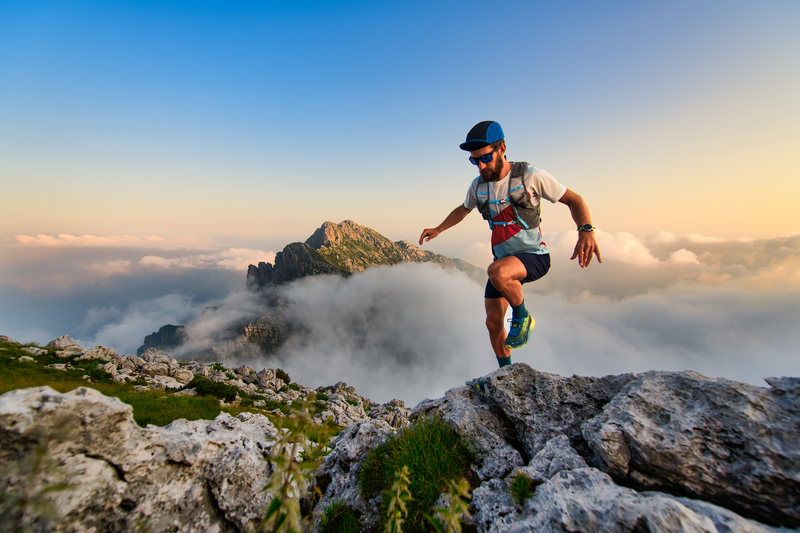
95% of researchers rate our articles as excellent or good
Learn more about the work of our research integrity team to safeguard the quality of each article we publish.
Find out more
ORIGINAL RESEARCH article
Front. Educ. , 06 October 2022
Sec. STEM Education
Volume 7 - 2022 | https://doi.org/10.3389/feduc.2022.953164
This article is part of the Research Topic Innovations in Remote and Online Education by Hydrologic Scientists View all 16 articles
The need to adapt quickly to online or remote instruction has been a challenge for instructors during the COVID pandemic. A common issue instructors face is finding high-quality curricular materials that can enhance student learning by engaging them in solving complex, real-world problems. The current study evaluates a set of 15 web-based learning modules that promote the use of authentic, high-cognitive demand tasks. The modules were developed collaboratively by a group of instructors during a HydroLearn hackathon-workshop program. The modules cover various topics in hydrology and water resources, including physical hydrology, hydraulics, climate change, groundwater flow and quality, fluid mechanics, open channel flow, remote sensing, frequency analysis, data science, and evapotranspiration. The study evaluates the impact of the modules on students’ learning in terms of two primary aspects: understanding of fundamental concepts and improving technical skills. The study uses a practical instrument to measure students’ perceived changes in concepts and technical skills known as the Student Assessment of Learning Gains (SALG) survey. The survey was used at two-time points in this study: before the students participated in the module (pre) and at the conclusion of the module (post). The surveys were modified to capture the concepts and skills aligned with the learning objectives of each module. We calculated the learning gains by examining differences in students’ self-reported understanding of concepts and skills from pre- to post-implementation on the SALG using paired samples t-tests. The majority of the findings were statistically at the 0.05 level and practically significant. As measured by effect size, practical significance is a means for identifying the strength of the conclusions about a group of differences or the relationship between variables in a study. The average effect size in educational research is d = 0.4. The effect sizes from this study [0.45, 1.54] suggest that the modules play an important role in supporting students’ gains in conceptual understanding and technical skills. The evidence from this study suggests that these learning modules can be a promising way to deliver complex subjects to students in a timely and effective manner.
Hydrologists and water resource engineers deal with intricate and complex problems that are situated in natural-human ecosystems with several interconnected biological, physical, and chemical processes occurring at various spatial and temporal dimensions. In recent years, there has been a movement to enhance hydrology education (CUAHSI, 2010). Therefore, there is a growing need to better equip the next generation of hydrologists and water resource engineers to handle such complicated problems (Bourget, 2006; Howe, 2008; Wagener et al., 2010; Ledley et al., 2015). Some of the key desired enhancements in hydrology and water resource engineering education require exposure to data and modeling tools, adoption of effective pedagogical practices such as active learning, and use of case studies to deliver real-world learning experiences (Habib and Deshotel, 2018). In their review of hydrology education challenges, Ruddell and Wagener (2015) stressed the need for structured methods for hydrology education, such as community-developed resources and data- and modeling-based curriculum. The increasing availability of digital learning modules that incorporate such attributes provide opportunities for addressing the desired enhancements. Recent examples of such growing resources in the field of hydrology and water resources include: Environmental Data-Driven Inquiry and Exploration (EDDIE; Bader et al., 2016); online modules from the HydroViz platform (Habib et al., 2019a,b); HydroShare educational resources (Ward et al., 2021); web-based simulation tools (Rajib et al., 2016); HydroFrame tools for groundwater education (HydroFrame-Education, n.d.); geoinformatics modules for teaching hydrology (Merwade and Ruddell, 2012); and the HydroLearn hydrology and water resources online modules (Habib et al., 2022).
The potential value of digital resources has been further highlighted during the COVID-19 pandemic when instructors were forced to switch to remote teaching and find resources to facilitate their teaching and support students’ learning (Loheide, 2020). However, the rapid acceleration of instructional resources available via the internet makes it difficult for instructors to assess the quality, reliability, and effectiveness of such resources. Instructors’ decisions to adopt certain digital resources are based on the digital resource’s potential to enhance student learning and alignment to the instructor’s learning objectives (Nash et al., 2012). Evidence of improved student learning is often cited as important factors that affect instructors’ adoption of education innovations (Borrego et al., 2010; Bourrie et al., 2014). Therefore, there is a need to continuously evaluate the emerging educational resources and assess their potential impact on students’ learning (Merwade and Ruddell, 2012; Ruddell and Wagener, 2015). The impact of a given instructional resource on students’ learning can be assessed in terms of two key components: (a) impact on conceptual understanding of fundamental topics in hydrology and water resources, and (b) impact on technical skills that students need to identify and solve problems (Herman and Klein, 1996; Woods et al., 2000; Kulonda, 2001; Entwistle and Peterson, 2004; Sheppard et al., 2006; Yadav et al., 2014). Moreover, it is important for instructors to weigh not only the impact on students, but also the cost of the instructional resources before adopting the materials (Kraft, 2020). Costs of resources may be direct, such as subscription fees, or indirect, such as the instructor’s time. Resources with high cost, even if they have an impact on student learning, may not be feasible for an instructor to adopt.
One way to support students’ learning is through authentic, high-cognitive demand tasks. High-cognitive demand tasks are defined by Tekkumru-Kisa et al. (2015) as those which require students to “make sense of the content and recognize how a scientific body of knowledge is developed” (p.663). High-cognitive demand tasks, which have been widely researched in mathematics and science education, are open-ended or unstructured and challenge students to use the knowledge they have gained to engage in the problem-solving process (Stein et al., 1996; Boston and Smith, 2011; Tekkumru-Kisa et al., 2020). Low-cognitive demand tasks are those that need little to no deep comprehension; examples include tasks that involve scripts (e.g., a list of instructions or procedures) or memorization (e.g., definitions, formulae) since the task has just one correct answer or is otherwise plainly and directly stated. The level of cognitive demand of a task can also be identified with the aid of Bloom’s Taxonomy (Anderson and Krathwohl, 2001). For instance, low-cognitive demand tasks can be characterized by the lower three levels of the taxonomy (i.e., remember, understand, and apply). Alternatively, tasks of high-cognitive demand are evocative of the higher levels of the taxonomy (i.e., analyze, evaluate, and create).
Authentic tasks are a subset of high-cognitive demand tasks. In contrast to problem sets, which often have one clean, neat answer, authentic tasks are ill-defined problems with real-world relevance which have multiple possible solutions (Herrington et al., 2003). Authentic can pertain to the types of problems students are asked to solve and the tools required to address those problems. Authentic tasks should involve the integrated applications of concepts and skills to emulate the tasks that professionals would perform (Brown et al., 2005; Prince and Felder, 2007). For example, if modelers use Hydrologic Engineering Center’s (HEC) Statistical Software Package (SSP) to perform frequency analyses, then the authentic task should incorporate the use of that program. Within engineering education research, authentic tasks are sometimes referred to as case-based instruction. In case-based instruction, the topic of the lesson or module is embedded in a case study to allow students to draw real-world connections and apply the information in realistic problem scenarios (Prince and Felder, 2007). This approach has the potential to enhance students’ understanding of principles and practices (Kardos and Smith, 1979; Kulonda, 2001) and increase their awareness of critical issues in the field (Mayo, 2002, 2004). Students who learn from cases have a higher conceptual understanding, can transfer their knowledge, and can solve real-world problems (Dori et al., 2003; Miri et al., 2007; Hugerat and Kortam, 2014). Furthermore, students benefit from the use of authentic tasks because it allows them to learn lessons while addressing problems, understand when to apply such lessons, and how to adapt the lessons for novel situations (Kolodner, 2006). Furthermore, the use of authentic problems may increase the problem’s relevance for students and their enthusiasm for finding a solution, as well as provide them with the opportunity to work on open-ended questions (Fuchs, 1970; Bransford et al., 2004). Open-ended assessments, where students are asked to participate in higher-cognitive demand tasks (e.g., analyze, evaluate, and create), can demonstrate the students’ level of understanding of the concepts. Authentic tasks also allow the instructor to provide opportunities for students to practice their skills and communicate about them in a professional context (Hendricks and Pappas, 1996; Pimmel et al., 2002).
These studies suggest that the use of authentic tasks in engineering education has great potential. However, there is limited research on how the inclusion of such strategies may support the development of conceptual understanding and technical skills in hydrology and water resources education specifically. The current study will evaluate a set of web-based learning modules developed as part of the HydroLearn platform (Gallagher et al., 2022; Habib et al., 2022). The modules cover a wide range of concepts and technical skills and incorporate authentic, high-cognitive demand tasks with the goal of developing students’ conceptual understanding and technical skills. The research question addressed in this study is: Are there differences in students’ self-reported learning gains in conceptual understanding and technical skills after participating in each of the online learning modules designed around authentic, high cognitive demand tasks?
The HydroLearn platform1 hosts nearly 50 authentic, online learning modules. The platform was specifically designed with a vision to influence adoption: compatibility, relative advantage, observability, trialability, and complexity (Rogers, 2003). The HydroLearn platform was developed using a deployment of the well-established open source edX platform, OpenEdx, (The Center for Reimagining Learning Inc, 2022) with hydrology education-driven enhancements, such as scaffolding wizards and templates to support the development of learning objectives, learning activities, and assessments (Gallagher et al., 2021; Lane et al., 2021). A unique feature of HydroLearn is that it allows instructors to adapt modules that were developed by other contributors and customize them for their own purposes, while following proper attribution and license requirements. This is intended to facilitate a wider use and dissemination of the learning resources beyond their own immediate developers, and thus promotes the concept of building a collaborative community of instructors around the concepts of open-source and open-access authentic learning content.
HydroLearn modules were created purposefully to: (a) represent key topics covered in undergraduate hydrology and water resources courses, (b) be used as is or customized according to the needs of the adopter, (c) integrate web-based, open-source tools, (d) be crafted in alignment with research on curriculum design, and (e) offer support for faculty adopters. Additionally, they are easy to implement as many instructors simply assign the chosen module to be completed outside of class. Although all modules are freely available on the platform, there are indirect costs to instructors such as needing time to review the modules before deciding to use them. Most HydroLearn modules were developed and peer-reviewed during a hackathon-style immersive workshop (see Gallagher et al., 2022 for details on this process). They were designed to incorporate at least one authentic, high-cognitive demand task that requires students to apply the conceptual understanding and technical skills gained throughout the module to devise a solution to the task. The modules have learning objectives, instructional content, and assessment tasks aligned with those objectives.
For the purposes of this study, we examined 15 of the HydroLearn modules, which were predominantly developed collaboratively during the COVID-19 pandemic by groups of instructors (2–3 instructors per module) who then used them for teaching at their respective institutions in primarily undergraduate classes. Five modules were not developed during the pandemic, of which one was developed by an individual. We chose these because they were used during the pandemic and had been used with enough students for us to draw inferences. These modules, which were written for upper-level undergraduate and early graduate students enrolled in water resources courses, cover a broad range of topics, such as physical hydrology, hydraulics, climate change, groundwater flow and quality, fluid mechanics, open channel flow, remote sensing, frequency analysis, data science, and evapotranspiration. Most of these modules can be completed individually and non-sequentially and were assigned by instructors to be completed outside of class time. Some modules could be completed in a week’s time, while others were assigned to be completed throughout an entire semester. Details about the topics, concepts and technical skills covered by the 15 modules are available in the Supplementary material. Supplementary Table 1 provides a short description of each module and its authentic task(s). Supplementary Table 2 lists the concepts and technical skills for the modules, all of which were identified by the module developers.
The modules examined in this study include a common set of characteristics: frequent self-assessment questions, learning activities structured around an authentic task, and open-source materials. All the modules contain frequent Check Your Understanding questions that allow students to assess their level of understanding of the learning material. These questions are intentionally placed to re-engage the student and provide immediate feedback (Woods et al., 2000). Another common component that the modules share is the inclusion of a set of Learning Activities, structured around an authentic task, which emulate the work a professional scientist or engineer would be doing in their career (Herrington et al., 2003). Although all the modules use open-source materials, the materials they use vary. For instance, open data and modeling platforms (Lane et al., 2021), remote sensing data and tools (Maggioni et al., 2020) professional engineering software (Polebitski and Smith, 2020), and real-world case studies that increase relevance and engagement for students (Arias and Gonwa, 2020; McMillan and Mossa, 2020).
A total of 299 participants, both graduate (n = 56) and undergraduate students (n = 243), used the 15 HydroLearn modules between the spring 2020 and fall 2021 semesters, consented to participate in our study, and had complete data. The participants in this study are the students whose instructors chose to use these 15 modules in their courses. Out of the total number of students, 57% (n = 171) identified as male, 38% (n = 113) identified as female, 1% (n = 4) identified as non-binary, 1% (n = 2) preferred not to answer, and 3% (n = 8) selected other; 77% (n = 228) identified as white, 3% (n = 9) identified as Black or African American, 2% (n = 6) identified as American Indian or Alaska Native, 16% (n = 48) identified as Asian, 1% (n = 3) identified as Native Hawaiian or Pacific Islander, 4% (n = 13) preferred not to answer, and 3% (n = 10) selected other. These students were at 15 different universities across the USA. Table 1 provides demographic details of the study participants organized by module.
Supplementary Table 3 provides details about the universities that participated in the study. We used the most recent student population reports in conjunction with the rankings from the National Association for College Admission Counseling (CollegeData n.d.) to assign the university to a size range. We considered a university small if the student population is less than 5,000, medium for populations between 5,000 and 15,000, and large for populations greater than 15,000 students.
Each student completed the Student Assessment of Learning Gains (SALG; Seymour et al., 2000) survey before they used the module (pre) and shortly after they finished the module’s final assignment (post). The SALG is a tool that can be used to measure the knowledge and understanding of key concepts and processes that students believe they have achieved as a result of participating in a particular module (Seymour et al., 2000). It can be customized to fit any pedagogical approach or discipline. The SALG instrument has been used to assess students’ gains in numerous studies, including some in the field of hydrology education (e.g., Endreny, 2007; Aghakouchak and Habib, 2010). Separate versions of the SALG were created for each module, and each version includes a list of concepts and skills aligned with the module’s learning objectives (see Supplementary Table 2).
The SALG is divided into two scales in which students self-report their understanding of concepts and competency in employing technical skills that are the subject of the module. The concepts statement begins with, “Presently, I understand the following concepts that will be explored in this module…” followed by items that represent the key concepts from the module. Students indicate to what degree they understand each item using a 6-point Likert scale rated from 1 – Not applicable to 6 – A great deal. For example, one of the concept items for the “Hydrologic Droughts and Drying Rivers” module is “Presently, I understand the following concepts that will be explored in this module…drought indices.” The concepts section in the SALG is followed by the skills section, which states, “Presently, I can…” followed by items that represent the technical skills students are exposed to through the use of the module. Students rate the skills items on the same Likert scale. “Presently, I can…Calculate drought indices using USGS streamflow data” is an example of a skill item from the “Hydrologic Droughts and Drying Rivers” module. A student’s responses to all items within each scale (i.e., one for conceptual understanding and one for technical skills) were averaged to determine their pre- and post-module scores.
A survey’s reliability is an important sign of the instrument’s capacity to produce reliable and consistent results [i.e., how closely related the set of items (e.g., concepts or skills) are for all students for one module]. The internal consistency of a survey can be measured using Cronbach’s alpha (Cronbach, 1984). For each scale, we determined the Cronbach’s alpha (i.e., concepts and skills for each module). If the scores are reliable, they will relate at a positive, reasonably high level, with Cronbach’s alpha ≥0.6 considered acceptable (Cresswell and Guetterman, 2019). The concept and skills scales’ reliabilities across all modules ranged from 0.74 to 0.95.
We opted to merge data acquired from the identical modules used at different universities in the study. Data were only combined if they came from the same module (i.e., no updates or alterations at all). We made this decision for several reasons, the first of which is that the sample size is frequently insufficient for analysis, particularly in graduate-level courses. Second, by evaluating all the data for students who had completed a specific module, we were able to determine whether students felt they had attained the concepts and skills taught in that module, regardless of their university. Furthermore, because most instructors assigned the modules to be completed outside of class time, the university that used the modules was relatively irrelevant.
To answer our research question and investigate if the modules lead to a change in concepts or skills, we examined the difference in means from pre to post using paired samples t-tests. The paired samples t-test is commonly used to examine the difference between paired means (Zimmerman, 1997). We first tested the data to ensure it met the assumption of normality by examining the skewness and kurtosis of each scale. If a scale was found to be non-normally distributed, we used the Wilcoxon signed-rank test (Wilcoxon, 1945) instead of a paired samples t-test to determine whether there were statistically significant differences from pre to post (Siegel, 1956). For normally distributed scales, we moved forward with the paired samples t-tests.
One disadvantage of running so many tests (n = 30, 2 for each of the 15 modules) is that it raises the likelihood of wrongly rejecting the null hypothesis (i.e., a Type I error). To correct for the increased probability of Type I error, we employed the Benjamini–Hochberg procedure (Benjamini and Hochburg, 1995). The Benjamini–Hochberg procedure is a straightforward, sequential approach for reducing the rate of false discovery and is dependent upon the proportion of false discoveries. A discovery is the number of non-zero confidence intervals in a data set. A discovery can demonstrate that the difference noticed in the samples is not only attributable to chance (Soriæ, 1989). In this study, the number of discoveries was equal to the number of tests; therefore, the false discovery rate was reduced to α, which for this study was set at α = 0.05. After the Benjamini–Hochberg correction factor was determined, each p-value had an associated Benjamini–Hochberg critical value. A variable was considered significant if the Benjamini–Hochberg correction factor was less than α.
After determining which tests were statistically significant using the Benjamini–Hochberg correction, we assessed the effect sizes to see if the changes between pre and post made practical sense (i.e., does this difference matter?). According to Warner (2012), pp. 35), “effect size is defined as an index of … the magnitude of the difference between means, usually given in unit-free terms; effect size is independent of sample size.” According to Cresswell and Guetterman (2019), the effect size is a way of determining the strength of a study’s conclusions about group differences or the link between variables. This study measured effect size using two methods: Cohen’s d (Cohen, 1988) for normally distributed scales and requivalent (Rosenthal and Rubin, 2003) for non-normally distributed scales. Cohen’s d describes the difference between the means in terms of standard deviations for normal distributions. In educational research, a value of 0.4 or higher is considered impactful (Hattie, 2009). For each effect size, we describe Cohen’s d in terms of size categories, which are small (0.2 – 0.49), medium (0.50 – 0.79), and large (≥0.8) (Cohen, 1988). Alternatively, requivalent is designed specifically for non-parametric procedures (among other situations) as an indicator of effect size. The size bins for r used in this study are described as r = 0.10 (small effect; effect explains 1% of the total variance), r = 0.30 (medium effect; effect explains 9% of the total variance) and r = 0.50 (large effect; effect accounts for 25% of the total variance) (Field, 2018).
Table 2 presents the reliability, pre- and post-mean scores and standard deviations, significance, and the effect size (Cohen’s d or requivalent) organized by module. We found that all but one of the scales had statistically significant and practically significant differences from pre to post. Only one scale (Physical Hydrology, conceptual understanding) did not have statistically significant differences from pre to post; however, it was approaching significance, p = 0.050, and the sample size was small (n = 8). Given the effect size of 0.85, the small sample size, and the p-value approaching significance, this suggests there was not enough power to detect statistical significance for this scale. A power analysis suggests that 16 students would be needed to find a statistically significant difference (Warner, 2012). To clarify, we do not have reason to think that this module is any less effective than the others. These results suggest that the students who participated in these modules felt that they had a greater understanding of the concepts and a greater ability to apply the skills after completing each module, as compared to before.
Table 2. Reliability, pre- and post-mean scores and standard deviations, significance, and the effect size (Cohen’s d or requivalent) organized by module.
One of the most important findings of our study is the magnitude of the effect sizes. We found that only one scale had a small effect size (Development of Design Storms, conceptual understanding). The remaining scales were fairly evenly divided between medium and large effect sizes. Moreover, the fact that the effect sizes of all the t-tests we conducted were greater than the Cohen’s d = 0.4 benchmark and requivalent benchmark r = 0.50 typically considered impactful in education research (Hattie, 2009; Field, 2018) suggests that these modules may have a substantial practical effect on students’ learning of concepts and skills.
Our results suggest that the students who participated in this study felt that they had greater conceptual understanding and technical skills after completing every one of the HydroLearn modules with the exception of Physical Hydrology conceptual understanding, as compared to before. Furthermore, the outcomes of this study align with previous research wherein students also used short modules designed to enhance their proficiency in applying technical skills to complete a task derived from the modules’ learning objectives (Pimmel, 2003). Our results also support the idea that learning may be greater when conceptual understanding is directly linked to a real-world problem, and students are required to use professional tools and technical skills to propose a solution to the problem, as suggested by Brown et al. (2005) and Prince and Felder (2007). Similar to past research (Hiebert and Wearne, 1993; Stein and Lane, 1996; Boaler and Staples, 2008), our study also found that student learning was greater in courses that include high-cognitive demand tasks that stimulate high-level reasoning and problem-solving. Additionally, Kraft (2020) suggested that researchers should look not just at effect size, but at effect size compared to the cost of an educational intervention. Implementing HydroLearn modules in a water resources or hydrology course costs the instructor some time to prepare, but there are no direct costs to using these modules, as they are all freely available on our website. The findings from this study suggest that HydroLearn modules provide a very cost-effective way to improve water resources and hydrology students’ understanding of key concepts and skills.
This study sought to answer the research question: Are there differences in students’ self-reported learning gains in conceptual understanding and technical skills after participating in each online learning module designed around authentic, high cognitive demand tasks? The results of this study suggest that students who completed these modules reported that they had a greater conceptual understanding of key topics and developed proficiency in technical skills required to solve authentic problems. Most notably, the effect sizes of this study [0.45, 1.54] surpass the average effect size found in education research (0.40). These results suggest that the modules may relate to the growth of students’ conceptual understanding and technical skills.
Instructors in the disciplines of hydrology and water resources are entrusted with preparing their students to become effective engineers in a relatively short time. We recommend that instructors consider augmenting traditional lectures with modules that use authentic high-cognitive demand tasks to develop students’ conceptual knowledge and specialized technical skills, such as those hosted on the HydroLearn platform. Exposing students to authentic, high cognitive demand tasks can help them connect mathematical theories or classroom lectures to complex, real-world problems, applications, or procedures and gain a deeper understanding of fundamental topics in the field and develop the expertise needed to solve complex engineering problems.
While this study cannot directly attribute the observed gains in conceptual understanding and technical abilities to the usage of the specific module that the students completed, the positive trends that emerged from this study provide some important insights into how students’ self-reported conceptual knowledge and technical skills grow following the use of an online learning module based on an authentic, high cognitive demand tasks. Moreover, this study cannot claim impact or effect based on the data collected because we did not use randomized control trials. Without randomized control trials, this study cannot make any causal claims as it is possible that participation in the courses, rather than the use of the HydroLearn modules, improved students’ conceptual understanding and technical skills. Also, we cannot rule out the possibility that external factors influenced the students’ self-reported results. It is possible that the students would have picked up on these concepts and skills anyway, and they simply happened to develop them between the pre and post-surveys. Finally, the exclusive use of self-report data can raise some concerns; however, this type of data is still widely used because it can be a convenient measure with some validity (Felder, 1995; Besterfield-Sacre et al., 2000; Terenzini et al., 2001). Future research should compare a control group of students who did not participate in the modules to a group who did. Further investigation could also include performing different analyses, such as multilevel modeling, examining the impact of the modules on learning by controlling for other factors (e.g., grade point average, demographics, or motivation for learning) to try to parse out the effects of using the module on students’ learning.
The datasets presented in this article are not readily available. Per the institutional IRB, the dataset collected cannot be shared or hosted on any server that is not owned by the university. Inquiries can be directed to EH, ZW1hZC5oYWJpYkBsb3Vpc2lhbmEuZWR1.
The studies involving human participants were reviewed and approved by the University of Louisiana at Lafayette Institutional Review Board. The patients/participants provided their written informed consent to participate in this study.
JB collected the data, performed the analysis, and contributed to the writing of the manuscript. MG conceived and designed the analysis and contributed to the writing and editing of the manuscript. EH conceived and designed the overall approach and contributed to the writing and editing of the manuscript. All authors contributed to the article and approved the submitted version.
This study was supported by the National Science Foundation (NSF) IUSE Award #1726965. The HydroLearn project was supported by the National Science Foundation under collaborative awards 1726965, 1725989, and 1726667.
We acknowledge and sincerely appreciate the efforts of all HydroLearn fellows who have contributed modules to the HydroLearn platform. We would also like to thank all Fellows and faculty who have collected and shared their student data. Lastly, a very special thank you goes to the students who participated in the study.
The authors declare that the research was conducted in the absence of any commercial or financial relationships that could be construed as a potential conflict of interest.
All claims expressed in this article are solely those of the authors and do not necessarily represent those of their affiliated organizations, or those of the publisher, the editors and the reviewers. Any product that may be evaluated in this article, or claim that may be made by its manufacturer, is not guaranteed or endorsed by the publisher.
Any opinions, findings, conclusions, or recommendations expressed in this material are those of the authors and do not necessarily reflect the views of the NSF.
The Supplementary Material for this article can be found online at: https://www.frontiersin.org/articles/10.3389/feduc.2022.953164/full#supplementary-material
Aghakouchak, A., and Habib, E. (2010). Application of a conceptual hydrologic model in teaching hydrologic processes. Int. J. Eng. Educ. 26, 963–973.
Anderson, L. W., and Krathwohl, D. R. (2001). A taxonomy for learning, teaching, and assessing: A Revision of Bloom’s Taxonomy of Educational Objectives. Harlow: Longman.
Bader, N. E., Soule, D., and Castendyk, C. (2016). Students, meet data: Using publicly available, high-frequency sensor data in the classroom. EOS. 97. Available online at: https://eos.org/science-updates/students-meet-data
Benjamini, Y., and Hochburg, Y. (1995). Controlling the false discovery rate: a practical and powerful approach to multiple testing. J. R. Statistical Soc. Ser. B 57, 289–300. doi: 10.1111/j.2517-6161.1995.tb02031.x
Besterfield-Sacre, M., Shuman, L. J., Wolfe, H., Atman, C. J., McGourty, J., Miller, R. L., et al. (2000). Defining the outcomes: a framework for EC-2000. IEEE Trans. Educ. 43, 100–110. doi: 10.1109/13.848060
Boaler, J. O., and Staples, M. (2008). Creating mathematical futures through an equitable teaching approach: the case of railside school. Teach. Coll. Rec. 110, 608–645. doi: 10.1177/016146810811000302
Borrego, M., Froyd, J. E., and Hall, T. S. (2010). Diffusion of engineering education innovations: a survey of awareness and adoption rates in US engineering departments. J. Eng. Educ. 99, 185–207. doi: 10.1002/j.2168-9830.2010.tb01056.x
Boston, M. D., and Smith, M. S. (2011). A ‘task-centric approach’ to professional development: enhancing and sustaining mathematics teachers’ ability to implement cognitively challenging mathematical tasks. ZDM Mathematics Educ. 43, 965–977. doi: 10.1007/s11858-011-0353-2
Bourget, P. G. (2006). Integrated water resources management curriculum in the united states: results of a recent survey. J. Contemporary Water Res. Educ. 135, 107–114. doi: 10.1111/j.1936-704X.2006.mp135001013.x
Bourrie, D. M., Cegielski, C. G., Jones-Farmer, L. A., and Sankar, C. S. (2014). Identifying characteristics of dissemination success using an expert panel. Decision Sci. J. Innov. Educ. 12, 357–380. doi: 10.1111/dsji.12049
Bransford, J. D., Ann, L., Brown, A., and Cocking, R. R. (2004). How People Learn Brain, Mind, Experience, and School Committee. Washington, DC: National Academy Press.
Brown, J. S., Collins, A., and Duguid, P. (2005). Situated cognition and the culture of learning. Subj. Learn. Prim. Curric. Issues English Sci. Math. 18, 288–305. doi: 10.4324/9780203990247
Cohen, J. (1988). Statistical Power Analysis for the Behavioral Sciences, 2nd Edn. Hillsdale, NJ: Lawrence Erlbaum Associates.
CollegeData. (n.d.). CollegeData College Sizes: Small, Medium, or Large. Natl. Assoc. Coll. Admiss. Couns. Available online at: https://www.collegedata.com/resources/the-facts-on-fit/college-size-small-medium-or-large (accessed May 22, 2021).
Cresswell, J. W., and Guetterman, T. C. (2019). Educational Research: Planning, Conducting, and Evaluating Quantitative and Qualitative Research, 6th Edn. Saddle River, NJ: Pearson.
CUAHSI (2010). Water in a Dynamic Planet: A Five-year Strategic Plan for Water Science. Washington DC: CUAHSI.
Dori, Y. J., Ral, R. T., and Tsaushu, M. (2003). Teaching biotechnology through case studies—can we improve higher order thinking skills of nonscience majors? Sci. Educ. 87, 767–793. doi: 10.1002/sce.10081
Endreny, T. A. (2007). Simulation of soil water infiltration with integration, differentiation, numerical methods and programming exercises. Int. J. Eng. Educ. 23, 608–617.
Entwistle, N. J., and Peterson, E. R. (2004). Conceptions of learning and knowledge in higher education: relationships with study behavior and influences of learning environments. Int. J. Educ. Res. 41, 407–428. doi: 10.1016/j.ijer.2005.08.009
Felder, R. M. (1995). A longitudinal study of engineering student performance and retention. IV. instructional methods. J. Eng. Educ. 84, 361–367. doi: 10.1002/j.2168-9830.1995.tb00191.x
Field, A. (2018). Discovering Statistics Using IBM SPSS Statistics, 5th Edn. London: Sage Publications Ltd.
Gallagher, M. A., Habib, E. H., Williams, D., Lane, B., Byrd, J. L., and Tarboton, D. (2022). Sharing experiences in designing professional learning to support hydrology and water resources instructors to create high-quality curricular materials. Front. Educ. 7:890379. doi: 10.3389/feduc.2022.890379
Gallagher, M. A., Byrd, J., Habib, E., Tarboton, D., and Willson, C. S. (2021). “HydroLearn: improving students’ conceptual understanding and technical skills in a civil engineering senior design course,” in Proceedings of the 2021 ASEE Virtual Annual Conference Content Access, Virtual Conference.
Habib, E., and Deshotel, M. (2018). Towards broader adoption of educational innovations in undergraduate water resources engineering: views from academia and industry. J. Contemp. Water Res. Educ. 164, 41–54. doi: 10.1111/j.1936-704X.2018.03283.x
Habib, E., Deshotel, M., Lai, G., and Miller, R. (2019a). Student perceptions of an active learning module to enhance data and modeling skills in undergraduate water resources engineering education. Int. J. Eng. Educ. 35, 1353–1365.
Habib, E., Tarboton, D., Gallagher, M., Williams, D., Lane, B., Ames, D., et al. (2022). HydroLearn. Available online at: https://www.hydrolearn.org/ (accessed July 12, 2022).
Habib, E. H., Gallagher, M., Tarboton, D. G., Ames, D. P., and Byrd, J. (2019b). “HydroLearn: An online platform for collaborative development and sharing of active-learning resources in hydrology education,” in Proceedings of the AGU Fall Meeting Abstracts, Vol. 2019, 13D–0898D.
Hattie, J. (2009). Visible Learning: a Synthesis of Meta-analysis Relating to Achievement. Milton Park: Routledge.
Hendricks, R. W., and Pappas, E. C. (1996). Advanced engineering communication: an integrated writing and communication program for materials engineers. J. Eng. Educ. 85, 343–352. doi: 10.1002/j.2168-9830.1996.tb00255.x
Herman, J. L., and Klein, D. C. D. (1996). Evaluating equity in alternative assessment: an illustration of opportunity-to-learn issues. J. Educ. Res. 89, 246–256. doi: 10.1080/00220671.1996.9941209
Herrington, J. A., Oliver, R. G., and Reeves, T. (2003). Patterns of engagement in authentic online learning environments. Australia’s. J. Educ. Technol. 19, 59–71. doi: 10.14742/ajet.1701
Hiebert, J., and Wearne, D. (1993). Instructional tasks, classroom discourse, and students’ learning in second-grade arithmetic. Am. Educ. Res. J. 30, 393–425. doi: 10.3102/00028312030002393
Howe, C. (2008). A creative critique of U.S. water education. J. Contemporary Water Res. Educ. 139, 1–2. doi: 10.1111/j.1936-704X.2008.00009.x
Hugerat, M., and Kortam, N. (2014). Improving higher order thinking skills among freshmen by teaching science through inquiry. Eurasia J. Math. Sci. Technol. Educ. 10, 447–454. doi: 10.12973/eurasia.2014.1107a
HydroFrame-Education (n.d.). (HydroFrame). Available online at: https://hydroframe.org/groundwater-education-tools/ (accessed July 19, 2022).
Kardos, G., and Smith, C. O. (1979). “On writing engineering cases,” in Proceedings of ASEE National Conference on Engineering Case Studies, Vol. 1, 1–6.
Kolodner, J. L. (2006). “Case-based reasoning,” in Cambridge Handbook of the Learning Sciences, ed. K. Sawyer (New York, NY: Cambridge University Press), 225–242. doi: 10.1017/CBO9780511816833.015
Kraft, M. (2020). Interpreting effect sizes of education interventions. Educ. Res. 49, 241–153. doi: 10.3102/0013189X20912798
Kulonda, D. J. (2001). Case learning methodology in operations engineering. J. Eng. Educ. 90, 299–303. doi: 10.1002/j.2168-9830.2001.tb00608.x
Lane, B., Garousi-Nejad, I., Gallagher, M. A., Tarboton, D. G., and Habib, E. (2021). An open web-based module developed to advance data-driven hydrologic process learning. Hydrol. Process. 35, 1–15. doi: 10.1002/hyp.14273
Ledley, T., Prakash, S., Manduca, C., and Fox, S. (2015). Recommendations for making geoscience data accessible and usable in education. Earth Sp. Sci. News 89, 1139–1157.
Loheide, S. P. II (2020). Collaborative graduate student training in a virtual world, EOS, 101. Available online at: https://par.nsf.gov/servlets/purl/10286981
Maggioni, V., Girotto, M., Habib, E., and Gallagher, M. A. (2020). Building an online learning module for satellite remote sensing applications in hydrologic science. Remote Sens. 12, 1–16. doi: 10.3390/rs12183009
Mayo, J. A. (2002). Case-based instruction: a technique for increasing conceptual application in introductory psychology. J. Constr. Psychol. 15, 65–74. doi: 10.1080/107205302753305728
Mayo, J. A. (2004). Using case-based instruction to bridge the gap between theory and practice in psychology of adjustment. J. Constr. Psychol. 17, 137–146. doi: 10.1080/10720530490273917
Merwade, V., and Ruddell, B. L. (2012). Moving university hydrology education forward with community-based geoinformatics, data and modeling resources. Hydrol. Earth Syst. Sci. 16, 2393–2404. doi: 10.5194/hess-16-2393-2012
Miri, B., David, B. C., and Uri, Z. (2007). Purposely teaching for the promotion of higher-order thinking skills: a case of critical thinking. Res. Sci. Educ. 37, 353–369. doi: 10.1007/s11165-006-9029-2
Nash, S., McCabe, B., Goggins, J., and Healy, M. G. (2012). “The use of digital resources in civil engineering education: Enhancing student learning and achieving accreditation criteria,” in Proceedings of the National Digital Learning Revolution (NDLR) Research Symposium, Limerick.
Pimmel, R. (2003). Student learning of criterion 3 (a)-(k) outcomes with short instructional modules and the relationship to bloom’s taxonomy. J. Eng. Educ. 92, 351–359. doi: 10.1002/j.2168-9830.2003.tb00780.x
Pimmel, R., Leland, R., and Stern, H. (2002). “Changes in student confidence resulting from instruction with modules on EC 2000 Skills,” in Proceedings of the 2002 ASEE peer Annual Conference, Montreal, QC.
Prince, M., and Felder, R. (2007). The many faces of inductive teaching and learning. J. Coll. Sci. Teach. 36, 14–20.
Rajib, M. A., Merwade, V., Luk Kim, I., Zhao, L., Song, C. X., and Zhe, S. (2016). A web platform for collaborative research, and education through online sharing, simulation, and visualization of SWAT models. Environ. Model. Softw. 75, 498–512. doi: 10.1016/j.envsoft.2015.10.032
Rosenthal, R., and Rubin, D. B. (2003). r equivalent: a simple effect size indicator. Psychol. Methods 8, 492–496. doi: 10.1037/1082-989X.8.4.492
Ruddell, B. L., and Wagener, T. (2015). Grand challenges for hydrology education in the 21 st Century. J. Hydrol. Eng. 20, 1–24. doi: 10.1061/(ASCE)HE.1943-5584.0000956
Seymour, E., Wiese, D., Hunter, A., and Daffinrud, S. M. (2000). “Creating a better mousetrap: On-line student assessment of their learning gains,” in Proceedings of the national meeting of the American Chemical Society (Amsterdam: Pergamon), 1–40.
Sheppard, S., Colby, A., Macatangay, K., and Sullivan, W. (2006). What is engineering practice? Int. J. Eng. Educ. 22, 429–438. doi: 10.4324/9781315276502-3
Soriæ, B. (1989). Statistical “discoveries” and effect-size estimation. J. Am. Stat. Assoc. 84, 608–610. doi: 10.1080/01621459.1989.10478811
Stein, M. K., Grover, B. W., and Henningsen, M. (1996). Building capacity for mathematical thinking and reasoning: an analysis of mathematical tasks used in reform classrooms. Amer. Edu. Res. J. 33, 455–488. doi: 10.3102/00028312033002455
Stein, M. K., and Lane, S. (1996). Instructional tasks and the development of student capacity to think and reason: an analysis of the relationship between teaching and learning in a reform mathematics project. Educ. Res. Eval. 2, 50–80. doi: 10.1080/1380361960020103
Tekkumru-Kisa, M., Stein, M. K., and Doyle, W. (2020). Theory and research on tasks revisited: task as a context for students’ thinking in the era of ambitious reforms in mathematics and science. Edu. Res. 49, 606–617. doi: 10.3102/0013189X20932480
Tekkumru-Kisa, M., Stein, M. K., and Schunn, C. (2015). A framework for analyzing cognitive demand and content-practices integration: task analysis guide in science. Wiley Period. Inc. J Res Sci Teach. 52, 659–685. doi: 10.1002/tea.21208
Terenzini, P. T., Cabrera, A. F., Colbeck, C. L., Parente, J. M., and Bjorklund, S. A. (2001). “Collaborative Learning vs. Lecture/Discussion: students’ reported learning gains. J. Eng. Educ. 90, 123–130. doi: 10.1002/j.2168-9830.2001.tb00579.x
The Center for Reimagining Learning Inc (2022). About The Open edX Project – Open edX. Available online at: http://openedx.org/about-open-edx/ (accessed September 12, 2022).
Wagener, T., Sivapalan, M., Troch, P. A., McGlynn, B. L., Harman, C. J., Gupta, H. V., et al. (2010). The future of hydrology: An evolving science for a changing world. Water Resour. Res. 46:W05301. doi: 10.1029/2009WR008906
Ward, A., Herzog, S., Bales, J., Barnes, R., Ross, M., Jefferson, A., et al. (2021). Educational resources for hydrology & water resources. Hydroshare. Available online at: https://www.hydroshare.org/resource/148b1ce4e308427ebf58379d48a17b91/
Warner, R. M. (2012). Applied Statistics: From Bivariate Through Multivariate Techniques. Thousand Oaks, CA: Sage Publications.
Wilcoxon, F. (1945). Individual comparisons by ranking methods. Biometrics Bull. 1, 80–83. doi: 10.1093/jee/39.2.269
Woods, D. R., Felder, R. M., Rugarcia, A., and Stice, J. E. (2000). The future of engineering education: Part 3. Developing critical skills. Chem. Eng. Educ. 34, 108–117.
Yadav, A., Vinh, M., Shaver, G. M., Meckl, P., and Firebaugh, S. (2014). Case-based instruction: improving students’ conceptual understanding through cases in a mechanical engineering course. J. Res. Sci. Teach. 51, 659–677. doi: 10.1002/tea.21149
Keywords: authentic tasks, high-cognitive demand tasks, online learning, conceptual understanding, technical skills
Citation: Byrd J, Gallagher MA and Habib E (2022) Assessments of students’ gains in conceptual understanding and technical skills after using authentic, online learning modules on hydrology and water resources. Front. Educ. 7:953164. doi: 10.3389/feduc.2022.953164
Received: 25 May 2022; Accepted: 06 September 2022;
Published: 06 October 2022.
Edited by:
Bridget Mulvey, Kent State University, United StatesReviewed by:
Amber Jones, Utah State University, United StatesCopyright © 2022 Byrd, Gallagher and Habib. This is an open-access article distributed under the terms of the Creative Commons Attribution License (CC BY). The use, distribution or reproduction in other forums is permitted, provided the original author(s) and the copyright owner(s) are credited and that the original publication in this journal is cited, in accordance with accepted academic practice. No use, distribution or reproduction is permitted which does not comply with these terms.
*Correspondence: Emad Habib, ZW1hZC5oYWJpYkBsb3Vpc2lhbmEuZWR1
Disclaimer: All claims expressed in this article are solely those of the authors and do not necessarily represent those of their affiliated organizations, or those of the publisher, the editors and the reviewers. Any product that may be evaluated in this article or claim that may be made by its manufacturer is not guaranteed or endorsed by the publisher.
Research integrity at Frontiers
Learn more about the work of our research integrity team to safeguard the quality of each article we publish.