interesting direction of international RSE research.
Furthermore, the three-factor structure of the ASER that fits the data of this study best needs to be confirmed in a German sample and internationally. Thus, the final structure, or even more specifically, the precise structural differentiation of RSE across relevant moderators, remains unresolved. This refers to the chicken-and-egg problem in empirical research on RSE structure: are we to begin with a specified theoretical structure? Or with no structure, which then can be freely developed empirically based on a content-valid item pool? How do we, then, judge findings on model fit and measurement non-invariance by means of traditional fit indices? Again, considering a lack of MI as a feature calls for elaborate methods that are fit for systematic investigations and judgments of said feature.
The present study emphasized measurement non-invariance in educational variables to be understood as a feature rather than a bug. The structural differentiation that we found in this study spotlights that RSE is complex and malleable. This Interpretation supports a desired feature of academic education: conceptual change in research within university training (Rochnia and Radisch, 2021) and, following, self-efficacy beliefs referring to research. Overall, we see four main areas where implications from our study become important.
The methodical implications of our endeavor to take a non-traditional perspective on measurement (non-)invariance are the basis for future research on any differentiation of latent constructs. If test developers seriously begin to consider non-invariance in measurement as a feature, strategies are needed to deal with naturally resulting poor model fit in the configural baseline model, and with other procedures that have been employed to judge latent construct measurement under a perspective of desired invariance. Common procedures and cut-off values employed in current MI analyses do the concept of MI as a feature injustice and need to be differentiated as well. In this regard, Rochnia and Radisch (2021) revive the AGB Typology by Golembiewski et al. (1976) that systematizes levels of change in measurement across time. By this means, Rochnia and Radisch (2021) refrain from considering a lack of invariance generally as a bug but emphasize that considering different types of change in measurement may hold relevant information in educational settings. Regarding concrete analysis methods for MI, LSEM (Hildebrandt et al., 2009), and moderated non-linear factor analysis (Bauer, 2017) allow the ability to test changes in the model parameter as effects of certain moderating variables, like semesters at university. However, while both approaches are well fit to investigate changes in meanings and emphases of latent constructs, they are not (yet) able to detect systemic changes in the factor structure between groups. Meaning, both approaches are fit to investigate parameter changes in the same model but are not fit to investigate changes in the model structure (e.g., from a two to a three-factor model). Furthermore, a developmental differentiation of RSE may constitute itself in a growing, more complex factor structure contingent on relevant moderating variables. This will render the test of invariant covariance matrices as suggested by Vandenberg and Lance (2000) relevant again—a step in MI analysis that has been omitted in most MI practice recently (Putnick and Bornstein, 2016). In addition, exploratory methods for structure analyses might be needed that can depict changes in the construct structure across a certain moderator.
The identified variance in construct structure and constitution may explain previously reported heterogeneous results. Factorial differences between different measures of RSE have been pointed out repeatedly (e.g., Forester et al., 2004; Livinţi et al., 2021). However, an explanation for these differences is lacking as of yet, but might be achieved by considering our differentiation hypothesis and examining the respective databases used for instrument development and factor analysis. The empirical structure of the Self-efficacy in Research Measure (Phillips and Russell, 1994) was based on the data from 219 doctoral students in counseling psychology. In contrast, the structure of the Research Self-Efficacy Scale (RSES, Bieschke et al., 1996) resulted from a factor analysis with the data from 177 doctoral students enrolled in various programs, namely biological sciences (32%), social sciences (28%), humanities (23%), and physical sciences (17%). Since our results suggest that students' academic discipline affects the RSE factor structure, the heterogeneous results from these two studies might be reconciled by considering disciplinary differentiation effects. This could be achieved by re-analyzing the factor structure of the RSES separated by discipline. A more feasible approach might be to systematically recognize and investigate the effects of the disciplines that participants are sampled from in future studies.
Furthermore, inconsistencies in the relations of RSE to other constructs have been pointed out regarding gender (Livinţi et al., 2021), research training environment, year in the program, interest in research, and research outcome expectations (Bieschke, 2006). Again, suchlike heterogeneous results may be reconciled by taking a closer look at sample characteristics that affect construct structure and meaning. Specifically, if the meaning of a latent construct, namely RSE, is inconsistent across samples, empirical relations to neighboring constructs may be inconsistent as well. In their recent meta-analysis, Livinţi et al. (2021) point out that student samples in RSE research are pretty heterogeneous, as participants are sampled from different training levels (undergraduate vs. graduate training) and from various disciplines such as counseling psychology, education, STEM education, and even law. Following, inconsistencies in construct relations may be explained under the differentiation hypothesis, since we found differentiation effects on the RSE structure for both of these dimensions, training level and discipline. The exemplary analysis of two studies on the relation between RSE and training environment does, indeed, reveal differences in sample characteristics. A non-significant relation reported by Phillips et al. (2004) is based on a sample of 84 individuals who had already accomplished their full doctoral degree (85%) or all requirements but the thesis. In contrast, a significant relation reported by Kahn (2001) is based on a sample of 149 doctoral students of which 50% were still in their first 2 years of training.
Concluding, in the context of theory development, the lack of MI in RSE measurement should caution us to compare findings on RSE structure, mean scores, or relations to other variables that stem from the data on students with different backgrounds. Similarly, practical implications drawn from empirical RSE research should be given very cautiously with regard to whether the targeted group that implications are drawn for is well-enough represented by the empirical sample. Therefore, future research should give more thought to considering potential sub-groups that have gone unregarded as of yet in order to reveal the differentiated picture of RSE that is needed. To do so, differentiated analyses are called for that are fit to model a lack of MI as a feature.
Considering MI between training levels will foster a process-oriented theory of RSE structure and development. The differentiation hypothesis that we propose in this article might be one unifying factor in RSE theory development. Concrete characteristics that affect RSE mean scores as well as structural differentiation can be deduced from previous research (e.g., Livinţi et al., 2021) and social cognitive career theory (Lent et al., 1994). They constitute person factors like interest and environmental factors like aspects of research training as antecedents to RSE development. Livinţi et al. (2021) identified these antecedents of RSE in the context of social cognitive career theory. The studies included in their meta-analysis, however, exhibit two weak spots: first, they stem from cross-sectional data. Second, they analyze RSE total scores only, without taking into account structural differentiation.
Our results demonstrate the importance of structural differentiation, not only based on main effects but also on complex interaction, for example between the discipline studied and time in the program. Future research should address such potential interaction effects of discipline and the amount of methods training on factor model parameters in more detail. In a way, this interaction describes the culture and aspiration under which research is taught and addressed within a university program. Are students introduced to research methods and research matters in their discipline? Are they given the opportunity for their own (mastery) experience? Do faculty model scientific behavior and attitudes, and do they, thereby, socialize students into a (discipline) specific way to conceptualize and approach research? The theory of the research training environment (Gelso, 1993) stems from an investigation of the science practitioner teaching in U.S. American psychology programs and incorporates those questions.
Indeed, previous researchers have identified these factors of the research training environment as a predictor of RSE in cross-sectional studies (e.g., Kahn and Schlosser, 2010). One study explored the longitudinal effect of the research training environment on changes in RSE after 1 year in graduate training: Kahn (2000) found effects for one training aspect, namely the student–mentor relation. In addition to Kahn, also Livinţi et al. (2021) conclude from their meta-analysis the need for further longitudinal studies. Analyzing students' research training environment might serve as an explanation for the interaction effect of discipline and training level on RSE differentiation. Longitudinal studies can in addition give insight into the intra-individual development of RSE, as well as delineate additional causal effects of possible predictors beyond the training environment (such as general self-efficacy, interest, or other person variables). Understanding causes, interactions, and onsets for this intra-individual change will help refine a theory of RSE development regarding both RSE scores and structural changes.
A fine-grained investigation of structural changes can help clarify how students think about research and how this affects their self-efficacy at different levels. This might help to enhance and customize methods education to the “zone of proximal development.” Again, longitudinal studies may investigate these intra-personal structural changes in RSE as an effect of a specific person and environmental factors.
Developing an understanding of RSE in academic education can serve several goals in higher education practice. On an individual scale, considering students' RSE can support our understanding of their individual needs regarding methods education. Specifically, insights from longitudinal studies will enable educators, faculty, and mentors to identify said “zones of proximal development” in regard to specific research tasks that students should be exposed to. This way, they can tailor methods education to students' needs, focusing on tasks in which students perceive low self-efficacy. In addition, students might use the differentiating facets of RSE for the self-assessment of their own development (Forester et al., 2004), especially in self-regulated learning settings. This can help them delineate what research tasks they should acquaint themselves with next. Hereby, focusing on self-efficacy development, beyond mere research knowledge, will draw both students' and educators' attention toward learning settings that deliberately incorporate its facilitation. Specifically, such settings should emphasize the main factors that influence self-efficacy beliefs. This is to facilitate mastery experiences through student activity and be aware of the communicated perspective on the value and epistemological nature of research. Our results show that the latent structure of RSE differs between students based on their disciplinary socialization. Thus, zones of proximal development are not only contingent on a student's training level, but also on other context factors that need to be considered in individual mentoring. Our results indicate that academic discipline is such a context factor, but systematic investigations of the longitudinal data on individual RSE development are needed to specify and confirm differentiating factors that operate on an individual level.
More generally, including RSE in higher education research may enhance our understanding of the psychological processes that underly successful academic development. Longitudinal studies should, thus, delineate specific factors in the training environment that prove promotive of RSE. Prospectively, these factors will enable faculty to foster students' RSE, following evidence-based reasoning. However, considering the differentiation hypothesis, RSE sensitive practices in methods training can never be simply carried over from one setting to another. Following that, university didactics need to develop approaches to fostering RSE that are customized to different disciplines and training levels. These customized approaches can only be delineated through longitudinal studies that systematically investigate possible differentiation effects that operate on the group level.
Once the role of RSE in higher educational processes is delineated, its assessment might be an asset to the evaluation of research training settings on a broader scale, such as settings that employ research-based learning in Europe (Wessels et al., 2021), or the scientist–practitioner approach in the U.S. (for a description see Jones and Mehr, 2007). Their focus on student activity (Huber, 2014) can be reasoned to particularly enable mastery experience. Therefore, research self-efficacy should be regarded as a core outcome of such student-active learning environments and should, thus, be considered in program evaluation.
Taken together, the findings reported here can add to the conclusion that further investigation of RSE differentiation is needed, worthwhile, and beneficial. Understanding causes and onsets for change in the RSE structure will help refine both, a theory of RSE development and university methods training.
The raw data supporting the conclusions of this article will be made available by the authors, without undue reservation.
Ethical review and approval was not required for the study on human participants in accordance with the local legislation and institutional requirements. The patients/participants provided their written informed consent to participate in this study.
KK: conceptualization (lead), investigation, methodology, data curation, formal analysis, writing—original draft preparation, and writing—review and editing (lead). FS-B: conceptualization (supporting), resources, and writing—review and editing (supporting). All authors contributed to the article and approved the submitted version.
The authors declare that the research was conducted in the absence of any commercial or financial relationships that could be construed as a potential conflict of interest.
All claims expressed in this article are solely those of the authors and do not necessarily represent those of their affiliated organizations, or those of the publisher, the editors and the reviewers. Any product that may be evaluated in this article, or claim that may be made by its manufacturer, is not guaranteed or endorsed by the publisher.
The Supplementary Material for this article can be found online at: https://www.frontiersin.org/articles/10.3389/feduc.2023.1092714/full#supplementary-material
1. ^See Supplementary material A for analyses of measurement invariance between U.S. American and German students.
2. ^The jangle fallacy describes “the use of two separate words or expressions covering in fact the same basic situation, but sounding different, as though they were in truth different” (Kelley, 1927, p. 64).
3. ^Here, only the main findings are reported to enhance readability. All details on fit statistics and difference tests for the nested MGCFA models are displayed in the Supplementary material B.
Bandura, A. (2006). “Guide for constructing self-efficacy scales,” in Self-efficacy Beliefs of Adolescents, eds F. Pajares and T. Urdan (Greenwich, CT: Information Age Publishing), 307–337.
Bauer, D. J. (2017). A more general model for testing measurement invariance and differential item functioning. Psychol. Methods 22, 507–526. doi: 10.1037/met0000077
Beierlein, C., Kovaleva, A., Kemper, C., and Rammstedt, B. (2012). Ein Messinstrument zur Erfassung subjektiver Kompetenzerwartungen Allgemeine Selbstwirksamkeit Kurzskala (ASKU). GESIS-Working Papers 17. (Köln: GESIS - Leibniz-Institut für Sozialwissenschaften), 1–24.
Bieschke, K. J. (2006). Research self-efficacy beliefs and research outcome expectations: Implications for developing scientifically minded psychologists. J. Career Assess. 14, 77–91. doi: 10.1177/1069072705281366
Bieschke, K. J., Bishop, R. M., and Garcia, V. L. (1996). The utility of the research self-efficacy scale. J. Career Assess. 4, 59–75. doi: 10.1177/106907279600400104
Chen, F. (2007). Sensitivity of goodness of fit indexes to lack of measurement invariance. Struct. Eq. Model. 14, 464–504. doi: 10.1080/10705510701301834
Chen, F., Curran, P. J., Bollen, K. A., Kirby, J., and Paxton, P. (2008). An empirical evaluation of the use of fixed cutoff points in RMSEA test statistic in structural equation models. Sociol. Methods Res. 36, 462–494. doi: 10.1177/0049124108314720
Choi, M. (2005). Self-efficacy and self-concept as predictors of college students' academic performance. Psychol. Schools 42, 197–205. doi: 10.1002/pits.20048
Clark, L. A., and Watson, D. (2019). Constructing validity: New developments in creating objective measuring instruments. Psychol. Assess. 31, 1412–1427. doi: 10.1037/pas0000626
De Feyter, T., Caers, R., Vigna, C., and Berings, D. (2012). Unraveling the impact of the Big Five personality traits on academic performance: The moderating and mediating effects of self-efficacy and academic motivation. Learn. Individ. Diff. 22, 439–448. doi: 10.1016/j.lindif.2012.03.013
Dickhäuser, O., Schöne, C., Spinath, B., and Stiensmeier-Pelster, J. (2002). Die Skalen zum akademischen Selbstkonzept Konstruktion und Überprüfung eines neuen Instrumentes. [The Academic Self Concept Scales: Construction and Evaluation of a New Instrument]. Zeitschrift für Differentielle und Diagnostische Psychologie 23, 393–405. doi: 10.1024//0170-1789.23.4.393
Finney, S. J., and Schraw, G. (2003). Self-efficacy beliefs in college statistics courses. Contemp. Educ. Psychol. 28, 161–186. doi: 10.1016/S0361-476X(02)00015-2
Fischer, R., and Karl, J. A. (2019). A primer to (cross-cultural) multi-group invariance testing possibilities in R. Front. Psychol. 10, 1507. doi: 10.3389/fpsyg.2019.01507
Forester, M., Kahn, J., and McInnis, M. (2004). Factor structures of three measures of research self-efficacy. J. Career Asess. 12, 3–16. doi: 10.1177/1069072703257719
Gelso, C. J. (1993). On the making of a scientist-practitioner: A theory of research training in professional psychology. Prof. Psychol. 24, 468–476. doi: 10.1037/0735-7028.24.4.468
Gess, C., Geiger, C., and Ziegler, M. (2018). Social-scientific research competency: Validation of test score interpretations for evaluative purposes in higher education. Eur. J. Psychol. Assess. 2018, a000451. doi: 10.1027/1015-5759/a000451
Golembiewski, R. T., Billingsley, K., and Yeager, S. (1976). Measuring change and persistence in humanaffairs: Types of change generated by OD designs. J. Appl. Behav. Sci. 12, 133–157. doi: 10.1177/002188637601200201
Hildebrandt, A., Lüdtke, O., Robitzsch, A., Sommer, C., and Wilhelm, O. (2016). Exploring factor model parameters across continuous variables with local structural equation models. Multivar Behav. Res. 51, 257–258. doi: 10.1080/00273171.2016.1142856
Hildebrandt, A., Wilhelm, O., and Robitzsch, A. (2009). Complementary and competing factor analytic approaches for the investigation of measurement invariance. Rev. Psychol. 16, 87–102.
Holden, G., Barker, K., Meenaghan, T., and Rosenberg, G. (1999). Research self-efficacy. J. Soc. Work Educ. 35, 463–476. doi: 10.1080/10437797.1999.10778982
Huang, C. (2013). Gender differences in academic self-efficacy: A meta-analysis. Eur. J. Psychol. Educ. 28, 1–35. doi: 10.1007/s10212-011-0097-y
Huber, L. (2014). Forschungsbasiertes, Forschungsorientiertes, Forschendes Lernen: Alles dasselbe? Ein Plädoyer für eine Verständigung über Begriffe und Unterscheidungen im Feld forschungsnahen Lehrens und Lernens. Das Hochschulwesen 62, 32–39.
Jones, J., and Mehr, S. (2007). Foundations and assumptions of the scientist-practitioner model. Am. Behav. Scientist 50, 766–777. doi: 10.1177/0002764206296454
Jöreskog, K. G. (1971). Simultaneous factor analysis in several populations. Psychometrika 36, 409–426. doi: 10.1007/BF02291366
Kahn, J. H. (2000). “Research training environment changes: Impacts on research self- efficacy and interest,” in Research Training in Counseling Psychology: New Advances and Directions. Symposium conducted at the Annual Convention of the American Psychological Association. Washington, DC.
Kahn, J. H. (2001). Predicting the scholarly activity of counseling psychology students: A refinement and Extension. J. Counsel. Psychol. 48, 344–354. doi: 10.1037/0022-0167.48.3.344
Kahn, J. H., and Schlosser, L. Z. (2010). The graduate research training environment in professional psychology: A multilevel investigation. Train. Educ. Prof. Psychol. 4, 183–193. doi: 10.1037/a0018968
Kahn, J. H., and Scott, N. A. (1997). Predictors of research productivity and science-related career goals among counseling psychology doctoral students. Counsel. Psychologist 25, 38–67. doi: 10.1177/0011000097251005
Kelley, T. H. (1927). Interpretation of Educational Measurements. Yonkers-on-Hudson, NY: World Book Company.
Klieme, K. E. (2021). “Psychological Factors in Academic Education – Development of the Self-Efficacy in Research Questionnaire,” in Hochschullehre im Spannungsfeld zwischen individueller und institutioneller Verantwortung. Tagungsband der 15. Jahrestagung der Gesellschaft für Hochschulforschung, eds C. Bohndick, M. Bülow-Schramm, D. Paul, and G. Reinmann (Wiesbaden: Springer VS), 309–322. doi: 10.1007/978-3-658-32272-4_23
Klieme, K. E., Lehmann, T., and Schmidt-Borcherding, F. (2020). “Fostering professionalism and scientificity through integration of disciplinary and research knowledge,” in International Perspectives on Knowledge Integration: Theory, Research, and Good Practice in Pre-service Teacher and Higher Education, ed T. Lehman (Leiden, Boston, MA: Brill; Sense Publishers), 79–107. doi: 10.1163/9789004429499_005
Lachmann, D., Epstein, N., and Eberle, J. (2018). FoSWE – Eine Kurzskala zur Erfassung forschungsbezogener Selbstwirksamkeitserwartung. Zeitschrift für Pädagogische Psychologie 32, 89–100. doi: 10.1024/1010-0652/a000217
Lent, R. W., Brown, S. D., and Hackett, G. (1994). Toward a unifying social cognitive theory of career and academic interest, choice, and performance. J. Voc. Behav. 45, 79–122. doi: 10.1006/jvbe.1994.1027
Livinţi, R., Gunnesch-Luca, G., and Iliescu, D. (2021). Research self-efficacy: A meta-analysis. Educ. Psychologist 56, 215–242. doi: 10.1080/00461520.2021.1886103
Marsh, H., Trautwein, U., Lüdtke, O., Köller, O., and Baumert, J. (2006). Integration of multidimensional self-concept and core personality constructs: Construct validation and relations to well-being and achievement. J. Personal. 74, 403–456. doi: 10.1111/j.1467-6494.2005.00380.x
Mason, L., Boscolo, P., Tornatora, M. C., and Ronconi, L. (2013). Besides knowledge: a cross-sectional study on the relations between epistemic beliefs, achievement goals, self-beliefs, and achievement in science. Instruct. Sci. 41, 49–79. doi: 10.1007/s11251-012-9210-0
Meredith, W. (1993). Measurement invariance, factor analysis and factorial invariance. Psychometrika 58, 525–543. doi: 10.1007/BF02294825
Mieg, H. A., Ambos, E., Brew, A., Lehmann, J., and Galli, D. (2022). The Cambridge Handbook of Undergraduate Research. Cambridge: Cambridge University Press. doi: 10.1017/9781108869508
Molenaar, D., Dolan, C. V., Wicherts, J. M., and van der Maas, H. L. J. (2010). Modeling differentiation of cognitive abilities within the higher-order factor model using moderated factor analysis. Intelligence 38, 611–624. doi: 10.1016/j.intell.2010.09.002
Oberski, D. (2014). lavaan.survey: An R package for complex survey analysis of structural equation models. J. Statist. Softw. 57, 1–27. doi: 10.18637/jss.v057.i01
O'Brien, K. M., Malone, M. E., Schmidt, C. K., and Lucas, M. S. (1998). “Research selfefficacy: Improvements in instrumentation,” in Poster Session Presented at the Annual Conference of the American Psychological Association. San Francisco, CA.
Papanastasiou, E. (2014). Revised-attitudes toward research scale (R-ATR). A first look at its psychometric properties. J. Res. Educ. 24, 146–159. doi: 10.1037/t35506-000
Pfeiffer, H., Preckel, F., and Ellwart, T. (2018). Selbstwirksamkeitserwartung von Studierenden. Facettentheoretische Validierung eines Messmodells am Beispiel der Psychologie. Diagnostica 64, 133–144. doi: 10.1026/0012-1924/a000199
Phillips, J. C., and Russell, R. K. (1994). Research self-efficacy, the research training environment, and research productivity among graduate students in counseling psychology. Counsel. Psychologist 22, 628–641. doi: 10.1177/0011000094224008
Phillips, J. C., Szymanski, D. M., Ozegovic, J. J., and Briggs-Phillips, M. (2004). Preliminary examination and measurement of the internship research training environment. J. Counsel. Psychol. 51, 240–248. doi: 10.1037/0022-0167.51.2.240
Putnick, D. L., and Bornstein, M. H. (2016). Measurement invariance conventions and reporting: The state of the art and future directions for psychological research. Develop. Rev. 41, 71–90. doi: 10.1016/j.dr.2016.06.004
R Core Team. (2022). R: A Language and Environment for Statistical Computing. Vienna: R Foundation for Statistical Computing. Available online at: https://www.R-project.org/ (accessed December 2, 2022).
Rammstedt, B., and John, O. (2005). Kurzversion des Big Five Inventory (BFI-K): Entwicklung und Validierung eines ökonomischen Inventars zur Erfassung der fünf Faktoren der Persönlichkeit. Diagnostica 51, 195–206. doi: 10.1026/0012-1924.51.4.195
Robitzsch, A. (2015). sirt: Supplementary Item Response Theory Models. R Package Version 1.8-9. Available online at: http://cran.r-project.org/web/packages/sirt/ (accessed June 3, 2022).
Rochnia, M., and Radisch, F. (2021). Die unveränderliche Veränderbarkeit und der unterschiedliche Unterschied – Varianz nachweisen mit Invarianz. Bildungsforschung 2021, 2. doi: 10.25539/bildungsforschun.v0i2.410
Rosseel, Y. (2012). lavaan: An R package for structural equation modeling. J. Statist. Softw. 48, 1–36. doi: 10.18637/jss.v048.i02
Royalty, G. M., and Reising, G. N. (1986). The research training of counseling psychologists: What the professionals say. Couns. Psychol. 14, 49–60. doi: 10.1177/0011000086141005
Stajkovic, A. D., Bandura, A., Locke, E. A., Lee, D., and Sergent, K. (2018). Test of three conceptual models of influence of the big five personality traits and self-efficacy on academic performance: A meta-analytic path-analysis. Personal. Individ. Diff. 120, 238–245. doi: 10.1016/j.paid.2017.08.014
Vandenberg, R. J., and Lance, C. E. (2000). A review and systhesis of the measurement invariance literature: Suggestions, practices, and recommendations for organizational research. Org. Res. Methods 2, 4–69. doi: 10.1177/109442810031002
Wessels, I., Rueß, J., Gess, C., Deicke, W., and Ziegler, M. (2021). Is research-based learning effective? Evidence from a pre–post analysis in the social sciences. Stud. High. Educ. 46, 2595–2609. doi: 10.1080/03075079.2020.1739014
Keywords: research self-efficacy, assessment, validity, measurement invariance, differentiation, MGCFA, local structural equation modeling, research training
Citation: Klieme KE and Schmidt-Borcherding F (2023) Lacking measurement invariance in research self-efficacy: Bug or feature? Front. Educ. 8:1092714. doi: 10.3389/feduc.2023.1092714
Received: 08 November 2022; Accepted: 12 January 2023;
Published: 06 February 2023.
Edited by:
Lan Yang, The Education University of Hong Kong, Hong Kong SAR, ChinaReviewed by:
Gregory Siy Ching, Fu Jen Catholic University, TaiwanCopyright © 2023 Klieme and Schmidt-Borcherding. This is an open-access article distributed under the terms of the Creative Commons Attribution License (CC BY). The use, distribution or reproduction in other forums is permitted, provided the original author(s) and the copyright owner(s) are credited and that the original publication in this journal is cited, in accordance with accepted academic practice. No use, distribution or reproduction is permitted which does not comply with these terms.
*Correspondence: Katrin Ellen Klieme, a2xpZW1lQHVuaS1icmVtZW4uZGU=
Disclaimer: All claims expressed in this article are solely those of the authors and do not necessarily represent those of their affiliated organizations, or those of the publisher, the editors and the reviewers. Any product that may be evaluated in this article or claim that may be made by its manufacturer is not guaranteed or endorsed by the publisher.
Research integrity at Frontiers
Learn more about the work of our research integrity team to safeguard the quality of each article we publish.