- 1Institute for Research Methods in Educational Science, University of Education, Karlsruhe, Germany
- 2Strategic Corporate Development and Communications, Karlsruhe Institute of Technology, Karlsruhe, Germany
- 3Psychological Assessment, TU Dortmund University, Dortmund, Germany
Introduction: Self-efficacy is an important predictor of teaching behavior. Within several research traditions (TPACK framework, school achievement tests), different ICT self-efficacy scales have been developed. The empirical structure of existing questionnaires has frequently been researched and discussed within the TPACK framework. However, for teacher ICT self-efficacy, non-instructional aspects as well as alternative instruments have rarely been investigated and compared to standard TPACK self-report scales.
Methods: In this study, we administer two sets of non-subject-specific ICT scales to a group of pre-service teachers (N = 165). We investigate the empirical structure using structural equation modeling.
Results: For both scales, the results show that instructional ICT self-efficacy forms a separate factor. For the remaining items, item difficulty and content drive further divisions. Further, more specific item formulations resulted in a higher range of scale means. Additionally, we find gender differences only on some non-instructional scales.
Discussion: Results confirm that the distinction between instructional vs. non-instructional ICT self-efficacy is important when developing or using questionnaires for pre-service teachers. Results also indicate that the usage of more specific task-related items is a promising alternative to general TPACK items.
Introduction
Technology has been used in classrooms and education for almost 100 years, with radios broadcasting educational content as early as the 1920s (Cuban, 1986). Since then, there has been a steady inflow of increasingly powerful information and communication technology (ICT) into classrooms. As a result, teachers faced new challenges such as introducing students to modern technology and media as well as using it in a meaningful manner to support and elevate students’ learning. This also means that teachers need 21st century skills and confidence in their technological competencies to adapt to these evolving needs and opportunities (Falloon, 2020).
This confidence to master complex situations is often subsumed under the term self-efficacy. Self-efficacy comprises the personal belief in one’s organization, abilities, and skills to handle a situation (Bandura, 1977). Self-efficacy is considered an important concept as it is a central predictor for an individual’s behavior and performance. Tschannen-Moran and Hoy (2001) report that teachers’ self-efficacy is related to student achievement. Self-efficacy is usually regarded domain-specific (Bandura, 2006). Questionnaires may address teachers’ self-efficacy (e.g., Tschannen-Moran and Hoy, 2001), academic self-efficacy (e.g., Pumptow and Brahm, 2021), or technology integration into teaching (e.g., Wang et al., 2004). Regarding teachers’ self-efficacy in ICT related knowledge and skills, questionnaires based on the TPACK framework (Technological, Pedagogical, And Content Knowledge, Mishra and Koehler, 2006) have often been used. However, these questionnaires have shortcomings: In addition to general problems of self-reports, there is an ongoing discussion about their factor structure. Further, the factor capturing general Technological Knowledge (TK) is usually treated as a unidimensional construct (see “The TPACK framework” below for a short description of the TPACK framework and its components). However, several theoretical frameworks, for example the DIGCOMP model by Ferrari (2013) and its current version DIGCOMP 2.2 (Vuorikari et al., 2022) as well as other instruments (for example the student questionnaire from the International Computer and Information Literacy Study ICILS, see Fraillon et al., 2014) suggest several dimensions within this factor. The DIGCOMP model (Ferrari, 2013; Vuorikari et al., 2022) distinguishes five competence domains (information, communication, content creation, safety, and problem solving) containing several competencies, respectively. The ICILS-studies focus on collecting and organizing vs. producing and exchanging information with several sub-domains. These sub-domains comprise knowledge about computers and safe use of information (Fraillon et al., 2014). In the 2018 study, computational thinking (i.e., the ability to solve problems by using computers) was added (Fraillon et al., 2020). Like the TK factor, these and other models focus on general ICT competencies. They propose similar competence domains, but the number and the labels of these domains as well as their structure (e.g., whether safety is considered a main topic or sub-domain) differ between the models (see Hatlevik et al., 2018, p. 109, or Rubach and Lazarides, 2019, for a similar argumentation).
In the following study, we address these issues and compare two traditions of self-report questionnaires on ICT self-efficacy and instruments developed within them. The first tradition builds on the TPACK model (Mishra and Koehler, 2006), which acts as a content starting point. The second tradition relates to the idea of self-efficacy (Bandura, 1977) as an underlying theory and was applied in both, smaller scientific studies (Reddy et al., 2021; Tzafilkou et al., 2021) and large-scale assessments like the ICILS study (Fraillon et al., 2014, 2020). By comparing these traditions on a conceptual level and on an empirical basis, we show that both traditions share similarities, but also exhibit differences, for example with regard to item formulations and factor structure. In particular, based on empirical results, we propose possible subdivisions of the purely technological domain. Additionally, the results are relevant for the design of ICT self-report questionnaires, as they point out important aspects of item formulation.
We start by shortly summarizing the TPACK model, associated instruments, as well as further ICT self-efficacy questionnaires. Throughout this article, we focus on non-instructional and instructional, non-subject-specific scales. We identify similarities and differences between the traditions and summarize findings on the role of gender in ICT self-efficacy reports. In an empirical study on exemplary questionnaires based mainly on existing instruments (Gerick et al., 2018; Schmid et al., 2020, see Appendix A for a detailed listing), we investigate the factorial structure of both instruments. Further, by looking at correlations between the resulting subscales and gender differences on them, we take a first step to examine the construct validity of these instruments.
Theoretical underpinnings and empirical background
Within the next sections, we outline theoretical frameworks on ICT competencies and ways to assess these competencies along with empirical findings. In particular, we will focus on the TPACK framework (Mishra and Koehler, 2006) and self-report questionnaires. We also consider alternative self-report questionnaires used less frequently within research and evaluation of pre-service teachers’ competencies and training programs. Finally, we summarize the findings on gender differences in ICT skills and self-efficacy, which play an important role in interpreting the results of self-report questionnaires.
The TPACK framework
Many frameworks on ICT competencies have been developed. There are general frameworks for example the DIGCOMP framework by Ferrari (2013), or its current version by Vuorikari et al. (2022) as well as frameworks within education (for example the theoretical framework of the ICILS studies, Fraillon et al., 2014); or the DigCompEdu framework by Punie and Redecker (2017). Within teacher education, the TPACK framework by Mishra and Koehler (2006) is widely used. Based on the idea of Shulman (1987) that content knowledge, pedagogical knowledge, and pedagogical content knowledge are central for teachers, Mishra and Koehler (2006) extended this concept to include technological knowledge and its intersections with the previously mentioned knowledge areas. The resulting framework consists of seven central knowledge areas (Mishra and Koehler, 2006; Schmidt et al., 2009; Valtonen et al., 2015; Backfisch et al., 2020; Rahmadi et al., 2020; Zhang and Tang, 2021):
TK: Technological Knowledge about standard technologies, possibilities, constraints, usage, and interest in these technologies (e.g., using standard software tools, adding or removing single technical components).
PK: Pedagogical Knowledge about teaching and learning as well as purposes, values, and aims of teaching on a general level.
CK: Content Knowledge about a specific subject matter (e.g., facts, concepts, theories, procedures, and content specific ways of inquiry).
TPK: Technological Pedagogical Knowledge as the intersection of technological and pedagogical knowledge (e.g., choosing a suitable technological tool for a pedagogical task).
PCK: Pedagogical Content Knowledge on how to teach and learn a specific content (e.g., suitable representational forms, typical prior knowledge of students).
TCK: Technological Content Knowledge how technology and a specific content relate to each other (i.e., how technology has changed ways of inquiry and representation).
TPCK: Technological Pedagogical Content Knowledge comprising all basic aspects (i.e., how technology can be used to support a content specific teaching approach).
According to Mishra and Koehler (2006), the term knowledge also includes attitudes, skills, and competencies (see Seufert et al., 2021, for a more detailed discussion). Mishra and Koehler (2006) state and show empirically that it is important to provide training for the combined aspects in their model—TPK, TCK, and especially TPCK.
In this study, we focus on TK and TPK for several reasons: First, as we focus on pre-service teachers, the transition from general technological knowledge to more teaching specific knowledge is a central point. Further, several content domains may require different subdivisions. Finally, we aim at comparing instruments from different research traditions. TK and TPK are dimensions frequently found in different areas of research on digital literacy. In many models, TK is split into further subcomponents. Siddiq et al. (2016) conclude that the different competency models and frameworks are based on similar common ideas of retrieving, processing, and producing information, communication aspects, and responsible ICT use. Regarding the TPACK model, these distinctions can be interpreted as one possible subdivision of the TK factor (Rubach and Lazarides, 2019).
Based on TPACK model and its practical implications for teacher training, different questionnaires and other instruments have been developed in order to assess ICT competencies, for example the instrument developed by Schmidt et al. (2009). They will be described in the next section.
TPACK questionnaires
Although different ways to measure TPACK exist and testing may seem attractive to circumvent possible biases of self-reports (e.g., Siddiq et al., 2016), using self-report measures is widespread in empirical research on teachers’ TPACK (Wang et al., 2018; Willermark, 2018): Self-reports are easily applicable, less time consuming, and the transparency of the operationalization and reliabilities are usually higher (Willermark, 2018). Items can be formulated on a more general level, being more flexible and less susceptible to knowledge changing rapidly in this field (see Richter et al., 2001 for an older instrument with many items that are outdated by now). Some authors use the term self-efficacy when referring to TPACK self-report questionnaires (Backfisch et al., 2020; Lachner et al., 2021: Technology-related self-efficacy; Lee and Tsai, 2010 for the TPCK-W survey; Scherer et al., 2021). As mentioned in the introduction, although self-report questionnaires have the disadvantage of being subjective, motivational aspects captured by these scales are a co-determinant of showing a behavior in a specific situation and have an additional value when compared to objective testing procedures.
Based on the TPACK framework (Mishra and Koehler, 2006) and the self-report questionnaire published by Schmidt et al. (2009), several versions of this instrument and competing questionnaires have been published (Chai et al., 2013; Voogt et al., 2013; Wang et al., 2018; Willermark, 2018, provide overviews of existing instruments). Most TPACK self-report questionnaires ask for agreement on generic statements in each domain and use 5–7 point Likert scales as response scales (Willermark, 2018).
Although or because these TPACK self-report questionnaires are often used, empirical findings point to several shortcomings. First, there is an ongoing discussion if the dimensions of TPACK self-reports correspond to the structure of the TPACK framework (Chai et al., 2013; Voogt et al., 2013; Valtonen et al., 2017; Wang et al., 2018; Willermark, 2018). Some of the resulting models found seven correlated factors corresponding to the seven content areas postulated by the TPACK model (Chai et al., 2013; Schmid et al., 2020). Few authors found additional dimensions (Koh et al., 2010) or a different subdivision (Valtonen et al., 2015). Many researchers found fewer dimensions with a tendency for items on technological knowledge (TPK, TCK, and/or TPCK) to load on a single factor. Differences in the findings might be due to samples differing by expertise levels or cultural backgrounds. Differing item wordings and different approaches to data analysis may have contributed to the diverging findings on the factorial structure as well.
Scherer et al. (2017) restrict themselves to the T-dimensions (TK, TPK, TCK, and TPCK) and explore their factor structure. They conclude that the items of the TPK, TCK, and TPCK subscales form a single factor. Concerning TK, the authors favor a nested model where the TK items belong to both a TK factor and a general TPACK factor. They encourage researchers to take a closer look at the TK factor for unlocking its meaning and unravel the overall factorial structure of the TPACK self-report scales. Willermark (2018) states that several researchers criticized the TK factor for “being vague and too extensive” (p. 316). Few attempts have been made so far: Lee and Tsai (2010) intended to differentiate between general technical and communicative technical knowledge, but these items formed a single TK factor in subsequent analyses. Within the body of research on ICT attitudes, it has been shown to be useful to distinguish between behavior-oriented and object-oriented attitudes (Scherer et al., 2018; Sailer et al., 2021). This distinction might also be applicable to single subscales of TPACK self-report questionnaires.
Partly depending on the relatedness of underlying constructs, several studies found moderate to strong factor correlations. Some authors state that the TK factor shows lower correlations than the other factors within themselves (e.g., Valtonen et al., 2017). There is a wide range of correlations found between these two factors, reaching from 𝑟TK TPK = 0.37 (Schmid et al., 2020) to 𝑟TK TPK = 0.79 (Canbazoğlu Bilici et al., 2013). Similar to the unstable factor structure above, determinants for these fluctuations in the correlations are not known. Besides different cultural or educational systems that may suggest more or less related knowledge areas, the quite generic wording of many TPACK items in self-report questionnaires leaves room for interpretation by the respondents. Looking at other, similar instruments from large-scale assessments, and comparing those to standard TPACK items, may yield further insights.
Other questionnaires assessing ICT self-efficacy
Self-report questionnaires on digital competencies are part of large-scale assessments such as the Programme for International Student Assessment (PISA) or the ICILS study. They contain thoroughly developed items and scales for digital competencies with additional empirical results available. The PISA studies do not focus primarily on digital competencies. However, single aspects like digital reading play an increasing role (OECD, 2017). Accompanying questionnaires contain items on media use as well as on attitudes and self-reported digital abilities (OECD, 2017) similar to items from the TPACK self-report questionnaires. Additionally to these instruments developed for large scale assessments, ICT self-efficacy scales for specific purposes have been developed (see Tzafilkou et al., 2021, for a study using ICT self-efficacy scales for teacher educators). Recently, ICT self-efficacy has been shown to be a predictor of computer information literacy and technology acceptance in students (Hatlevik et al., 2018; Reddy et al., 2021).
The ICILS studies (Fraillon et al., 2014, 2020) focus on ICT knowledge and associated measures of 8th graders. In the students’ questionnaire, in addition to performance tests, the students’ self-efficacy is surveyed on both basic, everyday tasks and advanced, complex and technical tasks (Fraillon et al., 2014; Gerick et al., 2018). The correlation between these scales was 𝑟basic advanced = 0.64 with minor fluctuations across countries (Rohatgi et al., 2016, for a reanalysis from the ICILS 2013 study).
For the teachers’ questionnaire, Scherer and Siddiq (2015) investigated the factorial structure of computer self-efficacy in a Norwegian sample with support for a three-factor model with a factor for basic skills, for advanced skills (operational and collaborative), and for computer use on instructional purposes, respectively. They also found high correlations between the factors (𝑟basic advanced = 0.81, 𝑟basic instructional = 0.74, 𝑟advanced instructional = 0.76).
Both, the students’ and the teachers’ scale, are rated on a three-point response scale with the options I do not think I can do this, I could work out how to do this, and I know how to do this. Thus, in contrast to the TPACK items mentioned above, they follow Bandura’s (2006) recommendations by using different degrees of confidence in one’s ability on the response scale, but with less answer categories than proposed by Bandura, and a variety of specific tasks within their items (see Hatlevik et al., 2018, p. 117, for some comments on the response scales for self-efficacy items).
Interim summary and comparison of instruments
Both self-report questionnaires from the TPACK framework and similar questionnaires from the self-efficacy tradition aim at measuring ICT competencies. Compared to tests, they are less objective but do capture additional motivational aspects (Backfisch et al., 2020). A deepened understanding how these questionnaires work, including their origin and the underlying constructs, helps to choose the appropriate questionnaire and to interpret its results properly. Comparing these instruments at a descriptive, theoretical level provides an important basis for comparison using empirical data:
As noted above, both sets of instruments stem from different lines of research. The TPACK framework proposed by Mishra and Koehler (2006) builds upon the taxonomy suggested by Shulman (1987). Shulman distinguishes between three central knowledge domains (PK, CK, and PCK) that teachers should master. TPACK extends this model to include TK as well as its overlap with the previous three knowledge domains. According to Shulman (1987), reasoning and action operate on this corpus of knowledge. The concept of self-efficacy in contrast is already more action–oriented (Bandura, 1977). Its subdivision is related to specific goals and situations a person may encounter. Abu Bakar et al. (2020) argue that TPACK can be used by a person to get a sense of his or her self-efficacy regarding ICT-use in teaching contexts. However, as the items themselves may not fully transport the underlying idea of the two research traditions, looking closer at the instruments themselves may uncover differences as well as similarities between them.
A first difference is the general approach: Whereas self-efficacy as a belief is primarily captured by using self-reports, for example by self-efficacy scales, TPACK as knowledge can be captured by tests or outcomes like lesson plans as well (Wang et al., 2018; Willermark, 2018). However, as mentioned above, TPACK self-report scales are frequently used, and have even recently been labeled as self-efficacy scales (e.g., Backfisch et al., 2020; Lachner et al., 2021; Scherer et al., 2021).
A second difference is in the target group of the questionnaires: Self-report questionnaires from the TPACK framework were developed to survey pre- and in-service teachers, whereas questionnaires from large-scale assessments are intended to survey either students or teachers. Especially the student questionnaires could be complementary to TPACK instruments for pre-service teachers who are in transition between being students and being professionals. Thus, within this paper, we investigate the relationships of selected items from large-scale assessments to items and scales from the TPACK framework.
A third, related difference is the face-content of the scales. Some address the use of media for everyday purposes, especially those directed at students, but also other questionnaires on self-reported digital abilities and competencies (e.g., van Deursen et al., 2014; Reddy et al., 2021). Other scales are directed at teachers and capture instructional and pedagogical use of media (Schmidt et al., 2009; Schmid et al., 2020; Tzafilkou et al., 2021). A further distinction described above is the focus on attitudes or tasks (Seufert et al., 2021). Usually, items formulated to capture self-efficacy like some ICILS scales (Fraillon et al., 2014; Gerick et al., 2018; Hatlevik et al., 2018) are more oriented towards tasks (Siddiq et al., 2016). Therefore, these items are also more specific and less generic as some items from TPACK self-report questionnaires or the PISA study (but see Reddy et al., 2021 for an exception). This may imply that respondents have less scope for interpretation (see for example Groves et al., 2009, for a general model of the response process), but also that these items may have to be adapted for the field of interest as self-efficacy is domain specific (Bandura, 2006).
Empirical findings from studies on teachers’ ICT competencies underline the important role of content similarity between several self-report scales (see Podsakoff et al., 2003 for critical comments on response biases due to content overlap): In most cases, medium to high correlations between different instruments have been observed. Empirical results suggest that TPACK self-reports and self-efficacy scales capture similar constructs, and that there are higher correlations when there is a larger overlap of face-content: Joo et al. (2018) investigated the correlations between two TPACK sub-scales, general teacher self-efficacy, and other scales belonging to the technology acceptance model in a South Korean sample. They found weak to moderate correlations between TPACK and teacher self-efficacy scales ranging from 0.35 to 0.50. Correlations between TPACK and teacher self-efficacy scales were higher for the teaching related teacher self-efficacy scale than for the administrative teacher self-efficacy scale. Abu Bakar et al. (2020) found relatively high correlations between a teaching related self-efficacy scale and different TPACK sub-scales (𝑟s ranging from 0.61 to 0.83). Kul et al. (2019) found slightly lower correlations between TPACK and self-efficacy on using Web 2.0 technologies for teaching (𝑟s between 0.39 and.76). Thus, content overlap of the scales might be a central source for the different correlation levels.
Finally, not only the item wording but also the response format differs between items: TPACK and PISA items use Likert-type response scales with differing levels of agreement. However, some TPACK self-report scales use response scales with differing levels of self-rated competence or formulate statements that ask for individual abilities to use digital media and technologies in a specific way (Voogt et al., 2013). On the other hand, ICILS self-efficacy items are rated on a three-point response scale with differing levels of confidence in accomplishing a specific task. It is known that features of the response scale may affect data quality (DeCastellarnau, 2018).
Gender differences on self-reported technology-related skills and competencies
A frequently discussed issue in both ICT skills and competency self-assessments is gender. Gender is known to be an important predictor of self-reported ICT related skills and digital competencies (Venkatesh and Davis, 2000; Sieverding and Koch, 2009; Hohlfeld et al., 2013; Gebhardt, 2019). Knowing the sensitivity of scales to gender is a relevant point for further analyses (e.g., including gender as a relevant covariate) and for interpreting the results of a questionnaire.
Within students, Gebhardt (2019) found little to no difference between male and female students concerning basic self-efficacy, but they did find gender differences favoring males in the advanced self-efficacy scale. This may reflect the results of gender differences in different item functioning, where tasks involving the creation of information favor female students, while technical tasks such as information security or conceptual understanding of computers favor male students’s (Gebhardt, 2019). Hatlevik et al. (2018) find gender differences on ICT self-efficacy among students in some, but not all countries. They argue that this may suggest that the traditional differences are declining.
Within adults, Sieverding and Koch (2009) examine gender effects in men and women assessing their own technological skills in comparison to others as well as in their ratings of other people’s skills. Their study focuses on a complex task and shows that men and women alike rate their own technological skills significantly higher if they compare themselves to a woman than if they compare themselves to a man. Independently of their comparisons to other people, women rate their own skills significantly lower than men do (Sieverding and Koch, 2009). There are similar findings in other cultures (e.g., Durndell and Haag, 2002). However, Hohlfeld et al. (2013) summarize that previous research has shown that expected gender-differences are usually more pronounced in adults than in children. Overall, the body of research suggests that men and women do only slightly differ in actual ICT skills but women tend to report lower skill-levels when asked about their own skills than men with a comparable level of ICT skills.
Some studies focusing on teachers found similar results. Within mathematics teachers, Abu Bakar et al. (2020) detected no significant differences in TPACK self-report scales and teaching related self-efficacy scales in a small sample, but small to medium effect sizes favoring men (𝑑TPACK = 0.29, 𝑑self-efficacy = 0.48). Scherer et al. (2017) found support for the invariance of TPACK self-report scales across gender. There were mean differences for gender on both the general TPACK scale and the TK scale. This difference was more pronounced on the TK factor than on the general factor with male teachers reporting higher values. Scherer and Siddiq (2015) found significant gender differences on the ICILS basic and advanced skills scales, but not on the instructional scale.
In summary, gender seems to be a relevant predictor in self-reported digital competencies, especially on scales on advanced technology. However, fewer or no gender differences were found for scales addressing instructional purposes.
Aims and research questions of the present study
Valid questionnaires capturing meaningful constructs in a differentiated manner provide a foundation for assessing and improving ICT related skills and self-efficacy in pre-service teachers. In order to investigate the factorial structure of non-subject-specific items on ICT self-efficacy from two instruments within pre-service teachers, we inspect the structure within each instrument as a first step (Figure 1). We are interested in whether instructional and non-instructional items form distinct factors, and whether the sub-division of non-instructional items suggested by previous findings holds empirically. Further, we examine factor correlations and gender differences to gain initial insights into the construct validity of the resulting scales.
1. Regarding items from the TPACK tradition (Schmidt et al., 2009; Schmid et al., 2020), we assume that teaching related and purely technology related items form different latent factors (Scherer et al., 2017). Schmid et al. (2020) developed a short version of the TPACK self-report questionnaire with a TK factor consisting of four items capturing interest in and attitudes towards digital media. However, the original scale also contained more behavior-oriented items, for example on solving technological problems. We assume that items of the TK factor by Schmid et al. (2020) and more behavior-oriented items like technological problem solving (PS) form separate factors (Scherer et al., 2018; Willermark, 2018). Therefore, we will estimate three competing models:
(A1) Single factor model.
(B1) Two factor model comprising a factor for TK and for TPK, respectively.
(C1) Three factor model comprising a factor for TK, PS, and TPK, respectively.
Based on previous research, we expect the last model to provide the best fit.
2. Regarding items from or in the style of the ICILS study (Fraillon et al., 2014, 2020; Gerick et al., 2018), we hypothesize that items may form two latent factors based on item difficulty, as factors in the ICILS study resemble either easy, everyday tasks or difficult, more advanced tasks. Similar to the findings from the TPACK framework and on ICILS-items (Scherer and Siddiq, 2015), we further assume that items with instructional content may be further extracted as an additional factor. Again, we will estimate three competing models:
(A2) Single factor model.
(B2) Two factor model with items on basic vs. advanced tasks.
(C2) Three factor model with items on basic, advanced, and instructional tasks.
As above, we expect the last model to provide the best fit.
3. Similar to a multitrait-multimethod approach (Campbell and Fiske, 1959), we examine correlations between the latent factors resulting from both preceding analyses. The correlation pattern should provide insights into the nomological network between the factors. We assume to find higher correlations between factors with a higher content overlap (resembling convergent vs. discriminant validity; possible aspects of face-content are instructional vs. non-instructional, and task vs. attitude orientation) or formulation of the response scale (resembling a method effect).
4. Gender: Self-reports of competencies as well as self-efficacy ratings are subject to gender differences, with males frequently reporting higher proficiency levels. This especially applies to scales that capture these aspects with regard to advanced technology use (e.g., Scherer and Siddiq, 2015; Scherer et al., 2017), but less with regard to basic and instructional use of ICT (e.g., Gebhardt, 2019; Abu Bakar et al., 2020). Based on previous findings, we assume to find
(a) strong associations between gender and factors capturing advanced non-instructional aspects favoring male students, and
(b) lower or even no associations between gender and factors addressing technology use for instructional purposes and less advanced technology-only aspects.
Materials and methods
Sample and procedure
The present study is based on a sample of 𝑁 = 165 pre-service teachers enrolled as students in a degree program at the University of Education Karlsruhe for becoming primary or secondary school teachers. They participated in an online survey as part of several courses in November 2020. The survey was conducted as a part of the University of Education Karlsruhe project which aims to develop and strengthen digital competencies within pre-service teachers. Participation in the study was voluntary and without compensation. The survey took place with the aim of providing lecturers and university executives with information about digital competencies of the students as a part of course and curriculum development. Participating courses included a lecture on media literacy, a lecture on research methods, and a seminar on computer use in mathematics education.
Due to the Covid-19 pandemic, we invited students to participate via mail, providing a link to the online questionnaire. The questionnaire consisted of a welcome page, an informed consent, a person specific code, demographic data, and several pages of items on self-reported technology-related competencies described below. Further, a motivation scale and feedback questions were included but are not considered here.
Out of the 181 questionnaires that were started, we excluded 16. Eleven participants only filled in the demographic data but stopped before answering the study specific items and three participants were excluded due to implausible responses (e.g., only the highest answer category). For one participant who did the survey three times, we used only the first trial. Finally, 165 questionnaires remained. Of these, 142 questionnaires were answered completely, and 152 participants filled in at least some of the ICILS items in the second part of the questionnaire.
Participants reported an average age of 22.8 years (SD = 4.0). Regarding gender, 146 persons (88.5%) reported being female, 18 (10.9%) reported being male, and one person reported being non-binary. This is a typical distribution of gender among pre-service teachers in these degree programs. The mean reported study semester was 3.1 (SD = 1.8). Further, 121 (73.3%) participants were enrolled for a Bachelor’s degree, and 44 (26.7%) for a Master’s degree. For future employment, 101 participants (61.2%) indicated that they were studying to become an elementary school teacher, and 64 (38.8%) to become a secondary school teacher.
Measures
We used several self-report measures for competencies in digital media. The survey started with TPACK items from two short self-report scales for TK and TPK (Schmid et al., 2020). We supplemented both sets of items with further items from a pilot study in a similar sample, where two factors emerged from an exploratory factor analysis: one factor addressing purely technological aspects, and a second factor addressing technology use for teaching. These items originated from several questionnaires (Schmidt et al., 2009; OECD, 2017). We used existing German translations for the wording of the items. Most items were slightly adjusted to fit the response pattern or the general wording of the questionnaire and the involved courses. Especially, the term technologies was replaced by digital media and further described as comprising both hard- and soft-ware components of technology. All items were rated on a 5-point Likert scale (1 = do not agree at all to 5 = fully agree). As part of the initial analysis, we removed items that contributed least to the internal consistency of TK and TPK scales. However, since this would have dropped all of Schmid’s TK items, these items were retained as a separate scale. Finally, three different scales with four items each entered the subsequent structural equation modelling (SEM) analysis: the TK scale contained in Schmid et al. (2020) capturing interest and general skills in using digital media, an additional scale on technological problem solving (PS) with general items on the ability to solve technological problems and install software, as well as a final scale primarily consisting of TPK items on employing digital media for instructional goals.
As a second set of items, items closer to Bandura’s (2006) idea of self-efficacy were adopted from or developed based on the student questionnaire of the ICILS 2013 study (Fraillon et al., 2014; Gerick et al., 2018): the first scale captures self-efficacy on basic tasks; the second scale captures self-efficacy on more advanced and complex tasks. Both scales are rated on the same response scale (1 = I do not think I can do that; 2 = I could figure out how to do that; 3 = I know how to do that.). In order to administer this scale within pre-service teachers, we added a further answer category 4 = I can teach others how to do that, adding a level of proficiency and rebinding the items to a teaching and learning context. Moreover, we added several items: Two items were adapted from van Deursen et al. (2014). We formulated five new items on technology use in teaching according to the content of the involved courses (producing an e-book, producing an explainer video, using virtual or augmented reality applications, developing a WebQuest, using mobile technologies for teaching outside classrooms).
Table 1 summarizes characteristics of the resulting scales. Regarding measures of reliability, we estimated Cronbach’s 𝛼 and McDonald’s 𝜔 (McDonald, 1999). All scales showed acceptable to high reliabilities within the boundaries of other TPACK self-report measures (α > 0.70, cf. Voogt et al., 2013) except the scale on basic self-efficacy, which was slightly below that value. Supplementary Tables 4 and 5 in Appendix A provide item wording and additional information.
Data analysis
We used the statistical software R (R Core Team, 2021) and the lavaan package on structural equation modeling (SEM; Rosseel, 2012) for data analysis. Due to non-normal data, we employed robust WLSMV estimates with pairwise deletion of data. Model fit criteria were used to assess model fit according to cut-off values reported in Marsh et al. (2005) for a moderate model fit (i.e., CFI ≥ 0.95, TLI ≥ 0.95, RMSEA ≤ 0.08, and SRMR ≤ 0.10) and for model comparisons.
First, we estimated three competing measurement models for the items from the TPACK self-report scales (Figure 1A1–C1), and for the items in the style of the ICILS self-efficacy scales (Figure 1A2–C2), respectively.
Based on existing studies of TPACK self-report questionnaires, we compared a model with a single factor, a model with one factor for technology-only items and a one factor for instructional items, and a third model with an additional factor resembling items on solving technological problems as a separate factor.
For the self-efficacy items, we also started with a single factor model. Then we followed the idea of difficulty factors in the ICILS study: We assigned all newly formulated items on pedagogical software use to the ICILS advanced self-efficacy scale as they consisted of relatively complex tasks (see Supplementary Table 5 for means of the respective items). Likewise, we assigned the easy items from van Deursen et al. (2014) to the base self-efficacy factor. Finally, we estimated a three-factor model, where the newly formulated items on instructional and pedagogic media tasks formed a separate factor. For the two-factor and the three-factor model of self-efficacy style items, we allowed for correlated error terms between two items addressing image editing and creating videos.
Additionally, we were interested in the nomological network of the resulting scales. The term nomological network was developed within the concept of construct validity by Cronbach and Meehl (1955): “According to construct validity theory, a construct is implicitly defined by its position in a network of other constructs that is deduced from theory and based on scientific laws—the ‘nomological net’” (Preckel and Brunner, 2017, 1). The nomological network is empirically examined by observing convergent and divergent validity through estimation of correlation patterns between scales as described by Campbell and Fiske (1959).
In our analysis, we investigated the nomological network by estimating a single SEM model that included the previously developed best-fitting measurement models, and comparing the correlations between the latent factors. We did not use path analysis to avoid causal interpretations of the network, which are possibly misleading in cross-sectional studies.
Finally, gender was included as an exogenous variable in this overarching model, as the number of male participants did not allow for multi-group comparisons. Gender was coded by 1 for female students and 2 for male students. Appendix B provides lavaan syntax and output of this most comprehensive model.
Results
To learn about possible factor structures within each of both sets of items, we compared three models for the TPACK (Figure 1A1–C1) and self-efficacy (Figure 1A2–C2) items, respectively. Additionally, for a direct comparison of the instruments and a first step towards construct validity, we inspected the correlational pattern of the resulting factors across both sets of items and gender differences on them.
TPACK items
For the TPACK items, the model with a single factor yielded the worst fit, whereas the fit of the model with separate TK and TPK dimensions was better (Table 2). The third model that contained an additional factor for problem solving achieved an acceptable model fit on all fit indices and the best fit of the three models (𝜒2(51) = 90.1, 𝑝 = 0.001, CFI = 0.981; TLI = 0.975; RMSEA = 0.068, 90% CI [0.044; 0.091]; SRMR = 0.065).
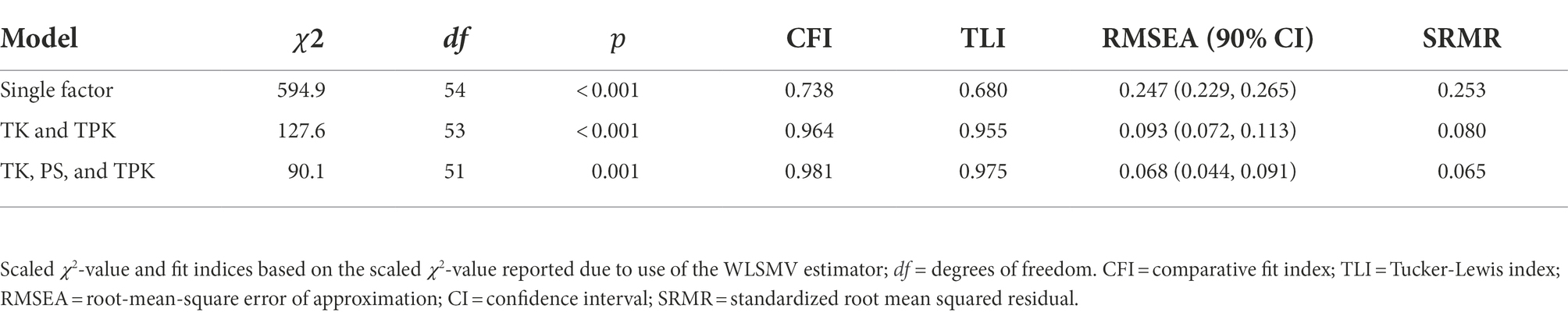
Table 2. Fit indices different numbers of factors in a CFA (general statements of technological and technological pedagogical knowledge, 𝑁 = 165).
Self-efficacy items
For the self-efficacy items (Table 3), again the model with a single factor yielded the worst fit, whereas the fit of the model with two factors representing the difficulty of the tasks similar to the original ICILS-scales was acceptable (𝜒2(168) = 232.5, 𝑝 = 0.001, CFI = 0.965; TLI = 0.960; RMSEA = 0.050, 90% CI [0.033; 0.065]; SRMR = 0.097). The third model that introduced an additional factor for pedagogical tasks improved model fit slightly (𝜒2(166) = 223.7, 𝑝 = 0.002, CFI = 0.969; TLI = 0.964; RMSEA = 0.048, 90% CI [0.030; 0.063]; SRMR = 0.095).
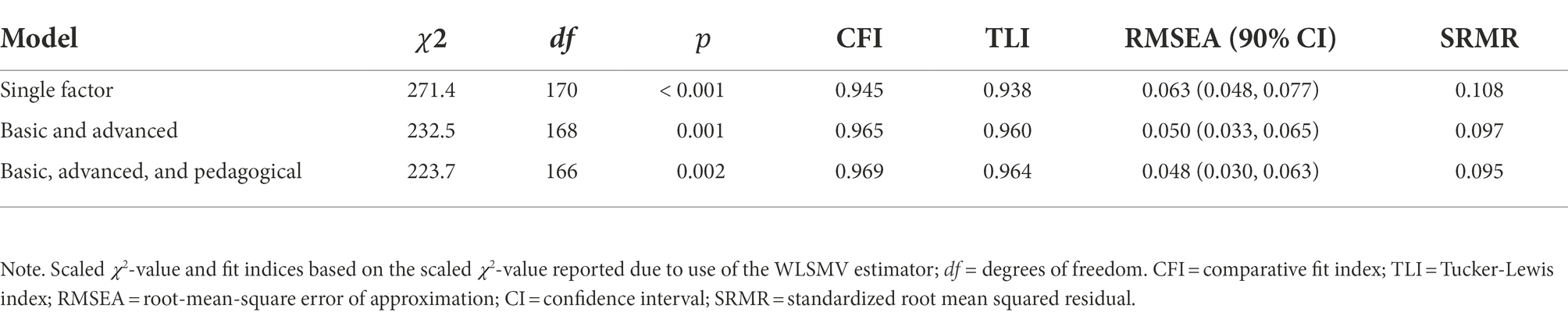
Table 3. Fit indices different numbers of factors in a CFA (task and software related statements, 𝑁 = 152).
Nomological network
Regarding the nomological network, we estimated a joint model containing six factors from preceding analyses, one error correlation, and correlations between all factors. The model showed acceptable fit (𝜒2(448) = 596.0, 𝑝 < 0.001; CFI = 0.960; TLI = 0.956; RMSEA = 0.045, 90% CI [0.035; 0.054]; SRMR = 0.091). Figure 2 depicts correlations between latent variables. All correlations were significant at the 0.05 level, ranging between 𝑟TK TPK = 0.17 and 𝑟S.E.Adv S.E.Ped = 0.85. Factors resembling self-efficacy style items yielded high correlations among themselves and with the PS factor. Furthermore, the PS factor was highly correlated with the TK factor (𝑟TK PS = 0.74).
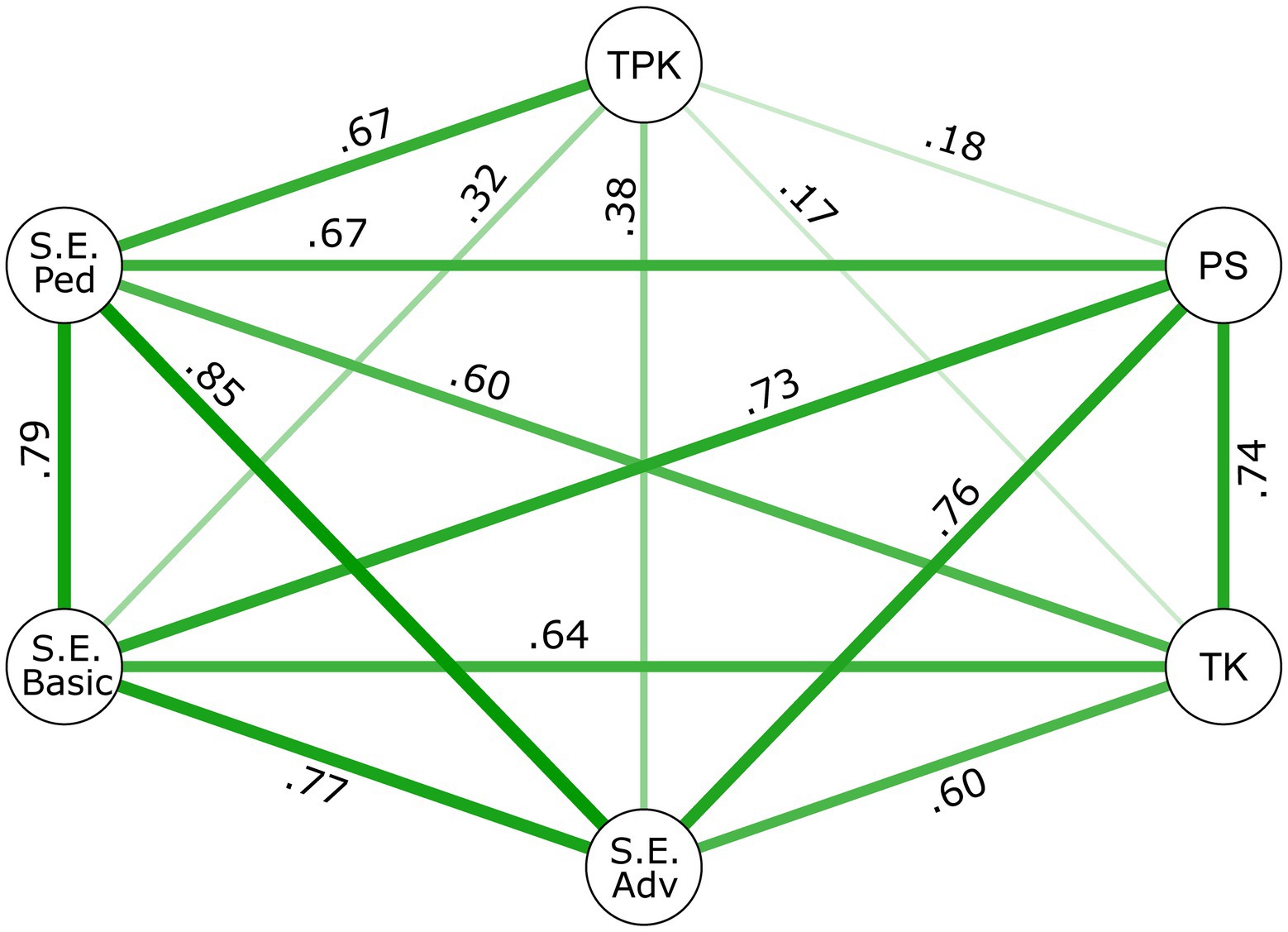
Figure 2. Associations between the latent variables. The widths of the lines indicate the strength of the correlations. All correlations are significant (p < 0.05, N = 165).
Gender
We included gender as a predictor for all six factors (Figure 3). We excluded a single person stating gender as non-binary. The model showed acceptable fit (𝜒2(474) = 619.6, 𝑝 < 0.001; CFI = 0.960; TLI = 0.958; RMSEA = 0.043, 90% CI [0.033;0.053]; SRMR = 0.094). All regression coefficients were positive, indicating higher values on latent factors for male participants. However, only the regression coefficients on TK, PS, and advanced self-efficacy were significant at the α-level of 0.05. Especially, regression coefficients for both factors comprising pedagogical aspects were close to zero.
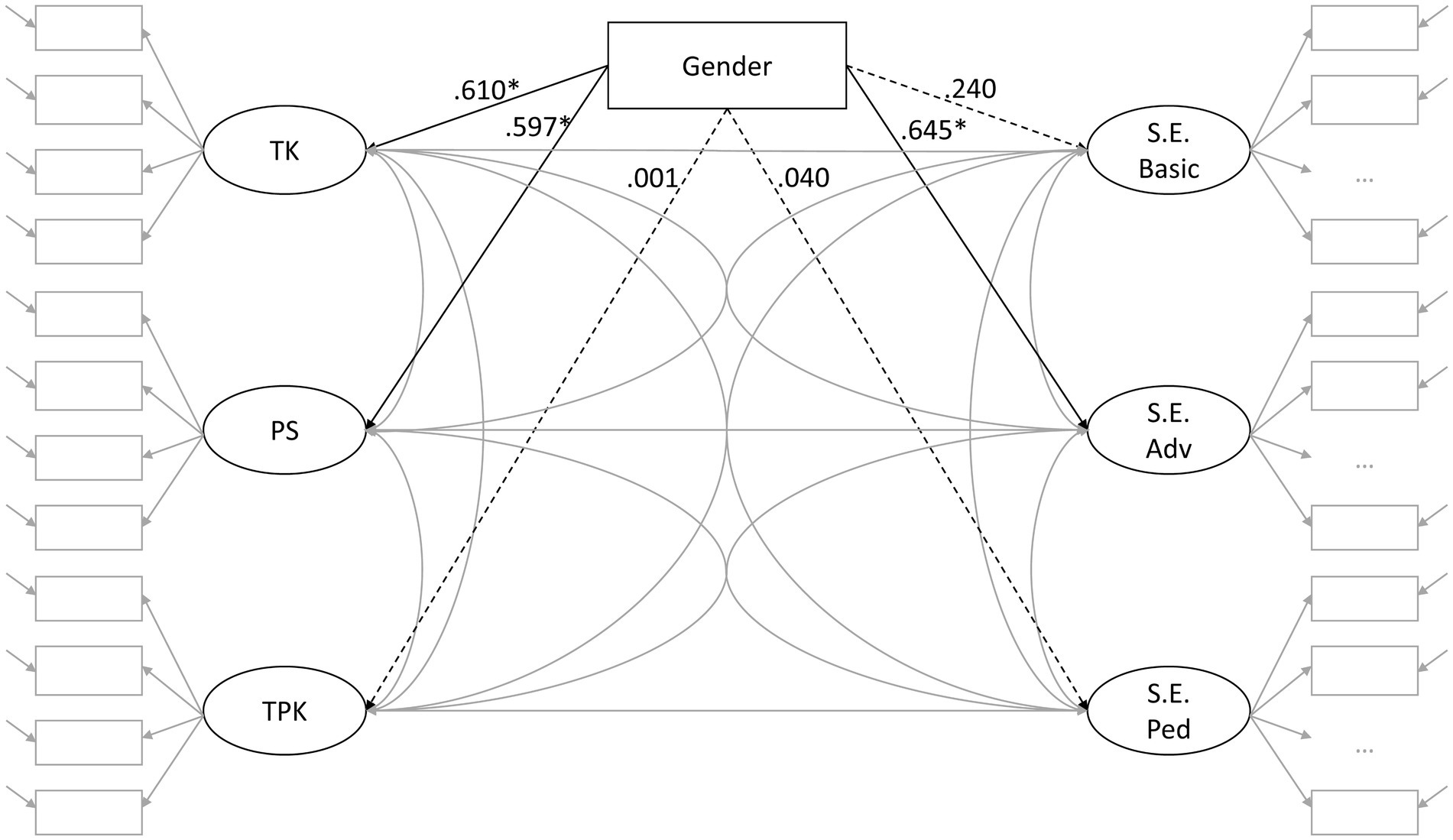
Figure 3. Standardized regression coefficients for gender (1: female, 2: male; *: significant at 𝛼 = 0.05, N = 164).
Discussion
Summary and interpretation of results
As self-report measures on ICT competencies are frequently used, knowledge of underlying factor structures and other properties of these instruments is important for selecting an appropriate questionnaire and interpreting its results. In terms of factor structures for non-subject-specific ICT self-efficacy, the results presented above suggest that instructional vs. non-instructional items should be considered as belonging to separate factors, and propose two possibilities how the non-instructional items could be further subdivided. This underlines the claims to focus on this factor in order to unlock its meaning (Scherer et al., 2017; Willermark, 2018). However, as allocation of these items differed between both sets of scales when viewed from the point of view of item formulations and properties, sensible division criteria represent a topic for further research, too. In the following, we first describe and discuss individual results following the research questions. Afterwards, we discuss limitations and shortcomings of the study on a general level.
Regarding the first research question on the items from the TPACK tradition and similar items (Schmidt et al., 2009; OECD, 2017; Schmid et al., 2020), fit indices presented in “TPACK items” and Table 2 favored a model with three factors (see also Model C1 in Figure 1): (1) The TK factor captures attitudes to and interest in technology. (2) The PS factor comprises items on solving technical problems including installing software, similar to Archambault and Barnett (2010) who found a factor with items on troubleshooting technical devices. (3) The TPK factor contains items with additional instructional content. Table 1 gives additional information on the obtained factors: All factors consist of four rather generally formulated items and show good reliabilities, except the TK factor, which resembles a rather heterogeneous construct. The range of scale means is quite narrow within 3.5–3.8, and students tended to select the fourth answer category, but rarely the first one. Overall, participants reported fairly high scores on these scales. This contrasts with the usually low test scores of this group of students in ICT performance tests (Senkbeil et al., 2021). Overestimation of ICT competencies in self-reports is quite common: For example, Tomczyk (2021) reports differences between objective and self-reported ICT competency measures within a sample. Differences between actual and self-reported competencies have been attributed to shortcomings of self-report measures, for example due to the Dunning–Kruger effect or response biases. The Dunning–Kruger effect describes the phenomenon that incompetent persons tend to overestimate their knowledge and abilities (see Mahmood, 2016 for a review on the Dunning–Kruger effect within information literacy; and Dunning, 2011 for a general explanation of this effect). Alternative explanations of these results include response bias, such as acquiescence bias, or simply the preference of certain answer categories (Kalton and Schuman, 1982; Knowles and Nathan, 1997; Podsakoff et al., 2003). Additionally assessments on global scales formulated in a generic manner tend to be higher (Ackerman et al., 2002). This becomes apparent in the current study when comparing the mean scores of the TPACK self-report scales with the results from the self-efficacy scales described next.
Regarding the second research question and items following the self-efficacy tradition (van Deursen et al., 2014; Gerick et al., 2018), results also favored a model with three factors (see “Self-efficacy items” and Table 3): (1) A first factor S.E. Basic consisting of items on everyday tasks, (2) a second factor S.E. Advanced with items on more complex technical tasks, and (3) an instructional factor (see Scherer and Siddiq, 2015, for similar results). See Model C2 in Figure 1 for a graphical representation of this model. According to Table 1, reliabilities for the self-efficacy scales tended to be lower than for the TPACK self-report scales, probably because most participants did not feel equally confident in all tasks. Compared to prior empirical results on these scales, reliability of the S.E. Basic scale is in the range reported in Fraillon et al. (2014), but lower than the reliabilities of the other two self-efficacy scales, although two items were added from van Deursen et al. (2014). An explanation is that most participants were quite confident in mastering these tasks, leading to a ceiling effect, and reducing variance in the items. Reliability of the S.E. Advanced scale is within the range reported by Fraillon et al. (2014). Regarding S.E. Pedagogical, the low reliability could be enhanced by adding further items, for example from the ICILS teacher questionnaire or similar self-efficacy scales for teachers (Scherer and Siddiq, 2015; Tzafilkou et al., 2021). In comparison to the TPACK self-report scales, the means of the resulting self-efficacy scales differed more, while standard deviations of the scales were lower. Particularly with regard to the advanced tasks, the participants were unsure whether they could manage them without additional effort. This may indicate that difficulty of these items resembles difficulty of tasks that serve as some kind of reference point during the answering process. Hopefully, due to the more specific item formulations, there is less room for interpretation of items (Podsakoff et al., 2003; Groves et al., 2009). As a consequence, response bias (Kalton and Schuman, 1982; Knowles and Nathan, 1997) and effects like the Dunning-Kruger effect (Dunning, 2011) could be mitigated.
A joint model of the two previously discussed three factor models addresses the third research question. According to Marsh et al. (2005) it shows an acceptable model fit. The correlation patterns of the nomological network in Figure 2 give insight into construct validity of the factors. In general, the correlations across instruments were in the range reported by Kul et al. (2019) and Abu Bakar et al. (2020). We found the highest correlations within self-efficacy style scales probably due to the common response scale and behavior-oriented phrases. Regarding the purely technological factors, the S.E. Advanced and the S.E. Basic scales also showed slightly higher correlations with the PS scale than with the TK scale. A possible explanation could be that the items contained in the PS scale are more behavior-oriented, and thus more similar to the S.E. items. On the other hand, the items of the TK scale are more attitude-oriented (see Appendix A for wording of the items). Within the TPACK self-report scales, correlations were considerably higher between the non-instructional factors. Surprisingly, correlations of these non-instructional factors with the instructional TPK factor were quite low, especially when compared to existing findings (e.g., Schmid et al., 2020). An explanation, similar to Valtonen et al. (2017), could be that participants are not aware of the possible impact of technological competencies on teaching with digital media. However, the TPK factor shows high correlations with the instructional self-efficacy scale, thus supporting its meaning. This is also in line with our hypothesis on the importance of content overlap for the correlational structure. Findings on the response format are mixed and confounded with behavior-oriented formulated items. In further research, content and response scales could be altered in a systematic manner in order to investigate the effects of each one. Additionally, including one or several more attitude related scales or scenario based assessments might yield further insights (Krauskopf and Forssell, 2018; Sailer et al., 2021).
Finally, the last model addressing the fourth research question on gender differences (see “Gender” and Figure 3) showed acceptable model fit according to Marsh et al. (2005). The results revealed significant gender differences on purely technological factors, with male pre-service teachers reporting higher values, except the factor comprising the simple tasks (S.E. Basic). This finding is in line with previous studies (Scherer and Siddiq, 2015; Scherer et al., 2017; Gebhardt, 2019). For example in the ICILS-study, there were no significant gender differences on the scale capturing basic ICT self-efficacy (Fraillon et al., 2014; Gebhardt, 2019). In contrast, Scherer and Siddiq (2015) did find gender differences favoring men when using tasks that were slightly more difficult. Thus, content of the tasks may be crucial when looking at gender differences. Looking at the data from the current study, it seems plausible that a ceiling effect also prevents gender differences on this scale.
For instructional factors, regression coefficients indicating gender differences were close to zero. These results are comparable to existing findings from both research traditions (Scherer and Siddiq, 2015; Scherer et al., 2017; Abu Bakar et al., 2020). As female pre-service teachers seem to be more comfortable using technology in an instructional context, this could be also a starting point for developing courses on technology use for this target group (for more suggestions for gender-sensitive ICT lessons, see the discussion of Tzafilkou et al., 2021). This is similar to the call by Mishra and Koehler (2006) to teach technology in a project style manner that automatically includes pedagogical and content-specific aspects.
Limitations and further research
A major restriction of the study is the small and specific sample with a high proportion of female participants, as only students from one university took part. In addition, participation was voluntary, which could also lead to a biased sample. A more diverse sample with pre-service teachers from several universities as well as in-service teachers might add variance to the answers and improve reliabilities as well as transferability of the results. Furthermore, data from teachers-in-training who have differentiated practical experience teaching with digital media could lead to a different structure. For larger samples, multi-group models and tests of measurement invariance between gender groups would have been possible, as well as more complex models. By using further scales or even performance tests, additional insights into the nomological network would be possible(see the multitrait-multimethod approach described in Campbell and Fiske, 1959). We started from two existing instruments guided by previous empirical findings. Starting from theoretical models (e.g., Ferrari, 2013; Punie and Redecker, 2017; Vuorikari et al., 2022) and adding or developing suitable scales could be an alternative to developing a framework that is both theoretically reasonable and empirically sound (see Rubach and Lazarides, 2019 for an example). Additionally to gender, predictors such as professional experience may affect the underlying network. As participants of the present study were still in university, it would be interesting to investigate how the nomological network develops during professional life and expertise development, for example in longitudinal studies (Valtonen et al., 2019).
Nevertheless, this study empirically compares instruments and bridges research traditions within digital competencies in schools and teacher education. This is a first step to a comprehensive empirical network of ICT-related constructs. Furthermore, in line with other studies, our findings suggest that different aspects might affect correlations between scales These comprise face content like behavior vs. attitude orientation (Scherer et al., 2018; Sailer et al., 2021) and concrete vs. generic tasks, but also item characteristics like difficulty (i.e., of the tasks) and the response scale used (DeCastellarnau, 2018). Beyond that, alignment and validity of measures are related topics relevant for scale construction. Concerning alignment, Rohatgi et al. (2016) and Gebhardt (2019) found moderate to high correlations in the ICILS study between self-reported self-efficacy for basic skills and test performances for students within gender groups in different samples. However, the correlation between self-efficacy for advanced tasks and the performance test was negative. Rohatgi et al. (2016) argue that this could be due to a missing alignment, as the performance test consisted of rather simple, everyday tasks. Alignment of the questionnaire may also become relevant when choosing a suitable instrument for research or evaluation purposes. By following the tradition of self-efficacy (Bandura, 2006), developing and using domain specific questionnaires fitting with the study’s purpose may enable to draw conclusions that are more specific and perhaps even allow gaining results that are closer to testing results, despite still showing the advantages of self-report measures like being economic and capturing motivational aspects. However, in a similar manner, as ICT will change in future, these scales should be updated to emerging technologies regularly. Another question would be whether more specific items and answer scales may circumvent problems with longitudinal use of these scales showing fluctuations in confidence rather than progress in skills and competencies across the course of studies (Mouza et al., 2017; Rienties et al., 2020; Weß et al., 2020).
Conclusion
Based on two sets of (1) non-subject-specific TPACK self-report items and (2) corresponding self-efficacy items on ICT use, we investigated factor structures, relationships within and between instruments, and gender differences on the resulting scales. For both lines of research, our results suggest that instructional and non-instructional facets should be distinguished, and propose possibilities, how non-instructional items can be subdivided into factors. As we found different possible subdivision of these items, a topic for further research is to investigate sensible distinctions of non-instructional items. To accomplish this, and in addition to theoretical considerations, our results revealed that both item content and item characteristics (e.g., item difficulties) are important aspects researchers should consider when constructing scales. Regarding planning studies or using evaluation instruments within the emerging field of assessing teachers’ digital competencies, task-oriented scales may be an alternative to rather general TPACK self-report scales. According to our results, task-oriented scales may require more items to achieve adequate reliabilitie, but might also be able to draw a more accurate picture of the participants as we observed a more pronounced answer behavior. Further correlations to corresponding general TPACK self-report scales were high. Thus, these scales may present an alternative to generic self-reports on ICT competencies that are tailored to specific interventions.
Data availability statement
The raw data supporting the conclusions of this article are available from the corresponding author upon request. Lavaan input and output for the model containing gender are provided in Appendix B.
Ethics statement
Ethical review and approval was not required for the study in human participants according to local legislation and institutional requirements. Participants were digitally informed about and consented to the study and associated privacy policies.
Author contributions
SH: data curation, data analysis, writing and revising of the draft (lead). AP: data curation, data analysis, and writing and revising of the draft. OK-H: supervision, project administration, writing and revising of the draft, and funding acquisition. All authors contributed to the article and approved the submitted version.
Funding
This project is part of the “QualitätsoffensiveLehrerbildung”, a joint initiative of the Federal Government and the Länder which aims to improve the quality of teacher training. The programme is funded by the Federal Ministry of Education and Research (grant number 01JA2027). The authors are responsible for the content of this publication. The article processing charge was funded by the Baden-Württemberg Ministry of Science, Research and Culture and the University of Education Karlsruhe in the funding programme Open Access Publishing.
Acknowledgments
We thank the students as well as the lecturers for their participation in and support of the study.
Conflict of interest
The authors declare that the research was conducted in the absence of any commercial or financial relationships that could be construed as a potential conflict of interest.
Publisher’s note
All claims expressed in this article are solely those of the authors and do not necessarily represent those of their affiliated organizations, or those of the publisher, the editors and the reviewers. Any product that may be evaluated in this article, or claim that may be made by its manufacturer, is not guaranteed or endorsed by the publisher.
Supplementary material
The Supplementary material for this article can be found online at: https://www.frontiersin.org/articles/10.3389/feduc.2022.999679/full#supplementary-material
References
Abu Bakar, N. S., Maat, S. M., and Rosli, R. (2020). Mathematics Teacher’s self-efficacy of technology integration and technological pedagogical content knowledge. J. Math. Educ. 11, 259–276. doi: 10.22342/jme.11.2.10818.259-276
Ackerman, P. L., Beier, M. E., and Bowen, K. R. (2002). What we really know about our abilities and our knowledge. Pers. Individ. Differ. 33, 587–605. doi: 10.1016/S0191-8869(01)00174-X
Archambault, L. M., and Barnett, J. H. (2010). Revisiting technological pedagogical content knowledge: exploring the TPACK framework. Comput. Educ. 55, 1656–1662. doi: 10.1016/j.compedu.2010.07.009
Backfisch, I., Lachner, A., Hische, C., Loose, F., and Scheiter, K. (2020). Professional knowledge or motivation? Investigating the role of teachers’ expertise on the quality of technology-enhanced lesson plans. Learn. Instr. 66:101300. doi: 10.1016/j.learninstruc.2019.101300
Bandura, A. (1977). Self-efficacy: toward a unifying theory of behavioral change. Psychol. Rev. 84, 191–215. doi: 10.1037/0033-295X.84.2.191
Bandura, A. (2006). “Guide for constructing self-efficacy scales” in Self-efficacy Beliefs of Adolescents. eds. F. Pajares and T. Urdan (Greenwich, CT: Information Age Publishing), 307–337.
Campbell, D. T., and Fiske, D. W. (1959). Convergent and discriminant validation by the multitrait-multimethod matrix. Psychol. Bull. 56, 81–105. doi: 10.1037/h0046016
Canbazoğlu Bilici, S., Yamak, H., Kavak, N., and Guzey, S. (2013). Technological pedagogical content knowledge self-efficacy scale (TPACK-SeS) for preservice science teachers: Construction, validation and reliability. Eurasian J. Educ. Res. 37–60.
Chai, C. S., Ng, E. M. W., Li, W., Hong, H.-Y., and Koh, J. H. L. (2013). Validating and modelling technological pedagogical content knowledge framework among Asian preservice teachers. Australas. J. Educ. Technol. 29, 31–51. doi: 10.14742/ajet.174
Cronbach, L. J., and Meehl, P. E. (1955). Construct validity in psychological tests. Psychol. Bull. 52, 281–302. doi: 10.1037/h0040957
Cuban, L. (1986). Teachers and Machines: The Classroom Use of Technology Since 1920. New York, NY: Teachers College Press.
DeCastellarnau, A. (2018). A classification of response scale characteristics that affect data quality: a literature review. Qual. Quant. 52, 1523–1559. doi: 10.1007/s11135-017-0533-4
Dunning, D. (2011). “The Dunning–Kruger Effect” in Advances in Experimental Social Psychology. eds. M. J. Olson and M. P. Zanna (Amsterdam: Elsevier), 247–296.
Durndell, A., and Haag, Z. (2002). Computer self efficacy, computer anxiety, attitudes towards the internet and reported experience with the internet, by gender, in an east European sample. Comput. Hum. Behav. 18, 521–535. doi: 10.1016/S0747-5632(02)00006-7
Falloon, G. (2020). From digital literacy to digital competence: the teacher digital competency (TDC) framework. Educ. Technol. Res. Dev. 68, 2449–2472. doi: 10.1007/s11423-020-09767-4
Ferrari, A. (2013). “DIGCOMP: a framework for developing and understanding digital competence in Europe” in JRC Scientific and Policy Reports, EUR 26035 EN. eds. Y. Punie and B. N. Brečko (Luxembourg: Publications Office of the European Union)
Fraillon, J., Ainley, J., Friedman, T., Gebhardt, E., and Schulz, W. (2014). Preparing for Life in a Digital Age: The IEA International Computer and Information Literacy Study International Report. 1st edn.. Cham: Springer International Publishing.
Fraillon, J., Ainley, J., Schulz, W., Friedman, T., and Duckworth, D. (2020). Preparing for Life in a Digital World: IEA International Computer and Information Literacy Study 2018 International Report. Cham: Springer International Publishing.
Gebhardt, E. (2019). Gender Differences in Computer and Information Literacy. Cham, Switzerland: Springer International Publishing.
Gerick, J., Vennemann, M., Eickelmann, B., Bos, W., Mews, S., and Verlag, W. (2018). ICILS 2013 Dokumentation der Erhebungsinstrumente der International computer and information literacy study [ICILS 2013 documentation of the survey instruments of the international computer and information literacy study.]. Available at: https://nbn-resolving.org/urn:nbn:de:101:1-2018121604490633561253
Groves, R. M., Fowler, F. J., Couper, M. P., Lepowski, J. M., Singer, E., and Tourangeau, R. (2009). Survey Methodology. 2nd ed.. Hoboken, NJ: Wiley.
Hatlevik, O. E., Throndsen, I., Loi, M., and Gudmundsdottir, G. B. (2018). Students’ ICT self-efficacy and computer and information literacy: determinants and relationships. Comput. Educ. 118, 107–119. doi: 10.1016/j.compedu.2017.11.011
Hohlfeld, T. N., Ritzhaupt, A. D., and Barron, A. E. (2013). Are gender differences in perceived and demonstrated technology literacy significant? It depends on the model. Educ. Technol. Res. Dev. 61, 639–663. doi: 10.1007/s11423-013-9304-7
Joo, Y., Park, S., and Lim, E. (2018). Factors influencing preservice teachers’ intention to use technology: TPACK, teacher self-efficacy, and technology acceptance model. Educ. Technol. Soc. 21, 48–59.
Kalton, G., and Schuman, H. (1982). The effect of the question on survey responses: a review. J. R. Stat. Soc. Ser. A (General) 145:42. doi: 10.2307/2981421
Knowles, E. S., and Nathan, K. T. (1997). Acquiescent responding in self-reports: cognitive style or social concern? J. Res. Pers. 31, 293–301. doi: 10.1006/jrpe.1997.2180
Koh, J. H. L., Chai, C. S., and Tsai, C. C. (2010). Examining the technological pedagogical content knowledge of Singapore pre-service teachers with a large-scale survey: Singapore pre-service teachers’ TPACK perceptions. J. Comput. Assist. Learn. 26, 563–573. doi: 10.1111/j.1365-2729.2010.00372.x
Krauskopf, K., and Forssell, K. (2018). When knowing is believing: a multi-trait analysis of self-reported TPCK. J. Comput. Assist. Learn. 34, 482–491. doi: 10.1111/jcal.12253
Kul, U., Aksu, Z., and Birisci, S. (2019). The relationship between technological pedagogical content knowledge and web 2.0 self-efficacy beliefs. Int. Online J. Educ. Sci. 11, 198–213. doi: 10.15345/iojes.2019.01.014
Lachner, A., Fabian, A., Franke, U., Preiß, J., Jacob, L., Führer, C., et al. (2021). Fostering pre-service teachers’ technological pedagogical content knowledge (TPACK): a quasi-experimental field study. Comput. Educ. 174:104304. doi: 10.1016/j.compedu.2021.104304
Lee, M.-H., and Tsai, C.-C. (2010). Exploring teachers’ perceived self efficacy and technological pedagogical content knowledge with respect to educational use of the world wide web. Instr. Sci. 38, 1–21. doi: 10.1007/s11251-008-9075-4
Mahmood, K. (2016). Do people overestimate their information literacy skills? A systematic review of empirical evidence on the Dunning-Kruger effect. Comminfolit 10:199. doi: 10.15760/comminfolit.2016.10.2.24
Marsh, H. W., Hau, K.-T., and Grayson, D. (2005). “Goodness of fit in structural equation models” in Contemporary Psychometrics: A Festschrift for Roderick P. McDonald. eds. A. Maydeu-Olivares and J. J. McArdle (Mahwah, NJ: Lawrence Erlbaum Associates Publishers), 275–340.
Mishra, P., and Koehler, M. J. (2006). Technological pedagogical content knowledge: a framework for teacher knowledge. Teach. Coll. Rec. 108, 1017–1054. doi: 10.1111/j.1467-9620.2006.00684.x
Mouza, C., Nandakumar, R., Ozden, S. Y., and Karchmer-Klein, R. (2017). A longitudinal examination of preservice teachers’ technological pedagogical content knowledge in the context of undergraduate teacher education. Action Teach. Educ. 39, 153–171. doi: 10.1080/01626620.2016.1248301
OECD. (2017). “ICT familiarity questionnaire for PISA 2018.” Available at: https://www.oecd.org/pisa/data/2018database/CY7_201710_QST_MS_ICQ_NoNotes_final.pdf
Podsakoff, P. M., MacKenzie, S. B., Lee, J.-Y., and Podsakoff, N. P. (2003). Common method biases in behavioral research: a critical review of the literature and recommended remedies. J. Appl. Psychol. 88, 879–903. doi: 10.1037/0021-9010.88.5.879
Preckel, F., and Brunner, M. (2017). “Nomological Nets” in Encyclopedia of Personality and Individual Differences. eds. V. Zeigler-Hill and T. K. Shackelford (Cham: Springer International Publishing), 1–4.
Pumptow, M., and Brahm, T. (2021). Students’ digital media self-efficacy and its importance for higher education institutions: development and validation of a survey instrument. Technol. Knowl. Learn. 26, 555–575. doi: 10.1007/s10758-020-09463-5
Punie, Y., and Redecker, C. (eds.) (2017). European Framework for the Digital Competence of Educators: DigCompEdu. Luxembourg: Publications Office of the European Union.
R Core Team. (2021). “R: A Language and Environment for Statistical Computing.” Vienna: R Foundation for Statistical Computing.
Rahmadi, I. F., Hayati, E., and Nursyifa, A. (2020). Comparing pre-service civic education teachers’ TPACK confidence across course modes. Res. Soc. Sci. Technol. 5, 113–133. doi: 10.46303/ressat.05.02.7
Reddy, P., Chaudhary, K., Sharma, B., and Chand, R. (2021). The two perfect scorers for technology acceptance. Educ. Inf. Technol. 26, 1505–1526. doi: 10.1007/s10639-020-10320-2
Richter, T., Naumann, J., and Groeben, N. (2001). Das Inventar Zur Computerbildung (INCOBI): Ein instrument Zur Erfassung von Computer literacy und Computerbezogenen Einstellungen Bei Studierenden Der Geistes-und Sozialwissenschaften [the inventory of computer literacy (INCOBI): an instrument to assess computer literacy and computer-related attitudes among students in the humanities and social sciences]. Psychol. Erzieh. Unterr. 48, 1–13.
Rienties, B., Lewis, T., O’Dowd, R., Rets, I., and Rogaten, J. (2020). The impact of virtual exchange on TPACK and foreign language competence: Reviewing a large-scale implementation across 23 virtual exchanges. Comput. Assist. Lang. Learn. 35, 1–27. doi: 10.1080/09588221.2020.1737546
Rohatgi, A., Scherer, R., and Hatlevik, O. E. (2016). The role of ICT self-efficacy for students’ ICT use and their achievement in a computer and information literacy test. Comput. Educ. 102, 103–116. doi: 10.1016/j.compedu.2016.08.001
Rosseel, Y. (2012). Lavaan: an R package for structural equation modeling. J. Stat. Softw. 48, 1–36. doi: 10.18637/jss.v048.i02
Rubach, C., and Lazarides, R. (2019). Eine Skala zur Selbsteinschätzung digitaler Kompetenzen bei Lehramtsstudierenden: Entwicklung eines Instrumentes und die Validierung durch Konstrukte zur Mediennutzung und Werteüberzeugungen zur Nutzung digitaler Medien im Unterricht [A Digital Literacy Self-Assessment Scale for Student Teachers: Development of an Instrument and Validation Through Constructs on Media Use and Value Beliefs on the Use of Digital Media in the Classroom]. Z. Bild. 9, 345–374. doi: 10.1007/s35834-019-00248-0
Sailer, M., Stadler, M., Schultz-Pernice, F., Franke, U., Schöffmann, C., Paniotova, V., et al. (2021). Technology-related teaching skills and attitudes: validation of a scenario-based self-assessment instrument for teachers. Comput. Hum. Behav. 115:106625. doi: 10.1016/j.chb.2020.106625
Scherer, R., Howard, S. K., Tondeur, J., and Siddiq, F. (2021). Profiling teachers’ readiness for online teaching and learning in higher education: Who’s ready? Comput. Hum. Behav. 118:106675. doi: 10.1016/j.chb.2020.106675
Scherer, R., and Siddiq, F. (2015). Revisiting teachers’ computer self-efficacy: a differentiated view on gender differences. Comput. Hum. Behav. 53, 48–57. doi: 10.1016/j.chb.2015.06.038
Scherer, R., Tondeur, J., and Siddiq, F. (2017). On the quest for validity: testing the factor structure and measurement invariance of the technology-dimensions in the technological, pedagogical, and content knowledge (TPACK) model. Comput. Educ. 112, 1–17. doi: 10.1016/j.compedu.2017.04.012
Scherer, R., Tondeur, J., Siddiq, F., and Baran, E. (2018). The importance of attitudes toward Technology for pre-Service Teachers’ technological, pedagogical, and content knowledge: comparing structural equation modeling approaches. Comput. Hum. Behav. 80, 67–80. doi: 10.1016/j.chb.2017.11.003
Schmid, M., Brianza, E., and Petko, D. (2020). Developing a short assessment instrument for technological pedagogical content knowledge (TPACK.Xs) and comparing the factor structure of an integrative and a transformative model. Comput. Educ. 157:103967. doi: 10.1016/j.compedu.2020.103967
Schmidt, D. A., Baran, E., Thompson, A. D., Mishra, P., Koehler, M. J., and Shin, T. S. (2009). Technological pedagogical content knowledge (TPACK). J. Res. Technol. Educ. 42, 123–149. doi: 10.1080/15391523.2009.10782544
Senkbeil, M., Ihme, J. M., and Schöber, C. (2021). Schulische Medienkompetenzförderung in Einer Digitalen Welt: Über Welche Digitalen Kompetenzen Verfügen Angehende Lehrkräfte? [Promoting Media Literacy in Schools in a Digital World: What Digital Competencies Do Prospective Teachers Have?]. Psychol. Erzieh. Unterr. 68, 4–22. doi: 10.2378/peu2020.art12d
Seufert, S., Guggemos, J., and Sailer, M. (2021). Technology-related knowledge, skills, and attitudes of pre- and in-service teachers: the current situation and emerging trends. Comput. Hum. Behav. 115:106552. doi: 10.1016/j.chb.2020.106552
Shulman, L. (1987). Knowledge and teaching: foundations of the new reform. Harv. Educ. Rev. 57, 1–23. doi: 10.17763/haer.57.1.j463w79r56455411
Siddiq, F., Hatlevik, O. E., Olsen, R. V., Throndsen, I., and Scherer, R. (2016). Taking a future perspective by learning from the past – a systematic review of assessment instruments that aim to measure primary and secondary school students’ ICT literacy. Educ. Res. Rev. 19, 58–84. doi: 10.1016/j.edurev.2016.05.002
Sieverding, M., and Koch, S. C. (2009). (Self-)evaluation of computer competence: how gender matters. Comput. Educ. 52, 696–701. doi: 10.1016/j.compedu.2008.11.016
Tomczyk, Ł. (2021). Declared and real level of digital skills of future teaching staff. Educ. Sci. 11:619. doi: 10.3390/educsci11100619
Tschannen-Moran, M., and Hoy, A. W. (2001). Teacher efficacy: capturing an elusive construct. Teach. Teach. Educ. 17, 783–805. doi: 10.1016/S0742-051X(01)00036-1
Tzafilkou, K., Perifanou, M. A., and Economides, A. A. (2021). Teachers’ trainers’ intention and motivation to transfer ICT training: the role of ICT individual factors, gender, and ICT self-efficacy. Educ. Inf. Technol. 26, 5563–5589. doi: 10.1007/s10639-021-10541-z
Valtonen, T., Sointu, E., Kukkonen, J., Kontkanen, S., Lambert, M. C., and Mäkitalo-Siegl, K. (2017). TPACK updated to measure pre-service teachers’ twenty-first century skills. Australas. J. Educ. Technol. 33, 15–31. doi: 10.14742/ajet.3518
Valtonen, T., Sointu, E., Kukkonen, J., Mäkitalo, K., Hoang, N., Häkkinen, P., et al. (2019). Examining pre-service teachers’ technological pedagogical content knowledge as evolving knowledge domains: a longitudinal approach. J. Comput. Assist. Learn. 35, 491–502. doi: 10.1111/jcal.12353
Valtonen, T., Sointu, E. T., Mäkitalo-Siegl, K., and Kukkonen, J. (2015). Developing a TPACK measurement instrument for 21st century pre-service teachers. Seminar.Net 11, 87–100. doi: 10.7577/seminar.2353
van Deursen, A., Helsper, E. J., and Eynon, R. (2014). Measuring Digital Skills: From Digital Skills to Tangible Outcomes [Project Report]. Enschede: University of Twente.
Venkatesh, V., and Davis, F. D. (2000). A theoretical extension of the technology acceptance model: four longitudinal field studies. Manag. Sci. 46, 186–204. doi: 10.1287/mnsc.46.2.186.11926
Voogt, J., Fisser, P., Pareja Roblin, N., Tondeur, J., and van Braak, J. (2013). Technological pedagogical content knowledge–a review of the literature. J. Comput. Assist. Learn. 29, 109–121. doi: 10.1111/j.1365-2729.2012.00487.x
Vuorikari, R., Kluzer, S., and Punie, Y. (2022). DigComp 2.2: the Digital Competence Framework for Citizens–With New Examples of Knowledge, Skills and Attitudes. EUR 31006 EN. Luxembourgh: Publications Office of the European Union.
Wang, L., Ertmer, P. A., and Newby, T. J. (2004). Increasing preservice teachers’ self-efficacy beliefs for technology integration. J. Res. Technol. Educ. 36, 231–250. doi: 10.1080/15391523.2004.10782414
Wang, W., Schmidt-Crawford, D., and Jin, Y. (2018). Preservice teachers’ TPACK development: a review of literature. J. Digital Learn. Teach. Educ. 34, 234–258. doi: 10.1080/21532974.2018.1498039
Weß, R., Priemer, B., Weusmann, B., Ludwig, T., Sorge, S., and Neumann, I. (2020). Der Verlauf von lehrbezogenen Selbstwirksamkeitserwartungen angehender MINT-Lehrkräfte im Studium [The Trajectory of Teaching-Related Self-Efficacy Expectations of Undergraduate STEM Teachers]. Zeitschrift für Pädagogische Psychologie 34, 221–238. doi: 10.1024/1010-0652/a000272
Willermark, S. (2018). Technological pedagogical and content knowledge: a review of empirical studies published from 2011 to 2016. J. Educ. Comput. Res. 56, 315–343. doi: 10.1177/0735633117713114
Keywords: TPACK, ICT self-efficacy, information and communication technology, self-efficacy, pre-service teachers, factorial structure, gender differences
Citation: Hahn S, Pfeifer A and Kunina-Habenicht O (2022) Multiple facets of self-rated digital competencies of pre-service teachers: A pilot study on the nomological network, empirical structure, and gender differences. Front. Educ. 7:999679. doi: 10.3389/feduc.2022.999679
Edited by:
Meryem Yilmaz Soylu, Georgia Institute of Technology, United StatesReviewed by:
Iris Backfisch, University of Tübingen, GermanyŁukasz Tomczyk, Jagiellonian University, Poland
Copyright © 2022 Hahn, Pfeifer and Kunina-Habenicht. This is an open-access article distributed under the terms of the Creative Commons Attribution License (CC BY). The use, distribution or reproduction in other forums is permitted, provided the original author(s) and the copyright owner(s) are credited and that the original publication in this journal is cited, in accordance with accepted academic practice. No use, distribution or reproduction is permitted which does not comply with these terms.
*Correspondence: Sonja Hahn, c29uamEuaGFobkBwaC1rYXJsc3J1aGUuZGU=