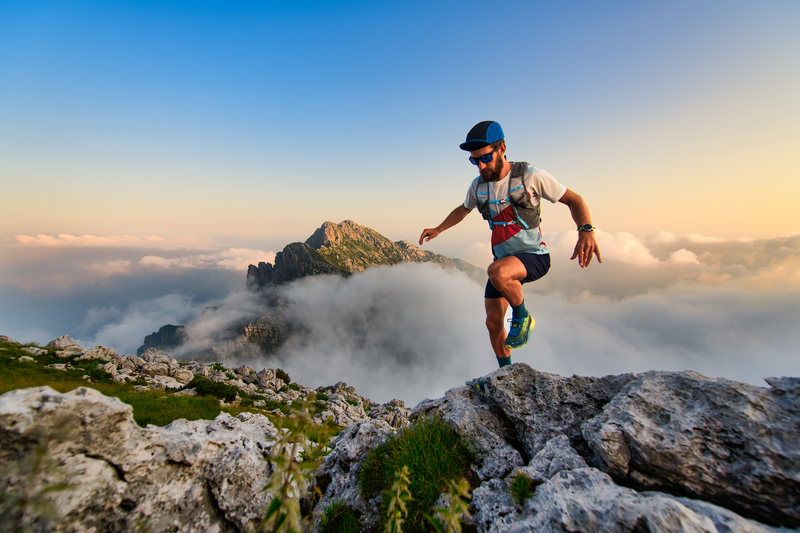
95% of researchers rate our articles as excellent or good
Learn more about the work of our research integrity team to safeguard the quality of each article we publish.
Find out more
ORIGINAL RESEARCH article
Front. Educ. , 20 October 2022
Sec. STEM Education
Volume 7 - 2022 | https://doi.org/10.3389/feduc.2022.942224
This article is part of the Research Topic Eye Tracking for STEM Education Research: New Perspectives View all 12 articles
Although eye tracking has been successfully used in science education research, exploiting its potential in collaborative knowledge construction has remained sporadic. This article presents a novel approach for studying collaborative knowledge construction in a simulation-based environment by combining both the spatial and temporal dimensions of eye-tracking data with video data. For this purpose, we have investigated two undergraduate physics student pairs solving an electrostatics problem in a simulation-based environment via Zoom. The analysis of the video data of the students’ conversations focused on the different collaborative knowledge construction levels (new idea, explication, evaluation, and non-content-related talk and silent moments), along with the temporal visualizations of the collaborative knowledge construction processes. The eye-tracking data of the students’ gaze, as analyzed by epistemic network analysis, focused on the pairs’ spatial and temporal gaze behavior. We illustrate how gaze behavior can shed light on collaborative knowledge construction in terms of the quantity of the talk (e.g., gaze behavior can shed light on the different activities of the pairs during the silent moments), quality of the talk (e.g., gaze behavior can shed light on the different approaches when constructing knowledge on physical phenomena), and temporality of collaborative knowledge construction processes [e.g., gaze behavior can shed light on (the lack of) attempts to acquire the supporting or contrasting evidence on the initial ideas on the physical phenomena]. We also discuss the possibilities and limitations of gaze behavior to reveal the critical moments in the collaborative knowledge construction processes.
In science education, simulation-based environments have been used to foster collaborative knowledge construction (CKC) and guide students in building on each other’s ideas and thoughts while learning about scientific phenomena (Schellens and Valcke, 2005; Liu et al., 2021). However, productive CKC processes in these environments rarely occur automatically (Jeong et al., 2019). Even though the (automatic) analysis of students’ verbal conversations could provide information to teachers and machines so that they can guide CKC processes (Lämsä et al., 2021b), many nontrivial issues, such as moving from the retrospective modeling of learning processes to predictive analytics, must be solved before these applications can be more broadly adopted (Schneider et al., 2021). In the field of multimodal learning analytics, various data modalities are combined to comprehensively understand if and how learning occurs (Olsen et al., 2020). Ultimately, the aim is to use this information to support learning.
Collaborative knowledge construction analysis has typically focused on the quantity and quality of conversations via coding the utterances of video data and evaluation of learning outcomes (Jeong et al., 2014, 2019); in this context, the temporal analysis of CKC has gained increased attention (Lämsä et al., 2021a). In addition to the conversations captured with video data, CKC research could benefit from eye tracking (Olsen et al., 2020). Although eye tracking has been successfully used in science education research (e.g., Hahn and Klein, 2022), only a few studies have investigated the role of gaze in CKC in science learning (for an exception, see Becker et al., 2021). Gaze similarity among students has been associated with higher-quality learning processes and outcomes (Olsen et al., 2020; Becker et al., 2021), although exceptions do exist (Liu et al., 2021). On the one hand, it is essential to develop improved gaze similarity indicators that reveal both the focus and timing of gaze and, thus, better reflect the kinematics of CKC in simulation-based environments. On the other hand, the contextual information of CKC processes (such as video data) and eye-tracking data would ensure that the processes are interpreted reliably (Molenaar, 2021).
In the current article, we introduce a methodology of combined video and eye-tracking data analysis to study CKC kinematics in a simulation-based environment. We discuss the possibilities of this methodology for designing pedagogical practices for science education that can help us in understanding and guiding these CKC processes.
Simulations work to model activities and processes by omitting irrelevant variables from the perspective of learning goals (Chernikova et al., 2020). Moreover, simulations provide users with certain control when they accomplish a given task (Chernikova et al., 2020). Simulations have been applied in practicing authentic practices and procedures, for example, in the aviation (Mavin et al., 2018) and healthcare sectors (Cook et al., 2013), and in various disciplines as a part of formal education (Chernikova et al., 2020). Simulations can be used in nondigital settings (e.g., simple patient simulations in healthcare) or digital settings (e.g., virtual reality flight simulations in aviation). In the current study, we focus on computer simulations in science education. In the context of science education, computer simulations are programs that provide a representation of a scientific phenomenon through a model (Clark et al., 2009; de Jong and Lazonder, 2014). Computer simulations such as PhET (University of Colorado Boulder, 2022b) or WISE (UC Berkeley, 2022) may improve learning outcomes by enhancing other forms of instruction, such as lectures or laboratories (Rutten et al., 2012; de Jong et al., 2013). Moreover, simulations may facilitate collaboration among students during CKC processes (Lämsä et al., 2018, 2020). This collaboration among students may be beneficial for gaining conceptual and procedural knowledge (Jensen and Lawson, 2011; Rutten et al., 2012).
In simulation-based environments, the user can interact with the simulation by exploring the effects of the given input variables to observe the effects on the output variables (Clark et al., 2009; de Jong and Lazonder, 2014). Within computer-mediated settings, the interaction between the students and simulation may take different forms (Figure 1). First, when using individual-based simulations, each student can individually interact with the simulation, requiring intensive verbal coordination of CKC processes between students (Figure 1A), which can be a challenge (Chang et al., 2017). Second, when using collaborative simulations, the students can interact with the simulation in a shared space collaboratively; hence, the coordination of CKC processes may be further fostered by assigning students distinct responsibilities (Figure 1B). Even though these latter simulation-based environments may benefit from the coordination of the CKC processes and, thus, facilitate interactions among students, they do not necessarily lead to higher-level CKC processes or learning outcomes compared with the former settings (Chang et al., 2017; Liu et al., 2021). Third, the rapid adoption of communication apps, especially during the COVID-19 lockdown, shifted face-to-face sessions to Zoom or Teams. Sessions with screen sharing can also foster the coordination of the CKC process, even if individual-based simulations are used (Stevenson et al., 2022). Although one student sharing the screen interacts with the simulation and others monitor the simulation view from the screen, the sharing student can mediate the interaction between the simulation and others by implementing requests from others in the simulation environment (Figure 1C). In the current study, we focus on this third scenario.
Figure 1. The interactions (double-sided arrows) among the students and simulation with the (A) individual-based simulations, (B) collaborative simulations, and (C) individual-based simulations used with the screen-sharing functionality in computer-mediated collaborative knowledge construction. The current study focuses on the faded scenario (C), in which student 1 is sharing and student 2 is monitoring the screen.
Although this scenario inevitably assigns different roles to the students in the CKC processes, both sharing and monitoring students should effectively utilize a simulation-based environment as an external resource for explicating and evaluating ideas and thoughts (Jeong and Hmelo-Silver, 2010). Usually, students share their ideas and thoughts without building on previous ones, meaning that critical explication and evaluation of others’ ideas and thoughts and other higher levels of CKC are rare (Yang et al., 2018). Students may also have challenges understanding visual representations of abstract concepts, such as fields (Klein et al., 2018). These challenges highlight the role of the teacher and simulation-based environment in guiding CKC (Lin et al., 2013; Lehtinen and Viiri, 2017). In this respect, the eye-tracking analysis provides a view of students’ visual attention that could provide teachers and simulation developers with information on unnecessary or distracting visual objects, helping guide CKC and improve these environments.
In the current paper, we refer to gaze as “the act of directing the eyes toward a location in the visual world” (Hessels, 2020, p. 856) and gaze behavior as gaze similarities and dissimilarities over time. Tatler et al. (2014, p. 6) have pointed out that “eye movements give us a window onto how perception operates across the course of a task, from the first intention to act and through the process of carrying out the task itself.” Strohmaier et al. (2020) showed that many studies using eye tracking to study learning processes assume that when a student’s gaze is focused on an artifact, the student processes the information being provided (see Just and Carpenter, 1980). This assumption, however, is a simplification because, even though the sharp image of the artifact is formed within a tiny area of the eye, which is called the fovea (Holmqvist et al., 2011, p. 21), humans can process information from the wider area around the artifact (parafoveal processing, Schotter et al., 2012).
One of the critical questions in CKC is how to capture a joint activity between pairs or small groups using eye tracking (e.g., Hayashi and Shimojo, 2021). So far, most studies have evaluated CKC processes by assessing how often students look at the same objects of the learning environment (Olsen et al., 2020; Becker et al., 2021; Sharma et al., 2021). For example, Becker et al. (2021) found that early gaze similarities concerning laboratory apparatus were positively associated with the learning outcomes in a collaborative laboratory. However, similar gaze patterns do not guarantee productive learning processes and outcomes (Schneider et al., 2018). For example, high gaze similarity may result in low-level CKC processes and poor outcomes if the similarity is related to irrelevant objects (a synthesis by Hahn and Klein, 2022, indicated this to be true when learning individually in simulation-based environments). Schneider et al. (2018) addressed this challenge in the literature by augmenting spatial information from eye-tracking data and verbal information from audio recordings into cross-recurrence graphs that indicate “how and the extent to which streams of information come to exhibit similar patterns in time” (Coco and Dale, 2014, p. 2). Simulations often visualize concepts that are abstract, nonlocal, and visually absent in the real world, such as fields and forces. Thus, rich visualizations result in several visual objects, which can complicate the interpretation and comparison of cross-recurrence graphs.
The analysis of students’ gaze behavior means identifying the temporal co-occurrences of their gaze events (see an overview of the temporal analysis methods in Lämsä et al., 2021a). For this purpose, an emerging method in the learning sciences is epistemic network analysis (ENA; Shaffer et al., 2016). The premise of ENA is that co-occurrences of (gaze) events are more important than the events as such (Shaffer et al., 2016; Andrist et al., 2018). ENA models the co-occurrences of the gaze events with nodes and edges: the areas of interest (AOIs) are depicted as nodes, and the co-occurrences of the students’ gaze events with these AOIs are depicted as the edges between nodes. An advantage of the ENA compared with other network analysis methods is that it allows for examining which (instead of how) nodes are connected (Bowman et al., 2021); from the perspective of CKC processes, this is important to understand which features of the simulation-based environment students are simultaneously looking at. Moreover, the ENA allows for comparisons of the networks by keeping the nodes and edges in the same location in the visualization of the networks (Bowman et al., 2021); this facilitates a comparison of the students’ gaze behaviors between the pairs or small groups and between the CKC levels.
In the current study, we introduce a novel approach for exploring CKC kinematics in a simulation-based environment. By kinematics, we refer to the connections between the CKC processes and gaze behavior without considering their dynamics, which would imply understanding the causes of the observed CKC processes or gaze behavior. To illustrate our approach, we use video data of student pairs’ conversations to understand their CKC processes from the perspectives of the (i) quantity of the talk, (ii) quality of the talk, and (iii) temporality. We then apply ENA to eye-tracking data to explore what insights the student pairs’ gaze behavior provides regarding these CKC processes. We answer the following research questions (RQs):
RQ1: What does the analysis of the video data tell about the pairs’ CKC processes?
RQ2: What does the pairs’ gaze behavior tell about these CKC processes?
The current study was conducted in an introductory electricity course at a Finnish university. We focus on the data from two student pairs who used the Charges and Fields PhET simulation (University of Colorado Boulder, 2022a) to solve an electricity problem (Figure 2). The students worked in different rooms via Zoom so that both saw the same assignment and simulation views of the split screen. One student shared (S) and the other monitored (M) the screen; in the rest of this paper, we refer to these students as 1S and 1M (the sharing student and monitoring student of pair 1, respectively), and 2S and 2M (the sharing student and monitoring student of pair 2, respectively). The pairs constructed knowledge of electric field properties in the presence of a static negative charge and positive charge that could be moved about. The students were supposed to apply the superposition principle to explain how the direction and magnitude of the nonlocal electric field change when moving the positive charge.
Figure 2. The assignment and simulation view the student pairs were looking at when they constructed knowledge on electric field properties in the presence of a negative static charge and positive movable charge. The areas of interest are labeled using colored shapes; the labels were not visible to the students. The students wrote their answers to the problem in the textbox on the left.
To answer RQ1, we video recorded the pairs’ conversations in Zoom and transcribed the pairs’ conversations (pair 1: 5.3 min. with 59 utterances; pair 2: 12.8 min. with 163 utterances) using the “unit of meaning” (Henri, 1992, p. 134) to identify episodes comprising a few utterances. We then applied Veerman and Veldhuis-Diermanse (2001) to analyze the CKC level through theory-driven content analysis. We coded the episodes (13 and 38 episodes) as either physics content–related talk, including the following CKC levels: (i) new idea, (ii) explication (elaboration on earlier ideas), and (iii) evaluation (critical discussion of and reasoning about earlier ideas), or non-content-related talk, including planning and technical talk (e.g., planning procedures or wondering how to invoke the simulation). The first author prepared a coding manual with the definitions and example excerpts of the codes. After this, the first author and the coauthor coded all the episodes of the pairs’ conversations, after which the disagreements (see the contingency table in Table 1) were resolved and definitions of the codes revised by all the authors (see Table 2).
Table 1. The contingency table shows the agreements and disagreements in the coding of the conversations between two coders.
Table 2. Coding manual for non-content-related talk and physics content–related talk that includes a code for each level of collaborative knowledge construction.
To answer RQ2, we collected the eye-tracking data using Tobii Pro Glasses 2 (sampling frequency 50 Hz), which are mobile wearable eye trackers. Eye tracking allowed free movement of the participants so that the gaze outside the computer screen could also be captured. The scene camera of the eye tracker had a resolution of 1,920 × 1,080 pixels, capturing 52° vertically and 82° horizontally. We used one-point calibration, and we verified the calibration by asking the participants to look at three different points on their surroundings (the points were left, right, and in front of them). We wanted to keep the data collection situation as authentic a learning situation as possible, and we did not use chinrests, control students’ distance to the computer screen, nor control the gaze angles; however, the learning situation and simulation-based environment (Figure 2) provided satisfactory conditions for eye-tracking data collection (e.g., distance to the computer screen was approximately 0.5–1.0 m, and the targets in the environment were located within narrow area so that no large gaze angles were needed that improved the accuracy of the eye tracking; Tobii Pro AB, 2017). The data were analyzed in Tobii Pro Lab (Tobii Pro AB, 2022). We used the Tobii I-VT (Attention) as a gaze filter, which is the default preset for wearable eye trackers. The velocity threshold parameter was 100°/second. Blinks and saccades were cleaned from the data, and only fixation data were used in the coding and further analyses.
To study the gaze behavior, we first watched eye-tracking recordings to explore where students divided their visual attention when solving the given problem. Based on this exploration and the expert analysis of the problem itself (five authors have master’s or doctoral degrees from physics), we divided the screen view into AOIs (see Figure 2 and Table 3; the keyboard was an AOI only for the sharing student). The formed AOIs allowed “local analysis” (Hahn and Klein, 2022, p. 5), which differentiates the irrelevant and relevant features of the simulation view (Figure 2 and Table 3). The fixation data were manually coded in Tobii Pro Lab into the different AOIs based on the screen capture in Figure 2. The coding was done fixation by fixation, clicking that AOI in the screen capture to which the student’s gaze was located in the eye-tracking recording. The coding decisions were made based on the set of objects in the screen capture (e.g., sensor, moving charge, and static charge), not on the absolute position of the fixation in the eye-tracking recording (e.g., if the student’s visual attention was on the moving charge, it was coded as such, even though the position of the moving charge in the eye-tracking recording would have differed from that presented in Figure 2). Fixations unrelated to any AOIs were coded as being “outside screen” and excluded from further analysis. Two researchers coded the fixation data of one student (562 fixations, of which 160 were “outside screen”). To check the interrater reliability of the coding, we then calculated Cohen’s kappa (Cohen, 1960) and Shaffer’s rho separately for each code (AOI; Table 3) so that a high agreement in one code did not hide a low agreement in another code (Shaffer, 2017; Eagan et al., 2020). Cohen’s kappa was >0.97 for all the codes (AOIs), indicating almost perfect agreement between the two coders (Shaffer’s rho was <0.05 for all the codes when we set 0.7 as a threshold value of Cohen’s kappa to indicate good reliability; Cicchetti, 1994).
Table 3. The areas of interest (AOIs) and their total fixation durations in percentages during the collaborative knowledge construction processes of pair 1 (1S and 1M, duration 5.3 min) and pair 2 (2S and 2M, duration 12.8 min).
Because the sampling frequency of the eye trackers was 50 Hz (a data point for each 20-ms interval), as a result, we had a time series of the gaze events in which all AOIs were assigned binary data for each 20-ms interval, here corresponding either to student visual attention (one) or the absence of student visual attention (zero). We excluded five AOIs (settings, objects, measuring tape, meters, and reset) because they rarely attracted students’ attention (Table 3); this exclusion also eased the interpretation of the epistemic networks by decreasing the number of nodes in the networks. For both student pairs, synchronization of the video and eye-tracking data enabled analysis of the CKC processes from the perspectives of the (i) quantity of the talk, (ii) quality of the talk, and (iii) temporality and gaze behavior.
To answer RQ1 and to study the quantity of the talk, we first calculated the relative amount of time that the pairs used for non-content-related talk and physics content-related talk, including the following CKC levels: (i) new idea, (ii) explication, and (iii) evaluation. We also calculated the relative amount of time for silent moments. Second, to study the quality of the talk, we examined the quality of the conversations at the different CKC levels in terms of whether students’ ideas (and explication and evaluation of those ideas) were correct or not in the context of the given problem (Figure 2). Third, we studied the temporality of the pairs’ CKC processes by visualizing CKC level and non-content-related talk as a function of time.
To answer RQ2, we applied ENA to synchronized, binary eye-tracking data (see Shaffer et al., 2016; Andrist et al., 2018). The AOIs served as the nodes of the network (Figure 2). We considered that the gaze events within a 2-s time interval were connected, so we used the moving windows of the size of a 100 rows (100 rows × 20 ms/row = 2 s). We chose this 2-s time interval based on previous studies on the gaze similarity of pairs (Richardson and Dale, 2005; Schneider et al., 2018). The unit of analysis was a pair at different CKC levels (along with during non-content-related talk and during silent moments), so we created the adjacency matrices for both pairs at each CKC level separately. The adjacency matrices represent the strength of the connections between the AOIs of the two students at the different CKC levels (along with during non-content-related conversations and during silent moments). We used weighted sums so that more connections between the AOIs within a moving window also resulted in stronger connections between these AOIs. When building epistemic networks, we did not visualize the connections between the AOIs of an individual student; in other words, if the student focused on several AOIs within the 2-s time interval, the connections between these AOIs were not visible in the epistemic networks (Andrist et al., 2018). We made this decision to facilitate the interpretation of the networks.
After the adjacency matrices for each unit of analysis had been created, the matrices were converted into adjacency vectors (Bowman et al., 2021) that were spherically normalized. This normalization eased the comparison of the networks when the duration of the CKC processes (and, thus, the number of gaze events) differed between pairs 1 and 2 (see Table 3). Finally, the dimensions of the adjacency vectors were reduced by singular value decomposition, after which the network nodes were positioned by applying an optimization method (see Bowman et al., 2021). The networks included two nodes for each AOI (see Table 3): one node for the sharing student and another for the monitoring student. The edges connecting the nodes provided a visualization of the gaze behavior: the thicker the edge, the more students had simultaneously focused on the corresponding AOIs within the two-second time interval. Figure 3 demonstrates this process with a fictional, simplified dataset. We performed the ENA in RStudio (Version 1.2.1335) by applying the rENA package (Marquart et al., 2021).
Figure 3. A fictional, simplified process for visualizing the epistemic network of two areas of interest (AOI1 and AOI2). S and M after the underscore refer to the gaze of the sharing student and monitoring student. (A) Weighted adjacency matrices represent the co-occurrences of gaze events in the two different time intervals (moving windows). (B) The cumulative adjacency matrix is calculated by summing the adjacency matrices presented in (A). The connections in the AOIs between the sharing and monitoring students are included in further analyses; the connections within an individual student are excluded (see the shadings in the matrices). (C) The arbitrary visualization of the epistemic network shows that the connections between AOI2_S and AOI1_M were stronger (a thicker edge between the nodes) than the connections between AOI1_S and AOI1_M (a thinner edge between the nodes). The sharing student focused their attention neither on the AOI1_S nor on the AOI2_S when the monitoring student was looking at AOI2_M.
In the following section, we cover the pairs’ CKC processes from the perspectives of the (i) quantity of the talk, (ii) quality of the talk, and (iii) temporality based on the analysis of the video data (RQ1, Section “Pairs’ collaborative knowledge construction processes based on the video data”). We then illustrate what insights the pairs’ gaze behavior provides regarding these CKC processes (RQ2, Section “Pairs’ collaborative knowledge construction processes: Insights based on gaze behavior”).
Figure 4 shows the relative amount of time that the pairs used for non-content-related talk and physics content–related talk, including the following CKC levels: (i) new idea, (ii) explication, and (iii) evaluation. The relative amount of time for silent moments is also shown in Figure 4. Pair 1 had more silent moments and less non-content-related talk, such as planning, than pair 2 (67% vs. 42 and 15% vs. 25%, respectively). Regarding the physics content–related talk, both pairs used a relatively similar amount of time to present new ideas (6 and 6%) and explicated those (13 and 17%), but pair 2 also evaluated the presented ideas 10% of the time.
Figure 4. The relative amount of talk (in %) at the different collaborative knowledge construction (CKC) levels. The amount of non-content-related talk and silent moments has also been marked.
Even though there were no differences between the pairs in the relative amount of physics content–related talk in presenting new ideas and explicating those (Figure 4), pair 1 exhibited low quality of physics content–related talk. The new ideas that the monitoring student (1M) presented to the problem did not include the magnitude of the electric field, instead focusing only on its direction. These ideas about the direction were also incorrect because 1M ignored the fact that the direction of the electric field was constantly changing when the positive charge was moved (starting time of the utterance at t = 1.9 min, see Figure 5A):
Figure 5. Visualization of the collaborative knowledge construction (CKC) process of (A) pair 1 and (B) pair 2. The duration of the CKC process was 5.3 min for pair 1 and 12.8 min for pair 2.
1M (Monitoring student): Well, inside those [the electric field lines], all of them are pointing toward the negative [static charge].
1S (Sharing student): Mm. Yes … And outside then … But does [the electric field] change if … Mm.
1M: Yes, so then it’s kind of … There, where the positive [moving charge] is, so then those [the electric field lines] are pointing away from its vicinity, but otherwise, it is always pointing toward the negative [static charge].
1S: Yes.
Later, pair 1 only pondered and explicated how 1M’s incorrect ideas could be formulated to write in the textbox (starting time at t = 2.3 min; see Figure 5A):
1S: So, hmm.
1M: For a), all [the electric field lines] point toward the negative [static charge].
1S: Yes.
In contrast, the monitoring student of pair 2 (2M) presented fair ideas of the problem, even though 2M also focused more on the direction of the electric field than its magnitude (starting time at t = 1.4 min, see Figure 5B):
2M (Monitoring student): But it [the electric field] is doing that kind of pendular motion there.
2S (Sharing student): So it is. Yeah.
In the explication level, 2M provided physical explanations for the presented ideas and thoughts (starting time at t = 2.3 min; see Figure 5B):
2M: Let’s write this neatly down so that the direction of the force starts to oscillate then and … Then in part a), inside … Hmm … The direction of the [electric] field is changing, of course, depending on their lengths. Or no, depending on the … Hmm … Kind of position where the moving charge is going. Thus, a kind of oscillatory motion emerges. Because it is rotating 180° or, ahem, pi radians, it is always on the side where they kind of constructively interfere and half of which are destructive.
Pair 2 also evaluated the presented ideas (see an example in Table 2), while this CKC level was absent in pair 1’s conversation.
Figure 5A shows that the CKC process of pair 1 moved straightforwardly from non-content-related talk to presenting new ideas and then to explication without evaluation. Non-content-related talk, including planning and coordinating actions as examples, was rare later in the CKC process (see Section “Quantity of the talk”). Thus, pair 1 made their conclusions based on their initial and incorrect ideas and thoughts (see Section “Quality of physics content–related talk”), which they only explicated on further (no transitions from explication to presenting new ideas). Thus, pair 1 failed to solve the problem shown in Figure 2 correctly because they concluded the following in their joint answer in the textbox:
The electric field inside the circumference of the circle always points toward the [static] charge Q1.
[Outside the circumference of the circle and] close to the [moving] charge Q2, the electric field points away. When charge Q2 moves further, the electric field again points toward the [static] negative charge.
The answer reveals that pair 1 did pay attention to the direction of the electric field but not to its magnitude. They also failed to notice how the direction of the electric field constantly changed when the positive charge moved around the negative charge.
Figure 5B shows that pair 2 had several transitions between the CKC levels and non-content-related talk, meaning that pair 2 frequently planned their actions (Section “Quantity of the talk”). These findings may relate to their problem-solving strategy, which separately considered the two aspects of the problem: the electric field inside (0–7 min) and outside the circle (7–13 min, Figure 5B; see also Figure 2). Pair 2 reached the highest CKC level when they evaluated their ideas in both parts of the problem. Pair 2 finally focused both on the magnitude and direction of the electric field, answering the problem more correctly:
[Inside the circumference of the circle], the direction [of the electric field] changes periodically; [and] the magnitude [of the electric field] increases when the [moving] charge Q2 approaches the [chosen] point a.
[Outside the circumference of the circle], when the [moving] charge Q2 is on the same side of the circumference of the circle as the [chosen] point a, the [electric] fields add up.
The answer illustrates that pair 2 made relevant observations on electric field properties, despite a few careless statements, such as that electric fields add up only under certain conditions (“when the [moving] charge Q2 is on the same side of the circumference of the circle as the [chosen] point a”). We now explore what kinds of insights the pairs’ gaze behavior provides on these three perspectives of the CKC processes that we covered in sections “Quantity of the talk”, “Quality of physics content–related talk”, and “Temporality of CKC processes”.
First, the pairs’ gaze behavior reveals that the silent moments had different purposes from the perspective of CKC (see section “Quantity of the talk”): Figure 6A indicates that pair 1 used these silent moments for writing their answer to the textbox (1S’s visual attention was on the keyboard, while 1 M’s visual attention was on the textbox). Figure 6B shows that pair 2 used these silent moments for working with the simulation (1S’s visual attention was on the sensor, moving charge, and static charge, while 1 M’s visual attention was on the sensor), and both students also focused their visual attention on the textbox. The difference between these two networks is presented in Figure 6C, indicating that pair 1 used more time for formulating their answer to the textbox and less for working with the simulation than pair 2.
Figure 6. Epistemic networks of the gaze behavior of (A) pair 1 and (B) pair 2 during silent moments. S and M after the underscore refer to the gaze of the sharing student (1S/2S) and the monitoring student (1M/2M). The difference between epistemic networks (A,B) is presented in (C). The red edges show the connections between the nodes that were stronger among pair 1 than among pair 2. The blue edges show the connections between the nodes that were weaker among pair 1 than among pair 2.
Second, the pairs’ gaze behavior indicates that the CKC processes during the non-content-related talk differed between the pairs, as was the case with the silent moments. The pairs’ gaze behavior in Figure 7 shows that the students in pair 1 paid more visual attention to the assignment and textbox than the students in pair 2 (Figures 7A,C). The students in pair 2 divided their visual attention more on the simulation view than the students in pair 1 (Figures 7B,C).
Figure 7. Epistemic networks of the gaze behavior of (A) pair 1 and (B) pair 2 during non-content-related talk. S and M after the underscore refer to the gaze of the sharing student (1S/2S) and monitoring student (1M/2M). The difference between epistemic networks (A,B) is presented in (C). The red edges show the connections between the nodes that were stronger among pair 1 than pair 2. The blue edges show the connections between the nodes that were weaker among pair 1 than pair 2.
In section “Quality of physics content–related talk,” we found that pair 1 did not present correct ideas about the direction of the electric field, and both pairs ignored the magnitude of the electric field at the beginning of their CKC processes. When presenting new ideas, pair 1 had gaze dissimilarities, so that both students paid attention to the moving charge but not simultaneously (Figure 8A). Pair 2 had gaze similarities, and they were both simultaneously paying visual attention to the moving charge (Figure 8B); these differences are also visible in the difference network in Figure 8C. It is remarkable that neither of the monitoring students paid attention to the sensor when they presented new ideas to the problem, even though the sensor provided information on the direction and magnitude of the electric field.
Figure 8. Epistemic networks of the gaze behavior of (A) pair 1 and (B) pair 2 when presenting new ideas. S and M after the underscore refer to the gaze of the sharing student (1S/2S) and monitoring student (1M/2M). The difference between epistemic networks (A,B) is presented in (C). The red edges show the connections between the nodes that were stronger among pair 1 than among pair 2. The blue edges show the connections between the nodes that were weaker among pair 1 than among pair 2.
The pairs’ gaze behavior during the explication shows their different approaches when constructing knowledge on the properties of the electric field. Figure 9A shows that both 1M and 1S focused on the textbox, with only a few fixations on the simulation view (note what we found in section “Quality of physics content–related talk”: pair 1 explicated how 1M’s incorrect ideas could be formulated in the textbox). In contrast, Figure 9B shows that 2S and 2M focused their attention on the sensor, while 2S also focused on the moving charge (note that pair 2 aimed to provide physical explanations of the presented ideas during the explication, as we found in section “Quality of physics content–related talk”). These differences between the pairs’ gaze behaviors are also visible in the difference network in Figure 9C.
Figure 9. Epistemic networks of the gaze behavior of (A) pair 1 and (B) pair 2 during explication. S and M after the underscore refer to the gaze of the sharing student (1S/2S) and the monitoring student (1M/2M). The difference between epistemic networks (A,B) is presented in (C). The red edges show the connections between the nodes that were stronger among pair 1 than among pair 2. The blue edges show the connections between the nodes that were weaker among pair 1 than among pair 2.
As we have seen in sections “Gaze behavior sheds light on the silent moments and non-content-related talk” and “Gaze behavior sheds light on the knowledge construction approaches”, both students of pair 1 focused on the assignment, textbox, and keyboard, except during the short phase when they presented new ideas regarding the problem and focused on the simulation view (Figure 8A). This kind of gaze behavior implies that pair 1 had a few moments when they could have questioned the presented incorrect ideas to the problem; for example, monitoring student 1M hardly focused their visual attention on the sensor that provided information on the direction and magnitude of the electric field. Even though the sharing student (1S) focused their attention on the sensor when they presented new ideas, 1S did not question 1M’s incorrect ideas about the problem (see section “Quality of physics content–related talk”). Based on the gaze behavior in the explication level (Figure 9A), neither 1M nor 1S tried to find supporting or contrasting evidence to the presented ideas because neither student consulted the simulation view during this CKC level.
Regarding pair 2, the edges (the blue lines) between the nodes (the AOIs) in Figures 6–10 show that pair 2 was more focused on the simulation view than pair 1 (particularly, see Figures 6C–10C). During the physics content–related talk, the visual attention of 1M and 1S was almost entirely on the simulation view (see new idea in Figure 8B, explication in Figure 9B, and evaluation in Figure 10). Pair 2 also used silent moments and non-content-related talk both for working with the simulation and formulating their solution to the problem in the textbox. This kind of gaze behavior constantly gave food for thought to the students (making new observations, explicating and evaluating those, and writing them down) that might be associated with the frequent transitions between the CKC levels and non-content-related talk that we found in section “Temporality of CKC processes.”
Figure 10. Epistemic networks of the gaze behavior of pair 2 during the evaluation (pair 1 did not evaluate their collaborative knowledge construction process). S and M after the underscore refer to the gaze of the sharing student (2S) and monitoring student (2M).
By combining video and eye-tracking data, we have introduced a novel approach to exploring CKC kinematics in a simulation-based environment. To illustrate our approach, we used video data of two student pairs’ conversations to understand their CKC processes from the perspectives of the (i) quantity of the talk, (ii) quality of the talk, and (iii) temporality (RQ1). We then applied ENA to eye-tracking data to explore how gaze behavior can shed light on CKC processes in terms of these three perspectives (RQ2). As examples, we found that gaze behavior can shed light on (i) the learning activities of the pairs during the silent moments and non-content-related talk; (ii) the chosen approaches when constructing knowledge on physical phenomena; and (iii) (the lack of) attempts to acquire the supporting or contrasting evidence on the initial ideas on the physical phenomena.
Many studies have indicated that students’ gaze similarities play a role in the learning processes and outcomes in collaborative learning settings (Schneider, 2019; Olsen et al., 2020; Becker et al., 2021). Our findings emphasize that instead of treating gaze similarity merely as a binary variable and investigating the extent to which students are or are not looking at the same objects, comprehensive attention should be paid to investigating how gaze behavior can facilitate or hinder the ongoing CKC processes. In our study, pair 1 had a straightforward transition from presenting new ideas to explicating them (RQ1), and they hardly consulted the simulation view at the higher levels of their CKC process (RQ2, Figure 9). From the perspective of guiding students in their CKC processes, it is crucial to capture the critical moments of their CKC processes, such as the phase in which pair 1 presented new idea about the problem (Sections “Quality of physics content–related talk” and “Gaze behavior sheds light on the knowledge construction approaches”, Figure 8). The analysis showed that student 1 M did not focus their visual attention on the sensor but only on the static and moving charges that did not provide information about the electric field. After that, pair 1 started the explication level by writing down their ideas and thoughts in the textbox, here without critical explication and evaluation of the presented ideas (Sections “Temporality of CKC processes” and “Gaze behavior sheds light on the temporality of CKC processes”, Figure 9). As a form of guidance in these situations, students could be made aware of each other’s gaze behavior and prompted to focus their visual attention on the relevant features of the simulation (Hayashi, 2020). The information on students’ gaze behavior and its relation to the CKC process can also help teachers and developers of educational technology, for example, in how to visualize abstract concepts, such as fields, so that the selected representations can be effectively utilized as external resources of learning (Klein et al., 2018).
When considering the gaze behavior of the pairs, attention should also be paid to student roles during the CKC process. In our study, both pairs had one student sharing the screen and another student monitoring the screen. These different roles were visible in the gaze behavior of the students of pair 2. We found that the sharing student’s (2S) gaze behavior was more scattered compared with the monitoring student’s (2M) gaze behavior during the physics content–related talk (Figures 8B, 9B). This behavior is logical because 2S had to divide their visual attention between multiple objects in the simulation view while controlling everything on the screen. Respectively, during the explication level, 2M was able to monitor the electric field by focusing their visual attention on the sensor (Figure 9B). From the perspective of these different roles, gaze dissimilarities between the students seem inevitable, emphasizing the consideration of contextual information on CKC processes when interpreting eye-tracking data and analysis (Liu et al., 2021). At best, the gaze dissimilarities between students could trigger critical discussion of the presented ideas and lead to higher CKC levels and improved learning outcomes.
Our study has certain limitations, such as using only two student pairs to illustrate our approach. This limit could be overcome in the future because the ENA scores (the summary statistic of the corresponding network) could be used to study the similarities and differences in the pairs’ gaze behavior with larger sample sizes (for more details, see Shaffer et al., 2016; Andrist et al., 2018). In these cases, it is important to include only the necessary AOIs (the nodes of the epistemic networks) into the analysis so that the epistemic networks are easily interpretable. Moreover, the eye-tracking data analysis has some limitations when the data are collected in authentic, uncontrollable settings, as in our case: for example, the students were able to move freely during the data collection, so their visual scene was constantly changing when they moved their head and moved the objects in the simulation view. In our study, we aimed to improve the validity and reliability of the interpretations by analyzing the video data from three perspectives: the quantity of the talk, the quality of the physics content–related talk, and the temporality of CKC processes; and then exploring how eye-tracking data and analysis can shed light on CKC processes in terms of these three perspectives.
Despite these limitations, our study has several implications for future research. We illustrated how gaze behavior reflects the overall progress of CKC processes (Sections “Temporality of CKC processes” and “Gaze behavior sheds light on the temporality of CKC processes”) and the different CKC levels (Sections “Quality of physics content–related talk” and “Gaze behavior sheds light on the knowledge construction approaches”). In particular, gaze behavior could be used to capture the different activities that the pairs (or groups, in general) conduct within a specific CKC level, during non-content-related talk, or during silent moments. For example, even though the students were silent even over half of the time (as was the case with pair 1), their gaze behavior during these silent moments may help us understand their success or failures in the CKC process. In our study, the gaze behavior of pair 1 indicated that they used these silent moments for writing their answers in the textbox, even though they had not made proper observations of the properties of the electric field. The gaze behavior of pair 2 indicated that they also used these silent moments for working with the simulation; this behavior might contribute to their iterative CKC process, in which they moved back and forth between the CKC levels and non-content-related talk.
As a methodological implication, we followed and extended Andrist et al.’s (2018) work by applying ENA to study gaze behavior in an authentic simulation-based environment. Our approach considered the spatial and temporal dimensions of the eye-tracking data, both of which provided essential information about the CKC processes. Our approach complements (instead of compensating for) the cross-recurrence quantification analysis, in which the focus is on the temporal alignment of students’ gazes, here without spatial information about their visual attention. Thus, our study provides a novel approach for exploring CKC processes by combining video data and both spatial and temporal information from eye-tracking data. In the future, these explorations, together with learning outcomes, should be further investigated with larger sample sizes and in more diverse contexts. Future studies should also focus not only on the kinematics, but also the dynamics of these constructs, hence examining whether and why similar gaze behavior can lead to dissimilarities in the CKC process and its quality. Visualizations of CKC processes and gaze behavior could help the teachers and developers of educational technology design, implement, and refine productive CKC processes in simulation-based environments with appropriate forms of guidance. Contrary to the mobile, wearable eye trackers that we used in the current study, screen-based eye trackers could ease eye-tracking data analysis and visualization in computer-supported settings. Through the understanding of gaze behavior, one could envision a future where teachers use such trackers to guide and synchronize students’ gaze in real time. Therefore, it is crucial to involve teachers and students in co-designing these visualization tools to increase their usability, transparency, and acceptability among practitioners (Buckingham Shum et al., 2019).
Typically, eye-tracking data analysis in CKC settings has focused on whether students are looking at the same objects but has done so without analyzing whether these objects are relevant to the problem at hand. We have illustrated how gaze behavior can shed light on CKC regarding the quantity of the talk, quality of the physics content–related talk, and temporality of CKC processes. These kinds of approaches may help teachers, researchers, and developers of educational technologies understand and guide CKC processes by showing the critical moments in these processes and revealing the features in the simulation environment that attract unnecessary visual attention. In the future, which kind of gaze-based indicators appropriately reflect the temporality of CKC processes and complement cross-recurrence quantification analyses should be considered. For example, when the CKC processes have low quality or move in the wrong direction, gaze dissimilarities could trigger critical discussion of the presented ideas and lead to higher CKC levels and better learning outcomes. Therefore, gaze dissimilarity can occasionally be essential for rising to higher-level CKC processes and for favorably advancing the solutions to a given problem.
The datasets presented in this article are not readily available because video and eye-tracking data analyzed cannot be public due to personal data protection. More information on the data can be requested from the corresponding author. Requests to access the datasets should be directed to am9uaS5sYW1zYUBvdWx1LmZp.
The study was reviewed and approved by the Human Sciences Ethics Committee of the University of Jyväskylä. The participants provided their written informed consent to participate in this study.
JL: conceptualization, methodology, formal analysis, investigation, data curation, writing (original draft, plus review, and editing), and visualization. JiK: conceptualization, investigation, data curation, and writing (review and editing). AL, PK, and TM: conceptualization and writing (review and editing).JaK: conceptualization, investigation, and writing (review and editing). RH: funding acquisition, conceptualization, and writing (review and editing). All authors contributed to the article and approved the submitted version.
This research was funded by the Academy of Finland (grant number 318905, the Multidisciplinary Research on Learning and Teaching profiles II of the University of Jyväskylä).
The authors declare that the research was conducted in the absence of any commercial or financial relationships that could be construed as a potential conflict of interest.
All claims expressed in this article are solely those of the authors and do not necessarily represent those of their affiliated organizations, or those of the publisher, the editors and the reviewers. Any product that may be evaluated in this article, or claim that may be made by its manufacturer, is not guaranteed or endorsed by the publisher.
Andrist, S., Ruis, A. R., and Shaffer, D. W. (2018). A network analytic approach to gaze coordination during a collaborative task. Comput. Hum. Behav. 89, 339–348. doi: 10.1016/j.chb.2018.07.017
Becker, S., Mukhametov, S., Pawels, P., and Kuhn, J. (2021). “Using mobile eye tracking to capture joint visual attention in collaborative experimentation,” in Physics Education Research Conference 2021 Proceedings. (eds.) M. Bennett, B. Frank, and R. Vieyra; College Park, US: American Association of Physics Teachers, 39–44.
Bowman, D., Swiecki, Z., Cai, Z., Wang, Y., Eagan, B., Linderoth, J., et al. (2021). “The mathematical foundations of epistemic network analysis,” in Proceedings of the Advances in Quantitative Ethnography: Second International Conference—ICQE 2020, eds. A. R. Ruis and S. B. Lee; Switzerland: Springer, 91–105
Buckingham Shum, S., Ferguson, R., and Martinez-Maldonado, R. (2019). Human-centerd learning analytics. J. Learn. Analy. 6, 1–9. doi: 10.18608/jla.2019.62.1
Chang, C.-J., Chang, M.-H., Liu, C.-C., Chiu, B.-C., Fan Chiang, S.-H., Wen, C.-T., et al. (2017). An analysis of collaborative problem-solving activities mediated by individual-based and collaborative computer simulations. J. Comput. Assist. Learn. 33, 649–662. doi: 10.1111/jcal.12208
Chernikova, O., Heitzmann, N., Stadler, M., Holzberger, D., Seidel, T., and Fischer, F. (2020). Simulation-based learning in higher education: a meta-analysis. Rev. Educ. Res. 90, 499–541. doi: 10.3102/0034654320933544
Cicchetti, D. V. (1994). Guidelines, criteria, and rules of thumb for evaluating normed and standardized assessment instruments in psychology. Psychol. Assess. 6, 284–290. doi: 10.1037/1040-3590.6.4.284
Clark, D., Nelson, B., Sengupta, P., and D’Angelo, C. (2009). Rethinking Science Learning Through Digital Games and Simulations: Genres, Examples, and Evidence. Washington, DC: Learning Science: Computer Games, Simulations, and Education Workshop Sponsored by the National Academy of Sciences.
Coco, M. I., and Dale, R. (2014). Cross-recurrence quantification analysis of categorical and continuous time series: an R package. Front. Psychol. 5:510. doi: 10.3389/fpsyg.2014.00510
Cohen, J. (1960). A coefficient of agreement for nominal scales. Educ. Psychol. Meas. 20, 37–46. doi: 10.1177/001316446002000104
Cook, D. A., Brydges, R., Zendejas, B., Hamstra, S. J., and Hatala, R. (2013). Technology-enhanced simulation to assess health professionals: a systematic review of validity evidence, research methods, and reporting quality. Acad. Med. 88, 872–883. doi: 10.1097/ACM.0b013e31828ffdcf
de Jong, T., and Lazonder, A. W. (2014). “The guided discovery learning principle in multimedia learning,” in The Cambridge Handbook of Multimedia Learning. ed. R. E. Mayer, vol. 1 (Cambridge: Cambridge University Press), 371–390.
de Jong, T., Linn, M. C., and Zacharia, Z. C. (2013). Physical and virtual laboratories in science and engineering education. Science 340, 305–308. doi: 10.1126/science.1230579
Eagan, B., Brohinsky, J., Wang, J., and Shaffer, D.W. (2020). “Testing the reliability of inter-rater reliability,” in Proceedings of the Tenth International Conference on Learning Analytics and Knowledge (LAK '20) (New York, USA: Association for Computing Machinery), 454–461
Hahn, L., and Klein, P. (2022). Eye tracking in physics education research: a systematic literature review. Phys. Rev. Phys. Educ. Res. 18:013102. doi: 10.1103/PhysRevPhysEducRes.18.013102
Hayashi, Y. (2020). Gaze awareness and metacognitive suggestions by a pedagogical conversational agent: an experimental investigation on interventions to support collaborative learning process and performance. Int. J. Comput.-Support. Collab. Learn. 15, 469–498. doi: 10.1007/s11412-020-09333-3
Hayashi, Y., and Shimojo, S. (2021). “Investigating gaze behavior of dyads in a collaborative explanation task using a concept map: Influence of facilitation prompts on perspective taking,” in Proceedings of the 14th International Conference on Computer-Supported Collaborative Learning—CSCL 2021. eds. C.E. Hmelo-Silver, B. De Wever, and J. Oshima (Bochum, Germany: International Society of the Learning Sciences), 149–152.
Henri, F. (1992). “Computer conferencing and content analysis,” in Collaborative Learning Through Computer Conferencing. The Najadan Papers. ed. A. R. Kaye (Berlin, Heidelberg: Springer-Verlag), 117–136.
Hessels, R. S. (2020). How does gaze to faces support face-to-face interaction? A review and perspective. Psychon. Bull. Rev. 27, 856–881. doi: 10.3758/s13423-020-01715-w
Holmqvist, K., Nyström, M., Andersson, R., Dewhurst, R., Jarodzka, H., and van de Weijer, J. (2011). Eye Tracking: A Comprehensive Guide to Methods and Measures. Oxford: Oxford University Press
Jensen, J. L., and Lawson, A. (2011). Effects of collaboration and inquiry on reasoning and achievement in biology. CBE Life Sci. Educ. 10, 64–73. doi: 10.1187/cbe.10-07-0089
Jeong, H., and Hmelo-Silver, C. E. (2010). Productive use of learning resources in an online problem-based learning environment. Comput. Hum. Behav. 26, 84–99. doi: 10.1016/j.chb.2009.08.001
Jeong, H., Hmelo-Silver, C. E., and Jo, K. (2019). Ten years of computer-supported collaborative learning: a meta-analysis of CSCL in STEM education during 2005–2014. Educ. Res. Rev. 28:100284. doi: 10.1016/j.edurev.2019.100284
Jeong, H., Hmelo-Silver, C., and Yu, Y. (2014). An examination of CSCL methodological practices and the influence of theoretical frameworks 2005–2009. Int. J. Comput.-Support. Collab. Learn. 9, 305–334. doi: 10.1007/s11412-014-9198-3
Just, M. A., and Carpenter, P. A. (1980). A theory of reading: from eye fixations to comprehension. Psychol. Rev. 87, 329–354. doi: 10.1037/0033-295X.87.4.329
Klein, P., Viiri, J., Mozaffari, S., Dengel, A., and Kuhn, J. (2018). Instruction-based clinical eye-tracking study on the visual interpretation of divergence: how do students look at vector field plots? Phys. Rev. Phys. Educ. Res. 14:010116. doi: 10.1103/PhysRevPhysEducRes.14.010116
Lämsä, J., Hämäläinen, R., Koskinen, P., and Viiri, J. (2018). Visualising the temporal aspects of collaborative inquiry-based learning processes in technology-enhanced physics learning. Int. J. Sci. Educ. 40, 1697–1717. doi: 10.1080/09500693.2018.1506594
Lämsä, J., Hämäläinen, R., Koskinen, P., Viiri, J., and Lampi, E. (2021a). What do we do when we analyse the temporal aspects of computer-supported collaborative learning? A systematic literature review. Educ. Res. Rev. 33:100387. doi: 10.1016/j.edurev.2021.100387
Lämsä, J., Hämäläinen, R., Koskinen, P., Viiri, J., and Mannonen, J. (2020). The potential of temporal analysis: combining log data and lag sequential analysis to investigate temporal differences between scaffolded and non-scaffolded group inquiry-based learning processes. Comput. Educ. 143:103674. doi: 10.1016/j.compedu.2019.103674
Lämsä, J., Uribe, P., Jiménez, A., Caballero, D., Hämäläinen, R., and Araya, R. (2021b). Deep networks for collaboration analytics: promoting automatic analysis of face-to-face interaction in the context of inquiry-based learning. J. Learn. Analy. 8, 113–125. doi: 10.18608/jla.2021.7118
Lehtinen, A., and Viiri, J. (2017). Guidance provided by teacher and simulation for inquiry-based learning: a case study. J. Sci. Educ. Technol. 26, 193–206. doi: 10.1007/s10956-016-9672-y
Lin, T.-J., Duh, H. B.-L., Li, N., Wang, H.-Y., and Tsai, C.-C. (2013). An investigation of learners’ collaborative knowledge construction performances and behavior patterns in an augmented reality simulation system. Comput. Educ. 68, 314–321. doi: 10.1016/j.compedu.2013.05.011
Liu, C. C., Hsieh, I. C., Wen, C. T., Chang, M. H., Fan Chiang, S. H., Tsai, M.-J., et al. (2021). The affordances and limitations of collaborative science simulations: the analysis from multiple evidences. Comput. Educ. 160:104029. doi: 10.1016/j.compedu.2020.104029
Marquart, C.L., Swiecki, Z., Collier, W., Eagan, B., Woodward, R., and Shaffer, D.W. (2021). rENA: epistemic network analysis (0.2.3). Available at: https://CRAN.R-project.org/package=rENA
Mavin, T. J., Kikkawa, Y., and Billett, S. (2018). Key contributing factors to learning through debriefings: commercial aviation pilots’ perspectives. Int. J. Train. Res. 16, 122–144. doi: 10.1080/14480220.2018.1501906
Molenaar, I. (2021). “Personalisation of learning: towards hybrid human-AI learning technologies,” in OECD Digital Education Outlook 2021: Pushing the Frontiers With Artificial Intelligence, Blockchains and Robots. ed. S. Vincent-Lancrin, vol. 1 (Paris: OECD Publishing), 57–78.
Olsen, J. K., Sharma, K., Rummel, N., and Aleven, V. (2020). Temporal analysis of multimodal data to predict collaborative learning outcomes. Br. J. Educ. Technol. 51, 1527–1547. doi: 10.1111/bjet.12982
Richardson, D. C., and Dale, R. (2005). Looking to understand: the coupling between speakers’ and listeners’ eye movements and its relationship to discourse comprehension. Cogn. Sci. 29, 1045–1060. doi: 10.1207/s15516709cog0000_29
Rutten, N., van Joolingen, W. R., and van der Veen, J. T. (2012). The learning effects of computer simulations in science education. Comput. Educ. 58, 136–153. doi: 10.1016/j.compedu.2011.07.017
Schellens, T., and Valcke, M. (2005). Collaborative learning in asynchronous discussion groups: what about the impact on cognitive processing? Comput. Hum. Behav. 21, 957–975. doi: 10.1016/j.chb.2004.02.025
Schneider, B. (2019). “Unpacking collaborative learning processes during hands-on activities using mobile eye-trackers,” in A Wide Lens: Combining Embodied, Enactive, Extended, and Embedded Learning in Collaborative Settings, 13th International Conference on Computer Supported Collaborative Learning (CSCL). eds. K. Lund, G.P. Niccolai, E. Lavoué, C. Hmelo-Silver, G. Gweon, and M. Baker (Lyon, France: International Society of the Learning Sciences), Vol. 1, 41–48.
Schneider, B., Dowell, N., and Thompson, K. (2021). Collaboration analytics—current state and potential futures. J. Learn. Analy. 8, 1–12. doi: 10.18608/jla.2021.7447
Schneider, B., Sharma, K., Cuendet, S., Zufferey, G., Dillenbourg, P., and Pea, R. (2018). Leveraging mobile eye-trackers to capture joint visual attention in co-located collaborative learning groups. Int. J. Comput.-Support. Collab. Learn. 13, 241–261. doi: 10.1007/s11412-018-9281-2
Schotter, E. R., Angele, B., and Rayner, K. (2012). Parafoveal processing in reading. Atten. Percept. Psychophysiol. 74, 5–35. doi: 10.3758/s13414-011-0219-2
Shaffer, D. W., Collier, W., and Ruis, A. R. (2016). A tutorial on epistemic network analysis: analyzing the structure of connections in cognitive, social, and interaction data. J. Learn. Analy. 3, 9–45. doi: 10.18608/jla.2016.33.3
Sharma, K., Olsen, J.K., Verma, H., Caballero, D., and Jermann, P. (2021). “Challenging joint visual attention as a proxy for collaborative performance,” in Proceedings of the 14th International Conference on Computer-Supported Collaborative Learning—CSCL 2021, eds. C.E. Hmelo-Silver, B. De Wever, and J. Oshima (Bochum, Germany: International Society of the Learning Sciences), 91–98.
Stevenson, M., Lai, J. W. M., and Bower, M. (2022). Investigating the pedagogies of screen-sharing in contemporary learning environments—a mixed methods analysis. J. Comput. Assist. Learn. 38, 770–783. doi: 10.1111/jcal.12647
Strohmaier, A. R., MacKay, K. J., Obersteiner, A., and Reiss, K. M. (2020). Eye-tracking methodology in mathematics education research: a systematic literature review. Educ. Stud. Math. 104, 147–200. doi: 10.1007/s10649-020-09948-1
Tatler, B. W., Kirtley, C., MacDonald, R. G., Mitchell, K. M. A., and Savage, S. W. (2014). “The active eye: perspectives on eye movement research” in Current Trends in Eye Tracking Research. eds. M. Horsley, M. Eliot, B. A. Knight, and R. Reilly (Switzerland: Springer International Publishing), 3–16.
Tobii Pro AB (2017). Eye tracker data quality report: Accuracy, precision and detected gaze under optimal conditions—Controlled environment: Tobii Pro glasses 2 firmware v1.61. Available at: https://www.tobiipro.com/siteassets/tobii-pro/accuracy-and-precision-tests/tobii-pro-glasses-2-accuracy-and-precision-test-report.pdf
Tobii Pro AB (2022). Pro Lab user manual v1.181. Available at: https://www.tobiipro.com/siteassets/tobii-pro/user-manuals/Tobii-Pro-Lab-User-Manual/
UC Berkeley (2022). Web-based inquiry science environment. WISE. Available at: https://wise.berkeley.edu/
University of Colorado Boulder (2022a). Charges and fields PhET simulation. Available at: http://phet.colorado.edu/sims/html/charges-and-fields/latest/charges-and-fields_en.html
University of Colorado Boulder (2022b). PhET interactive simulations. Available at: https://phet.colorado.edu/
Veerman, A., and Veldhuis-Diermanse, E. (2001). “Collaborative learning through computer-mediated communication in academic education,” in Proceedings of the Euro CSCL 2001 Conference. eds. P. Dillenbourg, A. Eurelings, and K. Hakkarainen; Maastricht, The Netherlands: University of Maastricht, 625–632.
Keywords: collaborative learning, epistemic network analysis, eye tracking, gaze, collaborative knowledge construction, multimodal data, simulation, video
Citation: Lämsä J, Kotkajuuri J, Lehtinen A, Koskinen P, Mäntylä T, Kilpeläinen J and Hämäläinen R (2022) The focus and timing of gaze matters: Investigating collaborative knowledge construction in a simulation-based environment by combined video and eye tracking. Front. Educ. 7:942224. doi: 10.3389/feduc.2022.942224
Received: 12 May 2022; Accepted: 03 October 2022;
Published: 20 October 2022.
Edited by:
Martin Rusek, Charles University, CzechiaReviewed by:
Anna Shvarts, Utrecht University, NetherlandsCopyright © 2022 Lämsä, Kotkajuuri, Lehtinen, Koskinen, Mäntylä, Kilpeläinen and Hämäläinen. This is an open-access article distributed under the terms of the Creative Commons Attribution License (CC BY). The use, distribution or reproduction in other forums is permitted, provided the original author(s) and the copyright owner(s) are credited and that the original publication in this journal is cited, in accordance with accepted academic practice. No use, distribution or reproduction is permitted which does not comply with these terms.
*Correspondence: Joni Lämsä, am9uaS5sYW1zYUBvdWx1LmZp
Disclaimer: All claims expressed in this article are solely those of the authors and do not necessarily represent those of their affiliated organizations, or those of the publisher, the editors and the reviewers. Any product that may be evaluated in this article or claim that may be made by its manufacturer is not guaranteed or endorsed by the publisher.
Research integrity at Frontiers
Learn more about the work of our research integrity team to safeguard the quality of each article we publish.