- 1Department of Psychology, Center for Intervention Research in Schools, Ohio University, Athens, OH, United States
- 2Reid Software, Athens, OH, United States
Technology-based supports offer promise for helping elementary school teachers implement Tier 2 interventions to address challenging student behavior. The Daily Report Card Online (DRCO) platform is a cloud-based web application designed to support teachers’ adoption and implementation of a high-quality daily report card (DRC) intervention through the use of professional development resources, guided intervention design workflows, algorithm-based decision-making tools, and real-time progress monitoring. We examined teacher adoption, adaptation, and implementation of a DRC intervention when using the DRCO platform with support from a consultant during the 2021–2022 school year. Participants were 29 teachers, 20 of whom used the DRCO to implement a DRC with a student (n = 20). The most frequently chosen target behaviors were student interruptions, non-compliance, and work completion. When using the DRCO platform, teachers achieved several procedures that align with evidence-based guidelines (e.g., screening, baseline tracking, setting achievable goals, tracking behaviors over time). However, goal criterion changes and shaping procedures were used less often than expected. Despite the option to track behaviors solely with technology, 60% of teachers tracked student behaviors via paper methods (e.g., printed the DRC card, used sticky notes). Adaptations were made by 40% teachers; however, all adaptations involved modifying printed materials to be more student-friendly (e.g., add clipart to the DRC) and did not change the guiding principles of the intervention. Tau-effect sizes for academic and behavioral target behaviors on the DRC showed small to moderate change over time and change in target behaviors showed some association with change in global teacher ratings. Lastly, we identified associations between teacher characteristics and adoption and implementation, as well as associations between implementation and student outcomes.
Introduction
Public school teachers have one of the most stressful jobs in the country (Johnson et al., 2005; Richards, 2012), and this has been exacerbated by the global COVID-19 pandemic (National Education Association, 2022). One of the most stressful aspects of teaching elementary school students is managing student social, emotional, and behavioral challenges (e.g., Greene et al., 2002; Corbin et al., 2019). Although there are evidence-based classroom strategies and interventions to address challenging student behavior (e.g., McLeod et al., 2017; Collier-Meek et al., 2019a; Owens et al., 2021), limited time and access to professional development (e.g., Collier-Meek et al., 2019b), insufficient skills in problem solving and data-driven decision making (e.g., Farley-Ripple and Buttram, 2015), and inadequate implementation supports and accountability (e.g., Long et al., 2016) are barriers to teachers adopting and implementing such interventions. Given the negative teacher and student outcomes associated with teacher stress (Hoglund et al., 2015; Arens and Morin, 2016; Oberle and Schonert-Reichl, 2016; Larson et al., 2018), finding tools to support teachers’ implementation of interventions to address challenging student behavior are needed.
Technological supports offer the promise of achieving this goal. The Daily Report Card-Online system (DRCO1) was co-developed with educators to address many of the above-described barriers. DRCO is a cloud-based web application designed to support teachers’ adoption and implementation of high-quality classroom interventions through the use of professional development resources, guided intervention design workflows, algorithm-based decision-making tools, and real-time progress monitoring. In previous studies, we examined rates of adoption, teacher factors associated with adoption, and student outcomes (Mixon et al., 2019; Owens et al., 2019). In this study, we leverage the data from 29 teachers during the 2021–2022 academic year to further explore nuances about the adoption, implementation, and adaptation processes to inform future expansion of this and other similar technologies. Using the Reach, Effectiveness, Adoption, Implementation, and Maintenance (RE-AIM) framework (Glasgow et al., 2019), we explore teachers’ use of the interactive wizards and decision-making tools; describe adaptations teachers made to assist with fit and feasibility; assess student outcomes using single-subject effect sizes representing change in target behaviors; and examine the association between student outcomes, teacher characteristics, and the above-described features.
Demands on teachers in the current context
Following school closures associated with the COVID-19 pandemic, teachers are facing unprecedented job demands. Some students lost 6–9 months of in person instruction (United Nations Educational, Scientific, and Cultural Organization, 2021), likely influencing academic achievement, and the social, emotional, and behavioral development of many children. Some kindergarten and first grade students missed out on a year of structured and scaffolded social and adaptive skills development (National Center for Education Statistics, 2022a). Many children also either witnessed mental health challenges in their parents or experienced challenges themselves (Institute of Education Sciences [IES], 2022). Thus, as teachers returned to school after school closures, they faced the demands of helping students get back on track academically, socially, emotionally, behaviorally; finding ways to re-establish home-school connections; and conducting their job amidst a political landscape where education was under attack and new policies created contentious debates about what can and cannot be said or taught in public schools (e.g., Zurcher, 2021; Field, 2022). It is important to investigate teachers’ attempts to address challenging student behavior in this context to better understand how to best support teachers and students through this unstable time.
Evidence-based classroom interventions
In many schools, teachers and support staff promote student social and behavioral success via a multi-tiered system of support (MTSS). Within this framework, teachers foster student success by establishing a predictable, positive, and safe school and classroom climate. At Tier 1, teachers foster success by implementing universal school-wide procedures for (a) teaching, acknowledging, and reinforcing positive behavioral expectations and (b) applying effective consequences that prevent escalation of challenging behavior, redirect it to expected behavior, and minimize inadvertent rewarding of such behavior (Nisar et al., 2022). Such strategies are effective in preventing and managing challenging behavior for the majority of students (Bradshaw et al., 2012; Pas et al., 2019). However, a subset of students needs a higher level of support (i.e., Tier 2 or 3) to succeed in school.
When a student is persistently struggling to meet classroom expectations, teachers can work with behavioral support staff to develop an individualized Tier 2 intervention (e.g., daily report card, check-in/check-out program) to help the student be successful in the classroom. When implementing a Tier 2 intervention, it is recommended that teachers and behavioral support staff use screening data to determine which students may need a targeted intervention, narrow the problems to a manageable set of target behaviors, and develop specific and achievable goals to shape behavior into the typical range over time (Center on PBIS, 2022). Many classroom-based Tier 2 interventions involve the use of antecedent-based interventions (e.g., review of expectations, goal setting) and use of consequence-based interventions (e.g., labeled praise, rewards, or loss of privileges), as both are effective in shaping challenging student behavior in elementary classrooms (e.g., Staff et al., 2021). To maximize the success of these interventions, teachers and support staff should also use data to make informed decisions about the effectiveness of the plan over time (Center on PBIS, 2022); however, this can be challenging if procedures to aid in data-driven decision making are not standard practice or user-friendly.
One specific Tier 2 intervention that has been well-studied and found to be effective for reducing challenging behavior and improving student success is the daily report card (DRC) [see Owens et al. (2020) for review]. When using a DRC, the teacher and student agree upon clearly defined target behaviors (e.g., respectful behavior, work completion) and set goals for daily success that are shaped over time as the student makes progress. It is recommended that the teachers track the target behaviors for 3–5 days before implementing the intervention to obtain a baseline sample. Baseline data can help teachers establish initial goal criterion that are achievable goals for each behavior (e.g., completes 50% of daily math assignments, respects others with seven or fewer violations). The teacher is encouraged to review the goals with the student at the start of the day, give feedback to the student at the point of performance throughout the day, review success at the end of the day, and send a report home to caregivers. Following shaping procedures, the goals for each target behavior are modified until the student’s behavior falls in the normative range. New goals can be added as previous goals are mastered.
Meta-analyses of single case design studies (Vannest et al., 2010; Pyle and Fabiano, 2017) and randomized group trials (Fabiano et al., 2010) evaluating the DRC intervention document that it is effective in reducing a wide variety of challenging behaviors and improving academic and classroom functioning. Two studies document benchmarks that can be expected within the first 2 months of intervention (i.e., moderate to large change in disruptive behavior; small change in academic performance), and others document the feasibility and acceptability of the intervention by teachers (Owens et al., 2008; Girio and Owens, 2009). Given this level of evidence, our team undertook a systematic approach to identify key components of the intervention and key skills needed to implement the intervention, then developed an interactive technology-based platform to enhance teachers’ access to professional development and implementation supports.
Daily report card online program
The Daily Report Card Online (DRCO) Program was developed in collaboration with teachers and school behavioral support staff prior to the COVID-19 pandemic. It was designed to supports teachers’ use of a DRC intervention and address several barriers to adoption and implementation. To overcome barriers to professional development training, the DRCO offers information and materials needed to understand important components of a DRC intervention, including video models of implementation. Teachers can access this content at their preferred time and pace. To address barriers related to obtaining guidance and support to implement a high-quality DRC individualized to the student’s needs (Long et al., 2016), the DRCO includes a screening process and an interactive wizard that guides teachers through a development process of selecting and prioritizing target behaviors, collecting baseline data about those behaviors, and using the baseline data to set individualized student goals. Once the DRC is created, teachers can choose to print blank cards each day for tracking and sending home, or they can track behavior in real time using the DRCO program and print the completed card at the end of the day to send home with students. To address limitations in problem solving and data-driven decision skills (e.g., Farley-Ripple and Buttram, 2015), the DRCO has graphs of student progress and algorithms that offer data-driven recommendations about when goals should be changed and by how much (i.e., to gradually shape student behavior into the normative range while ensuring student success). The DRCO platform was designed to put evidence-based resources in the hands of teachers; however, it is also a tool that can be used by school-based behavior support staff (e.g., school psychologists, MTSS team leaders) to make their consultation work with teachers more efficient, systematic, and consistent across students and classrooms.
We conducted two quantitative evaluations assessing rates of teacher adoption of a DRC intervention when using the DRCO platform with limited support from behavioral support staff (Mixon et al., 2019; Owens et al., 2019). These studies, which assessed adoption of the first and second iteration of the DRCO platform, demonstrated that a substantial portion of teachers (39–51%) developed a DRC intervention and implemented it for at least 8 weeks, producing a meaningful change in student behaviors with minimal supports other than access to the DRCO platform (Mixon et al., 2019; Owens et al., 2019). These studies suggested that the DRCO platform had promise as a standalone technology-based tool for increasing teachers’ awareness, access, and implementation of Tier 2 classroom interventions.
A follow-up qualitative study (McLennan et al., 2020) revealed themes related to potential barriers to teacher’s implementation, some of which were addressed in the third (current) iteration of the DRCO platform. For example, teachers recommended simplifying some of the user interface features (i.e., reducing the number of steps in the DRC Development Wizard) and enhancing functionality (i.e., adding an option to track behavior via mobile devices in real time). Both of these features were upgraded based on this teacher feedback.
In addition, we received feedback that some teachers experienced confusion or dissatisfaction with tracking or targeting the problematic behavior (e.g., instances of disrespect), when the expected school ethos is to focus on the positive (e.g., “catch students being good”). We responded to this feedback in three ways. First, we developed a brief video describing the importance of (a) phrasing target behaviors in the positive on the DRC (e.g., respects others) so that students know what behavior is desired, (b) setting a goal that places a limit on behaviors that violate this expectation (e.g., respects others with 5 or fewer mistakes), as this is necessary to reduce the disruptive behavior, and (c) simultaneously praising positive alternative behaviors that are incompatible with the disruptive behavior (e.g., sharing materials, appropriate tone of voice). Second, we redesigned one step within the DRC Development Wizard to clarify what behaviors the teachers will be tracking and praising and what behaviors are expected to decrease over time. Third, we added additional target behaviors to the DRC Development Wizard focused on increasing prosocial skills (e.g., sharing feelings, problem solving) so that teachers could focus on both reducing disruptive behaviors and increasing prosocial behaviors. Given these upgrades, exploration of teacher implementation with these features is warranted.
Although our previous studies provided important insights, there are limitations to the conclusions that can be drawn from them. First, although we obtained qualitative feedback on facilitators and barriers, we did not explicitly assess adaptations teachers made to their DRC intervention when using the DRCO platform. Such adaptations can highlight features that teachers view as important for feasibility and fit. Second, although we examined teacher characteristics associated with adoption and implementation, we did not connect these variables or other characteristics to student outcomes. In the current study, we seek to explore the relationships across a broader set of implementation behaviors and their association with student outcomes as a way to generate hypotheses for future research. Lastly, all previous studies examined the utility of the DRCO platform as a standalone tool (e.g., as a mechanism for putting resources in the hands of more teachers). However, as the platform has evolved, we see how this tool can be leveraged to enhance efficiencies in consultation with teachers. Thus, the current study explores teacher adoption, adaptations, and implementation of a DRC intervention when using the DRCO platform with a behavioral consultant. By investigating the utility of the DRCO across different use contexts, we can better understand what supports are most beneficial in improving the ease and fidelity of teachers’ implementation.
Leveraging technology for teacher-implemented classroom interventions
We are aware of only a few studies that have examined outcomes associated with technology-focused DRCs like the DRCO program (i.e., Williams et al., 2012; Yeo et al., 2018; Riden et al., 2021). Although the technologies used in these studies (i.e., email and google documents) aimed to reduce paper burden and facilitate communication among parents and teachers, and school staff more broadly, the technologies did not guide the initial development of the DRC, the shaping of student goals over time, or teacher implementation of the intervention. Further, the intervention evaluation in these studies was time limited (e.g., only a few weeks). Thus, these studies shed little light on the extent to which technology-based supports can facilitate general education teachers’ use of a DRC with elementary school students over the course of a year under typical classroom conditions.
Collectively, these studies among others examining other technology-based classroom interventions (e.g., Harrison et al., 2020; Kumm et al., 2021; Scheibel et al., 2022), offer an example of how a traditional paper-and-pencil based intervention (like a traditional DRC) can be transitioned to a technology-based program to promote teacher implementation and positive student outcomes. However, due to the nature of many of the previous studies’ designs and samples, research is still needed to better understand the relationship between technology-based platforms, teacher implementation, and student outcomes.
Current study
There are several implementation science frameworks for assessing and interpreting data related to intervention adoption, adaptation, and implementation (e.g., Damschroder et al., 2009; Aarons et al., 2011; Glasgow et al., 2019). Each framework underscores the importance of attending to the key features of the intervention and the implementer, the social context (inner setting and outer setting features), and multiple processes over time (exploration, decision making, adaptation, implementation strategies) to facilitate the translation of science into practice. In this study, we used the RE-AIM framework to guide our inquiry. The aims of this study are to explore teachers’ use of the interactive wizards and decision-making tools (Aim 1); describe adaptations teachers made to assist with fit and feasibility (Aim 2); assess student outcomes using single-subject effect sizes representing change in target behaviors (Aim 3); and examine the association between student outcomes, teacher characteristics, and the above-described features (Aim 4).
Materials and methods
Participants
General education teachers were recruited from five elementary schools across two school districts in the Midwest to participate in a year-long study focused on evaluating the effectiveness of a consultation program supporting teacher use of Tier 1 classroom management strategies and Tier 2 classroom interventions (i.e., a DRC). Across the schools, 31 of 107 teachers (28.9% response rate) consented to participate in the year-long study. Of those 31 teachers, one teacher withdrew before completing any self-report measures and one teacher withdrew after three consultation sessions, leaving 30 teachers with fall survey data and 29 teachers who participated all year. Both teachers both withdrew due to time constraints. Teachers identified as White/Caucasian (93.3%), Black/African American (3.3%), or prefer not to answer (3.3%), and non-Hispanic/Latine (93.1% with 6.9% preferring not to answer). Most participating teachers were female (96.7% with 3.3% preferring not to answer). On average, teachers had 11.4 years (SD = 9.9) of teaching experience and 6.5 years (SD = 7.3) at their current school. Some (36.7%) had obtained a Master’s degree. Teachers taught Kindergarten (13.3%), 1st (3.3%), 2nd (13.3%), 3rd (26.7%), 4th (10.0%), and 5th grade (33.3%).
Of participating teachers, 22 teachers obtained parent consent to implement a DRC intervention with one student using the DRCO program, and 20 teachers had sufficient DRC data for analyses (one student moved; one teacher discontinued the DRC after 8 days). The main reasons for teachers not initiating the DRC intervention included caregivers not consenting to the intervention, teacher needing to focus on Tier 1 behavior management, and teacher declining to use the DRC intervention.
The demographic profiles of teachers were similar across school districts. In school district A, 57.6% of teachers had a Master’s degree and teachers were 87% female, 84.7% White/European American, 5.8% bi-racial, 4.7% Black/African American, 1% Asian/Asian American, and 3.5% Hispanic/Latine of any race. In school district B, 62.3% of teachers had a Master’s degree and teachers were 87.5 were female and 100% White/European American and non-Hispanic/Latine. Relative to the profile of general education teachers in the districts, our sample was representative in terms of race, ethnicity, and percentage of females but consisted of fewer teachers with a Master’s degree (34.5% in the sample compared to 57.6–62.3% in the school districts).
In School District A, the elementary schools had approximately 490 students and 20 general education teachers per school, with an average class size of 24.5 students. In School District B, the elementary schools had approximately 545 students and 24 general education teachers per elementary school, with an average class size of 22.7. The demographic profiles of students (one per teacher) were different across the two school districts (see Table 1). Of the 20 total students in this study, 11 were in School District A and nine were in School District B.
Procedures
All procedures were approved by all school districts and the Institutional Review Board at the university. All general education teachers at the five participating schools were recruited to participate via staff meetings and follow-up emails beginning in spring 2021 and continuing into August 2021. Teachers who consented to the project represent Cohort 1 (of three Cohorts) of teachers participating in a larger study evaluating consultation. Consenting teachers completed a brief consultation process involving a 30-min interview, a survey, two classroom observations, and a goal-setting consultation session. This gave teachers an opportunity to experience the consultation prior to signing up for the year-long trial. To support teachers in the context of challenges associated with COVID-19, all teachers who completed this brief consultation process were invited to participate in the year-long consultation for the 2021–2022 school year (i.e., no study exclusion criteria were applied).
During the year-long consultation, teachers were randomly assigned to receive one of two consultation packages: (1) standard best practices problem-solving with brief performance feedback or (2) multi-component individualized consultation that included all features of the standard condition and integrated techniques borrowed from motivational interviewing and cognitive behavioral therapy to address barriers to implementation [see Owens et al. (2017) for details]. Consultants were clinical psychology graduate students and research associates trained in providing both consultation packages. Teachers in both conditions received up to 10 individualized consultation sessions to support use of Tier 1 classroom management strategies with the whole class and Tier 2 strategies (i.e., DRC intervention) with one student. The consultation sessions occurred approximately twice per month between August and December, and once per month between January and March. Teachers in both conditions were also observed two times, for 30 min each, between each consultation session. During sessions, the teacher and consultant reviewed observation data to discuss strengths and areas for growth with regard to implementation of Tier 1 and Tier 2 practices. Because this sample represents the first cohort of three, we do not comment on consultation condition differences in this paper.
All teachers were given access to the DRCO platform and were encouraged to identify one student with whom to implement a DRC intervention. Teachers were instructed to identify a student who exhibited elevated behavioral concerns (e.g., inattention, hyperactivity, impulsivity, oppositionality) and needed additional supports beyond Tier 1 strategies. Teachers were instructed not to select a student who was suspected or identified as having autism spectrum disorder or cognitive impairment (e.g., Intellectual Disability, placement in Multiple Disability unit). Selected students had to spend at least 50% of the day in the teacher’s classroom.
As teachers considered potential students in need of intervention, they completed a brief screening measure (Daily Behavior Rating—see Section “Measures”). Students who exhibited elevated risk in the opposition, engagement, and/or disruptiveness subscales of this screener were eligible for the teacher to pursue caregiver consent. Once caregivers consented, child assent was obtained. Then, consultants and teachers used the DRCO Wizard to develop the DRC intervention (i.e., select target behaviors) and prepare for collecting baseline data through the DRCO program. After at least 3 days of baseline data collection, another consultation session was conducted to help the teachers finalize the target behaviors and goals and plan for implementation. Teachers were encouraged via email and follow-up consultation sessions to implement the DRC intervention consistently and complete monthly progress monitoring measures on the student (see Section “Measures”) through March of the school year. Teachers could discontinue or add target behaviors at any time based on their own judgment of student progress. After the end of March, teachers continued to have access to the DRCO platform but did not receive consultations or email reminders related to use of the DRC intervention. Teachers completed a final set of progress monitoring measures at the beginning of April.
Teachers also completed self-report ratings on a variety of factors that were hypothesized to be predictive of intervention implementation in August and January. In the spring teachers who used the DRCO program completed acceptability ratings about the DRC intervention.
Measures
Demographic questionnaire
In the fall, teachers were asked to report their age, gender, race/ethnicity, highest level of educational attainment, and years of experience. Caregivers reported child gender, race/ethnicity, primary language spoken, and free/reduced lunch status.
Daily report card online platform use metrics
For Aim 1, we obtained several indicators of adoption and implementation from the DRCO platform. First, we determined if the teachers adopted the intervention (i.e., completed the DRCO Wizard and initiated a DRC intervention using the DRCO platform). Second, we obtained information on the type of DRC target behaviors teachers selected from the DRCO Development Wizard. Third, we calculated the number of days between the start of the baseline tracking and the start of the DRC intervention and the month of DRC intervention initiation. This metric is important because it reveals how efficiently teachers were able to initiate implementation of the DRC intervention. Fourth, we calculated an index of teacher implementation integrity across the year, defined as the number of school days for which teachers entered data about target behaviors, divided by the total number of eligible school days (e.g., without student absences and holidays) between the starting date and ending date of the DRC (last day of data entry). Fifth, we calculated the duration of target behavior as the number of school days between the first and last day data were entered for each target behavior. Lastly, we calculated the percent of eligible school days on which the student met their goal for each target behavior. For the last three indicators (implementation, duration, and achievement), we averaged indicators across all target behaviors for that teacher.
For Aim 2, we asked consultants to report on several indicators. First, they reported whether the teacher tracked daily DRC target behaviors in real time using the DRCO technology or on paper (and later entered the data into the DRCO platform). Second, because the consultants were familiar with the DRCO design, we asked them to describe any adaptation the teacher made when implementing the DRC intervention with their student (e.g., modifying printable resources to make it more student friendly or easier for the teacher to implement).
For Aim 3, we extracted the daily data that teachers tracked about target behaviors (e.g., daily frequencies of interruptions or out of seat behaviors; daily percent of work completed). Using these data, we calculated Taunon–overlap and Tau-U effect sizes [single-case effect size; Parker et al. (2011)] to assess change in each target behavior from baseline to each month of the DRC intervention (see details in Section “Analytic strategy” below).
Teacher knowledge of behavioral principles
In the fall, teachers completed an 11-item multiple-choice measure assessing teacher knowledge of behavioral principles. This measure includes items with high difficulty that differentiate teachers with high and low knowledge, are sensitive to change as a function professional development (Owens et al., 2014), and have discriminant validity in identifying teachers with disparate baseline characteristics (Owens et al., 2017). A percent correct score was calculated for each teacher. Teacher knowledge scores are used in Aim 4.
Teacher stress
In the fall and winter, teachers completed four items from the Teacher Concerns Inventory (TCI) (Fimian and Fastenau, 1988) that assess general work-related stress (α = 0.84 in fall; α = 0.82 in winter). Items are scored on a 5-point scale with higher scores indicating greater stress. The psychometric properties of the measure, including the factor structure and convergent validity are well-demonstrated [see Fimian and Fastenau (1988) for review]. TCI scores have been shown to be associated with teacher burnout, job satisfaction (Fimian and Fastenau, 1988), and counseling sought for work-related issues (Fimian and Krupicka, 1987). Teacher stress scores are used in Aim 4.
Teacher self-efficacy
In the fall, teachers completed the Teacher Self-Efficacy Scale (TSES) (Tschannen-Moran and Hoy, 2001), a 12-item measure (α = 0.92) that assesses teacher’s self-efficacy in three domains: student engagement (four items; α = 0.83), instructional strategies (four items; α = 0.87) and classroom management (four items; α = 0.84). Items are rated on a 9-point scale, with higher scores indicating greater self-efficacy in that domain. The three-factor structure has been replicated across independent samples (Tschannen-Moran and Hoy, 2001). The scores correlate in the expected direction (moderate in magnitude) with other self-efficacy scales (Tschannen-Moran and Hoy, 2001). There is evidence that scores are sensitive to change as a function of consultation with modeled coaching procedures (Tschannen-Moran and McMaster, 2009). Teacher self-efficacy scores are used in Aim 4.
Teacher and consultant working alliance
In winter, teachers completed the Working Alliance Inventory-Short Form Revised (WAI-SR) (Hatcher and Gillaspy, 2006). The WAI is a 12-item self-report instrument assesses the strength of a therapeutic alliance. Items, rated on a 7-point Likert scale (from never to always) are used to compute a total score (α = 0.94). For this study, we modified the item wording slightly to apply to the alliance between the teacher and the consultant. Working alliance scores are used in Aim 4.
Usage rating profile—intervention
The Usage Rating Profile-Intervention (URP-I) (Chafouleas et al., 2009; Briesch et al., 2013) assesses teacher perceptions of intervention acceptability. The URP-I has a replicated factor structure identifying six subscales that are internally consistent: Acceptability, Understanding, Feasibility, Family-School Collaboration, System Climate, and System Support. Items are rated on a 1–6 scale, and for 5–6 subscales, higher scores indicate higher acceptability. The exception is the Systems Support subscale where lower scores indicate that teachers feel they can implement the intervention without support from administrators or others. Teachers who implemented a DRC intervention completed the Acceptability (13 items; α = 0.96), Understanding (eight items; α = 0.89), Feasibility (eight items; α = 0.97), and Systems Support (six items; α = 0.62) subscales about the DRC intervention. URP-I scores are used in Aim 4.
Student progress monitoring
Daily behavior rating
To screen students and to monitor progress over time, teachers completed multi-item Daily Behavior Ratings on the DRCO platform (DBR) (Hustus et al., 2020; Matta et al., 2020). Two DBRs assess positive behaviors (Organizational Skills and Engagement; αs > 0.81 across all time points) and two DBRs assess problem behaviors (Opposition and Disruptiveness αs > 0.85 across all time points). Each DBR includes five items. For the positive behavior subscales, teachers indicated the frequency of these behaviors on a 7-point scale (0 = Never to 6 = Almost Always). For the problem behavior subscales, teachers indicated the degree to which each behavior was a problem on a 7-point scale (0 = Never to 7 = Almost Always). Students were considered to be a good candidate for a DRC intervention if they had moderate or high risk, defined as having three or more items scored two or lower on the Engagement subscale or having three or more items scored four or higher on either problem behavior subscale. This measure was completed prior to the start of the DRC intervention, monthly during intervention, and in the spring. Studies document the treatment sensitivity of these measures (Hustus et al., 2020; Matta et al., 2020) and that they are capable of identifying children with behavior problems (Daniels et al., 2020). DBR scores are used in Aim 4.
Strengths and difficulties questionnaire
Teachers completed the Strengths and Difficulties Questionnaire (SDQ) (Goodman, 2001), a 25-item measure assessing perceptions of student behaviors in five areas: emotional symptoms (αs range from 0.73 to 0.94 across timepoints), conduct problems (αs range from 0.60 to 0.80 across timepoints), hyperactivity/inattention (αs range from 0.61 to 0.87 across timepoints), peer relationship problems (αs range from 0.20 to 0.59 across timepoints), and prosocial behavior (αs range from 0.83 to 0.91 across timepoints). Items were rated on a 3-point Likert scale (0 = not true to 2 = certainly true) with higher scores on the emotional, conduct, hyperactivity/inattention, and peer relationship subscales indicating more problematic behaviors. A total problems score (αs range from 0.45 to 0.81 across timepoints) was calculated by summing the scores from the emotional, conduct, hyperactivity/inattention, and peer relationship subscales. This measure was completed prior to the start of the DRC intervention, monthly during intervention, and in the spring. The teacher version of this measure has acceptable internal reliability, high test-retest correlation, and predictive utility in identifying problems in elementary school students (Goodman, 2001; Owens et al., 2016). SDQ scores are used in Aim 4.
Analytic strategy
For Aim 1, we used descriptive statistics to summarize teacher use of the DRC intervention using implementation data collected via the DRCO platform. For Aim 2, descriptive statistics and qualitative descriptions were used to summarize teacher adaptations to the DRC intervention. For Aim 3, we calculated Taunon–overlap and Tau-U effect sizes [single-case effect size; Parker et al. (2011)] to assess change in each target behavior from baseline to each month of the DRC intervention. A month was indicated as a calendar month following the date the intervention was initiated (e.g., if the intervention was initiated on 10/20 then Month 1 of data would include any data from 10/20 to 11/19 and Month 2 would include any data from 11/20 to 12/19). Effect sizes for a particular intervention month were calculated if there were at least 10 days of DRC intervention data for that target behavior in the month. For intervention months that included winter break, DRC intervention data from the end of the month before winter break was carried over to the next intervention month to calculate up to 10 days of intervention data for the intervention month following winter break. This was done for five target behaviors (6% of total target behaviors). A similar procedure was conducted when the last month of intervention did not contain 10 days of intervention data due to the intervention being completed. In these cases, the intervention data from the last month was added to the previous month when calculating an effect size. This was done for 28 targets (35% of target behaviors). Among the 79 targets, 18 targets (23%) were missing one month of data, 12 targets (15%) were missing 2 months of data, and nine targets (11%) were missing three or more months of data due to inconsistent data entry (i.e., less than 10 days of data entered during an intervention month).
Taunon–overlap was used to calculate the effect size unless the baseline tau was greater than 0.1 [indicating substantial baseline trend; Vannest and Ninci (2015)], in which case Tau-U was used. For three target behaviors (4% of total target behaviors), baseline data was not collected. For these behaviors, baseline was estimated by taking the initial goal for the target behavior set by the teacher at the start of the intervention and repeating that for 5 days of baseline. Vannest and Ninci (2015) provide guidelines for interpreting Tau, indicating 0.20 as a small change, 0.20–0.60 as moderate change, and 0.60–0.80 as large change. To be consistent with previous studies [see Holdaway et al. (2020)], we used an effect size of 0.40 as a lower threshold for denoting an acceptable response to the intervention.
For Aim 4, we calculated correlations to examine associations between adoption, implementation, change in target behaviors, and change in teacher rating scales (i.e., the DBR and SDQ). In addition, we created various groupings of teachers and examined characteristics associated with those groups (e.g., teachers who adopted the DRC intervention and did not; teachers representing those whose student achieved a minimum threshold of success (Tau ≥0.4) and those who did not).
Results
Aim 1: Teachers’ use of the daily report card online platform
Across the DRC interventions for all 20 students, 79 target behaviors were reviewed to determine if there were sufficient data for analyses; we found that 66 target behaviors had at least 1 month with enough intervention data to calculate an effect size. Henceforth, we present data about these 66 target behaviors. Table 2 shows the types of behaviors that teachers selected to target on their DRC interventions. All 20 teachers added at least two target behaviors to the DRC; 19 teachers added three targets, but only eight teachers added a 4th target (five of whom also added a 5th target behavior and three of whom added a 6th target behavior). Reducing interruptions, non-compliance, and increasing work completion were the top three most frequently selected target behaviors. Across target behaviors, 6% of the targets were initiated in October, 37.8% in November, 18% in December, 33% in January.
On average, DRC interventions were initiated 15.73 days after the start of baseline tracking (SD = 16.29 days). More specifically, 21% of target behaviors were initiated within 5 days, 59% were initiated within 10 days, and 73% were initiated within 15 days. For the remaining 27%, in most cases, the teacher started tracking in the fall, but for a variety of different reasons (e.g., high rates of teacher and student absence, rescheduled consultation sessions), did not initiate the DRC intervention until December or January.
With regard to changes to goal criterion, 29–66 target behaviors (43.9%) had one change in the goal criterion over time, seven of 66 (10.6%) had two goal changes, and 30 of 66 (45.5%) did not make a goal criterion change. With regard to the number of days passed before a goal change, 19% of target behaviors were changed within 1 month, 47% were changed within 2 months, and the remaining were changed after 2 months or more.
On average, teachers implemented the DRC intervention, defined as entering data on days the target behavior was applicable, on 63% of school days (SD = 18%; range: 28% to 88%), and target behaviors remained on the DRC for an average of 80 school days (SD = 29; range: 40–143 days). On average, target students achieved their DRC goals on 71% of school days (SD = 18%; range: 33 to 100%).
On average, teacher acceptability ratings of the DRC intervention are positive: Acceptability: (M = 5.07, SD = 0.83); Understanding: (M = 5.38, SD = 0.55); Feasibility: (M = 5.06, SD = 0.83). On average, their ratings suggest that they do not feel that they need support from administrators to implement the intervention (M = 2.69, SD = 0.76).
Aim 2: Adaptations
Data about adaptations are presented in Table 3. Of the 20 teachers who used the DRCO platform, 12 teachers (60%) used it as is and made no adaptations. The remaining eight teachers (40%) made adaptations on materials being presented to students. In this subsample of teachers, three (37.5%) modified how they presented the printed DRC to the student by adding emojis and clipart next to each target behavior, or adding check boxes and laminating the card so that the student could track their behavior, two (25%) changed the size of the DRC card so they could tape it to the student’s desk, and three (37.5%) made and printed reward menus that differed from those offered on the DRCO platform. Of note, none of the adaptations changed the underlying principles of the intervention.
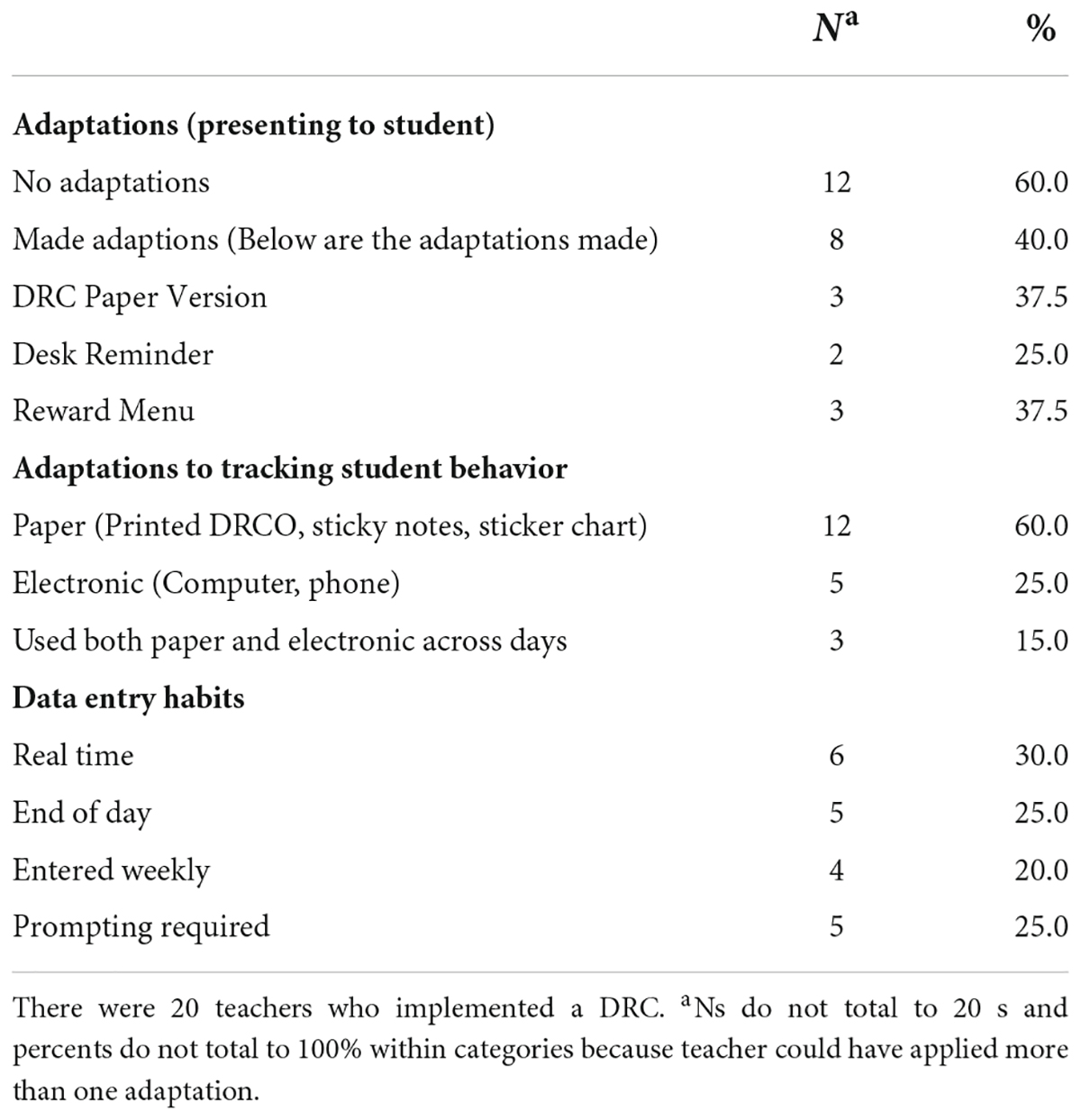
Table 3. Adaptations teacher made when implementing a daily report card (DRC) with daily report card online (DRCO).
Consultants also documented that 30% percent of teachers created 1–2 target behaviors in the DRCO Wizard that, ultimately, were not used on the DRC intervention. We think this evidence highlights the need that some teachers have to explore the system prior to settling on a target behavior that is well-matched to student behavior and teacher need.
Lastly, consultants documented the method each teacher used to track DRC target behaviors and how they entered data into the DRCO program. Across teachers, 12 (60%) tracked student behaviors through paper methods (printed DRCO, sticky notes, and sticker charts), five (25%) tracked through electronic methods (computer, phone), and three (15%) used multi-method of tracking (this includes a combination of paper and electronic methods). Among those teachers who tracked on paper, some teachers entered the data at the end of each day and others entered data at the end of the week. About 25% of teachers needed prompting to remind them to enter data.
Aim 3: Tau effect sizes representing change in target behaviors
Tau-effect sizes for DRC target behaviors (both behavioral, such as interruptions and non-compliance; and academic, such as work completion, work accuracy, and homework) are presented in Table 4. The effect sizes indicate that on average, both academic and behavioral target behaviors showed small but steady improvement over Month 1 to Month 4, with target behaviors that lasted into Months 3 and 4 showing moderate improvement relative to baseline.
Aim 4: Associations among teacher characteristics, implementation, and outcomes
With regard to teacher adoption, we compared the 20 teachers who had sufficient data to calculate effect sizes for DRC target behaviors to the 10 teachers who completed fall surveys but did not adopt a DRC/did not have sufficient data. The two groups of teachers did not differ significantly on any variable (i.e., years in the field, knowledge of behavioral principles, teacher self-efficacy). However, a few patterns generate hypotheses for future research. Namely, the average fall work-related stress subscale score was lower (M = 3.41; SD = 0.85) among adopters than among non-adopters (M = 3.70; SD = 0.86). Similarly, the average winter work-related stress subscale score was 3.33 (SD = 0.81) among adopters and 3.66 (SD = 0.86) among non-adopters. The Hedge’s g effect sizes for these group differences was 0.33 and 0.37, respectively. Further, the teacher-reported total working alliance score with the consultant was lower among non-adopters (M = 5.97; SD = 0.84) than adopters (M = 6.42; SD = 0.88; Hedge’s g = 0.66).
Second, we examined the correlations between teacher implementation (percent of days with data), target behavior duration, student goal achievement, and teacher characteristics (see Table 5). With regard to teacher implementation, a significant association was found with work-related stress in the fall (r = –0.48, p = 0.024), and teacher reported self-efficacy in student engagement (r = 0.50, p = 0.018) and classroom management (r = 0.59, p = 0.004). With regard to student goal achievement, a significant association was found with work-related stress in the winter (r = –0.46, p = 0.030). No significant associations were found between target behavior duration and any other variable. Several expected associations among teacher characteristics were found. Number of years teaching was associated with teacher reported self-efficacy in instructional strategies (r = 0.70, p = 0.001) and self-efficacy in classroom management (r = 0.54, p = 0.001). All dimensions of teacher reported intervention acceptability were associated with teacher-consultant working alliance (see Table 5).
Third, we examined the relationship between teacher implementation, target behavior duration, student goal achievement, and student outcomes (i.e., average Tau effect size across targets for Month 1 and Month 2). Teachers who observed an average Tau effect size across target behaviors of 0.4 or higher had stronger effect sizes in all months (Month 1 M = 0.20; Month 2 M = 0.56; Month 3 M = 0.46; Month 4 M = 0.53), than those whose target students did not achieve this level of initial success (Month 1 M = 0.05 Month 2 M = –0.05; Month 3 M = 0.24; Month 4 M = 0.03). Further, among the teachers who observed an average Tau effect size across target behaviors of 0.4 or higher, average implementation across the year was 67% (SD = 18%), target behaviors lasted on average 93 days (SD = 32 days), and target students achieved their goal, on average, 81% of days (SD = 12 day). In contrast, among the teachers whose target students did not achieve at least a 0.4 Tau effect size, implementation was 59% (SD = 16%), target behaviors lasted on average 64 days (SD = 16 days), and the students achieved their goal, on average, 59% of days (SD = 16). Although only some of these group differences are statistically significant (Month 2 Tau, duration, achievement), the Hedge’s g effect sizes are all greater than 0.5, suggesting that the magnitude of the difference warrants further investigation in larger samples.
We found little difference in teacher implementation, duration, or student goal achievement among those who tracked DRC target behaviors via the technology platform in real time (Implementation M = 64%; SD = 20; Duration M = 82 days; SD = 34; Student Achievement M = 68%; SD = 11%) as compared to those who tracked DRC target behaviors via paper (Implementation M = 62%; SD = 17%; Duration M = 80 days; SD = 29; Student Achievement M = 72%; SD = 20%). Although the average Tau effect size across all target behaviors in Month 1 were higher among those using the technology (M = 0.26; SD = 0.18) than those tracking on paper (M = 0.08; SD = 0.32), there were no differences in average effect sizes across target behaviors in Months 2, 3, or 4. Of note, however, those using technology had half as many days between baseline tracking and the start of the intervention (Target 1 M = 13 days SD = 11 days; Target 2 M = 12 days SD = 9 days) than those using paper (Target 1 M = 22 days SD = 20 days; Target 2 M = 25 days SD = 23 days).
Lastly, we examined the relationship between change in DRC target behavior and change in teacher-rated engagement, oppositional behavior, and disruptive behaviors from the DBRs and SDQs (that were completed in the fall and spring.). See Figures 1A–C for the pattern of DBR ratings among target students who did and did not achieve an average Tau effect size across DRC target behaviors of 0.4 or higher. See Figures 2A–C for the pattern of SDQ ratings across these same groups. Whereas, all students were rated by their teachers as generally being more engaged, less disruptive, and less oppositional in the spring than in the fall, the patterns suggest the change may have been greater for those who demonstrated greater change on their DRC target behaviors.
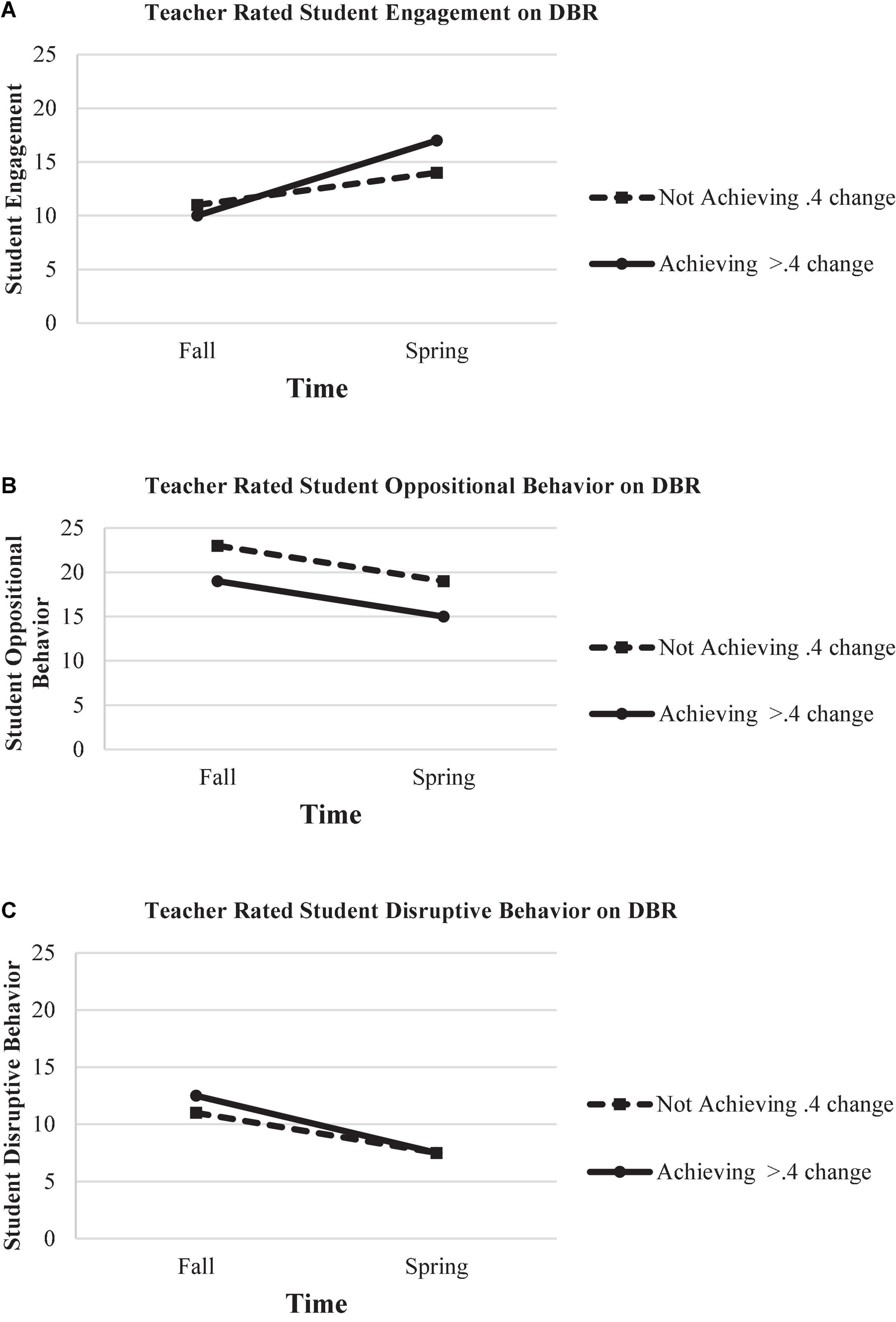
Figure 1. Teacher daily behavior rating (DBR) ratings among students achieving and not achieving change (Tau ≥0.4) in daily report card (DRC) target behaviors. (A) Teacher rated student engagement on DBR. (B) Teacher rated student oppositional behavior on DBR. (C) Teacher rated student disruptive behavior on DBR.
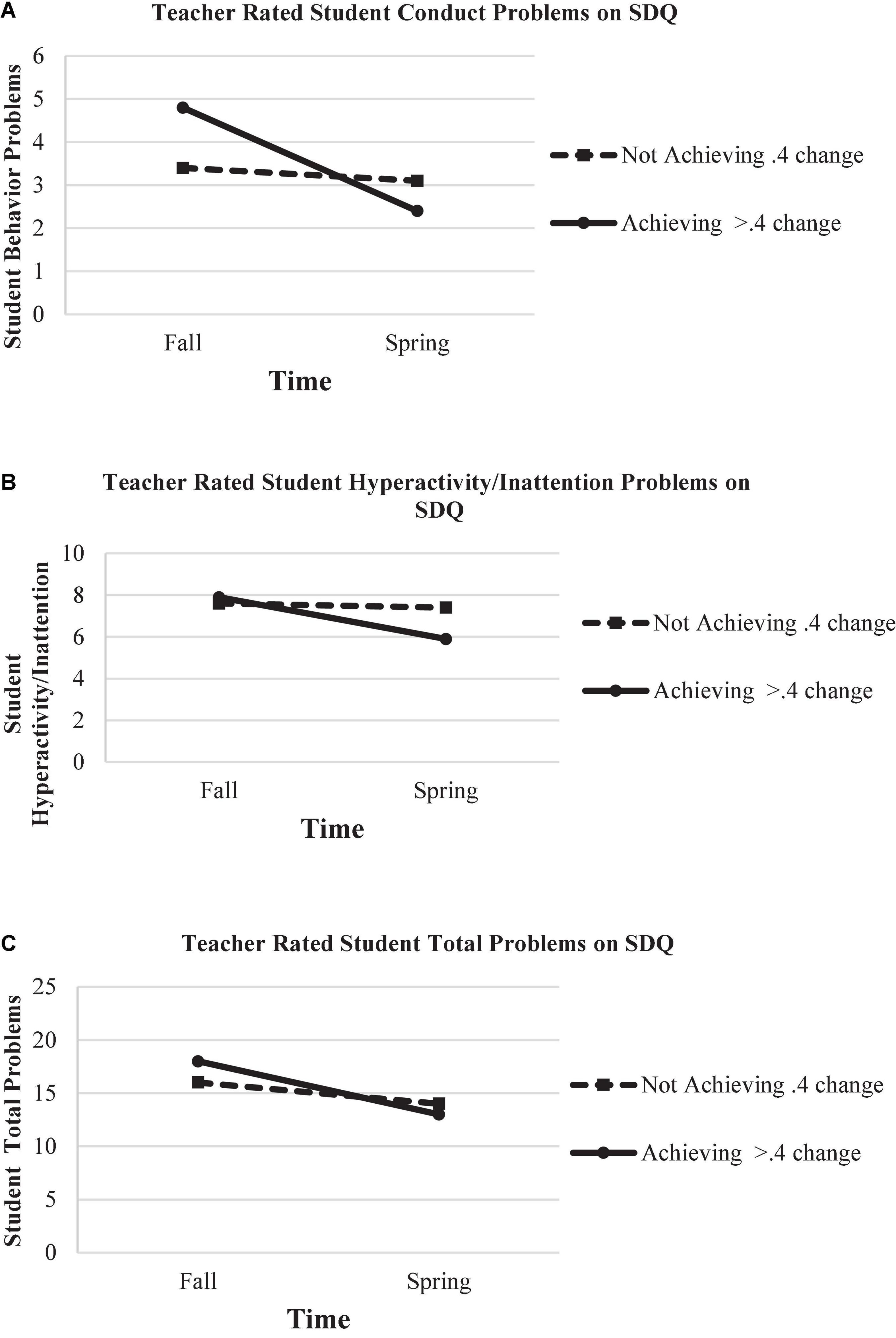
Figure 2. Teacher strengths and difficulties questionnaire (SDQ) ratings among students achieving and not achieving change (Tau ≥0.4) in daily report card (DRC) target behaviors. (A) Teacher rated student conduct problems on SDQ. (B) Teacher rated student hyperactivity/inattention problems on SDQ. (C) Teacher rated student total problems on SDQ.
Discussion
In the context of the global COVID-19 pandemic, elementary school teachers are facing unprecedented demands as they try to help their students make gains academically, socially, emotionally, and behaviorally. In this context, many students are likely to need Tier 2 interventions to be successful in the classroom. Technology-based supports, such as the DRCO platform offer promise for helping teachers follow recommended procedures (e.g., Center on PBIS, 2022) for implementing high quality Tier 2 interventions. However, research is needed to examine this promise. To contribute to this agenda, we examined teacher adoption, adaptation, and implementation of a DRC intervention when using the DRCO platform with the support of a consultant during the 2021–2022 school year. Below, we consider recommended guidelines for Tier 2 interventions as we interpret the results from the current study.
Recommended guidelines for tier 2 interventions
Screening
Aligned with MTSS recommendations (Center on PBIS, 2022), in the current study, 100% of students considered for a DRC intervention were screened for risk status on important variables related to student success (i.e., student engagement, oppositionality, and disruptive behavior) and found to be at risk for problems. This suggests that the DRCO program can help teachers and behavioral support staff successfully screen students in a systematic way.
Intervention focus
With regard to narrowing problems to a manageable set of target behaviors, most DRCs only included one to two target behaviors at a time. Although only targeting two behaviors at a time may ultimately slow student progress, addressing more than this may not be possible in the context of high teacher stress. This balance is important for behavior consultants to consider and explicitly discuss with teachers to navigate expectations.
The DRC target behaviors selected most frequently in the current sample were student interruptions, non-compliance, and work completion. This is consistent with previous studies on the DRC intervention (Owens et al., 2012; Holdaway et al., 2020) and aligns with teacher needs in the current context (i.e., reduce disruptive behaviors and improve academic performance). However, data in Table 2 demonstrates the importance of having a diverse array of target behaviors available in the DRCO Development Wizard so that teachers can match the intervention to the student impairment profile.
Data driven decisions
Consistent with evidence-based recommendations, baseline data were used to guide the development of the goal criterion for most target behaviors (96%), and most DRC interventions (73%) were initiated within 15 days after the start of baseline tracking. Technically, teachers can initiate the intervention within 3–5 days of baseline tracking. The longer span of time in this study is likely a function of the DRCO being used in the context of consultation. Namely, because consultation sessions occurred every other week, even if teachers had obtained 5 days of baseline data, many waited to discuss the data with a consultant before initiating the intervention. On one hand, this delay in DRC intervention initiation may be longer as a function of the research protocol. On the other hand, it may well represent practice, as those supporting teachers may not be able to meet with the teacher any more regularly than every 2–3 weeks.
The DRCO Development Wizard uses baseline data and algorithms to recommend target behavior goals that balance achievability (for student motivation) and gradual change over time. The student achievement data suggest that teachers used these guidelines to set achievable goals because on average, students achieved their goals on 71% of school days (SD = 18%; range: 33–100%). However, our results suggest that teachers may not have used elements of the platform to make changes in DRC goal criteria as much as intended by developers. Namely, a change in the goal criterion was not made for almost half (45.5%) of target behaviors. Among target behaviors that were changed, 19% were changed within 1 month, 47% were changed within 2 months, and the remaining were changed after 2 months or more. This pattern may have occurred, in part, because student behaviors were slower to change as a function of the COVID-19 context (i.e., high rates of student and teacher absence leading to less consistency in implementation and student behavior) and in part because of the research-based procedures. Anecdotally, consultants reported that they often had to prompt teachers to view the graphs and recommendations on the platform, offer guidance in interpreting them, and nudge them to consider a goal change. It is possible that because teachers could rely on the consultant, there was some diffusion of responsibility relative to if they were using the DRCO on their own. However, it may also suggest that technological tools need to provide greater guidance for teachers to use this support (e.g., scheduled reminders, instructions for interpreting the graphs). Because technology was not used to guide data-driven decisions in previous DRC studies (Williams et al., 2012; Yeo et al., 2018; Riden et al., 2021) and because these features of the DRCO program did not appear to be used by teachers as frequently as we had hoped, additional research is needed to explore ways to help teachers maximize these tools.
Teacher adaptations
Interestingly, 25% of teachers tracked student target behaviors directly via the DRCO platform, 60% tracked data through paper methods, and 15% used a combination of the two. Thus, despite feedback from previous users that real time data entry was preferred over paper tracking, many users in the current school districts preferred to print the DRC. Interestingly, we did not find many differences in implementation outcomes between those who used technology to track versus paper. Thus, it may not be technology per se that matters most for tracking but rather that teachers have different preferences, and matching that preference is most important for implementation quality. Thus, any technology platform intended to enhance the implementation of classroom interventions should be prepared to support such variety in teacher preferences. That said, it is interesting to note that teachers using technology had half as many days between baseline tracking and the start of the intervention than those using paper. Thus, if teachers are comfortable with technology, such a system seems to lead to efficiencies.
With regard to teacher preferences, it is also noteworthy that 60% of teachers used the DRCO as is and made no adaptations. However, among those who did make adaptations, they did so to enhance student engagement. These adaptations are important for developers to consider because it may be difficult to create “one size fits all” materials. Offering customization options for student engagement may also facilitate teacher engagement with the technology. With these results, we are considering DRCO updates including giving teachers the option to add visual pictures to each target behavior and the option to print a condensed version for student desks. We previously considered creating a student portal on the platform; however, with that comes additional security burdens, thus we have yet to pursue this option. It is noteworthy that all of the adaptations in the current study appeared to center around a teacher’s preference to creatively individualize the DRC intervention rather than modifying the intervention itself. Thus, the adaptations did not a sacrifice implementation quality.
Teacher implementation
On average, teacher implementation (percent of days the teacher tracked DRC behaviors) was 63% (range: 28–88%). This is slightly lower than that found in previous studies [75% in Owens et al. (2012); 89% in Holdaway et al. (2020)], but may likely represent the challenges that teachers were facing navigating the many demands placed on them during the COVID-19 pandemic. Indeed, we found that fall and winter work-related stress was higher among teachers who did not initiate a DRC intervention, and that implementation was significantly correlated with teacher work-related stress in the fall and student achievement of goals was significantly correlated with teacher work-related stress in the winter. Thus, stress is an important contextual factor to consider, even when providing technology supports designed to make implementation more feasible (Owens et al., 2019). Additionally, it is important to note that in the Owens et al. (2012) and Holdaway et al. (2020) studies, teachers used the DRCO program independently and were thus, the only ones responsible for developing and implementing DRC interventions. However, in the current study, teachers used the DRCO program within the context of consultation. As a result, it is possible that implementation rates were slightly lower than those found in previous studies because teachers were relying on their consultants to provide implementation supports. Further, although teachers rated the DRC intervention in the current study to be generally acceptable, understandable, and feasible, it is possible that the added consultation supports contributed to this positive perception. Because some research suggests that technology-based supports may be more cost effective than face-to-face supports (Owens et al., 2021), research that compares implementation and outcomes under differing technology-based conditions (e.g., with and without varying levels of support from behavioral consultants) is warranted.
Student outcomes and associations among variables
The Tau effect sizes presented in Table 4 demonstrate that students made small to moderate gains in target behaviors over time. The outcomes for target behaviors that lasted through Month 3 and Month 4, are similar to those found in the Holdaway et al. (2020) study. However, the outcomes for target behaviors in Month 1 and Month 2 are more modest than those found in the previous study. Although this difference could be a function of a variety of unmeasured factors, the COVID-19 context is a critical factor for interpreting the data. This sample includes young elementary school students (50% in third grade or younger) who experienced significant disruption in the last year and half of schooling. Thus, the impact of COVID-19 on social and behavioral maturity and on student-teacher relationships is important to consider.
Although the sample size is not sufficient to conduct statistical analyses, we noticed several trends connecting teachers’ implementation and student outcomes. Namely, teachers who achieved positive student outcomes had trends toward higher rates of implementation, greater success with each month of implementation, and greater change in teacher-rated student engagement and disruptive behaviors on the DBR and SDQ. Especially in the challenging context of the 2021–2022 school year, it is important to highlight that positive change in teacher-ratings on the DBR and SDQ as well as small to moderate gains in DRC target behaviors are commendable improvements. It is also important to acknowledge that there is evidence that teacher implementation and student outcomes may have a bi-directional effect on each other (Girio-Herrera et al., 2021), with teacher implementation leading to more positive student outcomes, and initial positive student outcomes reinforcing teacher implementation. This seems to be replicated in the patterns we found. Nonetheless, we also acknowledge that teachers with stronger implementation skills at baseline are likely to produce better outcomes at all times than teachers whose implementation skills are developing over time. Thus, if technology can support high quality teacher implementation (as noted above), then such technology warrants further development and evaluation.
Sample representation
The RE-AIM framework offers important guidance for researchers about how to communicate sample representation and how to consider its implications. Our general education teacher sample is representative in terms of percentage of females at the district and national level. However, our sample consists of fewer teachers with a Master’s degree (34.5% in sample compared to 57.6–62.3% in the school districts and 55% nationwide). When we advertised our project, we highlighted the benefits of consultation for early career teachers (particularly those who started their careers under virtual or hybrid conditions associated with the COVID-19 school closures), as they may have less experience applying high quality classroom management strategies. Indeed, 30% of our sample has been teaching for less than 5 years, shedding light on one potential reason for this sample’s underrepresentation of teachers with Master’s degrees. Compared to the district, our teacher sample was representative in terms of race and ethnicity.
Our demographic data suggest that our student sample likely represents students with a variety of lived experiences. Given the small sample size, it is difficult to draw conclusions based on district representativeness; however, data can be useful to districts as they consider equitable distribution of Tier 2 supports, allowing them to explore who is and who is not receiving interventions. This information may then be useful in understanding how biases may play a role in their broader referral or screening process. It is also worth noting that representation in research studies is likely to differ from district representation as a function of several complex dynamics. One salient characteristic is the lack of dual language learners in the current research sample. Despite having distributed flyers and consent forms in Spanish, there are still barriers to home-school engagement when caregivers and teachers do not share the same primary language; thus, likely leading to understandable hesitancies on the part of caregivers to enroll in research in this context. This is a reminder to school professionals, educators, and researchers about the additional actions we must take to better engage families whose primary language is not English. Exploration of sample representation is a useful exercise that should be given greater attention in research (Glasgow et al., 2019).
Limitations
Although the current study adds valuable findings to the literature related to technology-assisted classroom interventions, the results must be interpreted in the context of important limitations. First, the small sample size precluded our ability to conduct statistical testing. Second, teachers in the current sample were not randomly assigned to implementing a DRC intervention with or without the DRCO platform, thus there are limitations in the attributions we can make about the impact of the technology per se. In addition, we must interpret the data knowing that teacher use of the DRCO platform occurred in the context of a larger consultation project that involved regular one-on-one meetings with a consultant centered on improving the teacher’s use of Tier 1 and Tier 2 classroom management strategies. Thus, we cannot draw conclusions about teacher use of the DRCO program without acknowledging that some of the effects could have been influenced by consultation. It is possible that these findings would differ had the sample of teachers used the DRCO program as a standalone intervention.
Implications for future research and practice
The current study expands upon previous literature by exploring important outcomes associated with a technology-based classroom intervention (DRCO Platform). Collectively, the findings suggest that teachers are incredibly resilient despite experiencing additional challenges as a result of the COVID-19 pandemic. In this difficult context, many teachers implemented DRCs in a manner that aligns with recommended guidelines for screening, keeping a narrow focus, and data-driven decision making. On average, teachers implemented DRC interventions using the DRCO program on 63% of school days, their students were able to meet their goals on 71% of school days, and Tau effect sizes showed small to moderate improvements overtime, with trends that changes in target behaviors were associated with teacher ratings on the DBR and SDQ. This suggests that the DRCO platform may be a useful tool even in the context of the pandemic.
Although the average rate of teacher implementation was lower than that found in previous studies (Owens et al., 2012; Holdaway et al., 2020), teacher work-related stress was found to be associated with initial DRC adoption and implementation. This pattern is consistent with previous literature (Owens et al., 2019) and suggests that future research should continue to examine stress as an important contextual factor in understanding the feasibility of classroom interventions and technology-based tools. In addition, future research is needed to evaluate the extent to which enhancing some of the data-driven decision making tools (i.e., algorithms and graphs to assist in changing target behavior goals) can enhance teacher implementation and student outcomes.
Lastly, by tracking adaptations, we learned about teacher preferences for student-friendly materials and that no adaptations changed the key principles of the intervention. Future research should continue to explore intervention adaptation to better understand issues of fit and feasibility. These findings can then inform enhancements to technology-based tools, like the DRCO, to allow for individualization and to increase engagement for both students and teachers.
Data availability statement
The raw data supporting the conclusions of this article will be made available by the authors, without undue reservation.
Ethics statement
The studies involving human participants were reviewed and approved by Ohio University Institutional Review Board. Written informed consent to participate in this study was provided by the participants’ legal guardian/next of kin.
Author contributions
JO conceived of the research questions, wrote the initial draft of the introduction, edited and contributed to all parts of the manuscript, and was responsible for incorporating feedback from ML, KE, DM, SE, and JR and internal reviewers. ML wrote the first draft of the materials and methods section, was responsible for cleaning and preparing the data, conducted most of the analyses, assisted with writing the results section, and supervised the work of DM. KE co-wrote the introduction and discussion sections with JO and edited and contributed to all parts of the manuscript. DM worked under the supervision of ML and JO, conducted some analyses, and wrote parts of the results sections. SE assisted with the development of the research questions and edited and contributed to all parts of the manuscript. All authors contributed to the article and approved the submitted version.
Funding
This research was supported by the Institute of Education Sciences, U.S. Department of Education (R324A190154).
Acknowledgments
The authors express their sincere gratitude to the teachers who participated in this study, particularly given the many competing demands they faced as a function of the COVID-19 pandemic. The authors wish to acknowledge the contributions that Sam Girton has made to the development of the DRCO platform.
Conflict of interest
JR was employed by Reid Software at the time of this study. JR was also a software development contractor for Ohio University for the duration of the study.
The remaining authors declare that the research was conducted in the absence of any commercial or financial relationships that could be construed as a potential conflict of interest.
Publisher’s note
All claims expressed in this article are solely those of the authors and do not necessarily represent those of their affiliated organizations, or those of the publisher, the editors and the reviewers. Any product that may be evaluated in this article, or claim that may be made by its manufacturer, is not guaranteed or endorsed by the publisher.
Author disclaimer
The opinions expressed are those of the authors and do not represent views of the Institute or the U.S. Department of Education.
Footnotes
References
Aarons, G. A., Hurlburt, M., and Horwitz, S. M. (2011). Advancing a conceptual model of evidence-based practice implementation in public service sectors. Administr. Policy Ment. Health Ment. Health Serv. Res. 38, 4–23.
Arens, A. K., and Morin, A. J. S. (2016). Relations between teachers’ emotional exhaustion and students’ educational outcomes. J. Educ. Psychol. 108:800. doi: 10.1037/edu0000105
Bradshaw, C. P., Waasdorp, T. E., and Leaf, P. J. (2012). Effects of school-wide positive behavioral interventions and supports on child behavior problems. Pediatrics 130, e1136–e1145.
Briesch, A. M., Chafouleas, S. M., Neugebauer, S. R., and Riley-Tillman, T. C. (2013). Assessing influences on intervention implementation: revision of the usage rating profile-intervention. J. Sch. Psychol. 51, 81–96. doi: 10.1016/j.jsp.2012.08.006
Center on PBIS (2022). Tier 2. Positive Behavioral Interventions & Supports. Downers Grove, IL: Center on PBIS.
Chafouleas, S. M., Riley-Tillman, T. C., and Christ, T. J. (2009). Direct behavior rating (DBR): an emerging method for assessing social behavior within a tiered intervention system. Assess. Effect. Intervent. 34, 195–200. doi: 10.1177/1534508409340391
Collier-Meek, M. A., Johnson, A. H., Sanetti, L. H., and Minami, T. (2019a). Identifying critical components of classroom management implementation. Sch. Psychol. Rev. 48, 348–361.
Collier-Meek, M. A., Sanetti, L. M., and Boyle, A. M. (2019b). Barriers to implementing classroom management and behavior support plans: an exploratory investigation. Psychol. Sch. 56, 5–17.
Corbin, C. M., Alamos, P., Lowenstein, A. E., Downer, J. T., and Brown, J. L. (2019). The role of teacher-student relationships in predicting teachers’ personal accomplishment and emotional exhaustion. J. Sch. Psychol. 77, 1–12. doi: 10.1016/j.jsp.2019.10.001
Damschroder, L. J., Aron, D. C., Keith, R. E., Kirsh, S. R., Alexander, J. A., and Lowery, J. C. (2009). Fostering implementation of health services research findings into practice: a consolidated framework for advancing implementation science. Implement. Sci. 4, 1–15. doi: 10.1186/1748-5908-4-50
Daniels, B., Briesch, A. M., Volpe, R. J., and Owens, J. S. (2020). Content validation of direct behavior rating multi-item scales for assessing problem behaviors. J. Emotion. Behav. Disord. 29, 71–82. doi: 10.1177/1063426619882345
Fabiano, G. A., Vujnovic, R. K., Pelham, W. E., Waschbusch, D. A., Massetti, G. M., Pariseau, M. E., et al. (2010). Enhancing the effectiveness of special education programming for children with Attention Deficit Hyperactivity Disorder using a daily report card. Sch. Psychol. Rev. 39, 219–239. doi: 10.1080/02796015.2010.12087775
Farley-Ripple, E., and Buttram, J. (2015). The development of capacity for data use: the role of teacher networks in an elementary school. Teach. College Rec. 117, 1–34.
Field, K. (2022). Under New Laws, Some Teachers Worry Supporting LGBTQ Students will Get them Sued or Fired. New York, NY: USA Today.
Fimian, M. J., and Fastenau, P. S. (1988). The validity and reliability of the teacher stress inventory: a re-analysis of aggregate data. J. Organ. Behav. 11, 151–157.
Fimian, M. J., and Krupicka, W. M. (1987). Occupational stress and receipt of professional counseling in special education. Percept. Mot. Skills 65, 995–999. doi: 10.2466/pms.1987.65.3.995
Girio, E. L., and Owens, J. S. (2009). Teacher acceptability of evidence-based and promising treatments for children with attention-deficit/hyperactivity disorder. Sch. Ment. Health 1, 16–25. doi: 10.1007/s12310-008-9001-6
Girio-Herrera, E., Egan, T. E., Owens, J. S., Evans, S. W., Coles, E. K., Holdaway, A. S., et al. (2021). Teacher Ratings of acceptability of a daily report card intervention prior to and during implementation: relations to implementation integrity and student outcomes. Sch. Ment. Health 13, 69–83. doi: 10.1007/s12310-020-09400-y
Glasgow, R. E., Harden, S. M., Gaglio, B., Rabin, B., Smith, M. L., Porter, G. C., et al. (2019). RE-AIM planning and evaluation framework: adapting to new science and practice with a 20-year review. Front. Public Health 7:64. doi: 10.3389/fpubh.2019.00064
Goodman, R. (2001). Psychometric properties of the strengths and difficulties questionnaire (SDQ). J. Am. Acad. Child Adolesc. Psychiatry 40, 1337–1345.
Greene, R. W., Beszterczey, S. K., Katzenstein, T., Park, K., and Goring, J. (2002). Are students with ADHD more stressful to teach? J. Emotion. Behav. Disord. 10, 79–89.
Harrison, J. R., Kwong, C., Evans, S. W., Peltier, C., Mathews, L., and Chatman, T. (2020). Game-based self-management: addressing inattention during independent reading and written response. J. Appl. Sch. Psychol. 36, 38–61.
Hatcher, R. L., and Gillaspy, J. A. (2006). Development and validation of a revised short version of the working alliance inventory. Psychother. Res. 16, 12–25. doi: 10.1080/10503300500352500
Hoglund, W. L. G., Klingle, K. E., and Hosan, N. E. (2015). Classroom risks and resources: teacher burnout, classroom quality and children’s adjustment in high needs elementary schools. J. Sch. Psychol. 53, 337–357. doi: 10.1016/j.jsp.2015.06.002
Holdaway, A. S., Hustus, C. L., Owens, J. S., Evans, S. W., Coles, E. K., Egan, T. E., et al. (2020). Incremental benefits of a daily report card over time for youth with disruptive behavior: replication and extension. Sch. Ment. Health 12, 507–522. doi: 10.1007/s12310-020-09375-w
Hustus, C., Owens, J. S., Volpe, R., and Briesch, A. (2020). Treatment sensitivity of direct behavior rating multi-item scales in the context of a daily report card intervention. J. Emotion. Behav. Disord. 28, 29–42. doi: 10.1177/1063426618806281
Institute of Education Sciences [IES] (2022). School Responses to COVID19. Washington, DC: Institute of Education Sciences.
Johnson, S., Cooper, C., Cartwright, S., Donald, I., Taylor, P., and Millet, C. (2005). The experience of work-related stress across occupations. J. Manag. Psychol. 20, 178–187. doi: 10.1108/02683940510579803
Kumm, S., Talbott, E., and Jolivette, K. (2021). A technology-based self-monitoring intervention for secondary students with high-incidence disabilities. J. Spec. Educ. Technol. 36, 141–151.
Larson, M., Cook, C. R., Fiat, A., and Lyon, A. R. (2018). Stressed teachers don’t make good implementers: examining the interplay between stress reduction and intervention fidelity. Sch. Ment. Health 10, 61–76. doi: 10.1007/s12310-018-9250-y
Long, A. C., Sanetti, L. M. H., Collier-Meek, M. A., Gallucci, J., Altschaefl, M., and Kratochwill, T. R. (2016). An exploratory investigation of teachers’ intervention planning and perceived implementation barriers. J. Sch. Psychol. 55, 1–26.
Matta, M., Volpe, R. J., Briesch, A. M., and Owens, J. S. (2020). Five direct behavior rating multi-item scales: sensitivity to the effects of classroom interventions. J. Sch. Psychol. 81, 28–46. doi: 10.1016/j.jsp.2020.05.002
McLennan, J. D., Owens, J. S., Haines-Saah, R., Mitchell, S., and Hustus, C. (2020). Understanding barriers to teacher use of a daily report card intervention aided by online implementation support. Sch. Ment. Health 12, 826–840. doi: 10.1007/s12320-020-09389-4
McLeod, B. D., Sutherland, K. S., Martinez, R. G., Conroy, M. A., Snyder, P. A., and Southam-Gerow, M. A. (2017). Identifying common practice elements to improve social, emotional, and behavioral outcomes of young children in early childhood classrooms. Prevent. Sci. 18, 204–213. doi: 10.1007/s11121-016-0703-y
Mixon, C. S., Owens, J. S., Hustus, C., Serrano, V. J., and Holdaway, A. S. (2019). Evaluating the impact of online professional development on teachers’ use of a targeted behavioral classroom intervention. Sch. Ment. Health 11, 115–128. doi: 10.1007/s10566-018-9463-0
National Center for Education Statistics (2022a). Enrollment Rates of Young Children. Condition of Education. Washington, DC: U.S. Department of Education, Institute of Education Sciences.
National Center for Education Statistics (2022b). Public School Enrollment. Condition of Education. Washington, DC: U.S. Department of Education, Institute of Education Sciences.
National Education Association (2022). NEA Survey: Massive Staff Shortages in Schools Leading to Educator Burnout; Alarming Number of Educators Indicating they Plan to Leave Profession. Washington, DC: National Education Association.
Nisar, H., Elgin, D., Bradshaw, C., Dolan, V., Frey, A., Horner, R., et al. (2022). Promoting social and behavioral success for learning in elementary schools: Practice recommendations for elementary school educators, school and district administrators, and parents. Arlington, TX: 2M Research Services.
Oberle, E., and Schonert-Reichl, K. A. (2016). Stress contagion in the classroom? The link between classroom teacher burnout and morning cortisol in elementary school students. Soc. Sci. Med. 159, 30–37. doi: 10.1016/j.socscimed.2016.04.031
Owens, J. S., Coles, E. K., and Evans, S. W. (2014). “The role of teacher knowledge, skills, and beliefs in the implementation of classroom management skills,” in Proceedings of the Annual School Mental Health Research Summit, Pittsburgh, PA. doi: 10.1186/s13034-022-00470-1
Owens, J. S., Coles, E. K., Evans, S. W., Himawan, L. K., Girio-Herrera, E., Holdaway, A. S., et al. (2017). Using multi-component consultation to increase the integrity with which teachers implement behavioral classroom interventions: a pilot study. Sch. Ment. Health 9, 218–234. doi: 10.1007/s12310-017-9217-4
Owens, J. S., Holdaway, A. S., Serrano, V. J., Himawan, L. K., Watabe, Y., Storer, J., et al. (2016). Screening for social, emotional, and behavioral problems at Kindergarten entry: the utility of two teacher rating scales. Sch. Ment. Health 8, 319–331. doi: 10.1007/s12310-016-9176-1
Owens, J. S., Holdaway, A. S., Zoromski, A. K., Evans, S. W., Himawan, L. K., Girio-Herrera, E., et al. (2012). incremental benefits of a daily report card intervention over time for youth with disruptive behavior. Behav. Ther. 43, 848–861. doi: 10.1016/j.beth.2012.02.002
Owens, J. S., Hustus, C. L., and Evans, S. W. (2020). “The daily report card intervention: summary of the science and factors affecting implementation,” in Handbook of Research on Emotional & Behavioral Disabilities: Interdisciplinary Developmental Perspectives on Children and Youth, eds T. Farmer, M. Conroy, B. Farmer, and K. Sutherland (London: Routledge Press), 371–385.
Owens, J. S., Margherio, S. M., Lee, M., Evans, S. W., Crowley, D. M., Coles, E. K., et al. (2021). Cost-effectiveness of consultation for a daily report card intervention: comparing in-person and online implementation strategies. J. Educ. Psychol. Consult. 31, 382–409. doi: 10.1080/10474412.2020.1759428
Owens, J. S., McLennan, J. D., Hustus, C. L., Haines-Saah, R., Mitchell, S., Mixon, C. S., et al. (2019). Leveraging technology to facilitate teachers’ use of a targeted classroom intervention: evaluation of the daily report card.online (DRC.O) system. Sch. Ment. Health 11, 665–677. doi: 10.1007/s12710-019-09320-6
Owens, J. S., Murphy, C. E., Richerson, L., Girio, E. L., and Himawan, L. K. (2008). Science to practice in underserved communities: the effectiveness of school mental health programming. J. Clin. Child Adolesc. Psychol. 37, 434–447. doi: 10.1080/15374410801955912
Parker, R. I., Vannest, K. J., and Davis, J. L. (2011). Effect size in single-case research: a review of nine nonoverlap techniques. Behav. Modif. 35, 303–322. doi: 10.1177/0145445511399147
Pas, E. T., Ryoo, J. H., Musci, R. J., and Bradshaw, C. P. (2019). A state-wide quasi-experimental effectiveness study of the scale-up of school-wide positive behavioral interventions and supports. J. Sch. Psychol. 73, 41–55. doi: 10.1016/j.jsp.2019.03.001
Pyle, K., and Fabiano, G. A. (2017). Daily report card intervention and attention deficit hyperactivity disorder: a meta-analysis of single-case studies. Exception. Child. 83, 378–395. doi: 10.1177/0014402917706370
Richards, J. (2012). Teacher stress and coping strategies: a national snapshot. Educ. Forum 76, 299–316. doi: 10.1080/00131725.2012.682837
Riden, B. S., Taylor, J. C., Scheeler, M. C., Lee, D. L., and McCloskey, A. V. (2021). The effects of an electronic daily behavior report card on student’s challenging behavior. J. Spec. Educ. Technol. 36, 127–140. doi: 10.1186/s13054-016-1208-6
Scheibel, G., Zimmerman, K. N., and Wills, H. P. (2022). Increasing on-task behavior using technology-based self-monitoring: a meta-analysis of i-connect. J. Spec. Educ. Technol. [Epub ahead of print].
Staff, A. I., van den Hoofdakker, B. J., van der Oord, S., Hornstra, R., Hoekstra, P. J., Twisk, J. W. R., et al. (2021). Effectiveness of specific techniques in behavioral teacher training for childhood ADHD: a randomized controlled microtrial. J. Clin. Child Adolesc. Psychol. 50, 763–779. doi: 10.1080/15374416.2020.1846542
Tschannen-Moran, M., and Hoy, A. W. (2001). Teacher efficacy: capturing an elusive construct. Teach. Teach. Educ. 17, 783–805. doi: 10.1016/S0742-051X(01)00036-1
Tschannen-Moran, M., and McMaster, P. (2009). Sources of self-efficacy: four professional development formats and their relationship to self-efficacy and implementation of a new teaching strategy. Element. Sch. J. 110, 228–245.
United Nations Educational, Scientific, and Cultural Organization (2021). [Interactive Map of Total Duration of School Closures across the Globe]. Paris: United Nations Educational, Scientific, and Cultural Organization.
Vannest, K. J., Davis, J. L., Davis, C. R., Mason, B. A., and Burke, M. D. (2010). Effective intervention for behavior with a daily behavior report card: a meta-analysis. Sch. Psychol. Rev. 39, 654–672. doi: 10.1080/02796015.2010.12087748
Vannest, K. J., and Ninci, J. (2015). Evaluating intervention effects in single-case research designs. J. Counsel. Dev. 93, 403–411. doi: 10.1002/jcad.12038
Williams, K. L., Noell, G. H., Jones, B. A., and Gansle, K. A. (2012). Modifying students’ classroom behaviors using an electronic daily behavior report card. Child Fam. Behav. Ther. 34, 269–289.
Yeo, W., Goh, A., and Tan, C. (2018). Efficacy of online daily behaviour report card to decrease off-task behaviour for adolescents with attention-deficit/hyperactivity disorder. Austral. J. Spec. Inclusive Educ. 42, 143–157.
Keywords: daily report card, technology, adoption, adaptation, implementation, classroom intervention, teacher, DRC
Citation: Owens JS, Lee M, Eackles K, Medina D, Evans SW and Reid J (2022) Exploring teacher adoption, adaptation, and implementation of a daily report card intervention when using the daily report card online platform. Front. Educ. 7:981916. doi: 10.3389/feduc.2022.981916
Received: 29 June 2022; Accepted: 25 August 2022;
Published: 30 September 2022.
Edited by:
Gianluca Merlo, Institute of Didactic Technologies, Department of Human and Social Sciences, Cultural Heritage (CNR), ItalyReviewed by:
Davide Taibi, Institute of Didactic Technologies, Department of Human and Social Sciences, Cultural Heritage (CNR), ItalyNicola Lo Savio, Istituto Tolman, Italy
Copyright © 2022 Owens, Lee, Eackles, Medina, Evans and Reid. This is an open-access article distributed under the terms of the Creative Commons Attribution License (CC BY). The use, distribution or reproduction in other forums is permitted, provided the original author(s) and the copyright owner(s) are credited and that the original publication in this journal is cited, in accordance with accepted academic practice. No use, distribution or reproduction is permitted which does not comply with these terms.
*Correspondence: Julie Sarno Owens, owensj@ohio.edu