- Faculty of Hospitality and Tourism, Prince of Songkla University, Phuket, Thailand
The modern classroom takes on numerous forms and expands beyond the traditional brick-and-mortar walls. Educators are increasingly expected to integrate information and communications technology (ICT) and e-learning into the modern classroom. Several variables may influence instructors’ decision-making processes about ICT integration in the classroom. Smartphone technology provides students with accessibility, the ability to communicate with others, as well as to engage with classroom material. The study aims to examine how a variety of factors (perceived usefulness, perceived ease of use, subjective norm, and attitude) influence behavioral intention toward the use of smartphone technology. Two hundred and ninety survey responses were analyzed to identify the relationship between these factors based on an extended technology acceptance model. The empirical results of the study revealed that subjective norms and attitudes are significant predictors of behavioral intention toward the use of smartphone technology. The article concludes by presenting implications for educators, policymakers, and education researchers derived from the academic and practical discussions based on the findings.
Introduction
Educators are frequently challenged to incorporate ICT and e-learning into the modern classroom (Sandybayev, 2020). The demand for the application of ICT appears to be impending from all sides—commercial, political, and societal—and is motivated by the generally held belief that educational institutions can better meet the needs of all students than they do now (Arrosagaray et al., 2019). Gaining knowledge of what leads a teacher to actively utilize ICT in the classroom would be an excellent basis for new advancement and modernization of education (Ifinedo et al., 2020). Although, several variables may influence instructors’ decision-making processes about ICT integration in the classroom (Andyani et al., 2020). To start, many instructors consider that they must first acquire the necessary ICT skills (Falloon, 2020). Educators agree that they must not only be proficient in using technology, but also confident in their use in front of their digital native learners. Techataweewan and Prasertsin (2018) thus, digital literacy competence is expected to have a beneficial link with ICT implementation in the classroom, for both their growth and that of their students (Sánchez-Cruzado et al., 2021).
Smartphone technology provides students with accessibility, the ability to communicate with others, as well as to engage with classroom material (Techataweewan and Prasertsin, 2018; Andyani et al., 2020; Sánchez-Cruzado et al., 2021). Teachers’ attitudes toward the value of smartphone technology in the classroom are similarly expected to impact the implementation and application in their classrooms (Lawrence and Tar, 2018). This might present itself in two ways. First, if the teacher feels that smartphone technology enhances teaching and learning in general (Tondeur et al., 2018), and second, whether the instructor believes that using smartphone technology in the classroom would aid students, especially in the development of relevant current skills (Stal and Paliwoda-Pêkosz, 2019). The aforementioned factors may be predictive of smartphone technology deployment in the classroom, but they do not speak to any deliberate implementation (Mlambo et al., 2020).
In its place, teacher perceptions about teaching and learning may also predict deliberate implementation (Li et al., 2019). While the present literature observed a steadily increasing number of studies on the use of smartphone technology in an educational context, analysis of the literature revealed distinct research gap on the behavioral intentions by university students. Therefore, the purpose of this study is to explore what factors influence the behavioral intentions of students toward the use of smartphone technology in the classroom. Therefore, the study aims to explore how students assess how perceived ease of use, perceived usefulness, subjective norms, and attitudes influence behavioral intentions toward the use of smartphone technologies in the classroom.
Literature review
Positive impressions of ICT in the classroom and their influence on student success would, of course, be associated with the deployment of smartphone technology in the classroom (Fuchs, 2021a; Wilson et al., 2022). Educators that favor student-centered learning, which places the emphasis on the student rather than the teacher, may be more likely to promote smartphone technology in the classroom since it allows for greater levels of self-directed learning (Choy and Cheung, 2022). Furthermore, teachers that favor inquiry-based learning, in which students must probe deeper into more open-ended issues, may be more amenable to introducing smartphone technology into the classroom (Deák et al., 2021). This is due to the fact that technology allows students to delve deeper into concepts and ideas in ways that non-digital teaching instruments may be incapable of or ineffective at Fuchs (2021a). Educators that advocate for traditional methods of teaching and learning, such as content-learning (or rote-learning), in which students must memorize facts and details, may be less inclined to accept the introduction of smartphone technology into their classrooms (Lewis et al., 2018; Willis et al., 2019).
Many of the issues outlined can be resolved by employing active learning strategies that encourage students to actively participate in online course content (Lewis et al., 2018). Active learning is rarely connected with a particular type of learning environment (O’Connor, 2021). Nevertheless, there are numerous effective approaches for incorporating and practicing active learning in non-face-to-face settings, such as well-planned discussions, group work, and creating a collaborative environment that encourages and fosters a community of learning, such as the technology-enhanced flipped classroom method (Åhman et al., 2021). According to Molinillo et al. (2018), “it is vital to integrate active learning throughout the primary components of an online or hybrid course, such as discussions, assignments, and evaluations, to generate a high degree of student involvement” (p. 48). Active learning is a way of engaging students in higher-order thinking tasks (such as analysis, synthesis, evaluation, and reflection) through a variety of activities so that students acquire more than just passive learning (Sugeng and Suryani, 2020). Instead of listening to a lecture on a topic, students would discuss it among themselves, imagine how it may be implemented in practice, produce actual examples, and then present these examples (Fuchs, 2021b).
Smartphone technology is a tool that is frequently mentioned in the literature to enhance active learning (Aflalo et al., 2018). According to Baig et al. (2015), “smartphones are nowadays used as learning facilities in almost all fields, such as health, languages, engineering, and education” (p. 27). Smartphone technology is evolving at a fast pace and a smartphone can be used to carry out and access almost all learning activities (Fansury et al., 2020). The emergence of these instruments in higher education has followed a similar pattern of increasing usage (Fuchs, 2022). Although there has been little research on higher education students’ preferences for engaging in learning through this media (Gregorcic et al., 2018). The history of using smartphone technology in the classroom demonstrates that their use has steadily increased in as a result of the global coronavirus pandemic (Bautista-Vallejo et al., 2020). The usage of smartphone technology can encourage active, critically engaged, and reflective experiences, according to Fuchs (2021a). In the context of teaching and learning, practically all students own cell phones, which they may utilize as an aid in the learning process (Thornton and Houser, 2005). Several studies show the value of using smartphone technology in the classroom (Gan and Balakrishnan, 2018). It is noted that smartphone technology can help students locate learning resources and use learning applications at any time and from any location (Huang and Chiu, 2015).
The Technology Acceptance Model (TAM) has been widely employed as a strong and efficient methodological approach for assessing technological adoption among consumers (Buabeng-Andoh, 2018). Al-Emran and Graniæ (2021) further note that TAM is often viewed as a more superior choice to evaluate users’ perceptions. In particular, it is seen as more robust model of the Theory of Planned Behaviour (TPB) and Theory of Reasoned Action (TRA), and therefore, an advancement for measuring the acceptance of several technologies. TAM is utilized in this study because it can predict and explain users’ intentions to adopt technology (Al-Emran et al., 2018). The perceived usefulness and perceived ease of use are important factors in technology adoption belief (Elkaseh et al., 2016; Al-Emran et al., 2020). The degree to which a person feels that employing a technology will improve his or her performance is defined as perceived usefulness (Liaw and Huang, 2013; Al-Emran et al., 2021). Moreover, Yuan et al. (2016) define perceived ease of use as the extent to which the intended user feels the system or technology will require no effort. Prospective users may feel that a system or technology is advantageous while also believing that the system or technology is difficult to use. Thus, perceived simplicity of use is expected to impact perceived usefulness (Cho and Sagynov, 2015; Al-Emran, 2021).
Furthermore, Abramson et al. (2015) did a study to investigate the usage of e-learning throughout an extended TAM and discovered that perceived ease of use affected attitudes toward usage substantially. Extending the existing TAM has the possibility to create value by gaining further insights into variables that affect behavioral intentions of its users. Al-Qaysi et al. (2021) used an extended TAM to include perceived enjoyment as a variable to identify that it positively impacted the perceived usefulness of social media for learning purposes. Subjective norm is defined as an individual’s belief that the majority of significant people in his or her life feel he or she should or should not perform the behavior in question (Reynolds et al., 2015).
A person realizes that the more essential others feel it is for him or her to achieve something, the more he or she will attempt to do it (Huang et al., 2019). According to the literature, subjective norms have a considerable effect on perceived usefulness (Mou et al., 2017; AL-Nuaimi et al., 2022). Therefore, the study adopts a conceptual framework by extending the TAM adapted from Lee et al. (2003) as well as Maranguniæ and Graniæ (2015) with the factor subjective norm from the Theory of Reasoned Action (TRA) adapted from Madden et al. (1992) as well as LaCaille (2020) with as seen in Figure 1. Consequently, the following hypotheses were formulated:
H1. Perceived usefulness significantly impacts the attitude toward use.
H2. Perceived ease of use significantly impacts the attitude toward use.
H3. Perceived ease of use significantly impacts perceived usefulness.
H4. Perceived usefulness significantly impacts behavioral intentions.
H5. Subjective norm significantly impacts behavioral intentions.
H6. Attitude significantly impacts behavioral intentions.
Methodology
Procedure
The data collection took place in May 2022 at Haaga-Helia University of Applied Sciences in Finland. This study collected empirical data using a multilingual online survey that was distributed to undergraduate students via email to their personal email account of the university. Simple random sampling was used as a technique to recruit participants (Etikan et al., 2016), and responses were collected via a self-administered Google Forms survey (Mondal et al., 2018).
Sample
The sample consisted of full-time students enrolled in a 3-year Bachelor of Business Administration degree program. Following the removal of 17 responses due to missing or incomplete information, the total sample size was 290 students comprising of 69% female students (n = 200) and 31% male students (n = 90). Moreover, the majority of students were in their second year (n = 127) with the remainder in their first (n = 85) or third year (n = 78). The students were between 18 and 27 years old with an average age of 20.4 years.
Research instrument
The survey included seven socio-demographic profile questions as well as 17 items on a 5-point Likert-type scale (Joshi et al., 2015). The exact survey statements can be accessed in the Appendix 1. Eight statements (PU1-4; PEU1-4) were adopted from Fuchs (2021a) and modified to the context of this study and nine statements (ATT1-3; SN1-3; BI1-3) were adopted from Buabeng-Andoh (2018) and modified to the context of this study, respectively.
Data analysis
The open-source application JASP was used to do statistical analysis on the dataset based on good practice described by Love et al. (2019). Mean scores, lowest and maximum values, and standard deviation were used to examine the data descriptively. In addition, confirmatory factor analysis (CFA) was used to validate the factor structure of a collection of observed variables (Schmitt, 2011). The results of the CFA are reported in Figure 2. The individual factor loadings range between 0.54 and 0.88, which is a good measure (Schmitt, 2011). It means that between 29% (0.54∧2 = 0.29) and (0.88∧2 = 0.77) 77% of the observed variances (per individual factor) can be explained. Overall, 100% of the observed factor variances can be explained, wherein the variances between factors range from 0.60 to 0.98.
Results
To test the previously stated hypotheses, the Pearson correlation coefficient was calculated and the results are reported in Table 1 and Figure 3. The Pearson correlation coefficient requirements were all consistent and based on best practices by Benesty et al. (2009). Altogether, all six pair-wise combinations have a statistically significant relationship (0.001). According to Benesty et al. (2009), “an r-value between 0.1 and 0.4 indicates a minor correlation, whereas an r-value between 0.4 and 0.7 indicates a moderate connection. Finally, a strong correlation has an r-value of 0.7 or above” (p. 12). Based on the six pair-wise combinations, a moderate correlation exists between the factors perceived usefulness and behavioral intentions (0.561, p < 0.001), perceived ease of use and behavioral intentions (0.560, p < 0.001), and perceived usefulness (0.575, p < 0.001). A strong correlation was dedicated to the relationships between factors of perceived ease of use and perceived usefulness (0.822, p < 0.001), subjective norm and behavioral intentions (0.811, p < 0.001), as well as attitude and behavioral intentions (0.783, p < 0.001). Table 1 also includes the results of the Shapiro–Wilk test for bivariate normality and indicates that the result does not significantly deviate from a normal distribution.
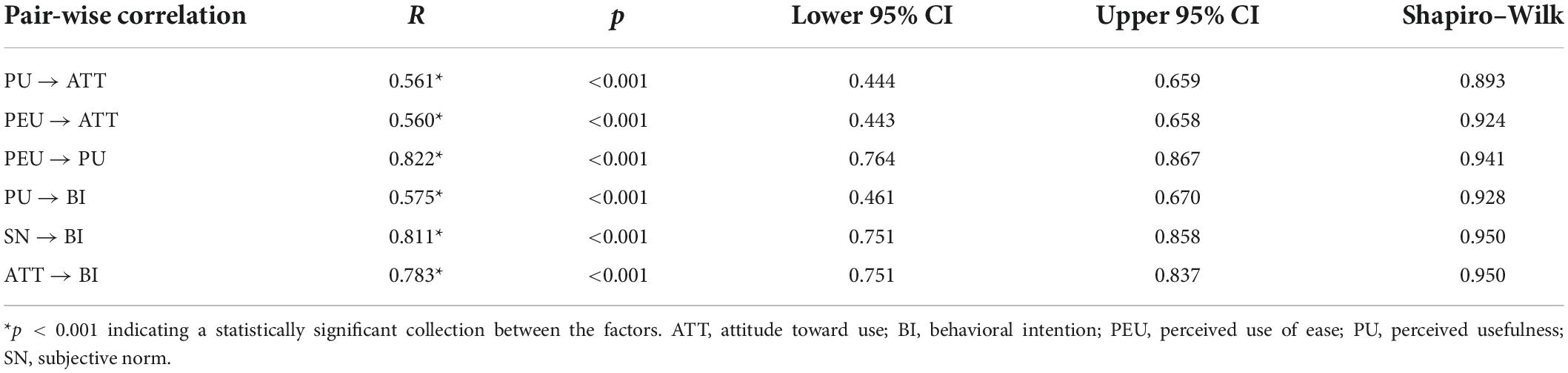
Table 1. Pearson’s correlation (r) based on the conceptual model influencing students’ behavioral intentions of using smartphone technology in the classroom.
The conceptual framework of the study uses an extended TAM with the addition of the factor subjective norm borrowed from the TRA. On that basis, the results of the statistical analysis were plotted into the new conceptual model as reported in Figure 2. The model highlights the statistically significant relationship between the variables. To sum up, there is a statistically significant correlation (p < 0.001) for all relationships within the model, and therefore, all six hypotheses are confirmed. Furthermore, the observed correlation between perceived ease of use and perceived usefulness, subjective norm, and behavioral intentions, as well as attitude and behavioral intentions is strong (larger than 0.80) based on the model. The statistical tests are summarized in Table 2.
Discussion and implications
The focus of this research was to explore what factors impact students’ behavioral intentions toward using smartphone technology in the classroom. Therefore, the study explored how students assess how perceived ease of use, perceived usefulness, subjective norms and attitudes influence behavioral intentions toward the use of smartphone technologies in the classroom. The previously stated results of the hypothesis tests revealed a statistically significant correlation between all six relationships. Moreover, three of these relationships exhibited a strong correlation. There are a variety of noteworthy findings that need to be discussed. First, the research confirmed the importance of subjective norms and attitudes toward behavioral intentions of using a specific technology as observed in similar contexts with different research settings (Schepers and Wetzels, 2007; Huang et al., 2020; AL-Nuaimi et al., 2022). As hybrid learning and virtual teaching become a norm (Fuchs, 2022), it is advised that faculty management give an introduction course for students who lack the necessary skills and knowledge to utilize smartphone technology, as well as a seminar on how to integrate the usage of a smartphone for educational reasons.
Second, the results of this study similarly affirm the findings of Abramson et al. (2015) who discovered that the perceived ease of use of e-learning affected attitudes toward usage substantially. In this study, there was a statistically significant relationship between the perceived ease of use of (smartphone) technology and the attitude toward that (smartphone) technology. Ardies et al. (2015) note that technology is more present than ever. Young consumers are curious in technical products, but their views on education and employment in technology are varied (Ardies et al., 2015). To boost students’ attitudes toward technology, we must first have a deeper grasp of the elements that influence attitudes. Improving students’ attitudes toward smartphone technology in the classroom requires a well-planned course design (Keržiè et al., 2019) as well as educating them about the intended use. Improving their understanding of how to integrate their smartphones with the coursework will improve their overall perception and hence positively impact their attitudes (Hansen et al., 2018). It is essential to encourage students to gain and build skills and competences that will allow them to be more confident in utilizing smartphone technology for their studies, as this will lead to more effective learning (Lawrence and Tar, 2018; Stal and Paliwoda-Pêkosz, 2019; Andyani et al., 2020).
Third, the outcome of this study supported other studies that demonstrated a positive relationship between perceived ease of use, perceived usefulness, subjective norms, and attitudes that influence behavioral intentions toward the use of smartphone technologies in the classroom. Educators need to ensure that particular activities involving smartphone technology are well-prepared and thoroughly explained to the students to increase understanding and usefulness. According to Torres Martín et al. (2021), many institutions are establishing specialized digital strategies in response to the tremendous shift toward the use of new technologies, but lack the vision, capacity, or commitment to properly apply them. It is critical to offer a good view of the technology’s usefulness to encourage students and boost their intentions to employ smartphone technology in their learning environment (Choi and Ji, 2015). Furthermore, students’ attitudes may not link this factor with being of equal value; hence, enhancing their comprehension would improve their behavioral intention.
Conclusion
The present study acknowledged a number of relationships that are expected to drive the integration of smartphone technology in educational settings. The results of the study revealed that subjective norms and attitudes are significant predictors of behavioral intention toward the use of smartphone technology. Although the research aim of the study was met and attests nurturing the use of smartphone technology in the classroom, there are a few shortcomings that provide potential for further research. There are certain limitations to this study that the reader should consider when assessing the conclusions and suggestions. Foremost, the empirical findings relate to a university in Finland and are not directly transferable to a different geographical context. However, the derived implications and recommendations provide value for other higher education institutions (HEI) as well as provide another baseline for education researchers.
Danish and Hmelo-Silver (2020) noted that “future research should increase the understanding of how smartphone technology can improve teaching and learning activities” (p. 12). In particular, more empirical evidence is needed to establish good practices that are transferable to a wider context or geographical coverage by further investigating affordances that positively and negatively affect the perceived usefulness and perceived ease of use. Another limitation is the lack of a specific context in which the students used smartphone technology. An exploratory interview study has the potential to discover a more meaningful and nuanced view of the “how” and “why” regarding behavioral intentions of smartphone technologies in the classroom. Lastly, this research concentrates on studying the students’ acceptance of smartphone technology only and disregards examining the educators’ acceptance of such technology.
Data availability statement
The raw data supporting the conclusions of this article will be made available by the authors, without undue reservation.
Ethics statement
The studies involving human participants were reviewed and approved by the Research Committee of the Faculty of Hospitality and Tourism, Prince of Songkla University. Written informed consent for participation was not required for this study in accordance with the national legislation and the institutional requirements.
Author contributions
KF: study conception and design, data collection, analysis and interpretation of results, and manuscript preparation.
Funding
The Faculty of Hospitality and Tourism, Prince of Songkla University supported this research project under the Fast Track Data Collection Grant.
Conflict of interest
The author declares that the research was conducted in the absence of any commercial or financial relationships that could be construed as a potential conflict of interest.
Publisher’s note
All claims expressed in this article are solely those of the authors and do not necessarily represent those of their affiliated organizations, or those of the publisher, the editors and the reviewers. Any product that may be evaluated in this article, or claim that may be made by its manufacturer, is not guaranteed or endorsed by the publisher.
Author disclaimer
Any opinions and conclusions expressed in this manuscript are solely the intellectual result of the author and do not reflect the viewpoint of the Faculty or University.
References
Abramson, J., Dawson, M., and Stevens, J. (2015). An examination of the prior use of e-learning within an extended technology acceptance model and the factors that influence the behavioral intention of users to use m-learning. Sage Open 5:2158244015621114. doi: 10.1177/2158244015621114
Aflalo, E., Zana, L., and Huri, T. (2018). The interactive whiteboard in primary school science and interaction. Interact. Learn. Environ. 26, 525–538. doi: 10.1080/10494820.2017.1367695
Åhman, S., Nguyen, J., Fuchs, K., and Aghaee, N. (2021). Student Response Systems in a Technology Enhanced Flipped Classroom: A Qualitative Investigation in Higher Education. Int. J. Learn. Teach. Educ. Res. 20, 86–101. doi: 10.26803/ijlter.20.9.6
Al-Emran, M. (2021). Evaluating the use of smartwatches for learning purposes through the integration of the technology acceptance model and task-technology fit. Int. J. Hum. Comput. Interact. 37, 1874–1882. doi: 10.1080/10447318.2021.1921481
Al-Emran, M., Al-Maroof, R., Al-Sharafi, M. A., and Arpaci, I. (2020). What impacts learning with wearables? An integrated theoretical model. Interact. Learn. Environ. 4, 1–21. doi: 10.1080/10494820.2020.1753216
Al-Emran, M., and Graniæ, A. (2021). “Is it still valid or outdated? A bibliometric analysis of the technology acceptance model and its applications from 2010 to 2020,” in Recent advances in technology acceptance models and theories, eds M. Al-Emran and K. Shaalan (Cham: Springer), 1–12. doi: 10.1007/978-3-030-64987-6_1
Al-Emran, M., Mezhuyev, V., and Kamaludin, A. (2018). Technology Acceptance Model in M-learning context: A systematic review. Comput. Educ. 125, 389–412. doi: 10.1016/j.compedu.2018.06.008
Al-Emran, M., Mezhuyev, V., and Kamaludin, A. (2021). Is M-learning acceptance influenced by knowledge acquisition and knowledge sharing in developing countries? Educ. Inform. Technol. 26, 2585–2606. doi: 10.1007/s10639-020-10378-y
AL-Nuaimi, M. N., Al Sawafi, O. S., Malik, S. I., Al-Emran, M., and Selim, Y. F. (2022). Evaluating the actual use of learning management systems during the covid-19 pandemic: an integrated theoretical model. Interact. Learn. Environ. 3, 1–26. doi: 10.1080/10494820.2022.2055577
Al-Qaysi, N., Mohamad-Nordin, N., and Al-Emran, M. (2021). Developing a comprehensive theoretical model for adopting social media in higher education. Interact. Learn. Environ. 12, 1–22. doi: 10.1080/10494820.2021.1961809
Andyani, H., Setyosari, P., Wiyono, B., and Djatmika, E. (2020). Does technological pedagogical content knowledge impact on the use of ICT in pedagogy? Int. J. Emerg. Technol. Learn. 15, 126–139. doi: 10.3991/ijet.v15i03.11690
Ardies, J., De Maeyer, S., Gijbels, D., and van Keulen, H. (2015). Students attitudes towards technology. Int. J. Technol. Design Educ. 25, 43–65. doi: 10.1007/s10798-014-9268-x
Arrosagaray, M., González-Peiteado, M., Pino-Juste, M., and Rodríguez-López, B. (2019). A comparative study of Spanish adult students’ attitudes to ICT in classroom, blended and distance language learning modes. Comput. Educ. 134, 31–40. doi: 10.1016/j.compedu.2019.01.016
Baig, M. M., GholamHosseini, H., and Connolly, M. J. (2015). Mobile healthcare applications: system design review, critical issues and challenges. Austral. Phys. Engin. Sci. Med. 38, 23–38. doi: 10.1007/s13246-014-0315-4
Bautista-Vallejo, J. M., Hernández-Carrera, R. M., Moreno-Rodriguez, R., and Lopez-Bastias, J. L. (2020). Improvement of memory and motivation in language learning in primary education through the interactive digital whiteboard (idw): The future in a post-pandemic period. Sustainability 12:8109. doi: 10.3390/su12198109
Benesty, J., Chen, J., Huang, Y., and Cohen, I. (2009). “Pearson Correlation Coefficient,” in Noise Reduction in Speech Processing. Springer Topics in Signal Processing, Vol. 2, (Heidelberg: Springer), doi: 10.1007/978-3-642-00296-0_5
Buabeng-Andoh, C. (2018). Predicting students’ intention to adopt mobile learning: A combination of theory of reasoned action and technology acceptance model. J. Res. Innov. Teach. Learn. 11, 178–191. doi: 10.1108/JRIT-03-2017-0004
Cho, Y. C., and Sagynov, E. (2015). Exploring factors that affect usefulness, ease of use, trust, and purchase intention in the online environment. Int. J. Manag. Inform. Syst. 19, 21–36. doi: 10.19030/ijmis.v19i1.9086
Choi, J. K., and Ji, Y. G. (2015). Investigating the importance of trust on adopting an autonomous vehicle. Int. J. Hum. Comput. Interact. 31, 692–702. doi: 10.1080/10447318.2015.1070549
Choy, D., and Cheung, Y. L. (2022). Comparison of primary four students’ perceptions towards self-directed learning and collaborative learning with technology in their English writing lessons. J. Comput. Educ. 3, 1–24. doi: 10.1007/s40692-022-00220-4
Danish, J., and Hmelo-Silver, C. E. (2020). On activities and affordances for mobile learning. Contemp. Educ. Psychol. 60:101829. doi: 10.1016/j.cedpsych.2019.101829
Deák, C., Kumar, B., Szabó, I., Nagy, G., and Szentesi, S. (2021). Evolution of new approaches in pedagogy and STEM with inquiry-based learning and post-pandemic scenarios. Educ. Sci. 11:319. doi: 10.3390/educsci11070319
Elkaseh, A. M., Wong, K. W., and Fung, C. C. (2016). Perceived ease of use and perceived usefulness of social media for e-learning in Libyan higher education: A structural equation modeling analysis. Int. J. Inform. Educ. Technol. 6, 192–199. doi: 10.7763/IJIET.2016.V6.683
Etikan, I., Musa, S. A., and Alkassim, R. S. (2016). Comparison of convenience sampling and purposive sampling. Am. J. Theoret. Appl. Stat. 5, 1–4. doi: 10.11648/j.ajtas.20160501.11
Falloon, G. (2020). From digital literacy to digital competence: the teacher digital competency (TDC) framework. Educ. Technol. Res. Devel. 68, 2449–2472. doi: 10.1007/s11423-020-09767-4
Fansury, A. H., Januarty, R., Wira, Ali, and Rahman, S. (2020). Digital content for millennial generations: Teaching the English foreign language learner on COVID-19 pandemic. J. Southwest Jiaotong University 3:55. doi: 10.35741/issn.0258-2724.55.3.40
Fuchs, K. (2021a). Preparing Students for Success in a Changing World: The Role of Virtual Whiteboards in the Modern Classroom. Educ. Q. Rev. 4, 151–158. doi: 10.31014/aior.1993.04.01.182
Fuchs, K. (2021b). Innovative Teaching: A Qualitative Review of Flipped Classrooms. Int. J. Learn. Teach. Educ. Res. 20, 18–32. doi: 10.26803/ijlter.20.3.2
Fuchs, K. (2022). An Exploratory Interview Study About Student Perceptions of Using Social Media to Facilitate Their Undergraduate Studies. Front. Educ. 7:834391. doi: 10.3389/feduc.2022.834391
Gan, C. L., and Balakrishnan, V. (2018). Mobile technology in the classroom: What drives student-lecturer interactions? Int. J. Hum. Comput. Interact. 34, 666–679. doi: 10.1080/10447318.2017.1380970
Gregorcic, B., Etkina, E., and Planinsic, G. (2018). A new way of using the interactive whiteboard in a high school physics classroom: A case study. Res. Sci. Educ. 48, 465–489. doi: 10.1007/s11165-016-9576-0
Hansen, J. M., Saridakis, G., and Benson, V. (2018). Risk, trust, and the interaction of perceived ease of use and behavioral control in predicting consumers’ use of social media for transactions. Comput. Hum. Behav. 80, 197–206. doi: 10.1016/j.chb.2017.11.010
Huang, F., Teo, T., Sánchez-Prieto, J. C., García-Peñalvo, F. J., and Olmos-Migueláñez, S. (2019). Cultural values and technology adoption: A model comparison with university teachers from China and Spain. Comput. Educ. 133, 69–81. doi: 10.1016/j.compedu.2019.01.012
Huang, F., Teo, T., and Zhou, M. (2020). Chinese students’ intentions to use the Internet-based technology for learning. Educ. Technol. Res. Devel. 68, 575–591. doi: 10.1007/s11423-019-09695-y
Huang, Y. M., and Chiu, P. S. (2015). The effectiveness of a meaningful learning-based evaluation model for context-aware mobile learning. Br. J. Educ. Technol. 46, 437–447. doi: 10.1111/bjet.12147
Ifinedo, E., Rikala, J., and Hämäläinen, T. (2020). Factors affecting Nigerian teacher educators’ technology integration: Considering characteristics, knowledge constructs, ICT practices and beliefs. Comput. Educ. 146, 103760. doi: 10.1016/j.compedu.2019.103760
Joshi, A., Kale, S., Chandel, S., and Pal, D. K. (2015). Likert scale: Explored and explained. Br. J. Appl. Sci. Technol. 7, 396–403. doi: 10.9734/BJAST/2015/14975
Keržiè, D., Tomaževiè, N., Aristovnik, A., and Umek, L. (2019). Exploring critical factors of the perceived usefulness of blended learning for higher education students. PLoS One 14:e0223767. doi: 10.1371/journal.pone.0223767
LaCaille, L. (2020). Theory of reasoned action. Encycl. Behav. Med. 2231–2234. doi: 10.1007/978-3-030-39903-0_1619
Lawrence, J. E., and Tar, U. A. (2018). Factors that influence teachers’ adoption and integration of ICT in teaching/learning process. Educ. Media Int. 55, 79–105. doi: 10.1080/09523987.2018.1439712
Lee, Y., Kozar, K. A., and Larsen, K. R. (2003). The technology acceptance model: Past, present, and future. Commun. Assoc. Inform. Syst. 12:50. doi: 10.17705/1CAIS.01250
Lewis, C. E., Chen, D. C., and Relan, A. (2018). Implementation of a flipped classroom approach to promote active learning in the third-year surgery clerkship. Am. J. Surg. 215, 298–303. doi: 10.1016/j.amjsurg.2017.08.050
Li, Y., Garza, V., Keicher, A., and Popov, V. (2019). Predicting high school teacher use of technology: Pedagogical beliefs, technological beliefs and attitudes, and teacher training. Technol. Knowl. Learn. 24, 501–518. doi: 10.1007/s10758-018-9355-2
Liaw, S. S., and Huang, H. M. (2013). Perceived satisfaction, perceived usefulness and interactive learning environments as predictors to self-regulation in e-learning environments. Comput. Educ. 60, 14–24. doi: 10.1016/j.compedu.2012.07.015
Love, J., Selker, R., Marsman, M., Jamil, T., Dropmann, D., Verhagen, J., et al. (2019). JASP: Graphical statistical software for common statistical designs. J. Statist. Softw. 88, 1–17. doi: 10.18637/jss.v088.i02
Madden, T. J., Ellen, P. S., and Ajzen, I. (1992). A comparison of the theory of planned behavior and the theory of reasoned action. Personal. Soc. Psychol. Bull. 18, 3–9. doi: 10.1177/0146167292181001
Maranguniæ, N., and Graniæ, A. (2015). Technology acceptance model: a literature review from 1986 to 2013. Univ. Access Inform. Soc. 14, 81–95. doi: 10.1007/s10209-014-0348-1
Mlambo, S., Rambe, P., and Schlebusch, L. (2020). Effects of Gauteng province’s educators’ ICT self-efficacy on their pedagogical use of ICTS in classrooms. Heliyon 6:e03730. doi: 10.1016/j.heliyon.2020.e03730
Molinillo, S., Aguilar-Illescas, R., Anaya-Sánchez, R., and Vallespín-Arán, M. (2018). Exploring the impacts of interactions, social presence and emotional engagement on active collaborative learning in a social web-based environment. Comput. Educ. 123, 41–52. doi: 10.1016/j.compedu.2018.04.012
Mondal, H., Mondal, S., Ghosal, T., and Mondal, S. (2018). Using Google forms for medical survey: A technical note. Int. J. Clin. Exp. Physiol. 5, 216–218. doi: 10.5530/ijcep.2018.5.4.26
Mou, J., Shin, D. H., and Cohen, J. (2017). Understanding trust and perceived usefulness in the consumer acceptance of an e-service: a longitudinal investigation. Behav. Inform. Technol. 36, 125–139. doi: 10.1080/0144929X.2016.1203024
O’Connor, N. (2021). Using active learning strategies on travel and tourism higher education programmes in Ireland. J. Hospital. Leis. Sport Tourism Educ. 29:100326. doi: 10.1016/j.jhlste.2021.100326
Reynolds, K. J., Subašiæ, E., and Tindall, K. (2015). The problem of behaviour change: From social norms to an ingroup focus. Soc. Personal. Psychol. Comp. 9, 45–56. doi: 10.1111/spc3.12155
Sánchez-Cruzado, C., Santiago Campión, R., and Sánchez-Compaña, M. (2021). Teacher digital literacy: The indisputable challenge after COVID-19. Sustainability 13:1858. doi: 10.3390/su13041858
Sandybayev, A. (2020). The impact of e-learning technologies on student’s motivation: Student centered interaction in business education. Int. J. Res. Tourism Hospital. 6, 16–24. doi: 10.20431/2455-0043.0601002
Schepers, J., and Wetzels, M. (2007). A meta-analysis of the technology acceptance model: Investigating subjective norm and moderation effects. Inform. Manag. 44, 90–103. doi: 10.1016/j.im.2006.10.007
Schmitt, T. A. (2011). Current methodological considerations in exploratory and confirmatory factor analysis. J. Psychoeducat. Assess. 29, 304–321. doi: 10.1177/0734282911406653
Stal, J., and Paliwoda-Pêkosz, G. (2019). Fostering development of soft skills in ICT curricula: a case of a transition economy. Inform. Technol. Devel. 25, 250–274. doi: 10.1080/02681102.2018.1454879
Sugeng, B., and Suryani, A. W. (2020). Enhancing the learning performance of passive learners in a Financial Management class using Problem-Based Learning. J. Univ. Teach. Learn. Pract. 17:5. doi: 10.53761/1.17.1.5
Techataweewan, W., and Prasertsin, U. (2018). Development of digital literacy indicators for Thai undergraduate students using mixed method research. Kasetsart J. Soc. Sci. 39, 215–221. doi: 10.1016/j.kjss.2017.07.001
Thornton, P., and Houser, C. (2005). Using mobile phones in English education in Japan. J. Comput. Assist. Learn. 21, 217–228. doi: 10.1111/j.1365-2729.2005.00129.x
Tondeur, J., Aesaert, K., Prestridge, S., and Consuegra, E. (2018). A multilevel analysis of what matters in the training of pre-service teacher’s ICT competencies. Comput. Educ. 122, 32–42. doi: 10.1016/j.compedu.2018.03.002
Torres Martín, C., Acal, C., El Honrani, M., and Mingorance Estrada, ÁC. (2021). Impact on the virtual learning environment due to COVID-19. Sustainability 13:582. doi: 10.3390/su13020582
Willis, R. L., Lynch, D., Fradale, P., and Yeigh, T. (2019). Influences on purposeful implementation of ICT into the classroom: An exploratory study of K-12 teachers. Educ. Inform. Technol. 24, 63–77. doi: 10.1007/s10639-018-9760-0
Wilson, C., Woolfson, L. M., and Durkin, K. (2022). The impact of explicit and implicit teacher beliefs on reports of inclusive teaching practices in Scotland. Int. J. Inclus. Educ. 26, 378–396. doi: 10.1080/13603116.2019.1658813
Yuan, S., Liu, Y., Yao, R., and Liu, J. (2016). An investigation of users’ continuance intention towards mobile banking in China. Inform. Devel. 32, 20–34. doi: 10.1177/0266666914522140
Appendix
SURVEY STATEMENTS |
Perceived Usefulness (PU); items adapted from Fuchs (2021a). |
PU1. Smartphone technology makes my classes more interesting. |
PU2. Smartphone technology makes my classes more fun. |
PU3. Smartphone technology makes my classes more active. |
PU4. Smartphone technology makes my classes more enjoyable. |
Perceived Ease of Use (PEU); items adapted from Fuchs (2021a). |
PEU1. I learn better during my class when using smartphone technology. |
PEU2. I would like my teacher to use smartphone technology more frequently. |
PEU3. I want to participate more in classes when using smartphone technology. |
PEU4. I learn more during the class when using smartphone technology. |
Attitude (ATT); adapted from Buabeng-Andoh (2018). |
ATT1. I look forward to class activities that require me to use smartphone technology. |
ATT2. I like working with smartphone technology during my university class. |
ATT3. I have positive feelings toward the use of smartphone technology in the classroom. |
Subjective Norm (SN); adapted from Buabeng-Andoh (2018). |
SN1. People who influence my behavior think that I should use smartphone technology. |
SN2. People who are important to me will support me to use smartphone technology. |
SN3. People whose views I respect support the use of mobile learning technology. |
Behavioral Intention (BI); adapted from Buabeng-Andoh (2018). |
BI1. I intend to use smartphone technology in the classroom in the future. |
BI2. I expect that I have to use smartphone technology in the classroom in the future. |
BI3. I plan to use smartphone technology in the classroom in the future. |
Keywords: technology-enhanced learning, higher education, undergraduate students, perceived usefulness, active learning, smartphone technology
Citation: Fuchs K (2022) Using an extended technology acceptance model to determine students’ behavioral intentions toward smartphone technology in the classroom. Front. Educ. 7:972338. doi: 10.3389/feduc.2022.972338
Received: 18 June 2022; Accepted: 29 August 2022;
Published: 20 September 2022.
Edited by:
Lee Kyung Oh, Sun Moon University, South KoreaReviewed by:
Mostafa Al-Emran, British University in Dubai, United Arab EmiratesMilan Kubiatko, J. E. Purkyne University, Czechia
Copyright © 2022 Fuchs. This is an open-access article distributed under the terms of the Creative Commons Attribution License (CC BY). The use, distribution or reproduction in other forums is permitted, provided the original author(s) and the copyright owner(s) are credited and that the original publication in this journal is cited, in accordance with accepted academic practice. No use, distribution or reproduction is permitted which does not comply with these terms.
*Correspondence: Kevin Fuchs, kevin.f@phuket.psu.ac.th