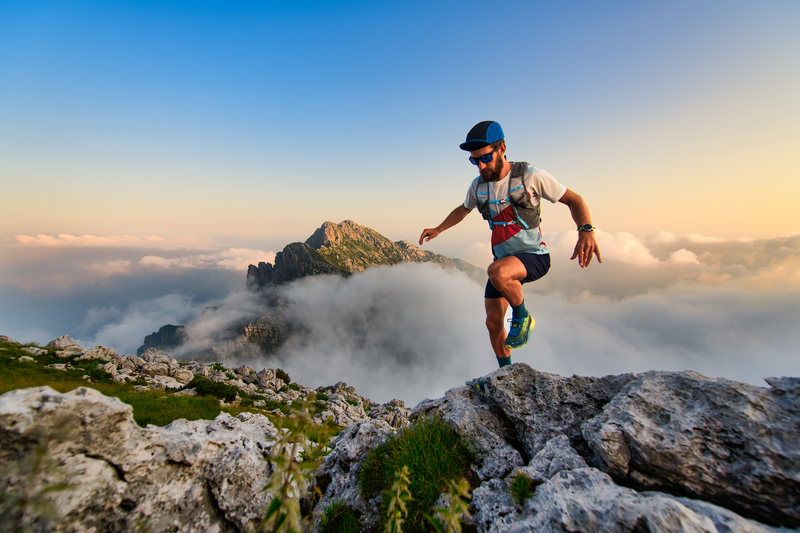
94% of researchers rate our articles as excellent or good
Learn more about the work of our research integrity team to safeguard the quality of each article we publish.
Find out more
ORIGINAL RESEARCH article
Front. Educ. , 29 July 2022
Sec. Digital Learning Innovations
Volume 7 - 2022 | https://doi.org/10.3389/feduc.2022.967117
This article is part of the Research Topic Digital Learning Innovations in Education in Response to the COVID-19 Pandemic View all 17 articles
It is rare to use “big data” in writing progression studies in the field of second language acquisition around the globe. The difficulty of recruiting participants for longitudinal studies often results in sample sizes that are too small for quantitative analysis. Due to the global pandemic, students began to face more academic and emotional challenges, and it became more important to track the progression of their writing across courses. This study utilizes big data in a study of over 4,500 students who took a basic English for Academic Purposes (EAP) course followed by an advanced one at a university in Hong Kong. The findings suggest that analytics studies can provide a range of insights into course design and strategic planning, including how students’ language use and citation skills improve. They can also allow researchers to study the progression of students based on the level of achievement and the time elapsed between the two EAP courses. Further, studies using mega-sized datasets will be more generalizable than previous studies with smaller sample sizes. These results indicate that data-driven analytics can be a helpful approach to writing progression studies, especially in the post-COVID era.
Students in higher education are often required to develop their academic writing skills by taking English for Academic Purposes (EAP) courses. Undergraduate programmes usually contain several of these courses. Various studies on undergraduate students have examined their development of EAP skills and/or language usage. They often deal with improvement after taking an EAP class (Archibald, 2001; Storch, 2009; Humphreys et al., 2012) or 1 year of undergraduate study (Knoch et al., 2014; Gan et al., 2015). However, there are frequently problems with recruiting participants in such test–retest studies, as students are usually unwilling to take tests without any benefit to themselves (Craven, 2012), resulting in the fairly limited use of mega-sized data to study the progression of literacy skills. The sample sizes of EAP progression studies range from approximately 25 (e.g., Storch, 2009) to 50 (e.g., Archibald, 2001; Humphreys et al., 2012) to just over 100 (Knoch et al., 2014). Recruitment of participants became an even more significant issue during the period of online education due to the COVID-19 pandemic. To complement their limited sample sizes, these studies often consult other data sources for further insight, such as coding academic essays (Storch, 2009; Knoch et al., 2014) and conducting student interviews (Humphreys et al., 2012; Gan et al., 2015). They have observed little improvement in students’ writing skills.
There is growing demand from practitioners and administrators for the use of big data methods, such as learning analytics, in progression studies to complement existing research methods and provide insights that can inform institution/department-level decision-making and strategic planning for student success. This is important for EAP courses as students in different academic disciplines are often offered the same course. Furthermore, when students take online classes, the evidence of their learning is primarily digital; therefore, big data analytics can be employed to obtain valuable insights. Learning analytics is “the measurement, collection, analysis and reporting of data about learners and their contexts, for purposes of understanding and optimizing learning and the environments in which it occurs” (Siemens and Baker, 2012). It helps identify at-risk students and improve learning outcomes (Hyland and Wong, 2017, p. 8). However, “its use and influence in language learning and teaching have thus far been minimal” (Thomas et al., 2017, p. 197). The power of learning analytics in an EAP context was demonstrated by various scholars before the pandemic (see Foung and Chen, 2019). The current study applies an innovative analytics approach to study the progression of students’ writing between two EAP courses.
The university where this study was conducted requires students to take two semester-long English language courses in their first 2 years. Local students (those from Hong Kong) take a secondary-school exit exam in English, the Hong Kong Diploma of Secondary Education (HKDSE English exam). At the research site, nearly half (49%) of the students admitted have obtained an overall Level 4 (equivalent to IELTS 6.31–6.51). While these students may not struggle with general language proficiency, they need to develop their writing skills to effectively handle university assignments (Morrison, 2014; Foung and Chen, 2019). This study explores these students’ progression from their first English course, Basic EAP (EAP1), to their second course, Advanced EAP (EAP2). While EAP1 teaches basic academic English skills, such as citing sources, writing simple argumentative essays and giving academic presentations, EAP2 requires students to write longer argumentative essays and present their research and views in a short oral defense. Since both courses include a take-home academic essay assessment and these are graded with the same set of assessment criteria, it is possible to study the progression of students’ academic writing skills from the first to the second course.
The current study aims to examine whether adopting data-driven analytics can provide useful insights to educational practitioners in the post-COVID era.
1. Did students’ writing skills improve between the two courses?
2. Did the time between the courses or students’ overall course grades affect the extent of students’ improvement? If yes, how?
3. How did the use of learning analytics contribute to the understanding of students’ writing development?
This study adopted a convenience sampling approach. Assessment data from the university’s learning management systems were retrieved for analysis, including the assessment results of 4,583 university students. Each EAP course lasts 13 weeks and meets for 3 h per week. Usually, EAP1 is taken in the first semester of Year 1. The departments in which students are enrolled can decide whether the two courses should be taken successively or with a semester or two in between.
Both EAP1 and EAP2 include an argumentative academic essay assignment. In EAP1, students are required to complete an 800-word academic essay using four sources on one of four given topics that they know well, such as education, transportation or the internet. EAP2 students are required to write a 1200-word essay that is related to an academic field, such as the use of genetic engineering or nuclear power, and incorporates a minimum of six academic sources. Each assessment is marked based on four criteria–content, organization, language and referencing–and uses the same grading descriptors (Table 1).
This study compares students’ performance on this writing assignment using a data-driven approach. Since both assessments are argumentative in nature and are marked with the same set of descriptors, they are considered comparable. The center offering these courses adopts stringent quality assurance mechanisms, including standardization exercises for all teachers, double-marking for new teachers and post-assessment moderation exercises. Teachers of different sections of the course are provided with a standardized site on the university management system and a set of course notes, which they use to deliver their lessons. These procedures ensure the reliability of the current study.
Both courses use the grade center in an online learning management system to record and disseminate grades. Each essay is marked according to the four criteria (Table 1) and receives a grade for each component, in addition to an overall grade. The university has adopted a common assessment system, according to which the following grades can be given: A +, A (“outstanding”), B +, B (“good”), C +, C (“satisfactory”), D +, D (“barely adequate”) and F (“inadequate”). With the help of IT colleagues, the take-home academic writing assessment grades were retrieved from the learning management system and converted to a scale of 0 to 4.5.
Unlike traditional studies, student progress was evaluated in two new ways. First, the researchers determined the number and proportion of students who earned the same grade, a lower grade and a higher grade for each assessment component. This tabulation is meaningful in view of the large sample size. They also computed the actual differences between the grades students received on the two assessments (and their effect sizes). In previous studies, such computations have not always been meaningful because their sample sizes were smaller.
For further analytics purposes, students were grouped in two ways: based on programme schedule and overall grades. In the former, students were grouped according to the time that elapsed between the two EAP courses in their degree programme. In practice, students who took EAP1 and EAP2 in two consecutive semesters were grouped together, while students who had at least one semester between the two courses formed another group. In addition, overall grades were considered. Students who achieved a grade of B (considered “good” at the university) or above in both courses were grouped together; all other students formed the second group. The semester during which students took the courses and their grades were stored in the learning management system by default and available for analysis in the current data-driven study.
The assessment results were subject to a series of analyses using IBM SPSS 21. Simple descriptive statistics and a paired sample t-test were used to answer the first part of RQ1. To compare the progress between the different groups, we adopted the independent sample t-test when necessary (RQ2).
Proper data cleaning procedures were applied to facilitate the use of the t-test, such as checking for normality and removing outliers. It should be noted that multiple (five) hypotheses were tested for RQ1, so the Bonferroni correction was applied to lower the alpha value from 0.05 to 0.01. Also, when examining the semester and grade factors, sample sizes between groups were unequal, so Welch’s statistics were used for the independent sample t-tests.
Critics may question the validity of using a rating (ordinal) scale to run inferential statistics tests. In fact, an ordinal variable can be treated as continuous when the underlying scale is assumed to be continuous and there are more than seven categories. In the current study, the scale used for the grades on the different components can be considered continuous and there are nine categories altogether. More importantly, it is common to use a rating scale (such as IELTS band scores or the band scores of writing tests) for inferential statistics in studies of writing progression (see Storch, 2009; Knoch et al., 2014; Gan et al., 2015).
In general, half of the students obtained a higher grade on the components of the EAP2 assessment, whereas roughly one-third received the same grade or a lower one (Table 2). It is important to note that more students received a higher grade on the skill-related components (content and referencing) than the proficiency-related components (language and organisztion).
Table 3 is an expanded version of Table 2, which presents the range of the grade changes. Overall, students demonstrated higher levels of grade increase (+ 3.0) than decrease (−2.0). The range of the grade change for “language” was the lowest of the four components (+ 2.0 to −2.0), while the range for “referencing” was the greatest (+ 3.0 to −2.0). Skill-related components featured a wider distribution (i.e., more students at the extremes) than proficiency-related components.
In addition, readers should be reminded that such analyses of range (as in Tables 3, 4) are only meaningful with a large sample size. Previous studies have often used absolute numbers to illustrate similar information to the data presented in Tables 3, 4 because using percentages to illustrate a proportion of a small sample (e.g., 30 participants) may not be very meaningful.
To understand the extent of students’ improvement, paired-sample t-tests were performed to compare their scores on each component of the EAP1 and EAP2 take-home essays. The results (Table 4) show that all mean differences were statistically significant, with medium to large effect sizes. Similar to the previous observation regarding the proportion of students who improved, there was a greater difference among skill-related components (from 0.33 to 0.44) than proficiency-related components (0.26).
It is evident from the statistical analyses that students improved to different extents on the various components. Referencing was the component that showed the greatest change, while changes in language use were minimal. In fact, this is the first time that referencing (including the technicalities of citation and the proper incorporation of sources) has been measured in a writing progression study using a scale. Most previous studies were conducted in a test setting, where citing sources was not required (Knoch et al., 2014). Archibald (2001) measured referencing based on the use of concrete examples; this definition is different from the referencing assessed in the current study. Although Storch (2009) also used coded data to explore how students used sources and paraphrasing in his progression study, he was more interested in the subtle changes in students’ linguistic skills, instead of the technicalities of citation defined in this study. Therefore, the current data-driven approach provides another dimension to the analysis of progress in referencing skills (one of the key components of academic literacy) and makes the current study unique. With a holistic scale to illustrate the improvement in students’ referencing skills, a more general evaluation could be made. These results will be important for course designers when considering how referencing skills should be presented in a course.
Other than referencing skills, the low degree of improvement in the “language” criterion is another interesting phenomenon. The current study was conducted using take-home assignments, but it had minimal practical differences from other studies. Without exception, past studies with test-based settings failed to find significant improvements in language (Archibald, 2001; Storch, 2009) and the current study unexpectedly echoed these findings. In the post-COVID era, language support may be deemed even more necessary, so more support should be provided to students.
The methodological difference between the current study with its big data approach and previous studies deserves further discussion. Typical progression studies compare the mean scores of different components and move on to “discourse measures,” which are computed based on the linguistic features of individual students’ writing (see Storch, 2009; Knoch et al., 2014). Some studies, such as Gan et al.’s (2015) study, have tabulated the extent of improvement in a detailed manner. However, with a relatively small sample size, the table could only include actual numbers, instead of percentages, to avoid misleading readers. This makes it hard for readers to interpret the extent of improvement in different components.
Also, obtaining consent to analyze students’ writing can be more challenging when classes are conducted online and teachers cannot develop a trusting relationship with students via the computer screen. With its comparatively large sample size, the current study tabulates the different levels of improvement for each assessment component (e.g., content and organization; see Tables 3, 4), which is useful information. For example, when the proportion of students in each category is observed, the differences in the range of various components become an indicator of variation (Table 3). The greater range evident in referencing (+ 3.0 to −3.0) means there was more variation in improvement or deterioration, whereas the smaller range in language (+ 2.0 to −1.5) means less variation. Even though the use of “discourse measures” in previous studies is a valid way to quantify a student’s linguistic patterns, the coding process can be time-consuming. More importantly, both the big-data approach (e.g., that of the current study) and the “discourse measures” approach lead to the same conclusion: improvement in language accuracy is minimal. This may suggest that the time-saving, big-data approach can be effective in writing progression studies.
To maximize the advantages of the big-data approach, the data were re-grouped based on when students took the courses and their overall grades. They were then used to explore if such analyses could provide insights for teaching and learning. More importantly, the following sections can illustrate the possibilities of data analytics in the post-COVID era.
The sample was divided into two groups: those who took the two courses consecutively (n = 3336) and those who took them with at least one semester in between (n = 1244). Table 5 illustrates the mean differences in the students’ scores on the different components of the take-home writing assessments. It indicates that, on average, both groups of students showed improvement in all components. These two datasets were then compared to find out which group of students showed more improvement.
All comparisons were statistically significant. Generally, there was a greater improvement among students who had at least one semester in between the two EAP courses than those who took them in succession. Once again, improvement was most obvious in the referencing component, with a mean difference of 0.22 points. In other words, students who took the two courses with a semester or two in between displayed a noticeably greater improvement in referencing skills than those who took the courses consecutively. The second-greatest difference was in the content component, another skill-related element. Proficiency-related components, including language and organization, revealed a smaller difference between the two groups of students. These results suggest that students who took the two courses consecutively did not improve as much as their counterparts who took them further apart.
The difference in assessment grades between students who took the two EAP subjects in succession and those who took them a semester or a year apart merits further discussion. As reflected in Table 5, students who had a “break” between the two EAP courses showed greater improvement in all four assessment components–content, organization, language and referencing–as well as their overall results, than those who did not. While all differences in the assessment scores were statistically significant, the greatest differences were associated with referencing and content. A plausible explanation for this result is that, during the “break” between the two EAP subjects, students continued to take five academic courses per semester and needed to write academic essays and reports for them. This means that they had the opportunity to practise the academic English skills they learned in the first EAP course; applying them in the context of other courses allowed them to polish these skills. During the “break” between the two EAP subjects, students may have experienced the language requirements of lecturers in other subjects (Ferris and Tagg, 1996), resulting in a deeper understanding of the instrumental relevance of EAP skills to their university studies and academic achievement.
Previous studies have rarely explored this factor. They have been more interested in how an EAP course or other intervention affects the progression of undergraduate students; even when they grouped students based on various factors, they often did not examine the semester during which students took the course. A traditional longitudinal design may not have been effective to analyze this factor, but it can be explored with a big-data approach, as in the current study. As previously mentioned, Craven (2012) had difficulties during the participant-recruitment process because few students were willing to take part in exams twice. If certain parameters for the participants (e.g., study patterns of English courses) are set, it will be even more difficult for traditional longitudinal studies to recruit student participants. In contrast, the current study made use of data already present in the university’s learning management system, including the semesters when students took EAP courses. The advantage of having readily available data will only be intensified in the post-COVID era. Analyzing this factor was easy and effective in the current data-driven study. It has the potential to help researchers understand writing progression in the post-COVID era.
Further analyses were conducted by comparing students who received high final course grades with those who did not. Students who did consistently well in both courses (with a grade of “B” or above) were grouped as “well-performing students,” whereas those who did not meet this requirement were grouped as “average students.” An independent sample t-test was conducted to see if these two groups of students showed different levels of progress. Table 6 lists the mean improvement levels of both groups of students. In general, both groups of students showed improvement. The mean differences between the two groups were then computed.
Surprisingly, the well-performing students did not achieve greater improvement in all aspects, only in three out of four components. Furthermore, not all comparisons were statistically significant. In other words, even though the well-performing students received high scores in the courses in general, they did not always make significantly greater improvements than the average students. In particular, they only showed improvement that was great enough to be statistically significant in content and organization. While both groups of students showed some improvement in referencing skills, the average students showed slightly greater improvement in this aspect; however, the difference was not statistically significant.
One further point to note is that the progression of students at all different levels of course performance was observed. Although, as expected, students in the well-performing group (i.e., students with higher overall English subject grades) improved more than their peers, our statistical analysis showed that students in the average group also made some progress, especially in the content and referencing aspects. The difference between the two groups of students’ progress in terms of language use was rather minimal. This is perhaps an area that subject leaders should pay attention to–for example, by considering how EAP courses, especially the second one, can challenge students to accelerate their language development.
The results of this study may extend the understanding of the differences between students with different proficiency levels. Knoch et al. (2014) grouped the 101 students in their study into three different proficiency groups to identify differences in progression among these groups. Their comparison was mainly conducted using manually coded linguistic features, such as the use of academic words and grammatical accuracy. They found no statistically significant results related to any of the linguistic features they studied. This echoes the findings of the current study, wherein no significant difference in language improvement was observed. However, it is important to note that the participants in the current study were grouped based on overall course grades, which can be attributed to both proficiency and effort. With such consistent results, the preliminary conclusion can be reached that the proficiency of students may not necessarily play a vital role in affecting their progression. Perhaps the more important question in the post-COVID era is which other engagement or demographic factors contribute to writing progression.
The present study provides a new and extensive expansion of traditional progression studies and demonstrates the potential of data analytics in the post-COVID era. With a larger sample size, the current data-driven study was able to tabulate and visualize students’ progression in different areas more effectively than studies using other approaches. In addition, the present study was able to make stronger claims by using inferential statistics and employed a range of readily available variables from the university’s learning management system to conduct sophisticated analyses without going through individual students’ essays. This demonstrates the advantages of using learning analytics to explore students’ progress. It also makes an important Contribution To The Field of second language acquisition around the globe. Data stored on learning management systems, even during online classes, can provide useful insights into the progression of their writing in the post-COVID era.
In spite of the effort to produce valid and reliable results, this study has several limitations. First, it only provides a macro picture of students’ progress. Since the study advocates the use of a big-data approach, the authors did not use other methods (such as focus group interviews) to triangulate the results obtained from the quantitative data. In reality, the research site has regular QA measures that it adopts every semester (e.g., student–staff feedback meetings and questionnaires). The results of this study offer a big-data perspective to enrich the QA process. Second, the progression study presented here is based on the fact that the two assessments are highly comparable, but they are not exactly the same. One difference between this study and previous ones is that previous studies have made use of standardized tests, whereas this study examines students’ progress on comparable course-based assessments. While the authors believe that differences between the assignments do not affect the validity of the study (see the Methodology section for details), the minimal differences between the assignments may limit its generalizability to a certain extent.
In light of the success of the current study, further research can take advantage of the big data era to explore students’ progression in other aspects of language learning, such as speaking and reading skills, in online courses. For example, researchers can examine interactions during online classes and how students’ use of language improves. This will help EAP practitioners understand the lessons learnt regarding online instruction during the pandemic and provide new insights for the post-COVID era.
The datasets presented in this article are not readily available because the authors have to observe the Data Governance Framework which approved this project. Requests to access the datasets should be directed to DF, ZGVubmlzLmZvdW5nQGdtYWlsLmNvbQ==.
Ethical review and approval was not required for the study involving human participants in accordance with the local legislation and institutional requirements. Written informed consent for participation was not required for this study in accordance with the national legislation and the institutional requirements.
Both authors planned and drafted the manuscript together.
The authors declare that the research was conducted in the absence of any commercial or financial relationships that could be construed as a potential conflict of interest.
All claims expressed in this article are solely those of the authors and do not necessarily represent those of their affiliated organizations, or those of the publisher, the editors and the reviewers. Any product that may be evaluated in this article, or claim that may be made by its manufacturer, is not guaranteed or endorsed by the publisher.
Archibald, A. (2001). Targeting L2 writing proficiencies: Instruction and areas of change in students’ writing over time. Int. J. English Stud. 1, 153–174.
Craven, E. (2012). “The quest for IELTS Band 7.0: Investigating English language proficiency development of international students at an Australian university,” in IELTS Research Reports Volume 13, (Melbourne: IDP), 1–61.
Ferris, D., and Tagg, T. (1996). Academic oral communication needs of EAP learners: What subject-matter instructors actually require. TESOL Quart. 30, 31–58. doi: 10.2307/3587606
Foung, D., and Chen, J. (2019). Disciplinary challenges in first-year writing courses: A big data study of students across disciplines at a Hong Kong university. Asian EFL J. 25, 113–131.
Gan, Z., Stapleton, P. A. U. L., and Yang, C. C. R. (2015). What happens to students’ English after one year of English-medium course study at university? Appl. Lang. Learn. 25, 71–91.
Humphreys, P., Haugh, M., Fenton-Smith, B., Lobo, A., Michael, R., and Walkinshaw, I. (2012). “Tracking international students’ English proficiency over the first semester of undergraduate study,” in IELTS Research Reports Online Series, 41. Available online at: http://gallery.mailchimp.com/d0fe9bcdc8ba233b66e1e0b95/files/Humphreys2012_ORR.pdf (accessed February 1, 2022).
Hyland, K., and Wong, L. (2017). “Faces of English language research and teaching,” in Faces of English Education, eds L. Wong and K. Hyland (New York, NY: Routledge), 1–9.
Knoch, U., Rouhshad, A., and Storch, N. (2014). Does the writing of undergraduate ESL students develop after one year of study in an English-medium university? Assessing Writing 21, 1–17. doi: 10.1016/j.asw.2014.01.001
Morrison, B. (2014). Challenges faced by non-native undergraduate student writers in an English-Medium university. Asian ESP J. 1, 137–175.
Siemens, G., and Baker, R. S. (2012). “Learning analytics and educational data mining: towards communication and collaboration,” in Proceedings of the 2nd International Conference on Learning Analytics and Knowledge, (New York, NY: ACM), 252–254. doi: 10.1145/2330601.2330661
Storch, N. (2009). The impact of studying in a second language (L2) medium university on the development of L2 writing. J. Second Lang. Writing 18, 103–118. doi: 10.1016/j.jslw.2009.02.003
Keywords: learning analytics, progression, improvement in writing, EAP (English for academic purposes), sequential analytics
Citation: Foung D and Chen J (2022) Tracing writing progression in English for academic purposes: A data-driven possibility in the post-COVID era in Hong Kong. Front. Educ. 7:967117. doi: 10.3389/feduc.2022.967117
Received: 12 June 2022; Accepted: 12 July 2022;
Published: 29 July 2022.
Edited by:
Mark Bedoya Ulla, Walailak University, ThailandReviewed by:
Paolo Nino Valdez, De La Salle University, PhilippinesCopyright © 2022 Foung and Chen. This is an open-access article distributed under the terms of the Creative Commons Attribution License (CC BY). The use, distribution or reproduction in other forums is permitted, provided the original author(s) and the copyright owner(s) are credited and that the original publication in this journal is cited, in accordance with accepted academic practice. No use, distribution or reproduction is permitted which does not comply with these terms.
*Correspondence: Dennis Foung, ZGVubmlzLmZvdW5nQGdtYWlsLmNvbQ==
Disclaimer: All claims expressed in this article are solely those of the authors and do not necessarily represent those of their affiliated organizations, or those of the publisher, the editors and the reviewers. Any product that may be evaluated in this article or claim that may be made by its manufacturer is not guaranteed or endorsed by the publisher.
Research integrity at Frontiers
Learn more about the work of our research integrity team to safeguard the quality of each article we publish.