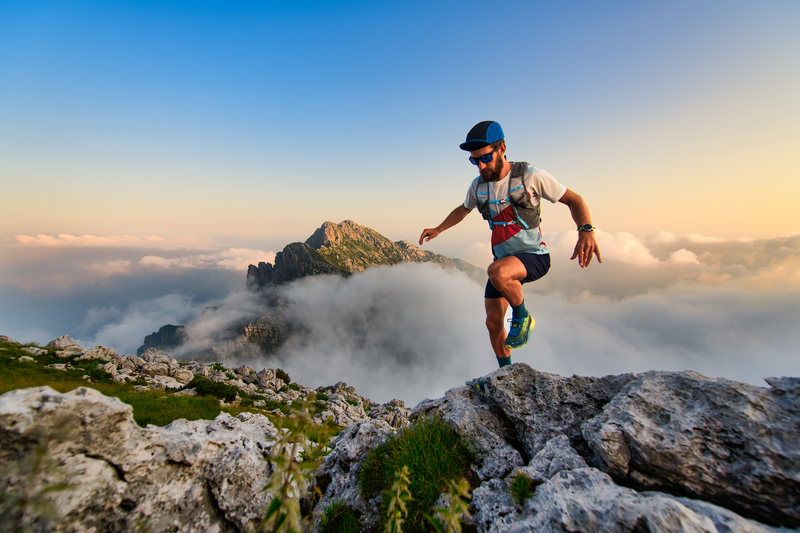
94% of researchers rate our articles as excellent or good
Learn more about the work of our research integrity team to safeguard the quality of each article we publish.
Find out more
ORIGINAL RESEARCH article
Front. Educ. , 07 December 2022
Sec. Teacher Education
Volume 7 - 2022 | https://doi.org/10.3389/feduc.2022.977788
This article is part of the Research Topic Evidence-Informed Reasoning of Pre- and In-Service Teachers View all 16 articles
In recent years, the call for an evidence-based practice has become more prevalent for educational professionals. However, educational professionals are rarely prepared for evidence-based practice; for example, teachers are not prepared to use and, thus, rarely do use scientific evidence in planning lessons. The knowledge and skills in appraising scientific literature, the basis of evidence-based practice, needs to be trained as early in professional education as possible. An effective training might start in university education of future educational professionals, engaging them in learning activities that foster their understanding of criteria that are used in appraising scientific literature and the skill to do so. However, we know little about the effect of different learning activities such as constructive or interactive learning in this context. Thus, this study investigated the influence of constructive versus interactive learning activities in the context of an intervention facilitating knowledge and skills in appraising scientific literature. This experimental study used a pre-posttest between-subject design with 105 participants. The students learned to evaluate scientific literature in an online learning environment. The results show that the inclusion of interactive versus constructive learning activities did not explain students’ learning in the intervention. The results implicate that the learning activities might not play a major role with learning contents such as evidence-based practice. However, the gain in skills and knowledge from pre- to posttest shows promising achievements in preparing future educational professionals in their evidence-based practice.
Reasoning with scientific evidence to solve practical problems is one of the core competences in a knowledge society (Fischer et al., 2014). Specifically, professionals are expected to understand the development of knowledge in their field and to incorporate new knowledge into their practice after a careful evaluation of its origin. The so-called evidence-based practice is already established in medicine (Sackett et al., 1996) and is considered one of its most important milestones (Dickersin et al., 2007). Decisions in medical care of individual or public health decisions are commonly expected to be made with “conscientious, explicit and judicious use of current best evidence” (Sackett, 1997, p. 3).
For the past 20 years, the call for education to follow disciplines such as medicine and to place more importance on scientific evidence in practical decisions has been growing (Slavin, 2008; Cook et al., 2012; Bromme et al., 2014; Brown and Zhang, 2016; Cain, 2016; Stark, 2017; Thomm et al., 2021). Educational professionals, especially teachers, are increasingly expected to identify relevant research, systematically evaluate their findings and implement evidence-based practices in classrooms (Detrich and Lewis, 2013).
One central aspect of evidence-based practice is the critical appraisal of the validity and applicability of the evidence (Sackett, 1997). Consequently, there is an effort to facilitate appraisal of scientific literature in, for example, instructional interventions. So far, studies investigating such interventions are mainly conducted in the field of medicine (e.g., Bradley et al., 2005; Kulier et al., 2012; Reviriego et al., 2014; Molléri et al., 2018) while there is little evidence for fostering appraisal of scientific literature in educational professionals. Moreover, studies investigating one group of educational professionals, teachers, found that they rarely use scientific evidence in professional decisions (Hetmanek et al., 2015a) but rather refer to anecdotal evidence (Menz et al., 2021). Similarly, pre-service teachers rather choose anecdotal evidence than scientific evidence as information source when giving advice for teaching (Kiemer and Kollar, 2021).
Thus, in order to meet the call for more evidence-based practice in education (e.g., Slavin, 2008; Cook et al., 2012; Bromme et al., 2014; Brown and Zhang, 2016; Cain, 2016; Stark, 2017; Thomm et al., 2021), future professionals in education need to be better prepared to use scientific evidence. This paper presents an approach to target one central aspect of using scientific evidence, the critical appraisal of scientific literature.
Based on the definition of evidence-based medicine as “the conscientious, explicit, and judicious use of current best evidence in making decisions about the care of individual patients” (Sackett et al., 1996, p. 71), we conceptualize evidence-based practice, independent from a specific discipline, as the conscientious, explicit, and judicious use of current best evidence in making decisions about one’s field of expertise. Thus, educators’ evidence-based practice will include the process of conscientiously, explicitly, and judiciously using the current best evidence in educational research in deciding, for example, on a teaching method in planning a lesson. One central task in working as a teacher is planning lessons. While experienced teachers do not need to plan every lesson from scratch, new learning goals or settings lead to teachers facing the decision on how to design a new lesson. In an evidence-based approach, at least some of these decisions would be made based on educational research that could provide a helpful insight “to identify the practices most likely to bring about positive student outcomes” (Cook et al., 2012, p. 498). In order to make evidence-based decisions in lesson planning, the educational professional starts by carefully analyzing the potential influencing factors, such as the learning goal of the lesson and characteristics of their pupils. The next steps include the search for potential evidence, appraising and selecting relevant, high quality evidence, and finally critically appraising the evidence and developing a lesson plan based on the insights gained from the evidence (see Trempler et al., 2015). While most educational professionals, for example teachers, are not able to spend the time needed to conduct a thorough search and evaluation of existing educational evidence in planning all educational interventions, these practices described above need to be integrated more often into the everyday practice of educational professionals to meet the call for more evidence-based practice in education.
A central aspect in this process is the critical appraisal of scientific literature (Sackett, 1997). In critically appraising scientific literature, the validity and usefulness of the evidence is evaluated (Sackett, 1997). For educational research, critically appraising the validity and usefulness of scientific literature can be adapted to appraising the quality of the research (validity) and the relevance (usefulness) of the research for a given problem (Hetmanek, 2014). Critically appraising the validity and usefulness of scientific literature requires (a) knowledge in criteria that are used in the appraisal as well as the (b) skill to correctly appraise the evidence. Research on sourcing and information integration from multiple resources includes further information such as the author of the text or the source of the evidence (e.g., Bråten et al., 2011; Thomm and Bromme, 2016). The evaluated information can be differentiated into first- and second-hand evaluation; in first-hand evaluation a claim is evaluated directly, while the second-hand evaluation targets the source, for example whether a claim or evidence is authored by a trustworthy expert (Bromme et al., 2010). Since first-hand evaluation requires prior domain-specific knowledge and skills, second-hand evaluation often is the necessary approach for laypeople (Bromme et al., 2010). While future educational professionals could also benefit from instruction in second-hand evaluation, because second-hand information such as trustworthiness of the source might also play a relevant role in educational professionals’ evidence-based practice, this paper will focus on first-hand evaluation. Educational professionals possess knowledge and skills related to education, learning, and teaching; thus, they are not laypersons with regard to content knowledge and skills in the field of education. Furthermore they are, as for example defined by standards for teachers and teacher education in many countries, expected to know methods of educational research (KMK, 2019), know basics in research, and exhibit research literacy (Révai, 2018). Future educational professionals need not only to develop professional knowledge and skills in education, but also a basic understanding of the scientific background of education and the skill to appraise this evidence. It is therefore beneficial for future educational professionals to learn how to directly evaluate scientific evidence from their area of expertise: education.
There are several approaches that could help derive criteria to evaluate scientific evidence, of which we will focus on two: the QUESTS dimensions teaching practices in medicine (Harden et al., 1999) and an instrument measuring the appraisal of scientific literature in evidence-based practice (Hetmanek, 2014; Trempler et al., 2015). QUESTS is an acronym that stands for the six dimensions that play a role in evaluating evidence in medical education: Quality, Utility, Extent, Strength, Target, and Setting. Quality refers to the rigor of the study design, with randomized controlled trials gaining more points than case studies or professional experience. The utility refers to the extent to which the object of investigation, for example an intervention, can be transferred to a different setting. The extent of the available evidence refers to the difference between multiple studies with similar outcomes or meta-analyses in comparison to single studies. The strength of a study refers to strength of the effect(s) found in a study. Furthermore, the dimensions on target and setting address the validity of a certain target or outcome and setting (Harden et al., 1999). The instrument measuring the appraisal of scientific literature in evidence-based practice (Hetmanek, 2014; Trempler et al., 2015) also includes the dimension of the validity of the target and the setting, differentiated in the dimension of quality between the rigor in conducting the study, and statistical rigor. The authors also added the fit of the intervention, the applicability of the intervention, appropriate measurement of the target, and fit of the participants with a given educational decision (Hetmanek, 2014; Trempler et al., 2015). In summary, these sets of criteria include relevance criteria and quality criteria. Relevance criteria target the fit between an educational decision and the evidence that is currently evaluated with regards to the teaching method, the learning objective, the participants, and the setting. Quality criteria target (a) whether the reported learning outcome is measured with an objective, reliable, and face valid test, (b) the statistical power, and (c) the design of the study (Hetmanek, 2014; Trempler et al., 2015). The criteria used to appraise scientific evidence might vary substantially between domains (see for discussion, e.g., Fischer et al., 2018); thus, these criteria are specific to critically appraising scientific research literature in education.
The cognitive processing of critically appraising scientific literature has not yet been specified in detail. Our conceptualization of the skill to critically appraise scientific literature is based on the model of information problem solving (e.g., Brand-Gruwel et al., 2005, 2009) that describes a process that is structurally similar to critically appraising scientific literature. Information problem solving describes skills needed to solve a problem by searching for information (for example on the internet), scan and process the information, and combine the information at the end of the process to solve a problem. Most important for this research is the fourth process of the information problem solving described as process information, during which one gains a deeper understanding of a piece of information and, as described in the subskill selecting, uses criteria to judge the usefulness and quality of the information (Brand-Gruwel et al., 2009). Selecting describes a skill that is central to the process of appraising scientific literature. Similarly to the selection of information on the internet, it is important in the critical appraisal of scientific literature to determine the usefulness of the evidence and the quality of information by judging how relevant a study is and how well it was conducted (Sackett, 1997; Hetmanek, 2014; Trempler et al., 2015). Appraising scientific literature provides the additional challenge that the information is given in the specific format of an empirical research article. Furthermore, the set of criteria used to appraise scientific evidence in terms of its relevance and quality is rather complex: For example, the fit between learning objectives indicates low relevance if one’s own educational decision aims at facilitating a cognitive skill and if the evaluated study reports motivational outcome measures. In this example, one needs to be able to identify the learning objective in the educational decision as well as the learning objective or measurement of the dependent variable in the empirical research article, and come to the correct inference that there is no overlap between them. As a second example, the design of a study is considered to be of high quality if the teaching method that is investigated in the study is varied between conditions while there are no further confounding variables that also vary between conditions. Here, one needs to know what an unconfounded design is and be able to detect whether the design described in an empirical research article only varies the independent variable between conditions or if there are confounding factors.
Evaluating information in an information problem solving process on the internet is difficult for students of all ages and, thus, rarely criteria-led (summarized by Brand-Gruwel et al., 2009). An analysis of think-aloud data showed that secondary students rarely appraise sources during information problem solving, and if they did, they only used a small selection of criteria in only a small percentage of cases (Walraven et al., 2009). Thus, it is not surprising that higher education students exhibit difficulties when asked to appraise empirical research articles in education (Trempler et al., 2015). Research showed that support in form of scaffolding (Raes et al., 2012), whole-task trainings (Frerejean et al., 2019), or long-term intervention programs (Argelagos and Pifarré, 2012) can support learners in their information problem solving. Interventions in related fields showed the potential of fostering scientific and evidence-based reasoning: For example, a short intervention teaching evidence-based medicine showed to improve medical students’ search for scientific literature (Gruppen et al., 2005), educational science students’ scientific argumentation was improved by engaging them in activities around an elaboration tool (Stark et al., 2009), and an intervention teaching heuristics in appraising and using scientific evidence to pre-service teachers fostered their evidence-based argumentation (Wenglein et al., 2015).
While there is little research investigating interventions teaching critical appraisal of scientific evidence in education, we expect higher education students to lack knowledge of appropriate criteria as well as the skill to apply these criteria in appraising literature. In line with the findings of intervention studies on information problem solving as well as studies in interventions in scientific reasoning, we expect higher education students to benefit from a training of their knowledge and skill in appraising scientific research articles.
In training critical appraisal of scientific literature, it might be advantageous to facilitate high cognitive engagement in learners. The so-called ICAP framework proposes that the way learners engage with learning material or the instruction influences their cognitive engagement and thereby their learning outcomes (Chi, 2009; Chi and Wylie, 2014; Chi et al., 2018). The hypothesis is based on a taxonomy of learning activities, ranging from passive, to active, to constructive, to interactive learning activities. Students learning passively receive information from teachers or learning material without further engaging with the information. Active learners engage with information to some degree by, for example, repeating or rehearsing it, taking notes, highlighting text, or stopping a video. Constructive learning activities are those activities in which students generate learning outputs, additional to the information given to them, for example in the form of formulating self-explanations, generating inferences, or drawing a concept map. Learning interactively describes at least two participants that take turns in a constructive learning process. In the ICAP framework, active learning activities are hypothesized to exceed passive learning activities because they require focused attention and, thus, more cognitive engagement by the learners than passive learning. Constructive learning activities are hypothesized to exceed active learning activities because they prompt a more active construction of individual knowledge. Interactive learning activities are hypothesized to exceed constructive learning activities because they require learners to frequently update their mental model because of the ongoing change in the information discussed (Chi and Wylie, 2014). This framework links the learners’ activities to the cognitive processes that they are engaged in during learning, it does not directly focus on the cognitive activity of a learner (which is distinct from other concepts using similar vocabulary such as the “cognitive activity” describing a state of deep learning, see Klieme and Rakoczy, 2008). There is some evidence about the hierarchy of learning activities, suggested by Chi and colleagues, based on the analysis of prior research (Chi, 2009; Chi and Wylie, 2014; Chi et al., 2018). Based on the publications of Chi and colleagues, some intervention studies investigated the effect of instructions aiming at different learning activities and found new insights into the effect of learning activities: Adding an instruction on how to interact for short periods of time with peers during a physics lecture was found to improve students’ conceptual knowledge about Newtonian dynamics concepts, but constructive instruction or a combination of constructive and interactive instructions were not found to be more beneficial than passive instruction (Henderson, 2019). An interactive learning activity also showed to foster better conceptual understanding of material science and engineering than a constructive learning activity (Menekse and Chi, 2019).
The beneficial role of interactive learning in comparison to constructive learning is called into question by meta-analyses that investigated whether constructive or interactive activities could be found in different instructional interventions: A meta-analysis investigating the effect of socio-cognitive scaffolding on domain-specific knowledge and collaboration skills included the presence of interactive prompts in a moderator analysis and found no significant difference for domain-specific knowledge nor collaboration skills (Vogel et al., 2017). Similarly, a meta-analysis on constructive and interactive instructions fostering scientific reasoning found no significant difference between the interventions on scientific reasoning outcome measures (Engelmann et al., 2016). Yet, a meta-analysis of interventions studies fostering domain knowledge with a preparing-to-teach and teaching intervention found that an interaction, and even the expectation of an interaction, was associated with a higher effect size than non-interactive teaching activities (Kobayashi, 2019). A meta-analysis of learning with videos found interactivity to be a significant moderator: There was no learning benefit found for interventions in which the control condition included more interactivity than the experimental condition and a particularly high effect on learning found for videos with interactive context (Noetel et al., 2021). Thus, while there is a body on literature supporting the ICAP hypothesis, the meta-analytic evidence found in different contexts indicates that the effect of learning activities on different outcome measures needs to be investigated more thoroughly.
Not only the outcome measures vary between studies, Chi and Wylie (2014) also found learning activities to be embedded in different learning situations and instructional approaches, such as individual or collaborative note taking, individual or collaborative building of concept maps, explaining examples or explaining own versus others answers. Beyond the investigation of learning activities, self-explaining in learning with examples has been shown to facilitate argumentation (Schworm and Renkl, 2007) and learning from texts in higher education teaching (Lachner et al., 2021), while there is also some research showing no benefit of self-explanations, for example in teaching critical thinking skills (van Peppen et al., 2018). However, a meta-analysis found a medium effect size of self-explanation prompts on learning (Bisra et al., 2018), providing evidence for the beneficial effect of self-explanations.
Self-explanations are most commonly used in problem solving, text comprehension tasks, or example-based learning (Bisra et al., 2018). Since example-based learning has been found to be beneficial in early skill acquisition (Renkl, 2014), we will focus on self-explanations in example-based learning. Example-based learning provides learners with the solution of a given problem and commonly the steps that lead to the solution (Renkl, 2014). Learning from examples is more effective if the learners self-explain the solution to a problem (summarized by Renkl, 2014). In the analysis of learning activities by Chi and Wylie (2014), self-explanations were often found to be constructive because they, for example, asked learners to explain steps in a worked-example to themselves. Comparisons between learning activities were mostly found between these constructive learning activities and passive activities, such as self-explaining versus rereading or explaining others’ solution versus just watching the solution. Alternatively, they were also found between constructive learning activities and interactive learning activities, such as explaining alone versus explaining with a partner (Chi and Wylie, 2014).
In the present study, we aim at testing the effect of constructive versus interactive learning activities in the context of an intervention that facilitates critical appraisal of scientific literature. Thus, the development of the intervention was guided by instructional approaches in which learning activities (Chi and Wylie, 2014) were investigated: The effect of different learning activities was found in intervention studies in which learning was implemented with note taking, concept mapping, or self-explaining (Chi and Wylie, 2014). Since we planned to integrate the learning activities in our practice tasks that was mainly aimed at early skill acquisition, we developed these tasks to ask the participants to explain a model solution to themselves or a learning partner; i.e. a version of example-based learning, (see Renkl, 2014), that only provided the solution not the steps that led to the solution. Thus, we embedded the learning activities in a type of task that has shown to foster learning outcomes similar to the ones in this study and provided a fruitful learning environment to investigate the effect of different learning activities in prior studies (Chi and Wylie, 2014). Furthermore, we designed this intervention to be integrated into a scenario that models a realistic situation in which educational professionals need to make evidence-based decisions (Hetmanek, 2014; Trempler et al., 2015).
There is evidence for the advantage of interactive learning activities in comparison to constructive learning activities (Chi and Wylie, 2014). However, the superiority of interactive activities over constructive activities has not yet been replicated in some fields related to the field of this study: For example, Engelmann et al. (2016) showed similar effect sizes in learning outcomes for interventions with constructive versus interactive learning activities. Similarly, Vogel et al. (2017) were not able to establish a difference in domain knowledge between interventions that prompted interactivity and those that did not. Thus, this study investigates the role of interactive versus constructive learning activities in supporting students in learning to appraise scientific evidence. The content of the intervention in this study was chosen since, in comparison to research in medicine (e.g., Bradley et al., 2005; Kulier et al., 2012; Reviriego et al., 2014; Molléri et al., 2018), there is little research on fostering evidence-based practice in education, for example on teachers making decisions in lesson planning based on scientific evidence. The existing literature supports the claim that educational professionals need more training in doing so: Prior research showed that teachers rarely use scientific evidence in professional decisions (Hetmanek et al., 2015a) and rather refer to anecdotal evidence (Kiemer and Kollar, 2021; Menz et al., 2021). Thus, this study also provides a first insight into learning the critical appraisal of scientific literature.
Our research questions are as follows:
Research question 1: To what extent does an intervention with interactive learning activities advance knowledge about scientific criteria in comparison to an intervention with constructive learning activities?
Research question 2: To what extent does an intervention with interactive learning activities advance the skill in critical appraisal of scientific literature in comparison to an intervention with constructive learning activities?
We hypothesized that the interactive learning activities facilitate a higher level of cognitive activities during the learning process in comparison to constructive learning activities and, therefore, lead to a higher gain in knowledge and skills (Chi and Wylie, 2014).
The sample size was calculated before data collection started, using the software G*Power 3 (Faul et al., 2007). The calculation was based on a target power of 80% and a medium effect size (d = 0.5) for the within-between interaction effect of an ANOVA with repeated measures and between-factor design. The estimation of the expected effect size was based on a medium effect (d = 0.64) found between an interactive and a constructive learning condition in an intervention study (see Menekse et al., 2013). In the condition with an interactive approach, the unit of analysis was a pair of learners and in the condition with the constructive approach, the unit of analysis was the individual learner. Thus, we aimed at a minimal sample size of 102 participants.
Participants were recruited via printed notices that were posted around the university campus and received 20 Euro for their participation. All participants met the following criteria: They were students at a university in their bachelor’s or master’s studies of educational sciences, psychology, teacher training, or an equivalent subject, who have not yet received a master’s degree. 105 students participated in this study (Mage = 24.50 years, SD = 4.03; 84% female), 49 students in teacher training, 34 psychology students, 14 educational sciences students, and 8 other students. The distribution of the participants’ study programs was similar in both conditions; we found no indicator of a systematic differences between knowledge or skills in participants from different study programs. No participant needed to be excluded from the data analysis.
This experimental study was conducted in a between-subject repeated measures design. The participants were randomly assigned to one of the conditions and they learned interactively (n = 56) or constructively (n = 49) during an intervention about reading scientific literature. The random assignment was based on a 2:1 ratio, since the unit of analysis in the interactive condition was the pair of learners and the unit of analysis in the constructive condition was the individual learner. However, participants were assigned to the condition before the appointments for the data collections were set (because only one condition could be implemented for each appointment) and the attendance was greater in the constructive condition; thus, the distribution of participants was unequal in favor of the constructive condition. The pre- and posttests were parallel tests. Which version participants received as pretest and which as posttest was counterbalanced. Due to organizational reasons, 43 participants received one order, 62 participants the other order of tests. Data indicated that the order of the tests was not relevant for the outcome.
The data collection took place at computer labs of a German university. At each data collection, a maximum of eight participants could take part in the session. The session took approximately 114 min to complete. An overview of the procedure of the experiment can be found in Figure 1.
The whole data collection was set in a fictional scenario in which the participants were asked to imagine themselves to be an educational professional who needs to make two educational decisions in designing a lesson: (a) present some content themselves or use the jigsaw technique and (b) use correct and erroneous or only correct video examples. The participants were asked to appraise a set of four pieces of scientific evidence from educational research in order to make each of the decisions. The evidence from educational research was presented in the form of structured briefs of scientific literature. The scenario was adapted from a competence test measuring skills of information selection and appraisal of scientific research articles (Hetmanek, 2014; Trempler et al., 2015). The first decision constituted the pretest and was referenced in the intervention. The second decision constituted the posttest. With which decision (a or b) learners worked with in the pretest and with which they worked with in the posttest was counterbalanced (see above). For each decision participants were given a short description of the learning content of the lesson, characteristics of the students and the setting. In a next step, they were asked to appraise a set of four pieces of scientific literature and make a decision regarding the teaching method based on this evidence. The intervention itself was nested between the two decisions building upon the first decision to create an authentic reference point for the participants.
The procedure was similar in both conditions: In a first phase, the participants were introduced to the scenario, the first decision, and filled out the pretest by evaluating the pieces of evidence related to the first decision. The second phase included the intervention. In the third phase, the participants were introduced to the second decision and filled out the posttest by evaluating the pieces of evidence related to the second decision.
The intervention started with a written four-page introduction to the criteria used in appraising scientific evidence. The introduction included the following criteria:
– evaluating the relevance of the evidence with respect to
a. the fit between the instructional methods that are part of the decision to be made and the instructional methods investigated in the piece of evidence,
b. the fit between the learning goal in the decision to be made and the dependent variable in the piece of evidence,
c. the fit between the learners’ age or level of prior knowledge specified in the decision to be made and the age or level of prior knowledge of the participants in the piece of evidence, and
d. the fit between the setting in the decision to be made and the setting in the piece of evidence.
– evaluating the quality of the evidence was specified in
e. the quality of the measurement of the dependent variable,
f. the statistical robustness of the results, and
g. whether the effect found in the study could be attributed to the instructional method investigated in the piece of evidence.
For each criterion, the introduction provided a short description on how to evaluate the degree to which a piece of evidence is relevant and of high quality. Subsequently, the participants were presented with model solution of the appraisal of the first piece of evidence from their pretest given by a fictional character that was described as knowledgeable. All participants were asked to compare the model solution of each criterion to the description in the introduction text and explain to themselves or their learning partner (depending on the condition) which information in the piece of evidence led to the example appraisal and why the knowledgeable person came to this result. Since each pretest consisted of four pieces of evidence, the participants moved through four model solutions. The learning phase was limited to 50 minutes.
The whole experiment was conducted in an online learning environment, except for the written introduction to the appraisal criteria that was given to the participants on a sheet of paper at the beginning of the second phase and was taken from the participants at the end of the second phase, before the posttest was conducted.
The manipulation of the independent variable was realized during the intervention phase: (a) Participants in the interactive conditions were prompted to explain the model solutions to their learning partner. In order to make sure that the pairs actually learned interactively, they were alternately scaffolded to explain the model solutions to their learning partner or to question the solution provided by the partner. (b) Participants in the constructive condition were prompted to explain the model solution to themselves. All participants were asked to take notes.
The knowledge was measured with a test containing two open questions asking the participants to recall criteria to appraise the relevance and quality of scientific literature. The test was developed for this study. The answers given by the participants were saved in written form and were coded according to the following categories: For the first question regarding the relevance of a study, a point was given for mentioning the independent variable (e.g., “jigsaw technique,” “task,” “lesson”), the dependent variable (e.g., “measurement,” “learning goal”), the characteristic of the students (e.g., “prior knowledge,” “students”) and the setting (e.g., “situation,” “field of application”). During the coding process, we added one additional category: mentioning the research question or object of the investigation in the piece of evidence. We did so, because many participants mentioned this category instead of recalling the independent and dependent variable. One of the initial categories, coding whether participants mentioned the quality of a study as a criterion for the relevance of a study, was dropped because it could not be coded reliably. For the second question regarding the quality of a study, one point was given for mentioning the quality of the measurement (e.g., “objectivity,” “reliability”), the clear design (e.g., “randomizing participants,” “execution of the experiment,” “validity”), and the statistical significance and/or power (e.g., “number of participants,” “power,” “statistical significance”). One point was given for each aspect mentioned, creating a knowledge score ranging between zero and nine points. The first author coded all data and the second author coded 10% of the data after a coding training to test the objectivity of the coding process. The agreement between coders showed to be ranged from acceptable to very good for all included categories (Cohen’s kappa ranging from 0.77 to 1.00). The same scale was administered as pre- and posttest.
The skill in appraising scientific evidence was measured by asking the participants to appraise four pieces of evidence, each by evaluating the evidence on seven dimensions. Each dimension consisted of a multiple-choice item with four response options each (very high, somewhat high, somewhat low, and very low), one of which was correct. The items asked the participants to appraise different aspects of the scientific text, for example (translated into English) “The study described in the structured brief investigates an educational intervention that matches the educational intervention in my decision.” or “The study described in the structured brief uses appropriate tests for its performance measures.” The measured dimensions matched the criteria taught during the intervention. The test was adapted from a sub-scale of a test for evidence-based practice in education, developed by Hetmanek (2014) and Trempler et al. (2015). For each item, we saved the response that was selected by the participants. The responses were aggregated into a scale in which (a) a correct response option was counted as one point, (b) a false response option leaning into the same direction as the correct response option was counted as half a point, (c) a false response option leaning into the other direction as the correct response option was counted as zero points. Based on the 4 × 7 items, the aggregation resulted in a score ranging from zero to 28 points. The reliability of the scales was low to medium for the pretest (α = 0.53/0.54) and posttest (α = 0.45/0.64) for both versions of the test. However, we decided against selecting or discarding items to achieve a more homogeneous measure. Each of the items covers a different important aspect of critically appraising scientific literature, discarding one or more aspects of the test could have increased the Cronbach’s alpha of the scale but would have decreased the validity of the test. Parallel scales were administered as pre- and posttest containing the exact same phrasing, targeting different sets of literature in pre- and posttest.
The analyses reported in this article were registered at the Open Science Framework (Engelmann et al., 2018). The registration was done after data collection but before any analysis was conducted.
In analyzing the learning pairs of the interactive condition, one person of each pair was randomly chosen and their data was used for the analysis.
Bayesian repeated measures ANOVAs with a between-subjects factor were used with priors kept at standard values, examining the BF10, compared to the null model and investigating the effects across all models. The interpretation of the results was based on van den Bergh et al. (2020) and Wagenmakers et al. (2018a). The grouping variable (interactive condition versus constructive condition) was the independent variable in analyzing both research questions. The score in knowledge was the dependent variable for the first analysis, the score in the skill measurement was the dependent variable in the second analysis. Q-Q plots for both variables did not indicate non-normality. We decided to utilize a Bayesian approach in comparison to classical null hypothesis statistical testing because it provides the opportunity to quantify the evidence that the data provides for the null as well as the alternative hypothesis: “In the Bayesian framework, no special status is attached to either of the hypotheses under test” (Wagenmakers et al., 2018b, p. 46). We would also like to draw conclusions about outcomes that do not support our hypotheses. A Bayesian approach allows for that by providing evidence in favor of the null hypothesis, in favor of the alternative hypothesis, or neither (Wagenmakers et al., 2018b). The strength of the evidence is also provided (e.g., moderate evidence, strong evidence, very strong evidence) in comparison to an (arguably arbitrary) level of significance.
The sequential analyses add evidential trajectories, showing how the evidence for one of the hypotheses increases, decreases, or remains the same with each additional datapoint (Marsman and Wagenmakers, 2017). Thus, the sequential analyses also give additional information about the number of data points that were needed to reach a certain Bayes factor. Since the sequential analysis could not be conducted in the repeated measures design, the learning gain was calculated as the difference between pre- and posttest score and the sequential analysis was conducted using a Bayesian independent and paired sample t-test.
All tests were conducted using the software JASP, Version 0.13.1.0 (JASP Team, 2020). Additionally, Cohen’s d of all reported mean differences were calculated (Cohen, 1988).
Research question 1: To what extent does an intervention with interactive learning activities advance knowledge about scientific criteria in comparison to an intervention with constructive learning activities?
The descriptive values of knowledge are displayed in Table 1. The results of the Bayesian repeated measures analysis of variance can be found in Table 2 for the comparison of each model to the null model and Table 3 for the average across all models. The BFincl (the inclusion Bayes factors) in Table 3 are of particular interest because they indicate the amount of evidence for the variable averaged over all models; thus, the BFincl can be interpreted as the evidence found in the data supporting a certain variable (van den Bergh et al., 2020). The results showed extreme evidence (Schönbrodt and Wagenmakers, 2018) for an effect of the time on knowledge (BFincl = 592595.75). However, the results regarding the condition (BFincl = 0.22) and the interaction between time and condition (BFincl = 0.21) provided moderate evidence for the null hypothesis (Schönbrodt and Wagenmakers, 2018). Thus, they provided evidence for the conclusion that knowledge is not affected by the difference between the constructive or interactive instruction. The results of the Bayesian independent and paired sample t-test regarding the learning gain in knowledge (see Figures 2, 3) are similar to the findings of the Bayesian repeated measures analysis of variance. The trajectories of the Bayes factors in the sequential analyses showed that these results were already present after approximately 40 participants.
Table 1. Descriptive values of knowledge and skills before (pretest) and after (posttest) the intervention.
The difference between the mean knowledge score in pre- and posttest would reflect a large effect size for constructive learners (d = 0.76) and a large effect size for interactive learners (d = 0.91); the difference between constructive and interactive learners in the pretest (d = 0.15) and posttest (d = 0.06) not even a small effect (see Cohen, 1988).
Research question 2: To what extent does an intervention with interactive learning activities advance the skill in critical appraisal of scientific literature in comparison to an intervention with constructive learning activities?
The descriptive values of the skill are displayed in Table 1. The Bayesian repeated measures analysis (see Table 4 for the comparison of each model to the null model and Table 5 for the average across all models) of variance showed extreme evidence (Schönbrodt and Wagenmakers, 2018) for an effect of the time on skill (BFincl = 3.020 × 107). However, the results regarding the condition (BFincl = 0.42) and the interaction between time and condition (BFincl = 0.36) provided anecdotal evidence for the null hypothesis (Schönbrodt and Wagenmakers, 2018). Thus, the data is not sufficiently informative to provide information on how skill might be affected by the difference between the constructive or interactive instruction. The results of the Bayesian independent and paired sample t-test regarding the learning gain in skill (see Figures 4, 5) are similar to the findings of the Bayesian repeated measures analysis of variance. The trajectories of the Bayes factors in the sequential analyses showed that these results were already present after approximately 40 participants regarding the effect of time, but stayed variate regarding the effect of the condition.
The difference between the mean skill score in pre- and posttest would reflect a large effect size for constructive learners (d = 0.93) and a large effect size for interactive learners (d = 1.05); the difference between constructive and interactive learners in the pretest (d = 0.35) and posttest (d = 0.17) would reflect a small and not even a small effect size, respectively (see Cohen, 1988).
This study aimed at testing the effect of an interactive versus a constructive approach in an intervention fostering knowledge and skills in appraising scientific literature.
The interactive condition did not outperform the constructive condition, contrasting our hypothesis. This is not coherent with the research on learning activities comparing passive, active, constructive, and interactive learning activities in interventions (Chi, 2009; Menekse et al., 2013; Chi and Wylie, 2014; Chi et al., 2018; Menekse and Chi, 2019). However, the Bayesian analysis only provided anecdotal to moderate evidence for the similarity of the constructive and interactive conditions. Based on these results, we conclude that the difference in learning with constructive or interactive learning activity alone is not sufficient to explain knowledge and skill gain in this content area. We expected the learners in the interactive conditions to benefit from frequently updating their mental model because of the ongoing change in the information discussed (Chi and Wylie, 2014) and we would expect that this mechanism would also take place in the intervention of this study. However, our operationalization of the learning environment might have affected the mechanism that is hypothesized to be caused by the interactive learning activities: prompting participants to frequently update their mental model because of the ongoing change in the information. The learners had to explain a model solution of the task (in appraising evidence) in both conditions. And while there was no other person in the constructive condition, there were written statements of another person that the students were asked to explain in both conditions. This factor could have caused students in both conditions to update their mental model more than once. In the ICAP framework it is hypothesized that there is a systematic relationship between the learning activity exhibited by the students and their cognitive process (Chi, 2009; Chi and Wylie, 2014; Chi et al., 2018). However, based on our results we would add that there might be other factors that also strongly influence this cognitive process, such as aspects of the learning task beyond the constructive versus interactive distinction. The prompt to update one’s mental model more frequently might also be given by learning material that asks learners to explain new information.
Furthermore, we found students in both conditions to show a rise of, approximately, one standard deviation in knowledge and skills in appraising literature after an intervention that took less than an hour, far beyond the scope of a mere retest effect that could be expected in cognitive abilities (Scharfen et al., 2018). Participating in an intervention that asked learners to explain model solutions (thus, a short version of example-based learning, Renkl, 2014) to themselves or to learning partner (thus, self-explained the causal connection between the overview of how to appraise scientific evidences and the model solutions, cf. Bisra et al., 2018) seems to advance students’ knowledge and skills in appraising scientific evidence. The results are consistent with prior research showing that scientific reasoning in general can be facilitated by interventions (Engelmann et al., 2016), more specifically, higher education students’ scientific reasoning skills (e.g., Gruppen et al., 2005; Stark et al., 2009; Wenglein et al., 2015). Whether there is a causal relationship between this intervention and the learning gain in knowledge and skills needs to be tested in a future experiment.
One aspect for further research might be the domain specificity of the task. In this intervention, we only used educational research articles reporting experimental or quasi-experimental intervention studies, investigating an effect of an instruction or educational support. Also, we only included participants who were familiar with the general topics of this intervention: students in teacher education, educational sciences, and psychology. We did so, because some degree of domain-specific knowledge is necessary for the first-hand evaluation of the evidence (Bromme et al., 2010). The intervention and the measurement of knowledge and skills in appraising scientific literature was kept narrow in range. The material of intervention and tests were about learning with examples and the jigsaw technique, all studies employed a quantitative approach, and the structured briefs of scientific articles were structured similarly, focusing on one main research question (Hetmanek et al., 2015b). Thus, we did not examine to which extent the effect of the intervention could be transferable to appraising scientific literature in educational sciences that employ a different methodological approach or scientific literature that was presented in a different format. A wider range of scientific literature might benefit from a combined approach of first-hand and second-hand evaluation skills. Moreover, teachers are expected to read beyond educational sciences: this includes literature about the subjects that they are teaching, e.g., biology, mathematics, or history. The intervention presented in this paper might not be completely dependent on the content of the literature that is to be appraised, since we integrated two different educational topics in the intervention. However, a change in the methodological approach of the studies or in the format of the presentation might change the effect of this intervention. Further research in this type of intervention should systematically broaden the types of scientific research articles that are used as scientific evidence.
The study presented in this paper has several limitations. First, the posttest was conducted right after the intervention. Thus, we cannot make any generalization about long-term effects of facilitating the appraisal of scientific literature.
Second, the reliability of the skill measurement was relatively low. This can be explained by the conceptual breath of the scale. Each item in the skill measure targets a different aspect in which the scientific literature is evaluated. Each item covered one aspect that was also targeted in the intervention. The validity of the measure for the intervention would be decreased by removing any of the items to gain a higher reliability. Moreover, the measure was adapted from a validated scale (Trempler et al., 2015) by only changing the response options from a nine-point scale to four options. The wording of some items was slightly changed. Furthermore, the pattern of results is rather similar in knowledge and skills, showing a relevant difference between pre- and posttest and very little difference between the conditions. Thus, we do not expect the rather low reliability of the skill measure to have significantly impacted the results found in this study.
Third, while the intervention targeted a complex and large area of knowledge and skills, this intervention took less than 2 h. We designed the intervention to fit our scale in trying to focus on core knowledge and skills in appraising central aspects of scientific evidence. Still, the duration of the intervention might have been insufficient to teach a more comprehensive idea of appraising scientific literature. A longer intervention could give participants more time to practice appraising the evidence, which could have led to more knowledge gain (as has been discussed for decades by, e.g., Anderson, 1981; Berliner, 1990; van Gog, 2013). Specifically, students working interactively might need more time and instructional support in evidence-based argumentation (Csanadi et al., 2021). However, the intervention was kept short in order to compare the effect of this intervention to interventions of similar length: The study that compared constructive and interactive learning activities and found a higher learning gain for interactive learning in comparison to constructive learning with an effect size of d = 0.64 gave the students 25–30 min in the learning phase (Menekse et al., 2013).
This study showed the limitations of the hypothesis that interactive learning activities are accompanied with higher learning gains (Chi and Wylie, 2014) in teaching critical appraisal of scientific evidence. We suggest to expand the ICAP hypothesis to include more dimensions that influence the underlying cognitive processes, such as characteristics of a learning task in differentiating constructive and interactive learning activities. Based on the results of this study, we hypothesize that interactive learning might not require a person to discuss with, interaction might also be achieved with learning material that imitates an interaction or implements other ways for the learners to frequently update their mental model because of the ongoing change in the information discussed (cf. Chi and Wylie, 2014). Future studies are necessary to (a) investigate how the learning material must be designed and implemented to reach interactive learning activities that initiate learning processes similar to the learning process in interactive learning activities in cooperative settings and (b) investigate the interactive components in interventions, such as the constructive condition in this intervention to understand which aspects of the material is actually responsible for the beneficial learning process.
Evidence-based decisions are considered important for educational professionals. In this study, we implemented an intervention that facilitated future educational professionals to appraise scientific evidence in order to make evidence-based decisions in day-today practice. This study suggests that an intervention implementing constructive or interactive learning activities in studying sample solutions and self-explaining the examples facilitated higher education students’ critical appraisal of scientific evidence. So far, the effect of the intervention was only observed in a measurement of appraising scientific literature. It would be interesting for future research to investigate the effect of this intervention on a broader measurement of scientific reasoning.
The data supporting the conclusions of this article will be made available by the first author.
Ethical review and approval was not required for the study on human participants in accordance with the local legislation and institutional requirements. The patients/participants provided their written informed consent to participate in this study.
KE, AH, and FF developed the study concept and the study design. KE and AH developed and adapted the material and coded the data. KE planned the data collection, processed the data, performed the data analysis, interpreted the results, and took the lead in writing the manuscript. KE, AH, BN, and FF contributed to interpreting the results and writing the manuscript. All authors approved the final version of the manuscript for submission.
This work was supported by the Elite Network of Bavaria [K-GS-2012-209], the Center for Advanced Studies at Ludwig-Maximilians-Universität München, and Stiftung Universität Hildesheim.
The authors declare that the research was conducted in the absence of any commercial or financial relationships that could be construed as a potential conflict of interest.
All claims expressed in this article are solely those of the authors and do not necessarily represent those of their affiliated organizations, or those of the publisher, the editors and the reviewers. Any product that may be evaluated in this article, or claim that may be made by its manufacturer, is not guaranteed or endorsed by the publisher.
Anderson, L. W. (1981). Instruction and time-on-task: a review. J. Curriculum Stud. 13, 289–303. doi: 10.1080/0022027810130402
Argelagos, E., and Pifarré, M. (2012). Improving information problem solving skills in secondary education through embedded instruction. Comput. Hum. Behav. 28, 515–526. doi: 10.1016/j.chb.2011.10.024
Berliner, D. C. (1990). “What’s all the fuss about instructional time” in The nature of time in schools: Theoretical concepts, practitioner perceptions. eds. M. Ben-Peretz and R. Bromme (Teachers College Press). 3–35.
Bisra, K., Liu, Q., Nesbit, J. C., Salimi, F., and Winne, P. H. (2018). Inducing self-explanation: a meta-analysis. Educ. Psychol. Rev. 30, 703–725. doi: 10.1007/s10648-018-9434-x
Bradley, P., Oterholt, C., Herrin, J., Nordheim, L., and Bjørndal, A. (2005). Comparison of directed and self-directed learning in evidence-based medicine: a randomised controlled trial. Med. Educ. 39, 1027–1035. doi: 10.1111/j.1365-2929.2005.02268.x
Brand-Gruwel, S., Wopereis, I., and Vermetten, Y. (2005). Information problem solving by experts and novices: analysis of a complex cognitive skill. Comput. Hum. Behav. 21, 487–508. doi: 10.1016/j.chb.2004.10.005
Brand-Gruwel, S., Wopereis, I., and Walraven, A. (2009). A descriptive model of information problem solving while using internet. Comput. Educ. 53, 1207–1217. doi: 10.1016/j.compedu.2009.06.004
Bråten, I., Strømsø, H. I., and Salmerón, L. (2011). Trust and mistrust when students read multiple information sources about climate change. Learn. Instr. 21, 180–192. doi: 10.1016/j.learninstruc.2010.02.002
Bromme, R., Kienhues, D., and Porsch, T. (2010). “Who knows what and who can we believe? Epistemological beliefs are beliefs about knowledge (mostly) attained from others,” in Personal Epistemology in the Classroom: Theory, Research, and Implications for Practice. eds. L. D. Bendixen and F. C. Feucht (Cambridge: Cambridge University Press), 163–193.
Bromme, R., Prenzel, M., and Jäger, M. (2014). Empirische Bildungsforschung und evidenzbasierte Bildungspolitik [educational research and evidence based educational policy]. Z. Erziehungswiss. 17, 3–54. doi: 10.1007/s11618-014-0514-5
Brown, C., and Zhang, D. (2016). Is engaging in evidence-informed practice in education rational? What accounts for discrepancies in teachers' attitudes towards evidence use and actual instances of evidence use in schools? Br. Educ. Res. J. 42, 780–801. doi: 10.1002/berj.3239
Cain, T. (2016). Research utilisation and the struggle for the teacher's soul: a narrative review. Eur. J. Teach. Educ. 39, 616–629. doi: 10.1080/02619768.2016.1252912
Chi, M. T. (2009). Active-constructive-interactive: a conceptual framework for differentiating learning activities. Top. Cogn. Sci. 1, 73–105. doi: 10.1111/j.1756-8765.2008.01005.x
Chi, M. T., Adams, J., Bogusch, E. B., Bruchok, C., Kang, S., Lancaster, M., et al. (2018). Translating the ICAP theory of cognitive engagement into practice. Cogn. Sci. 42, 1777–1832. doi: 10.1111/cogs.12626
Chi, M. T., and Wylie, R. (2014). The ICAP framework: linking cognitive engagement to active learning outcomes. Educ. Psychol. 49, 219–243. doi: 10.1080/00461520.2014.965823
Cohen, J. (1988). Statistical Power Analysis for the Behavioral Sciences (2. Auflage). Hillsdale, NJ: Erlbaum.
Cook, B. G., Smith, G. J., and Tankersley, M. (2012). “Evidence-based practices in education,” in APA Educational Psychology Handbook:Theories, Constructs, and Critical Issues Vol. 1. eds. K. R. Harris, S. Graham, T. Urdan, C. B. McCormick, G. M. Sinatra, and J. Sweller (American Psychological Association), 495–527.
Csanadi, A., Kollar, I., and Fischer, F. (2021). Pre-service teachers’ evidence-based reasoning during pedagogical problem-solving: better together? Eur. J. Psychol. Educ. 36, 147–168. doi: 10.1007/s10212-020-00467-4
Detrich, R., and Lewis, T. (2013). A decade of evidence-based education: where are we and where do we need to go? J. Posit. Behav. Interv. 15, 214–220. doi: 10.1177/1098300712460278
Dickersin, K., Straus, S. E., and Bero, L. A. (2007). Evidence based medicine: increasing, not dictating, choice. BMJ 334:s10. doi: 10.1136/bmj.39062.639444.94
Engelmann, K., Hetmanek, A., Neuhaus, B. J., and Fischer, F. (2018). Reading scientific articles: facilitating the evaluation of structured briefs of scientific literature. doi: 10.17605/OSF.IO/54F7J
Engelmann, K., Neuhaus, B. J., and Fischer, F. (2016). Fostering scientific reasoning in education–meta-analytic evidence from intervention studies. Educ. Res. Eval. 22, 333–349. doi: 10.1080/13803611.2016.1240089
Faul, F., Erdfelder, E., Lang, A. G., and Buchner, A. (2007). G* power 3: a flexible statistical power analysis program for the social, behavioral, and biomedical sciences. Behav. Res. Methods 39, 175–191. doi: 10.3758/BF03193146
Fischer, F., Chinn, C. A., Engelmann, K., and Osborne, J. (Eds.). (2018). Scientific Reasoning and Argumentation: The Roles of Domain-Specific and Domain-General Knowledge. New York, NY: Routledge.
Fischer, F., Kollar, I., Ufer, S., Sodian, B., Hussmann, H., Pekrun, R., et al. (2014). Scientific reasoning and argumentation: advancing an interdisciplinary research agenda in education. Frontline Learn. Res. 2, 28–45.
Frerejean, J., Velthorst, G. J., van Strien, J. L., Kirschner, P. A., and Brand-Gruwel, S. (2019). Embedded instruction to learn information problem solving: effects of a whole task approach. Comput. Hum. Behav. 90, 117–130. doi: 10.1016/j.chb.2018.08.043
Gruppen, L. D., Rana, G. K., and Arndt, T. S. (2005). A controlled comparison study of the efficacy of training medical students in evidence-based medicine literature searching skills. Acad. Med. 80, 940–944. doi: 10.1097/00001888-200510000-00014
Harden, R. M., Grant, J., Buckley, G., and Hart, I. R. (1999). BEME guide no. 1: best evidence medical education. Med. Teach. 21, 553–562. doi: 10.1080/01421599978960
Henderson, J. B. (2019). Beyond “active learning”: how the ICAP framework permits more acute examination of the popular peer instruction pedagogy. Harv. Educ. Rev. 89, 611–634. doi: 10.17763/1943-5045-89.4.611
Hetmanek, A. (2014). Evidenzbasierte Praxis im Bildungsbereich: Standortbestimmung und Vorarbeiten zur Förderung in drei empirischen Studien (Doctoral dissertation). München.
Hetmanek, A., Wecker, C., Kiesewetter, J., Trempler, K., Fischer, M. R., Gräsel, C., et al. (2015a). Wozu nutzen Lehrkräfte welche Ressourcen? [For what do teachers use which kind of resource?]. Unterrichtswissenschaft 43, 193–208.
Hetmanek, A., Wecker, C., Trempler, K., Kiesewetter, J., Gräsel, C., Fischer, M. R., et al. (2015b). Structured briefs provide a means to communicate research more efficiently to practitioners. Paper presented at 16th Biennial EARLI Conference “Towards a Reflective Society”, Limassol, Cyprus, 25–29 August 2015.
Klieme, E., and Rakoczy, K. (2008). Empirische Unterrichtsforschung und Fachdidaktik. Outcome-orientierte Messung und Prozessqualität des Unterrichts. Zeitschrift für Pädagogik 54, 222–237.
Kiemer, K., and Kollar, I. (2021). Source selection and source use as a basis for evidence-informed teaching. Z. Pädagog. Psychol. 35, 127–141. doi: 10.1024/1010-0652/a000302
KMK (2019). Standards für die Lehrerbildung: Bildungswissenschaften. Available at: https://www.kmk.org/fileadmin/Dateien/veroeffentlichungen_beschluesse/2004/2004_12_16-Standards-Lehrerbildung-Bildungswissenschaften.pdf
Kobayashi, K. (2019). Learning by preparing-to-teach and teaching: a meta-analysis. Jpn. Psychol. Res. 61, 192–203. doi: 10.1111/jpr.12221
Kulier, R., Gülmezoglu, A. M., Zamora, J., Plana, M. N., Carroli, G., Cecatti, J. G., et al. (2012). Effectiveness of a clinically integrated e-learning course in evidence-based medicine for reproductive health training: a randomized trial. JAMA 308, 2218–2225. doi: 10.1001/jama.2012.33640
Lachner, A., Jacob, L., and Hoogerheide, V. (2021). Learning by writing explanations: is explaining to a fictitious student more effective than self-explaining? Learn. Instr. 74:101438. doi: 10.1016/j.learninstruc.2020.101438
Marsman, M., and Wagenmakers, E. J. (2017). Bayesian benefits with JASP. Eur. J. Dev. Psychol. 14, 545–555. doi: 10.1080/17405629.2016.1259614
Menekse, M., and Chi, M. T. (2019). The role of collaborative interactions versus individual construction on students’ learning of engineering concepts. Eur. J. Eng. Educ. 44, 702–725. doi: 10.1080/03043797.2018.1538324
Menekse, M., Stump, G. S., Krause, S., and Chi, M. T. (2013). Differentiated overt learning activities for effective instruction in engineering classrooms. J. Eng. Educ. 102, 346–374. doi: 10.1002/jee.20021
Menz, C., Spinath, B., and Seifried, E. (2021). Where do pre-service teachers’ educational psychological misconceptions come from? Z. Pädagog. Psychol. 1:14.
Molléri, J. S., Bin Ali, N., Petersen, K., Minhas, N. M., and Chatzipetrou, P. (2018). Teaching students critical appraisal of scientific literature using checklists. In Proceedings of the 3rd European Conference of Software Engineering Education New York, NY: Association for Computing Machinery (pp. 8–17).
Noetel, M., Griffith, S., Delaney, O., Sanders, T., Parker, P., del Pozo Cruz, B., et al. (2021). Video improves learning in higher education: a systematic review. Rev. Educ. Res. 91, 204–236. doi: 10.3102/0034654321990713
Raes, A., Schellens, T., De Wever, B., and Vanderhoven, E. (2012). Scaffolding information problem solving in web-based collaborative inquiry learning. Comput. Educ. 59, 82–94. doi: 10.1016/j.compedu.2011.11.010
Renkl, A. (2014). Toward an instructionally oriented theory of example-based learning. Cogn. Sci. 38, 1–37. doi: 10.1111/cogs.12086
Révai, N. (2018). What difference do standards make to educating teachers? A review with case studies on Australia, Estonia and Singapore. OECD Educat. Work. Pap. 174:0_1-70.
Reviriego, E., Cidoncha, M. Á., Asua, J., Gagnon, M. P., Mateos, M., Gárate, L., et al. (2014). Online training course on critical appraisal for nurses: adaptation and assessment. BMC Med. Educ. 14, 1–10. doi: 10.1186/1472-6920-14-136
Sackett, D. L. (1997). “Evidence-based medicine,” in Seminars in Perinatology, vol. 21 (Philadelphia, PA: WB Saunders), 3–5.
Sackett, D. L., Rosenberg, W. M. C., Gray, J. A. M., Haynes, R. B., and Richardson, W. S. (1996). Evidence-based medicine: what it is and what it is not. BMJ 312, 71–72. doi: 10.1136/bmj.312.7023.71
Scharfen, J., Peters, J. M., and Holling, H. (2018). Retest effects in cognitive ability tests: a meta-analysis. Intelligence 67, 44–66. doi: 10.1016/j.intell.2018.01.003
Schönbrodt, F. D., and Wagenmakers, E. J. (2018). Bayes factor design analysis: Planning for compelling evidence. Psychonomic Bulletin and Review 25, 128–142.
Schworm, S., and Renkl, A. (2007). Learning argumentation skills through the use of prompts for self-explaining examples. J. Educ. Psychol. 99, 285–296. doi: 10.1037/0022-0663.99.2.285
Slavin, R. E. (2008). Perspectives on evidence-based research in education—what works? Issues in synthesizing educational program evaluations. Educ. Res. 37, 5–14. doi: 10.3102/0013189X08314117
Stark, R. (2017). Probleme evidenzbasierter bzw.-orientierter pädagogischer Praxis. [Problems of evidence-based or rather evidence-oriented educational practice]. Z. Pädagog. Psychol. 31, 99–110. doi: 10.1024/1010-0652/a000201
Stark, R., Puhl, T., and Krause, U. M. (2009). Improving scientific argumentation skills by a problem-based learning environment: effects of an elaboration tool and relevance of student characteristics. Evaluat. Res. Educat. 22, 51–68. doi: 10.1080/09500790903082362
Thomm, E., and Bromme, R. (2016). How source information shapes lay interpretations of science conflicts: interplay between sourcing, conflict explanation, source evaluation, and claim evaluation. Read. Writ. 29, 1629–1652. doi: 10.1007/s11145-016-9638-8
Thomm, E., Sälzer, C., Prenzel, M., and Bauer, J. (2021). Predictors of teachers’ appreciation of evidence-based practice and educational research findings. Z. Pädagog. Psychol. 35, 173–184. doi: 10.1024/1010-0652/a000301
Trempler, K., Hetmanek, A., Wecker, C., Kiesewetter, J., Fischer, F., Fischer, M. R., et al. (2015). Nutzung von Evidenz im Bildungsbereich – Validierung eines Instruments zur Erfassung von Kompetenzen der Informationsauswahl und Bewertung von Studien [Use of evidence in education - validation of a tool for measuring competences in information selection and evaluation of studies]. Z. Pädagogik 61, 144–166.
van den Bergh, D., Van Doorn, J., Marsman, M., Draws, T., Van Kesteren, E. J., Derks, K., et al. (2020). A tutorial on conducting and interpreting a Bayesian ANOVA in JASP. LAnnee Psychol. 120, 73–96. doi: 10.3917/anpsy1.201.0073
van Gog, T. (2013). “Time on task,” in International Guide to Student Achievement. eds. J. Hattie and E. M. Anderman (New York: Routledge)
Van Peppen, L. M., Verkoeijen, P. P. J. L., Heijltjes, A. E. G., Janssen, E. M., Koopmans, D., and van Gog, T. (2018). Effects of self-explaining on learning and transfer of critical thinking skills. Front. Educat. 3:100. doi: 10.3389/feduc.2018.00100
Vogel, F., Wecker, C., Kollar, I., and Fischer, F. (2017). Socio-cognitive scaffolding with computer-supported collaboration scripts: a meta-analysis. Educ. Psychol. Rev. 29, 477–511. doi: 10.1007/s10648-016-9361-7
Wagenmakers, E. J., Love, J., Marsman, M., Jamil, T., Ly, A., Verhagen, J., et al. (2018a). Bayesian inference for psychology. Part II: example applications with JASP. Psychon. Bull. Rev. 25, 58–76. doi: 10.3758/s13423-017-1323-7
Wagenmakers, E. J., Marsman, M., Jamil, T., Ly, A., Verhagen, J., Love, J., et al. (2018b). Bayesian inference for psychology. Part I: theoretical advantages and practical ramifications. Psychon. Bull. Rev. 25, 35–57. doi: 10.3758/s13423-017-1343-3
Walraven, A., Brand-Gruwel, S., and Boshuizen, H. P. (2009). How students evaluate information and sources when searching the World Wide Web for information. Comput. Educ. 52, 234–246. doi: 10.1016/j.compedu.2008.08.003
Keywords: evidence-based education, ICAP, appraisal of scientific literature, evidence evaluation, higher education
Citation: Engelmann K, Hetmanek A, Neuhaus BJ and Fischer F (2022) Testing an intervention of different learning activities to support students’ critical appraisal of scientific literature. Front. Educ. 7:977788. doi: 10.3389/feduc.2022.977788
Received: 24 June 2022; Accepted: 04 November 2022;
Published: 07 December 2022.
Edited by:
Martin Greisel, University of Augsburg, GermanyReviewed by:
Mario Mäeots, University of Tartu, EstoniaCopyright © 2022 Engelmann, Hetmanek, Neuhaus and Fischer. This is an open-access article distributed under the terms of the Creative Commons Attribution License (CC BY). The use, distribution or reproduction in other forums is permitted, provided the original author(s) and the copyright owner(s) are credited and that the original publication in this journal is cited, in accordance with accepted academic practice. No use, distribution or reproduction is permitted which does not comply with these terms.
*Correspondence: Katharina Engelmann, a2F0aGFyaW5hLmVuZ2VsbWFubkB1bmktaGlsZGVzaGVpbS5kZQ==
†ORCID: Katharina Engelmann, https://orcid.org/0000-0003-1697-9754
Andreas Hetmanek, https://orcid.org/0000-0002-9649-6711
Birgit J. Neuhaus, https://orcid.org/0000-0001-6031-9660
Frank Fischer, https://orcid.org/0000-0003-0253-659X
Disclaimer: All claims expressed in this article are solely those of the authors and do not necessarily represent those of their affiliated organizations, or those of the publisher, the editors and the reviewers. Any product that may be evaluated in this article or claim that may be made by its manufacturer is not guaranteed or endorsed by the publisher.
Research integrity at Frontiers
Learn more about the work of our research integrity team to safeguard the quality of each article we publish.