- 1Chair of Science Education, Faculty of Chemistry, University of Konstanz, Konstanz, Germany
- 2Thurgau University of Teacher Education, Kreuzlingen, Switzerland
Computational literacy is one of the 21st century skills–students must therefore acquire the appropriate competencies in computer science to meet the demands of 21st century society. To achieve this, teachers must be adequately trained. Furthermore, this also means that introductory computer science education concepts must also be integrated into the training of (pre-service) science teachers and anchored in the curriculum. This is particularly important because many scientific professions or research can no longer be done without basic computer knowledge and computational literacy. The central approach to educating future teachers is the university course of study. This paper aims to systematically provide an insight into which scientifically relevant publications address this issue. The essential criterion in this review is the restriction on science education or more general science, technology, engineering, and mathematics (STEM) education in schools or universities. Equally important is the specification that computational literacy (informatics competencies, information literacy, computer literacy, or computational thinking) are purposefully taught or trained. Only publications in the Web of Science database were included for quality assurance. This paper investigates to what extent scientifically valid research or knowledge is available at this junction, i.e., computational literacy in science education. Thereby, we distinguish between different approaches, such as effect studies on individual aspects, isolated practical contributions with a pronounced trial-and-error character, or systematic or model-based considerations in quantitative and qualitative studies. The results show common aspects, prominent trends, and promising approaches. However, possibly existing (subject-) didactic concerns should be considered in more detail and the dual perspective role of the students as future teachers. In addition to learning basic computer skills, the ability to teach them is also essential so that the scientific education of the students can benefit from them to the required extent.
Introduction
Computational literacy, computational thinking (CT), data literacy, for example, or more general informatics competencies are already indispensable competencies in the 21st century. In addition, they are increasingly becoming a basic prerequisite for successful and modern science teaching. This is especially true for primary and secondary classes, where new questions arise that cannot be addressed without these competencies. Therefore, it is long overdue that informatics competencies are more strongly anchored at universities and especially in teacher training in science, technology, engineering, and mathematics (STEM) subjects. Because in these subjects, the dependence on supporting disciplines has always been very pronounced. A comparison with mathematics shows clear parallels here: Mathematics is an essential subject in the group of natural and structural sciences. However, beyond that, mathematical concepts and competencies are in themselves already an essential component in the natural sciences since they often make it possible in the first place to describe a problem objectively and effectively and to determine a possible solution. Meanwhile, computer science also has this dual role. Like mathematics, the subject also belongs to this group. However, although computer science competencies play a crucial role in many aspects of scientific problems, they are still far from being integrated into education as they should be in the 21st century. The extent to which this also applies to science education will be examined more closely in this paper and under a very specific premise. Our main research questions are where and how computational literacy in science education plays a role in the existing literature and what commonalities or differences can be identified. This includes the important related question of how informatics competencies and concepts can be effectively integrated into science education to provide educators with helpful approaches. Based on this, we will discuss possible relevant conclusions or patterns and possibly further research questions. The need to focus from the natural sciences perspective arises mainly for two reasons: Firstly, it is not enough to teach only informatics content and competencies. For then, there is a real danger that transfer will fail, and only inert knowledge will emerge. If, for example, a student learns which control structures (for loop, if-else condition) exist, he or she is not yet able to formulate an algorithm that numerically solves a differential equation considering scientific framework conditions. Secondly, STEM curricula in various countries or regions explicitly demand that informatics competencies must be taught integratively in science subjects. It follows implicitly that prospective teachers have the possibility to build up knowledge and skills going beyond the levels of their perspective primary or secondary class students in this area already during their studies at university. And for this, instructors or teachers capable of integrating informatics competencies into their courses can be game-changers.
In order to be able to investigate relevant research questions at this crucial junction between computational literacy and STEM education, a specific review is needed that pays particular attention to the STEM perspective. Previous reviews are limited to CT in the narrower sense (Wang et al., 2021) or do not consider STEM education exclusively (Lyon and Magana, 2020). Thus, our contribution will consider a broader notion of CT but strictly aim at its integration in STEM education, gathering possible trends and promising approaches or examples.
Methodology
When studying the relevant literature, it became apparent early on that the terminology is inconsistent; therefore, a systematic analysis can be negatively affected by this circumstance. For instance, we have often found the term CT because it is often used as a synonym for various informatics competencies. Also, the distinction between computer literacy and data literacy is not clear-cut and sometimes even contradictory. However, one of the main aims of our paper is to cover the literature relevant to our research question. Therefore, we do not consider it useful to exclude papers that may use inconsistent terminologies if the relevance of the content of informatics competencies in STEM education is sufficiently present. At this point, it can already be seen that this topic has not yet been researched much, and therefore no uniform terminology has been established. For this reason, too, we will not make any corrections to the terms used in the papers in the following analysis but will retain the terminology of the respective authors. It must be stated in advance that the terms are not used uniformly throughout our review but rather in the context of the respective paper. Furthermore, it is neither possible nor ethically justifiable for us to reliably anticipate and reinterpret an author’s understanding or intention beyond the concrete text. Finally, this paper aims not to achieve uniformity but to identify and categorize relevant scientific contributions in the context of the research question based on specific keywords. In addition, after overseeing the relevant literature, we prefer the term computational literacy in this review paper to express the term informatics competencies in English in a suitable manner.
Based on this premise, we followed the four stages of the PRISMA standard (Page et al., 2021) for the external structure of the selection process (see Figure 1). First, all entries from the database are identified using keywords identifying the articles. As will be explained in more detail in Subsection “Identification: Keyword-based search and findings,” the check for duplicates is not necessary. Then, we excluded non-qualifying papers within a combined screening phase by screening the articles and deciding on the studies’ eligibility. At first, we did this by filtering only based on the title and abstract. Because we had already pre-selected using an appropriately restrictive search syntax, we did not have to screen out any papers at this point. In the second round of the screening phase, we used defined exclusion criteria to check eligibility as described in detail in Subsection “Screening and deciding on eligibility: Delimitation by exclusion” and excluded appropriate papers.
Identification: Keyword-based search and findings
Methodically, the database “Web of Science” was used, and the abstracts were searched for the key terms “informatics competence” (or synonymous terms, see also above) and “STEM education.” In addition, the search was refined by considering, for example, only English-language papers assigned to the category “education.” In this way, it can be ensured that the publications found have undergone at least a peer review process in terms of quality control and seriousness. Therefore, this database is chosen carefully. The Web of Science can be considered the most scientific database containing only quality journals that meet the criteria of being international, peer-reviewed, and recognized in the scientific community. Since, as mentioned at the beginning, computer science itself is a discipline of its own in the STEM field, the restriction that the focus should not be on computer science education or computer science itself, but rather on computational literacy in science education, should take effect as early as possible. Therefore, already here, we only considered papers that explicitly use the term “STEM education” and not just “STEM” in the abstract. Also, in this first look at the literature, we did not want to look too singularly at individual subjects such as physics or chemistry but rather at papers that take a broad, holistic approach to the natural sciences. We found 62 publications meeting these search criteria. You can read the concrete query term and the details from Table 1.
Furthermore, this setting guarantees that there can be no duplicates among the papers found. This is because the 62 papers met the specified search parameters and there were no duplicates or papers without peer review that we would have had to eliminate at this point.
Screening and deciding on eligibility: Delimitation by exclusion
At this stage, we could not identify any publications by screening the abstracts that could not be classified as relevant or helpful for our systematic review. In addition, as we searched for the keywords only in the abstract, no papers had to be excluded during the first phase of the screening, e.g., with the help of automated methods. After the first screening, these papers were examined more closely to decide on the papers’ eligibility and determine whether they satisfy the premise mentioned initially. Because of the dual role of computer science and its broad presence as an affine science that is often used on the practical side to look at problems, it has not been ensured up to this point that the publications are really about computational literacy being used specifically to support science education and its implementation. Therefore, three reasons for exclusion were formulated to identify only papers that show the role that computational literacy can have in the education of teachers, lecturers, and students (pupils) or the teaching of science subjects (see Figure 1).
Having a closer look at the 62 papers, we discovered that, although the papers often claim to discuss informatics content and competencies in STEM education, a closer analysis revealed that the publications do not meet the required parameters. The following excluding criteria were applied. We checked the abstract of each paper against the three criteria in the order given. If, for example, excluding criterion (a) was met, the paper was sorted out and not further checked against the following criteria. Only if a publication did not meet all three exclusion categories did we consider it in the systematic analysis (see Section “Analysis and evaluation”). We note at this point that we deliberately do not differentiate too strictly to what extent computer literacy is integrated. On the one hand, the data situation in this respect was too small from the beginning, and on the other hand, our goal in this early phase of the research is to show whether computer literacy is fundamentally integrated or not. Therefore, the following three exclusion criteria are only roughly based on these gradations: (a) missing functional or direct integration, (b) integration without application, and (c) no integration. In our view, an even more differentiated distinction seems to make sense only in later studies.
(a) The first criterion for excluding papers concerns publications primarily using digital tools without discussing relevance to computer science competencies: Because information technology is almost omnipresent in the 21st century, it is also more often a supportive element in STEM lessons. However, very often, there is a danger of remaining on the unreflective level from the perspective of the student (user): This situation is illustrated, for example, by Rajaei et al. (2012), wherein non-computer science STEM education information technology is explicitly used as a tool and not as an object of teaching. This can be compared to claiming the use of mathematical concepts in a lesson just because a calculator. Similarly, if CT is included in a lesson only because one uses a platform that has implemented this concept to visualize data (Akbar et al., 2018) it does not guarantee that students deal with CT actively. Thus, the concept remains in the background and is not actively processed by learners. More generally speaking, there is a risk of creating product knowledge because engagement with the underlying concepts only occurs through the platform and thus does not become the subject of active cognitive processing.
(b) Lack of or inappropriate focus on computational literacy: Another group of excluded publications, in contrast to the first, can be assigned to science education but without focusing on the specific teaching of or linking to computational literacy or related concepts in science education. Although Dziabenko and Budnyk (2019) show that informatics aspects play a role in the background when digital experiments and laboratories are integrated into science education, but the essential concepts from informatics remain mainly hidden or cannot be actively taken up and transferred to other cases. This is because they must be explicitly addressed and linked. This includes papers covering science education and possibly also partial aspects of computer science, but whose questions have a completely different focus. This is the case for the paper by Lamb et al. (2018), which aims at developing a model to predict later career choices in the STEM field, e.g., based on latent traits. The designing of serious educational games is used as an intervention approach.
(c) No (integration into) STEM education: Computer science content was taught independently without integrating natural science subjects. Mostly, this involved offerings for teachers or learners in computer science or computer science-related events or courses. In this context, computer science is either focused on as a standalone subject as one of the STEM subjects, or it is less concerned with science education in general or training issues of prospective teachers. In the latter case, the focus is then on teaching computer science as a subject and not, for example, on issues of didactics or subject didactics. For example, Kim et al. (2018) discuss informatics competence regarding how prospective teachers can learn and teach the concept of debugging, but without referencing science subjects or content. The paper is directly oriented toward computer science education to embed it more strongly in the education of teachers in general. Therefore, it is an attempt to promote computer science itself more deeply in education. Also, Leonard et al. (2016) explore the relevant competency of CT from an educational perspective but without integrating its use and applicability in science contexts.
The flow diagram (see Figure 1) shows that the second and third criteria are the most relevant ones, as they sort out the highest number of papers from the keyword-based database findings. This fact supports the relevance of our research question, as it shows that while there are a growing number of articles on computational literacy and STEM education, however, too few examine the integration of computational literacy into STEM education as called for in the introduction.
Finalizing the list of studies to include in the systematic review
After excluding irrelevant studies, 24 papers fulfilled the requirements (see Sections “Identification: Keyword-based search and findings” and “Screening and deciding on eligibility: Delimitation by exclusion”), which were then analyzed and categorized under certain aspects to prospectively work out possible questions that could be target-oriented for a further investigation of the importance of computational literacy in science education.
Analysis and evaluation
From now on we look closer at selected aspects only of the 24 publications mentioned above that fit the requirements of our central question: Where and, if applicable, how can computational literacy and related concepts be effectively integrated into science education or lessons? Table 2 gives an overview of these publications, including a brief description of the aspects relevant to our research topic, and Table 3 shows the number of papers per item.
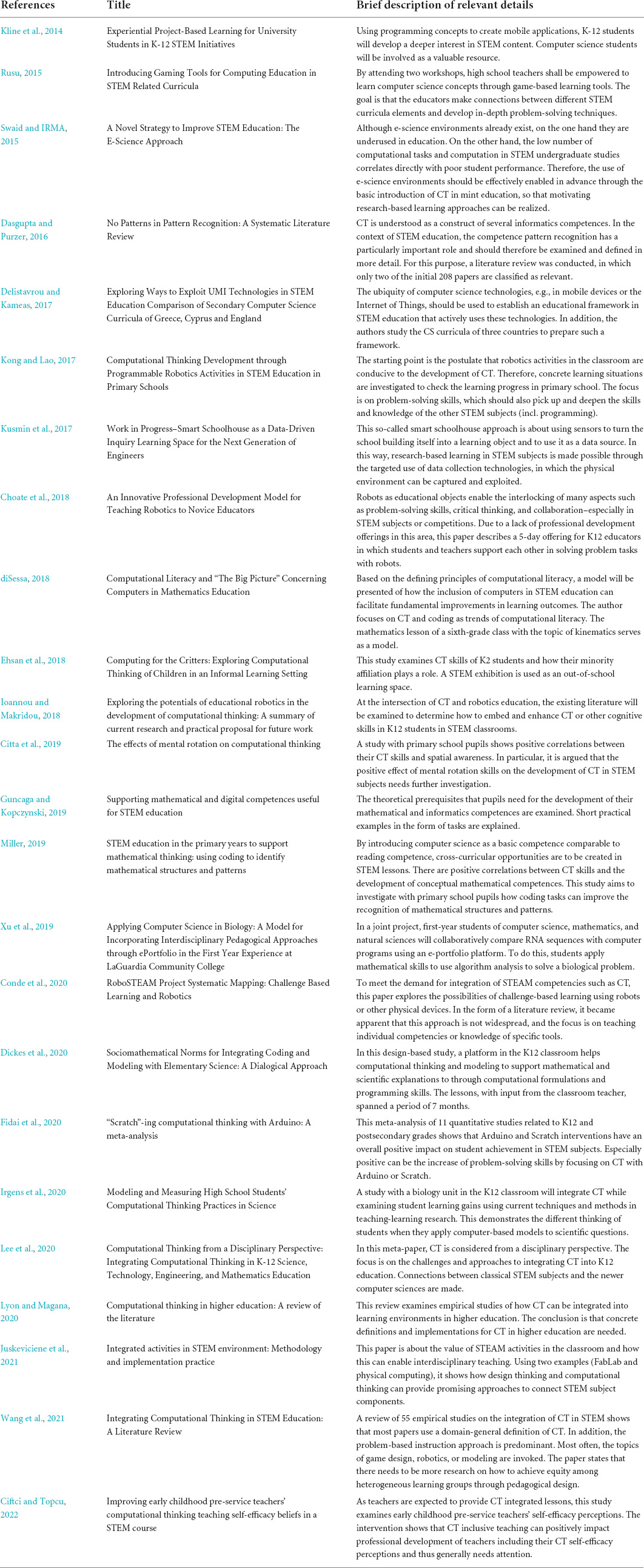
Table 2. List of papers analyzed with a brief description of relevant details (sorted by year of publication).
Theoretical or practical approach?
The majority emphasizes practical examples or interventions. On the one hand, there are isolated compact courses where K12 teachers are introduced to informatics competencies such as CT within 5 days using robotics courses (Choate et al., 2018). To increase the practical part, this paper explicitly emphasizes the challenging character with a special view of student competitions. In this way, students’ participation in competitions is increased by empowering their teachers to support them after additional training. On the other hand, CT is attempted to be systematically and broadly integrated into STEM education by incorporating appropriate learning opportunities through an e-science approach and active integration of computer science. While 13 papers follow this focus, eight articles consider the issue from a stronger theoretical emphasized perspective. For example, Lyon and Magana (2020) and Wang et al. (2021) review the literature to investigate how CT can be integrated into STEM education. This is limited to an isolated aspect of computational literacy, namely CT.
In contrast to this, however, the broad consideration of the whole STEM area is counterposed. In contrast to our paper, it is not ostensibly a matter of teaching computational literacy in science subjects–i.e., without computer science-related subjects. A different theoretical approach is taken by comparing computer science curricula from three European countries in a preliminary step to identify points of contact that enable a strengthening of STEM education supported by computer science technologies (Delistavrou and Kameas, 2017). It is remarkable that already at the beginning of the scientific investigation, the starting point is explicitly existing school curricula in computer science to search for synergies in other STEM subjects based on the associated competencies.
Few publications, such as those by Guncaga and Kopczynski (2019) and Juskeviciene et al. (2021), attempt to cover both theoretical analyses like literature reviews and practical studies or examples equally.
As a first interim result, we can state that we have found an increasing number of contributions from practice regarding our research questions, which are often based on isolated individual surveys or studies, but without focus on a systematically holistic approach, e.g., in the sense of a spiral curriculum or a theoretical model. Consequently, these two research approaches still seem to be primarily dealt with separately, suggesting that, in the absence of a sufficient number of publications and sound findings, fundamental questions remain unconsidered and make it challenging to incorporate them.
Furthermore, we can summarize the following aspects that have proven beneficial in several practical studies: Students must either be able to experience a concrete added value or there must be a need to address computational literacy from the context or the scientific content. This plays an important role, especially if the students are not interested in computer science per se. Consequently, the course teacher must ensure through the appropriate choice of scientific content and its didactic-methodical preparation that students can perceive computational literacy as purposeful and effective through active engagement. It is also beneficial if the effort or learning time is proportionate. For example, it is not helpful if 90% of the time in a course is spent learning computational literacy but only 10% is spent on transferring or linking it to scientific issues. The difficulty, however, is to ensure that students still have enough individual learning time to assimilate and process computational literacy sufficiently. Transparent learning objectives in the design of courses can help here. Finally, it can be positive to alternate between deductive and inductive phases regarding informatics competencies in order to allow for a stronger linkage. For example, the teacher can teach digital measurement in the beginning, with time discretization already implicitly integrated. And only later are the aspects of data type and memory finiteness explicitly taken up and generalized. However, it is important that computational literacy comes explicitly to the fore at some point.
Age
On the positive side, publications can be assigned to all three age groups in education according to their respective focus: Primary school students (seven papers), K-12 students (nine papers), and college students (four papers). But especially the last group shows a slight underrepresentation. In addition, there is a lack of targeted research limited to prospective teachers as there were too few studies exclusively focusing on pre-service teacher students in STEM subjects. Here, a considerable gap in the analyzed sample of the research literature emerges. This is because prospective teachers and student teachers in particular, play a crucial role if computational literacy is to be considered in science teaching in an integrative and contemporary manner. So it is obvious that the integration of computer literacy in special university or college courses explicitly addressed to pre-service teachers needs more attention.
It is easy to understand that approaches need to be appropriate to that age level in early childhood education, e.g., playful learning designs. Also, it can make use of out-of-school learning venues, e.g., in the form of an individual exhibition (Ehsan et al., 2018). Or you can use activities that include haptically engaging objects such as programmable robots (Kong and Lao, 2017). The specific role of such tools will be discussed in more detail later. As students get older, integrated education can be focused on the potential these concepts like CT offer them for later education or job search in specific branches (Lee et al., 2020), where direct integration of computational literacy into other sciences can bring a distinct competitive advantage. At the college level, one can increase effectiveness appropriately by demanding more student projects or offering interdisciplinary and collaborative work opportunities among students across disciplines (Xu et al., 2019). Based on this idea, it may be worthwhile to look more closely at how learners can positively contribute to each other so that informatics concepts can be profitably used in science education. The age level is also likely to play a crucial role here, of course. An interesting question would be, for example, how group work and inquiry-based learning can support the integration of computational literacy into STEM lessons. The effectiveness of such an intervention must be considered with special regard to age-specific prerequisites and conditions of the learners. In a further step, this should also influence how the didactic training of prospective teachers needs to be adapted.
The perspective of sustainability: Curricular or integrated?
The question of integration is closely linked to the sustainability of the targeted competence enhancements. Four papers explicitly include a curricular anchoring in their investigations right at the beginning as one possible option to achieve that goal. This is certainly also a prerequisite to taking up these competencies again and again following a spiral curricular approach, expanding them, and keeping them persistently available. It is striking that the two older publications by Rusu (2015) and Delistavrou and Kameas (2017) in this group of four focus on the technological possibilities of computer science and practical tools. Only Miller (2019) links computer science competencies like coding with a cross-curricula approach for all students to enable new learning approaches in STEM subjects. It is important to note that this approach does not combine computational literacy with science competencies but with mathematics competencies such as identifying mathematical structures and patterns.
On the one hand, it is understandable that computer science should be brought in as a mediator through mathematics because those competencies already play an essential role in science teaching and the natural sciences. In this way, synergy effects can arise, precisely since computer science is broadly integrated into the curricula of science teaching subjects and because computer science itself often uses mathematics as its language. But on the other hand, you cannot fully consider the peculiarities and characteristics of science education. In addition, there is the danger that sustainable usability under scientific problems is hindered if no direct connection is made. Following this idea, Dickes et al. (2020) focus more on science curricula, but the decisive mediation role of mathematics is still maintained to integrate informatics competencies like coding or modeling. To further support this goal, this intervention also uses practical tools such as a technical platform that enables agent-based programming and computational modeling.
From the point of view of sustainability and effectiveness, it must also be considered whether computational literacy should be taught integratively, i.e., directly in science lessons or in isolated informatics courses. We were able to assign 13 publications to the first approach. Consequently, these informatics competencies are always linked in the immediate context of science questions, activities, or content, as in the study by Ciftci and Topcu (2022). There were two early childhood pre-service teacher groups: one group received CT integrated STEM education, and the control group did not. A t-test measured significant positive effects in the intervention group. Often, the content orientation also determines the choice of an integrative approach, especially regarding the technologically dependent skills that use mostly specific tools or apps. Then, you need the informatics or natural science context to be able to show and use the practical added value. Two papers pick up exactly this idea by using robots to strengthen cognitive skills such as CT integratively in early year STEM education (Kong and Lao, 2017; Ioannou and Makridou, 2018).
Only four papers can be assigned to the opposite approach, focusing on the isolated teaching of skills. Although technical tools and apps can also play a substantial role in this group, for example, two papers rely on independent units in workshops or block programs (Rusu, 2015; Choate et al., 2018). They show that it can be relevant whether these technical tools should transport competencies themselves or–as in the case of these two papers–teachers should be empowered to develop or use other competencies. It should be noted that they also put particular focus on the game- or challenge-based activities or even on the students’ ability to participate in competitions. Conde et al. (2020) explicitly connect this approach on a technical level with robots and comparable physical devices. Alternatively, standalone offerings may be to realize extracurricular activities and environments (Ehsan et al., 2018) to embed real-world situations and experiences. Of course, mixed forms can occur when, for example, integrative offerings are additionally enriched by isolated courses. However, the evaluation of our sample suggests a preference for studies with majority integrative approaches.
The ambivalence of apps and tools
As we have already mentioned in some places, apps, platforms, robots, or other technical or digital aids and tools play an ambivalent role: On the one hand, it is often only the use of such tools that enables effective treatment of informatics competencies such (Kong and Lao, 2017; Choate et al., 2018)–especially in scientific contexts. In doing so, both teachers and students ideally become reflective experts and creators. This works better in education, the better the underlying concepts are integrated and can emerge. In addition, the didactic accessibility or preparation, e.g., in the form of special learning offers using the programming language Scratch1 (Fidai et al., 2020), may also be decisive. On the other hand, apps and technical aids are increasingly finding their way into everyday teaching because this is desired and can be implemented more easily due to the digitalization of society in general. The problem, however, is that the mere (and didactically unreflective) use of such tools certainly does not guarantee that informatics or science competencies can be taught correctly and effectively with added value. It is more likely that without sufficient basic computational literacy, both teachers and students will barely acquire situated product knowledge that neither enables competency promotion nor can be reused in an abstracted and reflective manner. In the worst case, teachers and students are only passive consumers and users. It is not expedient to demand the use of apps by teachers and students across the board as a standalone request (cf. Kline et al., 2014). This balancing act between product and concept knowledge concerning computational literacy in student teachers is discussed in more detail in a paper by Braun et al. (2021). The authors suggest that examples of use and concrete value-added references are crucial when teaching computational literacy. After explaining how asymmetric encryption works to (student) teachers, it must then be explained why information can be exchanged securely and confidentially via possibly insecure messenger services. In doing so, this informatics concept solves a privacy-related problem that many teachers often face. Finally, this paper proposes integrating informatics competencies into as many subjects as possible, as it is fundamental to learning and developing 21st century skills. Accordingly, student teachers must already be able to learn computational literacy early on in order to use it themselves and integrate it in a meaningful way for their students. This claim is in line with our research questions, whereby we focus explicitly on science subjects and can thus investigate differentiated points of contact and pick up on commonalities.
On focus: Concrete skills and competences
One question is fundamental: Which competencies and contents should be considered? When looking at the literature, one competence is currently particularly emphasized, at least from the perspective of computer science: CT. CT became a relevant competence in education (Wing, 2006), and several educational researchers have increasingly picked it up since that time if the topic is informatics competencies in STEM education. Our sample also supports this observation because 18 of the 24 papers address CT mainly, e.g., (Lee et al., 2020; Lyon and Magana, 2020), preferentially, e.g., (Dickes et al., 2020), or at least alongside other competencies. We assume the following reasons for this: First, a common basis for the definition of this competence has been laid by Wing and others, which is taken up repeatedly. Thus, it can have a unified frame of reference, which is essential for scientific collaboration. In addition, CT is very closely related to computers, both in its function as an object of instruction and as a technical tool. This makes a computer much more effectively applicable, especially in science education: to describe and solve physical problems, the language of mathematics is often invoked. The more complex and digital these descriptions and the solution approaches become, the more you fall back on computers and additional informatics competencies. In this sense, CT can be as important as other relevant mathematical competencies in STEM education (Guncaga and Kopczynski, 2019). As another justification, it can consider CT a door opener that can foster interest in and integration of further informatics content and competencies in specific contexts (Ehsan et al., 2018). Finally, CT taken for itself is universal enough and suitable to be trained directly with science competencies, for example, when it comes to systematic processing of information and problems.
Whereas, from a science education perspective, there does not seem to be such a clear and sole favorite so far. Nevertheless, we were able to identify eight papers that explore possible candidates for meaningful science competencies that you could consider effective model competencies: Pattern recognition because it is considered one aspect of CT (Dasgupta and Purzer, 2016). Also, Miller (2019) takes a specific look at this mathematical competence and assumes a close connection between CT on the one hand and pattern recognition and further mathematical concepts on the other hand, too. Mathematics again acts as a mediator, which is also true for the paper by diSessa (2018). In this paper, mathematical descriptions of movements serve as a scientific context. The premise is that informatics competencies such as CT or coding permanently change learning in STEM subjects.
In science education, spatial reasoning often plays a crucial role in the ability to mentally imagine rotations, e.g., in understanding the structure of molecules in chemistry or rotational movements in physics. According to the work by Citta et al. (2019) these abilities have a well exploitable connection to CT, which positive correlations can demonstrate in studies with 1st to 5th-grade students. Similarly, in biology, a study shows that students can benefit positively when CT activities are directly integrated into subject lessons (Irgens et al., 2020). Specifically, they implemented an empirical study using a 10-day biology unit at a high school, where learning analytics tools showed how students’ thinking changes using computer science. Another study by Xu et al. (2019), also in college biology, focuses on integrative activities by using specific computer programs when comparing RNA sequences. Remarkably, however, they focused on analyzing the competence of algorithms. In contrast to the previously discussed papers, CT is not the leading idea. In addition, the project approach is interdisciplinary, with computer science and science classes working together, and an e-portfolio platform is used to promote corresponding digital competencies in a situational manner simultaneously.
Finally, Kusmin et al. (2017) show another interesting way how informatics concepts can be broadly integrated into many STEM subjects: You use the schoolhouse itself as a data-rich physical and digital environment, i.e., this so-called smart schoolhouse itself becomes a teaching object and an instrument for this study at the same time, in which you investigate advantages and disadvantages of sensors as data sources with groups of experts from different backgrounds or jobs and combines this with data collection technologies and concepts from computer science. In this way, the topic of Big Data can be directly experienced by many STEM subjects in a variety of integrative ways.
Summary
In the following, we look at the most important similarities between the analyzed papers based on the item categories in Figure 2 and identify possible trends that can promote the successful integration of computational literacy in STEM:
The pairing of competencies: When integrating computational literacy into STEM lessons, it is advantageous if competencies from the respective subjects are specifically assigned. This makes it clear already when designing learning units that connecting aspects can maximize learning gains. In addition, a so-called red thread is established, which can also ensure transparency and clarity of purpose for the learners. As mentioned before, there is a big trend to focus on CT as a competence. However, it is important to note that this term is often representative of various other competencies that have a lot in common with CT.
Embedding in the curriculum: In many papers, one aspect is that the intervention is aligned with the curriculum. This can increase reuse and sustainability. It also offers the obvious advantage of using and formulating common prerequisites and objectives in a subject or a way to better ensure the interlocking of content by using an orientation framework for integration. Furthermore, it also has the advantage that cross-curricular lessons or projects can be realized. This is because many STEM curricula have now explicitly included links with other subjects in this subject group.
Problem orientation and use of tools: The trend to involve robots or smartphones is very noticeable. In this way, one can–at least at the beginning–increase the motivation of the students. In addition, haptic learning can be made possible in this way. Alternatively, a (learning) tool is placed in the foreground, for example with Scratch, or a (cloud) platform. Almost always, robots are used with a problem-based teaching approach. Building on this, the problem orientation is sometimes extended to participation in competitions. In this way, the students should delve deeper into a problem task and seek creative solutions collaboratively in competition with others.
Almost all studies focus only on a certain age group. In this way, both the age-related cognitive prerequisites can be considered, and specially tailored learning objects can be selected. In addition, it is then easy to map the learners’ world of experience, e.g., primary school pupils perceive smartphones quite differently than university students, and learning environments need to take this into account.
Marginal observations
In this section, we want to briefly mention some aspects that may also play a role in our research question and are already taken up by papers.
Another important goal in science education is to increase the proportion of women in STEM subjects. Therefore, it can be useful to ensure that this intention is not thwarted at an early stage. After all, you are linking two subject disciplines here in which women and girls are statistically less represented, which is even more pronounced in computer science in some cases. Therefore, there is a danger of reducing the proportion of women by combining those subjects in this way. A comparable idea has already been considered in the paper by Ehsan et al. (2018). They have also an additional focus on ethnic minorities, which have a low proportion among students in the statistics of STEM subjects, too. In addition, self-efficacy may play a relevant role for all student teachers (Ciftci and Topcu, 2022). This can especially affect teachers who have high values concerning their competencies in natural science subjects but have weaknesses in informatics or at least feel deterred by it.
Therefore, these aspects are important and should be considered at an early stage because the integration of computer science literacy in natural sciences must not impose additional obstacles for minorities or prospective teachers. In the worst case, this could exacerbate the shortage of well-trained teachers in STEM subjects. One way to address this may be to integrate computational literacy as low as possible, e.g., by making informatics content easy and direct to use or less subject-specific regarding (abstract) computer science topics (Braun et al., 2021).
Limitations of the review
“Information Literacy,” “Digital Literacy,” “Data Literacy” or even simply “Computer Science” are on the one hand used synonymously for the same competencies or different issues, on the other hand, they are also understood differently, or even alternative formulations are used. Consequently, it is difficult, especially in English-language literature, to fully grasp the term “computational literacy” on a universal basis. This ambiguity and inconsistency complicate research questions that focus on the clash of computer science and natural science education strongly. Therefore, identifying all corresponding papers is much more challenging since informatics content or tools are often used without distinct competence building, as we have already discussed in Section “Methodology.”
In extreme cases, informatic competence can seem to be adequately integrated with the use or introduction of provided tools, such as office applications or video editing programs, because these are immediately available in our digital age. However, it is sometimes not possible to decide whether computational literacy is being addressed in the background based on the abstract alone. For these two reasons, an important limitation of our review is that it cannot claim to have found all papers that properly address this specific topic. In this context, one of the strongly focused parameters of our research question also plays a role, as it is about the targeted teaching and integration of computational literacy into science education. Due to the dual role and the technical change, we claim to sort out rather strictly on this target in case of doubt. This is because we see a gap in scientific research here, which we want to investigate specifically. Furthermore, one can assume that the scientific vagueness in the terminology has also favored the focus on CT since a common and uniform definition exists here as a frame of reference.
In addition, we have deliberately used only the Web of Science search engine relevant to our field of science. This also ensures that only papers that have been subjected to a peer-review process are found. As a further restriction, we have limited ourselves to English-language publications because we want to ensure a minimum level of internationality in terms of addressees and relevance. In a second step, German-language publications can be consulted separately to consider country-specific circumstances.
Finally, we point out that the Corona pandemic at the time of the review has influenced the number of publications in the recent past: On the one hand, it can be assumed that computational literacy, in general, has become a stronger focus of science education research. On the other hand, it is precisely the natural sciences that are at a disadvantage because of their dependence on experiments. Studies or practical interventions often need to take place in real classrooms. Consequently, there might already be further theoretical considerations here, which could not yet be conducted or published due to a lack of empirical data. Anyway, you can see in Figure 3 that most papers found are published in 2020 following a remarkable decrease in the next year, although computer science in natural science education has certainly not become less important. The number for 2022 considers only paper if they were very available in the database before March 1st, 2022.
Conclusion and outlook
In reviewing and analyzing the literature, we have identified an essential gap in educational science research, as mentioned earlier. Although some papers first hint at possible approaches and relevant questions, fundamental or comprehensive studies are still missing. In a subject area that is also more subject to temporal change due to technological development, timely and systematic interventions are needed so that the education of teachers or students can be quickly adapted to the requirements of the 21st century. Because there is a broad consensus that informatics concepts and competencies are essential for effective science education or careers. Therefore, we need to address this issue of how to integrate them most effectively and appropriately. Teacher training certainly plays a determining role in this goal. This is because fundamentals already need to be integrated into education. Based on the literature discussed, there needs to be a clear focus on specific competencies to be able to investigate their linking potential with the other subject discipline. Suitable courses must be created. This concerns both the depth of content and the context orientation. In further steps, research questions should try to cover a systematic or curricular approach. Isolated and one-off offers or courses are not sustainable enough and thus run the risk of producing misconceptions or inert knowledge in the worst case.
Special features in this clash of two STEM subjects must also be considered: On the one hand, computer science has this dual role to come to the fore as its discipline in this composite of subjects. On the other hand, its integration into science education is urgently indicated and useful in a way comparable to mathematics as the language of natural science. Furthermore, the ambivalent dependence on computer-technical tools and aids must be considered carefully since it is precisely the way of dealing with them that determines whether a reflected and conscious teaching of computational literacy can succeed or not.
Finally, we derive a few concrete critical conclusions as points of orientation for future research projects. In doing so, we also take a special look at possible implications for the training of prospective teachers:
First, we point out that the partial focus on CT as a guiding idea is understandable and helpful, especially because CT is also suitable as a starting point to identify and look more closely at other concepts in parallel or building on it. But because our focus is on how to integrate computational literacy into science education, researchers must actively consider looking at it from this perspective as well, e.g., by taking up the other line of thought: looking at science competencies or contexts first, such as spatial reasoning or comparison of RNA sequences, and then looking for several informatics competencies that can be effectively linked. This way guarantees that the research and future development is not too one-sided and that the natural science competencies and content can be better developed holistically and within a spiral curriculum approach.
Furthermore, several different informatics competencies are certainly relevant per learning unit in science and not just one. This requires deeper expert knowledge on the part of the teachers. However, this realization can only be adequately taken into account with the change of perspective mentioned above. Applied to the teaching profession, this means that we need to train more science teachers in computational literacy. Especially those students who do not take computer science as an additional subject. Only in this way can science teaching be enriched in its entire breadth.
All in all, a focus on a guiding perspective such as CT is understandable and sensible since there are already common foundations and universal connecting points. However, natural science competencies as a starting point should equally be considered so that there is no imbalance in research and later implementation.
When embedding curricula, a cross-curricular approach is often also considered at the same time by selecting contents or competencies that enable interdisciplinary work. Such an approach is to be welcomed in principle but must be introduced cautiously. This is especially true if it is to be transferred to school teaching or the training of future teachers. It is important to realize that the integration of computational literacy already increases the cognitive load and that at least two disciplines are always present at the same time. If the interdisciplinary part is added to this, it can lead to excessive demands or even create unnecessarily high hurdles. In particular, we want to point out that the learners should have sufficient learning time to understand the integration in their own science subjects. Only then should the interdisciplinary approach be pursued in greater depth. For this reason, by the way, it is also elementary that before the actual integration of computational literacy into STEM lessons, computer science lessons are taught in the form of a separate subject at school.
As already mentioned, problem orientation is chosen as a teaching approach, e.g., when CT is taught with the help of robots. In our opinion, this–admittedly effective–pairing should not be anticipated so exclusively. There are other approaches to teaching: action-oriented, student-oriented (students’ world of experience), communicative, creative, and open or discovery-orientated. These alternative learning occasions have thus far received less attention without comprehensible justification. Because from the perspective of a pupil, motivation ends as soon as a solution is found. From an informatics perspective, however, further competencies could then be taken up, for example, a found algorithm can solve a problem. But only through a further analysis in terms of efficiency can a deeper understanding be found with the help of the optimization process. Furthermore, a different approach can be used to investigate creative learning objects, such as the above-mentioned smart schoolhouse, which do not arise solely from a problem orientation perspective.
Specific age groups require appropriate interventions and studies. But with a view to teacher training and transfer to the school classroom, it is imperative that research approaches vertically link the age boundaries involved. Instead of the age of the learners, the learning age with regard to experience with computational literacy could be used as an alternative, if one leaves out all too playful and childlike approaches. In other words: Science teachers are, to a certain extent, usually also beginners in computational subjects like their later pupils. It might therefore be helpful to create learning environments that provide prospective teachers with equivalent experiences to those their students will later experience in the classroom. For example, the training of subject specialists and educators must differ in such aspects in order to effectively design integrative STEM lessons.
In conclusion, we note that further research is needed in this area, with particular attention to the aspects mentioned above with respect to teacher education. In addition, we can also imagine other possible questions: Which scientific competence(s) can be considered as leading competencies like CT? Which technical language should be preferred in case of conflict: the informatics’ or the natural sciences’ language? Which exact prerequisites favor integration or create barriers in the process, for special groups of people? Should low-threshold or more specialized offerings be created in teacher training? What is the optimal ratio of implicit to explicit teaching of computational literacy in STEM subjects?
Author contributions
DB: conceptualization, methodology, investigation, data curation, formal analysis, writing–original draft, writing–review and editing, and visualization. JH: resources, writing–review and editing, supervision, and project administration. Both authors contributed to the article and approved the submitted version.
Funding
This research was funded by the Federal Ministry of Education and Research (project “edu4.0” in the framework of the joint “Qualitätsoffensive Lehrerbildung”, grant number 01JA2011). The APC was funded by the University of Konstanz.
Conflict of interest
The authors declare that the research was conducted in the absence of any commercial or financial relationships that could be construed as a potential conflict of interest.
Publisher’s note
All claims expressed in this article are solely those of the authors and do not necessarily represent those of their affiliated organizations, or those of the publisher, the editors and the reviewers. Any product that may be evaluated in this article, or claim that may be made by its manufacturer, is not guaranteed or endorsed by the publisher.
Footnotes
References
Akbar, M., Dura, L., Gates, A. Q., Ortega, A., Roy, M. K., Santiago, C., et al. (2018). “Sol y Agua: A game-based learning platform to engage middle-school students in STEM,” in Proceedings of the 48th IEEE Frontiers in Education Conference (FIE), (New York, NY: IEEE). doi: 10.1109/FIE.2018.8659071
Braun, D., Pampel, B., and Seiss, M. (2021). “Informatik-Grundlagen für Lehramtsstudierende,” in INFOS 2021 – 19. GI-Fachtagung Informatik und Schule, ed. L. Humbert (Bonn: Gesellschaft für Informatik), 193–202.
Choate, B., Dubosarsky, M., and Chen, K. (2018). “An innovative professional development model for teaching robotics to novice educators,” in Proceedings of the 48th IEEE Frontiers in Education Conference (FIE), (New York, NY: IEEE). doi: 10.1109/FIE.2018.8658771
Ciftci, A., and Topcu, M. S. (2022). Improving early childhood pre-service teachers’ computational thinking teaching self-efficacy beliefs in a STEM course. Res. Sci. Technol. Educ. 27:2036117. doi: 10.1080/02635143.2022.2036117
Citta, G., Gentile, M., Allegra, M., Arrigo, M., Conti, D., Ottaviano, S., et al. (2019). The effects of mental rotation on computational thinking. Comp. Educ. 141:103613. doi: 10.1016/j.compedu.2019.103613
Conde, M. A., Sedano, F. J. R., Fernandez-Llamas, C., Goncalves, J., Lima, J., and Garcia-Penalvo, F. J. (2020). “RoboSTEAM project systematic mapping: Challenge based learning and robotics,” in Proceedings of the IEEE Global Engineering Education Conference (IEEE EDUCON), (New York, NY: IEEE), 214–221. doi: 10.1109/EDUCON45650.2020.9125103
Dasgupta, A., and Purzer, S. (2016). “No patterns in pattern recognition: A systematic literature review,” in Proceedings of the IEEE Frontiers in Education Conference (FIE), (New York, NY: IEEE). doi: 10.1109/FIE.2016.7757676
Delistavrou, K. T., and Kameas, A. D. (2017). “Exploring Ways to Exploit UMI Technologies in STEM education comparison of secondary computer science curricula of greece, cyprus and England,” in Proceedings of the 8th IEEE Global Engineering Education Conference (EDUCON), (New York, NY: IEEE), 1824–1830. doi: 10.1109/EDUCON.2017.7943098
Dickes, A. C., Farris, A. V., and Sengupta, P. (2020). Sociomathematical norms for integrating coding and modeling with elementary science: a dialogical approach. J. Sci. Educ. Technol. 29, 35–52. doi: 10.1007/s10956-019-09795-7
diSessa, A. A. (2018). Computational Literacy and “The Big Picture” concerning computers in mathematics education. Math. Think. Learn. 20, 3–31. doi: 10.1080/10986065.2018.1403544
Dziabenko, O., and Budnyk, O. (2019). “GO-LAB ecosystem: Using online laboratories in a primary school,” in Proceedings of the 11th International Conference on Education and New Learning Technologies (EDULEARN), (Valenica: Iated-Int Assoc Technology Education & Development), 9276–9285. doi: 10.21125/edulearn.2019.2304
Ehsan, H., Ohland, C., Dandridge, T., and Cardella, M. (2018). “Computing for the critters: Exploring computational thinking of children in an informal learning setting,” in Proceedings of the 48th IEEE Frontiers in Education Conference (FIE), (New York, NY: IEEE). doi: 10.1109/FIE.2018.8659268
Fidai, A., Capraro, M. M., and Capraro, R. M. (2020). "Scratch"-ing computational thinking with Arduino: A meta-analysis. Think. Skills Creat. 38:14. doi: 10.1016/j.tsc.2020.100726
Guncaga, J., and Kopczynski, T. (2019). “Supporting mathematical and digital competences useful for stem education,” in E-Learning and Stem Education, ed. E. SmyrnovaTrybulska (Katowice: University Silesia), 409–419.
Ioannou, A., and Makridou, E. (2018). Exploring the potentials of educational robotics in the development of computational thinking: A summary of current research and practical proposal for future work. Educ. Inform. Technol. 23, 2531–2544. doi: 10.1007/s10639-018-9729-z
Irgens, G. A., Dabholkar, S., Bain, C., Woods, P., Hall, K., Swanson, H., et al. (2020). Modeling and measuring high school students’ computational thinking practices in science. J. Sci. Educ. Technol. 29, 136–160. doi: 10.1007/s10956-020-09811-1
Juskeviciene, A., Dagiene, V., and Dolgopolovas, V. (2021). Integrated activities in STEM environment: Methodology and implementation practice. Comput. Appl. Eng. Educ. 29, 209–228. doi: 10.1002/cae.22324
Kim, C., Yuan, J. M., Vasconcelos, L., Shin, M., and Hill, R. B. (2018). Debugging during block-based programming. Inst. Sci. 46, 767–787. doi: 10.1007/s11251-018-9453-5
Kline, R. L., Hill, J. H., Birney, L. B., Sarris, S., and Gauthier, J. (2014). “Experiential project-based learning for university students in k-12 stem initiatives,” Proceedings of the Integrated STEM Education Conference (ISEC), (Princeton, NJ: IEEE). doi: 10.1109/ISECon.2014.6891028
Kong, S. C., and Lao, C. C. (2017). “Computational thinking development through programmable robotics activities in STEM education in primary schools,” in Proceedings of the 25th International Conference on Computers in Education (ICCE) - Technology and Innovation - Computer-Based Educational Systems for the 21st Century, (Taoyuan City: Asia Pacific Soc Computers in Education), 984–989.
Kusmin, M., Saar, M., Laanpere, M., and Rodriguez-Triana, M. J. (2017). “Work in progress - smart schoolhouse as a data-driven inquiry learning space for the next generation of engineers,” in Proceedings of the 8th IEEE Global Engineering Education Conference (EDUCON), (New York, NY: IEEE), 1667–1670. doi: 10.1109/EDUCON.2017.7943072
Lamb, R., Annetta, L., Vallett, D., Firestone, J., Schmitter-Edgecombe, M., Walker, H., et al. (2018). Psychosocial factors impacting STEM career selection. J. Educ. Res. 111, 446–458. doi: 10.1080/00220671.2017.1295359
Lee, I., Grover, S., Martin, F., Pillai, S., and Malyn-Smith, J. (2020). Computational thinking from a disciplinary perspective: Integrating computational thinking in K-12 Science, technology, engineering, and mathematics education. J. Sci. Educ. Technol. 29, 1–8. doi: 10.1007/s10956-019-09803-w
Leonard, J., Buss, A., Gamboa, R., Mitchell, M., Fashola, O. S., Hubert, T., et al. (2016). Using robotics and game design to enhance children’s self-efficacy, STEM attitudes, and computational thinking skills. J. Sci. Educ. Technol. 25, 860–876. doi: 10.1007/s10956-016-9628-2
Lyon, J. A., and Magana, A. J. (2020). Computational thinking in higher education: A review of the literature. Comp. Appl. Eng. Educ. 28, 1174–1189. doi: 10.1002/cae.22295
Miller, J. (2019). STEM education in the primary years to support mathematical thinking: using coding to identify mathematical structures and patterns. Zdm-Math. Educ. 51, 915–927. doi: 10.1007/s11858-019-01096-y
Page, M. J., McKenzie, J. E., Bossuyt, P. M., Boutron, I., Hoffmann, T. C., Mulrow, C. D., et al. (2021). The PRISMA 2020 statement: an updated guideline for reporting systematic reviews. BMJ 372:n71. doi: 10.1136/bmj.n71
Rajaei, H., Aldakheel, E. A., and ASEE (2012). “AC 2012-4956: Cloud computing in computer science and engineering education,” in Proceedings of the ASEE Annual Conference, (Washington, DC: Amer Soc Engineering Education).
Rusu, A. (2015). “Introducing gaming tools for computing education in STEM related curricula,” in Proceedings of the 45th Annual Frontiers in Education Conference (FIE), (New York, NY: IEEE), 883–890. doi: 10.1109/FIE.2015.7344166
Swaid, S. I., and IRMA (2015). A Novel Strategy to Improve STEM Education: The E-Science Approach. Pennsylvania: IGI Global.
Wang, C. Z., Shen, J., and Chao, J. (2021). Integrating computational thinking in STEM education: A literature review. Int. J. Sci. Math. Educ. doi: 10.1007/s10763-021-10227-5
Xu, N., Keller, C., and Ye, Y. (2019). “Applying computer science in biology: A model for incorporating interdisciplinary pedagogical approaches through ePortfolio in the first year experience at laguardia community college,” in Proceedings of the 9th IEEE Integrated STEM Education Conference (ISEC), (New York, NY: IEEE), 316–323. doi: 10.1109/ISECon.2019.8882038
Keywords: computational literacy, science education, data literacy, information literacy, review, STEM education, informatics competencies
Citation: Braun D and Huwer J (2022) Computational literacy in science education–A systematic review. Front. Educ. 7:937048. doi: 10.3389/feduc.2022.937048
Received: 05 May 2022; Accepted: 09 August 2022;
Published: 30 August 2022.
Edited by:
Jorge Rodríguez-Becerra, Universidad Metropolitana de Ciencias de la Educación, ChileReviewed by:
Lisa M. Abrams, Virginia Commonwealth University, United StatesMirna Muñoz, Centro de Investigación en Matemáticas, Mexico
Copyright © 2022 Braun and Huwer. This is an open-access article distributed under the terms of the Creative Commons Attribution License (CC BY). The use, distribution or reproduction in other forums is permitted, provided the original author(s) and the copyright owner(s) are credited and that the original publication in this journal is cited, in accordance with accepted academic practice. No use, distribution or reproduction is permitted which does not comply with these terms.
*Correspondence: Daniel Braun, ZC5icmF1bkB1bmkta29uc3RhbnouZGU=; Johannes Huwer, am9oYW5uZXMuaHV3ZXJAdW5pLWtvbnN0YW56LmRl