- 1Department of Psychology, University of Virginia, Charlottesville, VA, United States
- 2UVA Library Research Data Services, University of Virginia, Charlottesville, VA, United States
In this study, we asked whether Montessori schools, which tend to have high student engagement, are associated with lower average rates of chronic absenteeism and/or smaller racial disparities therein relative to non-Montessori schools. Using data from the Civil Rights Data Collection, we identified a sample of Title 1 Montessori and non-Montessori schools with propensity score matching, and we used multilevel modeling to compare racial disparities in chronic absenteeism rates across school types. There was no significant difference in the average overall rates of chronic absenteeism across school types; nor were there sizable or significant differences in average racial disparities in the rates of chronic absenteeism between Black and White or Hispanic and White students, though Montessori schools had slightly lower average rates for White students. We discuss how shortcomings in the way chronic absenteeism data are collected limit intervention work by preventing researchers from answering questions about why students are chronically absent.
Introduction
Nationwide, 10–15% of students in K–12 education are chronically absent, meaning that they have missed at least 10% of the school year (Balfanz and Byrnes, 2012). There are a number of reasons why a student might miss school, including excused absences, like illness or family emergency, and unexcused absences, like skipping school to avoid a bully. In any case, chronic absenteeism is problematic because it is associated with lower academic performance for both the chronically absent student and their classmates, whose learning could be delayed when teachers must spend time catching up one student on material they missed (Gottfried, 2019). Chronic absenteeism may also perpetuate racial disparities in academic achievement; for example, one report found that being chronically absent was especially detrimental to Hispanic1 students’ reading achievement (Chang and Romero, 2008).
In this paper, we asked whether a specific alternative system of education, Montessori, is associated with (a) lower average overall rates of chronic absenteeism and/or (b) reduced racial disparities in chronic absenteeism. To date, only one other study has investigated attendance in Montessori schools specifically, and it reported that Montessori students tend to have higher attendance rates than their non-Montessori counterparts (Culclasure et al., 2018). This article is the first to compare chronic absenteeism rates between school types and to consider racial disparities therein. In answering our research questions, we used the same approach as in LeBoeuf et al. (in press) to succinctly analyze racial disparities in count outcomes using multilevel modeling with school-level data. Finally, we discuss the limitations of publicly available data for answering important questions related to chronic absenteeism—namely, why students are chronically absent.
Chronic absenteeism
Students with adverse childhoods, students with poor health or disabilities, and economically disadvantaged students are all more likely to be chronically absent (Balfanz and Byrnes, 2012; Gottfried and Gee, 2017; Stempel et al., 2017; Gee, 2018). Because each of these factors disproportionately impact students of color (relative to White students), rates of chronic absenteeism tend to be higher among Black students than White students (Gee, 2018). Racially disparate disciplinary practices may also help explain racial disparities in chronic absenteeism; students of color are suspended at disproportionately high rates (relative to White students), and these missed days are often recorded as unexcused absence (Davis et al., 2019). Nationwide, Black and Hispanic students are, respectively, 1.40 times and 1.17 times more likely than White students to be chronically absent (US Department of Education, 2019). Rates of chronic absenteeism tend to be highest in urban, low-income schools, which serve predominantly Black and Hispanic students (Balfanz and Byrnes, 2012). The effects of poverty, including worse health outcomes, lack of access to reliable transportation, etc., likely explain a large portion of why chronic absenteeism rates tend to be highest in low-income schools (Gee, 2018), which motivates research on this particular population of students.
Many of the risk factors associated with chronic absenteeism (poverty, illness, etc.) are well beyond what a school or district can control, which complicates intervention efforts. However, some districts have reported success in promoting attendance through incentive programs, like a school dance for students whose attendance is above a specified threshold (Epstein and Sheldon, 2002; Balfanz and Byrnes, 2012). Additionally, students with more positive attitudes toward school and with more involved parents tend to have higher attendance rates (Gottfried and Gee, 2017). Effective communication from schools to parents has also been linked to higher daily attendance rates and lower rates of chronic absenteeism (Epstein and Sheldon, 2002). Given these relations, a logical question is whether specific alternative education systems or pedagogies are especially adept at increasing student and family engagement, and thereby at encouraging school attendance. Moreover, knowing which students are most likely to miss school and why they do could reveal more avenues for targeted intervention. Effective interventions for lowering rates of chronic absenteeism must focus on both the overall rates and the racial disparities therein (Gee, 2018). As such, research evaluating an intervention targeting chronic absenteeism must consider whether it is appropriately lowering rates for all student demographic groups.
Montessori
Montessori schools are the most common alternative system of education worldwide (Lillard, 2019). They follow a model—including pedagogy, classroom materials, lessons, and teacher training—originally developed by physician/educator Maria Montessori in Italy early in the 20th century (Montessori, 1912). Montessori classrooms are known for having high levels of student self-determination and individualized instruction (Lillard, 2019). Montessori students exercise free-choice over what they work on and whether they work individually or with peers for 2.5–3-h work periods. Classrooms are mixed-age, with students spanning three-year age ranges, and students have the same teacher for three consecutive years. Every aspect of the classroom, including the schedule, the materials used, and lesson delivery, were thoughtfully designed by Maria Montessori with the goal of maximizing students’ interest, concentration, and intrinsic motivation (Montessori, 1912).
Attendance in Montessori schools
Montessori students, relative to conventional-school students, report feeling a stronger sense of community at school (Rathunde and Csikszentmihalyi, 2005; Lillard and Else-Quest, 2006) and enjoying schoolwork more (Lillard et al., 2017). Adults who attended Montessori schools also report remember liking school more than adults who attended non-Montessori schools (Snyder et al., 2022). Based on these findings, one might expect Montessori students to be more motivated to attend school and thus be less likely to be chronically absent, given that higher student engagement is associated with lower chronic absenteeism (Gottfried and Gee, 2017). Montessori schools are also associated with lower average suspension rates (LeBoeuf et al., in press; Culclasure et al., 2018). Suspensions are typically recorded as unexcused absences, so for students who are suspended multiple days in a given school year, days missed for suspension might push them over the threshold into being considered chronically absent (Davis et al., 2019). It’s possible that the lower suspension rates in Montessori schools also lead to fewer chronically absent students.
Parent engagement and communication is also related to chronic absenteeism (Epstein and Sheldon, 2002; Gottfried and Gee, 2017). Montessori wrote explicitly about the importance of parental involvement, and she placed high emphasis on this in her original schools (Montessori, 1912). To whatever extent current Montessori schools are still prioritizing parental involvement, we might expect to see lower rates of chronic absenteeism as a result. Research on parental involvement of Montessori students is limited, but Black and Hispanic parents of Montessori students report satisfaction with their children’s schools (Golann et al., 2019). Parents in this study also reported attending multiple parent-education sessions at their children’s school to learn about Montessori pedagogy. These types of session are common in Montessori schools because the Montessori system is likely different from what many parents experienced in school unless they attended a Montessori school themselves. These sessions may also function to improve rapport and communication between parents and schools. However, some Montessori parents also reported wanting more regular assurance that their children were reaching academic milestones and feeling uncertain of the school’s academic rigor (Golann et al., 2019), which may be a sign of a lack of communication. If parents are invested in the Montessori method and have consistent communication with the school, they might go to greater lengths to ensure that their children attend school, but if they question the academic program, they might be more permissive of their children missing school.
We know of only one study on attendance in Montessori schools (Culclasure et al., 2018). This study compared attendance rates of all public Montessori students in South Carolina to a sample of demographically matched non-Montessori students. Montessori students in the sample had significantly higher attendance rates than the non-Montessori students, after controlling for family income, race, gender, ESL status, special education status, and grade. The authors included race as a covariate in their OLS model, but they were not investigating potential racial disparities in attendance, so they did not report any results related to racial identity.
Montessori self-selection
Of the nearly 500 public Montessori schools in the United States, the vast majority are school-choice programs (Debs, 2016), meaning that they are either a charter or magnet school. The majority of Montessori parents, then, have either sought out a Montessori school specifically or selected a nearby Montessori school over their neighborhood’s public school. This decision might be driven by any number of factors (e.g., convenience, standardized test scores, word of mouth, etc.), but it might reflect characteristics of Montessori parents that differ, on average, from parents who do not opt for a Montessori school, and those differences could relate to school attendance. To date, only a couple of studies have attempted to measure differences between Montessori and non-Montessori parents with American samples (Fleege et al., 1967; Dreyer and Rigler, 1969), and neither reported observing differences. Both studies included parent questionnaires about general parenting practices, and both reported finding no differences between the two groups of parents. For instance, one study reports that “[n]o differences were found between the parents in these schools on such measures of social and parental attitudes and behavior as: achievement orientation, traditional family ideology, dogmatism, anomie, parent control behavior, or task oriented vs. person oriented values” (Dreyer and Rigler, 1969, p. 411). These two roughly 50-year-old studies are not sufficient evidence that there are no unobserved variables related to self-selection into a Montessori school that could confound results when comparing Montessori and non-Montessori students. Self-selection in Montessori schools, therefore, must be considered when comparing the two samples. Here, we use propensity score matching with school-level data to identify a sample of Montessori and non-Montessori schools that are statistically similar on a number of school characteristics related to chronic absenteeism (including school choice status) to compare school-level rates of chronic absenteeism. Propensity score matching is a useful option for reducing the risk of selection bias when other quasi-experimental methods and random assignment are not applicable (Fan and Nowell, 2011).
Method
Sampling and propensity score matching
We followed the same sampling and propensity score matching process as in LeBoeuf et al. (in press), using data from Civil Rights Data Collection’s (CRDC) 2017 survey. The CRDC considers a student chronically absent if they have missed 15 or more school days. The CRDC collects data biennially through a survey of every public school in the country—data is reported at the school-level, and completion of the survey is required by the Office of Civil Rights.
We were primarily interested in chronic absenteeism among students from low-income families, as they are more likely to be chronically absent than students from more affluent families (Gottfried and Gee, 2017). Public Montessori schools tend to enroll more economically advantaged students relative to their surrounding districts (Debs, 2016), so socioeconomic status (SES) presents a potential confound in this study. To limit the possibility for SES differences to bias results, we limited our sample to only include schools classified as receiving Title 1 funding, thereby increasing the likelihood that the student SES composition is similar across the two school types. The CRDC also only provides school-level data, which meant we could not identify specific students from low-income families, but focusing on Title 1 schools also made it easier to identify schools that had higher proportions of students from low-income families. Additionally, because we were interested in comparing rates across racial groups, we only included schools that had 5% or more White students and 5% or more Black students and/or 5% or more Hispanic students in order to reduce the likelihood that we would be comparing chronic absenteeism rates representing small numbers of students (these are the same diversity criteria as used in LeBoeuf et al., in press). We searched the CRDC database for schools with names containing the string “Montessori” that were classified as receiving Title 1 funding. This initial search yielded 151 schools. From the initial 151 schools, we made the following removals: 20 were removed because they did not meet our cutoffs for racial diversity; two were removed because their names on their school websites differed from their names in the CRDC datafile; 10 were removed because they were missing chronic absenteeism data; and three were removed because they were missing free or reduced-price lunch (FRPL) data (which we needed for our propensity score matching process). All of which left us with 116 Montessori schools in our sample.
We then identified non-Montessori comparison schools with propensity score matching. We assumed that students who live in the same area are likely to have access to the same public transportation system (or lack thereof), which could relate to school attendance. So our pool of potential matches included all schools in the CRDC’s database that were in the same ZIP Codes as the Montessori schools. We initially identified a pool of 1,268 non-Montessori schools. From this pool, 620 schools were removed because they either were not Title 1 schools or were below the racial-diversity threshold described previously; 17 schools were removed because they were missing free and reduced-price lunch data; three schools were removed because they were missing chronic absenteeism data; two schools were removed because they had unique programs or student populations (one school was entirely virtual and the other specifically served deaf and blind students). The final pool of potential matches included 626 schools.
As in LeBoeuf et al. (in press), we then estimated propensity scores (following Stuart, 2010) predicting status as a Montessori or non-Montessori school. The propensity score matching process was iterative, and we used a wide range of covariates: In lieu of extensive previous research indicating specific covariates associated with self-selection into Montessori schools, we followed the “kitchen sink” approach described in Steiner et al. (2015). We estimated propensity scores using the MatchIt R package (v4.2.0; Ho et al., 2011) multiple times using different combinations of the following variables (the same variables as used in LeBoeuf et al., in press): binary predictors indicating whether each school offered each grade from preschool to twelfth (since rates of chronic absenteeism vary by grade level (US Department of Education, 2019); the number of students in each school; the proportion of Black, White, Hispanic, and FRPL-qualifying students; the proportion of students with disabilities included in the Individuals with Disabilities Education Act (IDEA) and the proportion of students with disabilities protected by Section 504 of the Rehabilitation Act (504); and binary variables indicating magnet and charter school classification. We used nearest-neighbor matching without replacement, resulting in an equal number of Montessori and non-Montessori schools on each iteration. Within each iteration’s matched sample, we evaluated the standardized mean difference between the Montessori and matched non-Montessori schools on each of the variables listed above. Given that we (a) matched schools on the proportion of students who are Black, White, Hispanic, and FRPL-qualifying and (b) pulled non-Montessori matches from a pool of exclusively Title 1 schools from the same ZIP Codes as the Montessori schools, we argue that socioeconomic status is unlikely to be a meaningful source of bias in the results because average school SES is strongly associated with the surrounding neighborhood and the school’s racial demographics (Orfield and Frankenberg, 2014). Our final non-Montessori sample had no significant standardized mean difference from our Montessori sample on any of the variables listed above except for the proportion of schools that offered sixth grade).
We retrieved chronic absenteeism data for each school in the final sample based on the most recent available CRDC data (survey year: 2017). Our final sample included 232 schools (116 Montessori), representing 94,584 students. Table 1 shows the descriptive statistics of the sample prior to and after the matching process.
Analysis plan
Chronic absenteeism data from the CRDC include the raw count of students who were chronically absent, disaggregated by race, disability status, and gender. The CRDC defines chronic absenteeism as missing 15 or more school days. On average, ~90% of students in each school in our sample were Black, Hispanic, or White, and other racial groups were small, so we focused our analysis on chronic absenteeism of Black, White, and Hispanic students so as to avoid potentially noisy estimates for other racial groups. We summed the number of chronically absent Black, Hispanic, and White students to calculate the total number of chronically absent students in a school. Our resulting variable for total number of chronically absent students does not strictly refer to the absolute total number of chronically absent students at each school, because some schools had chronically absent students from other racial groups (albeit very few). Ten schools (seven Montessori; three non-Montessori) had no Black students, and two schools (one Montessori; one non-Montessori) had no Hispanic students. For these schools, the total number of chronically absent students was calculated based on data for White and Hispanic or White and Black students alone. The CRDC also reports the total number of students in a school and the percentage of students who fall into each racial category. We used these values to compute the total number of students in each racial group at each school.
We were interested in answering the following research questions: (1) whether overall rates of chronic absenteeism differ between school types and (2) whether racial disparities in chronic absenteeism rates differ between school types. To examine whether overall rates of chronic absenteeism differed, we ran the following negative binomial model [as is appropriate with count outcomes (Hilbe, 2014)] predicting counts of chronically absent students and using the log number of students within a school as an offset to account for differences in school size:
In this model, Montessori is a binary variable indicating whether a school is a Montessori school. Montessori refers to the average difference in log counts of chronically absent students between the Montessori and non-Montessori schools, and this value can be exponentiated to be interpreted as the multiplicative difference in the rates (i.e., a Montessori coefficient of −0.69, exponentiated to 0.50, would suggest the Montessori schools, on average, have 50% fewer chronically absent students as the non-Montessori schools).
To examine whether racial disparities in chronic absenteeism rates between racial groups varied across Montessori and non-Montessori schools, we used a multilevel negative binomial model as in LeBoeuf et al. (in press). We treated the number of chronically absent students in each racial group within a school as multiple nested measurements. We then constructed a multilevel model predicting the number of chronically absent students from dummy variables for Black and Hispanic (with White treated as the reference category), Montessori classification (with non-Montessori treated as the reference category), and the two-way interaction between Montessori classification and each race variable, fitting a random intercept for each school to account for dependencies in schools’ multiple measurements. We included the log count of students in each racial group within a school as an offset to account for differences in the sizes of each group:
Although the matched sets of Montessori and non-Montessori schools did not significantly differ on a number of variables (see Table 1), propensity score matching cannot perfectly or completely account for differences on those or additional unmeasured variables between Montessori and non-Montessori schools. We therefore ran Models 1 and 2 as written above, as well doubly robust versions of the models that included controls for charter and magnet classification, school size, percentages of Black, White, and Hispanic students, and school-level percentages of students with disabilities and FRPL-qualifying students. We found that some estimates varied between the non-doubly robust and the doubly robust models; to try and account for possible confounds as much as possible, we report the doubly robust results. In estimating both doubly robust models, we also bootstrapped the sample 5,000 times and estimated bias-corrected 95% confidence intervals around coefficients (and around predicted values for the multilevel model) by re-estimating the model on each resample. We bootstrapped schools to retain the clustered structure of the data. Not every school had three observations associated with it, so the sample sizes of bootstrap replicates varied slightly from resample to resample; the range of Ns (at the within-school racial-group measurement level) was from 665 to 693, with a median of 683 and an interquartile range of 4 [681, 685]. Models were estimated using the glmmTMB package (v1.1.4; Brooks et al., 2017) in R. Below, we report the observed coefficients from our model as well as the bias-corrected 95% confidence intervals around coefficients.
Results
Table 2 shows the average percent of chronically absent students in each group across school types. Full results from Model 1 are shown in Table 3. Exponentiating the Montessori coefficient from the doubly robust model indicated that the chronic absenteeism rate at Montessori schools was, on average, 0.84 times the rate at non-Montessori schools, magnet and charter school classification as well as the percents of Black, White, Hispanic, IDEA, and FRPL-qualifying students. However, the confidence interval for the Montessori coefficient includes zero, indicating this is not statistically significant difference. Charter schools were associated with higher rates of chronically absent students.
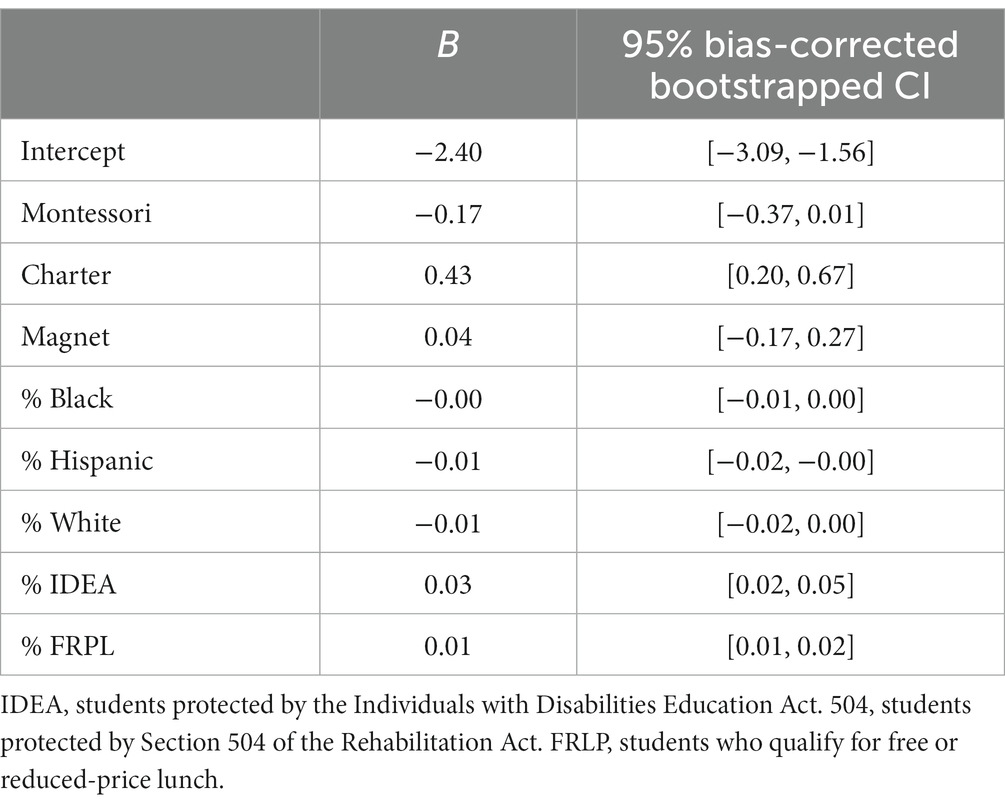
Table 3. Overall rates of chronic absenteeism across school types and bootstrapped (5000×) 95% bias-corrected confidence intervals.
Using a multilevel model (Model 2) in which we clustered counts of chronically absent Black, White, and Hispanic students within schools (i.e., a school with complete data had three associated measurements), we examined how disparities in chronic absenteeism rates between racial groups differed between Montessori and non-Montessori schools. Full results for the multilevel models are shown in Table 4. In Table 4, the coefficient for Montessori reflects the average difference in log counts of chronically absent White students (the reference group) across school types; exponentiating the coefficient of −0.20 indicated that, on average, the White Montessori student chronic absenteeism rate was 0.82 times that of White non-Montessori students. The main effects for Black and Hispanic reflect the estimated average differences in the log counts of chronically absent students from each of those groups and the log counts of chronically absent White students at non-Montessori schools. Exponentiating the coefficients for these terms indicated that 1.25 times and 1.31 times as many Black and Hispanic students were chronically absent in non-Montessori schools relative to White students. The interactions reflect differences in those disparities at Montessori schools. The differences between Black and White students and Hispanic and White students were not significantly different at Montessori vs. non-Montessori schools, meaning that the racial disparities in chronic absenteeism rates were not significantly different between the two school types. As shown in Figure 1, average chronic absenteeism rates for Black and Hispanic students were nearly identical across the two school types, though slightly lower for White students in Montessori schools.
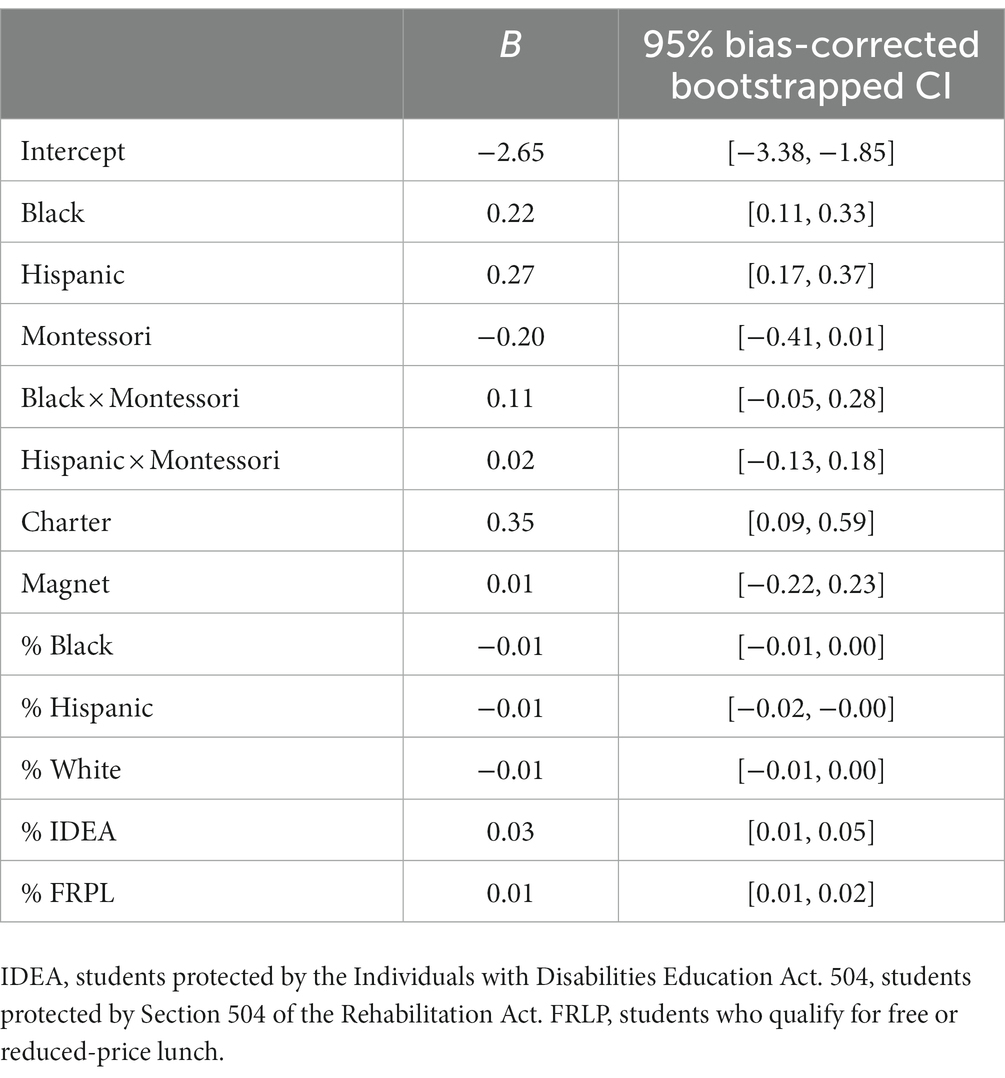
Table 4. Chronic absenteeism disparities between racial groups across Montessori and non-Montessori schools and bootstrapped (5000×) 95% bias-corrected confidence intervals for multilevel model.
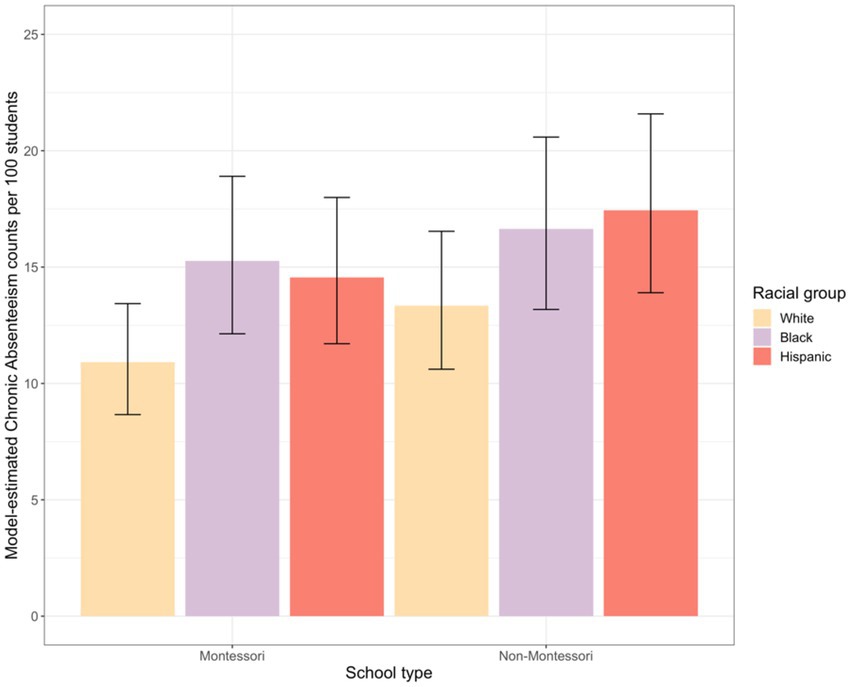
Figure 1. Differences in chronic absenteeism between racial groups across Montessori and non-Montessori schools. Errors bars represent bias-corrected 95% bootstrap confidence intervals. Predicted values were generated by setting the charter variable equal to 1, the magnet variable equal to 0, and continuous covariates equal to their means (in the non-bootstrapped data): 26.44 for percent Black students, 22.51 for percent Hispanic students, 40.22 for percent White students, 12.04 for percent IDEA, and 51.84 for percent FRPL.
Discussion
Rates of chronic absenteeism
Overall, we estimate that chronic absenteeism rates for Black, White, and Hispanic students taken together are 18% percent lower at Montessori than non-Montessori schools. We did not estimate a significant difference in the overall rates of chronic absenteeism between the two school systems, contra our hypothesis. However, as can be seen in Figure 1 and based on results from our multilevel model, rates of chronic absenteeism were slightly lower in the Montessori schools for White students specifically. We do not know why we did not observe a larger difference in the average rates of chronic absenteeism for all students across school sites. Other research has shown that Montessori implementation fidelity, or the degree to which a Montessori classroom adheres to Maria Montessori’s original vision, is associated with improved outcomes for students (Lillard, 2012; Lillard and Heise, 2016), and implementation can vary widely between Montessori schools (Daoust, 2004; Lillard, 2019). We did not have a measure of fidelity of implementation within these schools, so it’s possible that average fidelity was low in this particular sample; future work may want to consider whether fidelity of implementation at a Montessori school is associated with improved student attendance and lower rates of chronic absenteeism for students of all racial identities.
However, differences in fidelity of implementation would likely not explain why we observed a slight difference for White students specifically and yet almost no differences for Black and Hispanic students. Students of color are more likely to be chronically absent, due to racial disparities in other characteristics like socioeconomic status and health, making it particularly important that attendance interventions reduce racial disparities as well (Gee, 2018). The mean rates of chronic absenteeism observed in this sample are comparable with the national average (Balfanz and Byrnes, 2012), but even higher rates have been observed elsewhere in urban schools serving predominantly low-income students (McCluskey et al., 2004; Chang and Romero, 2008; Balfanz and Byrnes, 2012; Lenhoff and Pogodzinski, 2018). Montessori schools may benefit from providing additional attendance support to Black and Hispanic students both to shrink the disparities and because doing so would support the majority of public Montessori students—who are students of color (Debs, 2016). It is also noteworthy that the average rates of chronic absenteeism for Black and Hispanic students (across both school types) differed minimally. This similarity makes the slightly lower rates for White students stark, and it underscores the need for support for Black and Hispanic student attendance. Ultimately these findings reaffirm the importance of attending to both overall rates of chronic absenteeism and rates of specific groups of students when evaluating the efficacy of any attendance intervention (Gee, 2018).
Limitations and future directions
As described previously, characteristics associated with families who self-select into Montessori schools could impact school attendance, so self-selection bias a concern when comparing Montessori and non-Montessori student outcomes. It was impossible with these data to account for all variables related to Montessori self-selection, but by overrepresenting magnet and charter schools in our non-Montessori sample (relative to the population rate of magnet and charter schools) and by controlling for charter/magnet classification, we aimed to account for as much of the variance related to school choice (regardless of what type of school was chosen) as possible.
These data do not support any claims about why the Montessori schools did not have lower average rates of chronic absenteeism than the non-Montessori schools. Previous research indicates that chronic absenteeism can be reduced through effective school and family communication, incentive programs, and student engagement (Epstein and Sheldon, 2002; Balfanz and Byrnes, 2012; Gottfried and Gee, 2017). Montessori students tend to report higher school engagement and enjoyment (Rathunde and Csikszentmihalyi, 2005; Lillard et al., 2017), but these differences did not translate to lower chronic absenteeism in this sample.
More generally, these data also reveal nothing about why some students are chronically absent. Without knowing why many students are chronically absent, developing an effective intervention to meet those students’ needs is difficult. Improved record-keeping of why students miss school—while respecting students’ and families’ privacy—would aid this effort. For instance, schools could also track the number of days missed by students due to different reasons (health, transportation, etc.) and create targeted interventions based on which reasons are most frequent. It is also possible that many of the chronically absent students reflected in this data set were chronically absent in part because of days missed due to suspension, and counterproductively, in some cases, they may have been suspended for missing school (Davis et al., 2019). Students of color are suspended at disproportionately high rates, despite a lack of evidence that their behavior warrants suspension more often than White children (Skiba et al., 2002). Researchers interested in chronic absenteeism and discipline disproportionality would benefit from more detailed data collection about why students miss school to determine if racial disparities in absenteeism and disciplinary outcomes are related. Future research would also benefit from student-level data that allows for in-depth analysis of which students are at highest risk of being chronically absent and why (Gee, 2018).
Despite these limitations, this paper offers a first look at chronic absenteeism in Montessori contexts. Additionally, this paper models an approach to compare racial disparities in chronic absenteeism across school types or levels of an intervention.
Data availability statement
Publicly available datasets were analyzed in this study. This data can be found at: https://github.com/leboeuf77/Title1-Montessori.
Author contributions
LL came up with the research question, conducted the propensity score matching process, collected the data, and wrote the abstract, introduction, and discussion sections of the manuscript. JGG came up with the analysis plan and wrote the analysis code. JGG and LL wrote the analysis plan and results sections of the manuscript. AL provided feedback and guidance throughout the project. All authors contributed to the article and approved the submitted version.
Funding
While writing this manuscript, LL, JGG, and AL and their research were supported by Wend grant 2021–132 and a grant from the Wildflower Foundation to AL, a Master’s Thesis award from the American Montessori Society to LL, and a grant from the Institute of Education Sciences, U.S. Department of Education, through Grant R305A180181 to the American Institutes for Research and AL. The research reported here was supported by the Institute of Education Sciences, U.S. Department of Education, through Grant R305B200005 to the University of Virginia. The opinions expressed are those of the authors and do not represent views of the Institute or the U.S. Department of Education.
Conflict of interest
The authors declare that the research was conducted in the absence of any commercial or financial relationships that could be construed as a potential conflict of interest.
Publisher’s note
All claims expressed in this article are solely those of the authors and do not necessarily represent those of their affiliated organizations, or those of the publisher, the editors and the reviewers. Any product that may be evaluated in this article, or claim that may be made by its manufacturer, is not guaranteed or endorsed by the publisher.
Footnotes
1. ^This article, along with others cited in this manuscript, originally used the term Latino as a racial category to describe participants. The dataset used in our study used the term Hispanic. For consistency, we use the term Hispanic throughout our article, as a 2018 Pew Survey found that the majority of Latino/Hispanic individuals have no preference between the two terms, and Pew themselves, along with other similar organizations, use the terms interchangeably (Lopez et al., 2022).
References
Balfanz, R., and Byrnes, V. (2012). Chronic Absenteeism: Summarizing What We Know From Nationally Available Data. Baltimore: Johns Hopkins University Center for Social Organization of Schools. Educ. Digest 78, 4–9.
Brooks, M. E., Kristensen, K., van Benthem, K. J., Magnusson, A., Berg, C. W., Nielsen, A., et al. (2017). glmmTMB balances speed and flexibility among packages for zero-inflated generalized linear mixed modeling. R. Journal 9, 378–400. doi: 10.32614/RJ-2017-066
Chang, H. N., and Romero, M. (2008). Present, engaged, and accounted for: The critical importance of addressing chronic absence in the early grades. Report. National Center for Children in Poverty. New York, NY: Columbia University.
Culclasure, B., Fleming, D. J., Riga, G., and Sprogis, A. (2018). An Evaluation of Montessori Education in South Carolina’s Public Schools. Greenville, SC: The Riley Institute at Furman University.
Daoust, C. J. (2004). An examination of implementation practices in Montessori early childhood education. Doctoral Dissertation. University of California, Berkeley.
Davis, K. A., Allen-Milton, S., and Coats-Boynton, S. (2019). Making the case for results-based accountability as an intervention for chronic absenteeism in schools to improve attendance. Race Justice 9, 46–59. doi: 10.1177/2153368718816500
Debs, M. C. (2016). Racial and economic diversity in US public Montessori schools. J. Montessori Res. 2, 15–34. doi: 10.17161/jomr.v2i2.5848
Dreyer, A. S., and Rigler, D. (1969). Cognitive performance in Montessori and nursery school children. J. Educ. Res. 62, 411–416. doi: 10.1080/00220671.1969.10883885
Epstein, J. L., and Sheldon, S. B. (2002). Present and accounted for: improving student attendance through family and community involvement. J. Educ. Res. 95, 308–318. doi: 10.1080/00220670209596604
Fan, X., and Nowell, D. L. (2011). Using propensity score matching in educational research. Gift. Child Q. 55, 74–79. doi: 10.1177/0016986210390635
Fleege, U. H., Black, M., and Rackauskus, J. (1967). Montessori pre-school education (ED017320). ERIC. Available at: https://files.eric.ed.gov/fulltext/ED017320.pdf
Gee, K. A. (2018). Minding the gaps in absenteeism: disparities in absenteeism by race/ethnicity, poverty and disability. JESPAR 23, 204–208. doi: 10.1080/10824669.2018.1428610
Golann, J. W., Debs, M., and Weiss, A. L. (2019). “To be strict on your own”: Black and Latinx parents evaluate discipline in urban choice schools. Am. Educ. Res. J. 56, 1896–1929. doi: 10.3102/0002831219831972
Gottfried, M. A. (2019). Chronic absenteeism in the classroom context: effects on achievement. Urban Educ. 54, 3–34. doi: 10.1177/0042085915618709
Gottfried, M. A., and Gee, K. A. (2017). Identifying the determinants of chronic absenteeism: a bioecological systems approach. Teach. Coll. Rec. 119, 1–34. doi: 10.1177/016146811711900704
Ho, D. E., Imani, K., King, G., and Stuart, E. A. (2011). MatchIt: nonparametric preprocessing for parametric causal inference. J. Stat. Softw. 42, 1–28. doi: 10.18637/jss.v042.i08
LeBoeuf, L., Goldstein-Greenwood, J., and Lillard, A. S. (in press). Multilevel modeling resolves ambiguities in analyses of discipline disproportionality: A demonstration comparing Title 1 Montessori and non-Montessori schools. J. Res. Educ. Eff.
Lenhoff, S. W., and Pogodzinski, B. (2018). School organizational effectiveness and chronic absenteeism: implications for accountability. JESPAR 23, 153–169. doi: 10.1080/10824669.2018.1434656
Lillard, A. S. (2012). Preschool children’s development in classic Montessori, supplemented Montessori, and conventional programs. J. Sch. Psychol. 50, 379–401. doi: 10.1016/j.jsp.2012.01.001
Lillard, A. S. (2019). Shunned and admired: Montessori, self-determination, and a case for radical school reform. Educ. Psychol. Rev. 31, 939–965. doi: 10.1007/s10648-019-09483-3
Lillard, A. S., and Else-Quest, N. (2006). Evaluating Montessori education. Science 313, 1893–1894. doi: 10.1126/science.1132362
Lillard, A. S., and Heise, M. J. (2016). An intervention study: removing supplemented materials from Montessori classrooms associated with better child outcomes. J. Montessori Res. 2, 16–26. doi: 10.17161/jomr.v2i1.5678
Lillard, A. S., Heise, M. J., Richey, E. M., Tong, X., Hart, A., and Bray, P. M. (2017). Montessori preschool elevates and equalizes child outcomes: a longitudinal study. Front. Psychol. 8:1783. doi: 10.3389/fpsyg.2017.01783
Lopez, M. H., Krogstad, J. M., and Passel, J. S. (2022). Who is Hispanic. Available at: https://www.pewresearch.org/fact-tank/2022/09/15/who-is-hispanic/
McCluskey, C. P., Bynum, T. S., and Patchin, J. W. (2004). Reducing chronic absenteeism: an assessment of an early truancy initiative. Crime Delinq. 50, 214–234. doi: 10.1177/0011128703258942
Orfield, G., and Frankenberg, E. (2014). Increasingly segregated and unequal schools as courts reverse policy. Educ. Adm. Q. 50, 718–734. doi: 10.1177/0013161X14548942
Rathunde, K., and Csikszentmihalyi, M. (2005). Middle school students’ motivation and quality of experience: a comparison of Montessori and traditional school environments. Am. J. Educ. 111, 341–371. doi: 10.1086/428885
Skiba, R. J., Michael, R. S., Nardo, A. C., and Peterson, R. L. (2002). The color of discipline: sources of racial and gender disproportionality in school punishment. Urban Rev. 34, 317–342. doi: 10.1023/A:1021320817372
Snyder, A., LeBoeuf, L., and Lillard, A. S. (2022). “My name is Sally Brown, and I hate school!”: a retrospective study of school liking among conventional and Montessori school alumni. Psychol. Sch. 60, 541–565. doi: 10.1002/pits.22777
Steiner, P. M., Cook, T. D., Li, W., and Clark, M. H. (2015). Bias reduction in quasi-experiments with little selection theory but many covariates. J. Res. Educ. Effect. 8, 552–576. doi: 10.1080/19345747.2014.978058
Stempel, H., Cox-Martin, M., Bronsert, M., Dickinson, L. M., and Allison, M. A. (2017). Chronic school absenteeism and the role of adverse childhood experiences. Acad. Pediatr. 17, 837–843. doi: 10.1016/j.acap.2017.09.013
Stuart, E. A. (2010). Matching methods for causal inference: a review and a look forward. Stat. Sci. 25, 1–21. doi: 10.1214/F09-STS313
US Department of Education. (2019). Chronic absenteeism in the nation’s schools: a hidden educational crisis. Available at: https://www2.ed.gov/datastory/chronicabsenteeism.html
Keywords: racial disparities, chronic absenteeism, Montessori education, multilevel modeling, Title 1 schools
Citation: LeBoeuf L, Goldstein-Greenwood J and Lillard AS (2023) Rates of chronic absenteeism in Montessori and non-Montessori Title 1 schools. Front. Educ. 8:1059071. doi: 10.3389/feduc.2023.1059071
Edited by:
Arya Ansari, The Ohio State University, United StatesReviewed by:
Yusuf Kara, Southern Methodist University, United StatesQingqing Yang, The Ohio State University, United States
Copyright © 2023 LeBoeuf, Goldstein-Greenwood and Lillard. This is an open-access article distributed under the terms of the Creative Commons Attribution License (CC BY). The use, distribution or reproduction in other forums is permitted, provided the original author(s) and the copyright owner(s) are credited and that the original publication in this journal is cited, in accordance with accepted academic practice. No use, distribution or reproduction is permitted which does not comply with these terms.
*Correspondence: Lee LeBoeuf, ll3jt@virginia.edu